- Faculty of Forestry, Central South University of Forestry and Technology, Changsha, Hunan, China
Introduction: In the aftermath of a fire, prompt reforestation of the affected areas is crucial to mitigate economic losses and ecological impacts.
Methods: This paper introduces an ecological function assessment model leveraging the Back Propagation Neural Network (BPNN). The model's efficacy is validated through simulation comparison experiments. Subsequently, an analysis of the ecosystem's material circulation and energy flow capabilities is undertaken.
Results: Simulation outcomes reveal that our proposed model attains convergence by the 10th training iteration, with a loss function value of just 0.28, highlighting minimal training loss. This underscores the model's rapid convergence and impressive training performance. Our method proves superior to the comparison method in both initial and later operational phases. Notably, it offers a significantly faster response speed and boasts an accuracy rate exceeding 95%.
Discussion: Consequently, employing this model to analyze ecological function changes is deemed feasible. The analysis of ecosystem material circulation and energy flow capabilities reveals that while initial assessments show minimal change, scores exhibit a clear acceleration as the cycle progresses.
1 Introduction
The renewal of natural materials is an important means to continue forest resources and the basis for sustainable utilization of forests. The purpose of the investigation on the renewal of natural materials is to understand the natural renewal of forest areas, evaluate the renewal level, and provide scientific basis for forest ecological functions and organizing forestry production (Costa et al., 2018). In forest ecosystem, fire is the most serious and prone risk factor. Fire will not only damage forest resources, but also affect the stability and development of forest ecosystem, and also cause incalculable economic losses (Othoniel et al., 2015). At the moment of the fire, the whole forest was set on fire. At the same time, in the process of burning trees, it will have a great impact on the environment (Smith et al., 2015). Vegetation restoration after fire is an important problem that people are facing, which needs higher afforestation and regeneration technology as support to restore the diversity of vegetation and ensure the stable survival of forest ecosystem (Zhang et al., 2015). With the acceleration of economic construction in China, environmental protection has been put on the agenda, and forests play a great role in environmental protection and ecological maintenance (Qiting et al., 2015). Afforestation and regeneration in fire slash is an effective method to restore forest vegetation, and it is also the need to maintain the balance of forest ecosystem. It is of great significance to carry out afforestation regeneration in fire slash, so we should pay more attention to forest protection. It is a long process to correctly understand the reforestation of fire slash, and take effective remedial measures for fire slash, and analyze the reasons for the formation of fire slash in detail (Rosatti et al., 2018).
Renewing forest resources and maintaining the balance of ecosystem can avoid serious damage to forest resources and ensure the stable development of forest ecosystem (Coston-Guarini and Guarini, 2017). In order to ensure the continuous reproduction and protection function of wood, for a long time, people have carried out extensive research and application on forest artificial regeneration and made great achievements (Milievi et al., 2020). However, under some site conditions, or the regeneration of some tree species, or the restoration of the forest climax community that is most suitable for local natural conditions, artificial regeneration seems powerless (Woods, 2021). When burning trees, it will destroy soil, groundwater, atmosphere and animal habitat, and then break the stability of material circulation, information transmission and ecological balance. Artificial promotion of natural regeneration refers to the selection of mother trees for planting on the forested land or suitable forested land, or the use of the regenerative ability of forest nutrients left on the forested land, combined with artificial measures such as chopping grass, loosening soil and replanting, to create favorable conditions for providing seeds, seed germination and seedling growth, so as to achieve the purpose and method of natural material regeneration (Kimuyu et al., 2017). In this article, an ecological function assessment model based on BPNN is constructed, and the potential relationship among factors affecting ecological function is excavated. Based on this, the material circulation and energy flow capacity of the ecosystem are analyzed, and the influence of artificial promotion and renewal of fire slash on ecological function is studied from the perspective of materials science.
The restoration and reconstruction of fire slash forest ecosystem is the key research direction of forest fire ecology and restoration ecology. The size and spatial pattern of fire have great influence on vegetation restoration, and this influence is continuous (Pushkar, 2019). From another angle, fire is beneficial, which promotes the evolution and succession of forest ecosystem, makes those trees that should have been eliminated degenerate in advance, promotes the growth and development of new adaptive trees, and has far-reaching significance for the renewal of ecosystem (Richards et al., 2018). Regeneration of forestland is an important content of forest management according to law. Improving the long-term mechanism of supervision and management of regeneration of forestland and the afforestation and regeneration technology of fire slash can reduce the negative impact of fire on forest resources (Wang et al., 2020). Through the integration of anomaly detection and correlation analysis within deep learning frameworks, the exploration of forest ecological function issues reveals underlying patterns amidst extensive ecosystem datasets. This piece introduces an ecological function assessment model rooted in BPNN, showcasing noteworthy advancements and contributions:
(1) We delve into the current state and prevalent challenges of artificial promotion and ecological function enhancement in forest fire slash areas, thereby investigating technical strategies for afforestation and regeneration.
