- 1College of Desert Control Science and Engineering, Inner Mongolia Agricultural University, Hohhot, China
- 2Forestry College, Inner Mongolia Agricultural University, Hohhot, China
- 3Institutes of Forestry Sciences of Inner Mongolia Autonomous Region, Hohhot, China
- 4Key Laboratory of State Forest Administration for Desert Ecosystem Protection and Restoration, Hohhot, China
Introduction: The Kuye River Basin, a typical erosion area of the Loess Plateau region with the second largest tributary of the Yellow River Basin, faces significant environmental challenges and intense human activities. Balancing environmental sustainability and economic development in this region is urgent.
Methods: Therefore, this study takes this basin as the object and evaluates the landscape ecological risk and human activity intensity from 2000 to 2022 based on land use/land cover, population distribution and nighttime lighting data. And a geographically weighted regression model was used to reveal the correlation between the two.
Results and discussion: Results showed that the average landscape ecological risk index in 2000, 2015, and 2022 were 9.01×10-4, 5.61×10-4, and 7.40×10-4, respectively. This shows that the overall landscape ecological risk index is low in the first 15 years and then gradually increases over time. Cultivated land, grassland and construction land are the main landscapes, the expansion of 7.95 times construction land is a key factor in the intensification of human activities, mainly concentrated in the northwest, followed by a gradual expansion towards the south-east, and likewise the landscape ecological risks follow the same trend. Medium to high intensity human activities exacerbate landscape ecological risks, with a significant positive correlation, while low intensity human activities cause little damage to landscape ecology. To achieve sustainability, it is necessary to effectively control construction land and improve land use structure. Then, in the planning work, priority will be given to the northwest region with high human activity intensity and high landscape ecological risks, and the governance direction will gradually shift to the southeast region. These findings provide empirical evidence to support decision-making and underscore the need for comprehensive strategies to mitigate landscape ecological risks and promote sustainable development in the Kuye River Basin.
1 Introduction
Throughout the course of industrial civilization, individuals have achieved significant material gratification via the use of enhanced production. (1) Nevertheless, the excessive need for primary resources has imposed a significant strain on ecological systems (Hauschild et al., 2020; Hou et al., 2022). Research has demonstrated that worldwide terrestrial environments have experienced different levels of deterioration, such as higher levels of carbon emissions, erosion of soil, decrease in biodiversity, and extinction of species. These degradations are caused by a combination of natural and human-induced factors, and this pattern is expected to worsen in the future (Rapport and Maffi, 2011; Sutton et al., 2016; Cerretelli et al., 2018). The natural factors that influence and drive the evolution of regional ecosystems over a relatively brief timeframe are typically stable and resistant to significant sudden changes. However, due to rapid socio-economic development, human activities have become increasingly intense. These changes have a substantial impact on the land surface, indirectly modifying land use patterns and patches. Simultaneously, they have profound effects on landscape patterns and ecological processes. Consequently, there has been a substantial rise in ecological risks, posing a serious threat to regional ecological security and human well-being. Ultimately, this has led to the emergence of regional landscape ecological risk (Ding et al., 2012; Han and Li, 2019; Gurbuz et al., 2020; Jun et al., 2022). Alterations in land-use and land-cover are commonly related to changes in landscape ecological risk (Gong et al., 2015; Peng et al., 2015a). Anthropogenic influences, including governmental policy, urbanisation, and socioeconomic growth, have been found to exert a substantial influence on ecological risk in several instances (Sallis et al., 2006; Xiao et al., 2021; Wu et al., 2024). Nevertheless, the impact of the human footprint on landscape ecological risk exhibited an unpredictable pattern. For instance, various phases of urbanization might have contrasting impacts on the ecological risk of landscapes (Airiken et al., 2022; Fang et al., 2022). Increased green land size alone does not guarantee a reduction in risk, however optimising the entire spatial plan has been shown to have a significant impact (Weigand et al., 2023). The complex interplay between many factors is a significant obstacle in the field of ecological management (Lynch et al., 2008). Hence, it is imperative to conduct a thorough and unbiased depiction of landscape ecological risks, human activities, and their corresponding response levels. This scientific endeavour is crucial for achieving a harmonious relationship between humans and land, while also addressing the inherent contradictions within the territorial system. Moreover, it enables the exploration of regional sustainable development (Su et al., 2021).
It is well known that landscape ecological risk is defined as the unfavorable consequences of the cyclic interaction of landscape patterns and ecological processes under the influence of human activities (Angeler and Alvarez-Cobelas, 2005). The phrase “human activities” encompasses many forms of development, use, and conservation of the natural ecological environment by individuals for the purpose of meeting their own survival and development requirements (Wei et al., 2023a; Ren et al., 2024). The tight association between regional human activity intensity and variations in landscape ecological risk can be shown by quantitative and visual characterisation (Wu, 2023). The assessment of ecological risk in landscapes, which emerged in the 1990s, centres on the quantitative analysis of the spatial variability of risk in comparison to conventional ecological risk assessment. The findings of this study serve as a crucial foundation for facilitating the development and enhancement of regional ecological networks and the sustainable management of ecological environments (Cao et al., 2019; Yan et al., 2021; Chen X. D. et al., 2022). Landscape ecological risk assessment studies have effectively demonstrated the geographical and temporal variability and scale-related impacts of ecological risk by employing multi-objective, multi-scale, multi-hierarchical systems and various interaction linkages (Yang et al., 2021). After conducting extensive research for over three decades, scholars have identified a strong correlation between landscape patterns and ecological risk. This correlation has been established using the traditional ecological risk evaluation model and the theory of landscape ecology. Additionally, the evaluation method has been integrated with geostatistics. Furthermore, the scope of the research has been expanded to encompass various levels of administrative territorial units (Tian et al., 2023), different types of natural geographic units (Feng et al., 2022), as well as watershed units and tributaries (Zhao et al., 2022). The study material encompasses a wide range of topics, including the assessment of ecological riskiness in landscape patterns, the impact of risk on size, the degree of risk, the spatial-temporal distribution pattern, the movement of centers of gravity, analysis of cold and hot spots, and exploration of driving mechanisms. In the context of our research framework, we have expanded our analysis of landscape ecological risk receptors from a single-dimensional approach to a three-dimensional framework encompassing the ecological adaptive cycle (Zhao and Zeng, 2021), ESRISK (Cao et al., 2018), DPSIR (Shao et al., 2008), PSR (Zhang et al., 2011), risk “source-sink” (Wang et al., 2018a), landscape pattern-ecological process (Hu and Wang, 2007), and reciprocal feedbacks (Chen et al., 2003), among other multidimensional landscape ecological risk evaluation methods. The comprehensive examination of landscape ecological risk assessment can enhance our comprehension of the socio-economic and natural development trajectory of a given area, particularly in evaluating the consequences of substantial human choices on the regional ecological system. The acquisition of information through these analytical processes enables us to derive insights from previous experiences, recognise deficiencies, and plays a pivotal role in facilitating efficient risk management and fostering sustainable growth. Simultaneously, upon amalgamation, synthesis, and deduction, it has been observed that while the visualisation of spatial mapping technology pertaining to land use and landscape ecological risk is progressively advancing alongside the rapid advancement of 3S technology (Zhang et al., 2023), the progress in spatial data preparation concerning human activities remains relatively sluggish. This can be attributed to the diverse characteristics of human activities and the intricate nature of human behaviour. The lack of geographical data about the magnitude of human activities has significantly limited researchers’ ability to elucidate the intricate interplay between human activities and ecological dangers. Consequently, this has led to a prolonged period of study disengagement and isolation pertaining to these two domains. Hence, previous research has predominantly considered human activities as supplementary variables of information, with little quantitative examinations of the link between landscape ecological risk and human activity (Wang et al., 2017). The thorough use of both approaches may enhance the examination of the intricate interplay between individuals and land, while also establishing a connection between human welfare and alterations in the ecological environment. This, in turn, can offer more robust theoretical backing for the formulation of regional policies aimed at protecting the ecological environment.
Kuye River Basin, situated on the right bank of the North Main Stream of the Yellow River, is the second most significant tributary within the middle section of the Yellow River known as He Long. Additionally, it is a representative river within the erosion zone of the Loess Plateau. Kuye River Basin is a region that experiences frequent rainfall and is highly susceptible to large-scale floods. It serves as a primary source of floods in the northern main stream of the Yellow River. Additionally, it plays a crucial role in transporting sediment to the Yellow River, particularly coarse sediment. The area affected by soil and water erosion accounts for over 95% of the total basin area. Over the years, the average quantity of sand transported is 111 million tonnes. The highest yearly sand transit is 335 million tonnes, with a maximum sand concentration of 1,700 kg/m3. This sand primarily flows into the Yellow River as mud. In the present context, Kuye River Basin exemplifies a region characterised by significant human activity, notable economic progress, and exceptional ecological condition. The Shenfu-Dongsheng mega-coal field, which ranks as the ninth largest coal field globally, traverses the central region of the basin. The mining area encompasses approximately 28.51% of the total basin area, resulting in significant alterations to the geomorphological characteristics due to coal mining activities. Furthermore, the process of fast urbanisation has exacerbated the tensions between the preservation of arable land, economic growth, and ecological conservation within the basin. Consequently, the subsequent environmental issues have significantly impeded and postponed the region’s sustainable development. Hence, the primary objective of this research is to investigate the relationship between regional landscape ecological risks and human activities in the Kuye River Basin. The study seeks to examine the scale response and spatial and temporal correlation between these factors. Additionally, it aims to elucidate the development of human-land relations within the basin, offering insights for optimising regional national land space and ecological security. Furthermore, the findings of this study can contribute to the prevention of regional ecological risks and the restoration of ecological systems. That is, the aim of this study is to analyse the landscape ecological risks and the intensity of human activities in the Kuye River Basin and the interrelationships. So this article focuses on the sustainable development of the social economy and ecological environment in the Kuye River Basin. It employs ArcGIS and Fragstats software to assess and visualize land use structure, landscape ecological risks, and human activity intensity, along with their interrelationships, from 2000 to 2022. Then, based on the visualization results, identify key ecological protection areas such as landscape ecological risks and high-value areas of human activity intensity, and plan routes to ensure long-term regional stability and prosperity.
