- 1College of Resources and Environmental Sciences, China Agricultural University, Beijing, China
- 2Beijing Key Laboratory of Biodiversity and Organic Farming, China Agricultural University, Beijing, China
- 3Resources and Environment College, Tibet Agriculture and Animal Husbandry University, Nyingchi, China
- 4School of Foreign Studies, Nanchang University, Nanchang, Jiangxi, China
Plant communities are complex systems shaped by a combination of deterministic and stochastic ecological processes. Bryophytes are an essential component of plant diversity in natural ecosystems, yet our understanding of their community ecology needs to catch up to that of other organisms. The unique geological history, alpine climatic conditions, and high habitat heterogeneity of Tibet provide suitable areas for bryophytes to survive in the alpine regions. Therefore, field surveys were conducted across 184 plots in forest, thicket, and herbaceous vegetation of Tibet to investigate the role of deterministic processes such as biological interactions and abiotic effects, along with stochastic processes, in shaping the distribution of bryophyte assemblages. We employed various analytical methods, including mixed effects models, partial least squares path modeling, null model analysis, and neutral community models. The study showed that bryophyte richness was highest in forests. Bryophyte assemblages showed greater segregation in forest and thicket environments compared to herbaceous vegetation. As the influence of stochastic processes increased, that of deterministic processes decreased from forests through thickets to herbaceous vegetation. Deterministic processes were the main driving forces for the bryophyte assemblage pattern. Soil properties and climatic factors, particularly pH played a key role in determining bryophyte patterns in Tibet. This study has deepened our comprehension of how deterministic and stochastic ecological processes interplay and shape bryophyte distribution patterns in Tibet.
1 Introduction
Deterministic and stochastic processes play crucial roles in describing the mechanisms that shape the distribution and dynamics of species within ecosystems (Ovaskainen et al., 2017). Deterministic processes refer to ecological phenomena that are predictable and driven by consistent, deterministic factors. The abiotic and biotic conditions of an ecosystem, such as climate, competition and predation influence these processes. Deterministic processes assume that species’ presence and abundance are primarily governed by their adaptation to specific environmental conditions and their interactions with other species (Ovaskainen et al., 2017). In contrast, stochastic processes describe ecological phenomena that are unpredictable and influenced by random fluctuations or chance events. These processes include factors such as random environmental disturbances, demographic fluctuations, genetic drift, and colonization/extinction dynamics. Stochastic processes acknowledge the inherent uncertainty and variability in ecological systems and recognize that chance events can have significant impacts on species distribution and community dynamics (Maciel et al., 2017). The unified neutral theory of biodiversity and biogeography, which integrates speciation and ecological neutrality into MacArthur and Wilson’s theory, is an extreme example of the stochastic theory (Hubbell, 2011). Both deterministic and stochastic processes shape species distribution (Romme et al., 2016; Liu et al., 2018), and understanding these processes is essential for comprehending the current distribution of plant species.
Significant advancements have been made in understanding the assembly and distribution of seed plant communities (Nishizawa et al., 2022), illuminating processes such as neutral dynamics (Siefert et al., 2013), biotic, and environmental filtering (Jung et al., 2010). These insights have greatly enriched our understanding of plant community ecology. However, bryophytes, representing some of the earliest diverging lineages of land plants, exhibit unique ecological traits and life history strategies that may cause them to respond differently to these well-established processes. Unlike seed plants, bryophytes have a distinct physiological makeup, such as their poikilohydric nature, which allows them to survive in environments that would be hostile to most vascular plants. This fundamental difference in physiology and scale often results in different interactions with their environment and other organisms, potentially leading to different assembly and distribution patterns (Song et al., 2015). Despite their crucial roles in ecosystem functions such as water balance regulation and nutrient cycling (Ódor et al., 2013; Song et al., 2015), bryophytes have been relatively understudied, partly due to their small size and less conspicuous nature compared to vascular plants (Stehn et al., 2010). Given these considerations, our study aims to fill the substantial gap in our understanding of bryophyte assemblage dynamics, thereby enhancing our broader comprehension of plant community ecology and the unique challenges and mechanisms influencing bryophyte communities.
Bryophytes live in various types of vegetation, such as forests, thickets, and herbaceous vegetation (Scott, 1994). These vegetation types provide different habitat conditions and resources, thus providing different growth environments for bryophytes. In forest and thicket ecosystems, bryophytes can use the humidity and shade of the environment to find suitable growth space on the soil under the forest. Herbaceous vegetation is usually dominated by herbaceous plants, forming an open ground cover. Bryophytes can live on the surface of soil on grass or in symbiosis with herbaceous plants to form diverse bryophytes (Szövényi et al., 2004). Bryophytes’ remarkable adaptability allows them to thrive across diverse habitat conditions, making them vital subjects for biodiversity research (Turner and Pharo, 2005). Given their ecological significance, investigating these organisms in a region as ecologically unique and diverse as Tibet offers an exceptional opportunity to deepen our understanding of their ecological dynamics.
Tibet is located in southwestern China and is the highest and largest plateau on Earth. This unique geographical setting offers a wide range of microclimates and diverse vegetation types, from dense forests to sparse herbaceous cover. It is an exemplary natural laboratory for studying ecological adaptability. The extreme environmental conditions, coupled with variations in topography, vegetation cover, and human activities, create diverse vegetation types that are ideal for investigating how bryophytes adapt to both standard and harsh conditions. This rich variety allows our study to explore the dynamic patterns of local and regional bryophyte assemblages formed under the influence of both deterministic and stochastic processes. Based on these observations, we hypothesize that (1) the diversity of bryophytes varies significantly across different vegetation types due to distinct microclimatic conditions, (2) deterministic processes play a more dominant role than stochastic processes in shaping the spatial distribution of bryophyte assemblages, and (3) climatic factors are the main drivers of the assembly processes in bryophyte communities. Our research aims to test these hypotheses by addressing critical questions about bryophyte diversity and the factors influencing their assemblage processes.
2 Materials and methods
2.1 Study area
Tibet, known as the “Roof of the World,” is a region located in the high mountains of Asia. Its natural environment is characterized by immense beauty, extreme altitudes, and unique biodiversity, situated over 4,000 meters above sea level (Mo et al., 2004). Regionally, the climate in the southeast is warm and humid, and the climate in the northwest is cold and arid, with distinct dry and wet seasons, which leads to spatial differentiation of vegetation types (Yu and Xu, 2009). The primary ecosystem types in Tibet are forest, thicket, desert, grassland, etc (Chang, 1981).
2.2 Sampling and identification
We conducted field surveys and collected bryophytes from July to September in 2008, 2011, 2012, 2014, 2015, 2017, and 2019. We selected a sample site every 100 meters in elevation, which allowed us to capture the ecosystem characteristics and bryophyte diversity that change with the elevation gradient. We focused our study on the arid, semi-arid, and humid regions of Tibet to encompass various moisture gradients, which are crucial in shaping the structure of bryophyte communities. To better understand the distribution and diversity of bryophytes, we classified the areas surveyed, according to their physical features, into three vegetation types: forest, thickets, and herbaceous vegetation. This classification helped us to have a comprehensive understanding of the growth of bryophytes in these specific environments (Figure 1).
In forests, this included subtropical hardleaf evergreen broad-leaved, subtropical monsoon evergreen broad-leaved, and subtropical mountain coniferous forests. Thickets comprised subtropical evergreen broad-leaved shrubs, subalpine evergreen coniferous shrubs, subalpine hardleaf evergreen broad-leaved shrubs, and subalpine deciduous broad-leaved shrubs. The herbaceous vegetation included desert steppe with tufted dwarf grasses in the temperate zone, typical steppe of temperate tufted grasses, alpine sparse vegetation, alpine cushion vegetation, alpine Carex steppe, and alpine Kobresia meadow. Therefore, the study sites offered a wide range of growing environments, allowing for a more comprehensive understanding of how specific conditions under three types of vegetation influence the diversity of bryophytes. In each sample plot, the bryophytes on the type of soil substrate were generally investigated, and three quadrats were used to collect bryophytes on soils. The 0.5 × 0.5 m sampling frame with 100 grids was used to count and record species coverage. This standardized sampling method helped to collect consistent and comparable data. In total, 552 quadrats across 184 plots were used to investigate soil bryophyte species, ensuring the study’s extensive scope and large-scale sampling contributed to obtaining high-quality, statistically significant data. There are 44 plots in the forest, 48 plots in the thicket, and 92 plots in the herbaceous vegetation. Bryophyte specimens were collected from the sites, air-dried, and identified in the herbarium of China Agricultural University (BAU).