(2) Our study employs a BPNN-driven ecosystem assessment system to scrutinize forest ecological function data, unveiling the subtle influence of class structures and ecosystem factors concealed within dataset attributes on clustering outcomes.
(3) Leveraging a deep learning algorithm, our constructed model forecasts the test dataset, shedding light on intricate relationships between ecosystem challenges and their respective attributes.
This paper initially highlights the significance of post-fire reforestation. Subsequently, it establishes an ecological function evaluation model utilizing the BPNN and confirms its efficacy through rigorous simulation and comparative experiments. Following this, the paper employs this model to scrutinize the material circulation and energy flow capabilities of the ecosystem, thereby illustrating the model’s training proficiency and superiority. Ultimately, by analyzing the outcomes of the ecosystem’s material circulation and energy flow capabilities, the paper derives pertinent conclusions.
2 Related work
Studying the reconstruction of fire slash ecosystems is pivotal in forestry development research due to the profound impact of disasters. Numerous experts have conducted extensive research on various aspects of forest fire slash, including regeneration, site conditions, vegetation restoration, and succession laws. Their findings have been documented in a series of investigation reports and research papers. According to Palmquist et al., the restoration of forest ecosystems after a significant fire relies heavily on favorable climatic conditions (Palmquist et al., 2015). The spatial distribution and magnitude of fires exert persistent effects on vegetation, albeit varying in impact. Alternatively, fire plays a crucial role in promoting the renewal and succession of forest vegetation, thereby contributing to the regeneration of forest ecosystems.
Bremer et al. delved into the spatial distribution characteristics of diverse ecological factors and the influence of ecological changes, establishing assessment criteria and factors that led to the construction of a remote sensing monitoring and assessment model for ecological changes following forest fires (Bremer et al., 2016). Herrero et al. emphasized the importance of tailored regeneration measures based on vertical zones, advocating a combination of direct seeding afforestation and artificially promoting natural regeneration in alignment with the ecological characteristics of plant communities and their forest composition, distribution, growth, and regeneration patterns (Herrero and Torres, 2016).
From a community perspective, Liu et al. investigated forest succession across different regions and burning severities, leading to the development of a forest succession model tailored to various succession trends (Liu et al., 2017). Tarrason et al. introduced a model to determine the number of naturally regenerating trees in fire-affected secondary forests, facilitating the rationalization of restored forest structures through adjustments to uneven distributions and the implementation of classified forestry management (Tarrason et al., 2016).
Malekmohammadi et al. explored the relationship among various factors within the forest combustion ring, including types of combustible materials, fire sources, fire environments, and fire behaviors, outlining measures for forest restoration (Malekmohammadi and Jahanishakib, 2017). Li et al. examined the landscape-level impact of fires and the influence of fire disturbances on forest landscape patterns through an extensive analysis of fire-scarred trees (Li et al., 2019). Xu et al. emphasized the importance of managing forest ecosystems in fire slash restoration, aiming to achieve a balanced proportion of trees, shrubs, grasses, plants, and animals, thereby maintaining a virtuous cycle within the system (Xu et al., 2018). Furthermore, Herrero et al. studied the effects of fire on nutrient cycling within forest ecosystems, providing valuable insights for predicting vegetation restoration trends in fire-affected areas (Jiyu et al., 2022).
In this paper, we build upon this wealth of research by constructing an ecological function evaluation model based on BPNN. This model explores the laws and characteristics of forest ecosystem renewal and natural material renewal, delving into the underlying relationships between various factors that influence ecological function. Based on these findings, we analyze the material circulation and energy flow capabilities of the ecosystem, aiming to provide a comprehensive understanding of the post-fire ecological recovery process.
3 Ecological function assessment model based on BPNN
3.1 Function of natural material regeneration for afforestation in burned land
The natural factors that cause forest fires cannot be controlled artificially since ancient times, but what we can do is to minimize human factors and take remedial measures in time after the disaster to reduce the impact of forest fires. On the one hand, forest fire can really help some vegetation renewal, but the adverse effects of forest fire are far greater than this. When carrying out afforestation and regeneration in fire slash, we should consider the suitable growth environment of different tree species and formulate scientific regeneration methods. Fire is an important factor affecting forest ecosystem. The occurrence of fire leads to the decrease of trees in a large area of forest, which destroys the ecological balance in nature and hinders the material circulation and energy flow in the biosphere. In the reforestation technology of fire slash, soil improvement after fire slash is also a key work, and the trees with no use value and plants that can’t continue to grow should be removed in time to avoid the damage of pests to the original plants. Fire slash needs to be leveled and repaired effectively to provide better environmental conditions for the growth of plants, and different leveling should be adopted for sloping land peacefully to avoid the problem of soil erosion.
