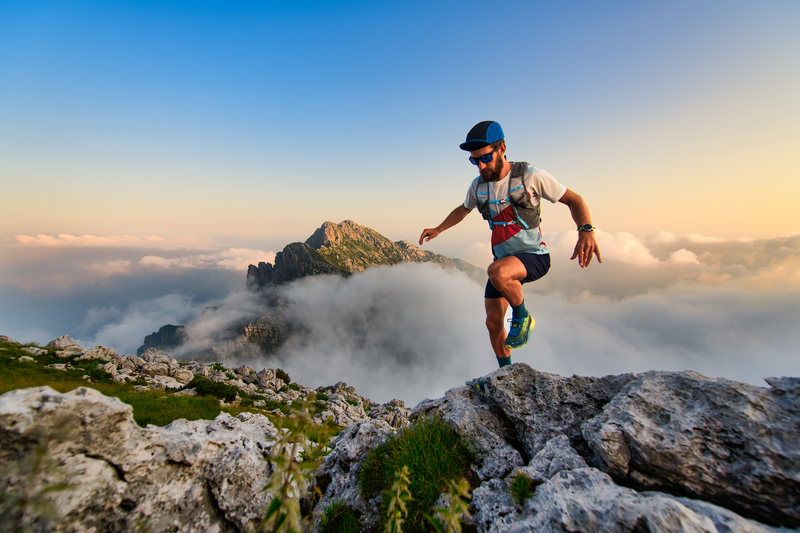
95% of researchers rate our articles as excellent or good
Learn more about the work of our research integrity team to safeguard the quality of each article we publish.
Find out more
METHODS article
Front. Ecol. Evol. , 09 October 2024
Sec. Models in Ecology and Evolution
Volume 12 - 2024 | https://doi.org/10.3389/fevo.2024.1400936
Numerous threats affect aquatic ecosystems at different biological organizational levels from individuals to ecosystems. Stresses occurring on the metabolism and physiological functions of individuals can have repercussions on the individual behavior, its ability to survive and reproduce, also known as the individual fitness, which may then influence the demography and spatial distribution of populations, and ultimately modify trophic flows and ecosystem functioning. In a context of a globally changing environment, predicting the life history traits and fitness of individuals can be relevantly performed with the association of laboratory experiments with Dynamic Energy Budget (DEB) theory, while modeling species interactions have proven to be an efficient tool to understand aquatic food webs using mass-balanced models such as linear inverse models (LIMs) or Chance and Necessity (CaN) models. However, while predictive results obtained on individuals can be provided with a thorough mechanistic interpretation, the propagation of the effects is most often limited to the closest biological hierarchical level, i.e., the population, and rarely to the food-web level. Furthermore, there is a need to understand how to avoid misleading approaches and interpretations due to the simplicity of experiments. For the moment, no clear methodology has stood out yet to do so. In this study, we provide a new methodology based on a combination of models (i.e., DEB, LIM, and CaN) aiming at upscaling information from laboratory experiments on individuals to ecosystems to address multiple ecological issues. This framework has a potential to enhance our understanding of higher-scale consequences of the effect of stressors measured at the sub-individual scale. This combination of models was chosen for the convergence of their framework but also their ability to consider a substantial portion of the projected uncertainty. The description of this methodology can help experimenters and modelers to jointly address a specific question involving upscaling from individual to ecosystem, proposes approaches, and gives tips on the pitfalls to avoid along the upscaling process.
Numerous threats affect aquatic ecosystems, such as climate change (IPCC, 2019, 2022), pollution (Islam and Tanaka, 2004; Häder et al., 2020), habitat degradation and loss (Turner et al., 1999; Geist and Hawkins, 2016), invasive species (Molnar et al., 2008; Gallardo et al., 2016), and resource overexploitation (Pauly et al., 2005; Crowder et al., 2008).
Their effects can be observed at different biological organizational levels from individuals to ecosystems. Climate change (e.g., temperature increase, acidification) is, for example, known to induce multiple stresses* [i.e. the physiological cascade of events that occurs when the organism is attempting to resist death or reestablish homeostatic norms in the face of insult (Schreck and Tort, 2016); for a definition of terms noted with a “*” symbol, see glossary in Supplementary Table S1] on the metabolism and physiological functions of individuals at various biological levels as follows: cells, tissues, and organs. Such alterations can have repercussions on the individual behavior, its ability to survive and reproduce, also known as the individual fitness, which may then influence the demography and spatial distribution of populations, and in fine modify trophic flows and ecosystem functioning (Koenigstein et al., 2016; Poloczanska et al., 2016; Lennox et al., 2019). Individuals could therefore be considered as a key unit of interest for understanding the mechanisms underlying the effects of perturbations on dynamic systems at higher levels of organization (Rijnsdorp et al., 2009; Martin et al., 2012).
In this context of a globally changing environment, predicting the life history traits and fitness of individuals can be relevantly performed using energy budget-based theories (Pörtner and Peck, 2010; Le Quesne and Pinnegar, 2012). Understanding and estimating the internal energy allocation under various conditions is paramount because of the profound impacts it can have on the individual fitness (Claireaux and Lefrançois, 2007). Among the theories able to describe the influence of external factors on individuals, Dynamic Energy Budget (DEB; Nisbet et al., 2000; Kooijman, 2010) theory has had a strong influence on how individuals were represented in terms of internal processes over the last decade (van der Meer et al., 2014). By offering a single and generic quantitative framework to dynamically describe the energy and mass budgets of all living organisms at the individual level, models based on the DEB theory (so-called DEB models) can provide valuable insights on global change impacts on energy allocation within organism and life history traits such as growth or fecundity (e.g., Thomas et al., 2016; Mounier et al., 2020a, b).
At a higher level of biological organization, improving our understanding on the influence of several environmental drivers on biomass-based trophic networks is particularly prominent and advocated as a necessity in today’s ecosystem conservation or management (Fath et al., 2019; Safi et al., 2019). Modeling species interactions has proven to be an efficient tool to understand aquatic food webs (Plagányi, 2007) despite their limits in “projecting the future” (Planque, 2016). Among ecosystem models, mass-balanced* (i.e., following the conservation law) ones and especially Ecopath with Ecosim (EwE; Christensen and Pauly, 1992; Christensen et al., 2005), linear inverse models (LIM; Vézina and Piatt, 1988; Niquil et al., 2011), or Chance and Necessity (CaN; Planque and Mullon, 2020) models form an important part of their diversity (e.g., Coll et al., 2015; Colléter et al., 2015; Stock et al., 2023). These kinds of models generally represent all the trophic levels involved in food webs from primary producers and dead organic matter to top predators and humans. Furthermore, their link with thermodynamic laws generates a strong theoretical basis (Ulanowicz, 2003; Kones et al., 2009; Saint-Béat et al., 2015). All of this allows modelers to assume how an environmental disturbance can propagate within a food web.
However, usually, while predictive results obtained on individuals (from observations or experiments for instance) can be provided with a thorough mechanistic interpretation, the propagation of the effects is most often limited to the closest biological hierarchical level, i.e., the population, and rarely to the food-web level (Stock et al., 2023). Also, inputs provided by experiments are often limited for higher trophic levels to specific ages/stages, mostly younger ones and thus, most of the time, not the main ages observed in situ. In consequence, there is a need to understand how impacts can differ with age/stage and how to avoid misleading approaches and interpretations due to the simplicity of experiments. Hence, when using a food-web mass-balanced* modeling approach, experimental results obtained from individuals can be used to adjust growth, consumption, and/or mortality of mono- or multi-specific trophic groups (Koenigstein et al., 2016), but no clear methodology has stood out to do so. In addition, these adjustments often rely on knowledge obtained from previous studies not designed for such endpoint. In this context, there is a necessity to design a clear framework to assess the repercussions of the effects of stress* induced by environmental factors observed at sub-individual and individual levels on trophic dynamics and food webs, thereafter named an upscaling methodology.
A wide range of effects of environmental stresses on trophic dynamics and ecosystems could be represented by a set of mathematical models integrating individual-level experimental data (Le Quesne and Pinnegar, 2012). This would furthermore be associated with a significant gain in mechanistic understanding. Developing such upscaling methodology still represents one of the most open challenges in ecology (Reuter et al., 2010), and remains particularly relevant and timely concerning the effects of environmental changes on aquatic ecosystems (Rijnsdorp et al., 2009; Pörtner and Peck, 2010).
To do so, the DEB theory matches the requirements to build a link from sub-individual experimental results to the food-web levels (Nisbet et al., 2000, 2012). Past studies already developed links toward the modeling of populations (e.g., Martin et al., 2012; Saraiva et al., 2014; Beaudouin et al., 2015), theoretical communities (Maury and Poggiale, 2013), or simplified food webs (Grangeré et al., 2010). However, a clear methodology to link DEB outputs with food-web modeling has, to our knowledge, not stood out yet.
In this direction, the present study aimed at proposing an upscaling methodology based on a combination of models to address multiple ecological issues starting from individual laboratory experiments, and thereafter linked to individual, then food-web scales in an aquatic environment. Furthermore, this framework was designed to consider a substantial portion of the projected uncertainty. Indeed, literature largely leaves most model assumptions implicit and deals with uncertainty unevenly often omitting this aspect in the interpretation of model results altogether (Gregr and Chan, 2015). Developing such formal framework therefore requires considering at least input data uncertainty (deYoung et al., 2004) with dedicated tools.
All the following sections are linked to arrows in Figure 1 representing the full methodology. Except when mentioned, every hierarchical step must be fulfilled before developing the next one. The present work was on linking hierarchical scales and not on presenting each type of models used in detail. Main references for each model type are provided in a dedicated section, and main processes, inputs, and outputs are given in Supplementary Table S2.
Figure 1. Description of the full upscaling methodology to simulate the consequences of the effects of stress* observed at the sub-individual levels on trophic and food-web dynamics. Boxes represent the different independent compartments of the methodology and arrows the transfer of data between the different boxes. Numbers on each arrow refer to the different sections of the present publication. CTRL, control.
