- 1Liaoning Provincial Key Laboratory for Hydrobiology, College of Fisheries and Life Science, Dalian Ocean University, Dalian, China
- 2Shanxi Key Laboratory of Earth Surface System and Environmental Carrying Capacity, College of Urban and Environmental Sciences, Northwest University, Xi’an, China
Understanding biodiversity changes, along with its driving factors and mechanisms, is crucial in biogeography and community ecology. Beta diversity is an indicator for discerning the compositional variations among communities and is essential role in fostering a comprehensive understanding of the ecological processes shaping biodiversity distribution patterns. This study quantitatively evaluated the beta diversity of macroinvertebrates in the Wei River Basin, focusing on: (i) contributions of turnover and nestedness to taxonomic, functional, and phylogenetic total beta diversity, (ii) correlations between these beta diversity dimensions, and (iii) the influences of local, climatic, and spatial factors on beta diversity. We analyzed macroinvertebrate data from 51 sampling locations in the Wei River Basin and explored the relationships between different beta diversity dimensions using the Mantel test. Additionally, we assessed the relative influence of local, climatic, and spatial factors on beta diversity through distance-based redundancy analysis and variance partitioning methods. The results indicated that turnover predominantly affects taxonomic and phylogenetic beta diversity, while nestedness mainly drives functional beta diversity. Functional beta diversity was more weakly associated with the other dimensions. Local environmental factors significantly influenced taxonomic and phylogenetic beta diversity, whereas spatial factors had a stronger influence on functional beta diversity. Our findings highlight the need for conservation strategies to integrate taxonomic, functional, and phylogenetic dimensions, transcending traditional species-level approaches.
1 Introduction
The biodiversity crisis is occurring globally (Thomas et al., 2004; Mantyka-Pringle et al., 2015; Segan et al., 2016), and the focus has turned to river ecosystems for their unique ecosystem functions and sensitivity to global change (Val et al., 2016). Rivers participate in the material cycle and provide ecosystem services such as fresh water supply, flood regulation, water purification, and fishery products (Acreman and Dunbar, 2004; Opperman et al., 2009; Kozak et al., 2016) (Alexander et al., 2000). However, river hydrology is affected by precipitation change caused by industrial production (Trenberth, 2011), groundwater exploitation (Konikow and Kendy, 2005), and agricultural irrigation (Rozelle et al., 1997). Furthermore, river landscape fragmentation is affected by urbanization, agricultural expansion, and hydropower stations (Fuller et al., 2015; Sahraoui et al., 2017). Moreover, water quality is polluted due to fertilizers and pesticides in agricultural runoff (Liu et al., 2021), municipal wastewater inputs, and industrial discharge (Gençer and Başaran, 2023; Mummidivarapu et al., 2023). River ecosystems are undergoing unexpected changes in the Anthropocene (Blocher et al., 2020). Thus, a better understanding of the river ecosystem response to environmental change is needed to preserve this important and sensitive ecosystem.
Based on the diversity stability hypothesis (which suggests that ecosystems with higher biodiversity tend to exhibit greater stability), understanding changes in river biodiversity might benefit river ecosystem maintenance (Allan and Flecker, 1993; Ives and Carpenter, 2007; Goertzen et al., 2022). In the 1960s, the American ecologist Robert. H. Whittaker proposed three different diversity scales: alpha diversity, beta diversity, and gamma diversity (Whittaker, 1960). Alpha diversity refers to the species richness of a particular community, beta diversity indicates the species composition variation among different communities, and gamma diversity represents the sum of species richness of multiple communities. Beta diversity aids the revelation of ecological processes compared to alpha and gamma diversity, species distribution patterns, and interactions between different habitats, thus revealing the community composition mechanism (Rosenzweig, 1995; Chen et al., 2010; Legendre and De Cáceres, 2013). Many previous works noted that the difference in species composition in different communities (total beta diversity) comprises species turnover (or replacement) and species loss (or gain) (Harrison et al., 1992; Williams, 1996; Lennon et al., 2002). Species turnover can in particular express the assembly of different species adapted to different environmental conditions across communities, whereas species loss reflects partial overlap in species composition among communities. Typically, high species losses can be attributed to factors such as dispersal limitations, dissimilar environments, and other influences (Jones et al., 2021). In 2010, Baselga proposed the BAS approach to decompose total beta diversity into turnover and nestedness components to distinguish between the contributions of these two processes to total beta diversity and explore how they affect species distribution patterns in different temporal and spatial dimensions (Baselga, 2010). These two components are intrinsically linked to critical ecological dynamics within river ecosystems, reflecting the effects of environmental gradients or habitat changes on species distributions (Soininen et al., 2007; Ulrich and Gotelli, 2007) and shifts in distributional patterns due to stochastic dispersal or extinction events (Alexander et al., 2000; Paine, 2006). While several studies utilizing the BAS methodology have examined the ecological underpinnings of community composition, investigations extending to functional and phylogenetic beta diversity remain limited.
Recent ecology research trends have incorporated functional and phylogenetic dimensions into beta diversity research, aiming to enrich the understanding of community composition shifts (Muvengwi et al., 2022). Traditional beta diversity metrics focus predominantly on species-level distinctions, clarifying the variation and distribution patterns within species compositions (Beaujour et al., 2024). Nonetheless, relying solely on analyses centered on the presence or absence of species might be inadequate to fully portray community dynamics and ecological processes. Therefore, there is a growing shift in research toward more integrated analyses, such as functional and phylogenetic beta diversity (Lin et al., 2024). Investigating the functional species’ beta diversity elucidates how they influence ecological processes and respond to environmental changes through their functional attributes. Communities, even those with compromised species diversity, can nonetheless uphold essential ecosystem processes and services by preserving functional diversity (Dı́az and Cabido, 2001). Additionally, phylogenetic diversity analyses highlight the importance of conserving evolutionarily unique species, ensuring that evolutionarily irreplaceable species are prioritized in conservation strategy formulation (Pellens et al., 2016). Functional and phylogenetic beta diversity studies present additional possibilities for understanding community composition. Nevertheless, there remains no current unified perspective on how abiotic factors affect these different beta diversity dimensions. Thus, there is an urgent need for further research to develop a more comprehensive and coherent understanding.
Organisms interact with their habitat constantly, collectively shaping the multi-scale biodiversity patterns of river ecosystems (Gorzel and Kornijów, 2007). Unraveling the influence of abiotic factors on community dynamics, particularly those related to local environmental conditions, topographic variables, spatial distribution, climate variations, and land use changes, is vital for deciphering riverine biodiversity ecological mechanisms (Rousi et al., 2013). Traditional studies have primarily focused on the influence of local environmental factors such as water chemistry and temperature on macroinvertebrate distributions, underscoring their foundational role in dictating species distributions. The significance of these local environmental factors is extensively acknowledged in ecological research and application (Liu et al., 2023). However, recent studies have revealed that, in addition to local environmental factors, spatial patterns and topographic factors also significantly mold biodiversity, especially in fluvial contexts (Peláez and Pavanelli, 2018). Spatial patterns are closely linked to dispersal limitation, and topographically related abiotic factors might also define change gradients in biological communities and functional characteristics (Vannote et al., 1980; Wang et al., 2023). Simultaneously, global changes represent a formidable biodiversity challenge (Liermann et al., 2012; Soininen et al., 2016; Dudgeon, 2019; Reid et al., 2019; Tickner et al., 2020; Comte et al., 2021; Caro et al., 2022; Mouton et al., 2022; Manfrin et al., 2023) in which global warming-driven alterations in precipitation and temperature patterns have directly affected river water and sediment dynamics (Arnell and Gosling, 2013; Schneider et al., 2013; Peng et al., 2020; Syvitski et al., 2022). Concurrently, land use changes such as deforestation, intensive farming, and urban expansion typically result in habitat degradation and species distribution shifts (Allan, 2004; Vörösmarty et al., 2010), influencing community stability and functionality. This new understanding has compelled ecologists to reassess the significance of abiotic factors in biodiversity conservation and ecosystem management.
In the present study, we conducted a field biodiversity survey in the Wei River Basin in May 2023 to gain a deeper understanding of macroinvertebrate beta diversity in the Wei River Basin and its drivers. Specifically, we concentrated on three dimensions of beta diversity: taxonomic, functional, and phylogenetic, alongside their respective turnover and nestedness. These diversity metrics represent distinct community facets and might exhibit varied responses to abiotic factors. As different dimensions of biodiversity might be interdependent, we hypothesized that taxonomic, functional, and phylogenetic beta diversity are significantly correlated (Hypothesis 1 [H1]). However, considering that different species can perform similar ecological functions, we anticipated lower functional beta diversity than taxonomic and phylogenetic beta diversity (H2). Acknowledging the critical role of environmental filtering in determining community structure, we expect that beta diversity is predominantly influenced by species turnover rather than nestedness (H3). Lastly, we proposed that beta diversity patterns are primarily shaped by local environmental factors as opposed to spatial factors and climatic factors (H4).