2 Overview of the study area and data sources
2.1 Overview of the study area
Kuye River Basin, situated on the right bank of the North Main Stream of the Yellow River, is the second largest tributary in the middle section of the Yellow River, between the River Dragon (Figure 1). It is also a representative river in the Loess Plateau erosion zone and a significant contributor to flooding in the North Main Stream of the Yellow River. Kuye River Basin is part of the characteristic vulnerable region of the Yellow River Basin and serves as a crucial zone for soil and water conservation management (Yuan et al., 2023). Recognizing and enhancing the ecological status is essential for fostering high-quality development in the Yellow River Basin. The river has its source in Mixed Tree Village, Chaideng Township, Dongsheng District, Ordos City, Inner Mongolia Autonomous Region. It flows in a southeast direction through 6 counties (banners, cities) in Ordos City and Yulin City, Shaanxi Province. It ends at Sha’an Village, Shenmu City, where it joins the Yellow River. The main stream of the river spans a length of 242km and covers an area of 8706km2. It has two major tributaries, the Wulanmulun River and Quince Niuchuan River. The upper sections of the river are characterised by sandy steppes, while the middle and lower sections are characterised by loess hills and gullies. The overall surface vegetation in the basin is limited, with a well-developed scouring gully that exhibits a predominantly dendritic distribution. The gully density in the valley ranges from 5-9km/km2, with depths exceeding 100m. From the geographical characteristics, watershed is located in Mao Wusu sand and loess plateau hills and gullies in the transition zone, the terrain is high in the northwest and low in the southeast, with an elevation of 800~1300m, soil structure is sparse, sparse natural vegetation, coupled with the type of climate belongs to the arid and semi-arid region, by the continental monsoon, the average multi-year precipitation is 415 mm, precipitation spatial and temporal distribution of extremely uneven, June to September precipitation accounted for 75%~81% of precipitation, the maximum monthly precipitation occurs in July and August, precipitation accounts for 50%~60% of the annual precipitation. The mean precipitation over multiple years is recorded at 415mm. The spatial and temporal distribution of precipitation exhibits significant disparities, with June to September constituting 75% to 81% of the total annual precipitation. Conversely, the months of July and August experience the highest monthly precipitation, equivalent to 50% to 60% of the total annual precipitation. The soil erosion problem in the Kuye River Basin is quite significant due to the occurrence of severe cold and minimal snow in winter, limited rainfall and early frost in spring and autumn, and frequent heavy rain in summer. This issue is further exacerbated by the effect of hydraulic erosion and wind erosion.
Kuye River Basin is renowned for its intricate geomorphic composition, characterised by significant soil erosion, recurrent droughts, substantial precipitation, dust storms, and substantial deposition of coarse silt towards the yellow region. The Shenfu Dongsheng mega-coal field, which is the eighth largest in the world, is located in the middle of the watershed. The mining area covers 28.51% of the entire watershed area. As a result, the watershed has become a central location for China’s long-term development of the Jin, Shaanxi, and Mongolia energy and heavy chemical industry. Nevertheless, due to the rapid pace of urbanisation and the expansion of industrial and mining activities, there has been a significant rise in population and the implementation of predatory management practices. Consequently, these factors have resulted in notable conflicts between human populations and land, exacerbated soil erosion, a substantial decline in the provision of ecological services and values, and the severe fragmentation and degradation of ecological landscapes.
2.2 Data sources
In this thesis, in an attempt to systematically study the response of landscape ecological risks and human activities, Kuye River Basin was taken as the research object, and specific studies were carried out with the help of remote sensing image data, digital elevation model elevation data and socio-economic data. Among them, (1) Landsat TM 4-5 and Landsat 8 OLI_TIRS satellite remote sensing image data were acquired through the Geo-spatial Data Cloud Platform (http://www.gscloud.cn). (2) The land use data of the study area in 2000, 2005, 2010, 2015 and 2022 were classified using remote sensing images. Referring to the national land use classification standard (GB/T 21010-2017) (Jiao and Yang, 2008), and according to the image accuracy and the actual situation of the study area, the land use types were divided into six categories, which are cultivated land, wood land, grassland, waters, construction land, and unutilized land, with a resolution of 30m. Using ENVI of Visualizing Images 5.3.1 software, the land use structure of the Kuye River Basin from 2000 to 2022 was derived by supervised classification and visual analysis of remote sensing images. (3) Digital elevation model data with a spatial resolution of 30 m from the Geospatial Data Cloud (http://www.gscloud.cn). (4) Data characterizing the intensity of human activities include not only land-use structure data, but also population distribution data and nighttime lighting data, which are derived from the China Population Spatial Distribution Kilometer Grid Dataset and Nighttime Lighting Dataset of the Resource and Environmental Science Data Registration and Publishing System (http://www.resdc.cn/). (5) Landscape factor data were obtained by analysis in Fragstats software (https://fragstats.org/index.php/downloads).
3 Method
3.1 Land use structure
3.1.1 Land use dynamics
The concept of land use dynamics pertains to the measurable alteration of land use categories within a specific timeframe, primarily indicating the magnitude of land use modifications and variations in the pace of change across different regions. This may be categorised into two types: single land use dynamics and comprehensive land use dynamics (Xiao et al., 2022). Equation 1 calculates the single land use dynamic attitude, which measures the rate of change of a certain land use type during a given time period in the region (Ordonez et al., 2014). The comprehensive land use dynamic attitude is a method employed to analyse the overall transfer of land use types during a specific time period (Wei et al., 2023b). This approach allows for the assessment of significant regional changes in comprehensive land use. Additionally, it facilitates the comparison of comprehensive land use changes between local areas and the entire region, as well as between local areas themselves. The calculation method for this analysis is as follows.
Where: LUTnow-before is the motivation of a single land use type in the study period; LUTnow and LUTbefore are the area of a land use type at the end and the beginning of the study period, respectively; Time is the study period; LUTsynthesize is the synthesized land use motivation; LUTx is the number of land use types of type x at the beginning; △LUTx-y is the absolute value of the area of land of type x converted to unutilized land types from the beginning to the end. LUTx-y is the absolute value of the area from the beginning to the end of the study period in which type x is transformed into unutilized land use types.
3.1.2 Land-use transfer matrix
The land use transfer matrix is a tool that illustrates the dynamic process of interconversion between different land use types within a specific region during a defined time period, both at the beginning and conclusion of the period (Zhang et al., 2016). The dataset encompasses both the static data pertaining to each category within a specific region at a particular moment, as well as more comprehensive information regarding the outflow and inflow of each category at the commencement and conclusion of the study period, as determined through the following calculations.
Where: S represents the land use area; n represents the number of land use types before and after the transfer; i,j (i,j = 1,2,…n) represents the area of land use types before and after the transfer, respectively; and SQij denotes the area of land use type i before the transfer that is converted to land use type j after the transfer.
3.2 Landscape ecological risk and human activity intensity calculation
3.2.1 Risk sample plot division
The ArcToolbox-Data Management Tools-Sampling-Create Fishnet method of operation in the ArcGIS computer mapping application programme was employed to compute the two indicators of landscape ecological risk and human activity intensity. The study area was partitioned into 417 square grids, each with a side length of 5km, based on the size of the evaluation units and the area of the study area. These evaluation units were utilized to calculate the landscape ecological risk index and the human activity intensity index, with the respective values located at the centre of each independent unit.
3.2.2 Calculation of landscape ecological risk index based on landscape pattern index
The Landscape Ecological Risk Index (LERI) is employed as a metric to assess the ecological risk resulting from the land use patterns within a given region. This index serves as a significant indication for establishing a connection between ecological risk and human activities. The index calculation incorporates explanatory variables that encompass both intrinsic and extrinsic factors that influence the ecological risk of landscapes. Extrinsic factors pertain to the level of disruption experienced by the ecosystem during periods of stress, as indicated by the degree of landscape disturbance. On the other hand, intrinsic factors pertain to the susceptibility of ecosystems during periods of pressure, as indicated by the degree of landscape vulnerability. Internal factors encompass the extent to which ecological systems are susceptible to stress, and are distinguished by the level of landscape vulnerability. The paper utilises Fragstats software to analyse the current conditions of the study area and existing research (Liao et al., 2022). It focuses on constructing a landscape ecological risk evaluation model for the Kuye River Basin, utilizing the landscape disturbance index and landscape vulnerability index. The model is calculated using the following formula:
The disturbance index of the i-th landscape type is denoted as Di. The weights of each landscape index are represented by a, b, and c, respectively. The sum of a, b, and c is equal to 1. This work utilises the findings of other studies, including Yufang (Yu et al., 2022), and incorporates the current conditions of the study region. The weight assigned to variable an is 0.5, the weight assigned to variable b is 0.3, and the weight assigned to variable an is 0.2. Landscape fragmentation is denoted by the symbol Fi. Landscape separation is denoted as Si, whereas landscape fractal dimension is denoted as FDi. In the context of landscape type i, Ni represents the quantity of patches, Ai represents the area, A represents the overall area (measured in hm2), and Pi represents the perimeter (measured in m) of landscape type i. The variable Ri represents the extent of landscape loss in landscape type i, while Vi represents the landscape fragility index of landscape type i. The landscape fragility index is assigned to various landscape types based on previous research findings (Guo et al., 2023). Specifically, unutilized land, waters, cultivated land, grassland, wood land, and construction land are assigned values of 6, 5, 4, 3, 2, and 1, respectively. The fragility index for each landscape type is then calculated after normalisation. The normalisation process was employed to produce the vulnerability index for each landscape type. The ecological risk index (ERI) represents the ecological risk of the i-th risk plot. Aki represents the area of the i-th kind of landscape in the k-th risk plot, whereas Ak represents the size of the k-th risk plot.
3.2.3 Analysis of spatiotemporal change in landscape ecological risk
The examination of spatiotemporal variations in the ecological risk value of the landscape is carried out through a three-step process. Ecological risk levels are categorised based on the presence of natural breaks. Natural breaks are well-suited for illustrating inherent patterns in the data, as they decrease the variability within each class and maximise the variability between different classes (Liang et al., 2018; Lei et al., 2020). All units from the years 2000, 2005, 2010, 2015, and 2022 were aggregated and categorised into ten periods based on the landscape ecological risk levels. Five risk levels were obtained by combining every two neighbouring periods.
