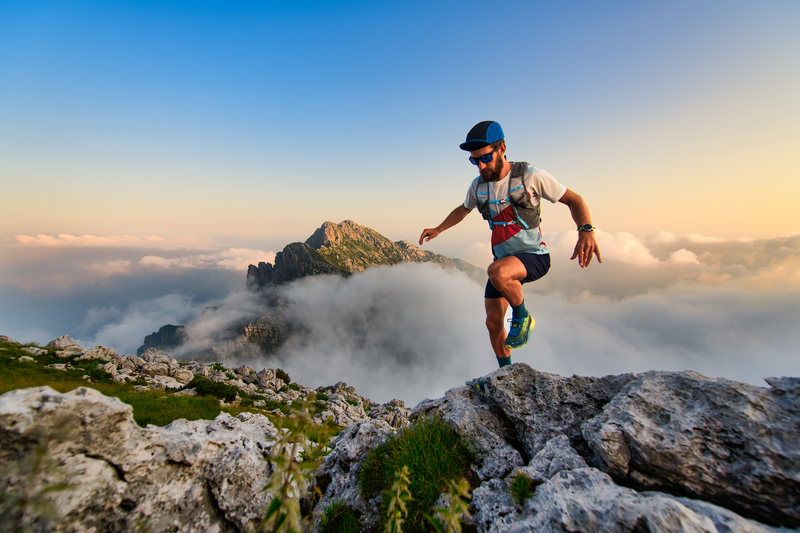
94% of researchers rate our articles as excellent or good
Learn more about the work of our research integrity team to safeguard the quality of each article we publish.
Find out more
REVIEW article
Front. Ecol. Evol. , 23 April 2024
Sec. Models in Ecology and Evolution
Volume 12 - 2024 | https://doi.org/10.3389/fevo.2024.1335452
Evolutionary biology was previously considered a historical science with predictions about evolutionary trajectories believed to be near impossible. The development of high throughput sequencing and data analysis technologies has challenged this belief, and provided an abundance of data that yields novel insights into evolutionary processes. Evolutionary predictions are now increasingly being used to develop fundamental knowledge of evolving systems and/or to demonstrate evolutionary control. Here we investigate the factors that make evolutionary repeatability more or less likely to increase the accuracy of evolutionary predictions. We identify outstanding questions and provide a potential starting point to determine how evolutionary repeatability is affected by genetic relatedness.
In the late 20th century prominent evolutionary biologist Stephen Jay Gould, popularised the theory that the random, stochastic nature of evolution made evolutionary processes unpredictable (Gould, 1989). However, many studies have now provided compelling evidence that challenges this view (Mabee et al., 2007; Losos, 2011; Stern, 2013). In the past two or three decades, through the use of high throughput sequencing and data analysis technologies, many studies have shown the repeatable nature of molecular processes at all stages of evolution (Di Bella et al., 2013; Qiang-long et al., 2014; Reuter et al., 2015). Numerous cases of documented parallel and convergent evolution (Figure 1) at the genotypic and phenotypic level (Conant and Wagner, 2003; Norton et al., 2007; Heyduk et al., 2019) support the existence of constrained evolutionary pathways (Mas et al., 2020).
Figure 1 Convergent and parallel evolution demonstrate evolutionary repeatability. (A) Parallel evolution: the evolution of similar phenotypes or genotypes in multiple independent populations, in response to similar selection pressures, from similar initial conditions. (B) Convergent evolution: the evolution of similar phenotypes or genotypes in multiple independent populations, in response to similar selection pressures, from different initial conditions.
The concept of evolutionary predictability is becoming more widely accepted (Lobkovsky and Koonin, 2012; Linnen, 2018; Marin-Bejar et al., 2021). Potential targets of evolutionary predictions include both the underlying genetic changes that cause adaptation and their resulting phenotypes (Martínez et al., 2007; Duan et al., 2020; Cano et al., 2023). Because of the inherent stochasticity of evolutionary processes, predictions have been shown to be more precise on short timescales (Cano et al., 2023; Wortel et al., 2023).
Evolutionary predictions are increasingly being developed as tools for use in medicine (Lipinski et al., 2016; Hosseini et al., 2019), agriculture (Cooper et al., 2014; Hawkins and Fraaije, 2021), biotechnology (Sandberg et al., 2019) and conservation (Fisher and Owens, 2004; Shefferson et al., 2018). Predictive models based on evolutionary theory can be used to aid vaccine development by predicting which influenza variant will dominate an incoming flu season (Agor and Özaltın, 2018; Morris et al., 2018; Yarmarkovich et al., 2020). Conservation efforts can be assisted in identifying which endangered species are at the greatest risk for extinction (Walters et al., 2012; Gallagher et al., 2015). Medicine would be made more efficacious if we can foresee which pathogens are most likely to evolve drug resistance (Mahfouz et al., 2020; Yang and Wu, 2022). Each of these possible applications requires the ability to accurately predict future outcomes and, in some circumstances, manipulate the path of evolution.
Evolution itself is an intricate process that involves the reciprocal interactions of stochastic and deterministic systems (Blount et al., 2018). Due to the complexity and historical nature of evolution, our ability to test and predict its outcomes in the natural world is limited (Gould, 1989). For this reason a fundamental understanding of evolutionary repeatability, the independent evolution of highly similar or identical genotypes or phenotypes, is of great interest (Venkataram and Kryazhimskiy, 2023). Repeatability has previously been proclaimed as evidence for evolution as a deterministic process, citing convergent and parallel evolution (Figure 1) as support for adaptation by natural selection (Harvey and Pagel, 1991; Losos, 2011). More recently, it has been argued that repeatability is not binary but rather existing on a quantifiable scale, with convergent and parallel evolution representing one extreme end of this continuum (Bolnick et al., 2018). This implies that knowledge of the extent to which evolution is repeatable, along with the factors that influence its repeatability, could ultimately allow us to predict evolution (Palmer and Kishony, 2013; Furusawa et al., 2018).
There are many examples of repeated adaptation from independent populations, species, or lineages. Instances of repeated evolution can be divided into two categories dependent on the relatedness of the starting strains – convergent and parallel evolution (Bolnick et al., 2018). Parallel evolution (Figure 1A) describes the evolution of similar traits in independently, but related evolving species (Bolnick et al., 2018; Simpson, 2019). Convergent evolution occurs when independent species who do not share a recent common ancestor, evolve similar traits (Bolnick et al., 2018). Examples of parallel evolution exist both in vitro and in the natural world (Ostrowski et al., 2008; Lieberman et al., 2011; Wong et al., 2012). Despite the growing interest in parallel evolution in recent decades, our understanding of the factors that influence the likelihood of parallel evolution remain limited (Blount et al., 2018; Bohutínská et al., 2021). Many studies demonstrate parallel evolution in strains with a range of genomic differences, showing no clear link between genetic relatedness and the likelihood of parallel evolution (Ostrowski et al., 2008; Dettman et al., 2013). Often many cases of parallel evolution occur in highly conserved genes, which would indicate that parallelism is linked to the target gene itself and uninfluenced by other genomic factors.