2 Materials and methods
2.1 Study area
The Wei River Basin is an integral tributary basin of the Yellow River and is situated in northwest China. The basin encompasses the main channel, the Wei River, and two significant secondary tributaries: the Jing River and the Beiluo River. The basin is characterized by a predominantly semi-arid continental monsoon climate, experiencing hot and rainy summers due to the western Pacific subtropical high pressure and cold, dry winters with minimal rainfall under the influence of Mongolian high pressure (Zhu et al., 2017). This basin is an ideal region for examining beta diversity patterns and their underlying mechanisms. This is primarily because the watershed spans a extensive area of 134,000 km², which constitutes 17.9% of the total Yellow River Basin. The basin features a diverse range of topographic elements, from towering mountains to expansive plains, and has an elevation gradient exceeding 3,000 meters, which aids comprehension and forecasting of the biome responses to spatial factors. Second, the upstream portion of the Wei River is characterized by low population density, minimal agricultural and industrial activities, reduced human intervention, and high vegetation cover. Contrastingly, the downstream plains are crucial agricultural production zones with higher urbanization and industrialization levels, leading to more pronounced environmental issues. This stark environmental contrast within the watershed provides an excellent opportunity to study how environmental filtering and human activities influence community composition.
2.2 Macroinvertebrate sampling
In May 2023, we selected 51 sites across the Wei River Basin for sample collection (Figure 1). At each site, we attached a D-shaped net closely to the riverbed, agitated the sediment upstream of the net, and dragged the net for 100 m against the current to gather qualitative samples. The collected samples were processed by filtering out impurities using a 60-mesh circular sieve. The organisms that passed this initial filtration were placed into white porcelain trays. The obtained macroinvertebrates were manually sorted on-site using tweezers. Subsequently, the samples were preserved in 100-mL wide-mouthed plastic bottles filled with 75% ethanol solution. In the laboratory, the samples were carefully sorted and identified using a microscope. The vast majority of the samples were identified down to the genus level.
2.3 Species traits
Trait information for all genus observed in this study was obtained from the literature (Vieira et al., 2006). Functional traits were chosen across four dimensions (life history, mobility, morphology, and ecology) to evaluate the genus’ functional diversity more comprehensively and predict their responses to environmental changes. After reviewing various studies, nine functional traits, including voltinism, female dispersal, and drift, were ultimately selected for analysis (Supplementary Appendix S3).
2.4 Environmental variables
Geographic coordinates were recorded at each sampling site, and 11 in situ environmental parameters, including stream width, flow velocity, and water depth, were measured directly. Furthermore, surface water samples were collected, acidified, and transported to the laboratory for analysis. In the lab, eight water chemistry parameters, including total nitrogen (TN), total phosphorus (TP), and sulfate ions (SO₄²⁻), were analyzed and measured. These data were first log-transformed (log₁₀(x+1)) to reduce the influence of outliers, and then z-score standardized (Müller and Guido, 2016). After these transformations, the data were incorporated into the abiotic dataset as variables reflecting local environmental conditions to ensure appropriate statistical treatment (Supplementary Appendix S1).
2.5 Climatic variables
The climatic variables in the abiotic dataset comprised three land use types and 19 bioclimatic variables obtained from remote sensing data. Specifically, the land use information was sourced from the 2021 China Landsat-based Annual Land Cover product (CLCD) (Yang and Huang, 2022). Referring to the methodologies of previous studies, we calculated the percentage of each land use type within a 1-km radius of each sampling point (Hilary et al., 2021). The original dataset contained six land cover types: cropland, forest, shrub, grassland, water, and impervious surfaces. However, for this study, we consolidated shrub, grassland, and forest into a single category (“greenfield”) and included impervious surfaces and cropland in our analysis. Simultaneously, we extracted 19 bioclimatic variables (BIO1–BIO19), including mean annual temperature, daily temperature difference, and annual precipitation, for each site from the WorldClim2 database. This database presents global average climate data at 30-s resolution covering the period from 1970 to 2000 (Fick and Hijmans, 2017). Before integrating these data into the abiotic dataset, we standardized the data scale and distribution using z-score transformations (Supplementary Appendix S1).
2.6 Spatial variables
The topographic information for each sampling location was extracted from the SRTM4.1 database at a resolution of 90 m. This database encompasses 10 topographic factors, including elevation, slope direction, and vector ruggedness measure (VRM) (Amatulli et al., 2018). In addition, we analyzed spatial eigenfunctions using a distance-based Moran eigenroot map (dbMEM) (Dray et al., 2006). This method reveals unmeasured large-scale changes due to natural dispersal or historical factors, such as dispersal dynamics at internal local scales or migration history at regional scales. The method represents geographic distances from small to large scales. In general, dbMEM vectors with large eigenvalues represent large-scale spatial patterns. In our study, 15 dbMEM eigenvectors were generated using the dbMEM function in the adespatial package and utilized for further analysis (Dray et al., 2018).
2.7 Data analysis
Beta diversity based on taxonomic units was computed using a presence/absence data-based approach utilizing the Sørensen similarity index (Sørensen, 1948). This method is utilized to measure differences in species composition between communities and uncover variation patterns within species composition. We implemented the beta function from the BAT package, enabling the decomposition of total taxonomic beta diversity into two principal components: turnover and nestedness (Cardoso et al., 2015). Turnover generally indicates the species replacement patterns between sites, reflecting how different communities are in terms of species composition. Nestedness relates to species loss or extinction processes, indicating how subsets of species from richer sites are present in poorer ones. This decomposition approach is essential for understanding community composition dynamics, biogeographic distribution patterns, and mechanisms for maintaining species diversity.
We extended this taxonomic unit-based approach to studying functional and phylogenetic beta diversity, again using the BAT package in R. We calculated functional beta diversity using Gower distances based on trait data, which are used to calculate trait differences between species (Laliberté and Legendre, 2010). Subsequently, we processed these trait distance matrices by principal coordinate analysis (PCoA). We combined them with the species presence/absence matrices to generate trait-based beta diversity matrices and their turnover and nestedness components. This process revealed the distribution patterns of functional traits of different specie.
Finally, phylogenetic beta diversity and its components were evaluated using the taxa2dist function from the vegan package to calculate the phylogenetic distances between species (Dixon, 2003). This step uses a matrix of interspecific taxonomic distances to assess the relative positions of species on a phylogenetic tree, which allows the quantitative analysis of the phylogenetic relationships of species in a community and their role in community construction. This approach facilitates the understanding of beta diversity and its turnover and nestedness components from a phylogenetic perspective, providing a unique perspective for exploring biodiversity evolution. Finally, we plotted ternary kernel density maps (Figure 2) of taxonomic, functional, and phylogenetic beta diversity and its decomposition components using the ggtern package in R to visualize the relationships between them (Hamilton and Ferry, 2018).
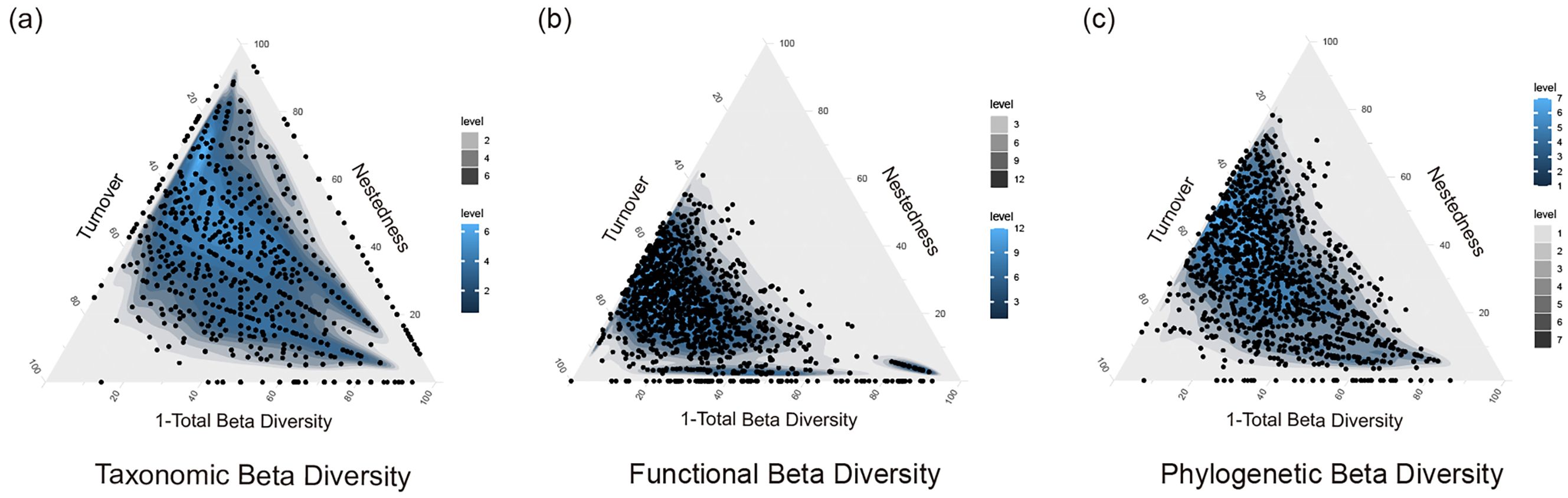
Figure 2. Ternary kernel density plots of beta diversity: (A) distribution of data points for '1-total beta diversity', turnover, and nestedness components of taxonomic level; (B) distribution of data points for '1-total beta diversity', turnover, and nestedness components of functional level; (C) distribution of data points for '1-total beta diversity', turnover, and nestedness components of phylogenetic level. The depth of the color represents the data point density, with the legend numbers progressively indicating levels of color depth, reflecting the gradual increase in density.