There are many reasons for the fire, most of which are uncontrollable factors. For the controllable factors, it is necessary to nip in the bud, minimize the damage caused by human factors to the forest, avoid fire hazards, and at the same time, do a good job in post-disaster remedial work in time to reduce the adverse effects of the fire. The reconstruction of fire slash forest ecosystem is the key research direction of forestry development. Once a forest fire occurs, it will bring serious losses to forest resources, and the size of the fire has a great impact on vegetation restoration (Jiyu et al., 2022). Before reforestation, a detailed investigation should also be made on the situation of fire slash. For those with natural regeneration conditions, the method of closing hillsides for afforestation can be adopted, and for those with artificial measures to assist closing hillsides for afforestation, the method of artificially promoting natural regeneration can be adopted. In forestry management, an important task is to restore afforestation and vegetation of fire slash, which is of great practical significance for ecological research (Jones et al., 2022). In the whole process of fire slash reforestation, it is very important to lead the work of leaders and clarify the division of labor and cooperation among staff. In the cleaning work of fire slash, the residues that need to be cleaned should be selected, and the residues that are of no use value should be cleaned to avoid the spread of pests and diseases. At the same time, fire has a positive effect on forest ecosystem. Because the composition of plant community is different in different degrees, the size of fire also has a great influence on the recovery of vegetation in the later period. The screening process of ecological function assessment indicators is shown in Figure 1.
When selecting tree species for post-fire reforestation, specific guidelines must be adhered to. For various soil types, it’s crucial to opt for tree varieties that can flourish in those conditions. Additionally, preference should be given to species possessing inherent fire resistance and robustness against external environmental fluctuations. Furthermore, the surrounding environment’s influence must be taken into account to ensure the chosen species can adapt seamlessly and prosper in the long run. In evaluating forest ecological function, advanced data mining techniques are employed to delve into the underlying issues, assess ecological function based on extensive datasets, and identify the key factors impacting it. This approach aids in addressing forest ecological challenges effectively. The artificial neural network, which mimics the structure and information processing of brain neurons, excels in automatically identifying the relationship between input and output data through rigorous training. This autonomous learning process eliminates the need for human intervention, requiring only fine-tuning of training parameters. For the identification algorithm of forest ecological function problems based on multi-source data, the forest resources with ecological function problems can be identified to some extent.
3.2 Significance of strengthening forest ecological function for wood supply
The large-scale reduction of forests will cause soil erosion, land desertification and other problems. Landslides, mudslides and perhaps the reduction of trees in many areas have inseparable reasons. Therefore, strengthening forest ecological function is not only beneficial to nature, but more importantly, creating a good living environment for future generations. In the process of forest growth, its growth form is often uncontrolled, so forest development is often based on natural laws. In the process of forest ecological function, we should not only rely on the natural growth of trees, but also supplement the application of artificial control measures to selectively control plant growth. Wood plays a vital role in construction sites, furniture manufacturers, shipbuilding, paper industry and so on. It is the raw material on which these industries depend. Fortunately, wood, a forest resource, is renewable, and its consumption is relatively small among all resources. However, in recent years, the vegetation coverage of many surfaces has shown a downward trend, and excessive logging will eventually lead to national disaster.
Forest ecological function is rich in content and requires high technology. Therefore, in the process of forest ecological function, advanced and scientifically used technology should be actively applied to promote forestry development. At the same time, a forest ecological function management system should be established and improved in combination with China’s national conditions and forest conditions. In the process of forest ecological function management, we should pay attention to updating the technical specifications of forest ecological function and basic forestry data in time. No matter what kind of imported resources are profiteering, although we can choose to import when there is a shortage of wood in our country, it is not a long-term solution. When forest resources disappear, importing more wood will not help. Wood supply and forest protection are not two conflicting concepts. The main purpose of forest ecological function is to develop a sustainable and healthy ecosystem, and wood supply is the key factor to develop China’s economy. Artificial tending requires clearing the trees with relatively small tree spacing and optimizing the structure of tree species. If the growth form of mature forest threatens the growth of effective trees, it can be selectively cut down, and at the same time, it is necessary to keep proper litter to ensure the rationality of forest structure.
3.3 Evaluation model of the impact of natural material renewal on ecological function
For a long time, most of the traditional trees have been planted artificially after harvesting. The proportion of the remaining natural broad-leaved forest in Yanping District has been decreasing year by year. Coupled with serious man-made damage, it has been replaced by a large area of artificial coniferous forest. The distribution of forest structure is extremely uneven, the ecosystem is deteriorating, and disasters such as wind, sand, water, drought and insects are intensifying. After the forest fire, it is necessary to clean up the residual branches and stumps in time to avoid pests. At the same time, the weak and degraded vegetation types should be eliminated, and the intact tree species, especially the precious tree species, should be protected to avoid secondary damage. Only by thoroughly cleaning up the places burned by the fire can we provide a good foundation for afforestation and regeneration in the later period. The focus of ecological function assessment is data analysis, and the basis is data collection. By establishing forest ecological function information base and archives, the ecological characteristics and laws of forest resources are observed and analyzed.