Frequently, the examination of parallel evolution within and among genetic backgrounds differs in the approaches employed (Wood et al., 2005; Vogwill and MacLean, 2015). When studying parallel evolution in similar genetic backgrounds, parallel evolution is commonly investigated using experimental evolution in controlled laboratory settings (Wood et al., 2005). On the other hand, in diverse genetic backgrounds, researchers often rely on phylogenetic and computational methods (Wood et al., 2005). By integrating these two approaches, a comprehensive understanding can be achieved. If genetic background does not affect parallelism we would anticipate that selection pressure would exert the most significant influence on evolutionary outcome, resulting in a similar path for all individuals (Vogwill and MacLean, 2015). A huge area of interest is determining to what extent relatedness is determined by distance from the shared common ancestor and finding where repeatability ends.
Arguably, the most prominent evolutionary framework is that provided by The Modern Synthesis (Huxley, 1942) an integration of the widely accepted principles of natural selection and inheritance patterns proposed by Charles Darwin (Darwin, 1859) and Gregor Mendel (Mendel, 1866) respectively. The Modern Synthesis is grounded in a few fundamental principles: (1) genetic variation is the source of all phenotypic variation, (2) genetic variation occurs randomly within the genome regardless of its fitness effects, (3) mutations that are accumulated and selected for over time are the basis of evolution, and (4) adaptation is as a direct result of natural selection (Futuyma, 2015, Futuyma, 2017; Hancock et al., 2021). The influence of natural selection in evolutionary outcomes is of great significance in modern synthesis (Futuyma, 2015; Liu, 2018). Natural selection favours beneficial mutations (Denamur and Matic, 2006; Gregory, 2009) and, to a certain extent, helps counteract the random nature of genetic variation (Caporale, 2003). Selectionists believe that, irrespective of the location and manner in which mutations arise in the genome, selection acts in a somewhat deterministic way (Rouzine et al., 2001; Lipinski et al., 2016; Blount et al., 2018).
An evolutionary prediction that adheres to the rules of The Modern Synthesis is as follows: if we expose bacteria to antibiotics, individuals harbouring a genetic mutation (either naturally or acquired) that reduces their susceptibility to the relevant antibiotic, will be able to survive in conditions with the antibiotic. Bacteria without this mutation will perish, leaving only resistant individuals to survive and proliferate when grown in the presence of antibiotics. Consequently, the resulting population will ultimately consist only of mutant individuals. This prediction is easily tested in a laboratory setting and allows for a measurable confirmation of predictive accuracy.
In the 1960s Motoo Kimura proposed the controversial neutral theory of evolution (Kimura, 1967). The central principle of his theory was that most genetic variation between and within species was due to the random accumulation of selectively neutral or nearly neutral mutations (Kimura, 1977; Nei et al., 2010). Kimura proposed that neutral mutations are the primary driver of genetic variation, leading to fixation, where a random mutation becomes predominant in the population due to genetic drift, independent of selection pressures (Kimura, 1967, Kimura, 1977). According to the neutral theory, the rate of molecular evolution is primarily determined by the mutation rate and population size, with Darwinian natural selection playing a minimal role (Kimura, 1987; Razeto-Barry et al., 2012; Jensen et al., 2019). Therefore, the mutation rate is equal to the rate of substitution of neutral mutations (Kimura, 1967; Jensen et al., 2019).
Although Kimura suggested that the majority of fixed genetic variation arises from neutral mutations, he explicitly recognises the existence of numerous deleterious mutations that are eliminated through natural selection (Nei et al., 2010). Neutral theory does not argue against the existence of natural selection, but rather recognises two distinct forms of selection: (1) purifying, which eliminates deleterious mutations, and (2) positive selection, which favours beneficial mutations (Takahata, 1996; Hughes, 2008). Neutral theory assumes that most genomes are well adapted for their local environments and therefore advantageous mutations are a rare occurrence, allowing them to be ignored when considering the rate of molecular evolution (Jensen et al., 2019; Kimura, 2020).
In nature, often the true evolutionary landscape is much more complex than described by either selectionists or naturalists (Wortel et al., 2023). Environmental influences can significantly impact selection (Hoffmann and Hercus, 2000; Melbinger and Vergassola, 2015). The maintenance of a large population of genetically diverse individuals is essential for natural selection to be the predominant driving force of evolution (Gravel, 2016; Vahdati et al., 2017; Raynes et al., 2018). Conflicting microevolutionary forces like genetic drift, mutation, recombination and migration (or gene flow) can inhibit the effects of natural selection by diminishing population size, genetic diversity or both (Allendorf et al., 2014; Gravel, 2016; Chen et al., 2018; Raynes et al., 2018). Small populations with low genetic variation are more susceptible to the random fixation of alleles by genetic drift, potentially amounting to maladapted communities (Kuo et al., 2009; Brigham and Schwartz, 2013).
Despite the increased availability of genetic data and our better understanding of the microevolutionary forces that influence evolution, there remains discussion around the use of the Neutral Theory as an explanation of evolution (Kern and Hahn, 2018; Jensen et al., 2019). A 2018 perspective article written by Kern and Hahn (Kern and Hahn, 2018) opposes the validity of the Neutral Theory, arguing that selection plays a stronger role in genetic variation than what it accounts for, specifically emphasizing the importance of selective sweeps and background selection. They argue that genetic drift and the dominance of neutral mutations alone do not adequately account for adaption (Leigh, 2007; Kern and Hahn, 2018). Their perspective was contested by Jensen et al. (2019), asserting that the Neutral Theory offers valuable insights into the observed patterns of genetic variation within populations, serving as a robust framework for understanding molecular evolution. It could be concluded that neither the Neutral Theory nor The Modern Synthesis, in isolation, provides a sufficient explanation of evolution (Barroso and Dutheil, 2023). Natural selection is limited by its environment and therefore is not always guaranteed to prevail (Lenormand, 2002; Endler and Rojas, 2009). However, genetic drift and the dominance of neutral mutations (as described by neutral theory) alone do not adequately account for adaptation (Leigh, 2007; Kern and Hahn, 2018). A comprehensive understanding of evolution requires consideration of the interplay between deterministic and stochastic forces, alongside the specific ecological context in which they operate.
