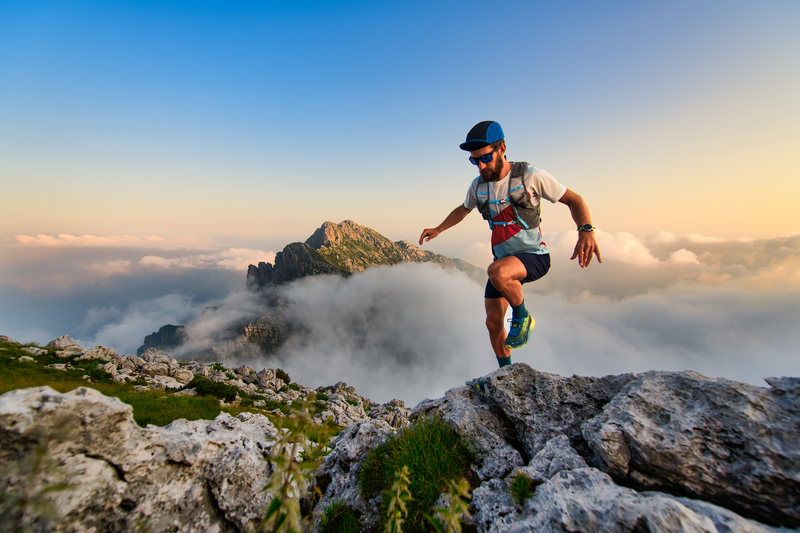
94% of researchers rate our articles as excellent or good
Learn more about the work of our research integrity team to safeguard the quality of each article we publish.
Find out more
ORIGINAL RESEARCH article
Front. Ecol. Evol. , 08 April 2024
Sec. Models in Ecology and Evolution
Volume 12 - 2024 | https://doi.org/10.3389/fevo.2024.1347066
Glycyrrhiza inflata Batalin is an important medical plant of the genus Glycyrrhiza. It is one of the key protected plants in China, distributed in the desert areas of southern Xinjiang and Dunhuang of Gansu Province. It has a strong resistance to drought, heat, and salt stresses, and plays a pivotal role in sand fixtion in desert areas. In this study, based on 157 valid distribution records and eight environmental factors including climate factors and altitude, the potential distribution area of G. inflata in the last glacial maximum, middle Holocen, modern, and future (2050) times in China were predicted, using the optimized MaxEnt model and ArcGis 10.2 software. The results showed that the predicted distribution area was highly consistent with the current distribution range, and the area under the receiver operating characteristic (AUC) curve was 0.986, indicating that the prediction performance was excellent. The key climatic factors affecting the distribution were precipitation in December and the average annual precipitation. Meanwhile, the suitable area of G. inflata in modern times was 1,831,026 km2, mainly distributed in Turpan-Hami Basin, Tarim Basin, and Dunhuang of Gansu Province, with Lop Nur Town of Xinjiang as the distribution center. In 2050, the potential suitable area forG. inflata in China will be 1,808,090 km2, 250,970 km2 of which will be highly suitable, which is 150,600 km2 smaller than that in modern times, with a reduction rate of 60.0%. Therefore, there is a trend of great reduction in the suitable area of G. inflata. From the last glaciation maximum to the middle Holocene, the geographical distribution center shifted to the southwest margin of the Kumtag Desert, Xinjiang, then later continued to shift to the southwest. This study will provide a basis for understanding the origin and evolution of G. inflata, developing conservation strategies to minimize the impacts of environment change, and utilizing plant resource.
Environmental factors greatly affect the distribution of species (Zhang et al., 2019). Among them, climate has an immediate and profound impact on geographical distribution and population density (Bellard et al., 2012; Bystriakova et al., 2014). The influence of different climates on species distribution has been used to clarify the relationship between the potential habitat of species and climatic conditions and the origin and evolution of species, which is of great significance for the utilization of species and the prediction of potential geographical distribution areas (Hou et al., 2023). Niche model, a common approach to explore the plant-environment and plant-climate interactions, is suitable for analyzing the distribution range and spread tendency of plants (Siller-Clavel et al., 2022). Based on the known distribution data of species and related environmental variables, a model can be constructed by a specific algorithm to evaluate the ecological needs of species, and the calculation results can be projected to a specific time and space to predict the actual and potential distribution areas (Beckerman et al., 2002; Phillips et al., 2006; Phillips and Dudı́k, 2008; Kong et al., 2019; Li et al., 2019; Liao et al., 2020; Qin et al., 2022; Li et al., 2024). This method has been widely used in the prediction of potential distribution area of species, biodiversity conservation, and prediction of invasive areas of alien species under climate change (Wang et al., 2008; Liu et al., 2016; Wang and Yaermaimaiti, 2022). Nowadays, MaxEnt, CLIMEX, DOMAIN, and BIOCLIM models have been widely used, and the MaxEnt model is the most representative and widely used ecological niche model (Zhu et al., 2013; Zhang et al., 2023). MaxEnt models determine the stable relationship between species and environment by calculating the state parameters with maximum entropy in the species-environment interaction system and deduces the suitable distribution of species (Niu et al., 2017; Fu et al., 2023). It can effectively deal with the complex interaction relationship between variables, and its prediction performance is excellent (Sun et al., 2018; Liu et al., 2020; He et al., 2021).
As one of the three medicinal Glycyrrhiza species recorded in the Chinese Pharmacopoeia (Editorial Committee of Flora of China and Chinese Academy of Sciences, 1998), G. inflata is mainly distributed in the Tarim Basin in southern Xinjiang and Turpan-Hami Basin in eastern Xinjiang, and sporadically distributed in Dunhuang, Gansu province (Li et al., 2015). Apart from the strong cold- and drought-resistance, this species also has a strong saline-alkali resistance. Therefore, it is an ideal plant to be used to improve the saline-alkali soil (Wang et al., 2022). In addition, G. inflata can be used to expel phlegm, relieve cough, detoxify, and treat parasitic malaria and leishmaniasis in traditional Chinese medical science (Lu, 2015). Glycyrrhiza plants are widely distributed in the temperate desert and the major river basins in Xinjiang, and its habitats are also the first choice for agricultural production. Therefore, from the 1850s to the 1990s, with the large-scale land reclamation in Xinjiang and the extensive collection of wild Glycyrrhiza plants, the distribution area of wild Glycyrrhiza plants reduced by 50%, and the reserves reduced by 75%. Subsequently, since the 1990s, Xinjiang’s cultivated land area was expanded again, reaching 54.29% of the total area of Xinjiang (He et al., 2018), resulting in a rapid decrease in the wild Glycyrrhiza population. G. inflata was listed as a key protected plant in China in 2022 (Wang et al., 2022). Habitat protection and artificial planting are important ways to protect Glycyrrhiza plants and meet the market needs, respectively (Jia et al., 2019).