This first step aims at describing the full life cycle of an individual for a species of interest as a function of food and temperature conditions as well as potential additional environmental stressors using a DEB model. DEB models are usually parameterized with data from multiple sources, including literature data or experiments originally designed for the model. Regarding parameter estimation methodologies, we refer the reader to, e.g., Marques et al. (2018); Boersch-Supan and Johnson (2019), and Robles et al. (2023), which all provide corresponding code libraries. Marques et al. (2018) provides details of the AmP procedure and the AmP portal (https://bio.vu.nl/thb/deb/deblab/add_my_pet/index.html) that contains more than 4000 animal species and the associated Matlab libraries DEBtool (2023) and AmPtool (2023) used to estimate these parameter sets. We also refer to other parameter estimation studies based on Bayesian methods such as those of Johnson et al. (2013) and Boersch-Supan and Johnson (2019) that are particularly designed to provide probability distribution of parameter values, but see also the work of Marques et al. (2019) for parameter distribution estimation associated with the AmP procedure (see arrow 3 and Section 2.2.1).
The DEB theory relies on the assumption that species from a wide range of taxonomic groups share the same mechanisms governing their metabolic organization, which can be described by a reduced set of equations for the standard DEB model (Figure 2; Supplementary Table S3; see Kooijman, 2010 for a full description of the model), i.e., the simplest model among the families of existing DEB models. The differences observed among closely related species are then assumed to be due to differences in the input parameter values. Several typified models are now available to capture the diversity of life stages and sexual dimorphism in animal species (Kooijman, 2014; Marques et al., 2018, https://bio.vu.nl/thb/deb/deblab/add_my_pet/index.html).
Figure 2. Schematic representation of the adult stage of the “standard” DEB model (from Kooijman, 2010 and Nisbet et al., 2012). Bold parameters represent energy flows, while other parameters represent fixed fractions. SDA, specific dynamic action. See Section 2.2.1 or Supplementary Table S3 for the definition of the parameters.
Despite having concepts that might be harder to measure empirically, the choice of proposing the DEB in our framework was directed by its ability to facilitate mechanistic understanding of process generating changes in internal flows of individuals, its generalization skills, and also because of the access to valuable resources: extensive literature, code libraries for parameter estimate, data, and operational models in AmP.
Arrow 1 in Figure 1. Laboratory experiments represent the first step of the framework as our goal is to upscale results obtained at the sub-individual level to evaluate their implications at higher organizational levels. Experiment here is understood as a procedure done in a controlled environment in which the effect of one or multiple environmental factors (e.g., diets, pollution; thereafter named conditions), each with one or several treatment levels, are tested at the individual (e.g., growth in length) or sub-individual (e.g., mitochondrial performance) scales. An experiment is, in our definition, always a combination of a control condition and one or several stress* conditions. For example, an experiment testing the effects of a depletion of essential fatty acids on the European seabass Dicentrarchus labrax would follow the growth of individuals knowing their everyday length and weight and their swimming performances, e.g., aerobic scope, basal metabolism. This would be realized with a set of individuals fed with normal fatty acid concentration, i.e., the control condition, and another set of individuals with several levels of fatty acid-depleted food, e.g., −10%, −15%, and −20%, i.e., the stress* conditions. This would be done in controlled environments ideally at different stress* and temperature conditions to obtain functional responses.
A priori, any kind of organism can be used for the experiment, as long as enough knowledge and data are available on the species to further develop a DEB model, and if the size and other traits of the species allow to realize controlled experiments. The purpose of this step is to use the knowledge obtained by the experiments in the development of a DEB model with different conditions. In addition, this knowledge can be a source of data to contribute to the estimate of parameters in the DEB model in control conditions, along with data from literature (see arrow 2 and Section 2.1.2.). All along the experiment, it is essential to follow both the temperature conditions (if not already considered as a stressor condition) and the ingested food. In terms of organism variables, it is necessary to monitor variables that allow the estimation of growth, development, maintenance and reproduction when relevant. Measurements performed both in length (when relevant) and weight during the experiment are particularly useful for a successful integration of experimental results into DEB models. However, considering the initial question, other types of variables can become primordial, e.g., the number of eggs produced if the ecological question explores reproductive success in specific stress conditions. Longitudinal measurements (i.e., repeated in time for the same individual) are also particularly useful when individuals can be marked. Also, to gather other quantitative variables, such as age and food consumption rate, metabolic rate at different temperatures would provide more precision to the following steps. Variables measured both in control and stress* conditions are the most useful to better understand the mode of action (MoA) of the stressor, i.e., the metabolic processes potentially impacted by the stressor.
The choice of age/stage on which experiments are done can be restricted (e.g., availability of individuals, size of the tanks), but one of the powerful benefits of DEB is the possibility to extrapolate results to a larger spectrum of sizes, ages, and stages. Also, when relevant according to the organism of interest, if males and females are discriminated in experiments, this can also be included in the DEB model by using a zoom factor that will differentiate individuals by altering the value of the maximum food assimilation rate in DEB, and the following different life stage thresholds: hatching, birth, metamorphosis, and puberty. Finally, results obtained for the different replicates/individuals will be paramount to tackle the uncertainty that will be considered in the upscaling process, even if an “average” individual can be used in the following modeling steps (see arrow 3 and Section 2.2.1.). Recent developments of the DEB model by, e.g., Boersch-Supan et al. (2017) and Koch and De Schamphelaere (2020) permit to catch the inter-individual variability and overcome the “average” individual approach.
At this step, it is also possible to use a pre-existing DEB model. In this case, if the experiment outputs were not included for the initial estimate of DEB parameters, the model still needs to fit well with the experimental data. Indeed, good fits with the new observations are a first requirement for the following comparison between the predicted individuals in control and stress* condition(s) that will permit deducing DEB parameters that can be influenced by the stress* and in which proportions.
Arrow 2 in Figure 1. Even if a particular experiment focuses on a particular life stage, the full life cycle of an organism will be embedded in a DEB model. Predictions, e.g., of the egg energy content as well as ultimate weight could be made with the same set of parameters. It is therefore commonly done to complement the experimental data of interest with previous experiments and field observations reported in the literature to estimate most accurately the parameter set that best fits all the different sources of data.
Experiments, when possible, often provide information on the younger stages, while field observations will more often provide information on the juvenile and adult stages. If the ecological question at aim in the foreseen work is oriented on the trophic network, it is reasonable to think that for macroorganisms, adults’ stages can represent most of the biomass in the natural environment, and therefore particular attention is required on fitting these older stages in the DEB. Nonetheless, exceptions can occur for some species that can be present in certain habitats only during specific stages, e.g., juvenile fish in nursery grounds.
Field observations useful for the calibration of DEB models are length- and weight-at-age of individuals, their ultimate length and weight, age-, length- and weight-at-maturity, and fecundity as a function of length (or weight) together with proxies for temperature and food conditions. When collecting such data from the literature, data from the spatial area of interest are, of course, more relevant but data from other populations can also be used considering some uncertainties in the estimation procedure (e.g., by using lower weight coefficients*, i.e., coefficients used during the estimation process depending on the confidence in the quality of each dataset and the priorities for an application for these datasets).
LIM modeling is a steady mass-balanced* trophic modeling approach useful to describe the structure and functioning of food webs at the habitat or ecosystem scales (Vézina and Piatt, 1988; Niquil et al., 2011). Its structure and flow mechanism are often identical to the ones described in Ecopath (we consider this case here). Combined with the Markov Chain Monte Carlo method* (i.e., a class of algorithms creating samples from a continuous random variable), it allows, contrary to Ecopath, (i) to consider the probability density function of flows in underdetermined systems and (ii) to avoid underestimations in both the size and complexity of the modeled food webs (Van den Meersche et al., 2009). Classically parameterized with data from multiple sources, LIM can, in the context of our methodology, be adjusted using outputs from the previous steps as described below. This procedure is here only performed for normal conditions to represent the structure of the food web, while the stress* conditions(s) are simulated using the CaN modeling approach, as explained below (see arrows 5 and 7 and Sections 2.3.1 and 2.3.3).
Arrow 3 in Figure 1. In a steady-state situation, the consumption Q (often defined in grams of carbon per kilometer per year (gC.km−2.year-−1) in LIM, which will constitute the reference for the following) of a consumer trophic group i is defined as:
where U (gC.km−2.year−1) is the unassimilated consumption, P (gC.km−2.year−1) is the production, and R (gC.km−2.year−1) is the respiration of the group. P includes all mortalities (i.e., predation, fishing, and other mortalities such as senescence or diseases), net migration and biomass accumulation, while R represents all the losses not attributable to feces (and N-wastes when defined in biomass) constituting U (Christensen et al., 2005; Figure 3). All these flows are often constrained as ratios of Q or the biomass B of the trophic group. It is worth noting that in LIM, natural mortalities other than predation or harvesting M0 (senescence, disease, etc.) are often considered negligible in LIM for secondary consumers (e.g., Chaalali et al., 2015) and are difficult to distinguish from unassimilated consumption. With the use of DEB modeling as input in the LIM, it is now theoretically feasible to clarify this distinction if values of U and P are fixed or precisely narrowed, M0 being grossly the difference between U without DEB-defined constraints and U with them. Note that this would slightly modify the estimated assimilation and production flows.
Figure 3. Schematic representation of the definition of flows for a trophic group in the LIM model. See section 2.2.1. for the definition of the parameters.
It is possible to use different outputs from DEB models to develop ecological threshold values for the species of interest in a given food web (Supplementary Table S4). However, special attention should be given to the definition of the different rates and the corresponding units. Output rates from DEB models are most of the time expressed on a daily basis, while food webs are often represented on a yearly basis in LIM, even if exceptions can occur (e.g., Saint-Béat et al., 2014; Haraldsson et al., 2018). For the following section concerning the dynamic representation of the food web (see arrows 5–7 and Sections 2.3.1–2.3.3), it is easier to represent annual flows. We will thus express DEB fluxes in an annual basis.
