- 1Instituto Tecnológico Vale, Belém, Brazil
- 2Globe Institute, Faculty of Health and Medical Sciences, University of Copenhagen, Copenhagen, Denmark
Introduction: The urgent need for effective environmental monitoring amid the escalating biodiversity crisis has prompted the adoption of molecular techniques like DNA metabarcoding. Through sequencing of taxonomically informative mitochondrial markers in bulk arthropod samples, metabarcoding allows assessment of arthropod diversity, which is crucial for ecosystem health evaluations, especially in threatened regions like the Amazon. However, challenges such as primer biases and reference database limitations persist.
Methods: Here, we assess the performance of four metabarcoding primer sets, two COI markers (ZBJ-ArtF1c/ZBJ-ArtR2c [Zeale]: 157bp, targeting arthropods, and mlCOIintF/jgHCO2198 [Leray]: 313bp, targeting metazoans) and two 16S markers (Ins16S_1shortF/Ins16S_1shortR [Ins16S]: 150bp, targeting insects, and Coleop_16Sc/Coleop_16Sd [EPP]: 105bp, targeting arthropods, mainly Coleoptera) in amplifying the taxonomic constituents of bulk arthropod samples, collected across different natural and anthropogenic habitats from the Brazilian Amazon biome. To evaluate primer performance, we used the indicators (i) amplification efficiency, (ii) primer specificity, i.e., the amount of non-target sequences, (iii) detected OTU richness, (iv) group coverage and (v) taxonomic resolution. Finally, we (vi) estimated the refinement in taxa recovery by additional amplifications.
Results: Despite lower specificity and contrasting results regarding OTU richness, the primer pairs returning larger fragments showed higher taxonomic resolution (Ins16S) and broader taxonomic coverage (Leray) than Zeale and EPP did. Furthermore, results demonstrated the complementarity of the Leray and the Ins16S primer sets from taxon-dependent studies.
Conclusions: Despite limitations, combining these primers could enhance biodiversity monitoring in the region. Considering incomplete reference gene banks, primers maximizing OTU richness (EPP and Leray) may be the best choice for taxon-independent surveys. These findings underscore the importance of primer selection and highlight the ongoing efforts to refine DNA metabarcoding for robust environmental assessments.
Highlights
● Different indicators were applied to evaluate primer performance in capturing arthropod diversity from bulk samples
● Findings underscored the superior performance of longer fragments over shorter ones in arthropod diversity recovery
● Key hurdle in metabarcoding assessments in the tropics is the lack of comprehensive reference databases
● Combining COI and 16S primers enhances taxonomic coverage and resolution in arthropod metabarcoding studies.
Introduction
In the looming biodiversity crisis, the imperative for comprehensive environmental monitoring has never been more critical (Montgomery et al., 2021; van Klink et al., 2022). Global ecosystems are under unprecedented threats, and the ability to accurately assess their health and integrity becomes a cornerstone in global conservation and restoration efforts (Mori et al., 2023), especially in the tropics, such as the mega-diverse Amazonian region. In this context, arthropods perform valuable ecosystem services and play a crucial role as bioindicators of environmental quality, and their abundance and diversity should be prioritized in monitoring for conservation purposes (Borges et al., 2021; Chowdhury et al., 2023; Hallmann et al., 2017). However, considerable time and fieldwork input, as well as scarcity of taxonomic expertise (Bevilacqua et al., 2012; Hortal et al., 2015; Pires et al., 2021) reduce the use of terrestrial invertebrates as indicators of environmental change or in conservation studies (Kallimanis et al., 2012). Amid this taxonomic bottleneck, the rise of molecular techniques, i.e., new generation biomonitoring, has provided new perspectives to make arthropod monitoring viable (Makiola et al., 2020; Zizka et al., 2020).
The DNA barcodes, or barcodes, are short DNA sequences that serve as molecular signatures used to help identify species or group them into possible species. Within the realm of new-generation biomonitoring, DNA metabarcoding has emerged as a promising tool (Deiner et al., 2017; Nørgaard et al., 2021; Taberlet et al., 2012; Tsuji et al., 2022). To achieve this, DNA is typically extracted from environmental samples e.g., soil, water or arthropod pools, after which informative mitochondrial DNA markers, that serve as barcode across taxonomic groups, are sequenced in parallel. Following high-throughput sequencing, computational workflows are used to process the resulting sequences and compare them to reference databases to ultimately identify the taxonomic constituents of the samples (Keck et al., 2023; Taberlet et al., 2012). Metabarcoding has been successfully applied on environmental samples to investigate the diversity of plants (Ariza et al., 2023; Vasar et al., 2023), arthropods (Hein et al., 2024; Hermans et al., 2022), as well as vertebrates (Gu et al., 2023; Lynggaard et al., 2019; Massey et al., 2022).
Although metabarcoding has demonstrated its efficacy in biodiversity monitoring, the technique provides some significant challenges (Elbrecht et al., 2019). First, the selection of which mitochondrial region to target is a crucial step. The target region depends on the objective of monitoring with stability to represent the species and variability to separate the taxa of interest (Bohmann et al., 2014; Elbrecht et al., 2016; Zhang et al., 2020). Commonly targeted regions for the detection of arthropods are the mitochondrial gene cytochrome c oxidase subunit I (COI) (Hebert et al., 2003) and the mitochondrial 16S rRNA regions (Clarke et al., 2014; Elbrecht et al., 2016). Compared to COI, the 16S region shows more conserved regions and lower mutation rates, which can lead to lower genetic variability between species or populations (Bose and Moore, 2023; Elbrecht et al., 2019). Another thing to consider is primer biases, i.e., the primers’ ability to PCR amplify evenly across the various taxa within targeted taxonomic group. Primer biases may distort estimates of species frequency, abundance, and diversity in metabarcoding studies (Mathieu et al., 2020).
Lastly, the availability of reference sequences for the mitochondrial region is crucial to allow taxonomic annotations (Clarke et al., 2014; Keck et al., 2023).
Thus, evaluating the performance (i.e. species resolution, primer bias and reference coverage) of primers targeting arthropods is essential to guarantee accurate and reliable results during environmental monitoring and biodiversity conservation (Alberdi et al., 2019), especially in mega-diverse tropical ecosystems (van der Heyde et al., 2022b, van der Heyde et al., 2022a). Generally, in-silico tests using reference genomes or DNA sequences stored in a database to predict the results of PCR reactions help scientists to design primers and predict what DNA fragments would be amplified, and in-vitro tests using template DNA are carried out to test the binding and subsequent PCR of the primer to target region (Lorusso et al., 2024). Additionally, primer performance should be compared using real samples, and different indicators should be used to provide a comprehensive view of how efficient and specific primers are in amplifying target DNA regions in-vivo. As the recovery of non-target taxa may result in assignment errors, primer specificity, i.e., the number of detected non-target taxa, will determine the degree of selectivity of the primer during amplification, avoiding false positives or negatives. During metabarcoding, sequences are frequently classified as Operational Taxonomic Units (OTUs) based on similarity. In a second step, OTUs receive taxonomic annotations by comparison with reference databases; taxonomic annotations may comprise different taxonomic levels, e.g., species or order levels. Lacking reference sequences result in ‘unassigned’ OTUs. OTU richness (i.e., the number of recovered OTUs per sample) and the group coverage (the range of identified organisms) within target organisms is another possibility to evaluate a given primer set. High OTU richness and group coverage ensure a more comprehensive representation of biological diversity in the sample. Taxonomic resolution, i.e., the number of OTUs identified to a specific taxonomic level, depends on the completeness and the availability of group- and region-specific reference libraries (Coissac et al., 2012). Incomplete libraries increase the chance of non-identification of specific OTUs and may explain the non-detection by metabarcoding of species supposedly present. Finally, similarity can be used to determine how similar the retrieved communities are to each other, while complementarity outlines the expected percentage of additional matches when more than one primer is applied. Similarity is expected to be greater between pairs of primers designed for the same region, and complementarity should be higher for primers from distinct regions.
Here, we aim to tackle the intricacies of DNA metabarcoding by comparing the performance of two distinct regions, COI and 16S, in surveying the diversity of arthropods in environmental monitoring in the megadiverse Brazilian Amazon. We used a comprehensive set of arthropod bulk samples spanning different trap types, ecosystems and seasons of the region (Lynggaard et al., 2020) to outline the in-vivo primer performance to monitor arthropod diversity in this realm. For the COI region, we examined the Zeale (ZBJ-ArtF1c/ZBJ-ArtR2c) and the Leray primers (mlCOIintF/jgHCO2198), and for the 16S region, Ins16S (Ins16S_1shortF/Ins16S_1shortR) and EPP (Coleop_16Sc/Coleop_16Sd) primers were applied (Supplementary Figure S1 from Supplementary Material). The following indicators were applied to evaluate primer performance: (i) amplification efficiency, (ii) primer specificity, i.e., the amount of non-target sequences, (iii) detected OTU richness, (iv) group coverage, and (v) taxonomic resolution. Finally, we estimated the refinement in taxa recovery by additional amplifications using (vi) similarity and complementarity. In doing so, we can recommend the most efficient primers for arthropod monitoring via metabarcoding in the megadiverse Amazonian forest.
Materials and methods
Geographic setting
Bulk arthropod samples analyzed in this study were collected in the Carajás National Forest located in Southeastern Pará State, Brazil (05°52’S–06°33’S, 49°53’W–50°45’W) (Figure 1). The Carajás National Forest is designated as a category VI Conservation Unit, permitting both sustainable use and mining activities. The region experiences a tropical climate characterized by rainy summers and dry, warm winters [Aw in the Koppen-Geiger classification (Alvares et al., 2013)]. The average temperature is 25.4°C, with an annual rainfall of approximately 2,000 mm, concentrated primarily in January, February and March. The prevalent vegetation types include Dense or Open Evergreen Forests. Notably, lateritic duricrusts, locally referred to as cangas, cap iron ore deposits and emerge on mountaintops, where they are adorned with a mega-diverse, endemic savanna-like vegetation (Gagen et al., 2019; Giulietti et al., 2019; Viana et al., 2016).