Additionally, the construction of the rate of risk change index aims to assess the disparity in the rate of growth in landscape ecological risk values across various units throughout different time periods. This analysis helps to detect the geographical and temporal variations in risk changes. The index of risk change rate refers to the mean yearly increment in the ecological risk value of a given unit, expressed as a percentage relative to the original value of ecological risk. Greater, positive index values signify accelerated rates of risk expansion, lesser values signify decelerated risk expansion, and negative values signify diminished risk. The computation of the rate of risk change index is as follows:
RRCi is the rate of risk change of unit i; LERV is the landscape ecological risk value; and are the landscape ecological risk index of unit k at time t1 and t2, respectively; and Δt is the time span from t1 to t2.
3.2.4 Calculation of the intensity of human activity
The assessment of human activities’ intensity serves as a reliable measure for evaluating the extent of human impact on the natural environment (Wang et al., 2009). The current approaches to quantifying the intensity of human activities may be categorised into two distinct spatial characterisation methods: direct spatialization and indirect spatialization. ground use change is a phenomenon that encompasses a wide range of human economic and social activities. It may be seen as the observable outcomes of human actions on the ground surface, serving as an effective means to measure the extent of human activity. Nevertheless, the approach of assessing the magnitude of human activities exclusively through land-use change poses challenges in accurately representing the diverse, intricate, and interconnected nature of human activities. Additionally, this method may introduce a certain level of endogenous influence when compared to landscape ecological risk evaluation, which also relies on land-use data. This study presents spatial distribution data of nighttime lighting and population, building upon previous research. It draws upon the findings of Rong Yi et al. (Rong et al., 2017) and combines three methods: Lohani list method, the Leopold matrix method, and the Delphi method. By averaging the coefficients, the study reduces coefficient error and assigns different coefficients to measure the intensity of impacts from various human activities on different land-use types. Finally, distinct coefficients were allocated to various land use categories in order to develop a complete evaluation model of human activity intensity in the Yangtze River Delta region. The formulas for these coefficients are as follows. Furthermore, the Jenks optimal natural fracture method was employed to classify human activity intensity into five distinct levels, namely low intensity, lower intensity, medium intensity, higher intensity, and high intensity, in order to visually represent the spatial and temporal evolution characteristics of human activity intensity from 2000 to 2022. This classification was based on the findings of previous research (Wang et al., 2021).
Where: HAI denotes the intensity of human activities; n, p, l are the index weights of the normalized nighttime lighting index NTL, population distribution data PD and land use data LU, respectively. We refer to the research results of Chen Hongjin et al. (Chen H. J. et al., 2022), and n, p and l are taken as 0.3, 0.3 and 0.4, respectively.
3.3 Spatial and temporal correlations between landscape ecological risk and human activity intensity
This research integrates an overview of the study area in the Kuye River Basin with geographical Statistics Tools to examine the geographical and temporal relationship between landscape ecological risk and the intensity of human activities in the region from 2000 to 2022.The Geographic Distribution-Center of Mean and Directional Distribution module of ArcGIS programme is used for measuring the geographic distribution. It is a tool for doing spatial statistics.The unique problem was analysed using the Geographically Weighted Regression Model of ArcGIS software, which is a spatial relationship modelling technique.
3.3.1 Geographic distribution of metrics
The Mean Center of Geographic Distribution module of the Spatial Statistics function of ArcGIS is used to identify the geographic center of a group of elements. The specific calculation formula is as follows:
The mean center can be expressed as:
Where: xi and yi are the coordinates of element i and n is equal to the total number of elements. The weighted average center expansion is:
Where: wi is the weight at element i. The tool also calculates the center of the third position if there is a Z attribute for each element:
The Directional Distribution module, located within the Spatial Statistics Functions of ArcGIS’s Metric Geographic Distribution, is utilized to provide a concise summary of the spatial attributes of geographic elements. This includes measures such as central tendency, dispersion, and directional tendency. By examining the distribution of these elements, we can determine if they are narrow and directional, enabling us to intuitively perceive the data’s tendency. The determination of the directional distribution, also known as the standard deviation ellipse, involves the computation of the standard deviation of the x- and y-coordinates relative to the mean’s centre. This calculation establishes the axis of the ellipse, thereby earning it the designation of a standard deviation ellipse.
3.3.2 Geographically weighted regression models
Geographically weighted regression is a variant of the conventional linear regression model (Kang et al., 2014). It incorporates the spatial location of the data into the equation for computation. This regression approach considers the specific attributes of the spatial variables and can efficiently adjust the weights at the local level. Each sample point is assigned an independent coefficient value, enhancing the accuracy of the obtained results in reflecting the real-world context. The formula for calculation is as follows:
Where: β0 (ui,vi) is the coordinates of the sampling point, βk (ui,vi) is the k-th regression coefficient on sampling point i, is the geographic location correlation function, which is obtained by using the weighting function in the estimation process, p is the number of explanatory variables, Xik is the value of explanatory variable Xk on point i, and ϵi is the random disturbance term.
4 Results
4.1 Land use structure
The analysis of the land use area and changes in the Kuye River Basin from 2000 to 2022, based on the interpretation of the five-phase remote sensing map, revealed that the primary land use types in the basin are cultivated land, grassland, and construction land. These land use types collectively account for an average of 85.63% of the total land use area over multiple years.
As shown in Figure 2, the area of wood and grassland accounted for the most, and the combined area of the both from 2000 to 2022 was 602576.5234hm2, 612346.2168hm2, 620415.5156hm2, 602723.5285hm2, and 576075.5507hm2, which accounted for 69.15%, 70.27%, 70.27%, 70.27%, 71.20%, 69.17%, and 66.12% of the total land use type, whose area and share were more than half of the total land use type, 71.20%, 69.17%, and 66.12%, respectively, with their areas and percentages reaching more than half of the total land use types. The land-use landscape pattern in the Kuye River Basin is spatially spread, including the majority of the basin, including its northern, central, and southern portions. However, it is more densely inhabited in the northwestern and western half of the central part of the basin, resulting in a distinct pattern. The cultivated land areas from 2000 to 2022, as seen in Figure 2, were as follows: 174236.2982hm2, 158599.294hm2, 151845.0701hm2, 149024.3916hm2, and 146599.1395hm2. These areas accounted for proportions of 20.00%, 18.20%, 17.43%, 17.10%, and 16.83% correspondingly. The spatial distribution of cultivated land in the watershed, as depicted in Figure 3, exhibits a notable degree of uniformity. Specifically, the southern, central, and northern districts of the watershed are predominantly occupied by cultivated land dedicated to grain cultivation. This agricultural activity serves as the primary source of sustenance for the social populations residing in Shenmu City, Fugu County, Yulin City, Shaanxi Province, Dongsheng District, Kangbash District, Dalate Banner, YijinhuoLuo Banner, Ordos City, Inner Mongolia Autonomous Region, and other regions within the Kuye River watershed area. Although the proportion of construction land is comparatively lower than that of grassland, there is a consistent upward trend. Specifically, the area of construction land in 2022 is 7.95 times greater than the area of construction land in 2000. This trend is indicative of the economic and social progress of urban areas, as depicted in Figure 2. Simultaneously, the Kuye River Basin exemplifies a region characterised by significant human activity, notable economic progress, and exceptional ecological condition. The Shenfu-Dongsheng mega-coal field, which ranks as the ninth largest coal field globally, traverses the central region of the basin. The mining area encompasses 28.51% of the entire basin area, resulting in significant alterations to the geomorphological characteristics due to coal mining activities. The extent of unutilized land areas has exhibited varying degrees of change between 2000 and 2022, with a general rising trajectory. These areas are mostly concentrated in the northern, northwest, and other regions, as seen in Figures 2, 3. Waters, being a significant resource for social production and ecological environment, are experiencing a gradual decline in both distribution range and area. This observation is supported by the analysis of five-phase remote sensing images, which reveal the following values: 25783.58hm2, 23336.7553hm2, 23072.5271hm2, 21206.1525hm2, and 21737.2323hm2 (Figures 2, 3). Additionally, it is evident that the water resources of the primary tributaries within the basin, namely the Wulanmulun River and Quince Niuchuan River, together with the remaining 19 tributaries, have seen varied levels of impact.
4.2 Land-use dynamics and transfers
According to the data presented in Figure 4, the land use patterns observed in the Kuye River Basin exhibit a consistent pattern characterised by three rises and three declines. Specifically, there is an upward trend in the extent of wood land, unutilized land, and construction land, whereas there is a downward trend in the areas of arable land, grassland, and waters. The period from 2000 to 2010 witnessed a significant prevalence of grassland, cultivated land, and wood land, accounting for a combined proportion of 89.15% in 2000, 88.48% in 2005, and 88.63% in 2010. From 2010 to 2022, there was a substantial alteration in the land cover composition of the watershed, characterised by a decrease in cultivated land, grassland, wood land, and waters. Among these, the area of cultivated land decreased by 0.29% from 151845.0701hm2 to 146599.1395hm2. The area of wood land decreased by 0.37% from 49,842.0514hm2 to 47,616.5412hm2. The area of grassland decreased by 0.62% from 570573.4642hm2 to 528459.0095hm2. Lastly, the area of waters decreased by 0.48% from 23072.5271hm2 to 21737.2323hm2. On the other hand, the amount of land that was not being used grew by 1.63% from 48,588.7927hm2 in 2010 to 58,120.255hm2 in 2022. Additionally, the amount of land used for building climbed by 12.55% from 27,444.8848hm2 in 2010 to 68,782.2425hm2 in 2022.
The phenomenon of land use transfers was marked by a notable expansion in the extent of developed land and unutilized land, accompanied by different degrees of reduction in unutilized land. Wood land and grassland constituted the majority of the transferred construction land, representing 5.17% and 28.41% of the total increase in construction land area, respectively. The extension of construction land within the watershed is closely linked to the notable impacts of escalating human activities, particularly urbanisation and rapid population rise. These factors contribute to an increased demand for construction land. Furthermore, the extensive extraction of coal in the Shendong and Shenfu coal fields within the Kuye River Basin, encompassing the establishment of production and residential areas for coal miners, transportation infrastructure, and supporting services, will result in a substantial expansion of construction land. Water, being a crucial prerequisite for the sustenance and advancement of human civilization, holds significant importance as a dynamic element within the natural and social milieu. It serves as an indispensable foundational natural resource that is indispensable for upholding the integrity of the Earth’s ecological system and facilitating the progress of the economic framework. However, over the span of 2000 to 2022, there has been a notable reduction of 4,046.3477 cubic meters in water availability. This decline can be attributed to various natural and socio-economic factors, including the extensive utilization of irrigation water for cultivating arable land, irrational water consumption for domestic purposes, and the water demand associated with the cultivation of wood and grassland. The reasons of water scarcity may be attributed to both environmental and socio-economic factors. These factors include the excessive use of irrigation water for cultivating arable land, the illogical consumption of water for home purposes, and the water demands associated with the expansion of wood land and grassland.