At present, many studies focus mainly on G. inflata’s pharmacology and chemical composition (Ablat et al., 2016; Guo et al., 2022; Zeng et al., 2023; Zhurinov et al., 2023). There has been a growing interest in the DIVA-Gis and MaxEnt models for predicting the potential distribution areas of Glycyrrhiza populations. Some scholars reported that climate change might lead to an increase in suitable habitats for some Glycyrrhiza species in the future, including G. inflata (Huang et al., 2023). In addition, Li et al. (2022) reported that the potential highly suitable habitat for Glycyrrhiza plants would decrease, and suitable area fragmentation would occur, with a steadily increasing trend. Currently, it is important to achieve a win-win relationship between Glycyrrhiza plant resource protection and utilization. However, the influencing factors affecting the spatial distribution of G. inflata are not clear. Therefore, to clarify the distribution of G. inflata and its response to future climate change, based on the existing field specimen collection points of G. inflata, this study used the MaxEnt model to predict the potential distribution areas in the past (last glacial maximum, the middle Holocene), contemporary, and future (RCP2.6-2050), and the ArcGIS software to determine the suitable area and distribution center (Booth et al., 2014). The Jackknife test, response curve and environmental limiting factor research were conducted to determine the climate limiting factors and suitable climatic conditions for G. inflata. The objectives of this study were: (i) To predict the potentially suitable area of G. inflata in China under current climate conditions; (ii) to analyze the main environmental factors which influence the geographical distribution of G. inflata; and (iii) to predict and compare the potentially suitable area and the change trends of G. inflata under different climate conditions in the past and future. This study will provide a scientific basis for the development and utilization of G. inflata resources.
Moat of the data on G. inflata distribution were collected by field surveys. and the latitude and longitude were determined by a GPS locator and recorded. A small amount of distribution data (10.83%) were downloaded from Global Biodiversity Information Network (http://www.gbif.org), Kunming Plant Research Institute Specification Database (http://kun.kingdonia.org), and Chinese Plants (http://frps.iplant.cn). After deleting redundant data, a total of 130 distribution points (Figure 1) were used for later analyses.
The data for a total of 67 climate variables (Table 1) were downloaded from the WorldClim database (http://www.worldclimorg). The soil data were downloaded from Harmonized World Soil Database v 1.2 (Wieder et al., 2014), Food and Agriculture Organization of the United Nations (http://www.fao.org/home/en/). The elevation data were obtained by downloading a 30 m-resolution DEM from the geospatial data cloud platform of Computer Network Information Center, Chinese Academy of Sciences (http://www.gscloud.cn). The GISMap (map based on a geographic coordinate system that is downloaded in real time from special online map services, such as OpenStreetMap) was used to cut climatic layers and convert them into ASC format. The current climatic variables used in the study were downloaded from the World Climatic Database and the spatial resolution of layers was 5 arc (about 9.2 km), climatic data for the Last Glacial Maximum of 22 ka, the Mid Holocene (about 6ka) and the future climate (2050) were from the WorldClim database. Besides, the CCSM4 model and rcp2.6 were selected to predict the future climate (2050) scenario. To maintain the comparability of the models in the spatial and temporal dimensions, the elevation and soil factors were unchanged in the prediction of future geographic distribution. The GCS_WGS_1984 projection coordinate system was adopted in this study.
In total, 80 environmental variables, including 67 climate variables, 12 soil variables, and altitude, were selected. Since collinearity between variables would lead to over-fitting of the distribution prediction model, principal component analysis and Spearman correlation analysis (Figure 2) were used to select variables with a correlation coefficient less than 0.8, and the variable with the largest contribution to the model was retained among the two variables with a correlation greater than 0.8 (Sun et al., 2023). After filtering, eight environmental factors were selected for model prediction including four climate factors (bio11, bio12, bio19, and Prec12) three soil factors (S-Esp, S-OC, and S-SAND), and one topographic factor (Alt).
The MaxEnt default parameter was used for modeling. The regularization multiplier (RM) and feature combination (FC) parameter (Cobos et al., 2019) of the MaxEnt model were adjusted by using the ENMTools package. The complexity of the model under various parameter conditions was analyzed, and the model parameters with the lowest complexity were selected. Then, the response curve and prediction results were comprehensively analyzed. Finally, the influence of the MaxEnt model complexity closely related to the RM and FC parameters on the prediction results was analyzed. There were five features in MaxEnt, namely linear (linear-L), quadratic (quadratic-Q), fragmentation (hinge-H), product (product-P), and threshold (threshold-T). The ENMTools package can evaluate the complexity of the model by testing the modified Akaike information criterion correction (AICc) value of the MaxEnt model under different parameter conditions. The AIC is a standard to measure the fitting ability of the model, which can balance the complexity and the fitting ability of the model. AIC information quantity criterion gives priority to the model with the minimum AIC value. The AIC value can be calculated by the lambdas file generated after running the MaxEnt model. The RM was set at 0.1-4, increasing by 0.1 each time. A total of 31 feature combinations (FC) were used to evaluate the fitting effect of MaxEnt model on the distribution data of G. inflata. Three-fourths of all distribution points were randomly selected for model construction and optimization, and the remaining points were used for the model test. MaxEnt models were compared and evaluated mainly by response curve and omission rate curve (Wang et al., 2023), and delta AIC was used to test model complexity and degree of fit. Then, the optimal combination for MaxEnt model operation was determined.
Based on AIC information criterion, when the RM of MaxEnt was 2 and the operating features were L and P, there was a minimum AIC value (Figure 3). So, the parameters were selected as the optimal parameter combination to construct MaxEnt model for predicting G. inflata distribution.
