- 1Division of Natural Sciences, New College of Florida, Sarasota, FL, United States
- 2Department of Ecology and Evolutionary Biology, Cornell University, Ithaca, NY, United States
We are nearing the 20-year anniversary of a groundbreaking paper which details how microsatellite marker development in Lepidoptera is “extremely difficult for no apparent reason.” How far have we come in these past 20 years? Microsatellites are still the marker of choice in many population genetics studies for their ease of use, high degrees of polymorphism, species-specificity, and low cost. The rise of next-generation sequencing technologies (e.g. 454, Illumina, PacBio, etc.) has greatly advanced our abilities to generate many microsatellite markers per species. In this paper, we summarize the improvements in marker development using next-generation technology. Using case studies, we review the use and implementation of microsatellite markers in different conservation programs. Lastly, we provide a guide to data interpretation of microsatellite data generated for butterflies, with the goal of supporting student researchers and conservation practitioners in evaluating the meaning in their data.
Introduction
Microsatellites have interested biologists since the 1980s (Tautz and Renz, 1984) and have proven to be a useful suite of markers for studies in population dynamics and conservation for several decades (Selkoe and Toonen, 2006). Microsatellites are short tandem repeats of DNA, typically between 1 and 6 bp long, that are produced by DNA slippage events during replication and which possess qualities that make them powerful genetic markers for conservation genetics studies: high levels of polymorphism, codominant inheritance, distribution throughout the nuclear genome, reliable genotyping, and easy amplification using polymerase chain reaction (PCR) (Vieira et al., 2016). Butterfly conservationists, like other practitioners, value these markers for their high information content and ability to address population-level questions (Saarinen, 2015). However, in contrast to their ease of use in many other taxa, the development of microsatellite markers in Lepidoptera has historically been “extremely difficult for no apparent reason” (Zhang, 2004). Quite interestingly, microsatellites in some other insects do not show the same difficulty, even when identical methods are used to isolate them (Nève and Meglécz, 2000). These studies indicate that Lepidopteran genomes (both butterflies and moths) might have lower frequencies of microsatellites compared to other taxonomic groups – even within the same Class.
Finding reliably-amplifying microsatellites in Lepidoptera is further confounded by their association with mobile elements (see Glossary of Terms). While microsatellites are associated with mobile elements in many taxa, in Lepidoptera, this association appears to produce highly similar flanking regions around multiple microsatellite loci (Zhang, 2004). This pattern presents two primary challenges for marker design. First, flanking regions that are variable across individuals or between taxa (see below) may not reliably anneal during PCR amplification, and second, flanking regions that are not unique lead to the amplification of microsatellites families rather than single-copies (Tay et al., 2010). These issues make amplification difficult, and can lead to a high rate of null alleles or extra banding patterns (which makes genotype scoring difficult to impossible) (Figure 1).
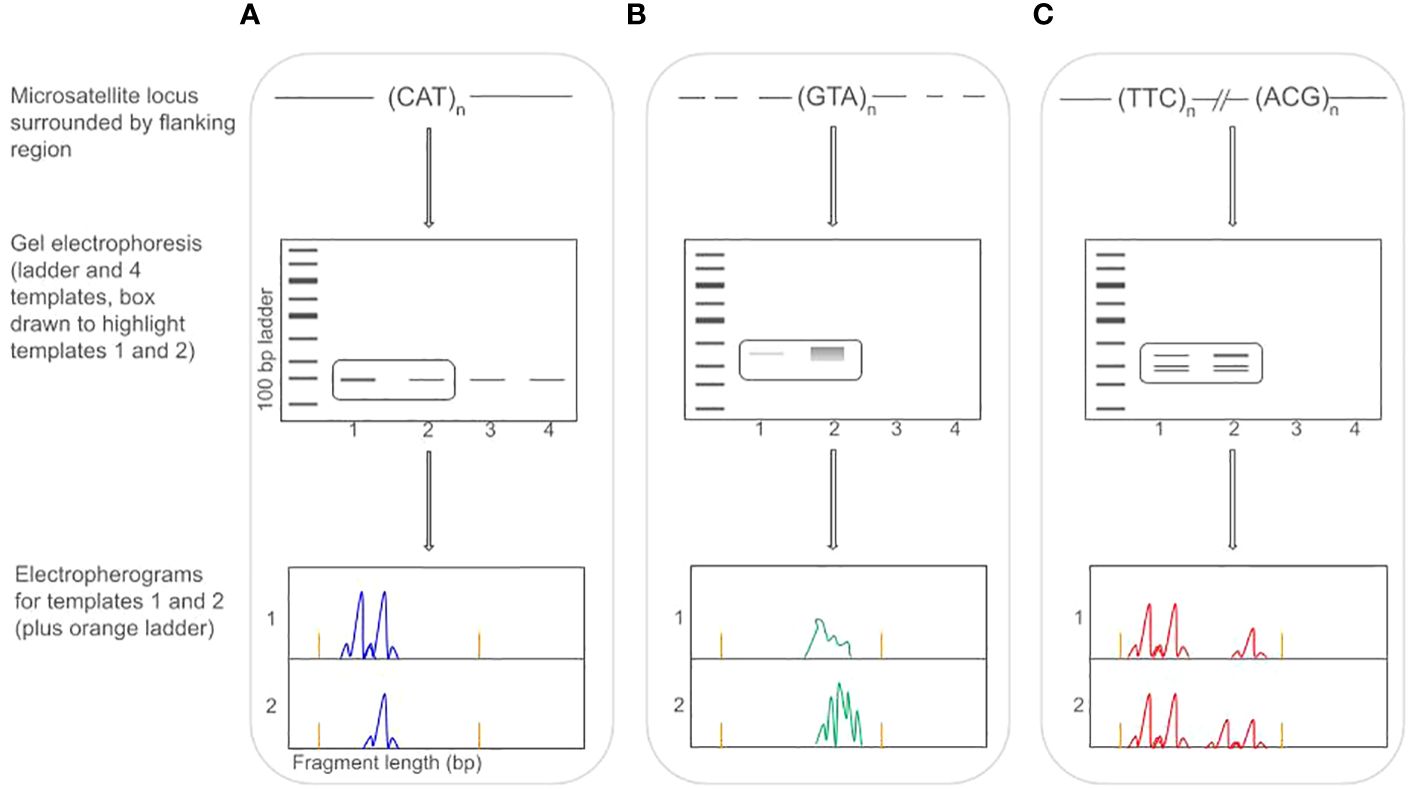
Figure 1 Workflow for genotyping Lepidoptera microsatellites showing three different microsatellite loci (each dyed with a different color fluorophore tag). Box (A) shows an ideal situation amplifying a perfect trinucleotide repeat (CAT). Primers anneal to the flanking region and all four templates produce a clear band (visible in gel electrophoresis). Electropherograms of templates 1 and 2 show a heterozygote and homozygote (respectively) at this locus (locus is dyed with a blue tag). Box (B) shows a different, perfect trinucleotide (GTA), but the flanking region is not fixed, and primers (linked with a green tag) may not anneal (leading to non-amplification in templates 3 and 4). Limited/weak annealing leads to low signal in template 1 (unscorable) and multiple primer annealing may lead to unscorable stutter bands (template 2). Box (C) shows two trinucleotide repeats that are genomically distant from each other, but which have identical flanking regions, leading primers to anneal and amplify both loci simultaneously (or not at all, as in templates 3 and 4). In the electropherogram, this leads to unscorable, complex banding patterns and possible large allele dropout/reduced amplification (with a red tag).
Taken together, the low incidence of microsatellites in the Lepidopteran genome coupled with incomplete flanking region fixation, makes identification and development of microsatellites challenging using traditional molecular methods (Meglecz et al., 2004). A variety of commercial services and university sequencing facilities have historically offered microsatellite library development for reasonable fees, yet have struggled to deliver large numbers of polymorphic, reliably-amplifying microsatellites in Lepidoptera. Other attempts to ameliorate the difficulty of microsatellite development in Lepidoptera include a “cross-amplification strategy” (Rutkowski et al., 2009). In this approach, microsatellite primers identified in one species are used in another (closely related) species, in an attempt to overcome technical difficulties, saving time and money (Rutkowski et al., 2009). However, this does not always work, as markers may fail to amplify due to variability in priming regions between taxa, or loci may be monomorphic (Rutkowski et al., 2009). Zakharov et al. (2007) tried to use microsatellite loci developed for other genera on Erynnis species (Lepidoptera: Hesperiidae) with limited success. The strategy of cross-amplification has been problematic across many taxonomic groups for reasons associated with the genetic distance between taxa resulting in poor amplification success (Primmer et al., 1996).
Next-generation sequencing can provide many more microsatellite loci and reduce traditional molecular laboratory limitations (Saarinen and Austin, 2010). In this paper, we 1) describe developments in microsatellite production using next-generation technologies, 2) demonstrate microsatellite utility in case studies in Lepidoptera of various life histories and conservation status, and 3) provide guidance on application and interpretation of genetic data generated from microsatellite for conservation studies for Lepidoptera.
Technical advances in marker development using next-generation technology
A major leap in our ability to identify usable microsatellite markers in Lepidoptera occurred with the move from enrichment-cloning techniques (traditional molecular laboratory approach) to next-generation sequencing-based techniques for marker development. By dramatically reducing the cost of sequencing large swaths of the genome or transcriptome de novo, next-generation sequencing technologies allow conservation practitioners to readily locate thousands of microsatellite markers and primers directly from sequence data (Castoe et al., 2012). These technical advances should facilitate the use of microsatellites in guiding butterfly management and improving molecular ecological studies.
A variety of next-generation sequencing technologies have emerged in the last several decades (reviewed in Satam et al., 2023). Early in the transition to next-generation sequencing techniques for microsatellite-based studies, Roche 454 pyrosequencing was the sequencing technology of choice (Guichoux et al., 2011). The method generated relatively long reads, increasing the likelihood that a microsatellite sequence together with both flanking regions would be captured in a single read, a requirement for primer design (Castoe et al., 2010; Guichoux et al., 2011). The 454 platform was discontinued in 2016; however, continued advances in sequencing technology expanded the range of platforms used for microsatellite discovery. Long-read technologies like PacBio are particularly suited for rapid microsatellite discovery because of the need for capturing both the microsatellite repeat region and DNA sequence upstream and downstream for primer design. Microsatellite markers can also be developed from sequenced transcriptomes, as in the fritillaries Euphydryas editha (Mikheyev et al., 2010) and E. aurinia (Smee et al., 2013), and the danaid butterfly Parantica sita (Hu et al., 2020, 2022) (Table 1). We note that sequencing technology continues to progress, and the advent of “third-generation” technologies like Nanopore sequencing will continue to influence conservation genetics methods. Practitioners should identify the appropriate technology for their use as platforms continue to evolve.