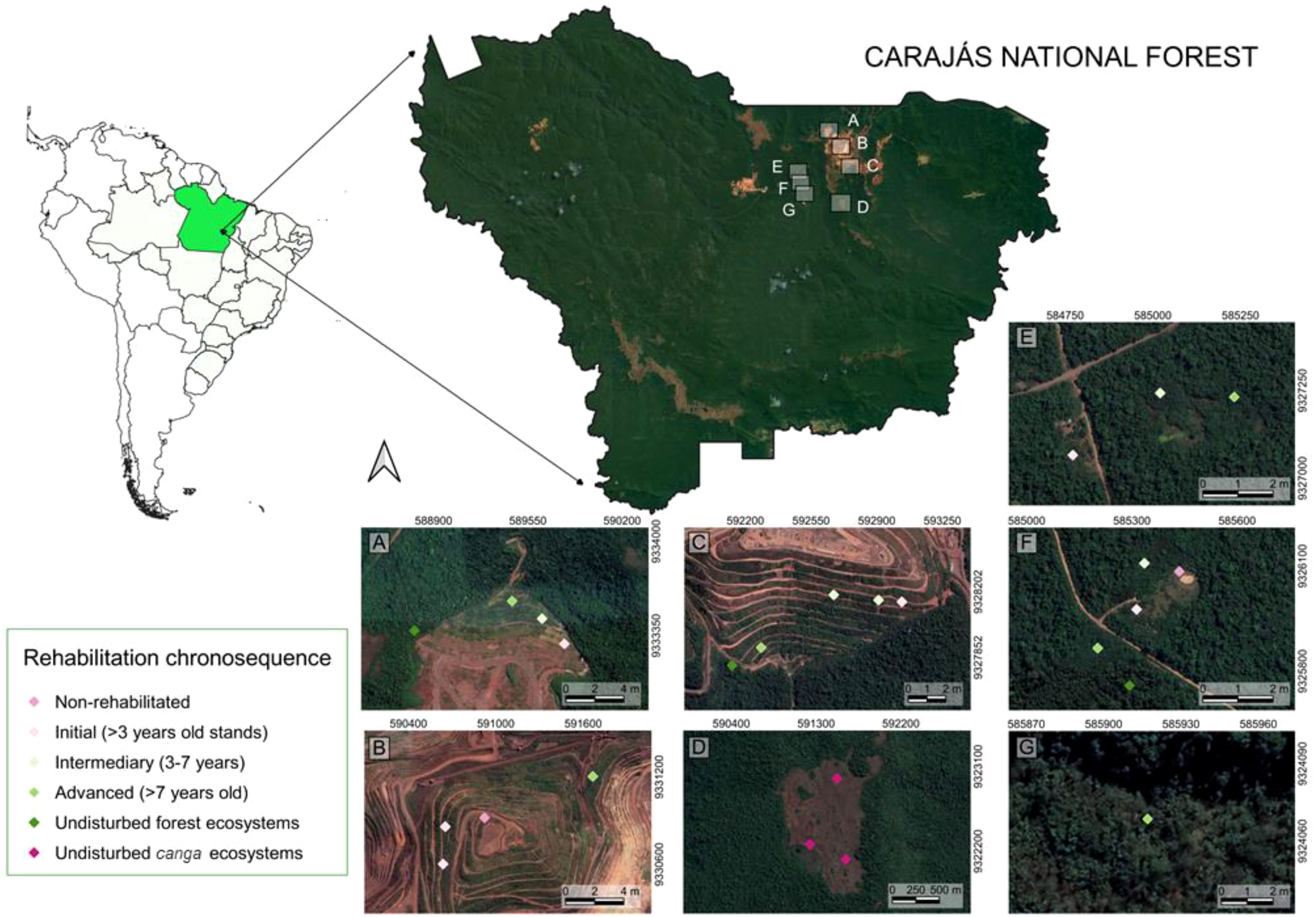
Figure 1. Localization and sampling points in the Carajás National Forest, Pará, Brazil. (A–C) Mining waste piles, (D) canga site, (E–G) sand quarries.
Gigantic reserves of iron, gold, copper, manganese, sand, and granite are exploited in the region, mainly by open-cast mines. Environmental rehabilitation of disused spaces, including closed mine pits and filled waste piles, is an essential aspect of mining operations’ environmental stewardship (Gastauer et al., 2018). This rehabilitation process is designed to stabilize benches, curtail soil erosion, and mitigate the environmental impacts associated with mining activities. Moreover, mine land rehabilitation aims to restore biodiversity and ecosystem services to their pre-mining levels. Various techniques, such as topsoil application, seedling planting, or hydroseeding, may be employed in this restoration endeavor. Ongoing monitoring of rehabilitated areas is conducted to assess the success of the rehabilitation efforts and determine the necessity of further interventions (Gastauer et al., 2022).
Sampling design
The samples utilized in this study encompass rehabilitation chronosequences from waste piles from the N4-N5 iron mining complex (Gastauer et al., 2021; Nascimento et al., 2020) and three sand quarries (Gastauer et al., 2019). Their primary purpose was to assess the effectiveness of rehabilitation activities (Lynggaard et al., 2020). All chronosequences consists of non-revegetated stands, early stages (1 to 3 years old stands, predominantly herbaceous vegetation), intermediate stages (3 to 7 years old, marked by shrub encroachment), advanced stages (post-canopy closure, > 7 years old), and reference canga and forest ecosystems.
The sampling protocol involved twenty-five sampling points from both chronosequences, with each point equipped with both a Malaise trap and a pitfall trap. Malaise traps were deployed for five days, while pitfall traps were left in the field for 24 hours. Due to logistic reasons, arthropods were preserved in 70% ethanol in pitfall traps and in propylene glycol in Malaise traps (Lynggaard et al., 2019). Sampling occurred in September 2017 (dry season) and April 2018 (rainy season), resulting in a total of 50 samples for each trap type. This comprehensive sampling design allows for testing potential influences of trap type or sampling season on primer performance.
PCR and sequencing
As described in Lynggaard et al. (2020), the DNA from all 98 samples was extracted following a nondestructive protocol (Nielsen et al., 2019). The extracted DNA was purified using a QiaQuick PCR Purification Kit (Qiagen, United Kingdom) following the manufacturer’s protocol with minor modifications, such as the addition of 50 μL of elution buffer and incubation at 37°C for 15 minutes before centrifugation.
Quantitative PCR (qPCR) was conducted prior to amplification to determine the ideal number of cycles, prevent excessive testing costs, and identify possible inhibitors (Krehenwinkel et al., 2017; Murray et al., 2015). For qPCR, a mixture of 1 μL of purified DNA, 0.6 μL of each primer, 1x Gold PCR Buffer (Applied Biosystems), 0.2 mM dNTP mixture (Invitrogen), 2.5 mM MgCl2 (Applied Biosystems), 0.75 U of AmpliTaq Gold (Applied Biosystems), 0.5 mg/mL bovine serum albumin (BSA; Bio Labs) with 1 μL of SYBR Green/ROX (Sigma−Aldrich) was prepared. DNA was amplified using 40 cycles, and further primer-specific parameters for qPCR were employed, as outlined in Table 1, but adding a dissociation curve instead of the extension of 72°C for 7 min. Primer-specific PCR amplification followed the protocol of qPCR but excluding the SYBR Green/Rox, using 27 to 35 cycles, depending on sampling season (Table 1).
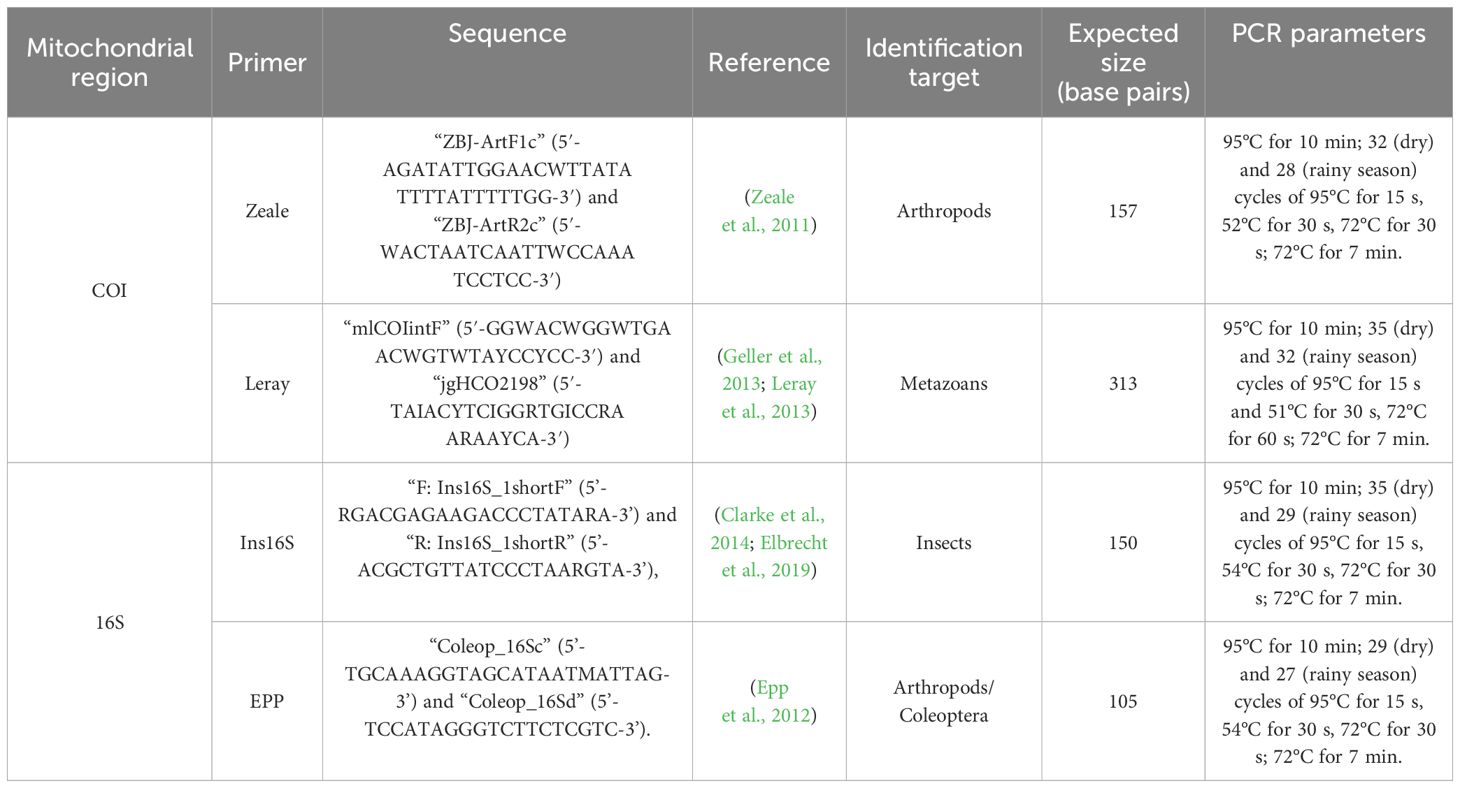
Table 1. Primers and PCR parameters used in this study to detect arthropod diversity from bulk arthropod samples collected in the Carajás National Forest, Pará, Brazil.