Figure 3 Optimal parameter combination for the MaxEnt model for predicting Glycyrrhiza inflata distribution.
The distribution point data of G. inflata were input, and the cropped environmental factors were layered into MaxEnt software. Three-fourths of the total points were randomly selected for modeling and 25% of the total points were used to verify the model. The maximum number of iterations was 1000, and the Bootstrap was 10. Response curves were plotted, and the jackknife method was used to analyze the relationship between environmental factors and G. inflata distribution. According to the suitability value, ArcGIS software was used to divide the distribution areas predicted by the constructed model into the following four grades: non-suitable areas (fitness value: 0%-20%), general suitable area (fitness value: 20%-50%), moderately suitable areas (fitness value: 50%-70%), and highly suitable areas (fitness value: 70%-100%). The receiver operating curve value was applied to evaluate the accuracy of the model (Zhang et al., 2020). The ArcGIS (Mondal et al., 2022) was used to narrow the distribution range of suitable areas in different periods to a single central point, and then the changes of distribution centers in different periods were calculated to analyze the evolution trend of G. inflata distribution.
The ROC curve was obtained by simulating the training set through the built-in function of MaxEnt software, and the area under the curve was the value of AUC. The AUC value was 0.986, and the standard deviation was ±0.002 (Figure 4). This indicates that the models had a good stability. According to the evaluation criteria (Liu et al., 2016), the overall prediction accuracy of the model reached an excellent level. This indicates that the model has a high accuracy in predicting the potential suitable areas for G. inflata.
Figure 4 ROC curve of the distribution of G. inflata predicted by MaxEnt model. AUC, Area under curve; ROC, Receiver operating characteristic.
The analysis results of climate variables of G. inflata (Figure 5) showed that there were eight major environmental factors: annual average precipitation(bio12), subsoil sodicity (S-Esp), subsoil sand fraction (S-SAND), precipitation in December (Prec12), average precipitation in the coldest season (bio19), average temperature in the coldest season (bio11), subsoil organic carbon (S-OC), and altitude(Alt). Among them, bio12, Prec12, and bio19 were precipitation factors; S-Esp, S-SAND, and S-OC were soil factors; bio11 was a temperature factor; and Alt was a topographic factor. If a single variable was used for model prediction (Cai et al., 2022), the normalized gain value, test gain value, and AUC value of average annual precipitation were the highest. The test gain value of average annual precipitation was greater than 2.1, the normalized gain value was greater than 1.9, and the area under ROC curve was greater than 0.95. Therefore, the average annual precipitation is the main climatic limiting factor affecting the distribution of G. inflata.
Figure 5 Jackknife test of the importance of environment variables in MaxEnt model. (S-SAND, subsoil sand fraction; S-OC, subsoil organic carbon; S-Esp, subsoil sodicity; Prec12, precipitation in December; bio19, average precipitation in the coldest season; bio12, average annual precipitation; bio11, average precipitation in the coldest season; alt, altitude).
The area of suitable habitats (Figure 6C) for G. inflata in modern times decreased by 401,126.1 km2 compared with that in the last glacial maximum (Figure 6A). The range of suitable habitats in modern times shrank in eastern Xinjiang, and the suitable habitat center moved to the vicinity of Lop Nur Town, Xinjiang. However, the area of highly suitable habitats in modern times decreased by 125,174.1 km2 compared with that in the last glacial maximum (31.2% of the current), and the highly suitable areas, such as Turpan-Hami Basin in Xinjiang, had little shrinkage, but the area around Tarim Basin in southern Xinjiang had obvious shrinkage. There was also a small area of highly suitable habitats in Alxa League of Inner Mongolia. This indicates the eastward spread of the G. inflata population. The moderately suitable area for G. inflata in modern times greatly reduced by 377,922.7 km2 (−125.8% of the current) compared with that in the last glacial maximum. The moderately suitable area in the Tarim Basin in Xinjiang and northern Inner Mongolia in modern times showed a reduction, while the moderately suitable area in Haixi of Qinghai Province was almost unchanged, compared with those in the last glacial maximum. The suitable area for modern G. inflata in modern times increased by 101,970.7 km2 (9.0% of the current) compared with that in the last glacial maximum, distributing in the Tarim Basin of Xinjiang. Most of the moderately suitable areas in northern Inner Mongolia during the last glacial maximum changed into general suitable areas in modern times.
Figure 6 Spatial distribution of Glycyrrhiza inflata under climate change scenarios. (A) Potential distribution areas in the last glacial maximum. (B) Potential distribution area in the middle Holocene. (C) Potential distribution area in modern times. (D) Potential distribution area in RCP2.6-2050.
The range of suitable habitats in modern times (Figure 6C) increased by 53,252.3 km2 compared with that in the middle Holocene (Figure 6B). The distribution of suitable habitats shifted slightly to the southwest, and the center shifted slightly to the southwest in Lop Nur Town, Xinjiang. However, the highly suitable area in modern times decreased by 31,115.7 km2 (7.8% of the current) compared with that in the middle Holocene, which mainly appeared in the southern Tarim Basin of Xinjiang, with sporadic reduction in Hotan and Kashgar. Compared with the middle Holocene, the moderately suitable area of G. inflata in modern times increased by 4,089.5 km2(1.4% of the current), mainly in Turpan and Xinjiang, compared with that in the middle Holocene, but reduced in the central Tarim Basin. The suitable area for G. inflata in modern times increased by 80,278.6 km2 (7.1% of the current) compared with that in the middle Holocene. The increase was mainly detected in the middle of the Tarim Basin, where the moderate suitable area shifted into the general suitable area.
The range of suitable habitats for G. inflata (Figure 6D) in 2050 reduced by 22,936.7 km2 (1.3% of the current) compared with that in modern times (Figure 6C), and the range of suitable habitats in 2050 shifted to the southwest. The highly suitable area in 2050 decreased by 150,600.1 km2 (mainly in the Turpan and Hami of Xinjiang, 60.0% of the current) compared with that in modern times. The moderately suitable area for G. inflata in 2050 increased by 35,916.4 km2 (10.7% of the current) compared with that in the modern times due to the highly suitable areas in Turpan and Hami of Xinjiang transforming to moderately suitable areas. However, the moderately suitable area in the central Tarim Basin mainly transformed to a general suitable area. The general suitable area in 2050 increased by 91,746.9 km2 (7.5% of the current) compared with that in modern times, due to the moderately suitable areas transforming to general suitable areas.