Next-generation sequencing has not only revolutionized microsatellite marker discovery; it is also beginning to reshape how microsatellite markers are genotyped. In traditional microsatellite genotyping, microsatellites are amplified using fluorescently-labeled primers, followed by analysis of PCR products using capillary electrophoresis. This approach provides genotypes in the form of microsatellite amplicon lengths, via an electropherogram (Figure 1). However, scholars have long cautioned about potential issues with microsatellite amplicon length data, particularly relating to homoplasy, but also to imperfect sequence repeats (Estoup et al., 2002; Selkoe and Toonen, 2006). Using amplicon length as an indicator of genotype assumes that homoplasy has not occurred; in other words, that alleles of the same length have the same evolutionary history. Microsatellites can violate this assumption when back-mutation occurs – e.g. an allele mutating from three to four repeats, and then a descendant of that allele mutating back to three repeats – occur (Estoup et al., 2002; Selkoe and Toonen, 2006; Vartia et al., 2016). While sequencing does not resolve issues of homoplasy, it does uncover imperfect repeats or other insertions in the genotyped sequence.
High throughput sequencing, due to its lower sequencing costs and longer read lengths, addresses the issue of potential homoplasy (Vartia et al., 2016; De Barba et al., 2017; Zhan et al., 2017; Tibihika et al., 2019). In this approach, microsatellite loci are amplified with PCR and the resulting amplicons are directly sequenced (De Barba et al., 2017). We emphasize that this approach can be particularly valuable in Lepidoptera, which may suffer from low polymorphism in microsatellite loci, since genotyping-by-sequencing can uncover “hidden” allelic diversity in these markers that would not be detected by capillary electrophoresis (Zhang, 2004; De Barba et al., 2017). The high throughput sequencing approach (SSRseq) also obviates the need for manual scoring of amplicon sizes from electropherograms; genotyping can be automated using programs like MEGASAT (Zhan et al., 2017; Lepais et al., 2020). Finally, SSRseq can greatly expand opportunities for multiplexing, lowering costs (De Barba et al., 2017).
Utility of microsatellites compared to RADseq in conservation genetics
The utility of microsatellites is limited in some cases, leading to the use of alternative markers such as single nucleotide polymorphisms (SNPs). A common method of de novo SNP discovery for conservation genetics is restriction associated DNA sequencing (RADseq), which uses enzymes to fragment DNA at restriction sites throughout the genome, followed by sequencing and SNP calling (Baird et al., 2008). The resulting RADseq markers have a number of advantages. Because there are many restriction sites in a genome, RADseq methods typically generate hundreds to thousands of markers per individual, many more than a typical microsatellite study, and the increased statistical power provided by this abundance of markers allows for the use of fewer samples (Jeffries et al., 2016). The large number of markers also ameliorates the limitation of each SNP being biallelic and thus having lower per-marker power than highly multi-allelic microsatellites (Helyar et al., 2011). However, because restriction sites must be conserved across individuals in a study, RADseq-derived SNPs are relatively slow evolving, which might limit their ability to detect recent population divergence but could improve their performance at deeper time scales (Andrews et al., 2016). On top of this, while a reference genome is not a strict requirement for RADseq-based methods, the use of a reference genome does allow for better filtering of SNP calls. This reduces their utility in species which lack genomic resources and references (Andrews et al., 2016).
In contrast to RADseq methods, studies using microsatellites have typically been limited to <25 markers (especially in endangered species, and in most Lepidopteran studies), which limits their power to detect weak genetic structure (Putman and Carbone, 2014; Jeffries et al., 2016). To overcome this constraint, larger sample sizes are required – which may be impossible to achieve in rare and endangered species. However, recent advances (like SSRseq) facilitate the development and sequencing hundreds of markers, making microsatellites more applicable to studies of rare or endangered species that have limited population sizes or where sampling is difficult (Bradbury et al., 2018). Microsatellites are known to have a high mutation rate that generates multiple alleles per marker, and this gives them greater power per sample than RADseq markers but can also lead to overestimates of genetic diversity. Studies have suggested that this makes microsatellites better able to detect recent changes to genetic diversity and structure, but also comes at the risk of inflating genetic diversity parameters (Hansson and Westerberg, 2002; Queirós et al., 2015). Museum specimens provide the only opportunity to characterize the genetic makeup of now-extirpated populations, which can provide valuable insights for modern conservation practitioners (Austin and Melville, 2006). Because historic specimens are characterized by degraded, fragmented DNA, genotyping microsatellite markers has a much higher rate of success than does the use of markers like RADseq SNPS. In particular, shorter microsatellite markers tend to have the greatest likelihood of recovery from degraded museum specimens (Strange et al., 2009; Nakahama and Isagi, 2017; Yuan et al., 2021). We note that practitioners seeking to incorporate museum specimens into genetic analyses will need to consult with curators in order to secure permissions for specimen use. Curators may be reluctant to allow specimens to be sampled for genetic material, even when minimally-destructive techniques are used (e.g. performing PCR amplification on a single leg removed from a specimen, and then returning the leg intact to the museum).
There are a number of practical benefits to microsatellites as well. While it is non-trivial to design microsatellites, the benchtop work involved is quicker and simpler than RADseq methods. Likewise, analyzing microsatellite data requires fewer computational resources than analyzing large SNP datasets. Since microsatellites are amplified with PCR, very small starting volumes of DNA are sufficient, as is degraded DNA. In contrast to RADseq markers, microsatellites typically evolve neutrally. This means they are well-suited to analyses aimed at uncovering the effects of demographic (i.e. neutral evolutionary) processes, like migration and genetic drift; however, it does prevent microsatellite markers from providing useful information about natural selection across the genome (Lemopoulos et al., 2019; Sunde et al., 2020; Hauser et al., 2021; Holmes et al., 2023).
The effectiveness of a particular marker type is context dependent, necessitating comparisons of markers within the same system. When evaluating genetic diversity and structure across populations, head-to-head comparisons of RADseq SNPs and microsatellites have shown few biologically meaningful difference between the two (Lemopoulos et al., 2019; Sunde et al., 2020; Hauser et al., 2021; Holmes et al., 2023). Microsatellites outperform RADseq SNPs when evaluating parentage in some contexts and not others (Flanagan and Jones, 2019), but RADseq derived SNPs may be better at detecting hybrid individuals, individual-level heterozygosity, and clustering (Zimmerman et al., 2020; Szatmári et al., 2021; Poelstra et al., 2022; Dufresnes et al., 2023). Combining both marker types can also be useful in testing biogeographic hypotheses because of the different mutation rates between the two marker types can capture different temporal processes (e.g., Holmes et al., 2023).
Notably, we are unaware of any contemporary direct comparisons of microsatellites to SNPs in insects, let alone in Lepidoptera. An evaluation by Schmidt et al. (2023) of bird, mammal, amphibian, reptile, and fish genetic data showed similarities in genetic diversity across microsatellites, mitochondrial DNA markers, and whole genome sequencing (WGS) in these vertebrate taxa. Research including insects (and Lepidoptera) in this area would be of use to practitioners in deciding which markers to use. Sucháčková Bartoňová et al. (2023) add to the call for additional incorporation of genomic information to butterfly conservation studies.
Microsatellite markers in Lepidopteran conservation: relevant case studies
The review paper “Microsatellites for ecologists: a practical guide to using and evaluating microsatellite markers” (Selkoe and Toonen, 2006) greatly “flattens the learning curve” for ecologists engaging with molecular data. In this comprehensive paper (cited 1,056 times per Ecology Letters), the authors provide a summative table of ecological questions that microsatellites can address, including assessing of parentage, evaluating inbreeding, assessing population bottlenecks, evaluating divergence time between populations, gene flow analysis, mating structures (e.g. panmixia), metapopulation dynamics, effective population size (current and historical), etc. Each of these ecological questions have direct conservation applications, but genetic data interpretation can present unknown challenges for conservation practitioners in the absence of clear guidance on statistical parameters, meta-analyses and reviews, and in the context of the jargon-filled discipline of population genetics. Saarinen (2015) presented a guide to molecular marker selection, development of field sampling protocols and post-sampling genetic processing, guidance on setting up conservation genetics programming for Lepidoptera and case studies for endangered North American species including Plebejus melissa samuelis (Lycaenidae), Neonympha mitchelli (Satyridae), and Cyclargus thomasi bethunebakeri (Lycaenidae).
Bridging the gap between Selkoe and Toonen (2006) and Saarinen (2015), we present additional case studies in Lepidoptera that used microsatellite markers to address ecological questions and follow with guidance on statistical interpretation. We highlight critical natural history characteristics (including larval host breadth, dispersal ability, voltinism, and metapopulation structure) that have implications for resulting population genetic information, as these traits shape the generalist–specialist ecological continuum of Lepidoptera (Habel and Schmitt, 2012).
Case study 1: the Poweshiek skipperling (Oarisma poweshiek), evaluating gene flow in an endangered North American prairie species
The Poweshiek skipperling butterfly is federally-endangered in the United States (occurring in the upper Midwestern U.S.) and provincially-endangered in Manitoba, Canada. Historically a common prairie specialist, larvae of this species have been observed feeding on a variety of grasses, while entirely inhabiting prairie fens, dry prairies, and mesic prairies in their range. The species is univoltine and adults have an extremely limited dispersal range, demonstrating an inability to cross even minor habitat barriers. Given the generalist feeding preference and low dispersal ability, Oarisma poweshiek (Parker, 1870) (Hesperiidae) can be classified as a relative intermediate on the generalist–specialist ecological continuum (Saarinen et al., 2016; Belitz et al., 2019). Loss of habitat, increased pesticide uses in agricultural areas, and climate change have all been named as causes for the rapid and precipitous decline of this species – resulting in the disconnection of the few remaining occupied prairie patches.
Saarinen et al. (2016) evaluated extant genetic diversity, tested alternative gene flow hypotheses and isolation by distance models, and considered the role of genetics in captive breeding and reintroduction (Table 1). They found moderately high levels of inbreeding and low levels of genetic diversity in each of the remaining populations (few alleles per locus, low observed heterozygosity, low allelic richness). Analyses revealed very few private alleles, indicating a lack of unique diversity in individual populations. Lack of divergence between populations was supported by non-significant pairwise FST and G’ST values and low variability of Jost’s D (Dest as a derived measure of differentiation). Additional statistics (PCoA, AMOVA, Mantel tests) provide further support that populations are not geographically structured and are genetically similar across the range. Taken together with additional Bayesian clustering results, there is strong support for a model of historically high gene flow across the range of hundreds of kilometers. Given the very low dispersal ability of this species, these results indicate that habitat connectivity was essential for population persistence and that the fragmentation and devastating loss of habitat has led to a precipitous drop in number of populations, size of populations, genetic diversity, and long-term population viability.
At present, very few populations of Oarisma poweshiek remain. Population sizes in all areas are small – exacerbating concerns of loss of genetic diversity to drift and the potential for inbreeding depression. Knowledge of limited dispersal ability and the importance of habitat connectivity are critical in supporting the conservation actions currently underway for this species (Delphey et al., 2016). Knowledge of extant diversity (i.e. allelic richness and private alleles) are necessary for head-starting and translocation efforts, which are critical to the species recovery plan.