Three PCR replicates were used for each sample extract and for the positive and negative controls. The positive controls consisted of DNA mixtures containing Locusta migratoria (order: Orthoptera), Tenebrio molitor (order: Coleoptera) and Galleria mellonella (order: Lepidoptera) for the dry period samples and L. migratoria, T. molitor, and Blaptica dúbia (order: Blattodea) for the rainy season. A negative control was included for every seven PCR sample product. PCR amplification was done using matching nucleotide tags (e.g. forward primer tag 1 – reverse primer tag 1 and so on). Each DNA extract was uniquely tagged, and PCR replicates of the same sample were given a different tag combination. These tags consisted of six to eight nucleotides added to the 5’ end of both the forward and reverse primers (Binladen et al., 2007).
The success of the DNA amplification was assessed through 2% agarose gel electrophoresis using GelRed and a 50 bp ladder to confirm fragment size and verify the absence of amplifications in negative controls. Amplicon pools consisted of PCR products of samples where two or more PCR replicates showed amplification, as well as negative and positive controls. After bead purification of the amplicon pools using the MagBio HighPrep magnetic bead kit (LabLife), libraries were built using the TagSteady protocol (Carøe and Bohmann, 2020). Purified libraries were quantified using the NEBNext Library Quant for Illumina Kit (New England Biolabs, Inc.). Finally, the libraries were sequenced on an Illumina MiSeq v2 platform aiming at 25,000 paired reads per PCR replicate.
Bioinformatics analyses
Each of the eight datasets, representing one of four primer sets from each of the two seasons, underwent individual analysis (Lynggaard et al., 2020). In the initial step, the triage process was executed using AdapterRemoval v2.3.3 (Schubert et al., 2016), eliminating Illumina adapters and low-quality stretches of sequence. Demultiplexing of sequences to PCR replicates and samples was done using the Begum package (Yang et al., 2021). A stringent filtering criterion was implemented, retaining only sequences present in at least two replicates and comprising a minimum of 10 sequences each (Alberdi et al., 2019). Moreover, sequences with a minimum length of 280 base pairs and a maximum of 330 were retained for the Leray data, 130 and 170 for Zeale and Ins16S, and 90 and 110 for the Coleop data.
To define optimal clustering values, SUMATRA and SUMACLUST were employed, transforming sequences into operational taxonomic units (OTUs) with 97% similarity (Mercier et al., 2013). To rectify potential misallocations of OTUs, the LULU algorithm was applied, identifying errors through the combination of similar sequences and patterns of co-occurrence. This process ensured that rare yet authentic OTUs persisted while addressing clustering errors (Frøslev et al., 2017).
The OTU sequences were compared to the NCBI GenBank database (https://www.ncbi.nlm.nih.gov/genbank/) using BLASTn with blast v2.13.10 using the following settings: -qcov_hsp_perc 80 and -perc_identity 84. To obtain a taxonomical identification of the sequences, the output was then imported into MEGAN Community Edition V6.24.20 using a weighted LCA algorithm with 80% coverage, top percent of 10 and a minimum score of 150.
Primer performance evaluation
Six indicators were chosen to assess the effectiveness of the four primers: (i) amplification efficiency, (ii) specificity, (iii) detected OTU richness, (iv) group coverage, (v) taxonomic resolution on the order and the species level, and (vi) similarity and complementarity. Target organisms of the applied primers are arthropods. Amplification efficiency refers to the success of amplification during the PCR, that is the number of successful amplification replicates. Only samples with three successfully amplifying replicates were for all primers were maintained for further analysis. Specificity was detected as the number of detected non-target classes or phyla. Detected OTU richness refers to the total number of retrieved OTUs classified as arthropods. To compare detected OTU richness among primers separately, we rarefied the number of OTUs based on the number of samples and the number of reads using R package iNEXT (Hsieh et al., 2016). Group coverage was evaluated as the number of arthropod orders revealed by each primer. Taxonomic resolution was represented by the number of OTUs detected by each primer identified to a specific taxonomic levels (orders, families, genera, and species). Where applicable, we carried out separate evaluations for the dry and the rainy season, and collection methods (Pitfall vs. Malaise). To outline similarity between primers, we used the Jaccard similarity index to quantify the proportion of common species among primers, and build a similarity dendrogram using phyloseq v.1.30.0 (McMurdie and Holmes, 2013) and vegan v.2.5-6 packages (Oksanen et al., 2017).
To address the question of the validity and cost-effectiveness of an additional sequencing with a different primer, the complementary hierarchy was established for the four primer pairs, focusing solely on the phylum Arthropoda. This assessment aimed to estimate the refinement in taxa recovery with each additional amplification.
Results
DNA sequencing
The number of reads per sample and per replication before and after filtering steps can be found in Supplementary Table S1 from Supplementary Material. In the rainy season, sequencing revealed a total of 1,194,607 reads in 51 input samples for Ins16S (after bioinformatic processing), 1,992,515 in 51 samples for EPP, 646,557 in 50 samples for Leray and 1,830,618 in 49 samples sequenced for Zeale. In the dry period, 47 samples generated a total of 3,650,501 reads for Ins16S, 36 samples for EPP with 4,176,526 reads, 41 samples for Leray with 2,222,120 reads and a total of 3,926,216 reads in 42 input samples for Zeale. As expected, all positive controls amplified only reads from the species used, and the negative controls did not detect contaminations.
Amplification efficiency
From all four primer pairs, EPP showed lowest amplification efficiency in both seasons (Supplementary Table S2), that is 47 out of 51 samples in the rainy season, and 36 out of 47 samples in the dry season (Supplementary Table S2 from Supplementary Material). Ins16S performed best (51 in rainy season and 47 in dry period), and Zeale (49 and 41 samples in rainy and dry season, respectively) and Leray (50 and 41 samples) were on intermediate positions (Supplementary Table S2).
Primer specificity
Although designed for the detection of insects and arthropods, both Ins16S and EPP exhibited detections beyond the arthropod realm (Figure 2). Ins16S reached into classes like Actinopteri (bony fish), while EPP extended its reach to classes such as Bivalvia (mollusks), Flavobacteria (bacteria), and Polychaeta (annelids). Leray, too, presented additional detections, albeit in smaller numbers, featuring a handful of fungal species and two bacteria. In contrast, Zeale delivered readings within the expected range only.
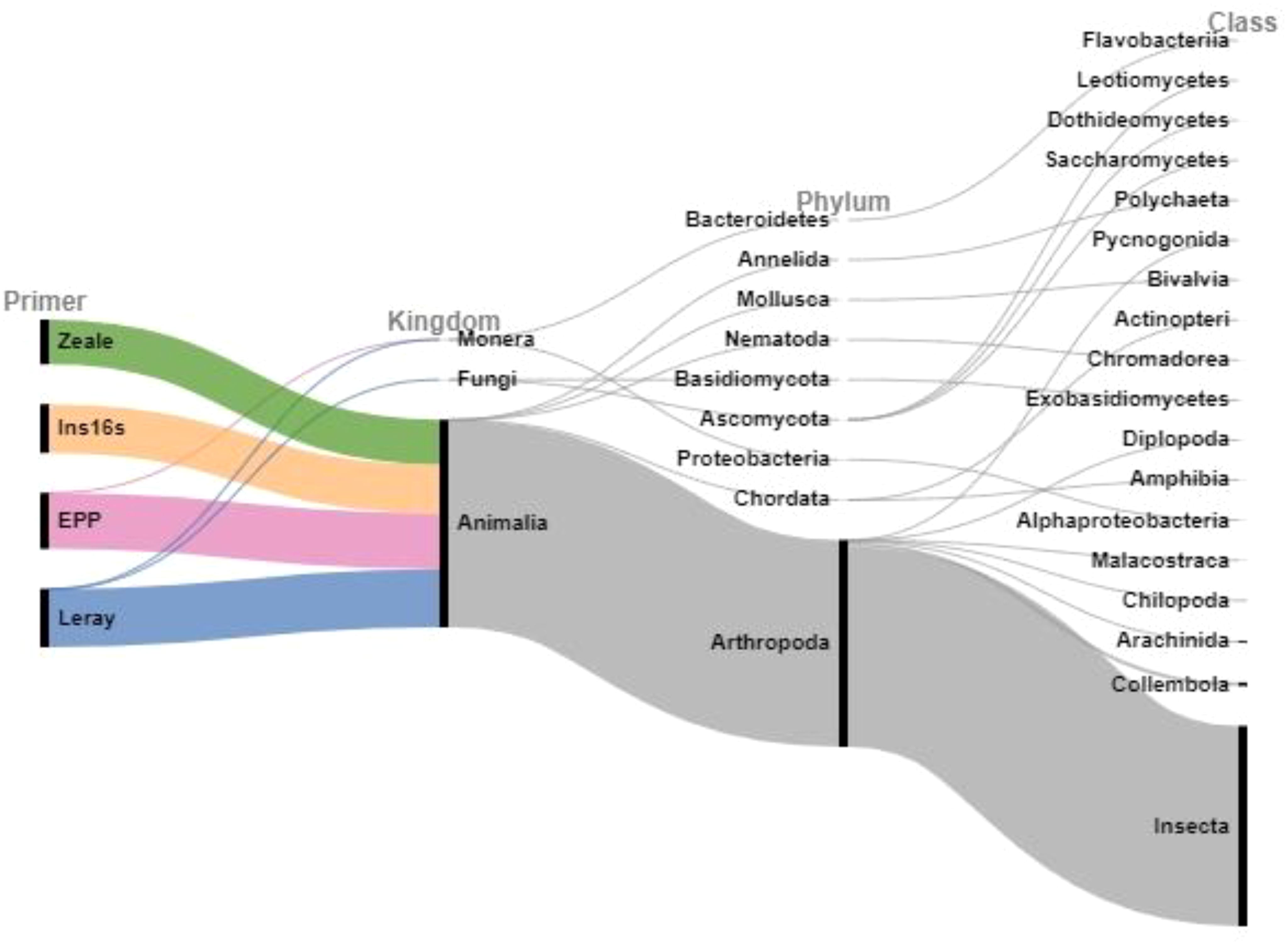
Figure 2. Taxonomic kingdoms, phylum and class annotations of DNA sequences detected by the four primers Ins16S, EPP (both 16S region), Zeale and Leray (both COI) in bulk arthropod samples from the Carajás National Forest, Pará State, Brazil. Bar width is proportional to the number of OTUs.
Detected OTU richness
OTU richness differed among primer pairs and sample season (Figure 3). Despite lower number of reads, Leray recovered more OTUs than EPP, Ins16S, and Zeale in the dry season. The number of detected OTUs in the rainy season was highest for EPP when samples were pooled and Malaise traps, followed by Leray, Zeale and Ins16S. For pitfall traps in both seasons, Leray (with lowest number of reads) performs best.