The transfer of individual’s values to a trophic group is not straightforward. LIM’s equations, similar to those of Ecopath, follow the ones of Winberg (1956) that were initially bioenergetic functions defined for individuals (Link, 2010). In a mass-balanced* ecosystem modeling framework like LIM, most inputs rely on observations made on individuals, e.g., Q/B ratio, R/B ratio, or diets. In this context, a trophic group could be considered as a single individual organism simplifying the diversity and demography of the group. This choice can be required for simplicity’s sake or due to the absence of precise data on the demography and ontogeny for several species. When comparing different parameterization of LIM models, we assume stable composition of size, age, and even species when the group is multi-specific. This issue was already treated by Christensen et al. (2005) in the “Capabilities and limitations” of the EwE User’s Guide with the proposition of using multiple life stanza by splitting the biomass pools when relevant, i.e., species or groups known to have a strong trophic ontogeny or size-dependent vulnerability to harvest. Moreover, recruitment is often difficult to predict in the aquatic environment and depends on many other parameters than the spawning stock biomass (Subbey et al., 2014). In consequence, population subdivision often might not be necessary because the less costly hypothesis for demography remains to keep it unchanged, except when valuable indices exist. However, changes in the production of a trophic group (for instance in the dynamic projection of the trophic network—see arrows 5–7 and Sections 2.3.1–2.3.3) due to environmental conditions and trophic interactions will be implicitly integrated in trophic flows as changes in the growth and reproduction of individuals. Inevitably, the processes represented by the model would imply changes in the recruitment and demography and even taxonomic diversity (when applicable) of the group in real life conditions, but are not here explicitly represented.
Outputs from DEB models do not solve the issue of linking individual scale outputs to populations, and assumptions must still be done. However, being available at all possible ages or lengths, these outputs can be produced for all sizes/ages of a population and weighted according to the known demography or a good indicator of it for each of the species present in the trophic group of interest to provide one value representative of each population. In the absence of knowledge on the demography, the easiest option is to keep the values obtained at a middle-adult stage with the DEB model representing most of the time for macroorganisms the main part of the biomass, e.g., the common length from the Fishbase database for fish (Froese and Pauly, 2000), if adult individuals are known to occur in the represented ecosystem. In addition, the vital rates (i.e., consumption, assimilation, egestion, production, respiration, excretion when relevant) only slightly evolve at the adult stage and thus limit the error due to uncertainty on demography. To obtain the annual DEB outputs, each flow is simply cumulated over the 365 days of every age or at the age of interest potentially divided by the average weight of the individual at that age to obtain its ratio per biomass (i.e., Q/B, P/B, R/B).
Cautions have to be taken if the trophic group is multi-specific. Similarly to the demography issue raised above for populations, assumptions have to be done to upscale from individual to trophic groups. In this case, the most straightforward one is to use the species for which experimental data are available as the reference and consider the results identical for all the species composing the group. This hypothesis seems reasonable because the species composing such group are supposed to be gathered according to their diet and vital rates’ similarities. Another solution would be to weight the values obtained from DEB modeling according to the biomass proportion of the species in the group to provide ecological thresholds* (i.e., minimum or maximum values of a constraint* defined by inequality) for the LIM. However, this would require having other constraints* (i.e., model input data to limit the solutions for the flows to possible values) value for the other species constituting the group, which is not always the case.
For a multi-age and multi-specific trophic group, a constraint* φ is defined as:
For each species s and age a composing the trophic group.
The better use of Q as constraint* for the LIM is to compute a value relatively to B of the trophic group, i.e., Q/B. To give the best flexibility to the LIM, we propose to use the thresholds* of P and R relatively to Q, i.e., the net growth efficiency P/Q and R/Q.
The conversion of DEB outputs to Q, P, and R is obtained from Nisbet et al. (2012), who worked on relating the DEB theory to other bioenergetic approaches to obtain, in our case, vital rates in gC.year−1 and biomass in gC following the LIM.
The relation between the representations of the consumption rate in DEB noted and LIM is the most straightforward. (derived from the “food consumption rate” C of Nisbet et al., 2012) is computed as:
where is the ingestion rate (J.year−1), is the molecular weight of carbon (g.mol−1), and is the chemical potential of food (J.mol−1).
The animal’s biomass in DEB is computed as the sum of the structure, reserve and (for adults) reproductive reserve:
where is the structural weight (g), is the reserve weight (g), is the weight of the reproduction buffer (g), is the density of the structural volume (g.cm−3), V the structural volume (cm3), is the molar weight of the reserve (g.mol−1), is the reserve energy (J), is the reproduction buffer energy (J), and is the chemical potential of reserve (J.mol−1).
The production rate in DEB (from the “growth” G of Nisbet et al., 2012) is computed as:
where is the assimilation rate (J.year−1), is the fraction of energy for growth that is fixed into structure, is the rate of energy allocated to growth (J.year−1), and the dissipation rate (J.year−1), which encompasses all processes not associated with the production of new reserve and structure (Figure 2).
If the currency used in the LIM is the carbon, the respiration rate in DEB (from the sum of “specific dynamic actions” S and “standard metabolism and activity” MA of Nisbet et al., 2012) is computed as:
where is the assimilation efficiency in the DEB, is the fraction of the ingestion rate transformed into feces, and is the chemical potential of feces (J.mol−1). If the LIM is defined in biomass, excretion has also to be considered following Equation 8 from Nisbet et al. (2012). This case is not tackled in our methodology.
The assimilation efficiency as defined in LIM obtained from the DEB and noted is computed as:
As explained above, the use of for the Q/B input in the LIM requires its division by the average weight of the individual during a year. The use of and as P/Q and R/Q ratio only requires their division by .
An equality equation* (defined as x = φ, with x as unknown flow and φ as empirical data) for the LIM can then be defined using DEB outputs for a given constraint* φ. A DEB model classically gives only one output and not the uncertainty around it. However, using only these values as equations in the LIM is giving too much credit on the DEB model predictions, while it is possible to consider its variability and uncertainty by building LIM inequations* (defined as x ≥ φ or x ≤ φ).
As previously evoked, there are ways to obtain more complex outputs than a single value from the DEB, i.e., the Bayesian method developed by Boersch-Supan et al (2017); Boersch-Supan and Johnson (2019) or the confidence interval definition proposed by Marques et al. (2019). If available, the outputs from these methods can provide much better estimates of the thresholds* provided for the LIM, even if we still have to set their limits, at least when using the Bayesian method (e.g., 5th and 95th percentiles of predictions). Once the thresholds* are defined by the modeler, a set of inequations* can thus be defined for a constraint* φ knowing the minimum and maximum values that xi can reach.
If the last option is not feasible, another one is to use an equivalent of the Ecopath pedigree* (i.e., a coded statement categorizing the origin of a given input and by the same token specifying the likely uncertainty associated with the input; Christensen et al., 2005; Supplementary Table S5), as previously done by Nogues et al. (2021), i.e., giving a fixed percentage of uncertainty ζ around the output considered as an average value. A typical set of inequalities* for φ can thus be obtained:
ζ has to be given according to the confidence the user has on DEB’s outputs to describe the species in the area of interest.
Arrow 4 in Figure 1. Despite the contribution of inputs from the previous steps, literature still constitutes a valuable source of information to evaluate the flow intensity within biomass-based trophic networks. These can belong to different sources, e.g., experimental studies, in situ observations, empirical relationships, other calculations from other values or even from other models when judged credible, which inform on the diet and vital rates of the groups. If there is no pre-existing LIM of the concerned ecosystem, all information necessary on how to build such model can be found in the work of Niquil et al. (2011).
The trophic network being static in LIM, the biomass is fixed, and a median or mean value is therefore to be given when having a time-series of biomass for the group observed. It is equivalent to consider that the variation of biomass is negligible throughout the time step of the model (e.g., the year). It is constituted by the different stages/ages of the species, except when the choice was made to use a multi-stanza group (see arrow 3 and Section 2.2.1).
Information on the species of interest, in addition to the DEB, is useful, at least concerning its/their diet. Concerning vital rates, the use of good quality thresholds* can be valuable in addition to outputs from the DEB because despite the potential of adding an equivalent of Ecopath pedigree* uncertainty around the predictions (see arrow 3 and Section 2.2.1.), DEB modeling still mostly relies on a single output when not using a methodology that considers uncertainty (see Section 2.2.1). By using additional literature-based thresholds*, the plausibility coverage by the thresholds* obtained from the DEB can be reduced.
CaN modeling is close to the concept of LIM modeling (Supplementary Table S6), but while the latter takes into account uncertainty on trophic flows in a static way, CaN modeling considers the uncertainty and variability of trophic relationship intensity in a dynamic way (Planque and Mullon, 2020). It can be performed using the RCaN package in the R environment (Drouineau et al., 2021; Drouineau et al., 2023). All the details on the implementation of parameters and constraints* in the CaN modeling are given by Mullon et al. (2009); Planque et al. (2014), and Lindstrøm et al. (2017).
In a CaN model, the variation in the biomass of a trophic group i is described as:
where γ is the assimilation efficiency* (i.e., the proportion of prey biomass ingested by i that can contribute to growth, reproduction, and maintenance), is the biomass of prey groups j consumed by i, is the biomass of i consumed by its predators, and are the imports and exports of i in or out of the system, respectively, represents the other losses* (i.e., a correction factor that accounts for variations in energy content between prey), and is the biomass of i.
Arrow 5 in Figure 1. A comparison of two LIM models could be done if biomass observations were available in the control and stress* conditions. However, as it is rarely the case, the purpose of the LIM step was to obtain a reliable balanced* version of the trophic network to simulate the control state that will serve as a basis for comparisons with the stress* condition(s) with CaN modeling. We do not consider that LIM is well adapted for projection in the future of scenarios as, except in situations of extreme biomass variation like during phytoplankton blooms (e.g., Haraldsson et al., 2018), LIM works with a static biomass, neglecting biomass variations, compared to flow values. By modifying vital rates of the species of interest directly from the DEB to the LIM, one would assume that all the biomasses of the different trophic groups would remain stable and that flows would adapt with this situation, which seems unlikely in reality. Here, to build projections of food webs under stress* condition(s), the variation of biomass needs to be estimated by a model due to the important effects of biomass variations on trophic network’s structure and functioning. The LIM step can even be eventually by-passed to use directly the CaN modeling, which is, in our context, the best tool to answer the question of trophic relationships in the transition from control to stress* conditions, but this would generate a minimized exploration of uncertainty on CaN input parameters.
Biomasses have to be set in our methodology for the initial year of the simulation. Otherwise, these would not be constrained and generate dynamics far from the goal of having a representation of the control condition. The simplest way is to use the B values used for the LIM. After this first year, no value is given for the biomasses for the rest of the simulation (see arrow 6 and Section 2.3.2).