The predicted results of suitable areas from the last glacial maximum to 2050 showed that the total suitable area for G. inflata decreased significantly until the middle Holocene and then changed little (Figure 7). The suitable areas in modern times reached 1,831,026 km2. The general suitable areas increased gradually. This is due to the conversion of moderately suitable and highly suitable areas to general suitable areas. In the middle Holocene, the moderately suitable areas decreased greatly and then increased slightly, but the highly suitable areas gradually decreased, especially by 2050. In 2050, the potential suitable area for G. inflata in China will be 1,808,090 km2, of which the highly suitable areas will be 250,970 km2, which is 150,600 km2 smaller than that in modern times, with a reduction rate of 60.0%. Therefore, there is a trend of great reduction in the suitable areas for G. inflata.
Figure 7 The potential suitable area of Glycyrrhiza inflata in different climate scenarios. LGM, Last glacial maximum; MID, Middle Holocene.
In the last glacial maximum, the distribution center of G. inflata was in the western margin of the Kumtag Desert, Xinjiang, and moved southwest along the Aqik Valley (40.2037° N, 91.9738° E) (Figure 8). By the middle Holocene, the distribution center was in the southeast of Lop Nur Town of Xinjiang (39.9072° N, 90.1943° E), and moved further southeast. Under modern climate conditions, the distribution center continued to move southwest along the paleochannel for more than 10 kilometers (39.833° N, 90.1201° E). In the future climate scenario (RCP2.6-2050), the distribution center would move to the southwest of Ruoqiang County (39.7589° N, 89.9719° E). In general, the distribution center of G. inflata continued to move to the southern margins of the Kumtag Desert in Xinjiang.
Figure 8 Major shifts in the distribution of suitable areas for G. inflata under different climate scenarios.
The changes in the distribution center of G. inflata showed that the distribution center of G. inflata moved to the southwest significantly from the last glacial maximum to the middle Holocene. After the middle Holocene, the temperature increased, the glaciers in the middle and high latitude mountains melted, and the climate became warm and humid. However, the distribution center of G. inflata was stable in the southeast of Lop Nur Town, Xinjiang, due to its limited influence on the habitats of G. inflata (Lu et al., 2013; Niu et al., 2017). G. inflata has strong resistance to light, drought, salt, and alkali stresses; therefore, the climate change had little impact on the habitats of G. inflata in the northern margin of the Kumtagh Desert and the margin of the Taklimakan Desert. It was also found that the distribution center of G. inflata showed a continuous movement to the southwest as the global temperature continued to rise. In the future, as the global temperature continues to rise, the distribution center of G. inflata will stably move to the southwest.
At present, the models for the evaluation of suitable plant and animal habitats mainly include niche model (Xu et al., 2015; Grev et al., 2019; Wu et al., 2022; Zhang et al., 2022), mechanism model, and regression model. Among them, the niche model pays more attention to species occurrence sites and environmental variables. To some extent, the MaxEnt model is a representative of the niche model (Wang et al., 2008; Lin et al., 2017). According to the normalized gain value, contribution rate, and single factor response curve in the results obtained by the optimized MaxEnt model, the main environmental factors affecting the distribution of G. inflata are average annual precipitation (bio12) and precipitation in December (Prec12). Precipitation especially is the main environmental factor affecting the distribution of G. inflata. G. inflata is only distributed in arid regions of Central Asia such as Uzbekistan, Turkmenistan, Kyrgyzstan, Tajikistan, and the arid areas of northwest China (West of Hexi Corridor in Gansu Province, Turpan-Hami Basin in eastern Xinjiang, Tarim Basin in southern Xinjiang). It can be seen that G. inflata can adapt to the arid environment. Huang et al. found that the precipitation in the driest month (bio14) had a significant effect on the distribution of Glycyrrhiza in China (Huang et al., 2023). This is similar to the results obtained in this study, indicating that precipitation is an important factor affecting the distribution of Glycyrrhiza under global climate change. In this study, the main precipitation variables that affected the distribution of G. inflata were the precipitation in December (32.9%) and the average annual precipitation (29.6%), and the main temperature variable was the average temperature in the coldest season (2.1%). This may be due to the fact that seeds of G. inflata are small, hard, and resistant to water stress (Zhou et al., 2017). After ripening in October, stratification is needed for winter, and soil moisture and temperature are very important for the germination of G. inflata seeds during the snowmelt season in the following year (Wang and He, 2004; Yang et al., 2016). G. inflata is a perennial herb with strong underground rhizomes, through which G. inflata propagated asexually (Wang and He, 2004). The annual precipitation in the Taklamakan Desert is only 20-70 mm, and plants can only grow if they can obtain sufficient water from groundwater, lakes, or rivers (Bruelheide et al., 2010). The lack of rainfall and the low groundwater table make it difficult for G. inflata survive, while too much water causes root rot and even death. Therefore, the clonal reproduction and growth of G. inflata are affected by the average annual precipitation (Zhang and Wang, 2005). From the distribution altitude of G. inflata, it can be seen that there is almost no G. inflata at high altitudes (more than 2000 meters) where there is a lack of heat.