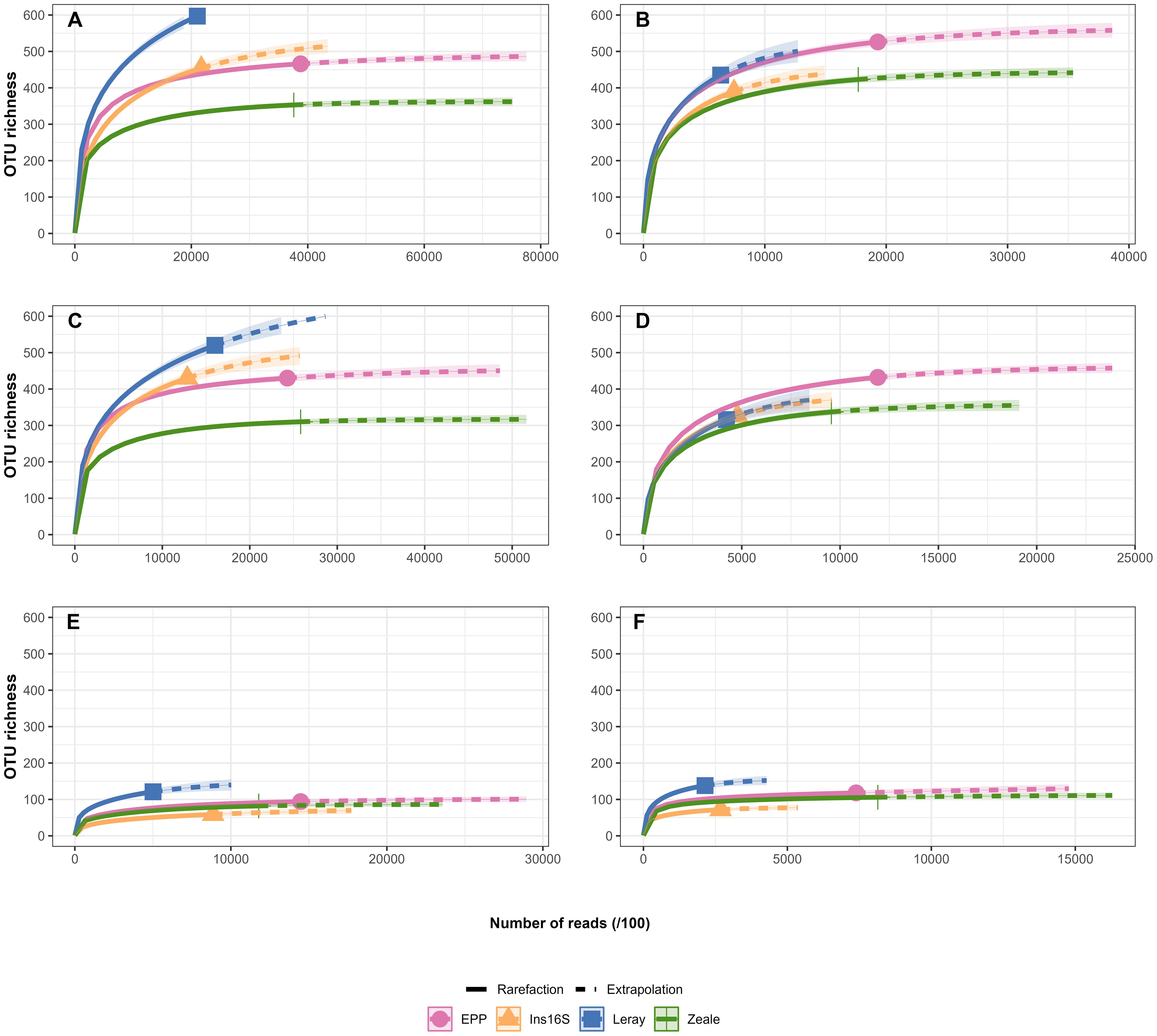
Figure 3. Total number of Operating Taxonomic Units (OTUs) and the taxonomic identification on the order (Or), family (F), genus (G), and species (S) level from bulk arthropod samples from the Carajás National Forest, Pará State, Brazil, amplified using four distinct primers. (A) Dry season, Malaise and pitfall traps pooled. (B) Rainy season, Malaise and pitfall traps pooled. (C) Malaise traps during the dry period. (D) Malaise traps during the rainy season. (E) Pitfall traps in the dry period. (F) Pitfall traps in the rainy season.
The rarefaction based on number of reads shows that Leray performs significantly better during dry season, followed by EPP, Zeale and Ins16S (Figure 3). However, in rainy season, EPP retrieves significantly more OTUs when only Malaise traps were analyzed. These patterns change slightly when rarifying based on the number of samples (Supplementary Figure S2), and Leray stands out for COI region, while EPP remains more efficient for 16S.
Group coverage
In the dry season, EPP outperformed Ins16S in detecting 19 distinct arthropod orders (Figure 4). The composition of these orders varied between the two primer pairs. Although both primer sets detected high frequencies of Lepidoptera and Diptera, EPP also primarily detected Coleoptera, whereas Ins16S detections were also dominated by Hymenoptera. During the rainy season, Ins16S detected more orders (17 in total, with Diptera and Coleoptera being dominant) compared to EPP, which detected 15 orders, mainly Diptera and Coleoptera.
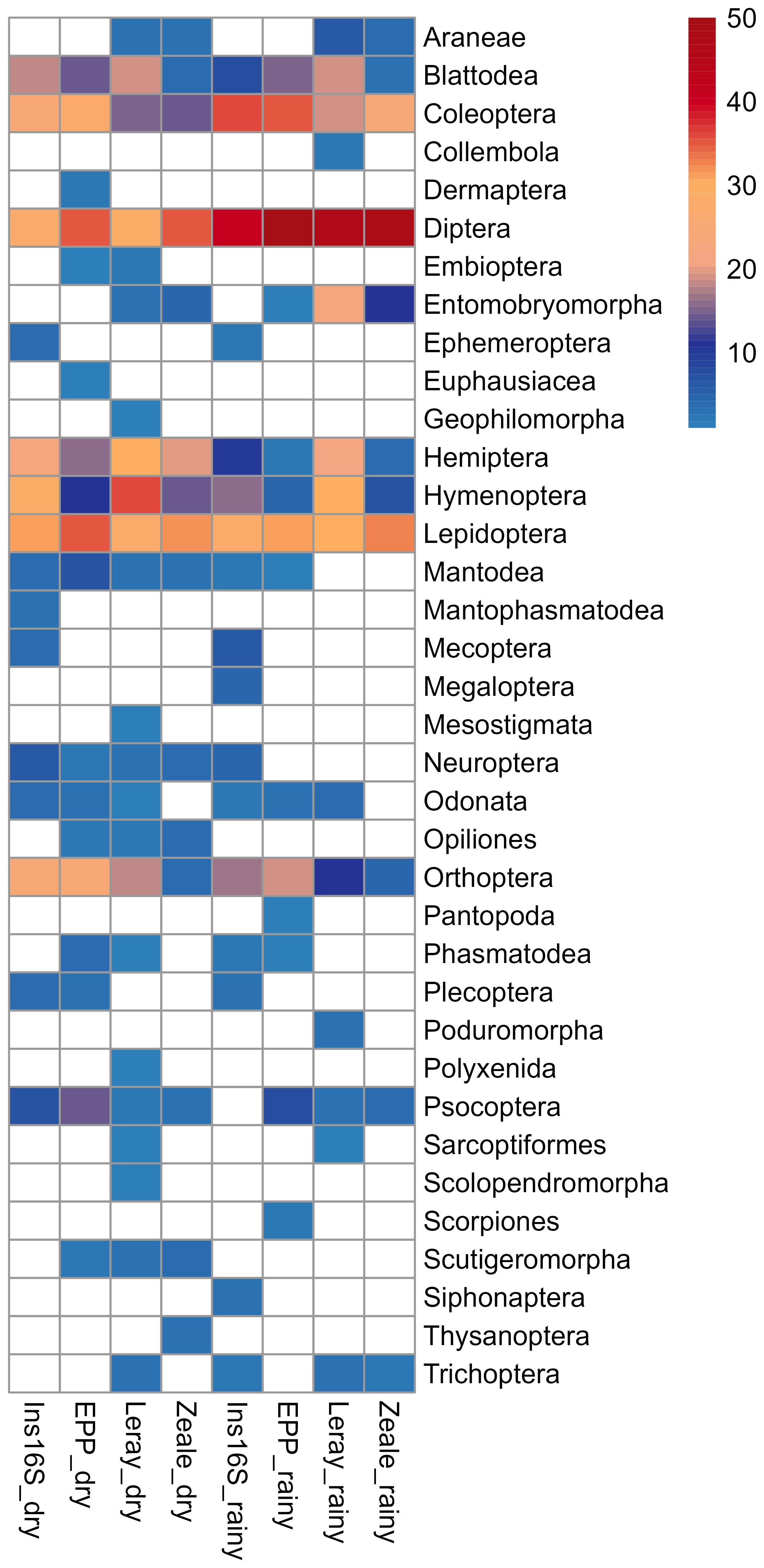
Figure 4. Frequency heatmap of recovered arthropod orders in the four primer sets from the Carajás National Forest, Eastern Amazon, Brazil, during the dry and the rainy season.
For the COI region, Leray primer covered 23 orders in the dry season, including some not detected by any other primer pair, such as Geophilomorpha (Myriapoda), Scolopendromorpha (Chilopoda), Mesostigmata (Acariformes), and Polyxenida (Diplopoda). Most frequent orders were Hymenoptera, Hemiptera, Lepidoptera and Diptera. In the rainy season, 15 orders were identified, with Diptera, Hymenoptera, and Lepidoptera being the most dominant. Similarly, Zeale primer showed the same pattern in both seasons, with Lepidoptera and Diptera as the most frequent orders. However, the number of detected orders was slightly higher in the dry season (15 orders) compared to the rainy season (11 orders) (Figure 4).
Taxonomic resolution
Taxonomic resolution exhibited variations among primers on all taxonomic levels (Figure 5). For the 16S region, Ins16S demonstrated the best coverage in both seasons, identifying approximately 40% of OTUs to species level, while EPP achieved a significantly lower coverage, just above 10% of detected OTUs. In the COI region, Leray outperformed, identifying approximately 13% of the OTUs to the species level, while Zeale identified less than 5% of the OTUs to species level (Figure 5).