Case study 2: the Marsh Fritillary butterfly (Euphydryas aurinia), characterizing impacts from translocations in a declining Eurasian generalist
Conservation practitioners may also be interested in how captive breeding and reintroduction (or translocation efforts) impact genetic characteristics of managed butterfly populations. This approach has been implemented for a British population of the Marsh Fritillary, Euphydryas aurinia (Rottemburg, 1775) (Nymphalidae) (Table 1). The species is a Eurasian butterfly with declining populations across its range (Petenian et al., 2005) and multiple studies have found metapopulation structure in E. aurinia (Warren, 1994; Anthes et al., 2003; Davis et al., 2021; Junker et al., 2021; Pertoldi et al., 2021; Konvicka et al., 2023). Population sizes fluctuate as a result of pressure from parasitoid wasps (Porter, 1983). Across the species’ range, larvae feed on many different host plants, but individual populations tend to be monophagous (Anthes et al., 2003; Konvicka et al., 2003; Meister et al., 2015). The British Isles are the home of a significant proportion of the butterfly’s population; despite legal protection and conservation efforts, it has undergone significant declines in this region due to changing agricultural practices (Porter and Ellis, 2011).
The species was functionally extirpated in Cumbria (NW England) by 2004, when captive breeding and reintroduction was undertaken (Davis et al., 2021). Because only a single butterfly family from Cumbria could be used for captive breeding, practitioners were concerned about inbreeding depression, and chose to include individuals from Argyll, in Scotland, into the captive breeding program (Davis et al., 2021). Offspring of that admixed captive population were reintroduced in 2007, and reintroduced populations have since successfully established (Davis et al., 2021). Results show that genetic diversity is higher in the reintroduced Cumbrian populations relative to their Scottish parent populations, possibly because the reintroduced individuals are descended from both Scottish and Cumbrian parent populations (Davis et al., 2021). Despite this admixture, reintroduced individuals are more genetically similar to their Cumbrian parent population than the Scottish parent populations, indicating that local genetic diversity has not been swamped (Davis et al., 2021). Moreover, no significant inbreeding was detected in the reintroduced populations, a sign of management success (Davis et al., 2021). Across Cumbrian populations, significant pairwise FST values range from 0.0204 to 0.1257. The authors argue that these data demonstrate the efficacy of assisted gene flow efforts in Lepidopteran conservation (Davis et al., 2021).
Case study 3: landscape genetics of the Spanish Moon Moth (Graellsia isabellae), a protected European specialist
Understanding how different habitat types influence species’ dispersal capabilities can help land managers prioritize areas for protection and, as an alternative to mark recapture studies, landscape genetics methods are useful in describing patterns of population structure across a landscape. Microsatellites allow for the detection of impact on a temporal scale suited for recent land-use changes (Wang, 2010; Epps and Keyghobadi, 2015).
The Spanish Moon Moth Graellsia isabellae (Graels, 1849) (Saturniidae) is an iconic, protected moth native to the mountains of Spain and France. The conservation status of this species is controversial: while G. isabellae is protected in the E.U., France, and Spain, the IUCN changed its status from threatened to data deficient in 1996, highlighting the need for further study (Baillie and Groombridge, 1996; Auger-Rozenberg et al., 1998; De Arce Crespo et al., 2010; Vila et al., 2010). In Northern Spain, populations of G. isabellae are protected within the Ordesa y Monte Perdido National Park (OMPNP), but it is unknown whether this is sufficient to protect the species at a broader geographic scale (González-Castellano et al., 2023). Graellsia isabellae is a night flying, univoltine specialist moth and larvae feed exclusively on Scots pine (Pinus sylvestris) and Black pine (Pinus nigra), the former being the only host in the Northern part of its range, including within OMPNP (Marí-Mena et al., 2016). Adults exhibit male-biased dispersal, and males can travel up to 2km every night (Marí-Mena et al., 2019). How these two factors, distribution of P. sylvestris and unique dispersal capabilities, interact may inform land managers whether buffer zones around the park should be established.
To explore whether patterns of genetic structure are associated with the distribution of habitat types in and around the OMPNP, nine microsatellite markers were used to describe gene flow between seventeen populations of G. isabellae (N=402) (González-Castellano et al., 2023) (Table 1). These data uncovered a significant West–East cline of pairwise genetic differentiation (FST = 0.038) and a strong correlation between genetic differentiation along this cline and pine tree cover. This pattern broke down when considering populations that were within six kilometers of each other, which is consistent with the fact that G. isabellae is limited to traveling two kilometers per night and only lives for six days, and implies dispersal occurs through a stepping stone model. Finally, gene flow among populations (as assessed using BAYESASS) supports the existence of a source population on the periphery of OMPNP that supplies migrants to population sinks within the national park and beyond (Wilson and Rannala, 2003). Together, these data support the maintenance of P. sylvestris connectivity and the establishment of buffer zones around parks to protect potential source populations.
These data inform our understanding of how individuals move across a landscape. The study by González-Castellano et al. (2023) incorporated data from multiple years, which helps reduce the concern that landscape genetics patterns are snapshots in time that might fluctuate dramatically over short temporal scales (Jangjoo et al., 2020). The use of microsatellites can also be of use in overcoming such concerns because they facilitate the incorporation of museum specimens into genetic studies, adding to the temporal coverage of the study.
Case study 4: studies in pest species place conservation findings in context
As with all organisms, Lepidopteran species fall along a continuum of rare-to-abundant, threatened-to-secure. Therefore, the results of conservation genetic studies can be placed in useful context through comparison with studies of abundant taxa (Table 1). Many microsatellite-based genetic studies have been conducted in Lepidopteran species that are agricultural pests. These species are abundant and typically geographically widespread, placing them at the opposite end of the scarcity continuum to species of conservation concern. Studies in these species tend to find relatively low global genetic differentiation indicative of high levels of gene flow (i.e., low global FST values). Thus, studies in pest Lepidoptera provide a valuable point of comparison for practitioners interested in the conservation, population, and landscape genetics of rare species.
The cotton bollworm (Helicoverpa armigera, Hübner) is a serious global agricultural pest, feeding on a wide variety of crops. The species is characterized by long-range dispersal (potentially more than 2000km), high fecundity, and a nearly global distribution (Riaz et al., 2021). Considerable microsatellite-based population studies characterize global populations (Tan et al., 2001; Ji et al., 2003; Scott et al., 2003, 2004; Ji et al., 2005; Weeks et al., 2010; Leite et al., 2016)., including research in Southern Australia which found no evidence for structuring across 14 sampled populations and with very low global FST (0.002) and no significant relationship between geographical distance and genetic distance, regardless of the statistic used (Endersby et al., 2007). These results are consistent with long-range migration and human transport in crop shipments (Endersby et al., 2007). The same pattern is evident in Brazil, where H. armigera was first detected in 2013 (Leite et al., 2017). Here, pairwise FST values across 17 populations indicate little to moderate differentiation, although most results were nonsignificant (significant pairwise FST values ranged from 0.034 to 0.114) (Leite et al., 2017). Again, the authors conclude that population genetic results are consistent with panmixia in Brazil, likely due to the species’ long-range dispersal capacity (Leite et al., 2017).
In contrast, Lepidoptera of conservation concern found in fragmented populations display elevated FST values consistent with population differentiation and reduction in gene flow. Even in the vulnerable, but high-dispersal-ability, regal fritillary Speyeria idalia (Drury 1773) (Nymphalidae), fragmented populations have elevated FST values (FST of 0.0202-0.0930) compared to populations located in areas of relatively continuous habitat (FST of 0.0017–0.0312) (Williams et al., 2003). These patterns are evident in other Lepidoptera of conservation concern (Keyghobadi et al., 2005).
A practical guide to data interpretation
Planning and workflow guides (Saarinen, 2015; Holderegger et al., 2019) should be used to develop sampling protocols, study design, collection of genetic data, and plans for analysis. With genetic data in hand, how can results be analyzed and used to answer conservation questions?
Avoiding common pitfalls
Studies of endangered Lepidoptera use non-lethal tissue samples (typically small wing fragments or a single leg) that yield low quantity and (potentially) low quality template DNA (Taberlet et al., 1999). Problems result in genotyping errors including allelic dropout (especially large allele dropout), false alleles, and mistaken alleles (Figure 1). Allelic dropout (false homozygosity) can be reduced by designing primers to amplify shorter fragments (smaller microsatellites) as they amplify at higher rates than longer loci and are preferred when using reduced quality DNA samples (Broquet et al., 2007). Microsatellites with dinucleotide repeats may also be more prone to false alleles, and tri and tetranucleotide repeat motifs should be selected for use. High heterozygosity loci (e.g. loci with many alleles) will also provide more information than loci with only 2–3 alleles per locus, providing more information and potentially saving template DNA use by reducing the number of loci needed for analyses (Taberlet et al., 1999).
Contamination may also negatively impact genetic evaluation in conservation studies (owing to low quantity and quality DNA). Contamination between samples (especially between historical and contemporary samples) is reduced by following ancient DNA laboratory protocols: using new aliquots of reagents (and monitoring reagents for contamination), using filter aerosol-resistant tips in pipettes and UV-cleaning pipettes, and separating pre- and post- PCR work stations (Taberlet et al., 1999). To account for low volumes (low quantity) of DNA stocks, multiplexing microsatellite PCR reactions can minimize valuable template use (but may not always be technically possible).
Despite knowing the problems associated with poor template quality, very few studies using microsatellites conduct any re-genotyping (Table 1) or calculation of genotyping error rate. We support the call by Valière et al. (2007) for projects using low quality DNA to include a pilot study to evaluate the genotyping error rate, impact of error rate on results, and simulation assessment to determine the number of PCR repetitions (per locus and per sample) necessary to provide 95% correct consensus genotypes. Multiple multilocus re-genotyping to generate a consensus genotype will add cost to a project, but a pilot study that assesses the number of PCRs to be repeated will help minimize costs. Valière et al. (2007) determined that six PCRs per locus per sample where necessary to correct up to 95% of genotyping error (based on deer fecal samples). Butterfly projects using wing tissue or leg fragments may not require as many PCR duplications, but a pilot study and evaluation will be essential to determine the extent of re-genotyping needed to achieve a determined level of accuracy for the specific conservation question. Taberlet et al. (1996) recommend three rounds of genotyping (with up to four additional sets depending on the results of the first three), while Pompanon et al. (2005) recommend a 5–10% of re-genotyping of blind samples (“multi-tube approach” of Taberlet et al., 1999). Ideally, duplicate samples of the same individual would be collected, but this is usually impractical when working with endangered Lepidoptera and PCRs may need to be repeated with the same DNA extract. Data may also be screened through programs (listed in Pompanon et al., 2005) to assess likelihood of genotyping error. To allow for re-genotyping, additional DNA template volume may need to be generated to overcome issues of limited template stock. Whole-genome amplification kits (e.g. GenomiPhi DNA Amplification Kit produced by Amersham Biosciences, Piscataway, NJ) have proven useful in generating more template volume and genotyping results are reliable when compared to those amplified from the original template. Caution should be exercised on degraded templates, however, as WGA kits will amplify degraded DNA as well (they do not fix sheared fragments) (Holbrook et al., 2005). Lastly, we encourage manual calling (or a manual check) of all allele calls in genotyping programs to minimize error due to mistaken alleles.