We investigated the relationships between taxonomic, functional, and phylogenetic beta diversity and their respective components using the mantel function from the vegan package in R. In the Mantel test, the statistic r represents the strength of the correlation between two diversity indicator matrices. A higher r-value indicates a stronger correlation. We ensured the statistical significance of our findings by conducting 9999 permutation tests.
We determined the effect of local environmental, climatic, and spatial variables on beta diversity and its components using the capscale function in the vegan package to conduct a distance-based redundancy analysis (db-RDA). Contrary to traditional redundancy analysis (RDA), which mainly relies on Euclidean distances, db-RDA facilitates the employment of non-Euclidean distances, such as the Bray-Curtis distance. The db-RDA significantly extends RDA applicability to statistical analyses in ecology (Legendre and Anderson, 1999).
Before formally conducting the db-RDA, we screened all abiotic datasets for variables that significantly affected changes in beta diversity and its components through forward selection (Blanchet et al., 2008). This process was based on 999 randomized permutation tests with p ≤ 0.05 as the criterion for determining significance. Variables exhibiting excessive covariance among the screened factors were excluded, adhering to the variance inflation factor (VIF) principle, with a threshold set at ≤10. Following this, variance decomposition analysis (VPA) was employed to determine the relative effects of local environmental, climatic, and spatial factors on beta diversity and its components more accurately (Figure 3). VPA is frequently utilized in ecology to uncover the different ecological processes influencing community assembly. It can determine the proportion of beta diversity attributed to specific abiotic factors, helping to identify the independent and interactive contributions of different categories of abiotic factors. We conducted this analysis using the varpart function from the vegan package.
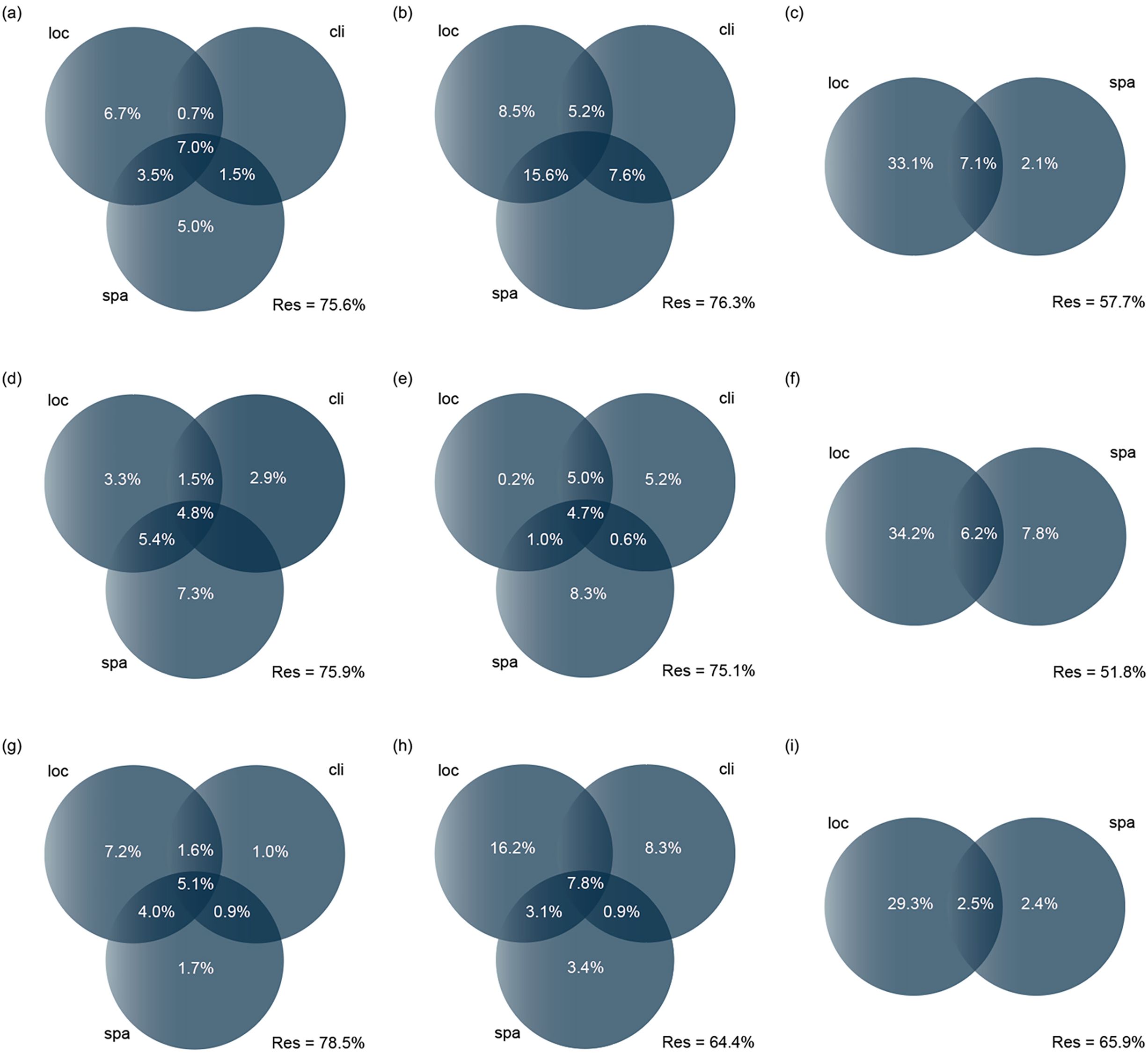
Figure 3. Relative contributions of local environmental factors (Local), climatic factors (Climatic), and spatial factors (Spatial) to taxonomic, functional, and phylogenetic beta diversity components (total, turnover, and nestedness). Adjusted R² values were used (values < 0 are not shown); res indicates unexplained variation (total variation = 100). Specifically: (A) represents total beta diversity at the taxonomic level, (B) represents turnover at the taxonomic level, (C) represents nestedness at the taxonomic level; (D) represents total beta diversity at the functional level, (E) represents turnover at the functional level, (F) represents nestedness at the functional level; (G) represents total beta diversity at the phylogenetic level, (H) represents turnover at the phylogenetic level, (I) represents nestedness at the phylogenetic level.
3 Results
3.1 Three aspects of beta diversity decomposition
We collected 90 macroinvertebrates (Supplementary Appendix S2) at the 51 sampling sites (average species richness: 10.88 per site). Calculating beta diversity and its components revealed that, as shown in Table 1 and Figure 2, at the taxonomic level, total beta diversity (mean ± SD: 0.70 ± 0.147) was mainly driven by turnover (mean ± SD: 0.38 ± 0.200) and complemented by nestedness (mean ± SD: 0.31 ± 0.217). For the functional level, total beta diversity (mean ± SD: 0.44 ± 0.138) was primarily driven by nestedness (mean ± S D: 0.23 ± 0.191), followed by turnover (mean ± SD: 0.21 ± 0.127). At the phylogenetic level, total beta diversity (mean ± SD: 0.57 ± 0.127) was mainly determined by turnover (mean ± SD: 0.31 ± 0.170) and complemented by nestedness (mean ± SD: 0.27 ± 0.187) (Table 1). This result demonstrated that taxonomic beta diversity was higher than phylogenetic beta diversity, which was higher than functional beta diversity, which supported H1.
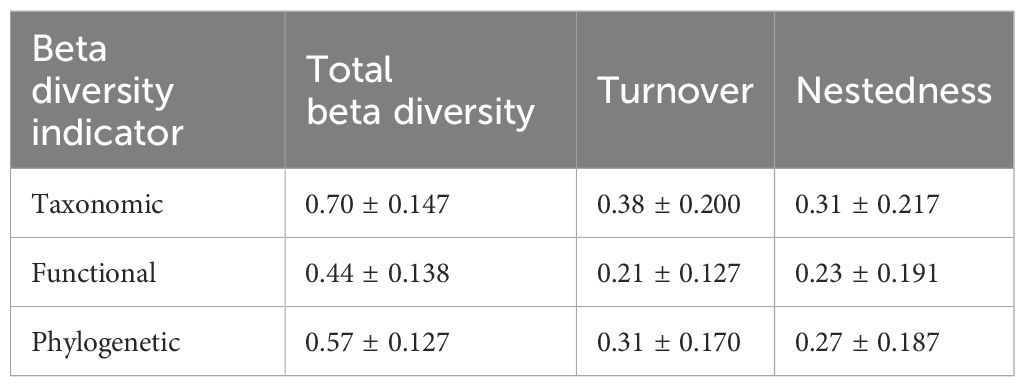
Table 1. Three dimensions (taxonomic, functional, and phylogenetic) of beta-diversity composition (i.e., total, turnover, and nestedness) of macroinvertebrate communities in the Wei River Basin.