4.3 Landscape pattern index and change characteristics
Aiming to analyze the landscape pattern index and change characteristics of the Kuye River Basin, we exported the land use remote sensing data in raster format to Fragstats software by using ArcGIS to calculate the number of patches and patch area of each land type in this paper. Afterwards, Equations 4–8 were combined to calculate landscape separation, landscape disturbance, landscape fragmentation and landscape loss to characterize the overall landscape pattern. According to the data presented in Figure 5, there was a consistent annual increase in the number of patches from 2000 to 2022. Additionally, the density of patches exhibited a pattern of initial decrease followed by subsequent increase. Specifically, the number of patches rose from 4713 in 2000 to 6522 in 2022. Conversely, the density of patches across all land types experienced a consistent annual decrease from 2000 to 2015, dropping from 0.0945 to 0.0771. With a further increase to 0.0788 in 2022, it can be deduced that landscape fragmentation is on the rise. This indicates that different types of landscapes are becoming more scattered after 2015 due to human disturbance and the spread of urban building in the watershed.
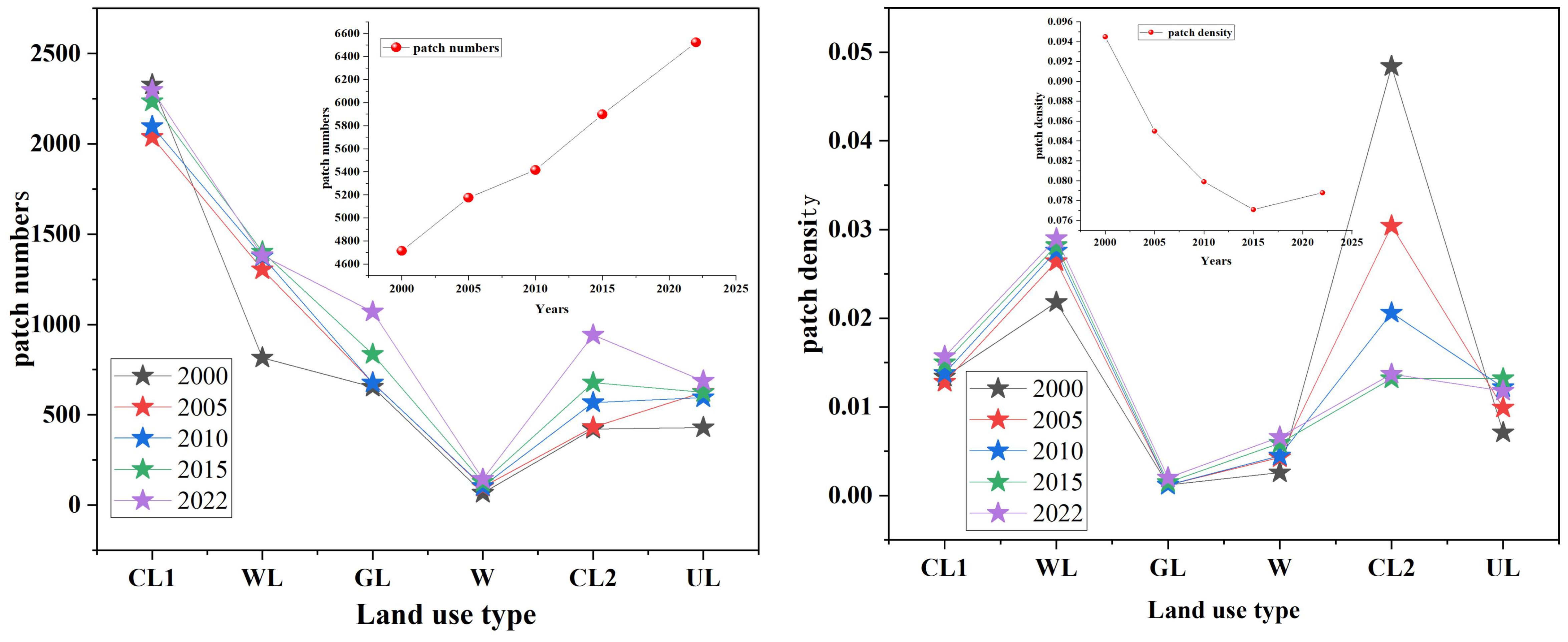
Figure 5. Number of landscape patches and density of patches in different years in the Kuye River Basin.
By analyzing Figure 6, we observed that the separation index exhibits a consistent pattern of decreasing and then increasing in response to changes in patch density. Notably, in 2022, the maximum value of 1.2252 indicates the highest level of landscape separation and fragmentation caused by human interference. The extent of landscape degradation, which indicates the extent of loss of ecological characteristics resulting from both natural and human-induced disturbances (Xu et al., 2016), exhibited a consistent upward trend from 2000 to 2022. This observation suggests that the degradation of ecological attributes in the Kuye River Basin is becoming more pronounced and severe, as evidenced by the respective degree of loss indices of 0.2391, 0.2588, 0.2750, 0.2827, and 0.2904. In summary, based on an analysis of the landscape pattern index, it can be observed that the watershed has experienced a progressive rise in anthropogenic disturbances over time. Consequently, the landscape pattern has become more intricate and fractured, exhibiting heightened levels of fragmentation.
Regarding each land use type (Figure 7), the Kuye River Basin experienced a decline in the area of cultivated land and grassland patches compared to an increase in the area of construction land patches between 2000 and 2022. Specifically, the area of cultivated land and grassland decreased by 27,696.96hm2 and 36,706.14hm2, respectively. The size of construction land patches has progressively grown, with a notable rise in the number of patches between 2010-2022 compared to 2000-2010. This growth may be attributed mostly to the rapid urbanisation of the Kuye River Basin in the last 12 years. The patch density and separateness indices for construction land have experienced a decrease from 0.0484 and 1.1016 in 2000 to 0.0137 and 0.2374 in 2022. However, it is worth noting that the degree of decrease in patch density and separateness indices between 2000 and 2010, which were 0.0278 and 0.6973 respectively, is significantly greater than the values observed between 2010 and 2022, which were 0.0069 and 0.1669. Specifically, following 2010, there was an increase in the extent of construction land, but it became subject to control within the watershed. This led to an increase in agglomeration, a decrease in segregation, and a shift in the development of construction land from dispersed to centralized. However, it is important to note that the level of human disturbance and fragmentation was intensifying, albeit at a reduced rate of increase. Water patches formed by the main tributaries Wulanmulun River and Quince Niuchuan River with 19 other tributaries decreased by 4041.81hm2, while the separation index of the watershed and the landscape loss index also decreased by 0.099 and 0.0254. In summary, the changes in the landscape pattern index for each land use change similarly show that the area of construction land is increasing year by year, and the landscape pattern tends to become more complex and fragmented, with increased fragmentation.
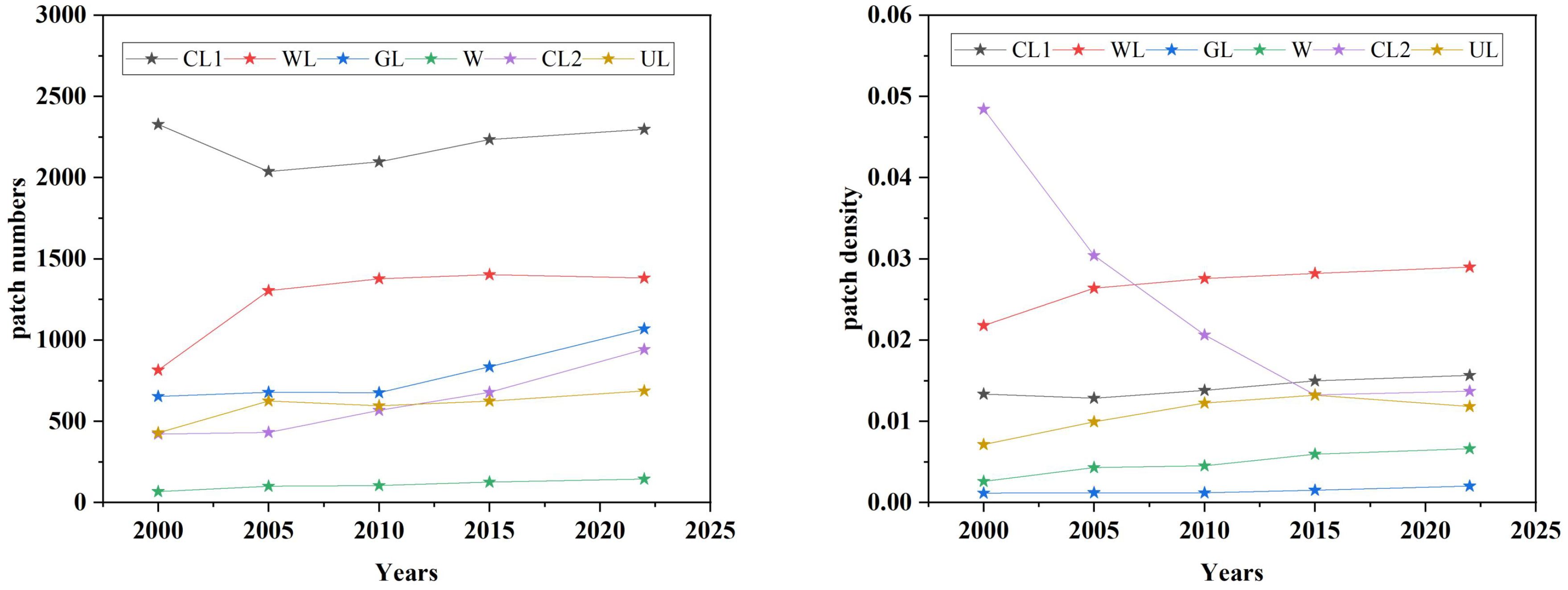
Figure 7. Number of landscape patches and patch density in the Kuye River Basin based on each land type.