There are two main reasons why we would want to predict evolution: (1) to test hypotheses to develop fundamental knowledge in evolving systems and/or (2) to demonstrate evolutionary control and prevent or encourage certain evolutionary outcomes. Most often general rules about evolutionary processes are discovered through the use of experimental evolution (Figure 2) (Wortel et al., 2023). Such experiments facilitate the identification of new and existing mutations, determine the speed of adaptation, and identify instances and causes of repeatability (Sandberg et al., 2019). Patterns of microbial adaptation have been identified through long term evolution experiments with bacteria (Gerrish and Lenski, 1998; Good et al., 2017). These studies have found that mutator genes are present in a greater frequency in pathogenic bacteria (LeClerc et al., 1996; Oliver et al., 2000). Changes in mutation rate can be indirectly modulated through natural selection (Giraud et al., 2001; Tenaillon et al., 2001; Peck and Lauring, 2018), maladapted individuals show the biggest and fastest increase in fitness (Couce and Tenaillon, 2015) and mutations with large beneficial fitness effects are rare (Imhof and Schlotterer, 2001). Although most evolution experiments are performed in vitro, these findings are often transferable to natural settings (Bons et al., 2020; Kelly, 2022), thus illustrating that a fundamental understanding of evolutionary processes enhances our ability to make evolutionary predictions that have practical applications.
Figure 2 Why do we want to predict evolution? (A) To develop fundamental knowledge, by testing hypotheses using experimental evolution. For example, testing to see if closely related strains evolve similar phenotypes. (B) To demonstrate evolutionary control and prevent or encourage certain evolutionary outcomes. For example, using predictions of which influenza phenotype will be the most dominant rather than basing the current vaccine off the dominant strain from the previous year. Adapted from Wortel et al. (2023), created in Biorender.com (2023). This figure is for illustrative purposes only and does not represent real data.
Accurately predicting evolutionary outcomes could provide the opportunity to prepare for and on occasion, manipulate desired outcomes. For example, the anticipation of which influenza strain will dominate an upcoming flu season necessitates the development of a vaccine specific to that particular strain (Agor and Özaltın, 2018). Through the use of world-wide surveillance and sequencing data, scientists produce an updated influenza vaccine to best control the seasonal influenza outbreak (Morris et al., 2018). In more extreme causes, predictions can be used to prevent evolution – understanding how antimicrobial resistance might occur can help doctors decide which course of treatment to prescribe patients (Vanderkooi et al., 2005; Her and Wu, 2018).
The nature of evolutionary predictions is context dependent. In some circumstances, predicting phenotype alone is sufficient – useful predictions do not always require identification of the underlying causal mechanism (Mas et al., 2020; Wortel et al., 2023). In other cases, such as the prediction of dominant flu variants, predictions at the genotypic level are required (Agor and Özaltın, 2018; Morris et al., 2018).
In a scenario that is conducive to high predictive accuracy, the influence of selection will surpass the stochastic mechanisms of mutation, genetic drift, recombination and ecological change (Wortel et al., 2023). Under these circumstances natural selection would prevail, and it would be feasible to suggest that we could accurately predict the evolution of traits that will increase population fitness (Stern and Orgogozo, 2008; Holmes, 2013; Nosil et al., 2020). Nosil et al. (2018) observed that more precise forecasts about evolutionary change can be made in traits whose frequency independently influenced an organism’s fitness, compared to those with additive fitness effects.
The genotype-fitness landscape, a crucial factor in evolution, represents the relationship between genotype and phenotype, specifically when phenotype, such as growth rate, is used as a proxy for fitness or another comparable proxy (de Visser and Krug, 2014; Hartl, 2014). Sewall Wright introduced the concept of the fitness landscape to illustrate interactions among mutations; it draws parallels between contours of physical topography and contours of organismal fitness (Wright, 1963). On a three-dimensional fitness landscape, the x- and y-axes represent the range of potential mutations, while the z-axis corresponds to the fitness level associated with each genotype (de Visser and Krug, 2014). The “ruggedness” of a fitness landscape is shaped by the prevalence of interactions among genes (Van Cleve and Weissman, 2015; de Visser et al., 2018). On a smooth landscape with a single peak each mutation has a constant effect on fitness, on rugged landscapes with multiple peaks, the magnitude and sign of each mutation is influenced by the presence of other mutations in the individual (Handel and Rozen, 2009; Szendro et al., 2013b).
In the natural environment, predicting evolution is a complex task that requires knowledge of various biological levels from DNA modifications to environmental interactions (Stoltzfus and McCandlish, 2017; Mas et al., 2020; Chevin et al., 2022). A myriad of genetic and environmental factors manipulate evolutionary trajectories (Stoltzfus and McCandlish, 2017; Govaert et al., 2019; Chevin et al., 2022). The inherent stochasticity of genetic mutation makes it difficult to predict the specific location and nature of genetic variation within the genome (Mas et al., 2020). Changes in a population’s environment can modify the strength and direction of selection (Wortel et al., 2023). In order to make accurate predictions about evolutionary outcomes, in-depth knowledge about the basic factors that contribute to evolution would be required for meticulous modelling.
At the molecular level, evolution is reliant on genetic variation – the ultimate source of which is genomic mutation (Arber, 2000; Barton, 2010). Mutation provides the raw material necessary for selection and genetic drift to act upon (Sprouffske et al., 2018). Mutations are typically described as being “random” in nature, with this randomness being a central tenet of evolutionary theory (Lenormand et al., 2009; Futuyma, 2017; Svensson and Berger, 2019; Liu and Zhang, 2022). In the context of mutation the term “random” that the generation of mutations is not altered as a direct response to organismal needs; it does not assume that all mutations are generated equally (Futuyma, 2017; Svensson and Berger, 2019). Their stochasticity is impacted by mutation rates (Drake et al., 1998), mutation biases (Stoltzfus and McCandlish, 2017), and epistasis, or linkage (Miton and Tokuriki, 2016; Starr and Thornton, 2016). Each of these factors will be discussed below.
The genomic mutation rate, determined by genome size and the rate of per-nucleotide site mutations, defines the average number of mutations that occur in a generation (Lynch, 2010; Peck and Lauring, 2018; Wortel et al., 2023). Typically mutation rate is inversely correlated with genome size (Lynch, 2010; Sung et al., 2012). Increased mutation rate enhances adaptive capacity while concurrently facilitating gene loss (Bradwell et al., 2013; Bourguignon et al., 2020). When compared to deletions at low mutation rates, some evidence has suggested that insertions are more beneficial for phenotypic evolution (Gupta et al., 2016).