Because genotyping errors may complicate interpretations of Hardy-Weinberg equilibrium (HWE), errors should be addressed before beginning any population analysis – failure to do so may collude interpretations of deviation from HWE and the very determinants of inbreeding and population sub-structuring (Pompanon et al., 2005). Bonferroni corrections should always be performed to assess significant deviation from HWE when performing multiple tests (e.g. across multiple loci).
If a microsatellite marker shows evidence of linkage disequilibrium (meaning it is linked to another locus), it is recommended to remove that marker from analysis so as not to conflate results (especially if there are few markers used in the study).
Defining population units
Before genetic diversity and similarity are assessed, it may be necessary to use genetic clustering strategies to define population units, as subsequent analyses are highly sensitive to population definition (Willi et al., 2022). Clustering analyses use genotype data to infer the number of distinct genetic groups present among samples. A variety of clustering analyses are possible, including Bayesian model-based approaches (e.g. STRUCTURE, Pritchard et al., 2000) and non-model-based, multivariate approaches (e.g. principal coordinates analysis, PCoA). These clustering analyses provide valuable information about the scale at which populations should be managed. For example, an analysis of the rare South Florida duskywing skipper (Ephyriades brunnea) (Hesperiidae) found that samples from mainland Florida and the Florida Keys form two distinct genetic clusters, indicating that these populations should be treated as two distinct units for management (Heffernan et al., 2023).
In addition to exploring patterns of genetic diversity within individual populations, it is important to understand the degree of differentiation vs. connection between populations. What is the extent of gene flow among populations? Does the species exist in panmixia, or is it divided into genetically distinct populations? Addressing these questions can assist practitioners in understanding potential risks of inbreeding depression and in planning interventions like translocation; it can also be useful in defining discrete management units, if genetic differentiation coincides with some form of ecological differentiation.
Because microsatellite markers evolve rapidly, they are well-suited to understanding recent and historic patterns of gene flow between populations. Population differentiation is typically measured using pairwise FST, with higher pairwise values indicating a greater degree of genetic fixation between populations (Figure 2). Related, derived statistics such as G’ST or G’’ST can also be used (but see Whitlock, 2011 for relative utility using different markers).The value of using FST and related metrics is that it provides a readily comparable measure of genetic drift due to limited gene flow and reduced population size. Interpreting FST values (or comparing FST values across study species) must consider landscape features (which may facilitate or hinder species dispersal), dispersal ability of the butterfly species, and the marker set used (Figure 2). Wright (1978) suggested general guidelines for FST interpretation of genetic differentiation (allele frequency divergence among subpopulations): FST of 0.00–0.05 → little, FST of 0.05–0.15 → moderate, FST of 0.15–0.25 → great, and FST above 0.25 → very great. However, these guidelines were developed based on bi-allelic markers (allozymes, and now SNPs) not hyper-variable microsatellites. Therefore, interpreting differentiation on this scale based on microsatellites should be done with caution.
Comparisons between species should also consider that microsatellite mutation rates may differ between species (Willi et al., 2022). The statistical power of the FST value is also critical in determining the significant and meaningful differentiation between populations.
Quantifying genetic diversity
A fundamental goal of conservation genetics is understanding the distribution of genetic diversity across populations (Figure 3). This allows practitioners to identify (1) populations with depleted genetic diversity – which may be at particular risk due to inbreeding depression and lack of evolvability – and (2) populations with relatively greater genetic diversity, which may be suitable source populations for captive breeding or translocation efforts. A number of different statistics derived from microsatellite genotypes are useful for describing genetic diversity within populations, including per-population allelic diversity, the observed heterozygosity per population, and the number of private alleles per population. Populations with greater genetic diversity are characterized by higher allelic diversity and higher observed heterozygosity, while the presence of private alleles indicates that a population harbors unique genetic diversity, which practitioners may wish to explicitly protect via management action. These metrics can also be used to compare wild populations to captive breeding colonies established for use in conservation, to ensure that the captive population’s genetic diversity is reflective of wild populations (Miller et al., 2014).
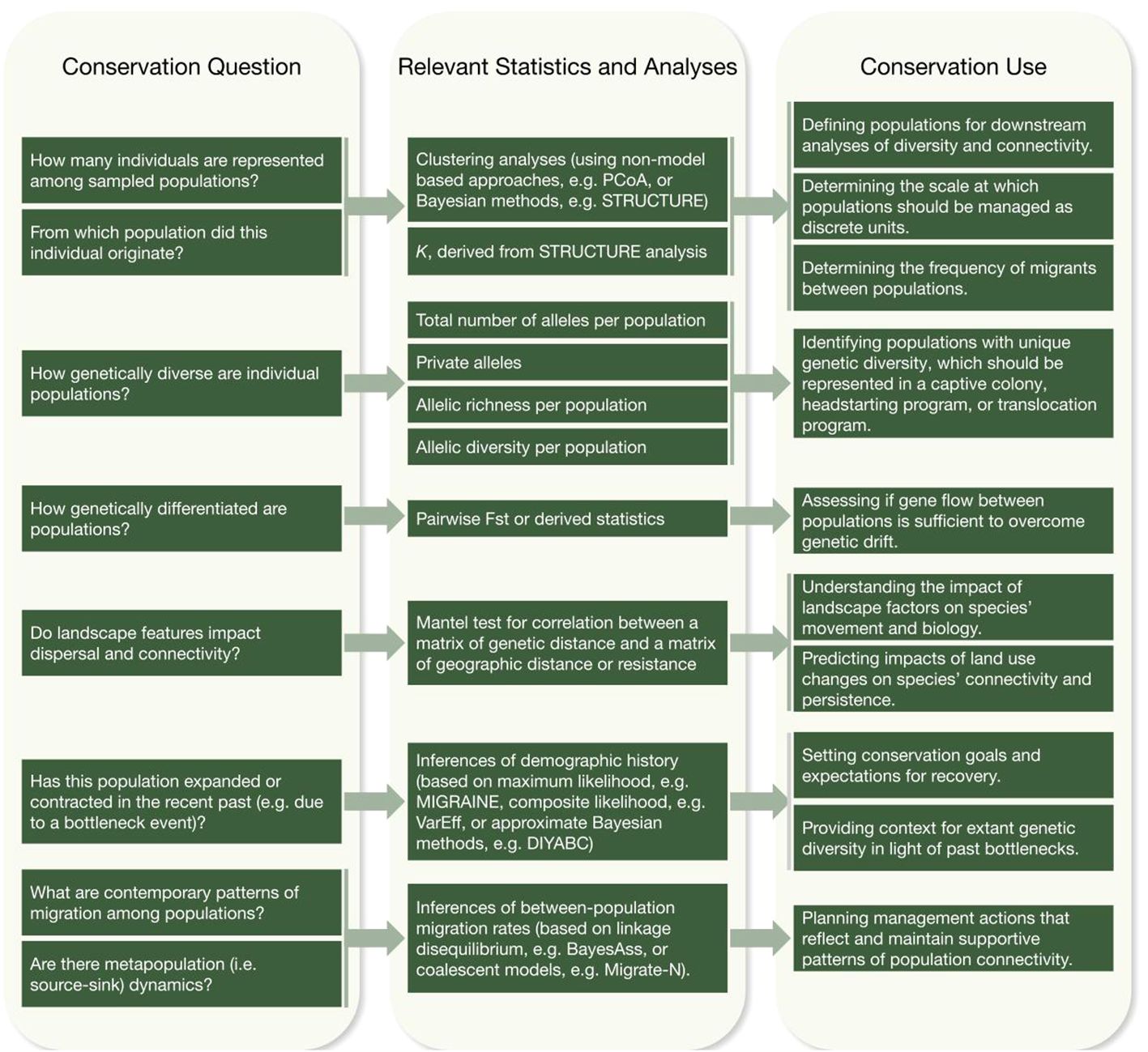
Figure 3 Making connections from conservation questions, through techniques, to applied conservation use.
Assessing geographic structure
Understanding the extent of genetic structure can clarify the factors shaping patterns of genetic differentiation. For instance, it may be useful to understand whether geographic distance structures genetic differentiation – in other words, whether the species experiences isolation-by-distance. Historically, patterns of isolation-by-distance have been assessed using a Mantel test. The Mantel test is a nonparametric test for correlation between two distance matrices: in this case, a matrix of geographic distances and a matrix of genetic distances (typically as measured by pairwise FST). The ability to detect isolation-by-distance in this manner is strongly influenced by the number of sampled populations (Jenkins et al., 2010). Newer strategies include information about landscape features and habitat types through tests for isolation-by-resistance, e.g. using CircuitScape (van Strien et al., 2015; McRae et al., 2016), the strip-based method developed by Emarsi and colleagues (Emaresi et al., 2011), or with the R package ResistanceGA, which implements linear mixed models with the maximum-likelihood population effect parameterization, accounting for the non-independence of values in pairwise distance matrices (Peterman, 2018).
Inferring historical demography
Conservation practitioners may also be interested in understanding historical demographic processes in species or populations of conservation concern. For example, has the species or population of concern been isolated for long periods, or is isolation a more recent phenomenon? A variety of programs can address these questions, including BayesAss, which infers recent migration rates among populations (Wilson and Rannala, 2003); the R package VarEff and the program MIGRAINE, both of which estimate past changes in effective population sizes (Leblois et al., 2014; Nikolic and Chevalet, 2014); and the program DIYABC, which allows users to compare support for competing models of population history (Cornuet et al., 2014). Contemporary effective population sizes can also be estimated from genetic data, using programs like NeEstimator (Do et al., 2014). The efficacy of these approaches will depend on the number of microsatellite markers and the number of sampled individuals; therefore, if conservation practitioners wish to use these methods, particular attention should be paid during the study design phase. For instance, modeling work demonstrates that the ability of BayesAss to accurately capture migration rates is maximized when few populations but many individuals are sampled, which may be challenging in Lepidoptera of conservation concern (Meirmans, 2014). Moreover, testing complex demographic scenarios can require many markers to achieve statistical power, meaning that conservation geneticists interested in these approaches may prefer to use SNPs, rather than microsatellite markers.
Utility of museum specimens
We emphasize the utility of incorporating genetic data from museum specimens into conservation genetics analyses, when possible (Tracy and Jamieson, 2011; Kekkonen, 2016; Lalonde and Marcus, 2020; Nakahama, 2021). Natural history collections are critical repositories of historic biological data, including genetic material, and their use can place contemporary results into meaningful context (Harper et al., 2006; Habel et al., 2009; Ugelvig et al., 2011; Kim et al., 2020; Davis et al., 2021). Microsatellites have proven to be very useful in recreating population histories and historical allele frequencies from museum specimens, as microsatellites are typically short in length and able to be amplified from older (low quality and low quantity) DNA (Saarinen and Daniels, 2012). For instance, recent bottleneck events and ensuing losses of genetic diversity can obscure historical patterns of spatial genetic structuring; by incorporating genetic data from museum specimens, these patterns may be elucidated and used in defining population units for conservation management (Nakahama, 2021). Reintroductions (a common conservation strategy) may also be assisted by genetic data from museum specimens. The black-veined white Aporia crataegi (Linnaeus 1758) (Pieridae) is a highly-dispersing generalist butterfly found from northwest Africa to far eastern Asia, but presumed extirpated from South Korea (Jugovic et al., 2017; Kim et al., 2020). Using a combination of microsatellite markers and mitochondrial gene sequences, Kim and colleagues determined that South Korean museum specimens are most genetically similar to extant populations in Mongolia, China, and Russia, making these populations the most suitable sources for any reintroduction efforts in South Korea (Kim et al., 2020).