Every iteration of the LIM is a potential version of the trophic network under the control conditions. If we aim to limit the number of simulations within the CaN modeling, it is safer to initialize the CaN parameters from the mean value of the flows obtained from the LIM (Saint-Béat et al., 2013). However, a way to take advantage of the uncertainty of the LIM is to use a given number of randomly chosen solutions of the vector of flows. By this operation, the uncertainty of the LIM would be spread on the main parameters of the CaN model. From each of these versions of the trophic network, multiple simulations would finally be done with the CaN modeling. This technical solution increases drastically the number of simulations to run and analyze but remains the most rigorous for uncertainty evaluation.
Multiple outputs from LIM can be used in the CaN modeling. Within this latter, the main parameters driving the simulations are the refuge biomass* (i.e., the absolute minimum biomass that a trophic group can reach), the prey digestibility* (i.e., a correction factor that accounts for variations in energy content between prey), the assimilation efficiency*, the satiation* (i.e., the maximum consumption rate per unit biomass of the predator), the inertia* (i.e., a parameter that expresses the variations in biomass from 1 year to the next are bounded between a maximum growth rate and a maximum mortality rate), and the other losses* (Lindstrøm et al., 2017).
Several constraints* can be included to represent the trophic network. Imports, often parameterized in LIM, can be added as a constant or bounded value, proportional or not to the biomass of the group. This is particularly useful if the user wants to represent the primary production. It is, however, possible to do like Lindstrøm et al. (2017) and represent the primary producers as constant net imports (see Drouineau et al. (2021) for the different ways to implement the constraints*). According to the type of ecosystem represented, the consumption of detritus can be significant and represents a substantial part of the energy source. In these conditions, the simplest way to represent detritus is to include them as trophic imports for the groups concerned, for example, as a ratio of their biomasses. In the following, Q for secondary consumers will thus include the trophic imports when existing.
Starting from a LIM, it is likely that the currency of the trophic network will be organic carbon. Therefore, there are two ways to operate the CaN modeling, either keeping carbon as a currency, which is not originally the case in CaN modeling (i.e., originally in biomass), or transferring the model into wet weight or any other desired currency. If the choice is the latter, all biomasses and flows have thus to be converted using conversion factors (e.g., Brey et al., 2010).
The refuge biomass* can be set to 1% of the initial biomass, as proposed by Planque et al. (2014).
If the model is kept in carbon, which seems the easiest way to proceed, prey digestibility* is set to one for all the trophic groups to mimic the LIM results. In this case, the assimilation efficiency* γ has a similar definition than for LIM and is for each group equal to:
based on the outputs of the LIM. Otherwise, both must be defined from literature in accordance with each other (Lindstrøm et al., 2017).
The satiation* σ is based on the Q/B ratio from the LIM and includes the trophic imports. Keeping this value for a trophic group is equivalent to assuming that this group has the same feeding rate than the one it could achieve under “safe” laboratory conditions. According to Christensen et al. (2005), such an assumption is reasonable at least for marine mammals that will always try to feed to satiation*, while it is rarely the case for fish (Ahrens et al., 2012). Depending on the knowledge on the satiation* of the different groups in a particular ecological context, the Q/B value can be increased by a certain percentage to obtain the satiation* parameter. The satiation* is computed as follows:
where λ is a ratio equal to or greater than 1 defined for each trophic group using literature or expert knowledge.
The inertia* ρ results from the P/B ratio at satiation* obtained from the LIM. Following the implementation of the inertia* in CaN modeling, the inertia* is computed as follows:
where σ is the previously computed satiation* for each trophic group.
The other losses* µ are obtained from the following computation:
Unless the user has insights about the amount of other natural mortalities, the other losses* are considered equal to the respiration flow from LIM. For primary producers, other mortalities are defined as:
where γ is the previously computed assimilation efficiency* (that considers losses to sediment organic matter in LIM), and I refers to the import flow.
The exports of trophic groups are also a common output from the LIM. They correspond to emigration but also to fish/harvest when occurring in a given ecosystem. Like imports, exports can be added as explicit constraints* for the CaN projections according to the user’s knowledge on the process.
Finally, constraints* can be described on the diet of the trophic groups. These constraints* can be added on trophic links as presented by Drouineau et al. (2021) for explicit structural constraints*, e.g., when a prey is always more abundant than another one in the diet of a predator. However, the use of constraints* on the diet has to be done carefully, because too many constraints* could lead to not consider the potential variation of diets among trophic groups in the projections, especially in new configurations such as the stress* state(s). On the other side, no constraints* could represent an underuse of the knowledge on the system and make the CaN model explore implausible situations. The choices of the thresholds to use thus have to be made by the user. One possibility is to add a constraint* only when the proportions of two preys in the diet of a predator differ by a fixed magnitude, e.g., when a prey is always 10 times more abundant than another in a diet. In addition, it can also be relevant to define a constraint* on the minimum predation undergone by a defined group.
Arrow 6 in Figure 1. According to the data availability on the biomass-based trophic network and the ecological question raised for the experimental study, partial or full time series, e.g., biomass from surveys, catches, primary production, etc. (see Drouineau et al., 2021 for examples of additional explicit constraints*), can be added to the CaN model to represent a given period. However, for the moment, CaN modeling does not allow users to change the values of inertia*, satiation*, and other losses* during a simulation. Therefore, it is not currently possible to design a simulation plan considering a time series for the stress* condition(s), except if these conditions already happened on a different time series than the one used for control condition, which is rarely the case. A solution is to start both control and stress* condition(s) with the same initial biomasses (see arrow 5 and Section 2.3.1). The model is then run for a given number of years free of time series constraints, i.e., close to the Non-Deterministic Network Dynamics (NDND) framework if considering only the biomasses (Planque et al., 2014; Lindstrøm et al., 2017), using potential additional permanent constraints* on the diets and/or import and export flows. In this case, time series can also be a way to calibrate* (i.e., adjust the parameters of a model by integrating the uncertainty of the parameters and/or the model to obtain a representation of the modeled system that satisfies a predefined criterion) the model with the control condition if the stability of the system is targeted, i.e., by modifying the ratios used to link consumption and satiation* and/or the thresholds of diet constraints*.
If the user does not use the values obtained from the LIM, it is still possible to use the empirical relationships given by Lindstrøm et al. (2017) to derive the values of inertia*, satiation*, and other losses* for every trophic group.
Arrow 7 in Figure 1. As previously demonstrated, LIM outputs can be used to obtain a range of values of parameters (assimilation efficiency*, satiation*, inertia*, other losses*) for all the trophic groups in the control condition. The projection of stress* condition(s) for the focus species or trophic group is then input with perturbations of these same parameters quantified from DEB outputs.
The relative variations of Q, P, and R in the stress* state(s) compared to the control state are used to proportionally modify the assimilation efficiency*, satiation*, inertia*, and other losses*. The other values remain the same, including potential constraints* on diets. Like previously exposed in Section 2.2.1, the modification is justified by the analogy between the individual variations and the ones undergone by the group. Therefore, any modification of Q, P, and R are expected to affect all individuals of the group with the same magnitude. Like described in Section 2.2.1, this magnitude is obtained by weighting the values predicted by the DEB model depending on the demography of the species and/or the species composing the trophic group. The assimilation efficiency*, satiation*, inertia*, and other losses* for a stress* condition ψ are defined as:
where , , and are obtained from the DEB model representing the stress* condition with the computations described in Section 2.2.1 (Equations 3–7) and 2.3.1 (Equations 11–15). If a secondary consumer is subject to trophic import, the constraint* can be modified accordingly.
The change in these three constraints* is supposed to modify the trajectories observed on projections performed with the CaN model. Depending on the trophic level of the group(s) modified following the methodology, cascading effects can be observed on the other compartments of the food web.
Other meta-parameters must be defined in the “sampleCaN” function of the RCaN package. For more details, see the work of Drouineau et al. (2021). It is noteworthy that the choice of the settings will greatly influence the time of sampling of the polytope* (i.e., a finite region of n-dimensional space bounded by hyperplanes), which could be limiting if multiple outputs of the LIM are used as input for the CaN modeling.
The presented framework is finally able to provide a comparison of the trophic network between the control and stress* conditions. The simulations can last for the number of years specified by the user with different purposes, e.g., to find stable states of the stress* state(s) or just to look at the trends of several parameters (Table 1).
Table 1. Potential outputs generated throughout the framework from experiments, DEB, LIM, and CaN modeling used directly within or outside of it.
Different indicators can be observed, like the biomasses of the different groups, the diet contributions or predation pressures, or even trophic network indicators (ENAs, Fath et al., 2019), etc. Note that producing ENAs would require balancing* yearly the network by changing as little as possible these values. Such automatic balancing* can be done with the “balance” function of the enaR package in the R environment (Lau et al., 2017) that uses the algorithm developed by Allesina and Bondavalli (2003). For examples of outputs, see e.g., the work of Drouineau et al. (2021) and Sivel et al. (2021).
Despite these final outcomes, several outputs of interest are also provided throughout the framework at different scales (Table 1). Experimental results, if executed for the purpose of the study, can provide outcomes out of the scope of the framework, e.g., concerning behavioral aspects not represented in DEB, LIM, nor CaN. In the same way, DEB outputs can be numerous and predict the life cycle of individuals through growth, maturation or reproduction features. Finally, LIM outputs are limited in comparison as they are only available for a control condition but can still provide reference states for multiple flows and ENAs on control condition.
In this study, we provide a new methodology aiming at upscaling information from laboratory experiments on individuals to food webs. This framework brings new insights on the potential of understanding higher-scale consequences of the effect of stressors measured at the sub-individual scale. This original approach extends past works on this topic, tackles a portion of uncertainty, but still remains constrained by some limitations.
Our methodology does not imply newly developed techniques or models, but mainly clarifies the usefulness of connecting different models and the ways to link them to address the question of the effects of environmental stresses* observed at the individual level on the food-web functioning.