2.3 Environmental variables
Annual mean temperature, annual precipitation, mean diurnal range of temperature and solar radiation were acquired from the WorldClim dataset (Fick and Hijmans, 2017) with a spatial resolution of 30 s (ca. 1 km) recorded. The potential evaporation (PET) was available from the CGIAR-CSI figshare with a spatial resolution of 30 s (ca. 1 km) recorded (Trabucco and Zomer, 2018). Water content, sand content, pH, organic carbon, and total nitrogen in soil were gained at http://openlandmap.org.
Solar radiation, potential evaporation, temperature, mean diurnal range of temperature, precipitation, nitrogen, pH, sand content, carbon, and water content on the soil of the 184 sample plots were extracted in ArcGIS 10.5.
2.4 Data analyses
Bryophyte coverage was calculated spatially across different plots. Coverage at each plot represents the total coverage summed from all quadrats within that plot. Richness data, similarly, were calculated based on the total number of species observed within each plot. This approach ensures that our analysis captures spatial variations in species coverage and richness across the study area, prior to any further statistical analysis. The differences in bryophyte diversity among forest, thicket, and herbaceous vegetation were investigated through the utilization of analysis of variance (ANOVA) and Fisher’s LSD test.
To avoid issues with collinearity, we analyzed the correlation among selected environmental factors—solar radiation, potential evaporation, temperature, mean diurnal range of temperature, precipitation, nitrogen, pH, sand content, carbon, and water content. We retained solar radiation, potential evaporation, temperature, mean diurnal range of temperature, precipitation, pH, sand content, and water content. We also ensured that all pairwise Pearson correlation coefficients (R) were below 0.8 (Supplementary Figure 1). Further analysis using partial least squares path modeling (PLSPM) revealed both direct and indirect impacts of environmental drivers on bryophyte assemblage stability (Figure 2; Supplementary Table 1).
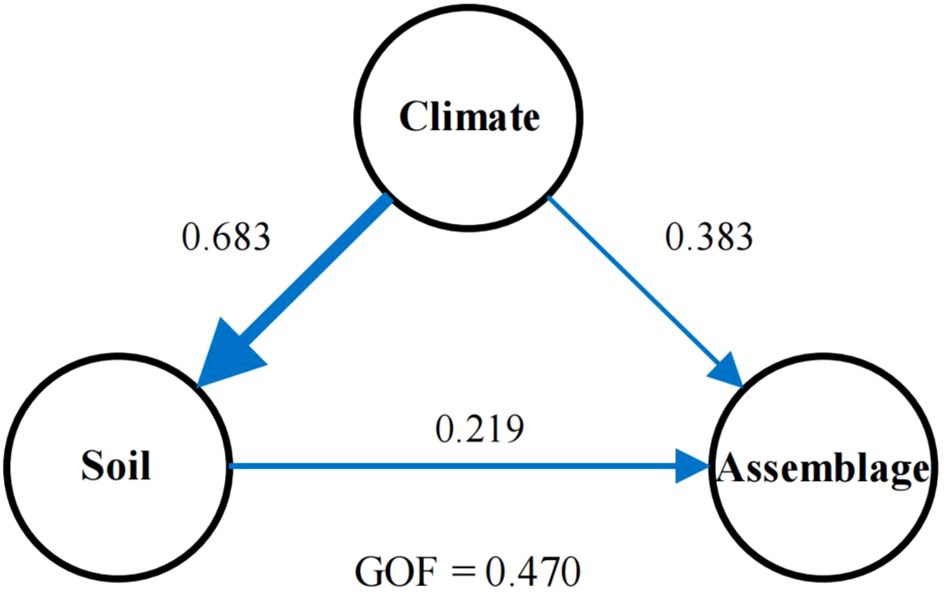
Figure 2. The direct and indirect effects of soil and climate on the bryophyte assemblage stability in the Tibet Plateau based on partial least squares path modelling. Each oval represents a latent variable (e.g., climate). The path with arrowhead and coefficient indicates a unidirectional cause-total effect between variables. Path coefficients are reflected by the widths of the arrows and the numbers next to the arrows. GOF, the goodness of fit.
Linear model was used to assess the environmental factors crucial for bryophyte richness across 184 plots. We address this type of collinearity problem by using glmm.hp. Through hierarchical partitioning, we can isolate the independent contribution of each predictor to the variation in the response variable. By implementing this approach, we ensured that our model’s conclusions are robust and accurately reflected the actual influences of each environmental factor across different vegetation subgroups, enhancing both the accuracy and interpretability of our findings. Environmental factors encompassed temperature, precipitation, mean diurnal temperature range, solar radiation, water content, sand content, pH, carbon, and (Dray and Dufour, 2007; Schielzeth and Nakagawa, 2013; Bates et al., 2014; Kuznetsova et al., 2015; Kassambara, 2022; Lai et al., 2022).
The Redundancy Analysis (RDA) was used to determine how closely the variation in bryophyte composition, derived from a species-plot-coverage matrix incorporating species coverage data for each plot, is related to the environmental conditions (Oksanen et al., 2013). The main purpose of conducting partial mantel correlations was to determine the strength and significance of the relationship between two sets of variables while accounting for the potential influence of confounding factors. We used partial mantel correlations (9,999 permutations) to determine the extent to which environmental factors significantly influenced the observed patterns in bryophyte assemblage composition (species-plot-coverage matrix) of forest, thicket, and herbaceous vegetation (Guillot and Rousset, 2013; Legendre et al., 2015).
The partial least squares path modeling (PLS-PM) approach was used to investigate the relationships between latent variables and assessed the direct and indirect associations among “climate”, “soil properties”, and “bryophyte assemblage stability” (Sanchez et al., 2013). Climate variables included temperature, potential evaporation, precipitation, and solar radiation. Soil properties were represented by metrics such as water content, sand content, and pH levels. Bryophyte assemblage stability was quantified using the community stability index (ICV), defined as the ratio of the mean relative coverage of all plant species in one particular plot to the standard deviation of this mean coverage (Tilman, 1999). This model assessed both the direct and indirect associations among these constructs to elucidate the underlying ecological drivers of bryophyte community dynamics. To assess the performance of model performance, we used the goodness of fit index (GoF) within the range of 0.40 to 1.00 (Wang et al., 2021).
In order to assess the clustering or overdispersion of bryophyte assemblages, the null model is examined using the checkerboard score (C-score) method (30,000 simulations) (Stone and Roberts, 1990); (Gotelli et al., 2015). We calculated the checkerboard score (C-score) and Standardized Effect Size (SES) for each vegetation type to determine patterns. The C-score is an ecological index utilized to characterize co-occurrence patterns and is a valuable tool for quantifying the tendency of interspecific repulsion among bryophyte species. Standardized effect size (SES) was used to standardize the C-score (Gotelli and McCabe, 2002). A higher standardized effect size (SES) value indicates overdispersion, while a lower SES value indicates underdispersion compared to the expected null value (Swenson, 2014; Mo et al., 2021).
The analysis was spatially conducted within each assemblage type, allowing us to evaluate community structure by comparing observed species frequencies against the model’s predictions. One thousand bootstrap replicates were generated, and the resulting distribution of fitting statistics was used to calculate the 95% confidence intervals. We partitioned the species within each dataset into three groups based on their occurrence frequency relative to the 95% confidence interval of the NCM predictions: above partition (more frequent), below partition (less frequent), and neutral partition (within the interval). The community connectivity quantifies the potential for species dispersal within the bryophyte assemblage, considering the geographical layout of the sampling plots. We calculated the geographical distance between sampling plots by using the latitude and longitude coordinates recorded. Subsequently, we evaluated the connectivity of the bryophyte assemblage by analyzing the degree to which individual plots within the sampling grid are interconnected through potential dispersal pathways (Yeh et al., 2015).
The Modified Stochasticity Ratio (MST) is a metric that quantifies the influence of stochastic and deterministic processes on bryophyte dynamics. We chose the MST over the standard Normalized Stochasticity Ratio (NST) due to the latter’s occasional calculation errors resulting in values outside the 0 to 1 range. Below the 50% threshold, deterministic factors dominate with predictable effects, while above the 50% threshold, stochastic factors become more influential, leading to species dynamics driven by environmental uncertainty and randomness (Ning et al., 2019). MST based on Bray-Curtis dissimilarity (MSTbray) was utilized to quantify the level of stochasticity in the assembly of ecological communities in the study. The code used for the analyses in this paper can be found in Supplementary Data Sheet 1.
3 Results
3.1 Richness of bryophytes
This study recorded 324 bryophyte species through investigation of sampling plots. The richness of bryophytes gradually decreased along the forest, thicket, and herbaceous vegetation (Figure 3). The highest richness of bryophytes was in forest, which significantly higher than that of herbaceous vegetation. It should also be noted that there was no significant difference in bryophyte richness between the thicket and both the herbaceous vegetation and the forest.