Conclusion
Butterfly conservation efforts continue to be plagued by data deficiencies: missing natural history information, unknown dispersal capacity, lack of population-level demographic information, and an absence of genetic data (Geyle et al., 2021; New, 2022; Rosa et al., 2023). By shedding light on patterns of population connectivity and diversity, conservation genetic studies can help address some of these gaps. However, prior to the advent of next-generation sequencing technologies, conservation genetic studies in butterflies faced a major challenge: difficulties developing suitable genetic markers.
Although microsatellite marker generation in Lepidoptera has become substantially easier, actually integrating genetic data into conservation practice remains challenging. For instance, the International Union for Conservation of Nature (IUCN) threats classification scheme, which is used to generate Red List assessments, does not include genetic concerns or threats (except “introduced genetic material”, e.g. the release of hatchery fish into wild gene pools) (Garner et al., 2020; IUCN – CMP Unified Classification of Direct Threats Version: 3.3, 2022). Although only evaluated in vertebrate species, Schmidt et al. (2023) demonstrate that genetic data (including from microsatellites) cannot be used to identify threat status. Furthermore, they found that Red List status did not indicate whether a species warranted genetic diversity protection. As more insect studies, and Lepidoptera in particular, generate genetic results, it will be critical to develop metrics to include assessments of genetic diversity into conservation policy. There also remains a potential disconnect between academic researchers engaged in conservation genetics studies, and conservation practitioners actively engaged in species conservation (Holderegger et al., 2019). This disconnect may potentially widen with the increasing use of SNP-based methods in conservation genetics, which are more computationally demanding than microsatellite-based analyses.
By summarizing microsatellite-based conservation genetics studies across Lepidoptera species, and by providing a guide to study design and data interpretation, we aim to narrow the disconnect between academic researchers and conservation professionals. We call for a standard reporting of microsatellite statistics to allow for comparisons across studies (including number of effective alleles, allelic richness, and FIS both per locus and per population). Whenever possible, descriptions of basic life history information (larval feeding breadth, adult dispersal, voltinism) should be included to further assist in data interpretation and comparison across studies (Figure 2). These comparisons are useful in setting conservation management goals (e.g. number of populations necessary for species viability, arrangements of populations, requirements for minimum population size to maintain genetic diversity, etc.) while considering critical life history information.
No conservationists question the importance of genetic data. Genetic diversity directly affects extinction risk. However, the incorporation of genetic data into conservation plans continues to be challenging, and genetic data is not a criterion for listing on the IUCN Red List. We hope that new technologies continue to support investigation of genetic diversity, and that review papers assist conservationists in data interpretation and incorporation into conservation strategies.
Author contributions
EH: Conceptualization, Data curation, Formal analysis, Project administration, Resources, Supervision, Visualization, Writing – original draft, Writing – review & editing. MB: Data curation, Formal analysis, Investigation, Methodology, Resources, Visualization, Writing – original draft, Writing – review & editing. NB: Formal analysis, Resources, Writing – original draft, Writing – review & editing.
Funding
The author(s) declare that no financial support was received for the research, authorship, and/or publication of this article.
Conflict of interest
The author(s) declare that no financial support was received for the research, authorship, and/or publication of this article.
Publisher’s note
All claims expressed in this article are solely those of the authors and do not necessarily represent those of their affiliated organizations, or those of the publisher, the editors and the reviewers. Any product that may be evaluated in this article, or claim that may be made by its manufacturer, is not guaranteed or endorsed by the publisher.
Glossary
References
Andrews K. R., Good J. M., Miller M. R., Luikart G., Hohenlohe P. A. (2016). Harnessing the power of RADseq for ecological and evolutionary genomics. Nat. Rev. Genet. 17, 81–92. doi: 10.1038/nrg.2015.28
Anthes N., Fartmann T., Hermann G., Kaule G. (2003). Combining larval habitat quality and metapopulation structure – the key for successful management of pre-alpine Euphydryas aurinia colonies. J. Insect Conserv. 7, 175–185. doi: 10.1023/a:1027330422958
Anton C., Zeisset I., Musche M., Durka W., Boomsma J. J., Settele J. (2007). Population structure of a large blue butterfly and its specialist parasitoid in a fragmented landscape. Mol. Ecol. 16, 3828–3838. doi: 10.1111/j.1365-294X.2007.03441.x
Auger-Rozenberg M. A., Géri C., Quencez C., Bastien C. (1998). Noxious Effect of Scots Pine Foliage on Diprion pini L. (Hym. Diprionidae) and Other Defoliators: Specificity and Prospects for Use in Forest Management. In Proceedings of Population Dynamics, Impacts, and Integrated Management of Forest Defoliating Insects, ed. McManus M. L., Liebhold A. M., USDA Forest Service, Northeastern Reserach Station, General Technical Report NE-247., 91–99
Austin J. J., Melville J. (2006). Incorporating historical museum specimens into molecular systematic and conservation genetics research. Mol. Ecol. Notes 6, 1089–1092. doi: 10.1111/j.1471-8286.2006.01443.x
Baillie J., Groombridge B. (Eds.) (1996). 1996 IUCN red list of threatened animals. IUCN, Gland Switzerland, 448p.
Baird N. A., Etter P. D., Atwood T. S., Currey M. C., Shiver A. L., Lewis Z. A., et al. (2008). Rapid SNP discovery and genetic mapping using sequenced RAD markers. PloS One 3, e3376. doi: 10.1371/journal.pone.0003376
Belitz M. W., Monfils M. J., Cuthrell D. L., Monfils A. K. (2019). Life history and ecology of the endangered Poweshiek skipperling Oarisma poweshiek in Michigan prairie fens. J. Insect Conserv. 23, 635–649. doi: 10.1007/s10841-019-00158-6
Bradbury I. R., Wringe B. F., Watson B., Paterson I., Horne J., Beiko R., et al. (2018). Genotyping-by-sequencing of genome-wide microsatellite loci reveals fine-scale harvest composition in a coastal Atlantic salmon fishery. Evol. Appl. 11, 918–930. doi: 10.1111/eva.12606
Broquet T., Menard N., Petit E. (2007). Noninvasive population genetics: a review of sample source, diet, fragment length and microsatellite motif effects on amplification success and genotyping error rates. Conserv. Genet. 8, 249–260. doi: 10.1007/s10592-006-9146-5
Cao L. J., Wen J. B., Wei S. J., Liu J., Yang F., Chen M. (2015). Characterization of novel microsatellite markers for Hyphantria cunea and implications for other Lepidoptera. Bull. Entomol. Res. 105, 273–284. doi: 10.1017/S0007485315000061
Castoe T. A., Poole A. W., de Koning A. P. J., Jones K. L., Tomback D. F., Oyler-McCance S. J., et al. (2012). Rapid microsatellite identification from Illumina paired-end genomic sequencing in two birds and a snake. PloS One 7, e30953. doi: 10.1371/journal.pone.0030953
Castoe T. A., Poole A. W., Gu W., Jason de Koning A. P., Daza J. M., Smith E. N., et al. (2010). Rapid identification of thousands of copperhead snake (Agkistrodon contortrix) microsatellite loci from modest amounts of 454 shotgun genome sequence. Mol. Ecol. Resour 10, 341–347. doi: 10.1111/j.1755-0998.2009.02750.x
Chen M. H., Dorn S. (2010). Microsatellites reveal genetic differentiation among populations in an insect species with high genetic variability in dispersal, the codling moth, Cydia pomonella (L.) (Lepidoptera: Tortricidae). Bull. Entomol. Res. 100, 75–85. doi: 10.1017/S0007485309006786
Cornuet J.-M., Pudlo P., Veyssier J., Dehne-Garcia A., Gautier M., Leblois R., et al. (2014). DIYABC v2.0: a software to make approximate Bayesian computation inferences about population history using single nucleotide polymorphism, DNA sequence and microsatellite data. Bioinformatics 30, 1187–1189. doi: 10.1093/bioinformatics/btt763
Davis M. L., Barker C., Powell I., Porter K., Ashton P. (2021). Combining modeling, field data and genetic variation to understand the post-reintroduction population genetics of the Marsh Fritillary butterfly (Euphydryas aurinia). J. Insect Conserv. 25, 875–886. doi: 10.1007/s10841-021-00354-3
De Arce Crespo J. I., Jiménez Mendoza S., Sánchez Fernández P. (2010). Recopilación de la información biogeográfica, análisis de patrones ecológicos, conservación y mapa potencial de Graellsia isabelae (Graells 1849) (Lepidoptera, Saturniidae) en la provincia de Cuenca, España. GRAELLSIA 66, 9–20. doi: 10.3989/graellsia.2010.v66.003
De Barba M., Miquel C., Lobréaux S., Quenette P. Y., Swenson J. E., Taberlet P. (2017). High-throughput microsatellite genotyping in ecology: improved accuracy, efficiency, standardization and success with low-quantity and degraded DNA. Mol. Ecol. Resour 17, 492–507. doi: 10.1111/1755-0998.12594
Delphey P., Runquist E., Harris T., Traylor-Holzer K., Miller P. S. (2016). Poweshiek skipperling and Dakota skipper: Ex situ feasibility assessment and planning workshop (Apple Valley, MN: IUCN/SSC Conservation Breeding Specialist Group).