Forbes et al. (2008) defined three broad classes of population models designed to mechanistically “relate individual-level responses to changes in population size and structure,” i.e., demographic models, energy budget models, and IBMs* (i.e., models in which each individual has a set of state variables or attributes and behavior). Each of them has advantages and disadvantages in their use for upscaling in terms of their generality, realism, and accuracy. Among them, IBMs* are maybe the most natural and has proven to be an efficient tool for the transfer from individual through population (DeAngelis and Grimm, 2014; Grimm et al., 2017), especially when associated with DEB models (Martin et al., 2012). Here, we propose something different from the IBM framework. Our orientation on steady mass-balanced* trophic models, such as LIM and CaN, was partly driven by the purpose to describe the full food web or ecosystem and not only a portion of it through the population. This choice reduced the potential of observing some emergent patterns likely to happen as when using IBMs*. Hybrid models mixing both IBM* and simplified food webs (e.g., Strauss et al., 2017; Morell et al., 2023) have already been implemented in some specific cases. However, our approach allowed the simulation of a larger potential of bottom–up and top–down dynamics with an explicit representation of the lowest and highest trophic levels.
The methodology for transfer across biological organization levels has, of course, to be chosen according to available data and models. In several past studies, the interest and techniques to perform general upscaling were described, and our methodology matches these past classifications by covering an important part of their diversity.
Carlotti and Poggiale (2010) described this transfer through the following two different techniques: “lumping” and replication. Contrary to replication, involving the multiplication of individual entities as in IBMs*, “lumping” is the simple use of characteristics from one organizational level, often the individual, to the upper organization level, e.g., the population, involving the use of an average individual. In our framework, this technique is used to represent the trophic group starting from the individual organism modeled with the DEB theory, by simply applying the same vital rates to the whole group of individuals. However, the diversity of ages or stages of individuals can be represented in the group using DEB outputs, which provides more than what is often realized in trophic modeling. Moreover, DEB modeling provides more flexibility and a deeper comprehension than empirical relationships to mimic stresses*, as it relies on experimental data finely representative of the species of interest and provides a mechanistic interpretation for the MoA of the stressor.
Using a terminology initially developed for IBM*, Reuter et al. (2005) described the properties of upscaling in qualitative terms as follows: (1) Collective properties, attributed equally to different organizational levels of the system, e.g., the scale up of energy fluxes from individual level to communities by Barneche et al. (2014). These are used in our framework when the biomasses of several individuals modeled with the DEB theory are summed to generate the initial trophic group on the food-web models. (2) Emergent properties* result from the activities of lower-level entities on a higher organizational level while not being present on the lower level. No aggregational emergent properties* (i.e., statistical properties that do not necessarily result from interactions of lower-level entities or non-linear feedback processes) are put forward in our framework, partly because our framework is based on models not spatially explicit, while connective emergent properties* (properties that necessarily depend on interactions and feedback processes between the represented organisms and between these organisms and their environment) among entities can be attributed to two main parts of our methodology. The first one is responsible for the relationships between the components of an organism’s metabolism obtained from the sub-individual experimental results and represented with the DEB modeling. The second one is the trophic control driving the final dynamic food web in comparison to the individual level from the DEB model.
Our methodology thus incorporates a suit of methods for upscaling through the transfer of information at the upper organizational level using intuitive and relevant hypothesis to link each node with its upper hierarchical level (Figures 2, 3; see also Supplementary Tables S4, S6 for equivalences between parameters of DEB, LIM, and CaN) and in fine to reach the dynamic food-web level while tackling uncertainty.
Here, we propose a set of models (DEB, LIM, and CaN), but the purpose of our methodology was not to be exhaustive in existing models and their ability to address ecological questions. Alternative models could be used given that formulation of processes and baseline assumptions are consistent between approaches. Otherwise, changes in the type of model used would require adaptations of the methodology that are out of the scope of this study (see, for instance, a comparison between the DEB theory and the biogeochemical model ERSEM of Marques et al., 2014). For example, it is not mandatory to stay within the LIM framework. Many Ecopath models are published, available, and provide similar outputs compared with LIM models. However, most often, they only provide the equivalent of one version of the network obtained from the LIM, except if uncertainty is accounted for (e.g., Guesnet et al., 2015; Steenbeek et al., 2018). But even in this case, the exploration of possible trophic network structure is limited in comparison to LIM due to the limited set of parameters that can be perturbed. Similarly, Ecosim (Walters et al., 1997) could theoretically be used for modeling food-web dynamics. However, contrary to RCaN, its trophic interactions are deterministic, and modifying vital rates and exploring uncertainty rates is not as straightforward as in RCaN, but a diversity of practices exists (see Stock et al., 2023 for a review). Using the EwE suite instead of our framework would most likely result in important biases on the simulation of the extent of possible impacts. Population level could also be added before the trophic network level to avoid our assumptions on demography using IBM or matrix population models (e.g., Klanjscek et al., 2006; Saraiva et al., 2014). It could be necessary to develop it if there is an important effect requiring it, e.g., migrations depending on the levels of maturity that could modify the demography of the population and/or its density.
Beyond the construction of a way to perform upscaling, we tried to consider uncertainty among the components of the framework. This development is crucial especially when inputs are of low quality but also because of the large number of components and processes involved in aquatic ecosystems that may modify the responses of individuals between experimental conditions and between the lab and the field (Planque, 2016). One good way to deal with uncertainty is to build a framework that includes the uncertainty from the beginning of the study and that is shared by all scientific partners throughout the process (Refsgaard et al., 2007).
The terminology of Walker et al. (2003) defines uncertainty according to its location, level, and nature. First, the different locations of uncertainty are partially tackled by our framework. The “context” uncertainty belongs to the user and cannot be optimized in the context of this paper, while “model” uncertainty is not explored with the use of only one set of models at each level. However, “input” and “parameter” uncertainties are reduced by the DEB if uncertainty is considered with the methodology of Boersch-Supan et al (2017); Boersch-Supan and Johnson (2019), or artificially by adding an equivalent of the Ecopath pedigree* value around the DEB predictions, and are inherent to the LIM and CaN approaches. “Model outcome” uncertainty (i.e., the accumulated uncertainty associated with the model outcomes) is captured within the thresholds of the final CaN uncertainty. Second, the level of uncertainty is explicitly described in the different models by the constraints* given that traduce the knowledge of the user. Finally, the nature of uncertainty is divided between epistemic uncertainty, mostly in the DEB and LIM, and stochastic uncertainty, traduced in the trophic relationships of the CaN modeling.
As evoked above, modeling an average individual in the DEB process can be problematic, and uncertainty approach has to be advocated (Boersch-Supan and Johnson, 2019). This is not only to represent the variability of individuals, but it is also crucial for the understanding of populations’ resilience that can vary in relation to the pool of individual traits, e.g., regarding global change (McKenzie et al., 2016). The DEB theory focuses on processes at an intermediate individual scale (Sousa et al., 2010). It assumes the choice of ignoring the molecular understanding of processes, such as those concerning the effect of pollution on the immune system. However, it captures the effects reflected on the energy transfer through large directions of the individual’s life cycle, growth, and reproduction principally. The response of individuals and the repercussions of individuals’ plasticity are one of the pros of our approach, which, however, does not fully capture the allometry influences on the food web, e.g., the influence of temperature on size and mass of individuals potentially influencing their diet and consequently ecosystem structure (Freitas et al., 2010; Palkovacs, 2011).
Our framework advocates for the use of laboratory experiments as basal inputs in association with the necessity to use numerical models to open for a larger scale. Small-scale aquariums are well adapted for individual levels, while obviously less useful for populations and communities, for which mesocosms permit more insights. Mesocosms can include multiple trophic levels at the same place and time, but they often lack the ability to represent networks with sufficient interacting groups under various conditions (Queirós et al., 2015). These ones are more challenging, costly, and finally lack predictive power (Forbes et al., 2008; Andersson et al., 2015; Queirós et al., 2015). Moreover, when experimental results are used as inputs for upscaling, these must be carefully treated to provide realistic projections. A classic example lies in the differences between the functional responses obtained from laboratory experiments and in situ observations (Morozov, 2010), which imply the use of well-defined upscaling methodologies (Carlotti and Poggiale, 2010). With a “lumping” aggregation approach from DEB, it may sometimes be possible to directly relate experimental observations to model parameters, while in other cases, links will be less clear (Le Quesne and Pinnegar, 2012). Models are known to be less structurally sensitive to the choice of a specific functional response if they include mass-balance* resource dynamics and individual maintenance (Aldebert et al., 2018). Using models like DEB could therefore be appropriate to at least reduce the uncertainty on such biological processes.
The DEB modeling used here is mostly adult focused because the methodology presented is based on mass-balanced* modeling, so the length of individuals is not explicit, unless multiple life stanza are defined or if the trophic network is only frequented by a precise stage of a species. If so, it could require an extensive focus on younger stages. If only a precise stage of a species is represented, a stronger focus has probably to be done on the hypothesis concerning the population effects and the imports and exports, which would have repercussions on the way constraints* are written, e.g., the proportionality of exports and imports.
The association of modelers and experimentalists is therefore globally required in aquatic sciences (Andersson et al., 2015; Koenigstein et al., 2016; McKenzie et al., 2016), especially when trying to develop a framework like the one presented here. The integration of physiology into models is needed to answer complex questions involving repercussions at different biological scales (McKenzie et al., 2016) involving multiple pathways by which stresses* can affect species (Stock et al., 2023). A paramount factor influencing a nonlinear transferability from experiments to in situ is the behavior of individuals in association with physiology (Pörtner and Peck, 2010; Cooke et al., 2014). Avoidance of areas or predators, ability to feed on preferential prey, or changes in the mono- or multi-specific movement may modify the values associated with consumption, production, or respiration in comparison with simple physiological results from experiments. This issue is not explicit in DEB representations but can be circumvented by bringing qualitative information concerning the variation of other components at the level of the trophic group (e.g., mortality by predation, predation abilities, etc.). These observations can complete the quantitative scenarios defined from the DEB model outputs with “qualitative” scenarios. This can be concretely realized by the increase or decrease of the different ratios. Qualitative scenarios have to be defined by the user knowing the effects different treatments can have on a species’ behavior or vulnerability involving a consensus between all specialists involved in the ecological questioning.