The credibility of the species distribution model to simulate the potential distribution area of species is closely related to the number and distribution range of species samples (Araujo et al., 2005). In this study, the data on valid geographical distribution points obtained from field investigations were used to predict the past, present, and future distribution of G. inflata. In modern times, the suitable areas for G. Inflata were mainly distributed around the Tarim Basin, Tarim River valley, and Konqi River valley in Xinjiang, and Guazhou, Jinta, and Dunhuang in Gansu Province. Suitable areas for G. Inflata were also scattered in the Ejin River basin of Inner Mongolia (Wang et al., 2021). From the perspective of the climate zone, the suitable areas for G. Inflata were concentrated in the middle-temperate arid zone. This is consistent with the actual distribution. In Qaidam Basin of Qinghai Province, there were middle-height suitable areas but no distribution records. This may be related to the high altitude and low temperatures, which are not suitable for the heliophilic properties of G. inflata. The prediction results showed that, in 2050, the total suitable area for G. inflata would decrease and the habitat fragmentation would be serious, especially in Turpan-Hami Basin. This may be related to the exacerbating water shortage in this region in the future (Zhang and Zhang, 2013). In addition, it was also found that the suitable areas were degraded, that is, the highly suitable areas were transferred to moderately and generally suitable areas in a large area, and most suitable areas were degraded to low and non-suitable areas. This is similar to the study results of Ephedra equisetina (Rong et al., 2023), Sabina centrasiatica (Wang et al., 2022), Populus xjrtyschensis (Wang et al., 2022), Kalidium (Liu et al., 2022), and Allium mongolicum (Lang et al., 2023). This may be due to that the increase of CO2 emissions and human interference such as excessive wild plant collection and land reclamation, which aggravates the habitat fragmentation of G. inflata and leads to the reduction of suitable areas. Yang et al. also verified this point by studying the genetic structure of the G. inflata population (Yang et al., 2016). This study found that the total habitat area of G. inflata showed a gradually decreasing trend. This may be due to the fact that, under the future climate scenario, the habitats of some species will continue to decrease (Wang et al., 2008; Wang and Yaermaimaiti, 2022), and these species will migrate to high latitude and altitude, along with the fragmentation of habitats (Li et al., 2022). However, high altitude and low temperature are limiting factors for G. inflata growth. Therefore, an overall decreasing trend was predicted in the future in this study. This result is contrary to Huang’s (Huang et al., 2023). This may be due to a slight increase in precipitation in arid areas due to global warming and glacier melting. However, in arid areas, temperature and evaporation increases, so a slight increase in precipitation can hardly offset the negative effect of temperature rise. This will eventually lead to the degradation of natural vegetation in deserts. Therefore, the prediction of suitable areas for plants in arid areas considering only precipitation difficult.
The climatic change in the Quaternary Ice Age has a great influence on the distribution of species. According to the results of model prediction, the distribution center of G. inflata moved to the southwest significantly from the last glacial maximum to the middle Holocene. due to the extensive existence of glaciers in the Tibetan Plateau, Kunlun Mountains, Altun Mountain, and Qilian Mountains in the last glacial maximum (Zhu and Qiao, 2016). The cooling of the climate led to the migration of G. inflata from north to south (northern margin of Kumtag Desert). From the mid-Holocene to the future, the change trend of distribution center tended to be stable, but the distribution center showed a trend of continuous southwest movement. This may be related to the continuous southwest shrinkage of the suitable areas for G. inflata, especially the severe shrinkage in the Turpan-Hami Basin. There is no river in the Turpan-Hami Basin. The water storage is small, the precipitation is low, and the evaporation is large, coupled with the excessive use of groundwater by human beings (Fang et al., 2022); therefore, water is the main limiting factor that makes this area gradually unsuitable for the growth of G. inflata. These results are consistent with those of Zhang et al. (2019).
G. Inflata is widely distributed in arid areas. However, according to this study results, its suitable area will shrink in the future, and the natural population degradation is serious, so it is particularly important to protect the germplasm resources of G. inflata. Considering the distribution characteristics, the change in highly suitable areas in the future, and the stable southeast movement of the distribution center (in the Lop Nur Town in Xinjiang), it is suggested to set up habitat protection zones in the Tarim Basin (Shaya County in north Aksu, Qira county in southern Hotan, east of Korla City, and south of Lop Nur Town), Turpan-Hami Basin (Daheyan in Turpan, Daquanwan in Hami), and Dunhuang in Gansu Province. This is consistent with the conservation recommendations based on the population genetic structure of Yang et al. (2016). According to the field survey results, it was found that there was still a certain G. Inflata distribution in the above areas, and the areas with less human activity can be prioritized as protected areas to maintain its natural habitats. In Daheyan in Turpan, Daquanwan in Hami, Mogao Town in Dunhuang, and Yangtak in Qiemo, G. inflata grows in deserts or salinized deserts. The G. Inflata population is gradually reducing. Therefore, in these places, in situ protection and scientific management is the best choice to preserve the species.
The hinterlands of rivers and tributaries in the arid zone, the main habitats for G. inflata, have been reclaimed as farmlands. G. inflata is considered a weed in canal banks or roadsides; habitat fragmentation and hybridization are serious. If a large area of farmland is restored to grassland, the cost will be high. At present, various Chinese herbal germplasm gardens and botanical gardens have been established successively. G. inflata has been planted in the Turpan Desert Botanical Garden, but the population size is very small. On the one hand, the existing facilities can be expanded; on the other hand, other highly suitable areas can be selected to increase the number of breeding bases to protect G. inflata germplasm resources and genetic homozygosity.
G. inflata is the most salt- and drought-tolerant medicinal species of the genus Glycyrrhiza; therefore, G. inflata should be prioritized when considering the maintenance of the ecology of harsh desert habitats. In this study, the MaxEnt model was used to simulate past, present, and future (2050) suitable areas for G. inflata. The findings were as follows: (1) The primary climatic factor influencing the geographic distribution of G. inflata was the average annual precipitation; (2) The Turpan-Hami Basin, Tarim Basin, and Dunhuang of Gansu Province were the main suitable areas for G. inflata in modern times, with Lop Nur Town in Xinjiang as the distribution center; (3) The potential highly suitable areas for G. inflata showed a continuous large-scale reduction trend in the past, present, and future; (4) The geographical distribution center of G. inflata shifted to the southwestern margins of the Kumtag Desert in Lop Nur Town, Xinjiang, and would continuously move southwest. The study results will provide a basis for future research for the conservation, breeding, and development of G. inflata for medical purposes. Long-term research is still necessary to support the scientific protection of G. inflata populations.
Publicly available datasets were analyzed in this study. Climate variables: WorldClim database, http://www.worldclim.com/. Soil factor data: Harmonized World Soil Database, https://www.fao.org/soils-portal/soil-survey/soil-maps-and-databases/harmonized-world-soil-database-v12/en. Elevation variables: Geospatial Data Cloud Platform of Computer Network Information Center, https://www.gscloud.cn/.