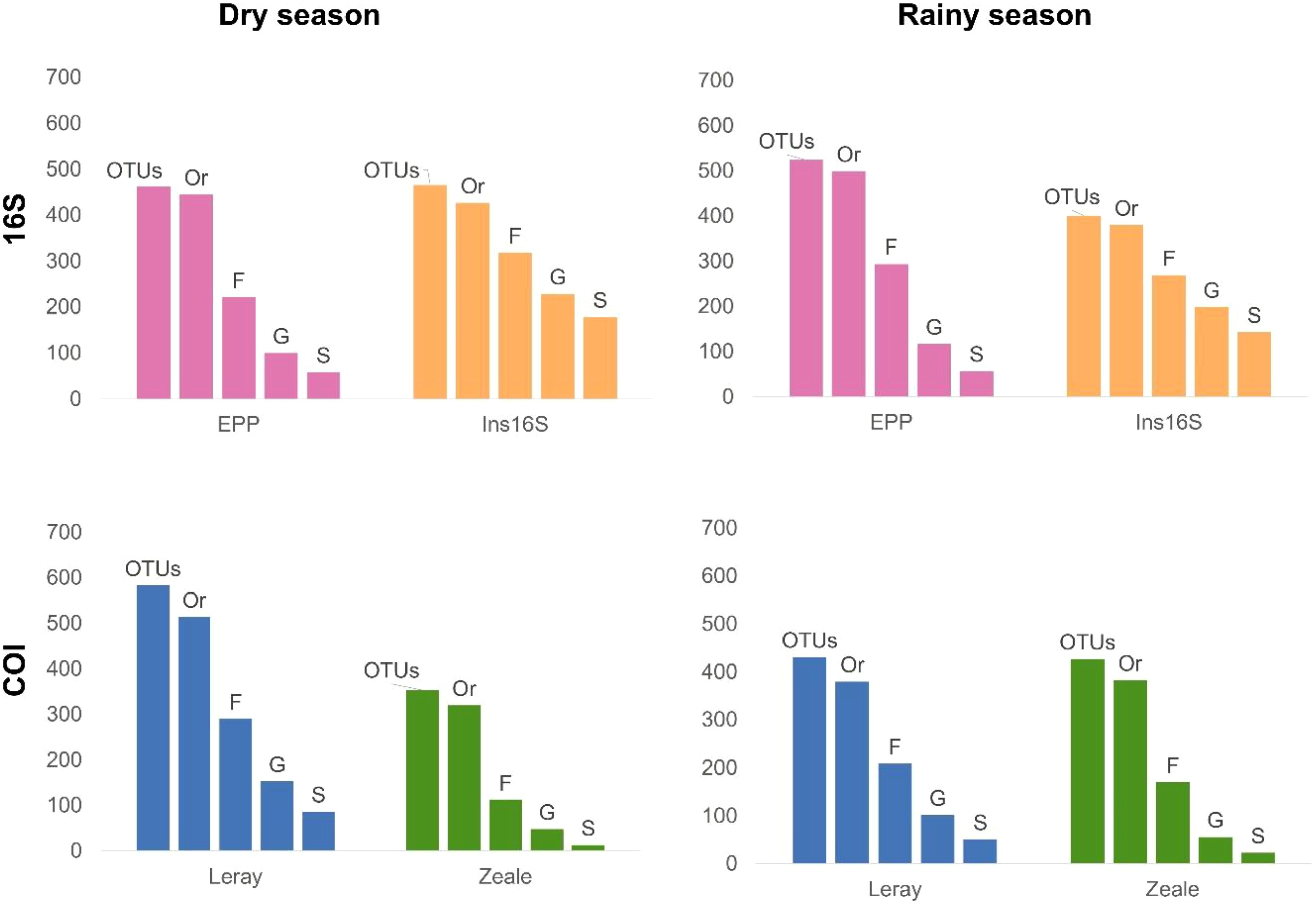
Figure 5. Total number of Operating Taxonomic Units (OTUs) and the taxonomic identification on the order (Or), family (F), genus (G), and species (S) level from bulk arthropod samples from the Carajás National Forest, Pará State, Brazil, amplified using four distinct primers.
Similarity and complementary
Similarity analysis showed similar trends for both the dry and the rainy season. Two clusters were formed: one with greater similarity between the 16S marker primers and the other with the COI marker primers.
To assess the improvement in identification with each successive round of amplification, we started our hypothetical trial with Ins16S given its capability to recover the highest number of OTUs identified to the species level (162 and 133 for the dry and rainy seasons, respectively). In sequence, the Leray primer contributed with an additional 67 and 34 new species, respectively. This was followed by EPP, which introduced another 42 and 32 species, and finally, Zeale, which augmented the species count by 21 in each period (Figure 6).
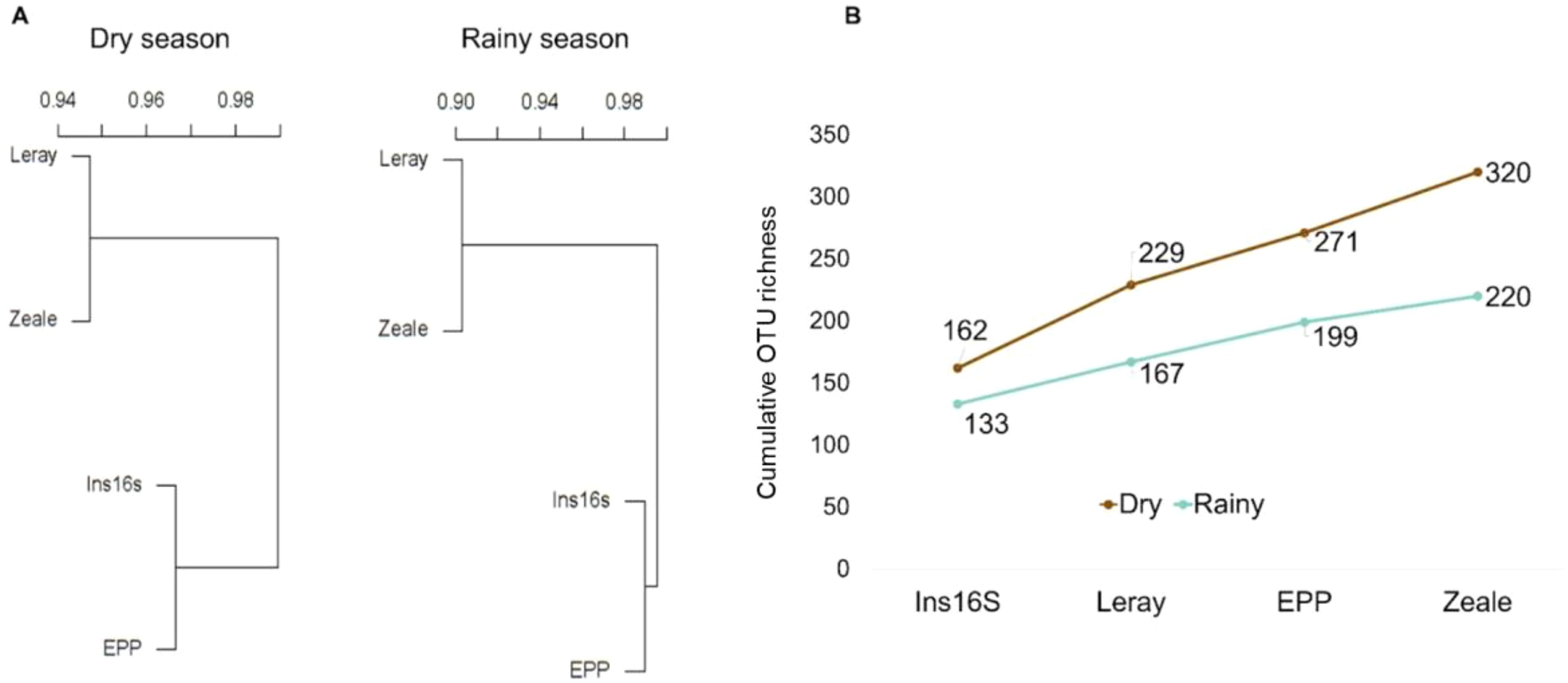
Figure 6. Similarity dendrogram of OTU communities (A) detected by four primer pairs from two distinct molecular regions, COI (Leray and Zeale) and 16S (Ins16S and EPP), based on the Jaccard index, and cumulated OTU richness of Amazonian arthropod bulk samples considering consecutive amplifications rounds (B).
Discussion
Our findings underscore the considerable influence of primer selection on arthropod OTU recovery in molecular environmental monitoring in the Amazon Forest. We detected differences in amplification efficiency among tested primers. Specifically, Ins16S demonstrated high efficiency in the 16S region by highest taxonomic coverage, while EPP recovered highest OTU richness in some of the analyzed scenarios. In the COI region, Leray exhibited better results, recovering more OTUs and assigning more names to sequences even though this primer detected a lower number of reads. Interestingly, there was no discernible impact of primer performance with respect to sampling seasonality, even though the number of recovered OTUs varied between seasons. Importantly, our analysis revealed that the choice of trap type, whether Malaise or pitfalls, as well as the preservation method used for each type of sampling, did not exert a noticeable influence on primer performance in the collected samples.
Several studies discuss differences in primer performance for environmental samples (Alberdi et al., 2018; Clarke et al., 2014; Collins et al., 2019; Elbrecht et al., 2016). Here, we observed differences in amplification efficiency among primers, with Ins16S and Leray performing better than further primer pairs. Amplification failure can be due to sample contamination or DNA degradation, but this would apply to all primers and not to a specific primer set only. In samples containing various species (bulk samples as used in this study), competition among different DNA templates can also be an occasional source of amplification failure, as shown in previous studies (Willassen et al., 2023; Mazurkiewicz et al., 2024). Primers designed to amplify a specific taxonomic group may fail when there is competitive DNA from other taxa in the same pool (Alberdi et al., 2018), which may explain lower amplification efficiency in Zeale and EPP.
Using taxon-independent methods for environmental monitoring (e.g., Wilkinson et al., 2024), most important criterion for primer performance is OTU richness, where EPP outperforms Ins16S in the 16S region. For the COI primers, we observed that the primer that generated more reads (Leray) retrieved a lower OTU richness. This may be due to efficient amplification of specific sequences combined with biases towards certain taxonomic groups, as shorter amplicons may have higher amplification efficiency but might co-amplify non-target organisms (Nakano, 2018), while longer amplicons allow for more accurate species identification but may have higher failure rates (Bylemans et al., 2018). Primer target region matters as well, as less conserved regions, such as the COI region, are expected to show higher diversity resulting from higher mutation rate than more conserved markers such as 16S, where multiple species may be pooled within a single OTU due to similarity threshold (Clarke et al., 2014). Finally, primer efficiency is a key factor, as not all primers may be equally effective across different species/OTUs (Pedro et al., 2023; Alberdi et al., 2018). All these factors may work together and guarantee higher performance of Leray primer for the COI and Ins16S for the 16S region when taxonomic annotations are desired within the study.