4.4 Landscape ecological risks
The landscape ecological risk is often categorized into five categories, namely the low level, lower level, medium level, high level, and higher level. This categorization serves to illustrate the varying degrees of risk associated with different methods and management measures. ArcGIS utilizes the Jenks techniques of natural breaks classification to automatically categorize the landscape ecological risk of the Kuye River Basin into five tiers based on the value of landscape ecological risk. The study identified five distinct categories of landscape ecological risk, namely the low risk level, lower risk level, medium risk level, higher risk level, and greatest risk level. The geographical distribution of multi-cores was observed in the landscape ecological risk levels of the Kuye River Basin. Utilized to provide a cartographic representation of the spatial arrangement of landscape ecological vulnerability in the designated research region spanning from 2000 to 2022, illustrating the alterations in the vulnerable area (Figure 8).
The Kuye River Basin had average landscape ecological risk levels of 9.01×10-4, 7.43×10-4, 7.24×10-4, 5.61×10-4, and 7.40×10-4 in the years 2000, 2005, 2010, 2015, and 2022, respectively. The results of this study indicate a consistent decline in ecological risk within the Kuye River Basin between the years 2000 and 2022, followed by a subsequent stabilisation at a comparatively low level. The collective proportion of the two phenomena at the five specific time intervals was 93.05%, 96.76%, 96.26%, 98.23%, and 93.52%, respectively. This corresponds to 93% to 98% of the total area affected by ecological risk in the entire watershed. Nevertheless, there was an observed rise in the level of landscape ecological risk subsequent to 2015, accompanied by a commensurate decline in the number of places characterized by lower and low landscape ecological risk. During the period from 2000 to 2015, the areas characterized by elevated landscape ecological risk were measured to be 6705.417hm2, 1614.559hm2, 2258.859hm2, and 1433.923hm2. Conversely, areas with high landscape ecological risk were recorded as 517.7411hm2, 509.7809hm2, 541.2127hm2, and 450.6185hm2. The time span from 2015 to 2022 witnessed a notable shift, as the proportion of both entities rose to 0.88% and the total area expanded from 7223.1581 hm2 in 2000 to 7654.494 hm2 in 2022. When doing a comparative analysis of the Risk Reduction Coefficient (RRC) for the Kuye River Basin over several time periods, it is seen that the total risk decreased at a faster rate during periods I (2000-2005) and IV (2015-2022). Conversely, the fall in overall risk was somewhat slower during period II (2005-2010), with RRC values of -3.52%, -4.83%, and -0.50%, respectively. During period III (2010-2015), the risk experienced a consistent annual rise of 0.44%.
From a geographical perspective, it can be observed that areas with high ecological risk are predominantly situated in the northern and southern portions of the basin. Conversely, regions with reduced ecological risk and low ecological risk are distributed over the majority of the basin, encompassing the eastern, western, southern, and northern areas. Geographically, these places with elevated ecological risk exhibit significant variations in height, latitudinal and vertical zonation, as well as substantial expansions of developed land (Figures 1, 3). The combined impacts of the increased construction land area of 60,133.3598hm2 and human intervention between 2000 and 2022 have resulted in the dispersion, finer, and fragmented distribution of landscape types in these regions. This has given rise to a distinct vertical band spectrum, characterized by fragmented landscape patches. Consequently, the ecological integrity and stability of the environment have been compromised, thereby increasing ecological risks. The landscape type in the Kuye River Basin is primarily characterized by wood land and grassland, which are distributed in the east-west and north-south regions of the low ecological risk area. The primary objective of this landscape type is to preserve a high level of landscape integrity and ensure the stability of wood land and grassland ecosystems. Additionally, it exhibits a strong resistance to external interference and poses a low level of ecological risk. Conversely, the region characterized by moderate ecological risk is mostly concentrated in the vicinity of the watershed.
4.5 Intensity of human activity
The level of human activities in the research region was classified into five classes: low intensity, lower intensity, medium intensity, higher intensity, and high intensity, in accordance with the spatially measurable operational approach to landscape ecological risk. The data shown in Figure 9 illustrates a notable rise in the level of human activities within the designated research region between the years 2000 and 2022. Notably, the period from 2015 to 2022 exhibited the most substantial growth, with a recorded rate of 12.07%.
The study area exhibits high-intensity human activities that align with the characteristics of high landscape ecological risk. The distribution area demonstrates a linear upward trend, with the growth area primarily consisting of construction land and the more densely populated northern district. Simultaneously, there is a gradual expansion towards the south. The relevant area, initially measuring 2613.5hm2, experienced an increase to 6039.2869hm2 in 2005. Subsequently, it further rose to 19519.5174hm2 in 2010 and 27507.1999hm2 in 2015. Finally, it experienced a rapid increase to 76,472.9192hm2 in 2022. During the time frame spanning from 2000 to 2010, the level of human activities had a relatively low intensity, mostly characterised by lower and low intensity activities, collectively accounting for 67.37% of the total. From 2010 forward, particularly starting from 2015, there was a prevalence of medium and greater intensities. The area of medium-intensity human activities was 485077.0675hm2 in 2015 and increased to 489734.0619hm2 in 2022. The area of higher-intensity human activities increased to 257428.3342hm2 and further increased to 187772.8694hm2. By 2022, the intensity scale of this phenomenon has reached a significant level, representing 8.83% of the total human activities within the watershed between 2000 and 2022. This is a substantial growth of 73,859.4192 hm2 in area, as opposed to the 2,613.5 hm2 recorded in 2000.
With the exception of the northern region, where human activities are concentrated, the landscape type primarily consists of wood and grassland. The absence of deforestation and land reclamation can be attributed to the implementation of integrated watershed management and ecological protection policies. Consequently, the surrounding areas experience minimal human activities, resulting in the preservation of their ecological systems. By 2022, the combined area of lower and low-intensity human activities is projected to reach 47,259.4238hm2 and 64,387.9941hm2, representing 5.46% and 7.44% respectively.
4.6 Correlation analysis between human activity intensity and landscape ecological risk
The spatial statistics tool module of ArcGIS software was employed to investigate the spatial influence link between landscape ecological risk and human activity intensity in the research region using the geographically weighted regression model. The research employed regression analysis to examine the relationship between landscape ecological risk and human activity intensity index. The dependent variable was landscape ecological risk, while the explanatory variable was human activity intensity index. The distance weight decay function was chosen as the Gaussian function kernel, and a geographically weighted regression model was utilized to assess the extent of human activity intensity’s influence. According to the data presented in Figure 10, it can be observed that the standardized residuals for most regions within the Kuye River Basin fall within the range of [-2.5,2.5]. It is important to note that if the absolute value of the standardized residuals exceeds 2.5, the reliability of the regression forecast for the respective area is compromised. This observation suggests that the model is appropriately fitted.
Regression coefficient spatial distribution map can reflect, spatially landscape ecological risk and human activity intensity of the influence of the relationship, when the regression coefficient is positive indicates that the landscape ecological risk and human activity intensity is positively proportional to each other, and vice versa. From the figure, it can be seen that the regression coefficient is positive in the northern part of the basin, especially in the northwestern region, part of the central part and the southeastern part, and the surrounding region, and the value of the regression coefficient is increasing after 22 years of development, especially from 2010 (Figure 11), and the values of the regression coefficient for the five time points of the period of 2000-2022 are in the ranges of [0.0688,0.4764], [0.0886, 0.5047], [0.0731,1.2096], [0.0445,0.6803], [0.1031,0.7343]. The landscape type primarily consists of construction land, and the ecological risk of the region is higher. This is indicated by a positive regression coefficient with a larger value, suggesting that the region is characterized by medium and high intensity human activities. This implies that as human activities intensify in the region, the ecological risk of the landscape also increases.
The mean centre of human activities was determined to be located in the middle north of the watershed using the Spatial Statistics Tools - Metrics Geographic Distribution - Mean Centre module of ArcGIS software. The xy coordinates of the mean centre of human activities at the start of the study, during the five time nodes from 2000-2022, were (446611.5473, 4228106.8413). At the conclusion of the study, the xy coordinates were (446371.7634, 4230844.2566). The central point of the mean landscape ecological risk was found to be situated in the central northern region of the watershed. According to Figure 12 of the distribution map of landscape ecological risk movement direction in ArcGIS, it can be observed that the average centre of landscape ecological risk is exhibiting a northward shift.
The findings from the Spatial Statistics Tools-Metrics Geographic Distribution-Directional Distribution (Standard Deviation Ellipse) module of ArcGIS software indicate that human activities are primarily concentrated in the northwestern region of the study area, as evidenced by the expansion of the large-scale construction land area (Figure 13). The escalation of human activities and the swift growth of other industrial enterprises have resulted in a rise in the level of human activities in the region, which subsequently expanded towards the southeast. This indicates a pattern of human activities moving from the northwest to the southeast. Similarly, the distribution of ecological risks in the landscape aligns with the direction of human activity. Consequently, this has caused a certain level of disturbance to the overall integrity, interconnectedness, and density of patches in the landscape, eventually increasing the ecological vulnerability of the environment. In contrast, the landscape type primarily consists of wood land and grassland, resulting in a relatively low human population density and low intensity of human activities. Consequently, the ecological risk associated with this landscape is also low. This can be attributed to the high altitude of the region, sparse population, and the absence of concentrated residential, industrial, mining, and other construction land in the distribution area. The coverage of wood land and grassland is high, and with the advancement of socio-economic development, there is a certain degree of expansion in the area of construction land, population growth, and the establishment of other industrial enterprises. However, these factors are unlikely to significantly impact the ecological risk of the landscape. The expansion of construction land area, population increase, and other industrial businesses, in conjunction with socioeconomic development, is unlikely to have a detrimental impact on the ecological risk of the landscape. Negative return coefficients range from [-0.4965,-0.1516], [-0.4905,-0.16660], [-0.8410,-0.0806], [-0.6872,-0.6251], and [-0.4957,-0.0155] during the period 2000-2022, respectively.