3.2 Correlations between taxonomic, functional, and phylogenetic beta diversity components
We assessed the correlations between different beta diversity scales using the Mantel test (Table 2). The results demonstrated a high correlation between taxonomic and phylogenetic total beta diversity (r = 0.918). However, the correlation between functional total beta diversity and the other two scales was relatively low (r = 0.668 and r = 0.658). Among the beta diversity decomposition components, the nestedness component demonstrated the highest correlation between taxonomic and functional, functional and phylogenetic, or taxonomic and phylogenetic beta diversity. The significant correlations shown between functional, taxonomic, and phylogenetic beta diversity and its decomposition components (p < 0.001) supported H2.
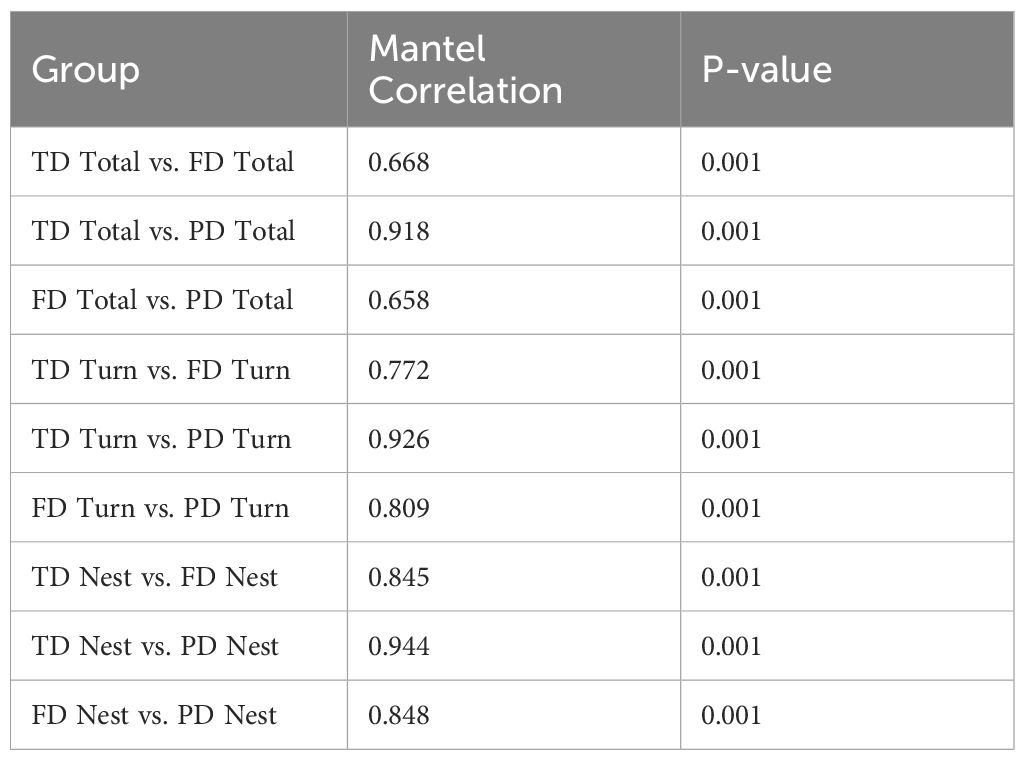
Table 2. Mantel correlation analysis between taxonomic, functional, phylogenetic beta diversity and its components.
3.3 Critical drivers of taxonomic, functional, and phylogenetic beta diversity components
We categorized abiotic factors into local environmental factors, including hydrologic and hydrochemical factors reflecting microhabitat differences in communities; climatic factors, which relate to global change and human activities, such as bioclimatic variables and land-use types; and spatial factors, which include dbMEM vectors reflecting spatial distribution patterns at large scales, and topographic features at small scales. Selection of multivariate distance matrix regression (db-RDA) models revealed that the screened local environmental, climatic, and spatial factors were statistically significant (p < 0.05) in permutation tests on taxonomic, functional, and phylogenetic beta diversity and its components (total, turnover, and nestedness).
For different levels of beta diversity, the variables selected for the db-RDA model exhibited significant variability. We screened key abiotic factors during the model construction process using forward selection and covariance. Ultimately, we selected nine local environmental factors, four climatic factors, and seven spatial factors as taxonomic beta diversity components. Six local environmental factors, five climatic factors, and six spatial factors were selected for functional beta diversity. We selected eight local environmental factors, four climatic factors, and seven spatial factors for phylogenetic beta diversity analysis (Table 3). These factors influenced the macroinvertebrate community structure in the Wei River Basin differently.
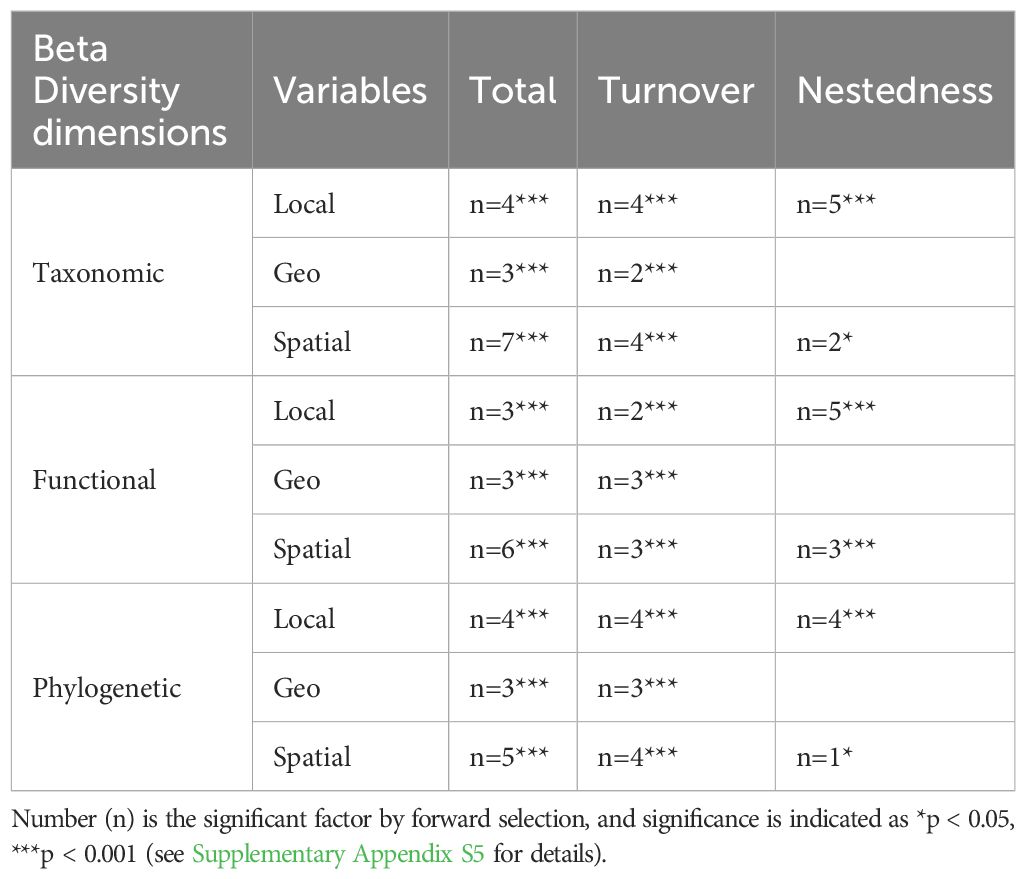
Table 3. Local environmental (Local), climatic factors (Climatic), and spatial (Spatial) factors based on the results of the forward selection of beta diversity facets and components.
Notably, the environmental factors screened for taxonomic and phylogenetic beta diversity were similar (Supplementary Appendix S5). For example, among the local factors, the parameters Na, SD, Width, TDS, DO, and Mg significantly affected the total and turnover components of taxonomic and phylogenetic beta diversity. This result indicates that these factors are critical for maintaining the species composition and the composition of the evolutionary history of communities. In contrast, local environmental factors influencing the nestedness component of beta diversity exhibited different characteristics, indicating that the mechanisms of environmental influence on species loss differ from those on species turnover. Additionally, seasonal differences in cropland and temperature emerged as common drivers of taxonomic, functional, and total phylogenetic beta diversity. Spatial factors such as MEM2, MEM3, MEM4, and MEM8 also exhibited commonality in driving macroinvertebrate communities at all three levels. The topographic factor was not selected in any forward selection model. This result indicates that the terrain variable might not be the dominant factor in explaining the beta diversity of biomes relative to other factors in the study area.