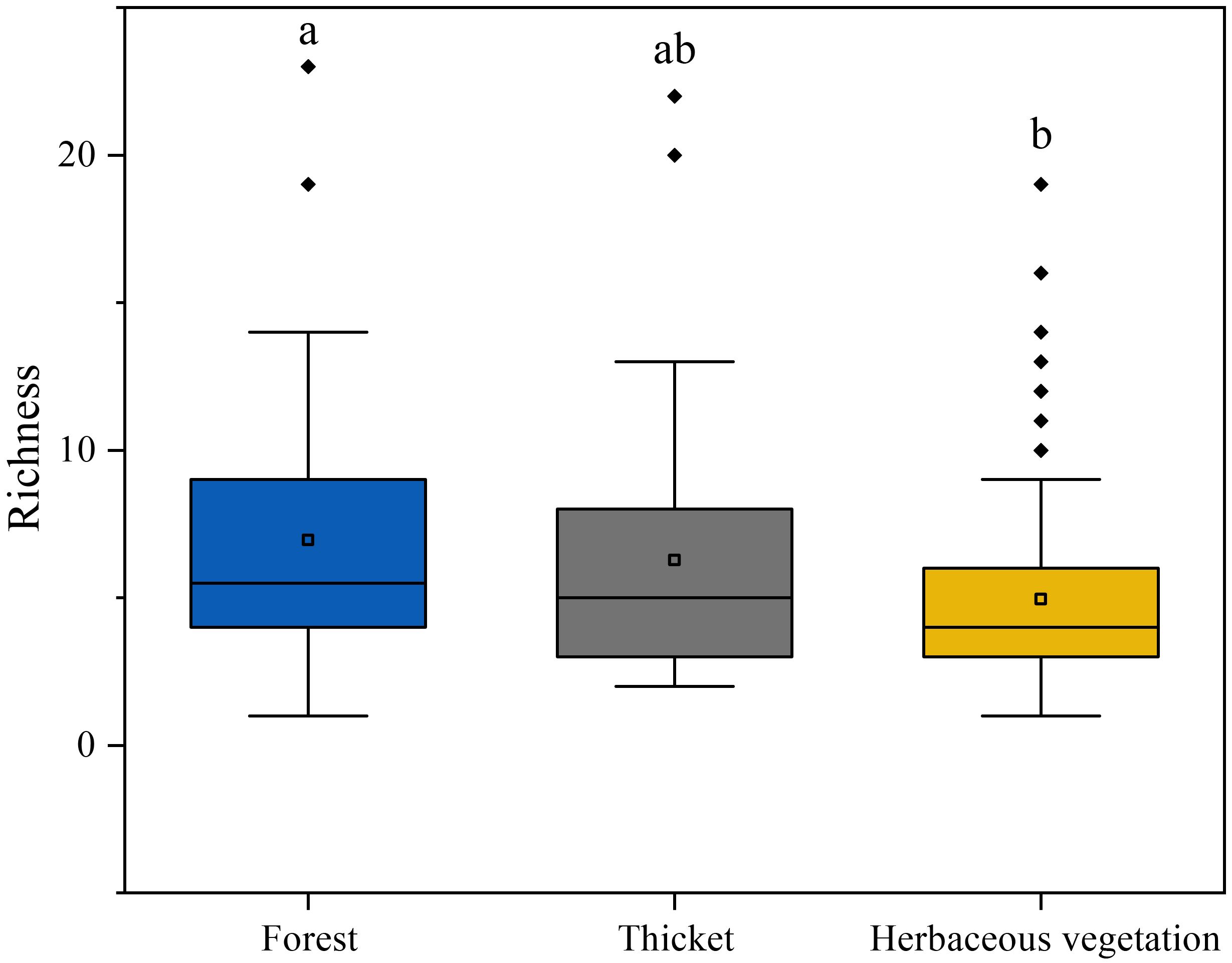
Figure 3. The bryophyte diversity differences among different vegetation types. Lowercase letters indicate significant differences among vegetation types at the 0.05 error probability level, as determined using Fisher’s LSD test. The boxes represent the range of values between the third and the first quartile. Outliers are values that do not fall within 1.5 times the interquartile range of the first and third quartiles (indicated by the whiskers). In each box, the horizontal black line represents the median, while the hollow point represents the mean value.
pH were the highest factors in explaining the bryophyte diversity variation in Forest, Thicket, and Herbaceous vegetation (Figure 4). The richness of bryophytes exhibited a negative correlation with solar radiation, mean diurnal range of temperature and pH, while showing a positive correlation with longitude, potential evaporation, precipitation and water content (Supplementary Figure 2).
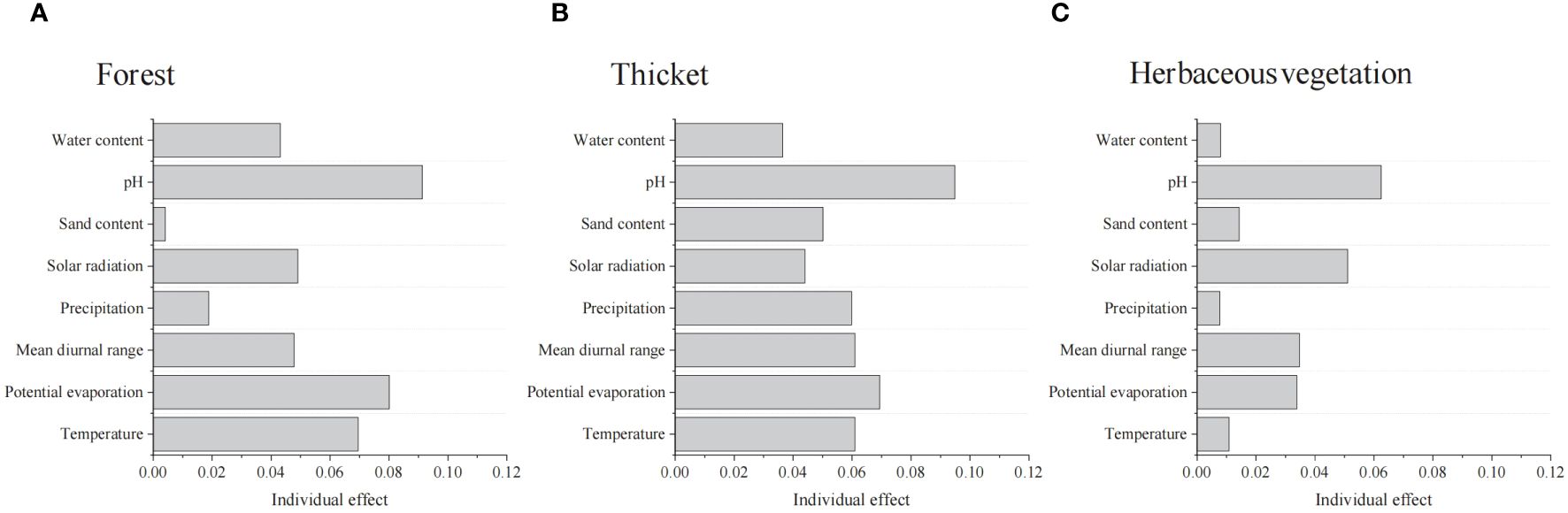
Figure 4. Summary of linear mixed effects model analyzing the effects of environmental factors on the bryophyte diversity of (A) forest, (B) thicket, and (C) herbaceous vegetation.
3.2 Relationship between bryophyte assemblages and environmental factors
The first two axes of Redundancy Analysis (RDA) accounted for 45.91% (28.84% + 17.07%) of the total variance (Figure 5). The bryophyte assemblage of forests displayed a positive correlation with high precipitation and water content levels. In contrast, the bryophyte assemblage of herbaceous vegetation was associated with elevated solar radiation, mean diurnal range of temperature, pH, and sand content. In bryophyte assemblage of forest, significant correlations were found between bryophyte assemblages and pH (0.132), mean diurnal range (0.102), and potential evaporation (0.076, Table 1). In bryophyte assemblage of thicket, significant results were observed for solar radiation (0.232), potential evaporation (0.197), pH (0.188), precipitation (0.131), mean diurnal range (0.121), and temperature (0.112).