GH: Writing – review & editing. Z-ZD: Conceptualization, Funding acquisition, Methodology, Software, Writing – original draft. W-BX: Conceptualization, Methodology, Writing – original draft. Y-XW: Supervision, Writing – review & editing. PY: Methodology, Writing – review & editing. Z-CM: Methodology, Writing – review & editing. H-BL: Writing – review & editing.
The author(s) declare financial support was received for the research, authorship, and/or publication of this article. This study was supported by the National Natural Science Foundation of China (Grant number: 31470191), the New Variety Cultivation Project of Shihezi University (Grant number: YZZX202106; ZZZC2022122; XPRU202104), the Science and Technology Project of Xinjiang Production and Construction Corps (Grant number: 2023AB052), and the Science and Technology Project of Huyanghe City (Grant number: QS2023008).
We would like to thank Dr. Shamshidin Abduriyim for his comments on an earlier version and the English of our manuscript.
The authors declare that the research was conducted in the absence of any commercial or financial relationships that could be construed as a potential conflict of interest.
All claims expressed in this article are solely those of the authors and do not necessarily represent those of their affiliated organizations, or those of the publisher, the editors and the reviewers. Any product that may be evaluated in this article, or claim that may be made by its manufacturer, is not guaranteed or endorsed by the publisher.
The Supplementary Material for this article can be found online at: https://www.frontiersin.org/articles/10.3389/fevo.2024.1347066/full#supplementary-material
Ablat S., Xuchao Y., Ablise M., Bingzhao R. (2016). Determination of licochalcone A in whole plant of Xinjiang Glycyrrhiza inflate by HPLC. Northwest Pharm. J. 31, 130–132. doi: 10.3969/j.issn.1004-2407.2016.02.006
Araujo M. B., Pearson R. G., Thuiller W., Erhard M. (2005). Validation of species-climate impact models under climate change. Global Change Biol. 11, 1504–1513. doi: 10.1111/j.1365-2486.2005.01000.x
Beckerman A., Benton T. G., Ranta E., Kaitala V., Lundberg P. (2002). Population dynamic consequences of delayed life-history effects. Trends Ecol. Evol. 17, 263–269. doi: 10.1016/S0169-5347(02)02469-2
Bellard C., Bertelsmeier C., Leadley P., Thuiller W., Courchamp F. (2012). Impacts of climate change on the future of biodiversity. Ecol. Lett. 15, 365–377. doi: 10.1111/j.1461-0248.2011.01736.x
Booth T. H., Nix H. A., Busby J. R., Hutchinson M. F. (2014). The first species distribution modelling package, its early applications and relevance to most current MAXENT studies. Diversity Distributions 20, 1–9. doi: 10.1111/ddi.12144
Bruelheide H., Vonlanthen B., Jandt U., Thomas F. M., Foetzki A., Gries D., et al. (2010). Life on the edge - to which degree does phreatic water sustain vegetation in the periphery of the Taklamakan Desert? Appl. Vegetation Sci. 12, 56–71. doi: 10.1111/j.1654-109X.2009.01050.x
Bystriakova N., Ansell S. W., Russell S. J., Grundmann M., Vogel J. C., Schneider H. (2014). Present, past and future of the European rock fern Asplenium fontanum: combining distribution modelling and population genetics to study the effect of climate change on geographic range and genetic diversity. Ann. Bot. 113, 453–465. doi: 10.1093/aob/mct274
Cai M., Yuan Y., Yuan J., Chen C., Yu J., Wang X. (2022). Authentic environmental factors analysis of Vitex rotundifolia based on maxEnt model. J. Chin. Medicinal Materials 45, 2065–2070. doi: 10.13863/j.issn1001-4454.2022.09.009
Cobos M. E., Peterson, A. T., Barve N., Osorio-Olvera L. (2019). kuenm: an R package for detailed development of ecological niche models using MaxEnt. PeerJ. 7, e6281. doi: 10.7717/peerj.6281
Editorial Committee of Flora of China, Chinese Academy of Sciences (1998). Flora of China Vol. 42 (Beijing: Science Press), 172.
Fang J., Chen L., Liu L., Liu Y. (2022). The cause of degradation of the oasis wetland in the Aiding Lake Basin and the prediction of its development trend. Ground Water 44, 91–93. doi: 10.19807/j.cnki.DXS.2022-01-025
Fu A., Gao E., Tang X., Liu Z., Hu F., Zhan Z., et al. (2023). MaxEnt modeling for predicting the potential wintering distribution of eurasian spoonbill (platalea leucorodia) under climate change in China. Animals 13, 856. doi: 10.3390/ani13050856
Grev M. E., Houadria M., Andersen A. N., Menzel F. (2019). Niche differentiation in rainforest ant communities across three continents. Ecol. Evol. 9, 8601–8615. doi: 10.1002/ece3.5394
Guo C., Su Y., Wang H., Cao M., Diao N., Liu Z., et al. (2022). A novel saponin liposomes based on the couplet medicines of platycodon grandiflorum–Glycyrrhiza uralensis for targeting lung cancer. Drug Delivery 29, 2743–2750. doi: 10.1080/10717544.2022.2112997
He K., Wu S., Yang Y., Wang D., Zhang S., Yin N. (2018). Dynamic changes of land use and oasis in Xinjiang in the last 40 years. Arid Land Geogr. 41, 1333–1340. doi: 10.12118/j.issn.1000-6060.2018.06.21
He P., Li Y., Zhu W., Meng F. (2021). MaxEnt model analysis of habitat suitability in Chuanxiong under climate change. Lishizhen Medicineand Materia Med. Res. 32, 3005–3009. doi: 10.3969/j.issn.1008-0805.2021.12.54
Hou J., Xiang J., Li D., Liu X. (2023). Prediction of potential suitable distribution areas of quasipaa spinosa in China based on MaxEnt optimization model. Biology 12, 366. doi: 10.3390/biology12030366
Huang S., Zhang W., Hong Z., Yuan Y., Tan Z., Wang Y., et al. (2023). Geographic distribution and impacts of climate change on the suitable habitats of glycyrrhiza species in China. Environ. Sci. pollut. Res. Int. 30, 55625–55634. doi: 10.1007/s11356-023-26232-w
Jia X., Wang C., Jin H., Zhao Y., Liu L., Chen Q., et al. (2019). Assessing the suitable distribution area of Pinus koraiensis based on an optimized MaxEnt model. Chin. J. Ecol. 38, 2570–2576. doi: 10.13292/j.1000-4890.201908.017
Kong W., Li X., Zou H. (2019). Optimizing MaxEnt model in the prediction of species distribution. Chin. J. Appl. Ecol. 30, 2116–2128. doi: 10.13287/j.1001-9332.201906.029
Lang X., Fan R., Li Q. (2023). Analysis of potential suitable areas of Allium mongolicum in Northern China. Acta Agrestia Sin. 31, 3525–3534. doi: 10.11733/j.issn.1007-0435.2023.11.031
Li X., Lu J., Yan P., Zhang F. (2015). Taxonomy and experimental biology of the genus glycyrrhiza L (Shanghai: Fudan University Press).