Our results emphasize the critical role of primer choice in DNA metabarcoding for bulk arthropod analyses, highlighting the need for a nuanced understanding of their specificities and performance (MacDonald and Sarre, 2017). For group coverage, the availability of reference databases for the specific regions is paramount (Zhang et al., 2020; Keck et al., 2023), which differs considerable among compared primers. Community ecology studies indicate the significance of orders such as Lepidoptera, Coleoptera, and Hymenoptera in monitoring recovering environments (Borges et al., 2021). Leray, identifying 23 orders in a single collection period, and Ins16S, identifying 16 orders, both including key orders, align with this ecological relevance. Although designed to target organisms beyond arthropods (metazoan) that were filtered out for our purpose, Leray showcased superior efficiency in capturing unique orders like Geophilomorpha, Scolopendromorpha, Mesostigmata and Sarcoptiformes (Acariformes), and Polyxenida (Diplopoda), many of them of elevated ecological significance (Gerlach et al., 2013). Specifically, mites (Acariformes) were identified as important ecological indicators, playing a pivotal role in monitoring assessments (Solascasas et al., 2022). In the context of rehabilitating areas, increased diversity at the order level as detected for Leray can be crucial for detecting diverse functional groups (Menta et al., 2014).
Remarkable are DNA sequences identified as Actinopteri, Lophogastrida, Euphausiacea and Decapoda that are somewhat unlikely to enter Malaise and pitfall sampling in terrestrial ecosystems. Decapods may wander off from nearby creeks into the bulk samples, and underlying reasons for further detections may be (1) contamination, (2) misclassification, or (3) refers to ingested DNA (e.g., Lynggaard et al., 2019). Contamination is somewhat unlikely as our laboratory procedures included negative controls at every step, minimizing the possibility of contamination during processing. Misclassification may result from incomplete reference databases, and a sequence from the sample may cluster (based on the 97% similarity criterion) with a sequence from the database and may explain the detection of maritime Lophogastrida and Euphausiacea, highlighting the need to invest in more complete databases. Ingested DNA may furthermore be an explanation for the presence of Actinopteri DNA in the samples.
Several studies with arthropods indicated the influence of seasonality on the diversity of identified taxa, especially in tropical zones (Hermans et al., 2022; Lynggaard et al., 2020; Zhu et al., 2010). Our results indicate visible differences regarding the number of species recovered and in the composition of these groups between one collection period and another. This observation is common in surveys of this type, regardless of sampling or identification method (Umair Sial et al., 2022). Differences can be explained by the interference of rainfall with the movement of arthropods, or variations in seasonal activity of distinct arthropod orders. These variations are closely linked to climatic conditions and the specific requirements of each group, playing a fundamental role in the ecology and population dynamics of these organisms (Lara-Pérez et al., 2023; Umair Sial et al., 2022). Despite the differences in richness and abundance of arthropod species around the year, no differences regarding the primer performance were detected between the analyzed seasons.
While DNA metabarcoding offers efficiency, potential biases can affect the results, spanning from collection and bench stages to bioinformatic processing. Biases, including false positives or negatives, underline the need for careful consideration in primer selection (González et al., 2023; Zinger et al., 2019). Here, we show that the performance of primers correlated with fragment size, with larger fragment sizes (313 and 150 bp for Leray and Ins16S, respectively) yielding better results, particularly because shorter amplicons increase the number of primer dimers and amplification of nonspecific sequences (Elbrecht et al., 2018, 2019).
Utilizing more than one primer for the same region has previously been recommended (Jeon et al., 2008; Zhang et al., 2018), as such procedures can enhance species detection and avoid under-representation of certain groups. Flexibility in marker selection is essential for environmental monitoring, emphasizing the importance of choosing a primer pair that can amplify a broad spectrum of organisms within its target region while maintaining specificity (Collins et al., 2019; MacDonald and Sarre, 2017). COI remains the preferred choice for DNA metabarcoding studies in animals due to its extensive reference library, and Leray, with its ability to detect a diverse array of orders, emerges as a powerful primer for environmental monitoring. Despite a relatively lower number of species identified at the species level, Leray’s taxonomic coverage provides crucial ecological insights for monitoring purposes (Borges et al., 2021). Combining Leray with the efficient species recovery capability of Ins16S could offer a comprehensive approach for community projection in environmental monitoring scenarios,. In contrast, Zeale provide less additional data to justify further sequencing rounds, considering the associated effort and expenditures.
Conclusion
The performance of 16S and COI depends on many factors. Primers revealing larger fragments retrieve higher taxonomic resolution to track arthropod communities in the megadiverse Carajás National Forest from Eastern Amazon. Ins16S offered substantial taxonomic resolution, whereas Leray outperformed with broader group coverage and OTU richness. On the other hand, the16S EPP primer stood out for its superior performance in terms of OTU richness in some of the scenarios analyzed – for taxon-independent surveys (or where reference libraries are highly incomplete as for the Carajás National Forest), this might indicate superior performance.
However, neither primer achieved full coverage, because the reference database for Amazonian arthropods is incomplete, hindering the match of most sequences obtained in this study. This highlights the challenge for the amplification of robust metabarcoding libraries covering undersampled areas such as the Amazon to enable comprehensive arthropod monitoring programs necessary for biodiversity management and conservation. To conclude, while in-silico and in-vitro analyses for primer performance evaluation are useful, in-vivo studies as presented here are essential for understanding primer performance in practice. This practical validation is crucial for ensuring accurate and reliable results. In the meanwhile, we suggest the combined use of Ins16S and Leray primer sets for comprehensive taxon-dependent community studies, leveraging their respective strengths while compensating for their limitations.
Data availability statement
The original contributions presented in the study are included in the article/Supplementary Material. Further inquiries can be directed to the corresponding author.
Ethics statement
The manuscript presents research on animals that do not require ethical approval for their study.
Author contributions
FVP: Data curation, Formal analysis, Methodology, Visualization, Writing – original draft. CL: Conceptualization, Data curation, Funding acquisition, Methodology, Writing – review & editing. LA: Data curation, Visualization, Writing – review & editing. YS: Visualization, Writing – review & editing. GO: Funding acquisition, Writing – review & editing. KB: Data curation, Funding acquisition, Writing – review & editing. MG: Conceptualization, Funding acquisition, Writing – review & editing.
Funding
The author(s) declare financial support was received for the research, authorship, and/or publication of this article. FVP is grateful to a scholarship from the ITV Resident of Pará program, MG acknowledges a CNPq productivity scholarship (Grant number 310865/2022- 0), and CL was supported by the National Council of Science and Technology in Mexico (CONACyT, Grant CVU 582667), the Danish Council for Independent Research (DFF grant 7079-00029B) and by a VILLUM FONDEN research grant (VIL41390).
Acknowledgments
Our sincere gratitude goes to Prof. Tom Gilbert for his invaluable logistical support and guidance. We furthermore acknowledge valuable comments and suggestions from both reviewers! We acknowledge ChatGPT (OpenAI) for its assistance in translating from Portuguese to English and enhancing the text structure. The authors carefully reviewed and revised the output, and final responsibility for the content remains solely with the authors.
Conflict of interest
The authors declare that the research was conducted in the absence of any commercial or financial relationships that could be construed as a potential conflict of interest.
The author(s) declared that they were an editorial board member of Frontiers, at the time of submission. This had no impact on the peer review process and the final decision.
Publisher’s note
All claims expressed in this article are solely those of the authors and do not necessarily represent those of their affiliated organizations, or those of the publisher, the editors and the reviewers. Any product that may be evaluated in this article, or claim that may be made by its manufacturer, is not guaranteed or endorsed by the publisher.
Supplementary material
The Supplementary Material for this article can be found online at: https://www.frontiersin.org/articles/10.3389/fevo.2024.1411388/full#supplementary-material
References
Alberdi A., Aizpurua O., Bohmann K., Gopalakrishnan S., Lynggaard C., Nielsen M., et al. (2019). Promises and pitfalls of using high-throughput sequencing for diet analysis. Mol. Ecol. Resour. 19, 327–348. doi: 10.1111/men.2019.19.issue-2
Alberdi A., Aizpurua O., Gilbert M. T. P., Bohmann K. (2018). Scrutinizing key steps for reliable metabarcoding of environmental samples. Methods Ecol. Evol. 9, 134–147. doi: 10.1111/2041-210X.12849