In this study, we proposed a framework designed to address an ecological question involving upscaling from individual to food web. The connection of experiments to a series of models is innovative as it permits to consider the effect of any stress* on individuals, focusing on a species or a trophic group, and spread them for a dynamic projection of aquatic food webs, while dealing with a non-negligible portion of uncertainty. This study does not have the pretention to give the one and only approach to follow to understand the effects of environmental factor from the individual level to the trophic web level, but proposes a framework of how to answer such ecological question in an aquatic system context. It can help experimenters and modelers to jointly address a specific question, proposes approaches, and gives tips on the pitfalls to avoid along the upscaling process.
The original contributions presented in the study are included in the article/Supplementary Material. Further inquiries can be directed to the corresponding author.
PB: Conceptualization, Methodology, Writing – original draft. NN: Conceptualization, Methodology, Writing – original draft, Supervision. EA: Methodology, Writing – review & editing. HC: Methodology, Writing – review & editing. AC: Methodology, Writing – review & editing. HD: Methodology, Writing – review & editing. JL: Methodology, Writing – review & editing. LP: Methodology, Writing – review & editing. BS-B: Methodology, Writing – review & editing. GL: Conceptualization, Methodology, Writing – original draft, Supervision. MV: Conceptualization, Methodology, Writing – original draft, Supervision.
The author(s) declare financial support was received for the research, authorship, and/or publication of this article. This work has been funded by the MEILH Project SAD volet 2, granted by the ‘Région Bretagne’, the INRAE institute, ‘Connétable-Chancerelle’ and the ‘Union des Fédérations départementales des associations agréées pour la pêche et la protection du milieu aquatique du Bassin Loire-Bretagne (UFBLB)’.
We thank Sébastien Hervé for the development of the main figure constituting this manuscript. We also thank Lucien Besnard, Laure Carassou, Luuk van der Heijden, Benoît Lebreton, François Le Loc’h and Florence Mounier for all the fruitful discussions that helped during the development of the methodology. Finally, we wish to thank the two anonymous reviewers and editor for helping us greatly improve the manuscript.
The authors declare that the research was conducted in the absence of any commercial or financial relationships that could be construed as a potential conflict of interest.
The author(s) declared that they were an editorial board member of Frontiers, at the time of submission. This had no impact on the peer review process and the final decision.
All claims expressed in this article are solely those of the authors and do not necessarily represent those of their affiliated organizations, or those of the publisher, the editors and the reviewers. Any product that may be evaluated in this article, or claim that may be made by its manufacturer, is not guaranteed or endorsed by the publisher.
The Supplementary Material for this article can be found online at: https://www.frontiersin.org/articles/10.3389/fevo.2024.1400936/full#supplementary-material
Ahrens R. N. M., Walters C. J., Christensen V. (2012). Foraging arena theory. Fish Fisheries 13, 41–59. doi: 10.1111/j.1467-2979.2011.00432.x
Aldebert C., Kooi B. W., Nerini D., Poggiale J.-C. (2018). Is structural sensitivity a problem of oversimplified biological models? Insights from nested Dynamic Energy Budget models. J. Theor. Biol. 448, 1–8. doi: 10.1016/j.jtbi.2018.03.019
Allesina S., Bondavalli C. (2003). Steady state of ecosystem flow networks: a comparison between balancing procedures. Ecol. Model. 165, 221–229. doi: 10.1016/S0304-3800(03)00075-9
AmPtool (2023). Software Package AmPtool. Available online at: http://www.github.com/add-my-pet/AmPtool/ (accessed July, 2023).
Andersson A., Kline D., Edmunds P., Archer S., Bednaršek N., Carpenter R., et al. (2015). Understanding ocean acidification impacts on organismal to ecological scales. Oceanography 25, 16–27. doi: 10.5670/oceanog.2015.27
Barneche D. R., Kulbicki M., FLoeter S. R., Friedlander A. M., Maina J., Allen A. P. (2014). Scaling metabolism from individuals to reef-fish communities at broad spatial scales. Ecol. Lett. 17, 1067–1076. doi: 10.1111/ele.12309
Beaudouin R., Goussen B., Piccini B., Augustine S., Devillers J., Brion F., et al. (2015). An individual-based model of zebrafish population dynamics accounting for energy dynamics. PLoS One 10, e0125841. doi: 10.1371/journal.pone.0125841
Boersch-Supan P. H., Johnson L. R. (2019). Two case studies detailing Bayesian parameter inference for dynamic energy budget models. J. Sea Res. 143, 57–69. doi: 10.1016/j.seares.2018.07.014
Boersch-Supan P. H., Ryan S. J., Johnson L. R. (2017). deBInfer: Bayesian inference for dynamical models of biological systems in R. Methods Ecol. Evol. 8, 511–518. doi: 10.1111/2041-210X.12679
Brey T., Müller-Wiegmann C., Zittier Z. M. C., Hagen W. (2010). Body composition in aquatic organisms - A global data bank of relationships between mass, elemental composition and energy content. J. Sea Res. 64, 334–340. doi: 10.1016/j.seares.2010.05.002
Carlotti F., Poggiale J.-C.. (2010). Towards methodological approaches to implement the zooplankton component in “end to end” food-web models. Progr. Oceanogr. 84, 20–38. doi: 10.1016/j.pocean.2009.09.003
Chaalali A., Saint-Béat B., Lassalle G., Le Loc’h F., Tecchio S., Safi G., et al. (2015). A new modeling approach to define marine ecosystems food-web status with uncertainty assessment. Prog. Oceanography 135, 37–47. doi: 10.1016/j.pocean.2015.03.012
Christensen V., Pauly D. (1992). Ecopath II - a software for balancing steady-state ecosystem models and calculating network characteristics. Ecol. Model. 61, 169–185. doi: 10.1016/0304-3800(92)90016-8
Christensen V., Walters C. J., Pauly D. (2005). Ecopath With Ecosim: a User’s Guide (Vancouver: Fisheries Centre, University of British Columbia), 154 p. Available at: www.ecopath.org. November 2005 edition.
Claireaux G., Lefrançois C. (2007). Linking environmental variability and fish performance: integration through the concept of scope for activity. Phil. Trans. R. Soc B 362, 2031–2041. doi: 10.1098/rstb.2007.2099
Coll M., Akoglu E., Arreguín-Sánchez F., Fulton E. A., Gascuel D., Heymans J. J., et al. (2015). Modelling dynamic ecosystems: venturing beyond boundaries with the ecopath approach. Rev. Fish Biol. Fisheries 25, 413−24. doi: 10.1007/s11160-015-9386-x
Colléter M., Valls A., Guitton J., Gascuel D., Pauly D., Christensen V. (2015). Global overview of the applications of the Ecopath with Ecosim modeling approach using the EcoBase models repository. Ecol. Model. 302, 42–53. doi: 10.1016/j.ecolmodel.2015.01.025
Cooke S. J., Blumstein D. T., Buchholz R., Caro T., Fernández-Juricic E., Franklin C. E., et al. (2014). Physiology, behavior, and conservation. Physiol. Biochem. Zoology 87, 1–14. doi: 10.1086/671165
Crowder L. B., Hazen E. L., Avissar N., Bjorkland R., Latanich C., Ogburn M. B. (2008). The impacts of fisheries on marine ecosystems and the transition to ecosystem-based management. Annu. Rev. Ecol. Evol. Syst. 39, 259–278. doi: 10.1146/annurev.ecolsys.39.110707.173406
DeAngelis D. L., Grimm V. (2014). Individual-based models in ecology after four decades. F1000Prime Rep. 6. doi: 10.12703/P6-39
DEBtool (2023). Software Package DEBtool. Available online at: http://www.github.com/add-my-pet/DEBtool_M/ (accessed July, 2023).
deYoung B., Heath M., Werner F., Chai F., Megrey B., Monfray P. (2004). Challenges of modeling ocean basin ecosystems. Science 304, 1463–1466. doi: 10.1126/science.1094858
Drouineau H., Planque B., Mullon C. (2021). RCaN : a software for Chance and Necessity modelling. Ecology. doi: 10.1101/2021.06.09.447734
Drouineau H., Planque B., Mullon C. (2023). RCaNmodel: an R package for chance and necessity modelling. J. Open Source Softw. 8, 4955 doi: 10.21105/joss.04955
Fath B. D., Asmus H., Asmus R., Baird D., Borrett S. R., de Jonge V. N., et al. (2019). Ecological network analysis metrics: The need for an entire ecosystem approach in management and policy. Ocean Coast. Manage. 174, 1–14. doi: 10.1016/j.ocecoaman.2019.03.007
Forbes V. E., Calow P., Sibly R. M. (2008). The extrapolation problem and how population modeling can help. Environ. Toxicol. Chem. 27, 1987. doi: 10.1897/08-029.1
Freitas V., Cardoso J. F. M. F., Lika K., Peck M. A., Campos J., Kooijman S. A. L. M., et al. (2010). Temperature tolerance and energetics: a dynamic energy budget-based comparison of North Atlantic marine species. Phil. Trans. R. Soc B 365, 3553–3565. doi: 10.1098/rstb.2010.0049
Froese R., Pauly D. (Eds.) (2000). FishBase 2000: concepts, design and data sources (Los Baños, Laguna, Philippines: ICLARM), 344 p.