5 Discussions and conclusions
5.1 Discussion
In recent years, there has been a shift in the focus of ecological risk assessment from primarily examining the effects of pollution on ecosystems to considering more significant factors related to human activities such as land use, urbanization, and demographic changes. In the 2000s, humans continued to seek economic advantages while progressively becoming aware of the importance of environmental conservation, resulting in a partial improvement in ecological quality (Wang and Sun, 2016). During the 2010s, the establishment of ecological civilization was implemented and yielded significant results, leading to a substantial reduction in ecological risk (Zhang et al., 2021; Ran et al., 2022). Additionally, the scope of research has expanded from a single population to encompass landscape and watershed scales (Fu and Lu, 2006; Peng et al., 2015b). The linkage between regional development and the ecological environment is established through landscape ecological risk assessment. This assessment examines the changes in the regional ecological environment due to watershed development and offers a quantitative foundation for decision-making in ecological and environmental management within the study area. Moreover, it serves as a scientific reference for the coordinated development of the region’s society, economy, and ecology (Jin et al., 2021; Ma and Liu, 2021). The objective of this study is to examine the ecological risk posed by human activities in the Kuye River Basin. Specifically, the focus is on the Yellow River, which erodes soil and covers over 95% of the basin’s total area. The Yellow River plays a crucial role in transporting sediments, particularly coarse sediments, and serves as a key channel for the construction of China’s “Jin-Shaan-Mengzhou Energy and Heavy Chemical Industry Base.” The study aims to promote the harmonious development of human activities and the ecological environment within the context of China’s rapid socio-economic development and comprehensive land use, including industrial, mining, and other industries. Simultaneously, as the focal point of the establishment of the “Jin, Shan, and Meng Energy and Heavy Chemical Base” in China in the forthcoming century, the expeditious advancement of the social economy, encompassing the mining industry, coupled with the optimal utilization of land resources, will facilitate the symbiotic progress of human endeavours and the ecological milieu.
Simultaneously, as the focal point of the establishment of the “Jin, Shan, and Meng Energy and Heavy Chemical Base” in China in the forthcoming century, the expeditious advancement of the social economy, encompassing the mining industry, coupled with the optimal utilization of land resources, will facilitate the symbiotic progress of human endeavours and the ecological milieu. This tendency can be linked to the dominance of wood land, grassland, and cultivated land types in the watershed, which have a significant influence on landscape patterns. The majority of the Kuye River is covered by grassland, encompassing the northern, central, and southern regions of the watershed. However, the western half of the northwestern and central areas exhibit a higher density of grassland, resulting in a distinctive land use landscape pattern within the watershed. Furthermore, the basin contains cultivated land in the southern, central, and northern districts. These districts are densely distributed and serve as the primary food source for various social groups in Shenmu City (a county-level city) and Fugu County of Yulin City in Shaanxi Province, as well as Dongsheng District, Kangbash District, Dalate Banner, and Yijinhuo Luo Banner of Ordos City in the Inner Mongolia Autonomous Region. These districts are part of the Kuye River Basin. The primary factor behind this is the introduction and execution of the Kuye River Basin Comprehensive Plan. This plan emphasises the integration of prevention and treatment, prioritising protection, highlighting key aspects, and enhancing basin management. It also emphasises the organic combination of wood land and grassland, engineering, and farming measures on a watershed scale. This approach aims to enhance environmental quality, vegetation cover, and land-use structure. Following the introduction and implementation of various programmes and engineering measures, such as the “National Ecological Environment Protection Outline,” “Yellow River Ecological Protection and Governance Battle Action Programme,” and “Inner Mongolia Yellow River Soil and Water Conservation Ecological Function Key Tributary of the Kuye River Basin Ordos Project Area Governance Project,” the Kuye River Basin wood land and grassland area have consistently improved. These types of land serve multiple ecological purposes (O’Connor and Kuyler, 2009), including water conservation (Zhang et al., 2022), soil preservation (Zhang et al., 2024), and species protection (Kivinen et al., 2022).
However, following 2015, the fast rise of population and the coal industry have resulted in an expansion of its territory and share. The upward trend in construction land suggests that the expansion of construction land in the region has been influenced by the growing human activities, particularly due to urbanization and rapid population growth in the watershed. This has resulted in an increased demand for construction land. The expansion of coal mining operations in Shenfu and other coal fields in the Kuye River Basin has led to a substantial growth in the construction land area due to the construction of production and living facilities. These facilities include residential land for coal miners, transportation land, and other services. Consequently, the escalation in human activities led to a decline in the rationality of landscape structure, with an augmentation of landscape fragmentation and an elevation in the risk value associated with landscape patterns. The findings of Ma Yingyi et al. (Ma and Liu, 2021) align with this observation. In the 21st century, significant phases of industrialization, urbanization, and infrastructural development have transpired, resulting in a sustained high demand for land development (Wang et al., 2018b). The study found that industrialization, urbanization, and infrastructural development has contributed to an increase in landscape ecological risk and human activity intensity in various regions (Cheng et al., 2023). Based on the findings of this study regarding the spatial and temporal relationship between landscape ecological risk and human activity intensity, it is observed that both landscape ecological risk and human activity exhibit a gradual expansion from the northwest to the southeast. This expansion is primarily observed in the northwestern region of the Kuye River Basin, as well as in certain parts of the central region and the southeast region. Additionally, the surrounding areas with a high intensity of human activities also demonstrate a positive regression coefficient. Consequently, areas characterized by intensive human activity and high activity levels exhibit a positive correlation with landscape ecological risk. The human activities in this region are extensive and substantial, and they have a direct correlation with the ecological risk of the landscape. In other words, high-intensity human activities elevate the degree of ecological risk in the landscape. Urbanisation has led to the dispersal of housing construction land and coal mining industry land, as well as an increase in hydropower projects (Liu et al., 2023). As a result, construction land has become a significant and abundant source of interference. This has led to the generation of a large amount of waste soil and waste residue, which contribute to soil erosion. This has caused extensive and profound soil erosion, as well as an increase in the ecological risk value of the landscape. However, the regulation of landscape ecological risk is relatively slow in addressing these issues. Hence, the aforementioned region has significant importance in the context of ecological restoration and management within the Kuye River Basin. In forthcoming planning endeavours, it is imperative to prioritize the regulation of regions characterized by high levels of human activity and elevated landscape ecological risks. The ecological management and building efforts will primarily target the northwestern section of the watershed, with a progressive shift towards the southeast direction. This research concept and methodology can be utilized in regions beyond the Kuye River Basin where landscape ecological risk and human activity intensity are elevated. Upon analyzing the regional land use structure, landscape ecological risks, and human activities along with their interrelationships, we can implement rational land use planning, preserve landscape integrity, mitigate landscape ecological risks, and identify critical management areas while devising management strategies, thereby transforming the conflict between humans and land into a harmonious coexistence (Wang, 2023).
This study aimed to develop human activity intensity indices that encompass natural, economic, and social levels. These indices were designed to accurately represent the characteristics of human activities in the study area. By avoiding the limitations of previous studies that focused on a single variable, this approach ensured a comprehensive representation of the indices. Additionally, we examined the spatial correlation between these indices by constructing landscape ecological risk indices. This method enhances the examination of landscape ecological risk by providing insights into ecological risk management based on land use patterns. Additionally, it analyses the impact of human activity intensity on regional landscape ecological risk, offering scientific assistance for regional scientific planning and development strategies within the framework of the “two mountains” theory. The selection of the geographically weighted regression model for regression analysis in the spatial correlation study is based on its superior fit. This approach addresses the limitation of ordinary linear regression in accounting for the spatial heterogeneity of indicators, thereby enhancing the accuracy of the research.
5.2 Conclusion
The classification of landscape types within the research region was conducted using Landsat 4-5TM and Land 8 OLI_TIRS series data, as well as ENVI software, with a focus on remote sensing data and landscape ecology. The landscape types were classified into six primary categories, including cultivated land, grassland, wood land, waters, construction land, and unutilized land. The research region was evaluated for landscape ecological risk using ArcGIS and Fragstats software. This assessment was conducted across three dimensions: a landscape disturbance index, landscape vulnerability index, and landscape loss index. The spatial correlation characteristics of landscape ecological risk and human activity intensity were analyzed using the geographically weighted regression model and spatial statistical function of ArcGIS software, based on the spatial distribution data of land use, night lighting, and population from 2000 to 2022. The study yielded some significant findings and conclusions.
(1) The human disturbances in the Kuye River Basin have had heightened impacts between 2000 and 2022, resulting in a more intricate and fragmented landscape pattern characterised by increasing fragmentation. The predominant landscape types in the Kuye River Basin have consistently been cultivated land, grassland, and construction land. The overall trend in these areas is a decline in the size of cultivated land and grassland patches, while the area of construction land patches is increasing. Construction land is gradually expanding from the northwest to the southeast of the basin.
(2) The Kuye River Basin has a pattern of declining and then rising landscape ecological risk. Specifically, the landscape ecological risk level of the basin saw a decline from 2000 to 2015, followed by an increase thereafter. Spatially, it has a distribution pattern characterised by “high values in the northern and southern regions and low values in the middle”. From a geographical perspective, it can be observed that areas with high ecological risk are predominantly situated in the northern and southern portions of the basin. Conversely, regions with reduced ecological risk and low ecological risk are distributed over the majority of the basin, encompassing the eastern, western, southern, and northern areas.
(3) An increasing ecological risk in the landscape has been observed in the northern part of the basin, particularly in the northwestern region, as well as in parts of the central and southeastern parts of the basin, and in the surrounding areas. This correlation is attributed to the medium-emphasis and high-intensity human activities in these areas. In the remaining areas of the watershed, there is a limited presence of human life, with minimal human activity and a low level of ecological danger to the landscape. The origins of this phenomenon can be attributed to the elevated altitude of the region, little human presence, and extensive covering of wooded areas and grasslands. The extension of building land area, population increase, and development of other industrial businesses do not pose a significant threat to the ecological danger of the landscape, as a result of socio-economic development.
Data availability statement
The original contributions presented in the study are included in the article/supplementary material. Further inquiries can be directed to the corresponding author.
Author contributions
YW: Data curation, Writing – original draft. FQ: Funding acquisition, Project administration, Writing – review & editing. LL: Formal Analysis, Writing – review & editing. XD: Supervision, Formal Analysis, Writing – review & editing.
Funding
The author(s) declare that financial support was received for the research, authorship, and/or publication of this article. Study on the hydraulic erosion process of thin overburden arsenic sandstone slopes in exposed arsenic sandstone areas (2024QN03062), Evolution of ecosystem structure and function and its impact on water and sediment processes in the watershed (2022 EEDS KJXM005-01), Study on Gully Slope Erosion Mechanism in Pisha Sandstone Area of Yellow River Basin (2021SHZR2545), Study on Erosion Process of Bare Bedrock-soil Composite Slope in Pisha Sandstone Area (41967008).
Conflict of interest
The authors declare that the research was conducted in the absence of any commercial or financial relationships that could be construed as a potential conflict of interest.