Alvares C. A., Stape J. L., Sentelhas P. C., De Moraes Gonçalves J. L., Sparovek G. (2013). Köppen’s climate classification map for Brazil. Meteorologische Z. 22, 711–728. doi: 10.1127/0941-2948/2013/0507
Ariza M., Fouks B., Mauvisseau Q., Halvorsen R., Alsos I. G., de Boer H. J. (2023). Plant biodiversity assessment through soil eDNA reflects temporal and local diversity. Methods Ecol. Evol. / Br. Ecol. Soc. 14, 415–430. doi: 10.1111/2041-210X.13865
Bevilacqua S., Terlizzi A., Claudet J., Fraschetti S., Boero F. (2012). Taxonomic relatedness does not matter for species surrogacy in the assessment of community responses to environmental drivers. J. Appl. Ecol. 49, 357–366. doi: 10.1111/j.1365-2664.2011.02096.x
Binladen J., Gilbert M. T. P., Bollback J. P., Panitz F., Bendixen C., Nielsen R., et al. (2007). The use of coded PCR primers enables high-throughput sequencing of multiple homolog amplification products by 454 parallel sequencing. PloS One 2, e197. doi: 10.1371/journal.pone.0000197
Bohmann K., Evans A., Gilbert M. T. P., Carvalho G. R., Creer S., Knapp M., et al. (2014). Environmental DNA for wildlife biology and biodiversity monitoring. Trends Ecol. Evol. 29, 358–367. doi: 10.1016/j.tree.2014.04.003
Borges F. L. G., Oliveira M., da R., de Almeida T. C., Majer J. D., Garcia L. C. (2021). Terrestrial invertebrates as bioindicators in restoration ecology: A global bibliometric survey. Ecological Indicators 125, 107458. doi: 10.1016/j.ecolind.2021.107458
Bose N., Moore S. D. (2023). Variable region sequences influence 16S rRNA performance. Microbiol. Spectr. 11, e0125223. doi: 10.1128/spectrum.01252-23
Bylemans J., Furlan E. M., Gleeson D. M., Hardy C. M., Duncan R. P. (2018). Does size matter? An experimental evaluation of the relative abundance and decay rates of aquatic environmental DNA. Environmental Science & Technology 52, 6408–6416. doi: 10.1021/acs.est.8b01071
Carøe C., Bohmann K. (2020). Tagsteady: A metabarcoding library preparation protocol to avoid false assignment of sequences to samples. Mol. Ecol. Resour. 20, 1620–1631. doi: 10.1111/1755-0998.13227
Chowdhury S., Dubey V. K., Choudhury S., Das A., Jeengar D., Sujatha B., et al. (2023). Insects as bioindicator: A hidden gem for environmental monitoring. Front. Environ. Sci. Eng. China 11. doi: 10.3389/fenvs.2023.1146052
Clarke L. J., Soubrier J., Weyrich L. S., Cooper A. (2014). Environmental metabarcodes for insects: in silico PCR reveals potential for taxonomic bias. Mol. Ecol. Resour. 14, 1160–1170. doi: 10.1111/men.2014.14.issue-6
Coissac E., Riaz T., Puillandre N. (2012). Bioinformatic challenges for DNA metabarcoding of plants and animals. Mol. Ecol. 21, 1834–1847. doi: 10.1111/j.1365-294X.2012.05550.x
Collins R. A., Bakker J., Wangensteen O. S., Soto A. Z., Corrigan L., Sims D. W., et al. (2019). Non−specific amplification compromises environmental DNA metabarcoding with COI. Methods Ecol. Evol. / Br. Ecol. Soc. 10, 1985–2001. doi: 10.1111/2041-210x.13276
Deiner K., Bik H. M., Mächler E., Seymour M., Lacoursière-Roussel A., Altermatt F., et al. (2017). Environmental DNA metabarcoding: Transforming how we survey animal and plant communities. Mol. Ecol. 26, 5872–5895. doi: 10.1111/mec.2017.26.issue-21
Elbrecht V., Braukmann T. W. A., Ivanova N. V., Prosser S. W. J., Hajibabaei M., Wright M., et al. (2019). Validation of COI metabarcoding primers for terrestrial arthropods (No. e27801v2). PeerJ Preprints. doi: 10.7287/peerj.preprints.27801v2
Elbrecht V., Hebert P. D. N., Steinke D. (2018). Slippage of degenerate primers can cause variation in amplicon length. Sci. Rep. 8, 1–5. doi: 10.1038/s41598-018-29364-z
Elbrecht V., Taberlet P., Dejean T., Valentini A., Usseglio-Polatera P., Beisel J.-N., et al. (2016). Testing the potential of a ribosomal 16S marker for DNA metabarcoding of insects. PeerJ 4, e1966. doi: 10.7717/peerj.1966
Epp L. S., Boessenkool S., Bellemain E. P., Haile J., Esposito A., Riaz T., et al. (2012). New environmental metabarcodes for analysing soil DNA: potential for studying past and present ecosystems. Mol. Ecol. 21, 1821–1833. doi: 10.1111/j.1365-294X.2012.05537.x
Frøslev T. G., Kjøller R., Bruun H. H., Ejrnæs R., Brunbjerg A. K., Pietroni C., et al. (2017). Algorithm for post-clustering curation of DNA amplicon data yields reliable biodiversity estimates. Nat. Commun. 8, 1–11. doi: 10.1038/s41467-017-01312-x
Gagen E. J., Levett A., Paz A., Gastauer M., Caldeira C. F., Valadares R. B., et al. (2019). Biogeochemical processes in canga ecosystems: Armoring of iron ore against erosion and importance in iron duricrust restoration in Brazil. Ore Geology Rev. 107, 573–586. doi: 10.1016/j.oregeorev.2019.03.013
Gastauer M., Caldeira C. F., Ramos S. J., Silva D. F., Siqueira J. (2019). Active rehabilitation of Amazonian sand mines converges soils, plant communities and environmental status to their predisturbance levels. Land Degradation Dev. 31 (5), 607–618. doi: 10.1002/ldr.3475
Gastauer M., Nascimento W. R., Caldeira C. F., Ramos S. J., Souza-Filho P. W. M., Féret J.-B. (2022). Spectral diversity allows remote detection of the rehabilitation status in an Amazonian iron mining complex. Int. J. Appl. Earth Observation Geoinformation 106, 102653. doi: 10.1016/j.jag.2021.102653
Gastauer M., Sarmento P. S., de M., Caldeira C. F., Castro A. F., Ramos S. J., et al. (2021). Shannon tree diversity is a surrogate for mineland rehabilitation status. Ecol. Indic. 130, 108100. doi: 10.1016/j.ecolind.2021.108100
Gastauer M., Souza Filho P. W. M., Ramos S. J., Caldeira C. F., Silva J. R., Siqueira J. O., et al. (2018). Mine land rehabilitation in Brazil: Goals and techniques in the context of legal requirements. Ambio. 48 (1), 74–88. doi: 10.1007/s13280-018-1053-8
Geller J., Meyer C., Parker M., Hawk H. (2013). Redesign of PCR primers for mitochondrial cytochrome c oxidase subunit I for marine invertebrates and application in all-taxa biotic surveys. Mol. Ecol. Resour. 13, 851–861. doi: 10.1111/men.2013.13.issue-5
Gerlach J., Samways M., Pryke J. (2013). Terrestrial invertebrates as bioindicators: an overview of available taxonomic groups. J. Insect Conserv. 17, 831–850. doi: 10.1007/s10841-013-9565-9
Giulietti A. M., Giannini T. C., Mota N. F. O., Watanabe M. T. C., Viana P. L., Pastore M., et al. (2019). Edaphic endemism in the amazon: vascular plants of the canga of carajás, Brazil. Botanical Review; Interpreting Botanical Prog. 85, 357–383. doi: 10.1007/s12229-019-09214-x
González A., Dubut V., Corse E., Mekdad R., Dechatre T., Castet U., et al. (2023). VTAM: A robust pipeline for validating metabarcoding data using controls. Comput. Struct. Biotechnol. J. 21, 1151–1156. doi: 10.1016/j.csbj.2023.01.034
Gu S., Deng Y., Wang P., Li C., Shi D., Wang S. (2023). Assessing riverine fish community diversity and stability by eDNA metabarcoding. Ecol. Indic. 157, 111222. doi: 10.1016/j.ecolind.2023.111222
Hallmann C. A., Sorg M., Jongejans E., Siepel H., Hofland N., Schwan H., et al. (2017). More than 75 percent decline over 27 years in total flying insect biomass in protected areas. PloS One 12, e0185809. doi: 10.1371/journal.pone.0185809
Hebert P. D. N., Ratnasingham S., de Waard J. R. (2003). Barcoding animal life: cytochrome c oxidase subunit 1 divergences among closely related species. Proc. R. Soc. London. Ser. B: Biol. Sci. 270, S96–S99. doi: 10.1098/rsbl.2003.0025
Hein N., Astrin J. J., Beckers N., Giebner H., Langen K., Löffler J., et al. (2024). Arthropod diversity in the alpine tundra using metabarcoding: Spatial and temporal differences in alpha- and beta-diversity. Ecol. Evol. 14, e10969. doi: 10.1002/ece3.10969
Hermans S. M., Lear G., Buckley T. R., Buckley H. L. (2022). Environmental DNA sampling detects between-habitat variation in soil arthropod communities, but is a poor indicator of fine-scale spatial and seasonal variation. Ecol. Indic. 140, 109040. doi: 10.1016/j.ecolind.2022.109040
Hortal J., de Bello F., Diniz-Filho J. A. F., Lewinsohn T. M., Lobo J. M., Ladle R. J. (2015). Seven shortfalls that beset large-scale knowledge of biodiversity. Annu. Rev. Ecology Evolution Systematics 46, 523–549. doi: 10.1146/annurev-ecolsys-112414-054400
Hsieh T. C., Ma K. H., Chao A. (2016). iNEXT: an R package for rarefaction and extrapolation of species diversity (Hill numbers). Methods Ecol. Evol. / Br. Ecol. Soc. 7, 1451–1456. doi: 10.1111/mee3.2016.7.issue-12
Jeon S., Bunge J., Leslin C., Stoeck T., Hong S., Epstein S. S. (2008). Environmental rRNA inventories miss over half of protistan diversity. BMC Microbiol. 8, 222. doi: 10.1186/1471-2180-8-222
Kallimanis A. S., Mazaris A. D., Tsakanikas D., Dimopoulos P., Pantis J. D., Sgardelis S. P. (2012). Efficient biodiversity monitoring: Which taxonomic level to study? Ecol. Indic. 15, 100–104. doi: 10.1016/j.ecolind.2011.09.024
Keck F., Couton M., Altermatt F. (2023). Navigating the seven challenges of taxonomic reference databases in metabarcoding analyses. Mol. Ecol. Resour. 23, 742–755. doi: 10.1111/1755-0998.13746
Krehenwinkel H., Wolf M., Lim J. Y., Rominger A. J., Simison W. B., Gillespie R. G. (2017). Estimating and mitigating amplification bias in qualitative and quantitative arthropod metabarcoding. Sci. Rep. 7, 1–12. doi: 10.1038/s41598-017-17333-x
Lara-Pérez L. A., Villanueva-López G., Oros-Ortega I., Aryal D. R., Casanova-Lugo F., Ghimire R. (2023). Seasonal variation of arthropod diversity in agroforestry systems in the humid tropics of Mexico. Arthropod-Plant Interact. 17, 799–810. doi: 10.1007/s11829-023-10001-0
Leray M., Yang J. Y., Meyer C. P., Mills S. C., Agudelo N., Ranwez V., et al. (2013). A new versatile primer set targeting a short fragment of the mitochondrial COI region for metabarcoding metazoan diversity: application for characterizing coral reef fish gut contents. Front. Zoology 10, 34. doi: 10.1186/1742-9994-10-34
Lorusso L., Piredda R., Mottola A., Intermite C., Ranieri L., Carpino S., et al. (2024). Authentication of seafood species on the ASFIS list (FAO) by in-silico evaluation of primers for metabarcoding. Food Control 165, 110663. doi: 10.1016/j.foodcont.2024.110663