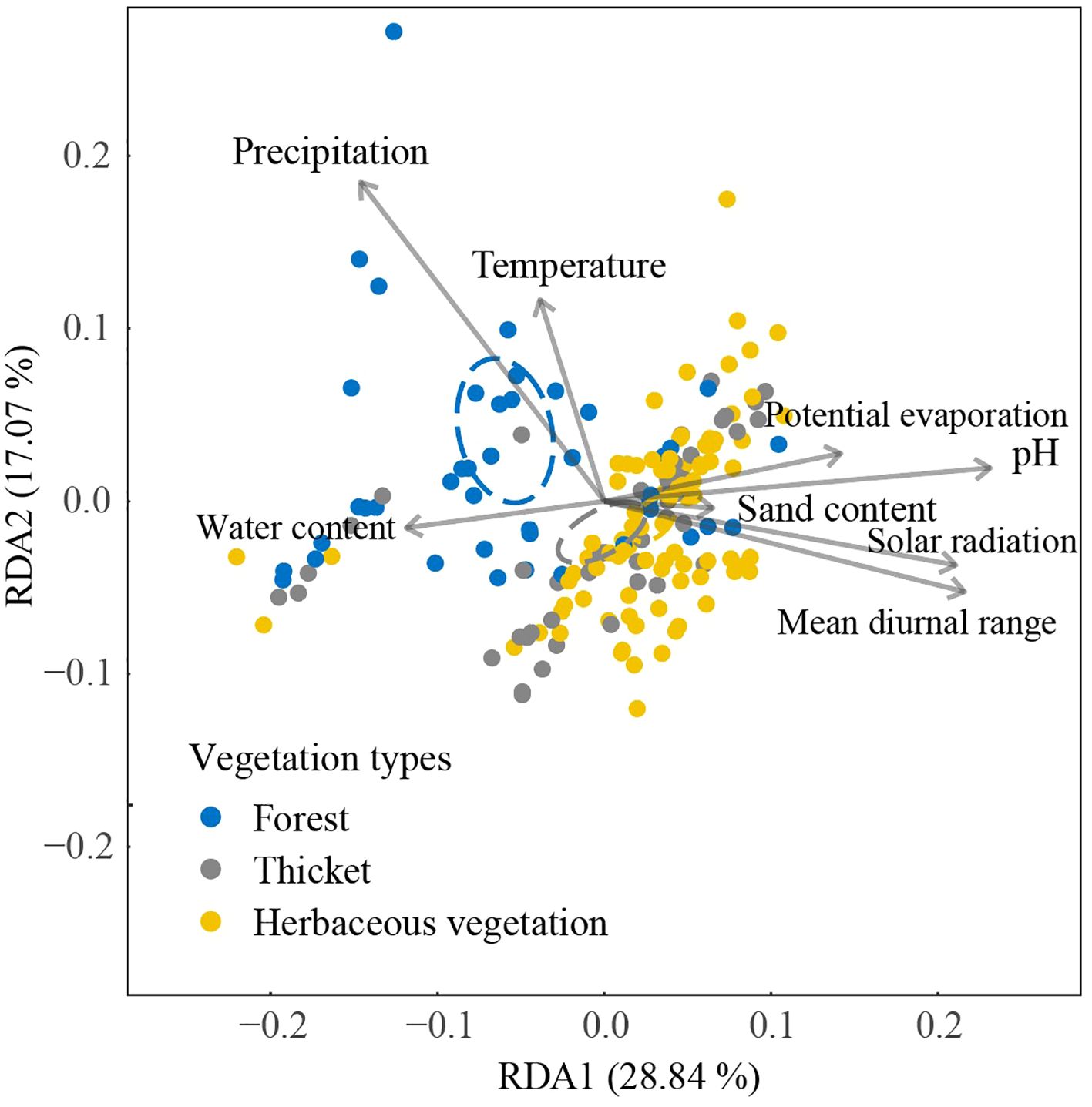
Figure 5. Relationship between bryophyte assemblage and environment based on dissimilarities among different vegetation types using RDA. The positions in ordination space are shown for 184 plots and environmental factors. The ellipses were calculated around barycenters with a confidence level of 0.95.
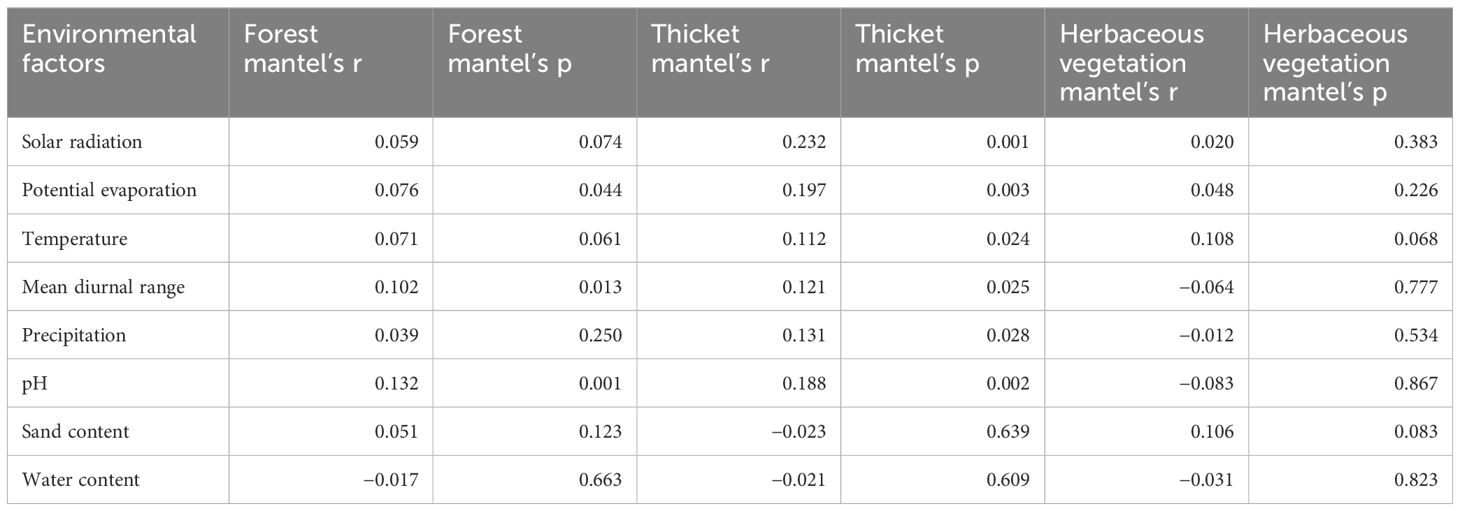
Table 1. Relationships among bryophyte assemblages in forest, thicket, and herbaceous vegetation and their environment.
Further analysis using partial least squares path modeling (PLSPM) revealed both direct and indirect impacts of environmental drivers on bryophyte assemblage stability (Figure 2, Supplementary Table 1). The stability of bryophyte assemblage was influenced by climatic and soil factors (Figure 2), as indicated by PLSPM, which explained a total effect of 0.512 and 0.226 on bryophyte assemblage stability in the climate and soil (Table 1). Specifically, the climate had a total effect of 0.691 on soil properties, leading to indirect changes in bryophyte assemblage stability (0.226, Table 1). Additionally, soil properties directly and positively affected bryophyte assemblage stability (0.324) (Figure 2) as well.
3.3 Bryophyte co-occurrence patterns
Bryophyte assemblages in forests (2.89%), thickets (1.48%), and herbaceous vegetation (3.05%) exhibited a notable disparity between the observed C-scores and values generated from random simulations, indicating a significant difference in co-occurrence patterns among these vegetation types (p < 0.05). Therefore, the co-occurrence patterns of bryophyte assemblages in different vegetation types were non-random (Figure 6).