The VPA revealed the respective degree rates of local environmental factors, geoclimatic factors, and spatial factors explaining the macroinvertebrate communities and the common explanatory components among taxonomic, functional, and phylogenetic beta diversity and their respective components (total, turnover, and nestedness) (Figure 3). Specifically, (a) the local environmental factors exhibited a high pure contribution across all beta diversity types (0.2%–34.2%), especially on the nestedness component (29.3%–34.2%). This result highlighted the central role of local factors in influencing different beta diversity types. (b) The pure contribution of spatial and climatic factors is smaller than that of local factors but could reach high values of 8.3%. This point emphasized that even spatial and climatic factors should not be overlooked in shaping macroinvertebrate community structure despite being less prominent than local factors. (c) The finding that spatial factors were even more influential than local factors in the analysis of functional beta diversity, especially in the total and turnover components, revealed the importance of spatial factors in influencing the functional level, reflecting specific patterns in the functional traits of species’ spatial distribution. (d) The common explanatory component of the three abiotic factors was 0%–15.6%, emphasizing the importance of integrated consideration of multiple abiotic factors in developing biodiversity conservation and management strategies. (e) For the taxonomic level, the model explained approximately 24.4% of total beta diversity, 23.7% of turnover, and 42.3% of nestedness, respectively. For the functional level, the model explained 24.1% of total beta diversity, 24.9% of turnover, and 48.2% of nestedness. For the phylogenetic level, the model explained 21.5% of total beta diversity, 35.6% of turnover, and 34.1% of nestedness. These results partially supported H3, suggesting that local factors may influence beta diversity more than climatic and spatial factors. However, these effects manifested differently across various level and components.
4 Discussion
4.1 Contribution of turnover and nestedness
Examining differences in beta diversity across different levels is crucial for gaining a deeper understanding of community ecology. In the present study, we delineated taxonomic, functional, and phylogenetic beta diversity in macroinvertebrates. The results revealed that taxonomic beta diversity was the highest, followed by phylogenetic and functional beta diversity, which was the lowest, supporting H1. Different species might perform similar ecosystem functions, leading to functional redundancy (Walker, 1992) and explaining the lower functional beta diversity compared to the higher taxonomic beta diversity. Functional redundancy plays a significant role in ecosystem stability, reducing sensitivity to external perturbations and facilitating quicker ecosystem recovery following disturbances (Biggs et al., 2020). Additionally, our results revealed higher phylogenetic beta diversity and lower functional beta diversity, which we hypothesize might stem from the tendency of species to maintain the ecological niche characteristics of their ancestors during evolution, known as niche conservatism (PNC) (Wiens et al., 2010; Crisp and Cook, 2012). A deeper understanding of this ecological niche conservatism is essential for revealing the evolutionary history of the Wei River Basin macroinvertebrates and is also crucial for formulating effective conservation measures to maintain phylogenetic diversity by preserving ecological niche conditions, ensuring long-term ecosystem health and stability.
H2 predicted that beta diversity would be driven primarily by turnover, and we discovered that the results differed from expectations. Turnover drove taxonomic and phylogenetic beta diversity, whereas functional beta diversity was driven by nestedness. The extensive watershed area had pronounced topographic differences, and climatic gradients might have induced higher environmental heterogeneity as the reason that species turnover dominated changes in the Wei River Basin macroinvertebrate community composition (Soininen et al., 2007). Functional beta diversity by nestedness might still be associated with functional redundancy. For example, trait composition in species-rich communities might be similar to that of more species-poor communities as many species perform similar ecological functions (Guillemot et al., 2011). This aspect leads to nestedness patterns between communities. Additionally, if different habitats within the Weir River Basin exhibit similarities in certain vital environmental factors (e.g., similar substrate types), functional traits adapted to these specific environmental conditions can occur across a wide range, developing functional nestedness patterns (Larsen and Ormerod, 2010). Identifying whether turnover or nestedness drives changes in taxonomic, functional, and phylogenetic beta diversity can reveal different aspects of differences in community species composition and their distributional dynamics across environmental change gradients to identify biodiversity patterns that might be overlooked from a single perspective alone, and target conservation strategies to protect unique species or maintain ecological function.
4.2 Relationship between functional, phylogenetic, and taxonomic beta diversity
Understanding the variation and interrelationships of beta diversity at different levels can guide more effective ecological conservation and restoration strategies. Our results revealed significant correlations among taxonomic, functional, and total phylogenetic beta diversity, implying that differential increases in macroinvertebrate species in the Wei River Basin would be accompanied by a concomitant increase in differences in functional and phylogenetic beta diversity, which supported H3. Based on this finding, the triple goal of conserving evolutionarily and functionally unique species by prioritizing areas with high species diversity and those with unique species can be achieved.
Taxonomic and phylogenetic beta diversity are highly correlated (Branco et al., 2020). Our study demonstrated the same trend, which might be due to phylogenetic niche conservatism (PNC) (Losos, 2008). Specifically, when specific types of environmental selection contribute to more frequent occurrences of specific phylogenetic lineages, the taxonomic and phylogenetic divergence of these species might increase simultaneously. Furthermore, although taxonomic and functional beta diversity were significantly correlated, the correlation coefficients were lower than those between taxonomic and phylogenetic beta diversity, which we hypothesized were due to functional redundancy causing decoupling between diversities (Oliveira et al., 2016). Functional beta diversity reveals the distribution and diversity of different functions in ecosystems, and exploring its relationship with taxonomic and phylogenetic beta diversity can aid in understanding the contribution of species or phylogenetic diversity to ecosystem functioning and services, and the potential effect of diversity loss on ecosystem functioning.
4.3 Contribution of three abiotic factors to beta diversity components
Deterministic and stochastic processes are commonly used in community ecology to explain biodiversity patterns (Thompson and Townsend, 2006). The former involves non-random determinants of species distribution and presence (environmental filtering and species interactions), leading to the predictive distribution of species along environmental gradients (Jongman et al., 1995). The latter views species existence and distribution as the result of stochastic events, emphasizing the role of drift, dispersal, and migration (Vellend et al., 2014). Deterministic processes predict that species composition changes are related to local environmental factors, while stochastic processes predict that species composition changes are linked to spatial factors due to dispersal limitation (Dumbrell et al., 2010; Powell et al., 2015).
Environmental filtering is vital in shaping riverine macroinvertebrate communities, especially from the perspective of species taxonomy and evolutionary history (Li et al., 2012; Rezende et al., 2014; Zhang et al., 2014). The VPA results revealed that mainly local environmental factors strongly influenced the taxonomic and phylogenetic beta diversity of the Wei River Basin macroinvertebrates. Specifically, the importance of this environmental influence increased significantly in the nestedness component, with local factors accounting for 33.1% and 29.3% of taxonomic and phylogenetic beta diversity, respectively. This result implied that species existence and distribution are not merely the result of random events but are determined by their adaptations to specific environmental conditions. In short, the presence of environmental filtering leads to a nestedness pattern of species, allowing certain well-adapted species to be widely distributed while others are restricted to specific environmental conditions (Viana et al., 2016).
The relative importance of deterministic and stochastic processes depends on the population dispersal capacity of the organisms studied (Guichard et al., 2014). We observed that although spatial factors (e.g., MEM) and topographic features (e.g., slope and surface roughness) significantly influenced the taxonomic and phylogenetic beta diversity of the Wei River Basin macroinvertebrates, the effects of these spatial factors appeared to be relatively minor compared to the role of local environmental factors. Other studies on dispersal limitation reported that beta diversity in groups of organisms with weaker dispersal capacity (e.g., fish) might reflect spatial processes more intensely and be less affected by environmental filtering effects. Contrastingly, groups of organisms with greater dispersal capacity (e.g., phytoplankton) might exhibit more robust environmental control and a weaker influence of spatial processes (Shurin et al., 2009). Based on this conclusion, we suggest that macroinvertebrates are less constrained by dispersal due to their ability to cross river corridors, e.g., by flight, effectively reducing the limitations imposed by spatial barriers (Li et al., 2021).
In addition to local factors and spatial elements driving community compositional mechanisms, an increasing number of recent studies have focused on human activities and global change. Therefore, we included climate factors and land use in our analysis and observed significant effects on total beta diversity and turnover, albeit the contribution of such factors was relatively low. Although the direct effect of climatic factors was relatively weak in our study, climatic factors variously affect river ecosystem structure and functioning in different geographic and ecological contexts (Döll and Zhang, 2010; Grimm et al., 2013). Therefore, although the composition and diversity of Wei River Basin macroinvertebrate communities seem to be mainly influenced by local factors, vigilance should be sustained regarding the indirect effects of global change factors on river ecosystems. Future studies should explore how global changes affect biodiversity in riverine ecosystems in different regions to understand the significance of these changes on ecosystem health and biodiversity conservation more fully.
Furthermore, we determined that spatial factors had a more significant effect on functional beta diversity, including its total beta diversity and turnover components, than local environmental factors. This result contrasted with the taxonomic and phylogenetic beta diversity, suggesting that stochastic processes primarily drive functional differences in riverine macroinvertebrate communities. We inferred that this pattern might similarly be related to functional redundancy. Although the functional characteristics of species are usually closely related to the environment in which they live, different species might carry similar functions into new habitats. Thus, when species dispersal limitation is insignificant, these functionally redundant species might be distributed across diverse geographic locations, allowing functional features to exhibit a wider spatial spread rather than taxonomically. On the other hand, there may be other environmental factors not accounted for in the analysis, which, if characterized by strong spatial structure, could have contributed to this outcome.These different results at the functional level emphasized the importance of resolving community-building mechanisms from multiple dimensions.