In this study, the BPNN is used to construct the ecological function evaluation model, which consists of input, hidden and output layers. The key ecological factors are selected in the input layer, and the time lag is analyzed by combining autocorrelation and partial autocorrelation functions to determine the appropriate lag input. The hidden layer captures the nonlinear relationship through multi-layer design and Sigmoid activation function. The output layer focuses on reflecting the material circulation and energy flow ability of the ecosystem. The model is optimized by random gradient descent algorithm, and early stop and regularization strategies are introduced to prevent over-fitting. In data preprocessing, we interpolate to complete missing data, standardize to eliminate dimensional differences, and use time correlation analysis method to select lagging inputs, thus ensuring the effectiveness, repeatability and generalization ability of the model.
After the restoration of fire slash, the diversity of vegetation will be maintained and the ecological cycle of forest will be promoted. Build a forest ecological security system to maintain the balance of the ecosystem. In the process of forest ecological function assessment, we should strengthen the application of network and big data to establish ecological function data files for forest resources. The BPNN model of ecological function assessment is shown in Figure 2.
Proper data gathering offers a dependable foundation for data mining, as ample datasets alone can bolster its credibility and persuasiveness. Through the process of clustering, key attributes can be delineated, thereby facilitating a more comprehensive elucidation of abnormal data causes. Given that the raw data dimensions of the chosen indicators diverge, posing challenges for comparison or yielding inconclusive results, they undergo dimensionless processing prior to grey correlation analysis, as shown in Equations 1, 2:
Calculate the correlation coefficient with Equation 3:
In the formula, and are the standardized values of ecological function indicators at time , respectively, and is the resolution coefficient. The larger the value, the smaller the resolution. Usually is the correlation coefficient of the moment.
Reforestation can reduce the accumulation of combustible materials by increasing vegetation coverage and biodiversity, and then slow down the spread of fire. The selection of specific fire-resistant tree species can form a natural fire barrier, improve soil structure, improve water retention capacity, and thus reduce fire risk (Iossa, 2022). In this study, BPNN algorithm is adopted. Because of its powerful nonlinear processing and self-learning ability, it can capture the complex relationship of ecological restoration in burned areas. In order to ensure the accuracy of the model, we have preprocessed the outliers. BPNN not only helps us to quantitatively analyze the changes of ecological functions, but also provides scientific support for future restoration strategies.
Fire plays a significant role in shaping forest ecosystems, and large-scale forest fires can have detrimental impacts on the forest environment. These fires disrupt the ecological balance among various forest components such as the atmosphere, water, and soil to different extents, thereby affecting material circulation, energy flow, and information transmission, ultimately leading to imbalances within the forest ecosystem. A sustainable society relies on several factors, including the continuous supply of physical resources, the harmonious integration of production, lifestyle, and ecological functions, effective macro-level societal control, coordinated efforts among various departments, and public awareness regarding supervision and participation. Let’s designate as an observable variable encompassing ecological variables denoted as . These variables are interconnected with the -dimensional vector . The measurement equation for this relationship is defined as follows (Equations 4, 5):
Herein, denotes the sample length, signifies the matrix, and corresponds to the vector. Moreover, is indicative of the vector, a continuously uncorrelated disturbance term possessing a mean of 0 and covariance matrix :
Typically, the components of are not directly observable; however, they can be expressed through a first-order Markov process. Thus, the state equation is formulated as follows (Equations 6, 7):
Within this context, stands for the matrix, signifies the vector, and denotes the matrix. Additionally, represents a randomly uncorrelated disturbance term with a mean of 0 for the vector and a covariance designated by :
Provided that economic progress does not outweigh the ecological system’s carrying capacity, swift economic growth and enhanced life satisfaction for individuals can coexist (Gilby et al., 2023). The long-term progression and succession of forest ecosystems hinge significantly on afforestation and the regeneration of areas affected by fire. Simultaneously, examining fire’s influence on forest ecosystems is crucial for their enhancement and preservation, further promoting their regeneration and advancement. Since fire-affected areas often harbor young tree species, special safeguards are essential. To ensure these young trees’ stable survival despite microclimatic fluctuations, it’s advisable to employ group planting methods while preserving the existing natural saplings.