Publisher’s note
All claims expressed in this article are solely those of the authors and do not necessarily represent those of their affiliated organizations, or those of the publisher, the editors and the reviewers. Any product that may be evaluated in this article, or claim that may be made by its manufacturer, is not guaranteed or endorsed by the publisher.
References
Airiken M., Zhang F., Chan N. W., Kung H. T. (2022). Assessment of spatial and temporal ecological environment quality under land use change of urban agglomeration in the North Slope of Tianshan, China. Environ. Sci. pollut. Res. 29, 12282–12299. doi: 10.1007/s11356-021-16579-3
Angeler D. G., Alvarez-Cobelas M. (2005). Island biogeography and landscape structure: Integrating ecological concepts in a landscape perspective of anthropogenic impacts in temporary wetlands. Environ. pollut. 138, 420–424. doi: 10.1016/j.envpol.2005.04.020
Cao Q. W., Zhang X. W., Lei D. M., Guo L. Y., Sun X. H., Kong F. E., et al. (2019). Multi-scenario simulation of landscape ecological risk probability to facilitate different decision-making preferences. J. Cleaner. Production. 227, 325–335. doi: 10.1016/j.jclepro.2019.03.125
Cao Q. W., Zhang X. W., Ma H. K., Wu J. S. (2018). Research progress of landscape ecological risk and evaluation framework based on ecosystem services: ESRISK. J. Geogr. 73, 843–855. doi: 10.11821/dlxb201805005
Cerretelli S., Poggio L., Gimona A., Yakob G., Boke S., Habte M., et al. (2018). Spatial assessment of land degradation through key ecosystem services: The role of globally available data. Sci. Total. Environ. 628-629, 539–555. doi: 10.1016/j.scitotenv.2018.02.085
Chen L. D., Fu B. J., Xu J. Y., Gong J. (2003). Landscape pattern identification method based on source-sink ecological process - landscape spatial load contrast index. Ecology 11, 2406–2413. doi: CNKI:SUN:STXB.0.2003-11-025
Chen H. J., Liu L., Zhang Z. Z., Liu Y., T,ian H., Kang Z. W., et al. (2022). The spatial-temporal correlation between human activity intensity and surface temperature on the northern slope of Tianshan Mountains. Geograph. J. 77, 1244–1259. doi: 10.11821/dlxb202205014
Chen X. D., Yang Z. P., Wang T., Han F. (2022). Landscape ecological risk and ecological security pattern construction in world natural heritage sites: a case study of Bayinbuluke, Xinjiang, China. Isprs. Int. J. Geo-Information. 11, 6. doi: 10.3390/ijgi11060328
Cheng X. M., Zhang Y. P., Yang G. F., Nie W. B., Wang Y. Y., Wang J., et al. (2023). Landscape ecological risk assessment and influencing factor analysis of basins in suburban areas of large cities - A case study of the Fuchunjiang River Basin, China. Front. Ecol. Evol. 11. doi: 10.3389/fevo.2023.1184273
Ding D., Sallis J. F., Conway T. L., Saelens B. E., Frank L. D., Cain K. L., et al. (2012). Interactive effects of built environment and psychosocial attributes on physical activity: A test of ecological models. Ann. Behav. Med. 44, 365–374. doi: 10.1007/s12160-012-9394-1
Fang Y., Wang J., Fu S. H., Zhai T. L., Huang L. Y. (2022). Changes in ecological networks and eco-environmental effects on urban ecosystem in China’s typical urban agglomerations. Environ. Sci. pollut. Res. 29, 46991–47010. doi: 10.1007/s11356-022-19226-7
Feng Z. H., Lai H. M., Wu D. F., Lin T. H., Chen C. L. (2022). Study on the spatial-temporal coupling mechanism of urban spatial expansion and landscape ecological risk-Taking Guangzhou as an example. Smart. City. 8, 7–11. doi: 10.19301/j.cnki.zncs.2022.08.003
Fu B. J., Lu Y. H. (2006). The progress and perspectives of landscape ecology in China. Prog. Phys. Geography-Earth. Environ. 30, 232–244. doi: 10.1191/0309133306pp479ra
Gong J., Yang J. X., Tang W. W. (2015). Spatially explicit landscape-level ecological risks induced by land use and land cover change in a national ecologically representative region in China. Int. J. Environ. Res. Public Health 12, 14192–14215. doi: 10.3390/ijerph121114192
Guo X. Y., Zhang Z. K., Zhang X. Q., Bi M., Das P. (2023). Landscape vulnerability assessment driven by drought and precipitation anomalies in Sub-Saharan Africa. Environ. Res. Lett. 18, 6. doi: 10.1088/1748-9326/acd866
Gurbuz I. B., Nesirov E., Ozkan G. (2020). Investigating environmental awareness of citizens of Azerbaijan: A survey on ecological footprint. Environ. Dev. Sustainabil. 23, 10378–10396. doi: 10.1007/s10668-020-01061-w
Han F. L., Li C. T. (2019). Environmental impact of tourism activities on ecological nature reserves. Appl. Ecol. Environ. Res. 17, 9483–9492. doi: 10.15666/aeer/1704_94839492
Hauschild M. Z., Kara S., Ropke I. (2020). Absolute sustainability: Challenges to life cycle engineering. Cirp. Annals-Manufactoring. Technol. 69, 533–553. doi: 10.1016/j.cirp.2020.05.004
Hou J. D., Zhou R., Ding F., Guo H. X. (2022). Does the construction of ecological civilization institution system promote the green innovation of enterprises? A quasi-natural experiment based on China’s national ecological civilization pilot zones. Environ. Sci. pollut. Res. 29, 67362–67379. doi: 10.1007/s11356-022-20523-4
Hu W. W., Wang G. X. (2007). Research progress on wetland landscape pattern and ecological process. Geosci. Prog. 9, 969–975. doi: CNKI:SUN:DXJZ.0.2007-09-011
Jiao K., Yang Z. S. (2008). Comparative analysis of the old and new standards of land use classification in China. Modern. Agric. Sci. Technol. 1, 296–297 + 300. doi: 10.3969/j.issn.1007-5739.2008.23.196
Jin M. T., Xu L. P., Xu Q. (2021). Multi-scenario landscape ecological risk assessment and prediction based on FLUS-Markov model-Taking Kezhou in southern Xinjiang as an example. Arid. Zone. Study. 38, 1793–1804. doi: 10.13866/j.azr.2021.06.3
Jun Z. J., Ming L. K., Qiang C. Y., Min W., Xin P. Z. (2022). Impacts of changing conditions on the ecological environment of the Shiyang River Basin, China. Water Supply. 22, 5689–5697. doi: 10.2166/ws.2022.197
Kang L. J., Di L. P., Deng M. X., Shao Y. Z., Yu G. N., Shrestha R. (2014). Use of geographically weighted regression model for exploring spatial patterns and local factors behind NDVI-Precipitation correlation. Iee. J. Selected. Topics. Appl. Earth Observ. Remote Sens. 7, 4530–4538. doi: 10.1109/JSTARS.2014.2361128
Kivinen S., Koivisto E., Keski-Saari S., Poikolainen L., Tanhuanpää T., Kuzmin A., et al. (2022). A keystone species, European aspen (Populus tremula L.), in boreal forests: Ecological role, knowledge needs and mapping using remote sensing. For. Ecol. Manage. 462. doi: 10.1016/j.foreco.2020.118008
Lei J. R., Chen Z. Z., Chen Y. Q., Chen X. H., Li Y. L., Wu T. T. (2020). Evolution of wetland landscape ecological security pattern in Hainan Island from 1990 to 2018. Ecol. Environ. J. 29, 293–302. doi: 10.16258/j.cnki.1674-5906.2020.02.010
Liang F. C., Liu H. R., Liu S. Y., Qi X. X., Liu L. M. (2018). The spatial reconstruction strategy of coastal landscape ecological security network in southern Fujian-Taking Jimei District of Xiamen City as an example. Econ. Geogr. 38, 231–239. doi: 10.15957/j.cnki.jjdl.2018.09.027
Liao G., He P., Gao X., Lin Z. Y., Huang C. Y., Zhou W., et al. (2022). Land use optimization of rural production-living-ecological space at different scales based on the BP-ANN and CLUE-S models. Ecol. Indic. 137, 108710. doi: 10.1016/j.ecolind.2022.108710
Liu Q., Yu F. H., Xia X., Zhang M. Y., Wang X. M., Mu X. M., et al. (2023). Landscape pattern evolution and driving forces of land use in Kuye River Basin from 1980 to 2020. Soil Water Conserv. Res. 30, 335–341. doi: 10.13869/j.cnki.rswc.2023.05.029
Lynch H. J., Hodge S., Albert C., Dunham M. (2008). The greater Yellowstone ecosystem: Challenges for regional ecosystem management. Environ. Manage. 41, 820–833. doi: 10.1007/s00267-007-9065-3
Ma Y. Y., Liu Z. F. (2021). Landscape ecological risk assessment and its dynamic response to urbanization in Jiangsu Province. J. Nanjing. Forestry. Univ. (Natural. Sci. Edition). 45, 185–194. doi: 10.12302/j.issn.1000-2006.202008049
O’Connor T. G., Kuyler P. (2009). Impact of land use on the biodiversity integrity of the moist sub-biome of the grassland biome, South Africa. J. Environ. Manage. 90. 1, 384–395. doi: 10.1016/j.jenvman.2007.10.012
Ordonez A., Martinuzzi S., Radeloff V. C., Williams J. W. (2014). Combined speeds of climate and land-use change of the conterminous US until 2050. Nat. Climate Change 4, 811–816. doi: 10.1038/NCLIMATE2337
Peng J., Dang W. X., Liu Y. X., Zong M. L., Hu X. X. (2015b). Research progress and prospect of landscape ecological risk assessment. J. Geogr. 70, 664–677. doi: 10.11821/dlxb201504013
Peng J., Zong M. L., Hu Y. N., Liu Y. X., Wu J. S. (2015a). Assessing landscape ecological risk in a mining city: a case study in Liaoyuan City, China. Sustainability 7, 8312–8334. doi: 10.3390/su7078312
Ran P. L., Hu S. G., Tong L. Y. (2022). Exploring changes in landscape ecological risk in the Yangtze River Economic Belt from a spatiotemporal perspective. Ecol. Indic. 137. doi: 10.1016/j.ecolind.2022.108744