Lynggaard C., Nielsen M., Santos-Bay L., Gastauer M., Oliveira G., Bohmann K. (2019). Vertebrate diversity revealed by metabarcoding of bulk arthropod samples from tropical forests. Environ. DNA (Hoboken N.J.) 1, 329–341. doi: 10.1002/edn3.v1.4
Lynggaard C., Yu D. W., Oliveira G., Caldeira C. F., Ramos S. J., Ellegaard M. R., et al. (2020). DNA-based arthropod diversity assessment in amazonian iron mine lands show ecological succession towards undisturbed reference sites. Front. Ecol. Evol. 8, 426. doi: 10.3389/fevo.2020.590976
MacDonald A. J., Sarre S. D. (2017). A framework for developing and validating taxon-specific primers for specimen identification from environmental DNA. Mol. Ecol. Resour. 17, 708–720. doi: 10.1111/men.2017.17.issue-4
Makiola A., Compson Z. G., Baird D. J., Barnes M. A., Boerlijst S. P., Bouchez A., et al. (2020). Key questions for next-generation biomonitoring. Front. Environ. Sci. Eng. China 7. doi: 10.3389/fenvs.2019.00197
Massey A. L., Bronzoni R. V., de M., da Silva D. J. F., Allen J. M., de Lázari P. R., et al. (2022). Invertebrates for vertebrate biodiversity monitoring: Comparisons using three insect taxa as iDNA samplers. Mol. Ecol. Resour. 22, 962–977. doi: 10.1111/1755-0998.13525
Mathieu C., Hermans S. M., Lear G., Buckley T. R., Lee K. C., Buckley H. L. (2020). A systematic review of sources of variability and uncertainty in eDNA data for environmental monitoring. Front. Ecol. Evol. 8. doi: 10.3389/fevo.2020.00135
Mazurkiewicz M., Pawłowska J., Barrenechea Angeles I., Grzelak K., Deja K., Zaborska A., et al. (2024). Sediment DNA metabarcoding and morphology provide complementary insight into macrofauna and meiobenthos response to environmental gradients in an Arctic glacial fjord. Mar. Environ. Res. 198, 106552. doi: 10.1016/j.marenvres.2024.106552
McMurdie P. J., Holmes S. (2013). phyloseq: an R package for reproducible interactive analysis and graphics of microbiome census data. PloS One 8, e61217. doi: 10.1371/journal.pone.0061217
Menta C., Conti F. D., Pinto S., Leoni A., Lozano-Fondón C. (2014). Monitoring soil restoration in an open-pit mine in northern Italy. Appl. Soil Ecology: A Section Agriculture Ecosyst. Environ. 83, 22–29. doi: 10.1016/j.apsoil.2013.07.013
Mercier C., Boyer F., Bonin A., Coissac E. (2013). SUMATRA and SUMACLUST: fast and exact comparison and clustering of sequences. Available online at: http://metabarcoding.org/sumatra. (accessed January 20, 2023)
Montgomery G. A., Belitz M. W., Guralnick R. P., Tingley M. W. (2021). Standards and best practices for monitoring and benchmarking insects. Front. Ecol. Evol. 8. doi: 10.3389/fevo.2020.579193
Mori A. S., Suzuki K. F., Hori M., Kadoya T., Okano K., Uraguchi A., et al. (2023). Perspective: sustainability challenges, opportunities and solutions for long-term ecosystem observations. Philos. Trans. R. Soc. London. Ser. B Biol. Sci. 378, 20220192. doi: 10.1098/rstb.2022.0192
Murray D. C., Coghlan M. L., Bunce M. (2015). From benchtop to desktop: important considerations when designing amplicon sequencing workflows. PloS One 10, e0124671. doi: 10.1371/journal.pone.0124671
Nakano M. (2018). 16S rRNA gene primer validation for bacterial diversity analysis of vegetable products. J. Food Prot. 81 (5), 848–859. doi: 10.4315/0362-028X.JFP-17-346
Nascimento F. S., Gastauer M., Souza-Filho P. W. M., Nascimento W. R. Jr., Santos D. C., Costa M. F. (2020). Land cover changes in open-cast mining complexes based on high-resolution remote sensing data. Remote Sens. 12 (4), 611. doi: 10.3390/rs12040611
Nielsen M., Gilbert M. T. P., Pape T., Bohmann K. (2019). A simplified DNA extraction protocol for unsorted bulk arthropod samples that maintains exoskeletal integrity. Environ. DNA (Hoboken N.J.) 1, 144–154. doi: 10.1002/edn3.2019.1.issue-2
Nørgaard L., Olesen C. R., Trøjelsgaard K., Pertoldi C., Nielsen J. L., Taberlet P., et al. (2021). eDNA metabarcoding for biodiversity assessment, generalist predators as sampling assistants. Sci. Rep. 11, 1–12. doi: 10.1038/s41598-021-85488-9
Oksanen J., Blanchet F. G., Friendly M., Kindt R., Legendre P., McGlinn D., et al. (2017).Vegan: community ecology package. Available online at: https://cran.r-project.org/package=vegan. (accessed January 20, 2023)
Pedro P. M., Cullen L. Jr., Prado F., Uezu A., Piper R., Faria C. M. A., et al. (2023). Ecological monitoring using Collembola metabarcoding with extremely low bycatch amplification. Ecol. Indic. 157, 111202. doi: 10.1016/j.ecolind.2023.111202
Pires M. M., Grech M. G., Stenert C., Maltchik L., Epele L. B., McLean K. I., et al. (2021). Does taxonomic and numerical resolution affect the assessment of invertebrate community structure in New World freshwater wetlands? Ecol. Indic. 125, 107437. doi: 10.1016/j.ecolind.2021.107437
Schubert M., Lindgreen S., Orlando L. (2016). AdapterRemoval v2: rapid adapter trimming, identification, and read merging. BMC Res. Notes 9, 88. doi: 10.1186/s13104-016-1900-2
Solascasas P., Azcárate F. M., Hevia V. (2022). Edaphic arthropods as indicators of the ecological condition of temperate grassland ecosystems: A systematic review. Ecol. Indic. 142, 109277. doi: 10.1016/j.ecolind.2022.109277
Taberlet P., Coissac E., Pompanon F., Brochmann C., Willerslev E. (2012). Towards next-generation biodiversity assessment using DNA metabarcoding. Mol. Ecol. 21, 2045–2050. doi: 10.1111/j.1365-294X.2012.05470.x
Tsuji S., Inui R., Nakao R., Miyazono S., Saito M., Kono T., et al. (2022). Quantitative environmental DNA metabarcoding shows high potential as a novel approach to quantitatively assess fish community. Sci. Rep. 12, 1–11. doi: 10.1038/s41598-022-25274-3
Umair Sial M., Zeeshan Majeed M., Atiq A., Farooq T., Aatif H. M., Jaleel W., et al. (2022). Differential efficacy of edaphic traps for monitoring arthropods diversity in subtropical regions. J. King Saud Univ. - Sci. 34, 101686. doi: 10.1016/j.jksus.2021.101686
van der Heyde M., Bunce M., Dixon K. W., Fernandes K., Majer J., Wardell-Johnson G., et al. (2022a). Evaluating restoration trajectories using DNA metabarcoding of ground-dwelling and airborne invertebrates and associated plant communities. Mol. Ecol. 31, 2172–2188. doi: 10.1111/mec.16375
van der Heyde M., Bunce M., Nevill P. (2022b). Key factors to consider in the use of environmental DNA metabarcoding to monitor terrestrial ecological restoration. Sci. Total Environ. 848, 157617. doi: 10.1016/j.scitotenv.2022.157617
van Klink R., August T., Bas Y., Bodesheim P., Bonn A., Fossøy F., et al. (2022). Emerging technologies revolutionise insect ecology and monitoring. Trends Ecol. Evol. 37, 872–885. doi: 10.1016/j.tree.2022.06.001
Vasar M., Davison J., Moora M., Sepp S.-K., Anslan S., Al-Quraishy S., et al. (2023). Metabarcoding of soil environmental DNA to estimate plant diversity globally. Front. Plant Sci. 14, 1106617. doi: 10.3389/fpls.2023.1106617
Viana P. L., Mota N. F. O., Gil A. S. B., Salino A., Zappi D. C., Harley R. M., et al. (2016). Flora das cangas da Serra dos Carajás, Pará, Brasil: história, área de estudos e metodologia. Rodriguésia 67, 1107–1124. doi: 10.1590/2175-7860201667501
Wilkinson S. P., Gault A. A., Welsh S. A., Smith J. P., David B. O., Hicks A. S., et al. (2024). TICI: a taxon-independent community index for eDNA-based ecological health assessment. PeerJ 12, e16963. doi: 10.7717/peerj.16963
Willassen E., Westgaard J.-I., Kongsrud J. A., Hanebrekke T., Buhl-Mortensen P., Holte B. (2023). Benthic invertebrates in Svalbard fjords—when metabarcoding does not outperform traditional biodiversity assessment. PeerJ 10, e14321. doi: 10.7717/peerj.14321
Yang C., Bohmann K., Wang X., Cai W., Wales N., Ding Z., et al. (2021). Biodiversity Soup II: A bulk-sample metabarcoding pipeline emphasizing error reduction. Methods Ecol. Evol. / Br. Ecol. Soc. 12, 1252–1264. doi: 10.1111/2041-210X.13602
Zeale M. R. K., Butlin R. K., Barker G. L. A., Lees D. C., Jones G. (2011). Taxon-specific PCR for DNA barcoding arthropod prey in bat faeces. Mol. Ecol. Resour. 11, 236–244. doi: 10.1111/j.1755-0998.2010.02920.x
Zhang G. K., Chain F. J. J., Abbott C. L., Cristescu M. E. (2018). Metabarcoding using multiplexed markers increases species detection in complex zooplankton communities. Evolutionary Appl. 11, 1901–1914. doi: 10.1111/eva.2018.11.issue-10
Zhang S., Zhao J., Yao M. (2020). A comprehensive and comparative evaluation of primers for metabarcoding eDNA from fish. Methods Ecol. Evol. / Br. Ecol. Soc. 11, 1609–1625. doi: 10.1111/mec.15060
Zhu X., Gao B., Yuan S., Hu Y. (2010). Community structure and seasonal variation of soil arthropods in the forest-steppe ecotone of the mountainous region in Northern Hebei, China. J. Mountain Sci. 7, 187–196. doi: 10.1007/s11629-010-0198-0
Zinger L., Bonin A., Alsos I. G., Bálint M., Bik H., Boyer F., et al. (2019). DNA metabarcoding-Need for robust experimental designs to draw sound ecological conclusions. Mol. Ecol. 28, 1857–1862. doi: 10.1111/mec.2019.28.issue-8
Keywords: primer selection, DNA in bulk samples, taxonomic coverage, malaise traps, DNA metabarcoding
Citation: Penner FV, Lynggaard C, Argolo LA, da Silva YKR, de Oliveira GC, Bohmann K and Gastauer M (2024) Navigating diversity: primer impact on arthropod recovery in molecular environmental monitoring in tropical Amazon. Front. Ecol. Evol. 12:1411388. doi: 10.3389/fevo.2024.1411388
Received: 02 April 2024; Accepted: 30 October 2024;
Published: 04 December 2024.
Edited by:
Mariana M. Vale, Federal University of Rio de Janeiro, BrazilReviewed by:
Daniel Dias Dornelas Do Carmo, University of São Paulo, BrazilDaniela Takiya, Federal University of Rio de Janeiro, Brazil
Copyright © 2024 Penner, Lynggaard, Argolo, da Silva, de Oliveira, Bohmann and Gastauer. This is an open-access article distributed under the terms of the Creative Commons Attribution License (CC BY). The use, distribution or reproduction in other forums is permitted, provided the original author(s) and the copyright owner(s) are credited and that the original publication in this journal is cited, in accordance with accepted academic practice. No use, distribution or reproduction is permitted which does not comply with these terms.
*Correspondence: Markus Gastauer, bWFya3VzLmdhc3RhdWVyQGl0di5vcmc=