Coordination typically involves enhancing the population’s quality and balancing environmental and developmental needs, aiming for harmony between humans, nature, and society. Alternatively, it signifies the level of harmony or alignment achieved during the coordinated development of systems or elements, reflecting a shift from chaos to orderliness. The main idea of grey relational analysis is to find out whether the numerical relations between systems or subsystems are closely related according to certain methods. Set the factor set and the assessment grade set of ecological function assessment object (Equations 8, 9):
A fuzzy assessment is conducted for each factor within , utilizing the grade index from the assessment set, ultimately yielding the assessment matrix, as shown in Equation 10:
In which represents ‘s membership degree with respect to . After determining the importance index of each factor, record it as (Equation 11):
Synthesis (Equation 12):
Following normalization, the subsequent outcomes are acquired (Equation 13):
In accordance with the grey relational model’s steps, the original data undergoes dimensionless processing, enabling the calculation of the correlation coefficient signifying the coupling degree between natural material renewal and environmental development. Subsequently, a correlation matrix linking natural material renewal and environmental indicators is derived, facilitating the computation of the average values for each row and column, thereby allowing for the observation of individual indicator correlations. From this correlation coefficient, the change curve depicting the coupling degree between natural material renewal and the environment is then obtained. This enables an analysis of the coupling degree’s trend over time between natural material renewal and the environment.
4 Result analysis and discussion
4.1 Performance simulation of ecological function assessment model
This study deeply realized the importance of data preprocessing, especially for non-ideal data sources. To this end, we have carefully used interpolation to fill in missing values, statistical methods to identify and eliminate abnormal values, and standardized the data to ensure the accuracy and reliability of the data. After constructing the ecological function evaluation model based on BPNN, we verified the effectiveness of the model through simulation and comparative experiments. During the experiment, we paid special attention to the material circulation and energy flow capacity of the ecosystem, and analyzed the changing trends of key indicators such as net primary productivity, soil organic carbon content and biodiversity index. These indicators are of great significance in evaluating the restoration of ecosystem functions and provide us with a comprehensive and in-depth understanding. Through comprehensive analysis of the changes of these key indicators, we have reached a scientific conclusion about the impact of different restoration measures on ecosystem functions, which provides strong theoretical support and practical guidance for future ecological restoration work.
Choose suitable neural network parameters and set up the corresponding neural network model. Next, we utilize the sample set to train the network, adjust its weights, and evaluate its performance to ascertain if the trained neural network aligns with our requirements. Following this, we procure sample data for processing, verify the attainment of the desired objective, and subsequently intervene to address ecological function issues pertaining to forest resources. The preprocessing of suboptimal data sources significantly enhances the efficiency of the ecological function assessment model and facilitates the knowledge discovery process. The establishment of an ecological archive database is an ongoing endeavor that requires continuous updating, timely collection and screening, as well as scientific analysis, summation, and evaluation. The ecological function assessment model has undergone experimental analysis, and the outcomes are presented in Figure 3.
From the convergence speed perspective, Figure 3 demonstrates that the model nearly achieves convergence by the 10th training iteration. This implies the model’s ability toswiftly grasp crucial features from the training data, rapidly approaching an optimal solution. The significance of this swift convergence lies in its practical applications, as it enhances model efficiency by reducing training durations. Remarkably, the loss function value depicted in Figure 3 stands at just 0.28. In the realm of machine learning, the loss function value serves as a pivotal indicator of model prediction accuracy. A lower loss function value signifies a smaller discrepancy between the model’s predictions and actual values, highlighting the model’s effectiveness in data fitting and learning its inherent patterns during training.
Turning to Figure 4, it is evident that the meticulously processed data exhibits notable advantages during the algorithm’s initial operational stages, resulting in a considerable enhancement in response speed. This underscores the model’s proficiency in promptly capturing essential data insights, thereby bolstering real-time decision-making capabilities. As the operation progresses, the model’s superiority becomes increasingly apparent, delivering impressive performance in terms of data fitting precision and predictive stability.
Figure 5 further verifies the high accuracy of the model. On the processed data, the accuracy of the model is above 95%, which fully proves the effectiveness of the pretreatment measures and model construction. This high accuracy not only provides us with reliable prediction results, but also lays a solid foundation for the subsequent analysis of ecological function changes.
Fuelled by the expansion of big data, the continuous growth of data holds significant importance for information management and screening. Prompt analysis and correction of data are imperative. It is essential to promptly eliminate false, outdated, and unsubstantiated information from the network while preserving authentic and dependable data. The early warning information is managed at different levels, and different levels of information are sent to staff at different levels, so as to establish and improve the tracking mechanism and management mechanism of dynamic ecological function, and lay the foundation for the smooth development of subsequent forest ecological function assessment.