Evidence of genetic variation in general DNA repair and replication processes (Miller, 1996; Horst et al., 1999; Friedberg et al., 2004) suggest it’s possible for an individual’s mutation rate to be under the influence of natural selection (Peck and Lauring, 2018). Despite the crucial role of mutations in long term adaptation, most mutations with phenotypic effects are deleterious (Zhang and Gerstein, 2003; Jiang et al., 2010). Therefore, mutation rates reflect an equilibrium between the cost of replication fidelity and the benefit of reducing these deleterious mutations (Nachman and Crowell, 2000; Lynch et al., 2016). In asexual species, the mutation rate that optimises the rate of adaptation depends on the strength of purifying selection against any deleterious mutations (Orr, 2000; Sniegowski et al., 2000). In 2010, Michael Lynch proposed a threshold that restricts the advantages associated with low mutation rates (Lynch, 2010). When the mutation rate reaches a level where additional beneficial mutations offer a smaller fitness advantage than the effects of random genetic drift, selection is unable to reduce the mutation rate any further (Lynch, 2010; Sung et al., 2012; Lehtonen, 2020).
Combined with population size, mutation rate determines mutation supply – the total number of mutations that occur per population per generation (Vahdati et al., 2017; van Dijk et al., 2017). When mutation supply is low, a significant amount of time is required for advantageous mutations with substantial fitness impacts to emerge and become established within a population (Ramiro et al., 2020; Wortel et al., 2023). In larger populations, where the mutation supply is higher (Vahdati et al., 2017) the effects of natural selection are stronger and therefore more effective at fixing beneficial mutations while removing deleterious mutations (Ohta, 1992; Lynch et al., 2016; Vahdati et al., 2017). The likelihood of parallel evolution resulting from selection is positively correlated with population size – a greater mutational supply Increases the chances of a beneficial, large-effect mutation occurring (Bailey et al., 2017).
It is now widely acknowledged that there is variation in mutation rates between genomic position and different classes of mutation (Svensson and Berger, 2019; Kustin and Stern, 2021; Cano et al., 2023; Wortel et al., 2023). This is termed mutation bias and is reflected by mutational patterns that occur at a greater rate than what would be expected under uniformity (Yampolsky and Stoltzfus, 2001; Cano et al., 2023). Given that mutation acts as the raw material for evolution, mutation biases may play a significant role in adaptation (Stoltzfus and Yampolsky, 2009; Cano and Payne, 2020).
Numerous studies surveying spontaneous mutation spectra have demonstrated that different DNA sequence changes are not generated equally (Schaaper and Dunn, 1987; Ossowski et al., 2010; Lee et al., 2012). There is a well-documented bias in many species towards transitions (purine to purine or pyrimidine to pyrimidine) in proportion to transversions (purine to pyrimidine and vice versa) (Morton, 1995; Wakeley, 1996; Katju and Bergthorsson, 2019; Payne et al., 2019). Different gene classes vary in their optimal mutation rates (Monroe et al., 2022; Horton and Taylor, 2023) housekeeping genes and other highly expressed genes, have characteristically low mutation rates because new mutations are likely to be deleterious to protein function (Drummond et al., 2005; Martincorena et al., 2012). The distribution of fitness effects (DFE) of mutations reveals the relative frequencies of different mutation types and their impact on fitness (Eyre-Walker and Keightley, 2007; Kousathanas and Keightley, 2013). Small effect mutations occur at a greater frequency than large effect mutations (Imhof and Schlotterer, 2001; Rockman, 2012; Hua and Springer, 2018; McDonough and Connallon, 2023) posing challenges for repeatability and predictability as multiple small effect mutations can collectively equal a single, large-effect mutation (Joyce et al., 2008; Sackman et al., 2017; Wortel et al., 2023). A recent study demonstrated that alterations in the direction of mutation biases can influence the distribution of fitness effects of mutations in E. coli (Sane et al., 2023). Asymmetries in mutation biases and the fitness effects of mutations (as a result of selection) influence evolutionary divergence, contributing to directional trends and parallel evolution (Rokyta et al., 2005; Stoltzfus and Yampolsky, 2009; Storz, 2016; Bailey et al., 2017).
At some genomic sites mutations occur at an elevated frequency compared to surrounding areas
(Rogozin and Pavlov, 2003; Miller et al., 2016). These sites are termed mutational hotspots and can be a result of various factors including repetitive DNA sequences (Bzymek and Lovett, 2001; McVean, 2010), the activity of specific DNA repair mechanisms (Perera et al., 2016; Nesta et al., 2021) or exposure to mutagens (Brash and Haseltine, 1982; Ziegler et al., 1993). The existence of these hotspots can cause position bias by creating concentrated areas of genetic variation, driving evolutionary change more rapidly than mutations occurring elsewhere within the genome (Galen et al., 2015; Baeissa et al., 2017; Shepherd et al., 2022). For example, a single point mutation at a highly mutable sight within the β-globin gene of Andean house wrens confers an evolutionary advantage by increasing haemoglobin’s oxygen affinity (Galen et al., 2015). Given no compelling evidence to suggest that this particular genetic change offered a superior evolutionary benefit compared to others within the same gene, it was inferred that the asymmetry in mutation frequency stemmed from an elevated mutation rate favouring its occurrence (Galen et al., 2015).
In the presence of strong mutation and selection biases, the number of mutations that reach high frequencies or become fixed within a population is limited (Storz, 2016; Schenk et al., 2022). Mutations achieve success either because they are more prevalent within the population (mutation bias), because they provide the greatest fitness advantage (selection bias), or as a combination of both (Gerrish and Lenski, 1998; Lynch et al., 2016; Horton and Taylor, 2023).
The impact that mutation biases may have on adaptation is determined by population genetics (Yampolsky and Stoltzfus, 2001; Cano and Payne, 2020) and other environmental circumstances, such as the strength of selection (Akashi, 1997; Vinogradov, 2004). Neutral theory proposes that in conditions of complete neutrality, genetic drift will be the driving force of evolution (Kimura, 1987). When mutational supply is high, strong selection favours the fixation of mutations that provide the greatest fitness advantage, thereby reducing the impact of mutation bias (Lynch et al., 2016; Vahdati et al., 2017). However, even in the presence of selection, a significant mutation bias reduces the pool of available mutations for selection, thereby enhancing the likelihood of repeatability in evolutionary outcomes (Yampolsky and Stoltzfus, 2001; Stoltzfus and Yampolsky, 2009; Cano et al., 2023; Xue et al., 2023).
Mutation bias as a driver for repeatability was demonstrated in replicating bacteriophage populations, where the least beneficial mutation was fixed in a population just as often as the mutation with the greatest fitness effect, purely due to their high frequency of occurrence (Sackman et al., 2017). However, the authors also noted that their ability to predict repeatability was greater when information about selection, in the form of DFE, was considered (Sackman et al., 2017).