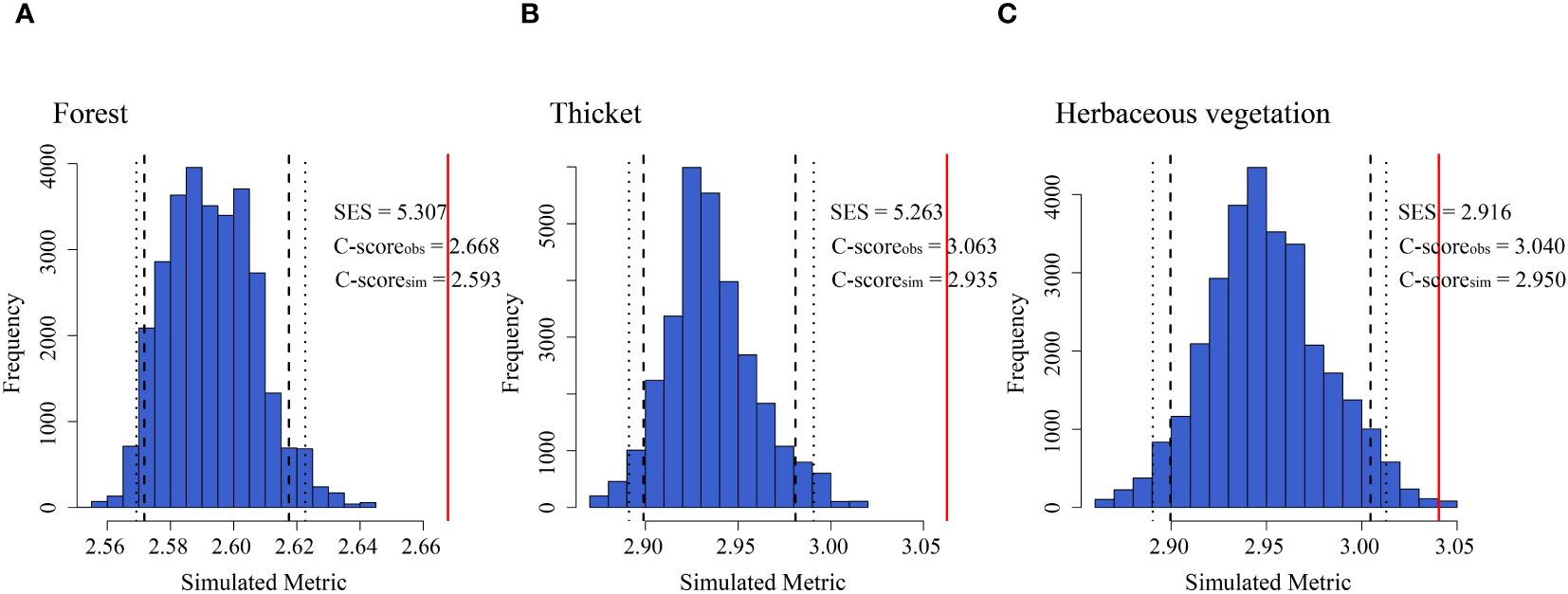
Figure 6. Co-occurrence patterns shaping the bryophyte assemblage assembly of (A) forest, (B) thicket, and (C) herbaceous vegetation. C-score metric using null models. The values of observed C-score (C-scoreobs) > simulated C-score (C-scoresim) indicate non-random co-occurrence patterns. Standardized effect size < −2 and > 2 represent aggregation and segregation, respectively. The blue bars indicate the observed C-score (C-scoreobs) and the simulated C-score (C-scoresim) for each type of vegetation. The red lines represent the standardized effect size (SES) of the C-scores.
3.4 Deterministic and stochastic processes affecting bryophyte assemblages
The neutral community model (NCM) provided a robust estimation of a substantial portion of the association between species occurrence frequency and their relative coverage fluctuations. Specifically, the NCM explained 0.120, 0.492, and 0.595 of the variances observed in the bryophyte assemblages within forest, thicket, and herbaceous vegetation, respectively. The degree to which the bryophyte assemblages were affected by stochastic processes increased gradually in the forest, thicket and herbaceous vegetation (Figure 7). The connectivity of bryophyte assemblages in herbaceous vegetation was considerably greater compared to those in forest and thicket (Supplementary Figure 3). The Modified Stochasticity Ratio based on the Bray-Curtis dissimilarity (MSTbray) index increased along the forest, thicket, and herbaceous vegetation (Figure 8). This indicated that the extent to which bryophyte assemblages were affected by deterministic processes decreases along forest, thicket, and herbaceous vegetation environments.
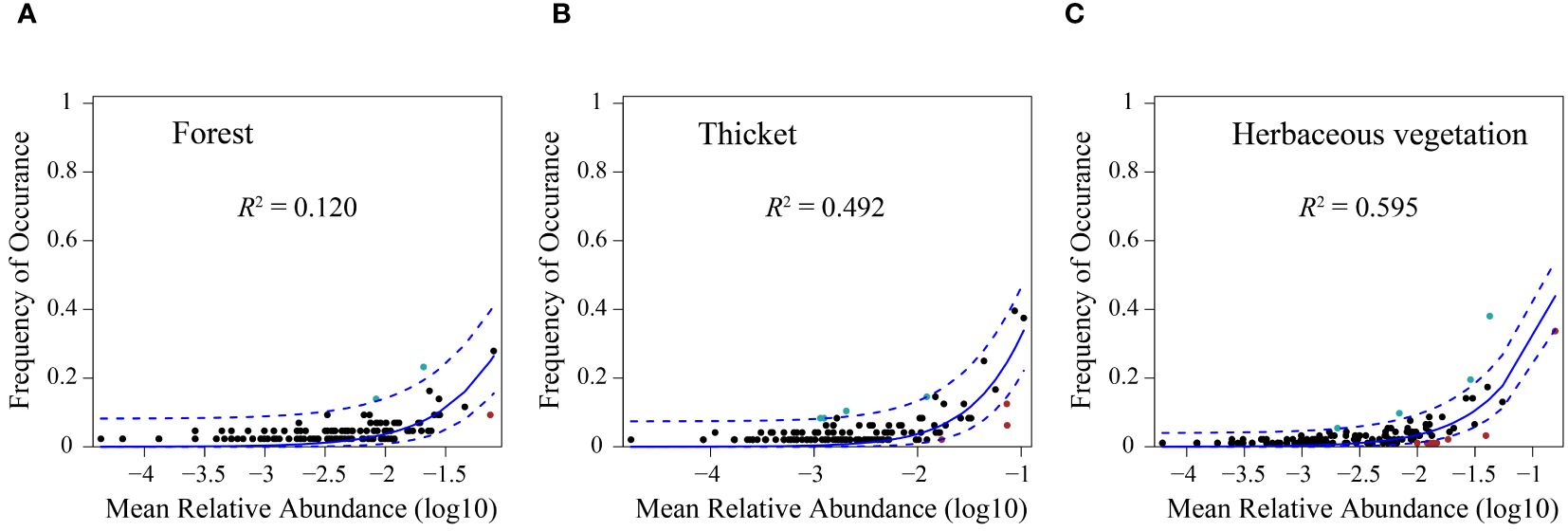
Figure 7. The fit of the neutral community model (NCM) of bryophyte assemblage. The predicted occurrence frequencies for (A) forest, (B) thicket, and (C) herbaceous vegetation, and all representing bryophyte assemblage from the forest, thicket, and herbaceous vegetation, respectively. The solid blue lines indicate the best fit to the NCM, and the dashed blue lines represent 95% confidence intervals around the model prediction. Species that occur more or less frequently than predicted by the NCM are shown in different colours. R2 indicates the fit to this model.
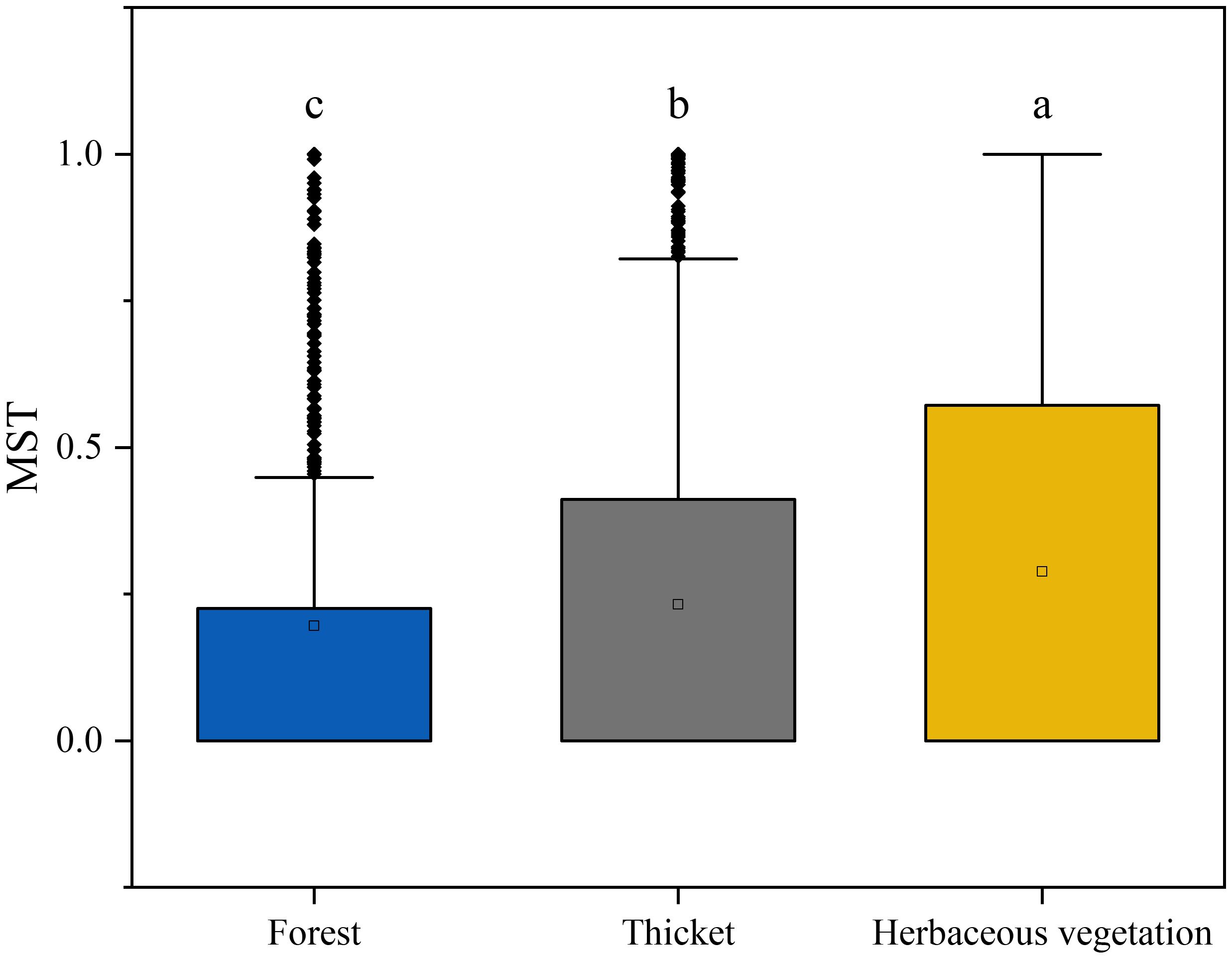
Figure 8. Bryophyte assemblage process measurements by the dominance test. The Modified Stochasticity Ratio (MST) was developed based on Bray-Curtis dissimilarity (MSTbray) with 50% as the boundary point between more deterministic (< 50%) and more stochastic (> 50%) assembly. Lowercase letters indicate significant differences at 0.05 error probability levels among vegetation types. The boxes represent the range of values between the third and the first quartile. Outliers are values that do not fall within 1.5 times the interquartile range of the first and third quartiles (indicated by the whiskers). In each box, the horizontal black line represents the median, while the hollow point represents the mean value.