This study provides crucial theoretical insights into the community assembly mechanisms of large benthic macroinvertebrate in the Wei River Basin. However, the reliance on a single sampling event may present some inherent limitations. Future research should incorporate investigations of seasonal variations and long-term dynamic changes to more comprehensively elucidate the community assembly mechanisms across different dimensions. Additionally, while our study primarily focused on local environmental factors, spatial factors, and climatic factors, the residuals from the Venn diagram indicate that at least 50% of the variance remains unexplained. This suggests the presence of other potentially significant drivers, such as microhabitat differences or more complex human activities, that may not have been fully captured in our analysis. Therefore, further exploration of these critical drivers is essential to gain a more complete understanding of biodiversity patterns in river ecosystems.
5 Conclusion
Our study provided insights into the distribution patterns of macroinvertebrate communities in the Wei River Basin, emphasizing how environmental filtering, dispersal limitation, and human activities affect different levels of beta diversity in the region. In particular, the apparent discrepancy between functional diversity and taxonomic and phylogenetic diversity reflected potential functional redundancy. Comprehensive analyses from multiple perspectives are essential for advancing ecological theory and developing biodiversity conservation strategies and sustainable ecosystem management. In biodiversity conservation practice, our results emphasized the need for conservation strategies to transcend the traditional species level but integrate communities’ taxonomic, functional, and phylogenetic dimensions. Thus, conservation programs should prioritize ecological regions rich in species diversity and have unique species and functional characteristics to protect biodiversity integrity and ecosystem service continuity. By revealing the detailed structure and drivers of macroinvertebrate diversity in the Wei River Basin, this study provided a scientific basis for ecosystem management and conservation. Research and conservation measures should comprehensively consider the multidimensional biodiversity landscape to achieve long-term ecosystem health and sustainable biodiversity conservation.
Data availability statement
The raw data supporting the conclusions of this article will be made available by the authors, without undue reservation.
Ethics statement
The manuscript presents research on animals that do not require ethical approval for their study.
Author contributions
JL: Conceptualization, Formal analysis, Methodology, Software, Visualization, Writing – original draft, Writing – review & editing. MM: Data curation, Methodology, Software, Writing – review & editing. LW: Data curation, Methodology, Software, Writing – review & editing. YJ: Investigation, Visualization, Writing – review & editing. YL: Writing – review & editing. XY: Funding acquisition, Project administration, Supervision, Writing – review & editing. GL: Funding acquisition, Project administration, Supervision, Writing – review & editing. JS: Funding acquisition, Project administration, Supervision, Writing – review & editing.
Funding
The author(s) declare that financial support was received for the research, authorship, and/or publication of this article. This work was funded by the National Natural Science Foundation of China (42230513, 41977193).
Conflict of interest
The authors declare that the research was conducted in the absence of any commercial or financial relationships that could be construed as a potential conflict of interest.
Publisher’s note
All claims expressed in this article are solely those of the authors and do not necessarily represent those of their affiliated organizations, or those of the publisher, the editors and the reviewers. Any product that may be evaluated in this article, or claim that may be made by its manufacturer, is not guaranteed or endorsed by the publisher.
Supplementary material
The Supplementary Material for this article can be found online at: https://www.frontiersin.org/articles/10.3389/fevo.2024.1410915/full#supplementary-material
References
Acreman M. C., Dunbar M. J. (2004). Defining environmental river flow requirements – a review. Hydrol. Earth Syst. Sci. 8, 861–876. doi: 10.5194/hess-8-861-2004
Alexander R. B., Smith R. A., Schwarz G. E. (2000). Effect of stream channel size on the delivery of nitrogen to the Gulf of Mexico. Nature 403, 758–761. doi: 10.1038/35001562
Allan J. D. (2004). Landscapes and riverscapes: the influence of land use on stream ecosystems. Annu. Rev. Ecology Evol. Systematics 35, 257–284. doi: 10.1146/annurev.ecolsys.35.120202.110122
Allan J. D., Flecker A. S. (1993). Biodiversity conservation in running waters. BioScience 43, 32–43. doi: 10.2307/1312104
Amatulli G., Domisch S., Tuanmu M.-N., Parmentier B., Ranipeta A., Malczyk J., et al. (2018). A suite of global, cross-scale topographic variables for environmental and biodiversity modeling. Sci. Data 5, 180040. doi: 10.1038/sdata.2018.40
Arnell N. W., Gosling S. N. (2013). The impacts of climate change on river flow regimes at the global scale. J. Hydrology 486, 351–364. doi: 10.1016/j.jhydrol.2013.02.010
Baselga A. (2010). Partitioning the turnover and nestedness components of beta diversity. Global Ecol. biogeography 19, 134–143. doi: 10.1111/j.1466-8238.2009.00490.x
Beaujour P. M., Loranger-Merciris G., Cézilly F. (2024). Sites and species contribution to the β-diversity of Odonata assemblages in Haiti: Implications for conservation. Global Ecol. Conserv. 50. doi: 10.1016/j.gecco.2024.e02816
Biggs C. R., Yeager L. A., Bolser D. G., Bonsell C., Dichiera A. M., Hou Z., et al. (2020). Does functional redundancy affect ecological stability and resilience? A review and meta-analysis. Ecosphere 11, e03184. doi: 10.1002/ecs2.3184
Blanchet F. G., Legendre P., Borcard D. (2008). Forward selection of explanatory variables. Ecology 89, 2623–2632. doi: 10.1890/07-0986.1
Blocher J. R., Ward M. R., Matthaei C. D., Piggott J. J. (2020). Multiple stressors and stream macroinvertebrate community dynamics: Interactions between fine sediment grain size and flow velocity. Sci. Total Environ. 717, 137070. doi: 10.1016/j.scitotenv.2020.137070
Branco C. C. Z., Bispo P. C., Peres C. K., Tonetto A. F., Krupek R. A., Barfield M., et al. (2020). Partitioning multiple facets of beta diversity in a tropical stream macroalgal metacommunity. J. Biogeogr. 47, 1765–1780. doi: 10.1111/jbi.13879
Cardoso P., Rigal F., Carvalho J. C. (2015). BAT – Biodiversity Assessment Tools, an R package for the measurement and estimation of alpha and beta taxon, phylogenetic and functional diversity. Methods Ecol. Evol. 6, 232–236. doi: 10.1111/2041-210X.12310
Caro T., Rowe Z., Berger J., Wholey P., Dobson A. (2022). An inconvenient misconception: Climate change is not the principal driver of biodiversity loss. Conserv. Lett. 15 (3). doi: 10.1111/conl.12868
Chen S., Ouyang Z., Xu W., Xiao Yi (2010). A review of beta diversity studies. Biodiversity Sci. 18, 323–335. doi: 10.3724/sp.J.1003.2010.323
Comte L., Olden J. D., Tedesco P. A., Ruhi A., Giam X. (2021). Climate and land-use changes interact to drive long-term reorganization of riverine fish communities globally. Proc. Natl. Acad. Sci. U.S.A. 118 (27). doi: 10.1073/pnas.2011639118
Crisp M. D., Cook L. G. (2012). Phylogenetic niche conservatism: what are the underlying evolutionary and ecological causes? New Phytol. 196, 681–694. doi: 10.1111/j.1469-8137.2012.04298.x
Dixon P. (2003). VEGAN, a package of R functions for community ecology. J. Vegetation Sci. 14, 927–930. doi: 10.1111/j.1654-1103.2003.tb02228.x
Dı́az S., Cabido M. (2001). Vive la différence: plant functional diversity matters to ecosystem processes. Trends Ecol. Evol. 16, 646–655. doi: 10.1016/S0169-5347(01)02283-2
Döll P., Zhang J. (2010). Impact of climate change on freshwater ecosystems: a global-scale analysis of ecologically relevant river flow alterations. Hydrol. Earth Syst. Sci. 14, 783–799. doi: 10.5194/hess-14-783-2010
Dray S., Blanchet G., Borcard D., Clappe S., Jombart G., Larocque G., et al. (2018). adespatial: Moran’s eigenvector maps and related methods for the spatial multiscale analysis of ecological communities. R package version 0.1-1.