Genome-wide association studies have led to the discovery that the evolution of traits is not always confined to one locus or pathway (Chebib and Guillaume, 2017; Csilléry et al., 2018; Mathieson, 2021). Many adaptation events occur via coordinated genetic changes at multiple genomic and regulatory loci (Pritchard and Di Rienzo, 2010; Pritchard et al., 2010; Stern, 2013). These polygenic traits, when subject to stabilising selection, are characteristically redundant, meaning that multiple genotypes can result in a single phenotype (Barghi et al., 2020; Rellstab et al., 2021; Yeaman, 2022). If redundancy is restricted due to a limited presence of mutations in the population or because only a small number of mutations are capable of influencing the phenotype, we can expect to observe a high level of repeatability (Yeaman, 2022). When redundancy is high, the genetic repeatability of adaptation is low, resulting in evolution becoming less predictable (Yeaman, 2022; Wortel et al., 2023).
Epistasis, non-additive interactions between mutations at different genomic loci (de Visser et al., 2011; Kondrashov and Kondrashov, 2015; Tufts et al., 2015; Miton and Tokuriki, 2016), adds further complexity to our ability to predict evolution (Wortel et al., 2023). Epistatic interactions introduce ruggedness into fitness landscapes (Kaltenbach and Tokuriki, 2014) and modify the phenotypic effects of mutations according to the genetic background in which they occur (Phillips, 2008; de Visser et al., 2011). The cumulative effects of a particular set of genetic mutations that alter protein function may rely on each successive step of the mutation process (Blount et al., 2008; Miton and Tokuriki, 2016). The beneficial effects of some mutations may be dependent on the previous establishment of specific permissive mutations (positive epistasis) (Gong et al., 2013; McKeown et al., 2014) or may be rendered deleterious when in the presence of other restrictive mutations (negative epistasis) (Salverda et al., 2011; Dickinson et al., 2013). Consequently, understanding the fitness effects of a mutation in one genetic background may not be applicable to or relevant for another genetic background (Miton and Tokuriki, 2016; Wortel et al., 2023).
Epistatic interactions can be divided into two main categories: magnitude epistasis and sign epistasis (Weinreich, 2005). Under magnitude epistasis, the strength of fitness effects of a mutation is altered by genetic background while the direction of the fitness effect remains irrevocably deleterious or beneficial (de Visser et al., 2011; Poelwijk et al., 2011; Lalić and Elena, 2013). In contrast, sign epistasis denotes epistatic interactions that reverse the direction of fitness effects of a mutation, changing it from deleterious to beneficial or vice versa (Desai et al., 2007; Lalić and Elena, 2013; Nghe et al., 2018). Sign epistasis can act as a genetic constraint for evolution, reducing the potential pathways for evolutionary adaptation (Weinreich, 2005; Nghe et al., 2018). For example, in Saccharomyces cerevisiae, two individually adaptive mutations in the genes MTH1 and HXT6/HXT7 become detrimental to population fitness when present simultaneously (Kvitek and Sherlock, 2011). The constraint imposed by reciprocal sign epistasis causes these mutations to remain mutually exclusive, even though adaptive mutations in these genes occurred independently in many experimental replicates (Kvitek and Sherlock, 2011). Predictability is increased because the number of potential evolutionary pathways is reduced (de Visser and Krug, 2014). However, the ruggedness that epistatic interactions introduce into populations can have the opposite effect on predictability (Poelwijk et al., 2011; Wortel et al., 2023). When multiple fitness peaks on the genotype-fitness landscape exist, the resulting genotype will be decided by whichever mutations fix first, thus reducing predictability (Poelwijk et al., 2011; Bank et al., 2016; de Visser et al., 2018). However, epistasis reduces the number of mutational pathways available, increasing predictability once a particular genotype has been fixed (Weinreich et al., 2006; Palmer et al., 2015; de Visser et al., 2018).
The adaptive “wrinkly spreader” phenotype displayed by Pseudomonas bacteria highlights the significance of genetic background in predicting evolution (Lind et al., 2019). This phenotype consistently emerges within three specific genetic pathways (McDonald et al., 2009; Lind et al., 2015; Van den Bergh et al., 2018). Elimination of these three pathways revealed the existence of 13 additional mutation pathways that lead to the same phenotype and showed similar fitness effects (Lind et al., 2015; Steenackers et al., 2016). The original three pathways were found to have higher mutation rates compared to the others, creating a mutation bias that imposed a genetic constraint (Lind et al., 2015). Understanding these biases can contribute to the genotype-fitness map, potentially providing a framework for making evolutionary predictions in other Pseudomonas strains (Lind et al., 2019; Wortel et al., 2023).
Most evolution experiments are performed in a laboratory setting, where all experimental conditions can be under stringent control (Adams, 2004; Kawecki et al., 2012; Ratcliff et al., 2012; Barrick and Lenski, 2013). In nature, evolving populations are complex biological systems of interactions between and within organisms, as well as between organisms and their direct environment (Beckerman et al., 2016; Gupta et al., 2017; Mas et al., 2020). In order to make accurate predictions about evolutionary outcomes, it is essential to consider the role that the environment plays in evolution (MacColl, 2011; Skinner, 2015; Baym et al., 2016). Changes in ecological factors can either inhibit or facilitate the evolvability of a population (Grant et al., 2017; Cabrerizo and Marañón, 2021). These changes can affect various factors of evolutionary dynamics including population size, the strength and direction of selection and the amount and distribution of genetic variation (Allendorf et al., 2014; Chen et al., 2018; Raynes et al., 2018).
The genetic characteristics of a population, and therefore its evolvability, are regulated through the interactions between genetic drift, gene flow and natural selection (Eckert et al., 2008). Demography and population dynamics, both internal and external, have the capacity to influence these microevolutionary forces (Tscharntke et al., 2012). The extent to which genetic variation is lost through the stochastic force of genetic drift is determined by fluctuations in population size (Eckert et al., 2008; Lohr et al., 2014). Gene flow is inhibited by populations that are spatially distinct and enhanced when there is direct proximity (Smadja and Butlin, 2011; Sexton et al., 2014).