4 Discussion
4.1 Environmental filtering
Bryophyte diversity and spatial distribution patterns are shaped by environmental factors such as climate and soil properties. In regions like Tibet, characterized by the world’s most robust solar radiation, bryophyte energy inputs are significantly influenced (Raabe et al., 2010). As poikilohydric organisms, bryophytes require moist environments for growth and reproduction; rapid evaporation of moisture in these regions makes it difficult for them to thrive (Liu et al., 2021). Additionally, high solar radiation can cause cellular damage, decreasing growth and reproduction rates. Bryophytes’ sensitivity to temperature fluctuation further challenges their survival in such regions. The relationship between mean daily temperature variation and bryophyte diversity is intricate, as species require specific temperature conditions (Király et al., 2013; Yeh et al., 2015). Tibet’s extreme temperature fluctuations may surpass the optimal range for several species, limiting their distribution and decreasing diversity (Aude and Poulsen, 2000; Brunialti et al., 2010), resulting in reduced richness in areas with higher mean diurnal temperature ranges (Supplementary Figure 2).
Soil pH, as a pivotal abiotic factor, plays a significant role in various bryophyte ecological processes, including nutrient availability and metal toxicity (Concostrina-Zubiri et al., 2022). Bryophytes, with their unique physiological characteristics of directly absorbing nutrients and water through surface cells, are particularly sensitive to changes in soil pH (Ren et al., 2021). This sensitivity is crucial in understanding how pH variations can impact bryophyte growth and survival. High or low pH levels alter the solubility of nutrients and toxins, which are directly absorbed and can significantly affect metabolic processes in bryophytes (Tyler and Olsson, 2016). Certain physiological traits of bryophytes make them especially susceptible to, or capable of adapting to, these pH changes. Research has indicated clear pH preferences for different bryophyte species, underscoring the selective pressure exerted by soil pH on community composition. Moreover, soil pH acts as an environmental filter within bryophyte communities, shaping community assembly by favoring species that can thrive within specific pH ranges. This filtering effect leads to distinct community dynamics, where only those species adapted to prevailing soil pH conditions can establish and persist, highlighting the critical role of soil pH in ecological processes and bryophyte richness in Tibet (Figure 4).
4.2 Co-occurrence patterns
The co-occurrence patterns of bryophyte assemblages in different vegetation types are non-random, and the co-occurrence patterns tend to be less segregated from forests to herbaceous vegetation (Figure 6). Several reasons might explain this finding. Bryophyte species may have varying ecological preferences or tolerances to environmental factors, leading to changes in their co-occurrence patterns. Forests are generally more structurally complex than thicket and herbaceous vegetation, providing a broader range of microhabitats, potentially allowing for more niche partitioning among bryophyte species and resulting in more segregated co-occurrence patterns (Pócs, 1982). Furthermore, the strength and type of interactions between bryophyte species may vary across different vegetation types; in the forest, competition is more intense, leading to more segregated patterns. Dispersal limitation also plays a role, as the dispersal abilities of bryophyte species differ across vegetation types due to variations in the surrounding landscape, leading to non-random co-occurrence patterns. Lastly, different vegetation types may experience distinct disturbance regimes, such as fire, wind, or herbivory, which can impact bryophyte species’ co-occurrence patterns (Maren et al., 2018). Generally, disturbance can promote species coexistence by creating opportunities for colonization and reducing competitive exclusion.
4.3 Deterministic processes and stochastic processes
Deterministic processes in ecology refer to non-random events and forces that shape assemblage composition and dynamics, such as competition, predation, and environmental filtering. Bryophyte assemblages in forests are typically influenced more by deterministic processes compared to those in herbaceous vegetation (Figure 8). In forests, which are typically found at lower elevations, can be attributed to several factors associated with bryophyte interactions and environmental filters, such as light, water, and nutrients (Turetsky et al., 2012). The competition for resources can be particularly intense in the understory of dense forest canopies, where bryophytes must compete with each other for limited resources (Silva et al., 2014; Valente et al., 2017). Bryophyte interactions with other members of the forest ecosystem, such as soil microbes and decomposers, can also play a role in determining assemblage composition and dynamics (Hamard et al., 2019). Environmental filters in forest ecosystems can also strongly influence bryophyte assemblage composition and dynamics (Ovaskainen et al., 2017). For example, soil chemistry, moisture levels, and light availability can all act as filters that limit the range of bryophyte species that can successfully colonize a given area (Fattorini and Halle, 2004). Because of the extreme environmental conditions in Tibet, deterministic processes play a more important role in bryophyte assemblages than stochastic ones (Figure 8). Bryophyte species in these environments are constantly adapting their adaptation strategies to survive the harsh climate and high altitude (Proctor et al., 2007; He et al., 2016). These adaptations lead to decisive processes that drive the composition and structure of bryophyte assemblages.
The dispersal ability of bryophytes is critical in stochastic processes, particularly since they tend to occupy spatially limited patches of habitat. The widespread distribution of many bryophyte species may result from frequent and sustained long-distance dispersal, with spores being the primary mode of dispersal (Lewis et al., 2017). In our study area, we also observed that the connectivity of bryophyte assemblage is higher in herbaceous vegetation compared to forests (Supplementary Figure 3). Studies indicate that bryophyte spores typically disperse under dry conditions, a strategy known as xerochastic dispersal, which is conducive to their transmission over greater distances. Dry conditions facilitate better airborne transportation of spores, especially in open areas such as high-elevation herbaceous vegetations where wind effects are more pronounced, thus enabling spores to travel further. Additionally, the ultrastructural features of spores, such as sporoderm thickness and plastid development, may also relate to their dispersal strategies across different habitats (Medina and Estébanez, 2014). Consequently, bryophytes could easily dispersal to other areas in the herbaceous vegetation.
Consequently, deterministic and stochastic processes provide insights into the factors that shape bryophyte assemblages in the Tibet region. Deterministic processes emphasize biological interactions as well as environmental filtering. In contrast, the neutral theory of stochastic processes emphasizes the role of dispersal and the importance of aspects of ecological stochastic events. These perspectives suggest that a combination of deterministic and stochastic processes influences bryophyte assemblages in Tibet.