Li S., Mo S., Hu X., Deng T. (2024). Prediction of potential suitable areas of endangered plant Abies ziyuanensis based on MaxEnt and ArcGIS. Chin. J. Ecol. 43(2), 533–541. doi: 10.13292/j.1000-4890.202402.004
Li Q., Wang X., Yan K., Liang Z., Xia P. (2022). Based on multiple environmental factors to explore the habitat distribution of licorice (glycyrrhiza uralensis) in different time and space. Biochem. Systematics Ecol. 105, 104490. doi: 10.1016/j.bse.2022.104490
Li W., Xu Z., Shi M., Chen J. (2019). Prediction of potential geographical distribution patterns of Salix tetrasperma Roxb. in Asia under different climate scenarios. Acta Ecologica Sin. 39, 3224–3234. doi: 10.5846/stxb201803020413
Liao J., Yi Z., LI S., Xiao L. (2020). MaxEnt modeling for predicting the potentially geographical distribution of Miscanthus nudipes under different climate conditions. Acta Ecologica Sin. 40, 8297–8305. doi: 10.5846/stxb201911092361
Lin Y., Xu X., Yi J., Lu Z. (2017). Potential predation and conservation vacancy analysis of Syrmaticus humiae in guangxi based on maxEnt model. Sichuan J. Zoology 36, 328–333. doi: 10.11984/j.issn.1000-7083.20170017
Liu X., LI J., Zhao C., Quan Z., Zhao X., Gong L. (2016). Prediction of potential suitable area of Ambrosia artemisiifolia L. in China based on MaxEnt and ArcGIS. J. Plant Prot. 43, 1041–1048. doi: 10.13802/j.cnki.zwbhxb.2016.06.023
Liu J., Liu Y., Zhang J., Wen Y., Chu G. (2022). Analysis of the potential distribution and suitability of five Kalidium species. Pratacultural Sci. 39, 133–148. doi: 10.11829/j.issn.1001-0629.2021-0308
Liu P., Wang L., Du Q., Du L. (2020). Estimation of potential suitable distribution area and the ecological characteristics of Eucommia ulmoides Oliv. in China. Acta Ecologica Sin. 40, 5674–5684. doi: 10.5846/stxb201907091450
Lu J. (2015). Salt tolerant mechanisms of three Glycyrrhiza Species. (Doctor) (Shihezi: Shihezi University).
Lu X., Lu J., Wu L., Li X. (2013). Germination responses of three medicinal licorices to saline environments and their suitable ecological regions. Acta Prataculturae Sin. 22 (2), 195–202.
Mondal B. K., Sahoo S., Das R., Mishra P. K., Abdelrahman K., Acharya A., et al. (2022). Assessing groundwater dynamics and potentiality in the lower ganga plain, India. Water 14, 2180. doi: 10.3390/w14142180
Niu Q., Lu J., Zhang A., Song F. (2017). ). A preliminary study on the symplast pathway of Na+ uptake in Glycyrrhiza inflate under different salinity stress. J. Shihezi Univ. 35, 493–498. doi: 10.13880/j.cnki.65-1174/n.2017.04.017
Phillips S. J., Anderson R. P., Schapire R. E. (2006). Maximum entropy modeling of species geographic distributions. Ecol. Model. 190, 231–259. doi: 10.1016/j.ecolmodel.2005.03.026
Phillips S. J., Dudík M. (2008). Modeling of species distributions with MaxEnt: new extensions and a comprehensive evaluation. Ecography 31, 161–175. doi: 10.1111/j.0906-7590.2008.5203.x
Qin W., Zhang H., Yang M., Luo H., Jiang S., Liu S. (2022). Study on Potential Distribution of Rubia argyi (Levl. et Van.) Hara ex L. A. Lauener et D. K. Based on MaxEnt Model and ArcGIS. Chin. Journalof Inf. On Traditional Chin. Med. 29, 1–4. doi: 10.19879/j.cnki.1005-5304.202111453
Rong W., Huang X., Niu P., Liu J., Yan R., Su J., et al. (2023). Potentially suitable areas for traditional Chinese medicinal material Ephedra equisetina based on MaxEnt model. Acta Ecologica Sin. 43, 8631–8646. doi: 10.20103/j.stxb.202209162641
Siller-Clavel P., Badano E. I., Villarreal-Guerrero F., Prieto-Amparán J. ,. A., Pinedo-Alvarez A., Corrales-Lerma R., et al. (2022). Distribution patterns of invasive buffelgrass (cenchrus ciliaris) in Mexico estimated with climate niche models under the current and future climate. Plants 11, 1160. doi: 10.3390/plants11091160
Sun R., Tong G., Zhang Q., Xu L., Sang Z., Li Y. (2023). A study on the suitable areas for growing apricot kernels in China based on the MaxEnt model. Sustainability 15, 9635. doi: 10.3390/su15129635
Sun L., Wang S., Wang Z. y., Feng F. (2018). Ecologicl suitable prediction of lyciumbarbarumL. Based on maximum entropy model. J. NingxiaUniversity 39, 143–147.
Wang Y., He R. (2004). Analysis on Glycyrrhiza uralensis Fisch and soil desertification. Chin. J. Eco-Agriculture 12 (3), 194–195.
Wang Q., Lu J., Zhang J., Xu Y., Xu K., Zhang J., et al. (2022). Soil microbial community structure and its influencing factors in original habitat of Glycyrrhiza inflata in different distribution areas. Acta Ecologica Sin. 42, 9780–9795.