Every organism is guaranteed to alter its environment in some way, however the exact impact is determined by each organism’s evolutionary past (Beckerman et al., 2016). Ecosystem modifications can then, in turn, directly influence evolutionary change, demonstrating that both processes exist on the same time scale (Hendry and Kinnison, 1999; Hairston et al., 2005; Wortel et al., 2023). The interplay between ecology and evolution is termed eco-evolutionary feedback (Govaert et al., 2019). A simple demonstration of eco-evolutionary feedback loops is the relationship between predator and prey (Govaert et al., 2019; van Velzen, 2020). Predation intensifies the selection pressure on prey, leading to the emergence of defensive traits, which subsequently influences the predator–prey dynamic (Cortez, 2018; Fryxell et al., 2019). Greater complexity in ecological systems, characterised by increased diversity and interactions, tends to promote greater stability (Tilman et al., 2006; Hector et al., 2010; Xu et al., 2021). However, this stability does not always directly translate into more predictable evolutionary outcomes (Yang et al., 2019). If a complex system has a multitude of chaotic dynamics, then it is likely that the final state of this system will be unable to be predicted because it is dependent on starting conditions (Fisher and Pruitt, 2020). Within chaotic systems, slight alterations in initial conditions and parameters can result in significantly magnified and unforeseeable consequences over time (Doebeli and Ispolatov, 2014); these systems rely entirely on their starting conditions and exhibit a high degree of sensitivity to them (Rego-Costa et al., 2017; Fisher and Pruitt, 2020). In a highly organised complex system, it is typically expected that the same final state will be reached regardless of the starting conditions (Fisher and Pruitt, 2020) the system’s dynamics are constrained by the structure and interactions within the system, leading to a consistent outcome (Anand et al., 2010; Mouquet et al., 2015).
Within a single population, eco-evolutionary feedback can be both intrinsic or extrinsic. Intrinsic feedback loops occur between population density and trait values, while extrinsic feedback results from resource limitations (Govaert et al., 2019). This frequency and density dependent selection suggests that fitness is dependent on both genotype proportion and density, not just the individual trait value (Travis et al., 2014; Govaert et al., 2019; Chevin et al., 2022). Changes in population densities shift selection pressure, causing a preference for different phenotypes that offer competitive abilities which are better suited to the new dynamic (Lankau and Strauss, 2011; Govaert et al., 2019). Negative frequency dependent selection (NFDS), the favouring of rare phenotypes within a population, is a powerful form of balancing selection that maintains many natural polymorphisms (Brisson, 2018; Govaert et al., 2019). On its own, strong NFDS can cause stablility in the frequency of genetic variations, or predictable frequency fluctutations and is therefore associated with predictable evolutionary outcomes (Rego-Costa et al., 2017; Chevin et al., 2022; Wortel et al., 2023). In the natural environment the predictability of evolutionary outcomes is dependent on the strength of NFDS and the stochasticisty of environmental changes (Travis et al., 2014; Chevin et al., 2022).
A population that is exposed to stress may adapt to avoid extinction through a process called evolutionary rescue (Gomulkiewicz and Holt, 1995; Bell, 2013). As a general rule, adaptation is more likely to occur when environmental changes (and thus exposures to stressors) are gradual (Bell and Gonzalez, 2011; Bell, 2013). In these circumstances the number of individuals exposed to selection remains high (Gonzalez et al., 2013). Rapid environmental changes may exceed the capacity of a population to persist and maintain their genetic diversity (Doak and Morris, 2010; Bell and Gonzalez, 2011). This was demonstrated by a 2011 study that showed yeast populations gradually exposed to an increase in salt stress had a greater survivability than those exposed abruptly (Bell and Gonzalez, 2011). Coupled with a more recent meta-analysis that demonstrated insufficient adaptation in the face of rapid climate change (Radchuk et al., 2019) it could be reasonable to assume that evolutionary predictability would be more accurate in instances of gradual change, where natural selection has a greater impact. However, in some studies using laboratory-based evolution, the opposite has been observed; populations exposed to gradually changing environments exhibited more diverse mutations (Lindsey et al., 2013; Vogwill et al., 2014). In rapidly changing environments, populations are bottlenecked as they move from one environment to another, leading to a limited set of observations as a consequence of the corresponding genetic drift (Lindsey et al., 2013). In an extreme instance, where ecological change is so rapid that there is no time for any adaptation – this would likely result in a predictable population extinction (Wortel et al., 2023).
A side effect of rapid ecological change is the altering of population size and genetic diversity through a bottleneck effect (Kazahari et al., 2023). As discussed previously, maintaining a large population is important for natural selection to be the primary evolutionary force (Gravel, 2016). Smaller, less diverse populations are more subject to the effects of random genetic drift and therefore are at risk of losing genetic variation through stochastic means (Brigham and Schwartz, 2013; Brown et al., 2013). Random events alter the efficacy of selection, which is the only selective force that acts in a deterministic manner (Witting, 2003; Lipinski et al., 2016). Expanding populations can overcome the effects of a population bottleneck by accumulating new mutations (Kazahari et al., 2023).
The predictability of a population can be influenced by the characteristics of its habitat, including environmental complexity and heterogeneity (Wortel et al., 2023). Greater habitat complexity introduces more selective pressures, which can lead to fitness trade-offs between traits when selection is antagonistic (Ghalambor et al., 2003; Roff and Fairbairn, 2012). Antagonistic selection pressures may allow for a greater number of adaptations which increases competition between individuals, reducing the rate of adaptation (Ghalambor et al., 2003; Merlo et al., 2020). The application of multiple selection pressures may lead to the fixation of unexpected traits within a single population. However, populations that evolved in environments with shared selection pressures were genetically similar to each other and demonstrated parallel evolution (Ord and Summers, 2015; Turner et al., 2018; Pilakouta et al., 2023). The extent of our ability to anticipate evolution is constrained by our understanding of environmental attributes and their historical impact on the evolution of comparable populations.
Microbial cells have rapid generation times and are able to maintain large population sizes, allowing them to readily adapt and evolve to changes in their environment (Bennett and Hughes, 2009) this characteristic makes microorganisms invaluable tools for studying fundamental evolutionary processes (Bennett and Hughes, 2009; McDonald, 2019). In the laboratory, adaptive evolution experiments provide scientists with the tools to study how microbes evolve in a completely controlled setting, with each environmental factor under strict regulation, but with the potential to be altered in a precise and direct manner (Kawecki et al., 2012). The general principles of laboratory evolution experiments date back to Reverend William Henry Dallinger’s work in the late 19th century (Dallinger, 1878) and remain attractive to modern scientists for the control allowed over everything from initial genotype to transfer protocols and selective pressure (Bruger and Marx, 2018; Wang et al., 2021).
A popular approach of adaptive laboratory evolution is prolonged cell culturing in the presence of a selection pressure (Sandberg et al., 2019). Over time the intensity of the selection pressure is slowly increased and individuals with the greatest fitness in their new environment are naturally selected to outcompete their less fit ancestors (Gresham and Dunham, 2014; Sandberg et al., 2019). Because microbial populations divide rapidly, they are able to maintain large populations (of approximately 109 cells per mL of liquid culture) (Sandberg et al., 2019) this type of experimentation provides wide environmental sampling and maintains genetic diversity (providing genotypes have risen to a reasonable frequency; Figure 3), resulting in a lineage of cells that are fitter than their starting ancestral strain (Teotónio et al., 2009; Hindré et al., 2012). Within the framework of experimental evolution, the concept of “fitness” relies on the specific growth environment chosen for the experiment (Liu et al., 2019; Sandberg et al., 2019). Depending on the preferred conditions, fitness parameters could range from growth rate to end point optical density (Kawecki et al., 2012; Liu et al., 2019).