Dray S., Legendre P., Peres-Neto P. R. (2006). Spatial modelling: a comprehensive framework for principal coordinate analysis of neighbour matrices (PCNM). Ecol. Model. 196, 483–493. doi: 10.1016/j.ecolmodel.2006.02.015
Dudgeon D. (2019). Multiple threats imperil freshwater biodiversity in the Anthropocene. Curr. Biol. 29, R960–R967. doi: 10.1016/j.cub.2019.08.002
Dumbrell A. J., Nelson M., Helgason T., Dytham C., Fitter A. H. (2010). Relative roles of niche and neutral processes in structuring a soil microbial community. ISME J. 4, 337–345. doi: 10.1038/ismej.2009.122
Fick S. E., Hijmans R. J. (2017). WorldClim 2: new 1-km spatial resolution climate surfaces for global land areas. Int. J. Climatology 37, 4302–4315. doi: 10.1002/joc.5086
Fuller M. R., Doyle M. W., Strayer D. L. (2015). Causes and consequences of habitat fragmentation in river networks. Ann. New York Acad. Sci. 1355, 31–51. doi: 10.1111/nyas.12853
Gençer E., Başaran C. (2024). Water quality assessment and pollution of Akarçay River, Türkiye. Kuwait J. Science. 51 (1), 100077. doi: 10.1016/j.kjs.2023.07.003
Goertzen D., Schneider A.-K., Eggers T. O., Suhling F. (2022). Temporal changes of biodiversity in urban running waters – Results of a twelve-year monitoring study. Basic Appl. Ecol. 58, 74–87. doi: 10.1016/j.baae.2021.11.007
Gorzel M., Kornijów R. (2007). The response of zoobenthos to “natural channelization” of a small river. Ecohydrology Hydrobiology 7, 59–70. doi: 10.1016/s1642-3593(07)70189-1
Grimm N. B., Chapin Iii F. S., Bierwagen B., Gonzalez P., Groffman P. M., Luo Y., et al. (2013). The impacts of climate change on ecosystem structure and function. Front. Ecol. Environ. 11, 474–482. doi: 10.1890/120282
Guichard F., Padial A. A., Ceschin F., Declerck S. A. J., De Meester L., Bonecker C. C., et al. (2014). Dispersal ability determines the role of environmental, spatial and temporal drivers of metacommunity structure. PloS One 9. doi: 10.1371/journal.pone.0111227
Guillemot N., Kulbicki M., Chabanet P., Vigliola L. (2011). Functional redundancy patterns reveal non-random assembly rules in a species-rich marine assemblage. PloS One 6, e26735. doi: 10.1371/journal.pone.0026735
Hamilton N. E., Ferry M. (2018). ggtern: ternary diagrams using ggplot2. J. Stat. Software Code Snippets 87, 1–17. doi: 10.18637/jss.v087.c03
Harrison S. P., Ross S. J., Lawton J. H. (1992). Beta diversity on geographic gradients in Britain. J. Anim. Ecol. 61, 151–158. doi: 10.2307/5518
Hilary B., Chris B., North B. E., Angelica Maria A. Z., Sandra Lucia A. Z., Carlos Alberto Q. G., et al. (2021). Riparian buffer length is more influential than width on river water quality: A case study in southern Costa Rica. J. Environ. Manage 286, 112132. doi: 10.1016/j.jenvman.2021.112132
Ives A. R., Carpenter S. R. (2007). Stability and diversity of ecosystems. Science 317, 58–62. doi: 10.1126/science.1133258
Jones H. H., Barreto E., Murillo O., Robinson S. K. (2021). Turnover-driven loss of forest-dependent species changes avian species richness, functional diversity, and community composition in Andean forest fragments. Global Ecol. Conserv. 32, e01922. doi: 10.1016/j.gecco.2021.e01922
Jongman R. H. G., Braak C. J. F. T., Tongeren O. (1995). Data Analysis in Community and Landscape Ecology (Cambridge: Cambridge University Press), vii–vxi.
Konikow L. F., Kendy E. (2005). Groundwater depletion: A global problem. Hydrogeology J. 13, 317–320. doi: 10.1007/s10040-004-0411-8
Kozak J. P., Bennett M. G., Piazza B. P., Remo J. W. F. (2016). Towards dynamic flow regime management for floodplain restoration in the Atchafalaya River Basin, Louisiana. Environ. Sci. Policy 64, 118–128. doi: 10.1016/j.envsci.2016.06.020
Laliberté E., Legendre P. (2010). A distance-based framework for measuring functional diversity from multiple traits. Ecology 91, 299–305. doi: 10.1890/08-2244.1
Larsen S., Ormerod S. J. (2010). Combined effects of habitat modification on trait composition and species nestedness in river invertebrates. Biol. Conserv. 143, 2638–2646. doi: 10.1016/j.biocon.2010.07.006
Legendre P., Anderson M. J. (1999). Distance-based redundancy analysis: testing multispecies responses in multifactorial ecological experiments. Ecol. Monogr. 69, 1–24. doi: 10.1890/0012-9615(1999)069[0001:DBRATM]2.0.CO;2
Legendre P., De Cáceres M. (2013). Beta diversity as the variance of community data: dissimilarity coefficients and partitioning. Ecol. Lett. 16, 951–963. doi: 10.1111/ele.12141
Lennon J. J., Koleff P., GreenwooD J. J. D., Gaston K. J. (2002). The geographical structure of British bird distributions: diversity, spatial turnover and scale. J. Anim. Ecol. 70, 966–979. doi: 10.1046/j.0021-8790.2001.00563.x
Li Z., Chen X., Jiang X., Tonkin J. D., Xie Z., Heino J. (2021). Distance decay of benthic macroinvertebrate communities in a mountain river network: Do dispersal routes and dispersal ability matter? Sci. Total Environ. 758, 143630. doi: 10.1016/j.scitotenv.2020.143630
Li F., Chung N., Bae M. J., Kwon Y. S., Park Y. S. (2012). Relationships between stream macroinvertebrates and environmental variables at multiple spatial scales. Freshw. Biol. 57, 2107–2124. doi: 10.1111/j.1365-2427.2012.02854.x
Liermann C. R., Nilsson C., Robertson J., Ng R. Y. (2012). Implications of dam obstruction for global freshwater fish diversity. BioScience 62, 539–548. doi: 10.1525/bio.2012.62.6.5
Lin Z., Liu G., Guo K., Wang K., Wijewardene L., Wu N. (2024). Scales matter: regional environment factors affect α diversity but local factors affect β diversity of macroinvertebrates in Thousand Islands Lake catchment area. Ecol. Indic. 158. doi: 10.1016/j.ecolind.2024.111561
Liu H., Cheng W., Xiong P., Li H., Liu Z., Ai J., et al. (2023). Temporal variation of plankton and zoobenthos communities in a freshwater reservoir: Structure feature, construction mechanism, associated symbiosis and environmental response. Ecol. Indic. 154. doi: 10.1016/j.ecolind.2023.110774
Liu L., Zheng X., Wei X., Kai Z., Xu Y. (2021). Excessive application of chemical fertilizer and organophosphorus pesticides induced total phosphorus loss from planting causing surface water eutrophication. Sci. Rep. 11, 1–8. doi: 10.1038/s41598-021-02521-7
Losos J. B. (2008). Phylogenetic niche conservatism, phylogenetic signal and the relationship between phylogenetic relatedness and ecological similarity among species. Ecol. Lett. 11, 995–1003. doi: 10.1111/j.1461-0248.2008.01229.x
Manfrin A., Pilotto F., Larsen S., Tonkin J. D., Lorenz A. W., Haase P., et al. (2023). Taxonomic and functional reorganization in Central European stream macroinvertebrate communities over 25 years. Sci. Total Environ. 889, 164278. doi: 10.1016/j.scitotenv.2023.164278
Mantyka-Pringle C. S., Visconti P., Di Marco M., Martin T. G., Rondinini C., Rhodes J. R. (2015). Climate change modifies risk of global biodiversity loss due to land-cover change. Biol. Conserv. 187, 103–111. doi: 10.1016/j.biocon.2015.04.016
Mouton T. L., Leprieur F., Floury M., Stephenson F., Verburg P., Tonkin J. D. (2022). Climate and land-use driven reorganisation of structure and function in river macroinvertebrate communities. Ecography 2022 (3). doi: 10.1111/ecog.06148
Müller A. C., Guido S. (2016). Introduction to machine learning with Python: a guide for data scientists. (O’Reilly Media, Inc: Sebastopol, California).
Mummidivarapu S. K., Rehana S., Rao Y. R. S. (2023). Mapping and assessment of river water quality under varying hydro-climatic and pollution scenarios by integrating QUAL2K, GEFC, and GIS. Environ. Res. 239, 117250. doi: 10.1016/j.envres.2023.117250
Muvengwi J., Fritz H., Mbiba M., Ndagurwa H. G. T. (2022). Land use effects on phylogenetic and functional diversity of birds: Significance of urban green spaces. Landscape Urban Plann. 225. doi: 10.1016/j.landurbplan.2022.104462
Oliveira B. F., Machac A., Costa G. C., Brooks T. M., Davidson A. D., Rondinini C., et al. (2016). Species and functional diversity accumulate differently in mammals. Global Ecol. Biogeography 25, 1119–1130. doi: 10.1111/geb.12471
Opperman J. J., Fargione J., Richter B. D., Mount J. F., Galloway G. E., Secchi S. (2009). Sustainable floodplains through large-scale reconnection to rivers. Science 326, 1487–1488. doi: 10.1126/science.1178256
Paine R. T. (2006). Metacommunities. Spatial dynamics and ecological communities. Integr. Comp. Biol. 46, 347–347. doi: 10.1093/icb/icj022
Peláez O., Pavanelli C. S. (2018). Environmental heterogeneity and dispersal limitation explain different aspects of β-diversity in Neotropical fish assemblages. Freshw. Biol. 64, 497–505. doi: 10.1111/fwb.13237
Pellens R., Faith D. P., Grandcolas P. (2016). “The future of phylogenetic systematics in conservation biology: linking biodiversity and society,” in Biodiversity Conservation and Phylogenetic Systematics: Preserving our evolutionary heritage in an extinction crisis. Eds. Pellens R., Grandcolas P. (Springer International Publishing, Cham), 375–383.