de Moura P. A., Quek S.-P., Cardoso M. Z., Kronforst M. R. (2011). Comparative population genetics of mimetic Heliconius butterflies in an endangered habitat; Brazil’s Atlantic Forest. BMC Genet. 12, 9. doi: 10.1186/1471-2156-12-9
De Ro A., Vanden Broeck A., Verschaeve L., Jacobs I., T’Jollyn F., Van Dyck H., et al. (2021). Occasional long-distance dispersal may not prevent inbreeding in a threatened butterfly. BMC Ecol. Evo 21, 224. doi: 10.1186/s12862-021-01953-z
Do C., Waples R. S., Peel D., Macbeth G. M., Tillett B. J., Ovenden J. R. (2014). NeEstimator v2: re-implementation of software for the estimation of contemporary effective population size (Ne) from genetic data. Mol. Ecol. Resour 14, 209–214. doi: 10.1111/1755-0998.12157
Dufresnes C., Dutoit L., Brelsford A., Goldstein-Witsenburg F., Clément L., López-Baucells A., et al. (2023). Inferring genetic structure when there is little: population genetics versus genomics of the threatened bat Miniopterus schreibersii across Europe. Sci. Rep. 13, 1523. doi: 10.1038/s41598-023-27988-4
Emaresi G., Pellet J., Dubey S., Hirzel A. H., Fumagalli L. (2011). Landscape genetics of the Alpine newt (Mesotriton alpestris) inferred from a strip-based approach. Conserv. Genet. 12, 41–50. doi: 10.1007/s10592-009-9985-y
Endersby N. M., Hoffmann A. A., McKechnie S. W., Weeks A. R. (2007). Is there genetic structure in populations of Helicoverpa armigera from Australia? Entomol. Exp. Appl. 122, 253–263. doi: 10.1111/j.1570-7458.2006.00515.x
Epps C. W., Keyghobadi N. (2015). Landscape genetics in a changing world: disentangling historical and contemporary influences and inferring change. Mol. Ecol. 24, 6021–6040. doi: 10.1111/mec.13454
Estoup A., Jarne P., Cornuet J.-M. (2002). Homoplasy and mutation model at microsatellite loci and their consequences for population genetics analysis. Mol. Ecol. 11, 1591–1604. doi: 10.1046/j.1365-294x.2002.01576.x
Flanagan S. P., Jones A. G. (2019). The future of parentage analysis: From microsatellites to SNPs and beyond. Mol. Ecol. 28, 544–567. doi: 10.1111/mec.14988
Garner B. A., Hoban S., Luikart G. (2020). IUCN Red List and the value of integrating genetics. Conserv. Genet. 21, 795–801. doi: 10.1007/s10592-020-01301-6
Geyle H. M., Braby M. F., Andren M., Beaver E. P., Bell P., Byrne C., et al. (2021). Butterflies on the brink: identifying the Australian butterflies (Lepidoptera) most at risk of extinction. Austral Entomol 60, 98–110. doi: 10.1111/aen.12525
González-Castellano I., Marí-Mena N., Segelbacher G., Lopez-Vaamonde C., González-López J., Fagúndez J., et al. (2023). Landscape genetics of the protected Spanish Moon Moth in core, buffer, and peripheral areas of the Ordesa y Monte Perdido National Park (Central Pyrenees, Spain). Conserv. Genet. 24, 767–782. doi: 10.1007/s10592-023-01536-z
Gratton P., Sbordoni V. (2009). Isolation of novel microsatellite markers for the clouded Apollo (P. mnemosyne Linnaeus 1758; lepidoptera, papilionidae). Conserv. Genet. 10, 1141–1143. doi: 10.1007/s10592-008-9728-5
Guichoux E., Lagache L., Wagner S., Chaumeil P., Léger P., Lepais O., et al. (2011). Current trends in microsatellite genotyping. Mol. Ecol. Resour 11, 591–611. doi: 10.1111/j.1755-0998.2011.03014.x
Habel J. C., Rödder D., Schmitt T., Nève G. (2011). Global warming will affect the genetic diversity and uniqueness of Lycaena helle populations. Glob. Change Biol. 17, 194–205. doi: 10.1111/j.1365-2486.2010.02233.x
Habel J. C., Schmitt T. (2012). The burden of genetic diversity. Biol. Conserv. 147, 270–274. doi: 10.1016/j.biocon.2011.11.028
Habel J. C., Zachos F. E., Finger A., Meyer M., Louy D., Assmann T., et al. (2009). Unprecedented long-term genetic monomorphism in an endangered relict butterfly species. Conserv. Genet. 10, 1659–1665. doi: 10.1007/s10592-008-9744-5
Hansson B., Westerberg L. (2002). On the correlation between heterozygosity and fitness in natural populations. Mol. Ecol. 11, 2467–2474. doi: 10.1046/j.1365-294x.2002.01644.x
Harper G. L., Maclean N., Goulson D. (2003). Microsatellite markers to assess the influence of population size, isolation and demographic change on the genetic structure of the UK butterfly Polyommatus bellargus. Mol. Ecol. 12, 3349–3357. doi: 10.1046/j.1365-294x.2003.02012.x
Harper G. L., Maclean N., Goulson D. (2006). Analysis of museum specimens suggests extreme genetic drift in the adonis blue butterfly (Polyommatus bellargus). Biol. J. Linn. Soc. 88, 447–452. doi: 10.1111/j.1095-8312.2006.00632.x
Hauser S. S., Athrey G., Leberg P. L. (2021). Waste not, want not: Microsatellites remain an economical and informative technology for conservation genetics. Ecol. Evol. 11, 15800–15814. doi: 10.1002/ece3.8250
Heffernan E., Markee A., Truglio M. R., Barkdull M., Steele Cabrera S., Daniels J. (2023). Population genetic structure of a rare butterfly in a fragmented south florida ecosystem. Insects 14, 321. doi: 10.3390/insects14040321
Helyar S. J., Hemmer-Hansen J., Bekkevold D., Taylor M. I., Ogden R., Limborg M. T., et al. (2011). Application of SNPs for population genetics of nonmodel organisms: new opportunities and challenges. Mol. Ecol. Resour. 11 Suppl 1, 123–136. doi: 10.1111/j.1755-0998.2010.02943.x
Holbrook J. F., Stabley D., Sol-Church K. (2005). Exploring whole genome amplification as a DNA recovery tool for molecular genetic studies. J. Biomol Tech 16, 125–133.
Holderegger R., Balkenhol N., Bolliger J., Engler J. O., Gugerli F., Hochkirch A., et al. (2019). Conservation genetics: Linking science with practice. Mol. Ecol. 28, 3848–3856. doi: 10.1111/mec.15202
Holmes I. A., Monagan I. V., Westphal M. F., Johnson P. J., Davis Rabosky A. R. (2023). Parsing variance by marker type: Testing biogeographic hypotheses and differential contribution of historical processes to population structure in a desert lizard. Mol. Ecol. 32, 4880–4897. doi: 10.1111/mec.17076
Hu P., Huang C.-L., Luo M.-X., Hsu Y.-F., Wang R.-J. (2020). Development and characterization of novel microsatellite markers in chestnut tiger butterfly Parantica sita (Lepidoptera: Nymphalidae) using next-generation sequencing. Appl. Entomol. Zool. (Jpn.) 55, 281–286. doi: 10.1007/s13355-020-00675-w
Hu P., Lu L., Hu S., Da W., Huang C.-L., Zhang H., et al. (2022). Differentiation of the chestnut tiger butterfly parantica sita (Lepidoptera: nymphalidae: danainae) in China. Front. Ecol. Evol. 10. doi: 10.3389/fevo.2022.846499
Jangjoo M., Matter S. F., Roland J., Keyghobadi N. (2020). Demographic fluctuations lead to rapid and cyclic shifts in genetic structure among populations of an alpine butterfly, Parnassius smintheus. J. Evol. Biol. 33, 668–681. doi: 10.1111/jeb.13603
Jeffries D. L., Copp G. H., Lawson Handley L., Olsén K. H., Sayer C. D., Hänfling B. (2016). Comparing RADseq and microsatellites to infer complex phylogeographic patterns, an empirical perspective in the Crucian carp, Carassius carassius, L. Mol. Ecol. 25, 2997–3018. doi: 10.1111/mec.13613
Jenkins D. G., Carey M., Czerniewska J., Fletcher J., Hether T., Jones A., et al. (2010). A meta-analysis of isolation by distance: relic or reference standard for landscape genetics? Ecography 33, 315–320. doi: 10.1111/j.1600-0587.2010.06285.x
Jeong S. Y., Kim M. J., Park J. S., Jeong N. R., Kim S.-S., Oh K.-C., et al. (2020). Population genetic characterization of the endangered silver skipper, Leptalina unicolor (Lepidoptera: Hesperiidae), using novel microsatellite markers and mitochondrial DNA gene sequences. J. Asia Pac Entomol. 23, 781–790. doi: 10.1016/j.aspen.2020.07.007
Ji Y.-J., Wu Y.-C., Zhang D.-X. (2005). Novel polymorphic microsatellite markers developed in the cotton bollworm Helicoverpa armigera (Lepidoptera: Noctuidae). Insect Sci. 12, 331–334. doi: 10.1111/j.1005-295X.2005.00040.x
Ji Y. J., Zhang D. X., Hewitt G. M., Kang L., Li D. M. (2003). Polymorphic microsatellite loci for the cotton bollworm Helicoverpa armigera (Lepidoptera: Noctuidae) and some remarks on their isolation. Mol. Ecol. Notes 3, 102–104. doi: 10.1046/j.1471-8286.2003.00366.x
Jugovic J., Crne M., Luznik M. (2017). Movement, demography and behaviour of a highly mobile species: A case study of the black-veined white, Aporia crataegi (Lepidoptera: Pieridae). Eur. J. Entomol. 114, 113–122. doi: 10.14411/eje.2017.016
Junker M., Konvicka M., Zimmermann K., Schmitt T. (2021). Gene-flow within a butterfly metapopulation: the marsh fritillary Euphydryas aurinia in western Bohemia (Czech Republic). J. Insect Conserv. 25, 585–596. doi: 10.1007/s10841-021-00325-8
Kekkonen J. (2016). “Temporal genetic monitoring of declining and invasive wildlife populations: current state and future directions,” in Problematic Wildlife. Ed. Angelici F. M. (Springer International Publishing, Cham), 269–294. doi: 10.1007/978-3-319-22246-2_13
Keyghobadi N., Roland J., Strobeck C. (2005). Genetic differentiation and gene flow among populations of the alpine butterfly, Parnassius smintheus, vary with landscape connectivity. Mol. Ecol. 14, 1897–1909. doi: 10.1111/j.1365-294X.2005.02563.x
Keyghobadi N., Unger K. P., Weintraub J. D., Fonseca D. M. (2006). Remnant populations of the regal fritillary (Speyeria idalia) in Pennsylvania: local genetic structure in a high gene flow species. Cons. Gen. 7, 309–313. doi: 10.1007/s10592-006-9127-8
Kim M. J., Cho Y., Wang A. R., Kim S.-S., Choi S.-W., Kim I. (2020). Population genetic characterization of the black-veined white, Aporia crataegi (Lepidoptera: Pieridae), using novel microsatellite markers and mitochondrial DNA gene sequences. Conserv. Genet. 21, 359–371. doi: 10.1007/s10592-020-01257-7
Konvicka M., Hula V., Fric Z. (2003). Habitat of pre-hibernating larvae of the endangered butterfly Euphydryas aurinia (Lepidoptera: Nymphalidae): What can be learned from vegetation composition and architecture? Eur. J. Entomol. 100, 313–322. doi: 10.14411/eje.2003.050