Figure 3 Important parameters of experimental evolution. (A) Batch cultivation requires the dilution of culture into fresh media. These experiments are easy to establish and allow for up to 1000s of replicates. (B) Continuous cultivation includes mechanisms for the constant supply of nutrients. This procedure is costly and doesn’t allow for a high number of replicates. Adapted from McDonald (2019), created using BioRender.com.
An additional benefit is the construction of a “living fossil record” by freezing replicate populations at each experimental step, allowing for direct comparisons to be made between different strains, time points and experiments (Lenski et al., 1991; Hindré et al., 2012; Batut et al., 2013; Sandberg et al., 2019). When a strongly advantageous mutation occurs, it often overtakes the population in a selective sweep (Kawecki et al., 2012; Sandberg et al., 2019; Stephan, 2019). Occasionally, genetically diverse populations can experience clonal interference, where competition between beneficial mutations results in the extinction of the less successful mutations (Fogle et al., 2008; Maddamsetti et al., 2015). Although endpoint strains generally display higher fitness compared to midpoint strains (Spencer et al., 2008) studying each time point from the frozen “fossil record” offers a valuable opportunity to understand the adaptive steps taken and identify the causal mutations involved (Lang and Desai, 2014; Sandberg et al., 2016, Sandberg et al., 2019). To aid in the identification of causal mutations, replicate strains are often evolved simultaneously – mutations that are present in multiple strains are more likely to be adaptive and not as a result of chance (Bailey et al., 2015; Maddamsetti et al., 2015).
Adaptive laboratory evolution provides the potential to identify genome-wide mutations and is not limited to just targeted sites (Packer and Liu, 2015; Arnold, 2018). This is particularly beneficial when there is inadequate information about the mechanisms responsible for a specific mutation and removes the risk of having too limited a scope of investigation (Kawecki et al., 2012). Coupled with the advancement of high throughput sequencing technologies, that have made whole genome sequencing cost effective and readily available, and the generation of a living fossil record by freezing subsequent populations or isolates at each step, adaptive evolution experiments provide immense amounts of genetic data (Gresham and Dunham, 2014; Shendure et al., 2017).
Selecting the correct evolutionary environment in an adaptive evolution experiment is critical because it determines the selection pressure (Van den Bergh et al., 2018). Laboratory evolution experiments tend to be performed using one of two popular methods: serial dilution (batch cultivation) and continuous culturing (Figure 3) (van Dijk et al., 2019). Batch cultivation (Figure 3A) can be performed in standard laboratory equipment (Lenski, 2017; McDonald, 2019). Several environmental factors can be easily controlled and the use of equipment such as deep multi-well plates allows for hundreds of microbial cultures to be grown in parallel (Hindré et al., 2012). However, the simplicity of batch culturing, although in some ways beneficial, can lead to irregulation in population density, growth rate, nutrient supply and variation in environmental conditions such as pH and oxygen availability (Van den Bergh et al., 2018).
Continuous cultivation (Figure 3B), such as during cultivation in a chemostat, allows more stringent control over environmental conditions such as population size and growth rates, avoiding bottlenecks; it is also possible to link nutrient supply to specific environmental conditions (Gresham and Dunham, 2014; Jeong et al., 2016). Chemostat bioreactor machines are highly specialised, rendering them very labour intensive and costly to run (McDonald, 2019). When choosing between each experimental method, the most important consideration is whether growth in nutrient sufficient or potentially nutrient limited conditions is desired (Sandberg et al., 2019).
Evolution has classically been viewed as a process too slow to be studied directly (Van den Bergh et al., 2018). Historical studies of evolution were typically performed by indirect comparisons of living species and/or fossils (Knoll, 1992; Van den Bergh et al., 2018). It is now evident that certain species, in particular microorganisms, can undergo evolutionary changes at significantly shorter time scales (Lenski et al., 1991). Observations of these adaptive changes in real time has provided novel insights into evolutionary responses that were previously unattainable (Bennett and Hughes, 2009; McDonald, 2019). Early evolution experiments focused on more complex eukaryotic organisms (Van den Bergh et al., 2018). A few key characteristics of microorganisms render them highly practical for use in evolution experiments.
Arguably of the greatest importance are their fast reproduction rates, large population sizes and minimal growth requirements (Kawecki et al., 2012; Van den Bergh et al., 2018). Successful evolution experiments require high levels of control and the ability to perform experimentation with replicate populations (Bennett and Hughes, 2009). Large, highly replicated populations of microorganisms can be easily cultured in parallel to reach many generations on short time scales (Dragosits and Mattanovich, 2013; Sandberg et al., 2019). Maintaining large population sizes reduces the chance of random mutation fixation as a result of bottlenecks and provides a greater chance of obtaining a beneficial mutation (Li and Roossinck, 2004; Wein and Dagan, 2019). The more rapid reproduction is, the faster beneficial mutations are spread and harmful mutations are eliminated (Lynch et al., 2016; Vahdati et al., 2017). Fast reproduction rates allow many generations to be achieved on a short time scale (Bennett and Hughes, 2009; Sandberg et al., 2019). Perhaps the most well-known example of experimental evolution in E. coli is Richard Lenski’s long term evolution experiment spanning 35 years and nearing 75,000 generations (Lenski et al., 1991; Lenski and Travisano, 1994; Good et al., 2017; Lenski, 2023). An equivalent experiment in mice, which began just 5 years later, has only reached 80 generations (Swallow et al., 1998).
An additional important attribute of microorganisms is their relatively small genomes. Many species of microbes have already been completely sequenced, with their genetic information readily available (Bennett and Hughes, 2009). In order to determine whether or not an observed mutation is as a result of real time adaptation, comparison to the ancestral strain is essential. The advancements of high throughput sequencing and data analysis technologies has significantly improved the accessibility and cost-effectiveness of sequencing entire genomes (Metzker, 2010; Qin, 2019). This provides the potential to understand adaptation at the molecular level. By preserving samples from various time points throughout the experiment, it becomes possible to compare genomes at each stage of adaptation (Van den Bergh et al., 2018). This repository also acts as a contingency for unseen events and allows for the resumption of evolution from a specific standpoint (Batut et al., 2013; Sandberg et al., 2019).