4.2 Influence of natural material renewal on ecological function
Natural material renewal and ecological function constitute intricate, interconnected systems that demand more than mere qualitative analysis. Hence, developing a specific evaluation model to illustrate the relationship between natural material renewal and the environment facilitates quantitative analysis. This enables us to comprehend, assess, and address the environmental and economic situations, as well as the level of their interaction. By pinpointing constraints and bottlenecks, we can take targeted, prioritized, and incremental measures toward achieving sustainable development. In this context, BPNN serves as a tool to evaluate the material cycles and energy flows within ecosystems. Figure 6 illustrates the variations in these scores under natural development conditions. Meanwhile, Figure 7 reveals how these scores evolve with the enhancement of both artificial and natural material renewal efforts.
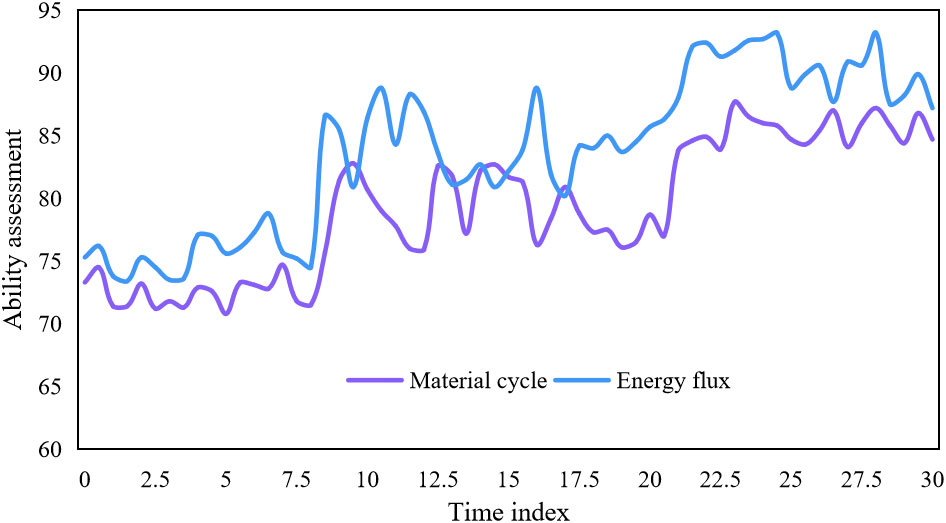
Figure 7 Scoring of material circulation and energy flow under the promotion of artificial regeneration and natural material regeneration.
The naturally developed ecosystem’s material circulation and energy flow capacity underwent predominantly chaotic variations, with minimal improvement in ecological function. In contrast, examination of the cultivated ecosystem’s material circulation and energy flow simulation results reveals noticeable progress in ecological function enhancement. While initial assessments did not indicate significant alterations in the ecosystem’s material circulation and energy flow, prolonged observation revealed a marked acceleration trend in scoring.
According to the results presented in Table 1, there was a noteworthy upward trend observed in several key ecosystem indicators during the post-fire recovery phase. These indicators include net primary productivity, soil organic carbon content, biodiversity index, material circulation rate, and energy flow efficiency. The steady enhancement of these vital metrics indicates the successful implementation of vegetation restoration efforts in facilitating carbon sequestration, soil quality enhancement, biodiversity augmentation, and efficient material and energy circulation within the ecosystem. This, in turn, has established a firm foundation for the stable and sustainable growth of the ecosystem.
The rapid convergence and small training loss of the model mean that we can evaluate the functional state of the ecosystem more efficiently, which is of great significance for monitoring and responding to ecological changes in time. In addition, our research also provides a new perspective for understanding the complex interaction within the ecosystem. The utilization of data can refine resource allocation, elevate decision-making standards and operational efficiency, and foster innovation in assessing forest ecological functions. By employing scientific methodologies, we can enhance the connectivity of data and information, thereby enabling us to scrutinize the variables within the dataset, grasp the fundamental details of forest resources, and devise precise ecological function assessments tailored to the context and insights provided by forest resources. The main findings of this study are that our model has high accuracy and efficiency in ecological function assessment. However, we also realize that the performance of the model in some complex ecosystems may be limited, which will be an important direction of future research.
The preliminary observation shows that in the first stage, the changes of material circulation and energy flow are not significant. However, considering that these processes may accelerate with the passage of time, we further analyzed the long-term data. By comparing the material circulation rate and energy flow efficiency in different time periods, it is found that these ecological processes have indeed shown an obvious acceleration trend after a period of recovery. This discovery emphasizes the importance of time factor in evaluating the process of ecosystem restoration, and provides beneficial enlightenment for future ecological restoration and management strategies. Forestry classification management strategy is a management method aiming at the supply and demand of wood products in regional ecosystem. This strategy aims to improve the yield and quality of wood products by optimizing the allocation of forest resources, while protecting the stability and diversity of ecosystems. According to the ecological function and economic value of different forest types, it can formulate corresponding management measures, such as cutting plan and planting plan. For example, in some areas, the implementation of forestry classification management strategy has successfully increased the supply of wood products and protected the ecological environment. The application of this strategy can provide useful reference for other similar areas.