Konvicka M., Hula V., Vlasanek P., Zimmermann K., Faltynek Fric Z. (2023). Within-habitat vegetation structure and adult activity patterns of the declining butterfly Euphydryas aurinia. J. Insect Conserv. 27, 335–346. doi: 10.1007/s10841-023-00459-x
Konvickova H., Spitzer L., Fric Z. F., Kepka P., Lestina D., Novotny D., et al. (2023). Perishing rich, expanding poor: Demography and population genetic patterns in two congeneric butterflies. Mol. Ecol. 32, 575–594. doi: 10.1111/mec.16784
Lalonde M. M. L., Marcus J. M. (2020). How old can we go? Evaluating the age limit for effective DNA recovery from historical insect specimens. Syst. Entomol. 45, 505–515. doi: 10.1111/syen.12411
Leblois R., Pudlo P., Néron J., Bertaux F., Reddy Beeravolu C., Vitalis R., et al. (2014). Maximum-likelihood inference of population size contractions from microsatellite data. Mol. Biol. Evol. 31, 2805–2823. doi: 10.1093/molbev/msu212
Leite N. A., Corrêa A. S., Alves-Pereira A., Campos J. B., Zucchi M. I., Omoto C. (2016). Cross-species amplification and polymorphism of microsatellite loci in Helicoverpa armigera and Helicoverpa zea (Lepidoptera: Noctuidae) in Brazilian cropping systems. Genet. Mol. Res. 15, 1–12. doi: 10.4238/gmr.15027890
Leite N. A., Correa A. S., Michel A. P., Alves-Pereira A., Pavinato V. A. C., Zucchi M. I., et al. (2017). Pan-American Similarities in Genetic Structures of Helicoverpa armigera and Helicoverpa zea (Lepidoptera: Noctuidae) With Implications for Hybridization. Environ. Entomol. 46, 1024–1034. doi: 10.1093/ee/nvx088
Lemopoulos A., Prokkola J. M., Uusi-Heikkilä S., Vasemägi A., Huusko A., Hyvärinen P., et al. (2019). Comparing RADseq and microsatellites for estimating genetic diversity and relatedness - Implications for brown trout conservation. Ecol. Evol. 9, 2106–2120. doi: 10.1002/ece3.4905
Lepais O., Chancerel E., Boury C., Salin F., Manicki A., Taillebois L., et al. (2020). Fast sequence-based microsatellite genotyping development workflow. Peer J. 8, e9085. doi: 10.7717/peerj.9085
Marí-Mena N., Lopez-Vaamonde C., Naveira H., Auger-Rozenberg M.-A., Vila M. (2016). Phylogeography of the spanish moon moth graellsia isabellae (Lepidoptera, saturniidae). BMC Evol. Biol. 16, 139. doi: 10.1186/s12862-016-0708-y
Marí-Mena N., Naveira H., Lopez-Vaamonde C., Vila M. (2019). Census and contemporary effective population size of two populations of the protected Spanish Moon Moth (Graellsia isabellae). Insect Conserv. Divers. 12, 147–160. doi: 10.1111/icad.12322
McRae B. H., Shah V. B., Edelman A. (2016). Circuitscape: modeling landscape connectivity to promote conservation and human health. Nat. Conservancy. 14, 1–14. doi: 10.13140/rg.2.1.4265.1126
Meglecz E., Petenian F., Danchin E., Coeur D’Acier A., Rasplus J.-Y., Faure E. (2004). High similarity between flanking regions of different microsatellites detected within each of two species of Lepidoptera: Parnassius apollo and Euphydryas aurinia. Mol. Ecol. 13, 1693–1700. doi: 10.1111/j.1365-294X.2004.02163.x
Meirmans P. G. (2014). Nonconvergence in Bayesian estimation of migration rates. Mol. Ecol. Resour 14, 726–733. doi: 10.1111/1755-0998.12216
Meister H., Lindman L., Tammaru T. (2015). Testing for local monophagy in the regionally oligophagous Euphydryas aurinia (Lepidoptera: Nymphalidae). J. Insect Conserv. 19, 691–702. doi: 10.1007/s10841-015-9792-3
Mikheyev A. S., Vo T., Wee B., Singer M. C., Parmesan C. (2010). Rapid microsatellite isolation from a butterfly by de novo transcriptome sequencing: performance and a comparison with AFLP-derived distances. PloS One 5, e11212. doi: 10.1371/journal.pone.0011212
Miller M. P., Pratt G. F., Mullins T. D., Haig S. M. (2014). Comparisons of Genetic Diversity in Captive Versus Wild Populations of the Federally Endangered Quino Checkerspot Butterfly (Euphydryas editha quino Behr; Lepidoptera: Nymphalidae). Proc. Entomol Soc. Washington 116, 80. doi: 10.4289/0013-8797.116.1.80
Nakahama N. (2021). Museum specimens: An overlooked and valuable material for conservation genetics. Ecol. Res. 36, 13–23. doi: 10.1111/1440-1703.12181
Nakahama N., Isagi Y. (2017). Availability of short microsatellite markers from butterfly museums and private specimens. Entomol. Sci. 20, 3–6. doi: 10.1111/ens.12215
Nève G., Meglécz E. (2000). Microsatellite frequencies in different taxa. Trends Ecol. Evol. 15, 376–377. doi: 10.1016/s0169-5347(00)01921-2
New T. R. (2022). “Facilitating conservation Progress,” in Insect diversity, declines and conservation in Australia Fascinating Life Sciences (Springer International Publishing, Cham), 163–189. doi: 10.1007/978-3-030-90134-9_9
Nikolic N., Chevalet C. (2014). Detecting past changes of effective population size. Evol. Appl. 7, 663–681. doi: 10.1111/eva.12170
Pertoldi C., Ruiz-Gonzalez A., Bahrndorff S., Renee Lauridsen N., Nisbeth Henriksen T., Eskildsen A., et al. (2021). Strong isolation by distance among local populations of an endangered butterfly species (Euphydryas aurinia). Ecol. Evol. 11, 12790–12800. doi: 10.1002/ece3.8027
Petenian F., Meglécz E., Genson G., Rasplus J.-Y., Faure E. (2005). Isolation and characterization of polymorphic microsatellites in Parnassius apollo and Euphydryas aurinia (Lepidoptera). Mol. Ecol. Notes 5, 243–245. doi: 10.1111/j.1471-8286.2005.00891.x
Peterman W. E. (2018). ResistanceGA: An R package for the optimization of resistance surfaces using genetic algorithms. Methods Ecol. Evol. 9, 1638–1647. doi: 10.1111/2041-210X.12984
Poelstra J. W., Montero B. K., Lüdemann J., Yang Z., Rakotondranary S. J., Hohenlohe P., et al. (2022). RADseq data reveal a lack of admixture in a mouse lemur contact zone contrary to previous microsatellite results. Proc. Biol. Sci. 289, 20220596. doi: 10.1098/rspb.2022.0596
Pompanon F., Bonin A., Bellemain E., Taberlet P. (2005). Genotyping errors: causes, consequences, and solutions. Nat. Rev. Genet. 6, 847–859. doi: 10.1038/nrg1707
Porter K. (1983). Multivoltinism in Apanteles bignellii and the influence of weather on synchronisation with its host Euphydryas aurinia. Entomol. Exp. Appl. 34, 155–162. doi: 10.1111/j.1570-7458.1983.tb03311.x
Porter K., Ellis S. (2011). Securing viable metapopulations of the Marsh Fritillary butterfly, Euphydryas aurinia, (Lepidoptera: Nymphalidae) in Northern England. J. Insect Conserv. 15, 111–119. doi: 10.1007/s10841-010-9328-9
Primmer C. R., Moller A. P., Ellegren H. (1996). A wide-range survey of cross-species microsatellite amplification in birds. Molec. Ecol. 5, 365–378. doi: 10.1046/j.1365-294X.1996.00092.xLLEG
Pritchard J. K., Stephens M., Donnelly P. (2000). Inference of population structure using multilocus genotype data. Genetics 155, 945–959. doi: 10.1093/genetics/155.2.945
Putman A. I., Carbone I. (2014). Challenges in analysis and interpretation of microsatellite data for population genetic studies. Ecol. Evol. 4, 4399–4428. doi: 10.1002/ece3.1305
Queirós J., Godinho R., Lopes S., Gortazar C., de la Fuente J., Alves P. C. (2015). Effect of microsatellite selection on individual and population genetic inferences: an empirical study using cross-specific and species-specific amplifications. Mol. Ecol. Resour 15, 747–760. doi: 10.1111/1755-0998.12349
Riaz S., Johnson J. B., Ahmad M., Fitt G. P., Naiker M. (2021). A review on biological interactions and management of the cotton bollworm, Helicoverpa armigera (Lepidoptera: Noctuidae). J. Appl. Entomol 145, 467–498. doi: 10.1111/jen.12880
Rosa A. H. B., Ribeiro D. B., Freitas A. V. L. (2023). How data curation and new geographical records can change the conservation status of threatened Brazilian butterflies. J. Insect Conserv. 27, 403–414. doi: 10.1007/s10841-023-00464-0
Rutkowski R., Sielezniew M., Szostak A. (2009). Contrasting levels of polymorphism in cross-amplified microsatellites in two endangered xerothermophilous, obligatorily myrmecophilous, butterflies of the genus Phengaris (Maculinea) (Lepidoptera: Lycaenidae). Eur. J. Entomol. 106, 457–469. doi: 10.14411/eje.2009.058
Saarinen E. (2015). “Butterfly Conservation Genetics,” in Butterfly conservation in North America. Ed. Daniels J. C. (Springer Netherlands, Dordrecht), 75–101. doi: 10.1007/978-94-017-9852-5_5
Saarinen E. V., Austin J. (2010). When technology meets conservation: increased microsatellite marker production using 454 genome sequencing on the endangered Okaloosa darter (Etheostoma okaloosae). J. Hered. 101, 784–788. doi: 10.1093/jhered/esq080
Saarinen E. V., Daniels J. C. (2012). Using museum specimens to assess historical distribution and genetic diversity in an endangered butterfly. Anim. Biol. 62, 337–350. doi: 10.1163/157075612X624176
Saarinen E. V., Reilly P. F., Austin J. D. (2016). Conservation genetics of an endangered grassland butterfly (Oarisma poweshiek) reveals historically high gene flow despite recent and rapid range loss. Insect Conserv. Divers. 9, 517–528. doi: 10.1111/icad.12192
Satam H., Joshi K., Mangrolia U., Waghoo S., Zaidi G., Rawool S., et al. (2023). Next-generation sequencing technology: current trends and advancements. Biol. (Basel) 12, 997–1022. doi: 10.3390/biology12070997
Schmidt C., Hoban S., Hunter M., Paz-Vinas I., Garroway C. J. (2023). Genetic diversity and IUCN Red List status. Conserv. Biol. 37, e14064. doi: 10.1111/cobi.14064
Scott K. D., Lange C. L., Scott L. J., Graham G. C. (2004). Isolation and characterization of microsatellite loci from Helicoverpa armigera Hübner (Lepidoptera: Noctuidae). Mol. Ecol. Notes 4, 204–205. doi: 10.1111/j.1471-8286.2004.00617.x
Scott K. D., Wilkinson K. S., Merritt M. A., Scott L. J., Lange C. L., Schutze M. K., et al. (2003). Genetic shifts in Helicoverpa armigera Hübner (Lepidoptera: Noctuidae) over a year in the Dawson/Callide Valleys. Aust. J. Agric. Res. 54, 739. doi: 10.1071/AR02164
Selkoe K. A., Toonen R. J. (2006). Microsatellites for ecologists: a practical guide to using and evaluating microsatellite markers. Ecol. Lett. 9, 615–629. doi: 10.1111/j.1461-0248.2006.00889.x