Peng T., Tian H., Singh V. P., Chen M., Liu J., Ma H., et al. (2020). Quantitative assessment of drivers of sediment load reduction in the Yangtze River basin, China. J. Hydrology 580. doi: 10.1016/j.jhydrol.2019.124242
Powell J. R., Karunaratne S., Campbell C. D., Yao H., Robinson L., Singh B. K. (2015). Deterministic processes vary during community assembly for ecologically dissimilar taxa. Nat. Commun. 6, 8444. doi: 10.1038/ncomms9444
Reid A. J., Carlson A. K., Creed I. F., Eliason E. J., Gell P. A., Johnson P. T. J., et al. (2019). Emerging threats and persistent conservation challenges for freshwater biodiversity. Biol. Rev. Camb Philos. Soc. 94, 849–873. doi: 10.1111/brv.12480
Rezende R. S., Santos A. M., Henke-Oliveira C., Gonçalves J. J. F. (2014). Effects of spatial and environmental factors on benthic a macroinvertebrate community. Zoologia (Curitiba) 31, 426–434. doi: 10.1590/s1984-46702014005000001
Rosenzweig M. L. (1995). Species Diversity in Space and Time (Cambridge: Cambridge University Press).
Rousi H., Laine A. O., Peltonen H., Kangas P., Andersin A.-B., Rissanen J., et al. (2013). Long-term changes in coastal zoobenthos in the northern Baltic Sea: the role of abiotic environmental factors. ICES J. Mar. Sci. 70, 440–451. doi: 10.1093/icesjms/fss197
Rozelle S., Huang J., Zhang L. (1997). Poverty, population and environmental degradation in China. Food Policy 22, 229–251. doi: 10.1016/S0306-9192(97)00011-0
Sahraoui Y., Foltête J.-C., Clauzel C. (2017). A multi-species approach for assessing the impact of land-cover changes on landscape connectivity. Landscape Ecol. 32, 1819–1835. doi: 10.1007/s10980-017-0551-6
Schneider C., Laizé C. L. R., Acreman M. C., Flörke M. (2013). How will climate change modify river flow regimes in Europe? Hydrology Earth System Sci. 17, 325–339. doi: 10.5194/hess-17-325-2013
Segan D. B., Murray K. A., Watson J. E. M. (2016). A global assessment of current and future biodiversity vulnerability to habitat loss–climate change interactions. Global Ecol. Conserv. 5, 12–21. doi: 10.1016/j.gecco.2015.11.002
Shurin J. B., Cottenie K., Hillebrand H. (2009). Spatial autocorrelation and dispersal limitation in freshwater organisms. Oecologia 159, 151–159. doi: 10.1007/s00442-008-1174-z
Soininen J., Jamoneau A., Rosebery J., Passy S. I. (2016). Global patterns and drivers of species and trait composition in diatoms. Global Ecol. Biogeography 25, 940–950. doi: 10.1111/geb.12452
Soininen J., McDonald R., Hillebrand H. (2007). The distance decay of similarity in ecological communities. Ecography 30, 3–12. doi: 10.1111/j.0906-7590.2007.04817.x
Sørensen T. J. (1948). A method of establishing groups of equal amplitude in plant sociology based on similarity of species content and its application to analyses of the vegetation on Danish commons. Biologiske skrifter. 5, 1–34
Syvitski J., Ángel J. R., Saito Y., Overeem I., Vörösmarty C. J., Wang H., et al. (2022). Earth’s sediment cycle during the Anthropocene. Nat. Rev. Earth Environ. 3, 179–196. doi: 10.1038/s43017-021-00253-w
Thomas C. D., Cameron A., Green R. E., Bakkenes M., Beaumont L. J., Collingham Y. C., et al. (2004). Extinction risk from climate change. Nature 427, 145–148. doi: 10.1038/nature02121
Thompson R., Townsend C. (2006). A truce with neutral theory: local deterministic factors, species traits and dispersal limitation together determine patterns of diversity in stream invertebrates. J. Anim. Ecol. 75, 476–484. doi: 10.1111/j.1365-2656.2006.01068.x
Tickner D., Opperman J. J., Abell R., Acreman M., Arthington A. H., Bunn S. E., et al. (2020). Bending the curve of global freshwater biodiversity loss: an emergency recovery plan. Bioscience 70, 330–342. doi: 10.1093/biosci/biaa002
Trenberth K. E. (2011). Changes in precipitation with climate change. Climate Res. 47, 123–138. doi: 10.3354/cr00953
Ulrich W., Gotelli N. J. (2007). Null model analysis of species nestedness patterns. Ecology 88, 1824–1831. doi: 10.1890/06-1208.1
Val J., Chinarro D., Pino M. R., Navarro E. (2016). Global change impacts on river ecosystems: A high-resolution watershed study of Ebro river metabolism. Sci. Total Environ. 569-570, 774–783. doi: 10.1016/j.scitotenv.2016.06.098
Vannote R. L., Minshall G. W., Cummins K. W., Sedell J. R., Cushing C. E. (1980). The river continuum concept. Can. J. Fisheries Aquat. Sci. 37, 130–137. doi: 10.1139/f80-017
Vellend M., Srivastava D. S., Anderson K. M., Brown C. D., Jankowski J. E., Kleynhans E. J., et al. (2014). Assessing the relative importance of neutral stochasticity in ecological communities. Oikos 123, 1420–1430. doi: 10.1111/oik.01493
Viana D. S., Figuerola J., Schwenk K., Manca M., Hobæk A., Mjelde M., et al. (2016). Assembly mechanisms determining high species turnover in aquatic communities over regional and continental scales. Ecography 39, 281–288. doi: 10.1111/ecog.01231
Vieira N. K., Poff N., Carlisle D. M., Moulton S. R., Koski M. L., Kondratieff B. C. (2006). A database of lotic invertebrate traits for North America. US Geological Survey Data Series. 187, 1–15.
Vörösmarty C. J., McIntyre P. B., Gessner M. O., Dudgeon D., Prusevich A., Green P., et al. (2010). Global threats to human water security and river biodiversity. Nature 467, 555–561. doi: 10.1038/nature09440
Walker B. H. (1992). Biodiversity and ecological redundancy. Conserv. Biol. 6, 18–23. Available at: http://www.jstor.org/stable/2385847.
Wang A., He P., Long X. (2023). Spatial differentiation of fish functional groups in the Yangtze River. Biodiversity Sci. 31(10). doi: 10.17520/biods.2023095
Whittaker R. H. (1960). Vegetation of the siskiyou mountains, oregon and California. Ecol. Monogr. 30, 279–338. doi: 10.2307/1943563
Wiens J. J., Ackerly D. D., Allen A. P., Anacker B. L., Buckley L. B., Cornell H. V., et al. (2010). Niche conservatism as an emerging principle in ecology and conservation biology. Ecol. Lett. 13, 1310–1324. doi: 10.1111/j.1461-0248.2010.01515.x
Williams P. H. (1996). Mapping variations in the strength and breadth of biogeographic transition zones using species turnover. Proc. R. Soc B. 263, 579–588. doi: 10.1098/rspb.1996.0087
Yang J., Huang X. (2022). The 30 m annual land cover datasets and its dynamics in China from 1990 to 2021. Earth System Sci. Data 13, 3907–3925. doi: 10.5281/zenodo.5816591
Zhang Y., Zhang J., Wang L., Lu D., Cai D., Wang B. (2014). Influences of dispersal and local environmental factors on stream macroinvertebrate communities in Qinjiang River, Guangxi, China. Aquat. Biol. 20, 185–194. doi: 10.3354/ab00560
Keywords: biodiversity protection, metacommunity, beta diversity, turnover, nestedness
Citation: Li J, Ma M, Wang L, Jin Y, Liu Y, Yin X, Liu G and Song J (2024) Ecological drivers of taxonomic, functional, and phylogenetic beta diversity of macroinvertebrates in Wei River Basin of northwest China. Front. Ecol. Evol. 12:1410915. doi: 10.3389/fevo.2024.1410915
Received: 02 April 2024; Accepted: 30 September 2024;
Published: 17 October 2024.
Edited by:
Francois Munoz, Université Grenoble Alpes, FranceReviewed by:
Tiziana Di Lorenzo, National Research Council (CNR), ItalyJiaxin Yang, Nanjing Normal University, China
Copyright © 2024 Li, Ma, Wang, Jin, Liu, Yin, Liu and Song. This is an open-access article distributed under the terms of the Creative Commons Attribution License (CC BY). The use, distribution or reproduction in other forums is permitted, provided the original author(s) and the copyright owner(s) are credited and that the original publication in this journal is cited, in accordance with accepted academic practice. No use, distribution or reproduction is permitted which does not comply with these terms.
*Correspondence: Xuwang Yin, eWlueHV3YW5nQGRsb3UuZWR1LmNu; Jinxi Song, amlueGlzb25nQG53dS5lZHUuY24=