Wang L., Parhat A., Li L., Tohti J., Liu Z. (2021). Genetic diversity ISSR analysis of Glycyrrhiza inflata from Xinjiang. Chin. traditional herbal Drugs 52, 6975–6982. doi: 10.7501/j.issn.0253-2670.2021.22.024
Wang Y., Xie L., Zhou X., Chen R., Zhao G., Zhang F. (2023). Prediction of the potentially suitable areas of leonurus japonicus in China based on future climate change using the optimized MaxEnt model. Ecol. Evol. 13, e10597. doi: 10.1002/ece3.10597
Wang X., Xu W., Ouyang Z., Liu J., Xiao Y., Chen Y., et al. (2008). The application of Ecological-Niche factor analysis in giant pandas (Ailuropoda melanoleuca) habitat assessment. Acta Ecologica Sin. 28 (2), 821–828.
Wang L., Yaermaimaiti Y. (2022). Prediction of the potential distribution of two endemic tree species in Xinjiang of western China under future climate scenarios. J. Beijing forestry Univ. 44, 10–21. doi: 10.12171/j.1000–1522.20210301
Wieder W. R., Boehnert G. B., Bonan M. (2014). Regridded Harmonized World Soil Database v1.2. Data set (Oak Ridge, Tennessee, USA: from Oak Ridge National Laboratory Distributed Active Archive Center). Available at: http://daac.ornl.gov.
Wu X., Wang M., Li X., Yan Y., Dai M., Xie W., et al. (2022). Response of distribution patterns of two closely related species in Taxus genus to climate change since last inter-glacial. Ecol. Evol. 12, e9302. doi: 10.1002/ece3.9302
Xu Z., Peng H., Peng S. (2015). The development and evaluation of species distribution models. Acta Ecologica Sin. 35, 557–567. doi: 10.5846/stxb201304030600
Yang L., Chen J., Hu W., Yang T., Zhang Y., Yukiyoshi T., et al. (2016). Population genetic structure of Glycyrrhiza inflata B. (Fabaceae) is shaped by habitat fragmentation, water resources and biological characteristics. PloS One 11, e0164129. doi: 10.1371/journal.pone.0164129
Zeng J., Huang Y., Zhou L., Liang X., Yang C., Wang H., et al. (2023). Histone deacetylase GiSRT2 negatively regulates flavonoid biosynthesis in glycyrrhiza inflata. Cells 12, 1501. doi: 10.3390/cells12111501
Zhang T., Huang Z., Peng Y., Wang Y., Wang P., Wang S., et al. (2020). Prediction of potential suitable areas of Actinidia arguta in China based on MaxEnt model. Acta Ecologica Sin. 40, 4921–4928. doi: 10.5846/stxb201909161921
Zhang X., Jiang Y., Bi Y., Liu X., Li X., Sun T. (2022). Identification of potential distribution area for Hippophae rhamnoides subsp. sinensis by the MaxEnt model. Acta Ecologica Sin. 42, 1420–1428.
Zhang X., Li Y., Wang Y., Cai H., Zeng H., Wang Z. (2019). Influence of future climate change in suitable habitats of tea in different countries. Biodiversity Sci. 27, 595–606. doi: 10.17520/biods.2019085
Zhang Q., Shen X., Jiang X., Fan T., Liang X., Yan W. (2023). MaxEnt modeling for predicting suitable habitat for endangered tree keteleeria davidiana (pinaceae) in China. Forests 14, 394. doi: 10.3390/f14020394
Zhang D., Wang H. (2005). Preliminary study on the growth pattern of several clonal plants in desert zones of Xinjiang. Arid zone Res. 22 (2), 219–224. doi: 10.13866/j.azr.2005.02.018
Zhang H., Zhang Y. (2013). Optimal allocation of water resources in the tuha basin base on concept of circular economy of water resources. Water Conservancy Sci. Technol. Economy 19, 27–29.
Zhou Y. (2017). Physiological and bochemiical mechanism of gycyrrhiza in response to drought stress.(Doctor) (Inner Mongolia: Agricultural University).
Zhu G., Liu G., Bu W., Gao Y. (2013). Ecological niche modeling and its applications in biodiversity conservation. Biodiversity Sci. 21, 90–98. doi: 10.3724/SP.J.1003.2013.09106
Zhu G., Qiao H. (2016). Effect of the MaxEnt model’s complexity on the prediction of species potential distributions. Biodiversity Sci. 24, 1189–1196. doi: 10.17520/biods.2016265
Keywords: Glycyrrhiza inflata, MaxEnt model, potential distribution, geographical distribution center, climate factors
Citation: Du Z-z, Xu W-b, Wang Y-x, Yan P, Ma Z-c, Huang G and Li H-b (2024) Prediction of the potential distribution area of Glycyrrhiza inflata in China using a MaxEnt model. Front. Ecol. Evol. 12:1347066. doi: 10.3389/fevo.2024.1347066
Received: 30 November 2023; Accepted: 18 March 2024;
Published: 08 April 2024.
Edited by:
Hong-Hu Meng, Chinese Academy of Sciences (CAS), ChinaReviewed by:
Hong-Xiang Zhang, Chinese Academy of Sciences (CAS), ChinaCopyright © 2024 Du, Xu, Wang, Yan, Ma, Huang and Li. This is an open-access article distributed under the terms of the Creative Commons Attribution License (CC BY). The use, distribution or reproduction in other forums is permitted, provided the original author(s) and the copyright owner(s) are credited and that the original publication in this journal is cited, in accordance with accepted academic practice. No use, distribution or reproduction is permitted which does not comply with these terms.
*Correspondence: Gang Huang, SHVhbmdHYW5nQHNoenUuZWR1LmNu; Hong-bin Li, bGloYkBzaHp1LmVkdS5jbg==
†These authors have contributed equally to this work and share first authorship
Disclaimer: All claims expressed in this article are solely those of the authors and do not necessarily represent those of their affiliated organizations, or those of the publisher, the editors and the reviewers. Any product that may be evaluated in this article or claim that may be made by its manufacturer is not guaranteed or endorsed by the publisher.
Research integrity at Frontiers
Learn more about the work of our research integrity team to safeguard the quality of each article we publish.