5 Conclusions
In this study, we used a combination of deterministic and stochastic processes to investigate the key factors influencing the diversity and assemblages of bryophytes in Tibet. Our results showed that the richness of bryophytes gradually decreased along the forest, thicket, and herbaceous vegetation, with the richness of bryophytes in forests being significantly higher than that in herbaceous vegetation. The co-occurrence patterns of bryophyte assemblages were more segregated in forests and thickets than in herbaceous vegetation. Stochastic processes increasingly influenced bryophyte assemblages within forest, thicket, and herbaceous vegetation environments. In deterministic processes, climate and soil properties were key factors in shaping the stability of bryophyte assemblages. Climate directly impacted the stability of these assemblages, while also indirectly influencing them by altering soil properties. pH was considered as the primary drivers of bryophyte diversity across all vegetation types. These findings contribute to a better understanding of the ecological processes of bryophytes in Tibet.
Data availability statement
The original contributions presented in the study are included in the article/Supplementary Material. Further inquiries can be directed to the corresponding author.
Author contributions
JG: Conceptualization, Data curation, Formal analysis, Methodology, Software, Validation, Visualization, Writing – original draft, Writing – review & editing. XTS: Conceptualization, Data curation, Investigation, Supervision, Writing – original draft, Writing – review & editing, Formal analysis, Methodology, Software. YY: Conceptualization, Data curation, Formal analysis, Investigation, Methodology, Resources, Supervision, Writing – review & editing. XHS: Conceptualization, Resources, Supervision, Validation, Visualization, Writing – review & editing. YL: Conceptualization, Data curation, Investigation, Methodology, Writing – review & editing. XMS: Conceptualization, Data curation, Funding acquisition, Investigation, Project administration, Resources, Supervision, Validation, Visualization, Writing – original draft, Writing – review & editing.
Funding
The author(s) declare financial support was received for the research, authorship, and/or publication of this article. This work was funded by the Tibet Autonomous Region Science and Technology Project (XZ202101ZD0003N), the Natural Science Foundation of China (Grant No. 41771054), the Flexible Talent Support Project of Tibet Agricultural and Animal Husbandry University (Grant No. 604419044), the Joint Scientific Research Fund Project of China Agricultural University-Tibet Agriculture and Animal Husbandry University, Chinese Universities Scientific Fund (Grant No. 2022TC126).
Acknowledgments
We extend our heartfelt gratitude to Youfang Wang (School of Life Science, East China Normal University), Min Li (College of Life Sciences, Hebei Normal University), and Xiaorui Wang (College of Resources and Environment, Shijiazhuang University) for their valuable contributions in collecting bryophyte specimens and assisting with partial specimen identification. We also express our appreciation to Shanshan Song, Yanbin Jiang, Yu Sun, Jin Kou, Baoguang Guo, Yingjie Fan, Ling Liu, Mengzhen Wang, Xinyuan Jiang from China Agricultural University, and Heping Ma from Tibet Agricultural and Animal Husbandry University for their assistance in collecting bryophyte specimens in Tibet.
Conflict of interest
The authors declare that the research was conducted in the absence of any commercial or financial relationships that could be construed as a potential conflict of interest.
Publisher’s note
All claims expressed in this article are solely those of the authors and do not necessarily represent those of their affiliated organizations, or those of the publisher, the editors and the reviewers. Any product that may be evaluated in this article, or claim that may be made by its manufacturer, is not guaranteed or endorsed by the publisher.
Supplementary material
The Supplementary Material for this article can be found online at: https://www.frontiersin.org/articles/10.3389/fevo.2024.1376263/full#supplementary-material
References
Aude E., Poulsen R. (2000). Influence of management on the species composition of epiphytic cryptogams in Danish fagus forests. Appl. Veg. Sci. 3, 81–88. doi: 10.2307/1478921
Bates D., Mächler M., Bolker B., Walker S. (2014). Fitting linear mixed-effects models using lme4. arXiv preprint arXiv:1406.5823. 67, 1–48. doi: 10.18637/jss.v067.i01
Brunialti G., Frati L., Aleffi M., Marignani M., Rosati L., Burrascano S., et al. (2010). Lichens and bryophytes as indicators of old-growth features in Mediterranean forests. Plant Biosyst. 144, 221–233. doi: 10.1080/11263500903560959
Chang D. (1981). The vegetation zonation of the Tibetan plateau. Mt. Res. Dev. 1, 29–48. doi: 10.2307/3672945
Concostrina-Zubiri L., Berdugo M., Valencia E., Mendoza B. J., Maestre F. T. (2022). Decomposition of dryland biocrust-forming lichens and mosses contributes to soil nutrient cycling. Plant Soil. 481, 23–34. doi: 10.1007/s11104-022-05481-7
Dray S., Dufour A. (2007). The ade4 package: implementing the duality diagram for ecologists. J. Stat. Software 22, 1–20. doi: 10.18637/jss.v022.i04
Fattorini M., Halle S. (2004). The dynamic environmental filter model: how do filtering effects change in assembling communities after disturbance. Assembly rules Restor. ecol.: Bridging Gap between Theory Pract., 96–114.
Fick S. E., Hijmans R. J. (2017). Worldclim 2: new 1-km spatial resolution climate surfaces for global land areas. Int. J. Climatol. 37, 4302–4315. doi: 10.1002/joc.5086
Gotelli N. J., Hart E. M., Ellison A. M. (2015). Ecosimr: null model analysis for ecological data (R package version 0.1. 0).
Gotelli N. J., McCabe D. J. (2002). Species co-occurrence: a meta-analysis of jm diamond’s assembly rules model. Ecology 83, 2091–2096. doi: 10.1890/0012-9658(2002)083[2091:SCOAMA]2.0.CO;2
Guillot G., Rousset F. (2013). Dismantling the mantel tests. Methods Ecol. Evol. 4, 336–344. doi: 10.1111/2041-210x.12018
Hamard S., Robroek B. J., Allard P., Signarbieux C., Zhou S., Saesong T., et al. (2019). Effects of sphagnum leachate on competitive sphagnum microbiome depend on species and time. Front. Microbiol. 10, 2042. doi: 10.3389/fmicb.2019.02042
He X., He K. S., Hyvönen J. (2016). Will bryophytes survive in a warming world? Perspect. Plant Ecol. Evol. Systematics 19, 49–60. doi: 10.1016/j.ppees.2016.02.005
Hubbell S. P. (2011). The unified neutral theory of biodiversity and biogeography (mpb-32) (Princeton University Press, Princeton, NJ, USA). doi: 10.1515/9781400837526
Jung V., Violle C., Mondy C., Hoffmann L., Muller S. (2010). Intraspecific variability and trait-based community assembly. J. Ecol. 98, 1134–1140. doi: 10.1111/j.1365-2745.2010.01687.x
Kassambara A. (2022). Package ‘ggpubr’: ‘ggplot2’ based publication ready plots (R package, version 0.4. 0).
Király I., Nascimbene J., Tinya F., Ódor P. (2013). Factors influencing epiphytic bryophyte and lichen species richness at different spatial scales in managed temperate forests. Biodivers. Conserv. 22, 209–223. doi: 10.1007/s10531-012-0415-y
Kuznetsova A., Brockhoff P. B., Christensen R. H. B. (2015). Package ‘lmertest’. R Package version 2, 734. doi: 10.32614/CRAN.package.lmerTest
Lai J., Zou Y., Zhang S., Zhang X., Mao L. (2022). Glmm. Hp: an r package for computing individual effect of predictors in generalized linear mixed models. J. Plant Ecol. 15, 1302–1307. doi: 10.1093/jpe/rtac096
Legendre P., Fortin M. J., Borcard D. (2015). Should the mantel test be used in spatial analysis? Methods Ecol. Evol. 6, 1239–1247. doi: 10.1111/2041-210X.12425
Lewis L. R., Ickert-Bond S. M., Biersma E. M., Convey P., Goffinet B., Hassel K., et al. (2017). Future directions and priorities for arctic bryophyte research. Arct. Sci. 3, 475–497. doi: 10.1139/as-2016-0043
Liu C., Dudley K. L., Xu Z. H., Economo E. P. (2018). Mountain metacommunities: climate and spatial connectivity shape ant diversity in a complex landscape. Ecography 41, 101–112. doi: 10.1111/ecog.03067
Liu L., Jiang Y., Song X., Tang J., Kou J., Fan Y., et al. (2021). Temperature, not precipitation, drives the morphological traits of didymodon rigidulus in Tibet. Ecol. Indic. 133, 108401. doi: 10.1016/j.ecolind.2021.108401
Maciel J. R., Sanchez-Tapia A., Siqueira M. F. D., Alves M. (2017). Palaeodistribution of epiphytic bromeliads points to past connections between the Atlantic and amazon forests. Bot. J. Linn. Soc 183, 348–359. doi: 10.1093/botlinnean/bow020
Maren I. E., Kapfer J., Aarrestad P. A., Grytnes J., Vandvik V. (2018). Changing contributions of stochastic and deterministic processes in community assembly over a successional gradient. Ecology 99, 148–157. doi: 10.1002/ecy.2052
Medina N. G., Estébanez B. (2014). Does spore ultrastructure mirror different dispersal strategies in mosses? A study of seven iberian orthotrichum species. PloS One 9, e112867. doi: 10.1371/journal.pone.0112867
Mo S. G., Zhang B. P., Cheng W. M., Tan Y., Xiao F., Wu H. Z. (2004). Major environmental effects of the Tibetan plateau. Prog. Geogr. 23, 88–96. doi: 10.11820/dlkxjz.2004.02.011
Mo Y., Peng F., Gao X., Xiao P., Logares R., Jeppesen E., et al. (2021). Low shifts in salinity determined assembly processes and network stability of microeukaryotic plankton communities in a subtropical urban reservoir. Microbiome 9, 128. doi: 10.1186/s40168-021-01079-w
Ning D., Deng Y., Tiedje J. M., Zhou J. (2019). A general framework for quantitatively assessing ecological stochasticity. Proc. Natl. Acad. Sci. 116, 16892–16898. doi: 10.1073/pnas.1904623116
Nishizawa K., Shinohara N., Cadotte M. W., Mori A. S. (2022). The latitudinal gradient in plant community assembly processes: a meta-analysis. Ecol. Lett. 25, 1711–1724. doi: 10.1111/ele.14019
Ódor P., Király I., Tinya F., Bortignon F., Nascimbene J. (2013). Patterns and drivers of species composition of epiphytic bryophytes and lichens in managed temperate forests. For. Ecol. Manage. 306, 256–265. doi: 10.1016/j.foreco.2013.07.001
Oksanen J., Blanchet F. G., Kindt R., Legendre P., Minchin P. R., O Hara R. B., et al. (2013). Package ‘vegan’ Vol. 2 (Community ecology package, version), 1–295.