5 Conclusions
Forest protection and wood supply is a long-term and lasting project. Vegetation restoration after fire is an important problem that people are facing, which needs higher afforestation and regeneration technology as support to restore the diversity of vegetation and ensure the stable survival of forest ecosystem. In this article, an ecological function assessment model based on BPNN is constructed, and the performance of the algorithm is simulated and compared, which verifies the effectiveness of the model. The simulation results show that it is feasible to use this model to analyze the change of ecological function. It can be seen from the analysis of the results of material circulation and energy flow ability of ecosystem that although the assessment of material circulation and energy flow of ecosystem did not change obviously in the early stage, the score showed an obvious acceleration trend after the cycle was prolonged. Therefore, reforestation of fire slash to enhance the fire resistance of trees can improve the survival rate of vegetation and strengthen the growth ability of trees, and maintain the diversity of biological communities as much as possible. After correctly understanding and evaluating the particularity of each region, adopting the forestry classified management strategy is conducive to improving the regional ecosystem and solving the shortage of wood products, and at the same time, it can also solve the contradiction between the social demand for various benefits of forestry and the single management goal of forestry enterprises.
Noise points may come from errors or natural variations in data collection, while abnormal points may represent real but rare events in the ecosystem. The distinction between the two is very important for accurate assessment of ecological functions. Future research can explore more advanced noise point and abnormal point detection technology to improve the accuracy of ecological function assessment. In addition, it is also a direction worth exploring in the future to study how these points affect the performance of clustering algorithm and how to optimize the algorithm to deal with these points.
Data availability statement
The original contributions presented in the study are included in the article/supplementary material. Further inquiries can be directed to the corresponding author.
Author contributions
XC: Conceptualization, Data curation, Investigation, Software, Writing – original draft. FL: Formal analysis, Funding acquisition, Methodology, Resources, Supervision, Writing – original draft, Writing – review & editing.
Funding
The author(s) declare financial support was received for the research, authorship, and/or publication of this article. This work was supported by the National Natural Science Foundation of China (31470659).
Acknowledgments
The authors would like to show sincere thanks to those techniques who have contributed to this research.
Conflict of interest
The authors declare that the research was conducted in the absence of any commercial or financial relationships that could be construed as a potential conflict of interest.
Publisher’s note
All claims expressed in this article are solely those of the authors and do not necessarily represent those of their affiliated organizations, or those of the publisher, the editors and the reviewers. Any product that may be evaluated in this article, or claim that may be made by its manufacturer, is not guaranteed or endorsed by the publisher.
References
Bremer L. L., Farley K. A., Chadwick O. A. (2016). Changes in carbon storage with land management promoted by payment for ecosystem services. Environ. Conserv. 1, 1–10. doi: 10.1017/S0376892916000199
Costa F. V., Blüthgen N., Viana-Junior A. B. (2018). Resilience to fire and climate seasonality drive the temporal dynamics of ant-plant interactions in a fire-prone ecosystem. Ecol. Indic. 93, 247–255. doi: 10.1016/j.ecolind.2018.05.001
Coston-Guarini J., Guarini J.-M. (2017). A roadmap for a quantitative ecosystem-based environmental impact assessment. ICES J. Mar. Sci. 74, 2012–2023. doi: 10.1093/icesjms/fsx015
Gilby B. L., Henderson C. J., Olds A. D., Ballantyne J. A., Cooper T. K. A., Schlacher T. A. (2023). Cross-ecosystem effects of coastal urbanisation on vertebrate assemblages and ecological function. Anim. Conserv. 26 (1), 126–136. doi: 10.1111/acv.12807
Herrero M. L., Torres R. C. (2016). Do wildfires promote woody species invasion in a fire-adapted ecosystem? Post-fire resprouting of native and non-native woody plants in Central Argentina. Environ. Manage 57, 308–317. doi: 10.1007/s00267-015-0616-8
Iossa G. (2022). The ecological function of insect egg micropyles. Funct. Ecol. 2022, 36. doi: 10.1111/1365-2435.14023
Jiyu L., Colloff M. J., David F. (2022). The effects of rehabilitation treatments on landscape function within a softwood plantation after fire: implications for catchment management. For. Sci. 2022, 2. doi: 10.1093/forsci/fxab060
Jones E., Chasmer L., Devito K., Rood S, Hopkinson C. (2022). Ecological impacts of shortening fire return intervals on boreal peatlands and transition zones using integrated in situ field sampling and lidar approaches. Ecohydrology 15 (3). doi: 10.1002/eco.2403
Kimuyu D. M., Sensenig R. L., Chira R. M. (2017). Spatial scales influence long-term response of herbivores to prescribed burning in a savanna ecosystem. Int. J. Wildland Fire 26, 287. doi: 10.1071/WF16152
Li W., Jiang R., Zhao Y. (2019). Water ecological environment protection under changing environment: A systematic review and bibliometric analysis. J. Coast. Res. 93, 9. doi: 10.2112/SI93-002.1
Liu R., Liu J., Zhao J. (2017). Ground-active arthropod recovery in response to size of shrub plantations in a desertified grassland ecosystem. Polish J. Ecol. 65, 410–422. doi: 10.3161/15052249PJE2017.65.3.008
Malekmohammadi B., Jahanishakib F. (2017). Vulnerability assessment of wetland landscape ecosystem services using driver-pressure-state-impact-response (DPSIR) model. Ecol. Indic. 82, 293–303. doi: 10.1016/j.ecolind.2017.06.060
Milievi S., Vlahovi M., Kragovi M. (2020). Removal of copper from mining wastewater using natural raw material—Comparative study between the synthetic and natural wastewater samples. Minerals 10, 753.