Gallardo B., Clavero M., Sánchez M. I., Vilà M. (2016). Global ecological impacts of invasive species in aquatic ecosystems. Glob Change Biol. 22, 151–163. doi: 10.1111/gcb.13004
Geist J., Hawkins S. J. (2016). Habitat recovery and restoration in aquatic ecosystems: current progress and future challenges: Aquatic restoration. Aquat. Conserv: Mar. Freshw. Ecosyst. 26, 942–962. doi: 10.1002/aqc.2702
Grangeré K., Lefebvre S., Bacher C., Cugier P., Ménesguen A. (2010). Modelling the spatial heterogeneity of ecological processes in an intertidal estuarine bay: dynamic interactions between bivalves and phytoplankton. Mar. Ecol. Prog. Ser. 415, 141–158. doi: 10.3354/meps08659
Gregr E. J., Chan K. M. A. (2015). Leaps of faith: how implicit assumptions compromise the utility of ecosystem models for decision-making. BioScience 65, 43–54. doi: 10.1093/biosci/biu185
Grimm V., Ayllón D., Railsback S. F. (2017). Next-generation individual-based models integrate biodiversity and ecosystems: yes we can, and yes we must. Ecosystems 20, 229–236. doi: 10.1007/s10021-016-0071-2
Guesnet V., Lassalle G., Chaalali A., Kearney K., Saint-Béat B., Karimi B., et al. (2015). Incorporating food-web parameter uncertainty into Ecopath-derived ecological network indicators. Ecol. Model. 313, 29–40. doi: 10.1016/j.ecolmodel.2015.05.036
Häder D.-P., Banaszak A. T., Villafañe V. E., Narvarte M. A., González R. A., Helbling E. W. (2020). Anthropogenic pollution of aquatic ecosystems: Emerging problems with global implications. Sci. Total Environ. 713, 136586. doi: 10.1016/j.scitotenv.2020.136586
Haraldsson M., Gerphagnon M., Bazin P., Colombet J., Tecchio S., Sime-Ngando T., et al. (2018). Microbial parasites make cyanobacteria blooms less of a trophic dead end than commonly assumed. ISME J. 12, 1008–1020. doi: 10.1038/s41396-018-0045-9
IPCC (2019). “Summary for policymakers,” in IPCC Special Report on the Ocean and Cryosphere in a Changing Climate. Eds. Pörtner H.-O., Roberts D. C., Masson-Delmotte V., Zhai P., Tignor M., Poloczanska E., Mintenbeck K., Alegría A., Nicolai M., Okem A., Petzold J., Rama B., Weyer N. M. (Cambridge University Press, Cambridge, UK and New York, NY, USA), 3–35. doi: 10.1017/9781009157964.001
IPCC (2022). “Summary for policymakers,” in Climate change 2022: Impacts, adaptation, and vulnerability: contribution of working group II to the sixth assessment report of the intergovernmental panel on climate change. Eds. Pörtner H.-O., Roberts D. C., Poloczanska E. S., Mintenbeck K., Tignor M., Alegría A., et al. (Cambridge, UK and New York, NY, US: Cambridge University Press), pp. 3–33.
Islam S., Tanaka M. (2004). Impacts of pollution on coastal and marine ecosystems including coastal and marine fisheries and approach for management: a review and synthesis. Mar. pollut. Bull. 48, 624–649. doi: 10.1016/j.marpolbul.2003.12.004
Johnson L. R., Pecquerie L., Nisbet R. M. (2013). Bayesian inference for bioenergetic models. Ecology 94, 882–984. doi: 10.1890/12-0650.1
Klanjscek T., Caswell H., Neubert M. G., Nisbet R. M. (2006). Integrating dynamic energy budgets into matrix population models. Ecol. Model. 196, 407–420. doi: 10.1016/j.ecolmodel.2006.02.023
Koch J., De Schamphelaere K. A. C. (2020). Estimating inter-individual variability of dynamic energy budget model parameters for the copepod Nitocra spinipes from existing life-history data. Ecol. Model. 431, 109091. doi: 10.1016/j.ecolmodel.2020.109091
Koenigstein S., Mark F. C., Gößling-Reisemann S., Reuter H., Poertner H.-O. (2016). Modelling climate change impacts on marine fish populations: process-based integration of ocean warming, acidification and other environmental drivers. Fish Fish 17, 972–1004. doi: 10.1111/faf.12155
Kones J. K., Soetaert K., van Oevelen D., Owino J. O. (2009). Are network indices robust indicators of food web functioning? A Monte Carlo approach. Ecol. Model. 220, 370–382. doi: 10.1016/j.ecolmodel.2008.10.012
Kooijman S. A. L. M. (2010). Dynamic Energy Budget Theory for Metabolic Organization, 3rd edn. Cambridge, UK: Cambridge University Press. doi: 10.1017/CBO9780511805400
Kooijman S. A. L. M. (2014). Metabolic acceleration in animal ontogeny: An evolutionary perspective. J. Sea Res. 94, 128–137. doi: 10.1016/j.seares.2014.06.005
Lau M. K., Borrett S. R., Hines D. E., Singh P. (2017). Package ‘ enaR .’ Tools for Ecological Network Analysis. Available at: http://cran.nexr.com/web/packages/enaR/enaR.pdf.
Lennox R. J., Crook D. A., Moyle P. B., Struthers D. P., Cooke S. J. (2019). Toward a better understanding of freshwater fish responses to an increasingly drought-stricken world. Rev. Fish Biol. Fisheries 29, 71–92. doi: 10.1007/s11160-018-09545-9
Le Quesne W. J. F., Pinnegar J. K. (2012). The potential impacts of ocean acidification: scaling from physiology to fisheries*: Potential acidification impacts on fisheries. Fish Fisheries 13, 333–344. doi: 10.1111/j.1467-2979.2011.00423.x
Lindstrøm U., Planque B., Subbey S. (2017). Multiple patterns of food web dynamics revealed by a minimal non-deterministic model. Ecosystems 20, 163–182. doi: 10.1007/s10021-016-0022-y
Link J. S. (2010). Adding rigor to ecological network models by evaluating a set of pre-balance diagnostics: A plea for PREBAL. Ecol. Model. 221, 1580−91. doi: 10.1016/j.ecolmodel.2010.03.012
Marques G. M., Augustine S., Lika K., Pecquerie L., Domingos T., Kooijman S. A. L. M. (2018). The AmP project: Comparing species on the basis of dynamic energy budget parameters. PLoS Comput. Biol. 14, e1006100. doi: 10.1371/journal.pcbi.1006100
Marques G. M., Lika K., Augustine S., Pecquerie L., Kooijman S. A. L. M. (2019). Fitting multiple models to multiple data sets. J. Sea Res. 143, 48–56. doi: 10.1016/j.seares.2018.07.004
Marques G. M., Mateus M., Domingos T. (2014). Can we reach consensus between marine ecological models and DEB theory? A look at primary producers. J. Sea Res. 13, 1385–1101. doi: 10.1016/j.seares.2014.09.007
Martin B. T., Zimmer E. I., Grimm V., Jager T. (2012). Dynamic Energy Budget theory meets individual-based modelling: a generic and accessible implementation: DEB theory in an IBM context. Methods Ecol. Evol. 3, 445–449. doi: 10.1111/j.2041-210X.2011.00168.x
Maury O., Poggiale J.-C. (2013). From individuals to populations to communities: A dynamic energy budget model of marine ecosystem size-spectrum including life history diversity. J. Theor. Biol. 324, 52–71. doi: 10.1016/j.jtbi.2013.01.018
McKenzie D. J., Axelsson M., Chabot D., Claireaux G., Cooke S. J., Corner R. A., et al. (2016). Conservation physiology of marine fishes: state of the art and prospects for policy. Conserv. Physiol. 4, cow046. doi: 10.1093/conphys/cow046
Molnar J. L., Gamboa R. L., Revenga C., Spalding M. D. (2008). Assessing the global threat of invasive species to marine biodiversity. Front. Ecol. Environ. 6, 485–492. doi: 10.1890/070064
Morell A., Shin Y. J., Barrier N., Travers-Trolet M., Halouani G., Ernande B. (2023). Bioen-OSMOSE: a bioenergetic marine ecosystem model with physiological response to temperature and oxygen. Prog. Oceanogr. 216, 103064.
Morozov A. Y. (2010). Emergence of Holling type III zooplankton functional response: Bringing together field evidence and mathematical modelling. J. Theor. Biol. 265, 45–54. doi: 10.1016/j.jtbi.2010.04.016
Mounier F., Loizeau V., Pecquerie L., Drouineau H., Labadie P., Budzinski H., et al. (2020a). Dietary bioaccumulation of persistent organic pollutants in the common sole Solea solea in the context of global change. Part 2: Sensitivity of juvenile growth and contamination to toxicokinetic parameters uncertainty and environmental conditions variability in estuaries. Ecol. Model. 431, 109196. doi: 10.1016/j.ecolmodel.2020.109196
Mounier F., Pecquerie L., Lobry J., Sardi A. E., Labadie P., Budzinski H., et al. (2020b). Dietary bioaccumulation of persistent organic pollutants in the common sole Solea solea in the context of global change. Part 1: Revisiting parameterisation and calibration of a DEB model to consider inter-individual variability in experimental and natural conditions. Ecol. Model. 433, 109224. doi: 10.1016/j.ecolmodel.2020.109224
Mullon C., Fréon P., Cury P., Shannon L., Roy C. (2009). A minimal model of the variability of marine ecosystems. Fish Fisheries 10, 115–131. doi: 10.1111/j.1467-2979.2008.00296.x
Niquil N., Saint-Béat B., Johnson G. A., Soetaert K., van Oevelen D., Bacher C., et al. (2011). “Inverse modeling in modern ecology and application to coastal ecosystems,” in Treatise on Estuarine and Coastal Science. eds. Wolanski E., McLusky D. S. (Wal-tham, MA: Academic Press), 115–133. doi: 10.1016/B978-0-12-374711-2.00906-2
Nisbet R. M., Jusup M., Klanjscek T., Pecquerie L. (2012). Integrating dynamic energy budget (DEB) theory with traditional bioenergetic models. J. Exp. Biol. 215, 1246–1246. doi: 10.1242/jeb.071845
Nisbet R. M., Muller E. B., Lika K., Kooijman A. L. M. (2000). From molecules to ecosystems through dynamic energy budget models. J. Anim. Ecol. 69, 913–926. doi: 10.1111/j.1365-2656.2000.00448.x
Nogues Q., Raoux A., Araignous E., Chaalali A., Hattab T., Leroy B., et al. (2021). Cumulative effects of marine renewable energy and climate change on ecosystem properties: Sensitivity of ecological network analysis. Ecol. Indic. 121, 107128. doi: 10.1016/j.ecolind.2020.107128
Palkovacs E. P. (2011). The overfishing debate: an eco-evolutionary perspective. Trends Ecol. Evol. 26, 616–617. doi: 10.1016/j.tree.2011.08.004
Pauly D., Watson R., Alder J. (2005). Global trends in world fisheries: impacts on marine ecosystems and food security. Phil. Trans. R. Soc B 360, 5–12. doi: 10.1098/rstb.2004.1574
Plagányi E. E. (2007). Models for an ecosystem approach to fisheries (Rome: FAO), 108p. FAO Gisheries Technical Paper. No. 477.