Ovaskainen O., Tikhonov G., Norberg A., Guillaume Blanchet F., Duan L., Dunson D., et al. (2017). How to make more out of community data? A conceptual framework and its implementation as models and software. Ecol. Lett. 20, 561–576. doi: 10.1111/ele.12757
Pócs T. (1982). “Tropical forest bryophytes,” in Bryophyte ecology. Ed. Smith A. J. E. (Chapman and Hall, London, UK), 59–104.
Proctor M. C., Oliver M. J., Wood A. J., Alpert P., Stark L. R., Cleavitt N. L., et al. (2007). Desiccation-tolerance in bryophytes: a review. bryologist 110, 595–621. doi: 10.1639/0007-2745(2007)110[595:DIBAR]2.0.CO;2
Raabe S., Müller J., Manthey M., Dürhammer O., Teuber U., Göttlein A., et al. (2010). Drivers of bryophyte diversity allow implications for forest management with a focus on climate change. For. Ecol. Manage. 260, 1956–1964. doi: 10.1016/j.foreco.2010.08.042
Ren J., Liu F., Luo Y., Zhu J., Luo X. Q., Liu R. (2021). The pioneering role of bryophytes in ecological restoration of manganese waste residue areas, southwestern China. J. Chem. 2021, 1–19. doi: 10.1155/2021/9969253
Romme W. H., Whitby T. G., Tinker D. B., Turner M. G. (2016). Deterministic and stochastic processes lead to divergence in plant communities 25 years after the 1988 yellowstone fires. Ecol. Monogr. 86, 327–351. doi: 10.1002/ecm.1220
Sanchez G., Trinchera L., Sanchez M. G., FactoMineR S. (2013). Package ‘plspm’ (Citeseer: State College, PA, USA).
Schielzeth H., Nakagawa S. (2013). Nested by design: model fitting and interpretation in a mixed model era. Methods Ecol. Evol. 4, 14–24. doi: 10.1111/j.2041-210x.2012.00251.x
Scott G. (1994). Elementary reflections on the biology of bryophytes. Victorian Naturalist 111, 112–115.
Siefert A., Ravenscroft C., Weiser M. D., Swenson N. G. (2013). Functional beta-diversity patterns reveal deterministic community assembly processes in eastern n orth a merican trees. Glob. Ecol. Biogeogr. 22, 682–691. doi: 10.1111/geb.12030
Silva J. B., Dos Santos N. D., Pôrto K. C. (2014). Beta-diversity: effect of geographical distance and environmental gradients on the rocky outcrop bryophytes. Cryptogamie Bryologie 35, 133–163. doi: 10.7872/cryb.v35.iss2.2014.133
Song L., Ma W. Z., Yao Y. L., Liu W. Y., Li S., Chen K., et al. (2015). Bole bryophyte diversity and distribution patterns along three altitudinal gradients in Yunnan, China. J. Veg. Sci. 26, 576–587. doi: 10.1111/jvs.12263
Stehn S. E., Webster C. R., Glime J. M., Jenkins M. A. (2010). Elevational gradients of bryophyte diversity, life forms, and community assemblage in the southern appalachian mountains. Can. J. For. Res. 40, 2164–2174. doi: 10.1139/X10-156
Stone L., Roberts A. (1990). The checkerboard score and species distributions. Oecologia 85, 74–79. doi: 10.1007/BF00317345
Swenson N. G. (2014). Functional and phylogenetic ecology in r (New York: Springer Science & Business Media). doi: 10.1007/978-1-4614-9542-0
Szövényi P., Hock Z. S., Tóth Z. (2004). Phorophyte preferences of epiphytic bryophytes in a stream valley in the carpathian basin. J. Bryol. 26, 137–146.
Tilman D. (1999). The ecological consequences of changes in biodiversity: a search for general principles. Ecology 80, 1455–1474. doi: 10.2307/176540
Trabucco A., Zomer R. J. (2018). Global aridity index and potential evapo-transpiration (et0) climate database v2. Cgiar consortium for spatial information (cgiar-csi) (CGIAR-CSI GeoPortal).
Turetsky M. R., Bond Lamberty B., Euskirchen E., Talbot J., Frolking S., McGuire A. D., et al. (2012). The resilience and functional role of moss in boreal and arctic ecosystems. New Phytol. 196, 49–67. doi: 10.1111/j.1469-8137.2012.04254.x
Turner P. A., Pharo E. J. (2005). Influence of substrate type and forest age on bryophyte species distribution in tasmanian mixed forest. Bryologist. 108, 67–85. doi: 10.1639/0007-2745(2005)108[67:IOSTAF]2.0.CO;2
Tyler T., Olsson P. A. (2016). Substrate ph ranges of south swedish bryophytes—identifying critical ph values and richness patterns. Flora 223, 74–82. doi: 10.1016/j.flora.2016.05.006
Valente E. D. B., Pôrto K. C., Bastos C. J. P. (2017). Habitat heterogeneity and diversity of bryophytes in campos rupestres. Acta Bot. Bras. 31, 241–249. doi: 10.1590/0102-33062016abb0450
Wang W., Hou Y., Pan W., Vinay N., Mo F., Liao Y., et al. (2021). Continuous application of conservation tillage affects in situ n2o emissions and nitrogen cycling gene abundances following nitrogen fertilization. Soil Biol. Biochem. 157, 108239. doi: 10.1016/j.soilbio.2021.108239
Yeh Y. C., Peres Neto P. R., Huang S. W., Lai Y. C., Tu C. Y., Shiah F. K., et al. (2015). Determinism of bacterial metacommunity dynamics in the southern East China Sea varies depending on hydrography. Ecography 38, 198–212. doi: 10.1111/ecog.00986
Keywords: bryophyte, biodiversity, deterministic processes, stochastic processes, neutral community model, Tibet
Citation: Gu J, Song X, Ye Y, Shao X, Liao Y and Shao X (2024) Exploring the driving factors of bryophyte assemblage distribution patterns in Tibet. Front. Ecol. Evol. 12:1376263. doi: 10.3389/fevo.2024.1376263
Received: 25 January 2024; Accepted: 15 July 2024;
Published: 12 August 2024.
Edited by:
Pavel Kindlmann, Charles University, CzechiaReviewed by:
Matthew Michalska-Smith, University of Minnesota Twin Cities, United StatesNaven Narayanan Venkatanarayanan, University of Minnesota Twin Cities, United States
Copyright © 2024 Gu, Song, Ye, Shao, Liao and Shao. This is an open-access article distributed under the terms of the Creative Commons Attribution License (CC BY). The use, distribution or reproduction in other forums is permitted, provided the original author(s) and the copyright owner(s) are credited and that the original publication in this journal is cited, in accordance with accepted academic practice. No use, distribution or reproduction is permitted which does not comply with these terms.
*Correspondence: Xiaoming Shao, c2hhb3htQGNhdS5lZHUuY24=
†These authors have contributed equally to this work and share first authorship