Seraphim N., Barreto M. A., Almeida G. S. S., Esperanço A. P., Monteiro R. F., Souza A. P., et al. (2016). Genetic diversity of Parides ascanius (Lepidoptera: Papilionidae: Troidini): implications for the conservation of Brazil’s most iconic endangered invertebrate species. Conserv. Genet. 17, 533–546. doi: 10.1007/s10592-015-0802-5
Sielezniew M., Patricelli D., Rutkowski R., Witek M., Bonelli S., Buś M. M. (2015). Population genetics of the endangered obligatorily myrmecophilous butterfly Phengaris (=Maculinea) arion in two areas of its European range. Insect Conserv. Diversity 8, 505–516. doi: 10.1111/icad.12129
Sigaard P., Pertoldi C., Madsen A. B., Søgaard B., Loeschcke V. (2008). Patterns of genetic variation in isolated Danish populations of the endangered butterfly Euphydryas aurinia. Biol. J. Linn. Soc. 95, 677–687. doi: 10.1111/j.1095-8312.2008.01078.x
Smee M. R., Pauchet Y., Wilkinson P., Wee B., Singer M. C., ffrench-Constant R. H., et al. (2013). Microsatellites for the marsh fritillary butterfly: de novo transcriptome sequencing, and a comparison with amplified fragment length polymorphism (AFLP) markers. PloS One 8, e54721. doi: 10.1371/journal.pone.0054721
Strange J. P., Knoblett J., Griswold T. (2009). DNA amplification from pin-mounted bumble bees (Bombus) in a museum collection: effects of fragment size and specimen age on successful PCR. Apidologie 40, 134–139. doi: 10.1051/apido/2008070
Sucháčková Bartoňová A., Linke D., Klečková I., de G. Ribeiro P., Matos-Maraví P. (2023). Incorporating genomics into insect conservation: Butterflies as a model group. Insect Conserv. Diversity 16, 427–440. doi: 10.1111/icad.12643
Sunde J., Yıldırım Y., Tibblin P., Forsman A. (2020). Comparing the performance of microsatellites and RADseq in population genetic studies: analysis of data for pike (Esox lucius) and a synthesis of previous studies. Front. Genet. 11. doi: 10.3389/fgene.2020.00218
Szatmári L., Cserkész T., Laczkó L., Lanszki J., Pertoldi C., Abramov A. V., et al. (2021). A comparison of microsatellites and genome-wide SNPs for the detection of admixture brings the first molecular evidence for hybridization between Mustela eversmanii and M. putorius (Mustelidae, Carnivora). Evol. Appl. 14, 2286–2304. doi: 10.1111/eva.13291
Taberlet P., Griffin S., Goossens B., Questiau S., Manceau V., Escaravage N., et al. (1996). Reliable genotyping of samples with very low DNA quantities using PCR. Nucleic Acids Res. 24, 3189–3194. doi: 10.1093/nar/24.16.3189
Taberlet P., Waits L. P., Luikart G. (1999). Noninvasive genetic sampling: look before you leap. Trends Ecol. Evol. 14, 323–327. doi: 10.1016/S0169-5347(99)01637-7
Tan S., Chen X., Zhang A., Li D. (2001). Isolation and characterization of DNA microsatellite from cotton bollworm (Helicoverpa armigera, Hübner). Mol. Ecol. Notes 1, 243–244. doi: 10.1046/j.1471-8278.2001.00090.x
Tautz D., Renz M. (1984). Simple sequences are ubiquitous repetitive components of eukaryotic genomes. Nucleic Acids Res. 12, 4127–4138. doi: 10.1093/nar/12.10.4127
Tay W. T., Behere G. T., Batterham P., Heckel D. G. (2010). Generation of microsatellite repeat families by RTE retrotransposons in lepidopteran genomes. BMC Evol Biol 10, 144. doi: 10.1186/1471-2148-10-144
Tibihika P. D., Curto M., Dornstauder-Schrammel E., Winter S., Alemayehu E., Waidbacher H., et al. (2018). Application of microsatellite genotyping by sequencing (SSR-GBS) to measure genetic diversity of the East African Oreochromis niloticus. Conserv. Genet. 20, 357–372. doi: 10.1007/s10592-018-1136-x
Tracy L. N., Jamieson I. G. (2011). Historic DNA reveals contemporary population structure results from anthropogenic effects, not pre-fragmentation patterns. Conserv. Genet. 12, 517–526. doi: 10.1007/s10592-010-0158-9
Ugelvig L. V., Nielsen P. S., Boomsma J. J., Nash D. R. (2011). Reconstructing eight decades of genetic variation in an isolated Danish population of the large blue butterfly Maculinea arion. BMC Evol. Biol. 11, 201. doi: 10.1186/1471-2148-11-201
Valière N., Bonenfant C., Toïgo C., Luikart G., Gaillard J.-M., Klein F. (2007). Importance of a pilot study for non-invasive genetic sampling: genotyping errors and population size estimation in red deer. Conserv. Genet. 8, 69–78. doi: 10.1007/s10592-006-9149-2
van Strien M. J., Holderegger R., Van Heck H. J. (2015). Isolation-by-distance in landscapes: considerations for landscape genetics. Heredity 114, 27–37. doi: 10.1038/hdy.2014.62
Vartia S., Villanueva-Cañas J. L., Finarelli J., Farrell E. D., Collins P. C., Hughes G. M., et al. (2016). A novel method of microsatellite genotyping-by-sequencing using individual combinatorial barcoding. R. Soc Open Sci. 3, 150565. doi: 10.1098/rsos.150565
Vieira M. L. C., Santini L., Diniz A. L., de Freitas Munhoz C. (2016). Microsatellite markers: what they mean and why they are so useful. Genet. Mol. Biol. 39, 312–328. doi: 10.1590/1678-4685-GMB-2016-0027
Vila M., Latasa T., Pino J. J., Verhulst G. (2009). Characterization of ten polymorphic microsatellite markers for the endemic Chapman’s ringlet, Erebia palarica (Lepidoptera: Nymphalidae). Eur. J. Entomol. 106, 485–490. doi: 10.14411/eje.2009.061
Vila M., Marí-Mena N., Yen S.-H., Lopez-Vaamonde C. (2010). Characterization of ten polymorphic microsatellite markers for the protected Spanish moon moth Graellsia isabelae (Lepidoptera: Saturniidae). Conserv. Genet. 11, 1151–1154. doi: 10.1007/s10592-009-9905-1
Wang I. J. (2010). Recognizing the temporal distinctions between landscape genetics and phylogeography. Mol. Ecol. 19, 2605–2608. doi: 10.1111/j.1365-294X.2010.04715.x
Warren M. S. (1994). The UK status and suspected metapopulation structure of a threatened European butterfly, the marsh fritillary Euphydryas aurinia. Biol. Conserv. 67, 239–249. doi: 10.1016/0006-3207(94)90615-7
Weeks A. R., Endersby N. M., Lange C. L., Lowe A., Zalucki M. P., Hoffmann A. A. (2010). Genetic variation among Helicoverpa armigera populations as assessed by microsatellites: a cautionary tale about accurate allele scoring. Bull. Entomol. Res. 100, 445–450. doi: 10.1017/S0007485309990460
Whitlock M. C. (2011). G’ST and D do not replace FST. Mol. Ecol. 20, 1083–1091. doi: 10.1111/j.1365-294X.2010.04996.x
Willi Y., Kristensen T. N., Sgrò C. M., Weeks A. R., Ørsted M., Hoffmann A. A. (2022). Conservation genetics as a management tool: The five best-supported paradigms to assist the management of threatened species. Proc. Natl. Acad. Sci. U.S.A. 119, 1–10. doi: 10.1073/pnas.2105076119
Williams B. L., Brawn J. D., Paige K. N. (2003). Landscape scale genetic effects of habitat fragmentation on a high gene flow species: Speyeria idalia (Nymphalidae). Mol. Ecol. 12, 11–20. doi: 10.1046/j.1365-294x.2003.01700.x
Wilson G. A., Rannala B. (2003). Bayesian inference of recent migration rates using multilocus genotypes. Genetics 163, 1177–1191. doi: 10.1093/genetics/163.3.1177
Wright S. (1978). Evolution and the genetics of populations, volume 4: Variability Within and Among Natural Populations (Variability Within & Among Natural Populations). New edition (Chicago: University of Chicago Press).
Yuan S. C., Malekos E., Hawkins M. T. R. (2021). Assessing genotyping errors in mammalian museum study skins using high-throughput genotyping-by-sequencing. Conserv. Genet. Resour. 13, 303–317. doi: 10.1007/s12686-021-01213-8
Zakharov E. V., Hellmann J. J., Romero-Severson J. (2007). Microsatellite loci in the Propertius duskywing, Erynnis propertius (Lepidoptera: Hesperiidae), and related species. Mol. Ecol. Notes 7, 266–268. doi: 10.1111/j.1471-8286.2006.01576.x
Zeisset I., Damm Als T., Settele J., Boomsma J. J. (2005). Microsatellite markers for the large blue butterflies Maculinea nausithous and Maculinea alcon (Lepidoptera: Lycaenidae) and their amplification in other Maculinea species. Mol. Ecol. Notes 5, 165–168. doi: 10.1111/j.1471-8286.2004.00872.x
Zhan L., Paterson I. G., Fraser B. A., Watson B., Bradbury I. R., Nadukkalam Ravindran P., et al. (2017). megasat: automated inference of microsatellite genotypes from sequence data. Mol. Ecol. Resour. 17, 247–256. doi: 10.1111/1755-0998.12561
Zhang D.-X. (2004). Lepidopteran microsatellite DNA: redundant but promising. Trends Ecol. Evol. 19, 507–509. doi: 10.1016/j.tree.2004.07.020
Zima J., Lestina D., Jansta P., Petru V., Tropek R. (2014). Isolation and characterisation of microsatellite markers for Mylothris jacksoni knutsoni (Lepidoptera: Pieridae), an endemic butterfly of the Gulf of Guinea Highlands. Conserv. Genet. Resour. 6, 763–764. doi: 10.1007/s12686-014-0209-5
Keywords: Lepidoptera, microsatellite, next-generation, observed heterozygosity, population genetics
Citation: Heffernan E, Barkdull M and Brady N (2024) Microsatellites for butterfly conservation: historical challenges, current relevance, and a guide to implementation. Front. Ecol. Evol. 12:1344065. doi: 10.3389/fevo.2024.1344065
Received: 24 November 2023; Accepted: 23 April 2024;
Published: 09 May 2024.
Edited by:
Max Post van der Burg, United States Department of the Interior, United StatesReviewed by:
Martin Konvicka, University of South Bohemia in České Budějovice, CzechiaSara J. Oyler-McCance, United States Department of the Interior, United States
Copyright © 2024 Heffernan, Barkdull and Brady. This is an open-access article distributed under the terms of the Creative Commons Attribution License (CC BY). The use, distribution or reproduction in other forums is permitted, provided the original author(s) and the copyright owner(s) are credited and that the original publication in this journal is cited, in accordance with accepted academic practice. No use, distribution or reproduction is permitted which does not comply with these terms.
*Correspondence: Emily Heffernan, eheffernan@ncf.edu
†These authors have contributed equally to this work and share first authorship