Othoniel B., Rugani B., Heijungs R. (2015). Assessment of life cycle impacts on ecosystem services: promise, problems, and prospects. Environ. Sci. Technol. 50, 1077–1092. doi: 10.1021/acs.est.5b03706
Palmquist K. A., Peet R. K., Mitchell S. R. (2015). Scale-dependent responses of longleaf pine vegetation to fire frequency and environmental context across two decades. J. Ecol. 103, 998–1008. doi: 10.1111/1365-2745.12412
Pushkar S. (2019). Modeling the substitution of natural materials with industrial byproducts in green roofs using life cycle assessments. J. Cleaner Prod. 227, 652–661. doi: 10.1016/j.jclepro.2019.04.237
Qiting Z., Hao C., Yongyong Z. (2015). Impact factors and health assessment of aquatic ecosystem in Upper and Middle Huai River Basin. J. Hydraulic Eng. 46, 1019–1027.
Richards S., Dawson J., Stutter M. (2018). The potential use of natural vs commercial biosorbent material to remediate stream waters by removing heavy metal contaminants. J. Environ. Manage. 231, 275–281. doi: 10.1016/j.jenvman.2018.10.019
Rosatti G., Zorzi N., Zugliani D. (2018). A Web Service ecosystem for high-quality, cost-effective debris-flow hazard assessment. Environ. Model. Softw. 100, 33–47. doi: 10.1016/j.envsoft.2017.11.017
Smith R. J., Benavides J. C., Jovan S. (2015). A rapid method for landscape assessment of carbon storage and ecosystem function in moss and lichen ground layers. Bryologist 118, 32–45. doi: 10.1639/0007-2745-118.1.032
Tarrason D., Ravera F., Reed M. S. (2016). Land degradation assessment through an ecosystem services lens: Integrating knowledge and methods in pastoral semi-arid systems. J. Arid Environ. 124, 205–213. doi: 10.1016/j.jaridenv.2015.08.002
Wang Z., Yang P., Qi G. (2020). An experimental study of a nearly perfect absorber made from a natural hyperbolic material for harvesting solar energy. J. Appl. Phys. 127, 233102. doi: 10.1063/5.0005700
Woods P. J. (2021). Aligning integrated ecosystem assessment with adaptation planning in support of ecosystem-based management. ICES J. Mar. Sci. 2021, 2.
Xu Z., Li T., Bi J. (2018). Spatiotemporal heterogeneity of antibiotic pollution and ecological risk assessment in Taihu Lake Basin, China. Sci. Total Environ. 643, 12–20. doi: 10.1016/j.scitotenv.2018.06.175
Keywords: ecosystem, ecological function, fire slash, natural material renewal, natural materials
Citation: Cai X and Liu F (2024) Effects of different fire slash artificial promotion regeneration and natural material regeneration on ecological function. Front. Ecol. Evol. 12:1338166. doi: 10.3389/fevo.2024.1338166
Received: 14 November 2023; Accepted: 18 April 2024;
Published: 03 May 2024.
Edited by:
Sandeep Samantaray, National Institute of Technology Srinagar, IndiaReviewed by:
Abinash Sahoo, Odisha University of Technology and Research, IndiaHumaira Hamid, National Institute of Technology, India
Copyright © 2024 Cai and Liu. This is an open-access article distributed under the terms of the Creative Commons Attribution License (CC BY). The use, distribution or reproduction in other forums is permitted, provided the original author(s) and the copyright owner(s) are credited and that the original publication in this journal is cited, in accordance with accepted academic practice. No use, distribution or reproduction is permitted which does not comply with these terms.
*Correspondence: Falin Liu, bGl1ZmFsaW42ODA2ODBAMTYzLmNvbQ==