Rapport D. J., Maffi L. (2011). Eco-cultural health, global health, and sustainability. Ecol. Res. 26, 1039–1049. doi: 10.1007/s11284-010-0703-5
Ren H. Y., Liu Y. J., Wang X. H., Sun H. R., Fu H. (2024). Evolution of land use landscape pattern and influencing factors in rapid urbanization areas: A case study of Suzhou City. J. Northeast Normal. Univ. (Natural. Sci. Edition). 56, 143–153. doi: 10.16163/j.cnki.dslkxb202201170001
Rong Y., Li C., Xu C., Yan Y. (2017). Spatial differentiation of ecosystem service value changes and human activities in the process of urbanization-a case study of Huanghua City. J. Ecol. 36, 1374–1381. doi: 10.13292/j.1000-4890.201705.00
Sallis J. E., Cervero R. B., Ascher W., Henderson K. A., Kraft M. K., Kerr J. (2006). An ecological approach to creating active living communities. Annu. Rev. Public Health 27, 297–332. doi: 10.1146/annurev.publhealth.27.021405.102100
Shao C. F., Ju M. T., Zhang Y. F., Li Z. (2008). Evaluation of eco-environmental security in Tianjin Binhai New Area based on DPSIR model. J. Saf. Environ. 5, 87–92. doi: 10.3969/j.issn.1009-6094.2008.05.024
Su J., Yin H. W., Kong F. H. (2021). Ecological networks in response to climate change and the human footprint in the Yangtze River Delta urban agglomeration, China. Landscape Ecol. 36, 2095–2112. doi: 10.1007/s10980-020-01129-y
Sutton P. C., Anderson S. J., Costanza R., Kubiszewski I. (2016). The ecological economics of land degradation: Impacts on ecosystem service values. Ecol. Econ. 129, 182–192. doi: 10.1016/j.ecolecon.2016.06.016
Tian Y. N., Ma L., Wu Q. (2023). Land use evolution and landscape ecological risk assessment in Inner Mongolia section of the Yellow River Basin. Ecol. Sci. 42, 103–113. doi: 10.14108/j.cnki.1008-8873.2023.05.013
Wang H. (2023). Regional ecological risk assessment of the Yellow River Delta High-efficiency Eco-economic Zone, China, with respect to human production-living disturbance. Environ. Dev. Sustainabil. doi: 10.1007/s10668-023-03986-4
Wang J. L., Chen C. L., Ni J. P., Xie D. T., Shao J. A. (2018a). Evaluation of agricultural non-point source pollution resistance and spatial pattern of ‘source-sink’ risk in small watershed. Acta Agric. Eng. 34, 216–224 + 306. doi: CNKI:SUN:NYGU.0.2018-10-027
Wang J., Guan S. Q., Yan W. B., Jiao B. B. (2021). Spatio-temporal evolution characteristics and carbon emission effects of production-living-ecological land in Qinghai Province. Environ. Sci. Technol. 44, 212–218. doi: 10.19672/j.cnki.1003-6504.2021.04.027
Wang J., Lin Y. F., Glendinning A., Xu Y. Q. (2018b). Land-use changes and land policies evolution in China’s urbanization processes. Land. Use Policy 75, 375–387. doi: 10.1016/j.landusepol.2018.04.011
Wang M., Sun X. F. (2016). Potential impact of land use change on ecosystem services in China. Environ. Monit. Assess. 188, 4. doi: 10.1007/s10661-016-5245-z
Wang J. Z., Zhang G. H., Nie Z. L., Yan M. J. (2009). Quantitative assessment of human activity intensity in plain area of Hutuo River Basin. Resour. Environ. Arid. Area. 23, 41–44. doi: CNKI:SUN:GHZH.0.2009-10-008
Wang T., Zhang C., Yu X. Y., Cao X. W. (2017). Land use change and its impact on landscape ecological risk in Erhai Lake Basin. J. Ecol. 36, 2003–2009. doi: 10.13292/j.1000-4890.201707.029
Wei C. J., Wang Y. F., Cai X. S. (2023b). Study on dynamic evolution of land use and ecological environment in Shandong Province. J. Appl. Basic. Eng. Sci. 31, 1183–1189. doi: 10.16058/j.issn.1005-0930.2023.05.010
Wei J. Y., Yu R., Fu D. Y., Su Y., Wang Q. (2023a). Spatial-temporal correlation analysis of landscape ecological risk and human activity intensity in Chengdu-Chongqing urban agglomeration. J. Anhui. Agric. Univ. 50, 887–896. doi: 10.13610/j.cnki.1672-352x.20230915.001
Weigand M., Wurm M., Droin A., Stark T., Staab J., Rauh J., et al. (2023). Are public green spaces distributed fairly? A nationwide analysis based on remote sensing, open street map and census data. Geocarto. Int. 38, 1. doi: 10.1080/10106049.2023.2286305
Wu H. F. (2023). Study on human activity intensity and land use landscape pattern change in Yuncheng City. Kunming. Univ. Sci. Technol. doi: 10.27200/d.cnki.gkmlu.2023.001244
Wu W. L., Huang Y., Zhang Y., Zhou B. (2024). Research on the synergistic effects of urbanization and ecological environment in the Chengdu-Chongqing urban agglomeration based on the Haken model. Sci. Rep. 14, 117. doi: 10.1038/s41598-023-50607-1
Xiao X. Y., Huang X., Jiang L. L., Jin C. X. (2022). Empirical study on comparative analysis of dynamic degree differences of land use based on the optimization model. Geocarto. Int. 37, 9847–9864. doi: 10.1080/10106049.2022.2025919
Xiao Y., Li Y., Huang H. (2021). Conflict or coordination? Assessment of coordinated development between socioeconomic and ecological environment in resource-based cities: Evidence from Sichuan province of China. Environ. Sci. pollut. Res. 28, 66327–66339. doi: 10.1007/s11356-021-15740-2
Xu K. P., Wang J. J., Chi Y. Y., Liu M., Lu H. J. (2016). Countermeasures for land use optimization and sustainable utilization in Yunnan-Guizhou Plateau based on comprehensive ecological risk. Ecology 36, 821–827. doi: 10.7666/d.Y1795500
Yan Y. C., Ju H. R., Zhang S. R., Chen G. K. (2021). The construction of ecological security Patterns in coastal areas based on landscape ecological risk assessment-a case study of Jiaodong Peninsula, China. Int. J. Environ. Res. Public Health 18, 22. doi: 10.3390/ijerph182212249
Yang G., Zhang Z. J., Cao Y. H., Zhuang E. N., Yang K., Bai Z. K. (2021). Spatio-temporal heterogeneity of landscape ecological risk in large open-pit mining areas in northern Shanxi. Ecology 40, 187–198. doi: 10.13292/j.1000-4890.202101.003
Yu F., Liu J., Xia L. H., Long X. C., Xu Z. W. (2022). Landscape ecological risk assessment based on LUCC in Weibei highland of Shaanxi. China Environ. Sci. 42, 1963–1974. doi: 10.19674/j.cnki.issn1000-6923.20220112.002
Yuan Y., Sheng Y., Liu L. F., Wang S., Li J., An L. (2023). Study on the spatial and temporal evolution characteristics of habitat quality and its influence mechanism in the Cave Wild River Basin. For. Grass. Resour. Res. 6, 67–74. doi: 10.13466/j.cnki.lczyyj.2023.06.008
Zhang X. M., Du H. M., Wang Y., Chen Y., Ma L., Dong T. X. (2021). Watershed landscape ecological risk assessment and landscape pattern optimization: Take Fujiang River Basin as an example. Hum. Ecol. Risk Assess. 27, 9-10, 2254–2276. doi: 10.1080/10807039.2021.1970511
Zhang Z. Y., Ge H. L., Li X. N., Huang X. Y., Ma S. L., Bai Q. F. (2023). Spatiotemporal patterns and prediction of landscape ecological security in Xishuangbanna from 1996-2030. PloS One 18, 11. doi: 10.1371/journal.pone.0292875
Zhang J. Y., Su W. C., Zhang F. T. (2011). Evaluation of land ecological security in the Three Gorges Reservoir Ecological Economic Zone based on PSR model. China Environ. Sci. 31, 1039–1044. doi: CNKI:SUN:ZGHJ.0.2011-06-035
Zhang C., Wang L., Zhang Q. X., Chen G. F. (2016). Study on land use transfer matrix and spatial change in Buha River Basin. Water Conservancy. Hydropower. Technol. 47, 6–11. doi: 10.13928/j.cnki.wrahe.2016.05.002
Zhang Y. G., Zhao Y., Chen Q. W., Zhu Y. J., Liu B., Zhang X. M., et al. (2024). Capacity of forests and grasslands to achieve carbon neutrality in China. Forests 15, 6. doi: 10.3390/f15061060
Zhang X. W., Zhu W. W., Yan N. N., Wei P. P., Zhao Y. F., Zhao H., et al. (2022). Research on service value and adaptability zoning of grassland ecosystem in Ethiopia. Remote Sens. 14, 11. doi: 10.3390/rs14112722
Zhao Y., Tao Z., Wang M. N., Chen Y. H., Wu R., Guo L. (2022). Landscape ecological risk assessment and planning enlightenment of Songhua River Basin based on Multi-Source heterogeneous data fusion. Water 14, 24. doi: 10.3390/w14244060
Keywords: land use/land cover, human activity intensity, landscape metrics, landscape ecological risk assessment, correlation, Kuye River basin
Citation: Wu Y, Qin F, Li L and Dong X (2024) Exploring landscape ecological risk with human activity intensity and correlation in the Kuye River Basin. Front. Ecol. Evol. 12:1409515. doi: 10.3389/fevo.2024.1409515
Received: 02 April 2024; Accepted: 07 November 2024;
Published: 04 December 2024.
Edited by:
Pedzisai Kowe, Midlands State University, ZimbabweReviewed by:
Subhanil Guha, National Institute of Technology Raipur, IndiaTatenda Musasa, Midlands State University, Zimbabwe
Copyright © 2024 Wu, Qin, Li and Dong. This is an open-access article distributed under the terms of the Creative Commons Attribution License (CC BY). The use, distribution or reproduction in other forums is permitted, provided the original author(s) and the copyright owner(s) are credited and that the original publication in this journal is cited, in accordance with accepted academic practice. No use, distribution or reproduction is permitted which does not comply with these terms.
*Correspondence: Xiaoyu Dong, ZG9uZ3hpYW95dTIwMTlAMTI2LmNvbQ==
†ORCID: Fucang Qin, orcid.org/0009-0008-0431-6615