Planque B. (2016). Projecting the future state of marine ecosystems, “la grande illusion”? ICES J. Mar. Sci. 73, 204–208. doi: 10.1093/icesjms/fsv155
Planque B., Lindstrøm U., Subbey S. (2014). Non-deterministic modelling of food-web dynamics. PLoS One 9, e108243. doi: 10.1371/journal.pone.0108243
Planque B., Mullon C. (2020). Modelling chance and necessity in natural systems. ICES J. Mar. Sci. 77, 1573–1588. doi: 10.1093/icesjms/fsz173
Poloczanska E. S., Burrows M. T., Brown C. J., García Molinos J., Halpern B. S., Hoegh-Guldberg O., et al. (2016). Responses of marine organisms to climate change across oceans. Front. Mar. Sci. 3. doi: 10.3389/fmars.2016.00062
Pörtner H. O., Peck M. A. (2010). Climate change effects on fishes and fisheries: towards a cause-and-effect understanding. J. Fish Biol. 77, 1745–1779. doi: 10.1111/j.1095-8649.2010.02783.x
Queirós A. M., Fernandes J. A., Faulwetter S., Nunes J., Rastrick S. P. S., Mieszkowska N., et al. (2015). Scaling up experimental ocean acidification and warming research: from individuals to the ecosystem. Glob Change Biol. 21, 130–143. doi: 10.1111/gcb.12675
Refsgaard J. C., van der Sluijs J. P., Højberg A. L., Vanrolleghem P. A. (2007). Uncertainty in the environmental modelling process – A framework and guidance. Environ. Model. Software 22, 1543–1556. doi: 10.1016/j.envsoft.2007.02.004
Reuter H., Hölker F., Middelhoff U., Jopp F., Eschenbach C., Breckling B. (2005). The concepts of emergent and collective properties in individual-based models—Summary and outlook of the Bornhöved case studies. Ecol. Model. 186, 489–501. doi: 10.1016/j.ecolmodel.2005.02.014
Reuter H., Jopp F., Blanco-Moreno J. M., Damgaard C., Matsinos Y., DeAngelis D. L. (2010). Ecological hierarchies and self-organisation – Pattern analysis, modelling and process integration across scales. Basic Appl. Ecol. 11, 572–581. doi: 10.1016/j.baae.2010.08.002
Rijnsdorp A. D., Peck M. A., Engelhard G. H., Möllmann C., Pinnegar J. K. (2009). Resolving the effect of climate change on fish populations. ICES J. Mar. Sci. 66, 1570–1583. doi: 10.1093/icesjms/fsp056
Robles J. F., Chica M., Filgueira R., Aguera A., Damas S. (2023). MultiCalib4DEB: A toolbox exploiting multimodal optimisation in Dynamic Energy Budget parameters calibration. ArXiv:2301.07548. doi: 10.48550/arXiv.2301.07548
Safi G., Giebels D., Arroyo N. L., Heymans J. J., Preciado I., Raoux A., et al. (2019). Vitamine ENA: A framework for the development of ecosystem-based indicators for decision makers. Ocean Coast. Manage. 174, 116–130. doi: 10.1016/j.ocecoaman.2019.03.005
Saint-Béat B., Baird D., Asmus H., Asmus R., Bacher C., Pacella S. R., et al. (2015). Trophic networks: How do theories link ecosystem structure and functioning to stability properties? A review. Ecol. Indic. 52, 458–471. doi: 10.1016/j.ecolind.2014.12.017
Saint-Béat B., Dupuy C., Agogué H., Carpentier A., Chalumeau J., Como S., et al. (2014). How does the resuspension of the biofilm alter the functioning of the benthos–pelagos coupled food web of a bare mudflat in Marennes-Oléron Bay (NE Atlantic)? J. Sea Res. 92, 144–157. doi: 10.1016/j.seares.2014.02.003
Saint-Béat B., Vézina A. F., Asmus R., Asmus H., Niquil N. (2013). The mean function provides robustness to linear inverse modelling flow estimation in food webs: A comparison of functions derived from statistics and ecological theories. Ecol. Model. 258, 53–64. doi: 10.1016/j.ecolmodel.2013.01.023
Saraiva S., van der Meer J., Kooijman S. A. L. M., Ruardij P. (2014). Bivalves: From individual to population modelling. J. Sea Res. 94, 71–83. doi: 10.1016/j.seares.2014.06.004
Schreck C. B., Tort L. (2016). “The concept of stress in fish,” in Biology of Stress in Fish - Fish Physiology, vol. 35 . Eds. Schreck C. B., Tort L., Farrell A. P., Brauner C. J. (Academic Press, Cambridge, MA), 1–34. doi: 10.1371/journal.pone.0106523
Sivel E., Planque B., Lindstrøm U., Yoccoz N. G. (2021). Multiple configurations and fluctuating trophic control in the Barents Sea food-web. PLoS One 16, e0254015. doi: 10.1371/journal.pone.0254015
Sousa T., Domingos T., Poggiale J.-C., Kooijman S. A. L. M. (2010). Dynamic energy budget theory restores coherence in biology. Phil. Trans. R. Soc B 365, 3413–3428. doi: 10.1098/rstb.2010.0166
Steenbeek J., Corrales X., Platts M., Coll M. (2018). Ecosampler: A new approach to assessing parameter uncertainty in Ecopath with Ecosim. SoftwareX 7, 198–204. doi: 10.1016/j.softx.2018.06.004
Stock A., Murray C. C., Gregr E. J., Steenbeek J., Woodburn E., Micheli F., et al. (2023). Exploring multiple stressor effects with Ecopath, Ecosim, and Ecospace: Research designs, modeling techniques, and future directions. Sci. Total Environ. 869, 161719. doi: 10.1016/j.scitotenv.2023.161719
Strauss T., Gabsi F., Hammers-Wirtz M., Thorbek P., Preuss T. G. (2017). The power of hybrid modelling: An example from aquatic ecosystems. Ecol. Model. 364, 77–88. doi: 10.1016/j.ecolmodel.2017.09.019
Subbey S., Devine J. A., Schaarschmidt U., Nash R. D. M. (2014). Modelling and forecasting stock–recruitment: current and future perspectives. ICES J. Mar. Sci. 71, 2307–2322. doi: 10.1093/icesjms/fsu148
Thomas Y., Pouvreau S., Alunno-Bruscia M., Barillé L., Gohin F., Bryère P., et al. (2016). Global change and climate-driven invasion of the Pacific oyster (Crassostrea gigas ) along European coasts: a bioenergetics modelling approach. J. Biogeogr. 43, 568–579. doi: 10.1111/jbi.12665
Turner S. J., Thrush S. F., Hewitt J. E., Cummings V. J., Funnell G. (1999). Fishing impacts and the degradation or loss of habitat structure: Degradation or loss of habitat structure. Fisheries Manage. Ecol. 6, 401–420. doi: 10.1046/j.1365-2400.1999.00167.x
Ulanowicz R. E. (2003). Some steps toward a central theory of ecosystem dynamics. Comput. Biol. Chem. 27, 523–530. doi: 10.1016/S1476-9271(03)00050-1
Van den Meersche K., Soetaert K., Van Oevelen D. (2009). xsample: an R function for sampling linear inverse problems. J. Stat. Softw. 30. doi: 10.18637/jss.v030.c01
van der Meer J., Klok C., Kearney M. R., Wijsman J. W. M., Kooijman S. A. L. M. (2014). 35 years of DEB research. J. Sea Res. 94, 1–4. doi: 10.1016/j.seares.2014.09.004
Vézina A., Piatt T. (1988). Food web dynamics in the ocean. I. Best-estimates of flow networks using inverse methods. Mar. Ecol. Prog. Ser. 42, 269–287. doi: 10.3354/meps042269
Walker W. E., Harremoës P., Rotmans J., van der Sluijs J. P., van Asselt M. B. A., Janssen P., et al. (2003). Defining uncertainty: A conceptual basis for uncertainty management in model-based decision support. Integrated Assess. 4, 5–17. doi: 10.1076/iaij.4.1.5.16466
Walters C., Christensen V., Pauly D. (1997). Structuring dynamic models of exploited ecosystems from trophic mass-balance assessments. Rev. Fish Biol. Fisheries 7, 139–172. doi: 10.1023/A:1018479526149
Keywords: aquatic food-webs, chance and necessity modelling, ecology, dynamic energy budget, laboratory experiments, linear inverse modelling, upscaling
Citation: Bourdaud P, Niquil N, Araignous E, Cabral H, Carpentier A, Drouineau H, Lobry J, Pecquerie L, Saint-Béat B, Lassalle G and Vagner M (2024) Linking individual experiments and multiscale models to simulate physiological perturbations on aquatic food webs. Front. Ecol. Evol. 12:1400936. doi: 10.3389/fevo.2024.1400936
Received: 20 March 2024; Accepted: 09 September 2024;
Published: 09 October 2024.
Edited by:
Antonio Bodini, University of Parma, ItalyReviewed by:
S. Lan Smith, Japan Agency for Marine-Earth Science and Technology (JAMSTEC), JapanCopyright © 2024 Bourdaud, Niquil, Araignous, Cabral, Carpentier, Drouineau, Lobry, Pecquerie, Saint-Béat, Lassalle and Vagner. This is an open-access article distributed under the terms of the Creative Commons Attribution License (CC BY). The use, distribution or reproduction in other forums is permitted, provided the original author(s) and the copyright owner(s) are credited and that the original publication in this journal is cited, in accordance with accepted academic practice. No use, distribution or reproduction is permitted which does not comply with these terms.
*Correspondence: Pierre Bourdaud, cC5sLmUuYm91cmRhdWRAZ21haWwuY29t; cGllcnJlLmJvdXJkYXVkQGZyYW5jZS1lbmVyZ2llcy1tYXJpbmVzLm9yZw==
†These authors share last authorship
Disclaimer: All claims expressed in this article are solely those of the authors and do not necessarily represent those of their affiliated organizations, or those of the publisher, the editors and the reviewers. Any product that may be evaluated in this article or claim that may be made by its manufacturer is not guaranteed or endorsed by the publisher.
Research integrity at Frontiers
Learn more about the work of our research integrity team to safeguard the quality of each article we publish.