Experimental evolution with microbes can be used as a low complexity model system to test evolutionary theories (Hoang et al., 2016). Microbial evolution can provide insights into the processes of natural selection (Teotónio et al., 2009) genetic drift (Ebert, 1998; Heffernan and Wahl, 2002) bet hedging strategies (Beaumont et al., 2009; Graham et al., 2014) and the emergence of specialist phenotypes (Kassen, 2009). These systems have generated biological results that have been used to understand the genetic basis of adaptation (Long et al., 2015) optimise microbial traits for biotechnology (Chen and Arnold, 1993; Sandberg et al., 2019) and test the phenotypic dynamics of microbes (Lenski, 2017; Remigi et al., 2019). Results obtained from microbial evolution experiments can be applicable for medically relevant phenomena, including cancer progression, which shares a similar clonal pattern observed in bacteria (Nowell, 1976; Sprouffske et al., 2012; Van den Bergh et al., 2018). Microorganisms themselves also have an implication in human health: bacterial strains have been associated with cancer (Vogelmann and Amieva, 2007; Zella and Gallo, 2021) the human microbiome can influence health and disease (Pflughoeft and Versalovic, 2012; Shreiner et al., 2015) and antimicrobial resistance is becoming of increasing concern (Dadgostar, 2019; Morrison and Zembower, 2020).
Despite all of the successful applications of laboratory evolution experiments (Lenski et al., 1991; Beaumont et al., 2009; Ratcliff et al., 2012; Vogwill et al., 2014), the method does not come without limitations. Evolution experiments are often conducted with population sizes that may not accurately represent the genetic diversity and population sizes that are found in nature (Kawecki et al., 2012; LaBar and Adami, 2016; McDonough and Connallon, 2023). Having a lower initial starting diversity and mutational supply could reduce the possible evolutionary trajectories for adaptation, comparative to what might be possible in nature (Handel and Rozen, 2009; Jiang et al., 2010; Gossmann et al., 2012). Smaller populations are also at a greater risk of being affected by genetic drift, allowing mutations to reach fixation due to random chance, and therefore masking the effects of natural selection (Tremblay and Ackerman, 2001; Brigham and Schwartz, 2013; Brown et al., 2013).
The short time scales inherent to experimental evolution present a significant limitation in accurately portraying the gradual pace of evolutionary change observed in natural populations (Bailey and Bataillon, 2016). Evolutionary change often unfolds over extended periods and numerous generations involving complex interactions within and between organisms that shape evolutionary trajectories (Åkesson et al., 2021). Evolution experiments are often conducted within very controlled laboratory settings where generation times might be artificially accelerated (Bromig and Weuster-Botz, 2023) and interactions between ecological variables are removed (Gresham and Dunham, 2014; Fisher and Lang, 2016). As a result, slower evolutionary dynamics are at risk of being overlooked, and more intricate selection dynamics could unintentionally be omitted (Lindsey et al., 2013; Bailey and Bataillon, 2016). As a consequence, extrapolating findings from short-term studies to long-term real-world scenarios requires consideration and caution.
Parallel (and convergent) evolution has frequently been attributed to selection (Diaz Caballero et al., 2015; Marvig et al., 2015; Bailey et al., 2018). However, many studies failed to consider the effect of mutation biases and heterogeneous mutation rates, incorrectly attributing repeated evolution to a selection pressure (Zou and Zhang, 2015). High levels of parallel evolution could occur when more mutable regions contribute a greater proportion of the available genetic variants that will eventually be selected upon for adaptation (Press et al., 2019). Therefore, our ability to predict evolution lies in the answer to the question of which plays a more important role in parallel evolution: mutation, selection, or both (Orr, 2005)?
If selection were the major driving force of parallel evolution, it would have to be able to reduce the number of evolutionary possibilities down to just one single, viable option (Lässig et al., 2017). The extent to which this is possible varies with biological level: the most repeatable phenotype is fitness itself (provided there is a strong enough selection pressure and sufficient genetic diversity of this selection to act on) (Tenaillon et al., 2012; Orgogozo, 2015; Venkataram and Kryazhimskiy, 2023). Laboratory populations that are evolved in parallel often each exhibit different single nucleotide polymorphisms and amino acid changes, demonstrating that they follow divergent genetic pathways (Colosimo et al., 2005; Smith and Rausher, 2011; James et al., 2021). This is unsurprising, even at the gene level there are many mutational changes that can confer similar functional effects (Steenackers et al., 2016; Scribner et al., 2020). However parallel-evolving populations do demonstrate some genetic convergence, frequently evolving mutations across the same genes or operons, at the gene rather than the nucleotide level (Jatsenko et al., 2010; Vogwill et al., 2014; Blair et al., 2015). The differences in genetic and phenotypic repeatability demonstrates that the level of parallelism is dependent on biological level (Bailey et al., 2015, Bailey et al., 2017; Gutierrez et al., 2019). Genetically, there are many possible evolutionary pathways that can lead to the same phenotype (Jatsenko et al., 2010; Vogwill et al., 2014). In contrast, because of directional selection, phenotype may be directed towards a single phenotypic path (Rieseberg et al., 2002; Hayden et al., 2014; Lässig et al., 2017).
Making the most accurate predictions about evolutionary outcomes requires meticulous modelling based on genetic and environmental data. Modern sequencing provides copious amounts of genomic information that can be used to train computational models. With whole population genome sequencing we gain the ability to track the genetic history of entire populations and identify variants present at low frequencies. In combination with high throughput assays of fitness and phenotype, this computational data can be used to link genetic and phenotypic data to variations in fitness with various forms of modelling.
Agent-based models, or individual-based models, simulate individual agents (organisms) interacting with each other and their environment (Stillman and Goss-Custard, 2010; Moustakas and Evans, 2013). ABM can be used to study how various factors, including selection pressure, genetic variation and environmental conditions influence the emergence of parallel evolution (Moustakas and Evans, 2013; Urban et al., 2016). By simulating the behaviour of these individuals over time, ABM can provide insights into the dynamics of evolutionary processes and make predictions about the future of evolutionary trajectories (Crooks and Heppenstall, 2012; Nemiche et al., 2013; DeAngelis and Diaz, 2019). For example, agent-based simulation modelling of colorectal cancer has demonstrated that intratumor heterogeneity is due to a high mutation rate and the presence of a stem cell hierarchy (Niida and Iwasaki, 2022). Varying parameters to simulate specific conditions provides a framework to test hypotheses and make informative predictions about evolutionary outcomes.
Population genetic models measure changes in allele frequency over time within an entire population (MacLean et al., 2010). Through the consideration of factors such as mutation rates, genetic drift, gene flow and natural selection, these models can simulate the dynamics of allele frequencies and genotype distributions (Yuan et al., 2012) and therefore be used to predict how populations might evolve in certain scenarios. Commonly used models include the Hardy–Weinberg Equilibrium, used to measure the consistency of allele populations from generation to generation in the absence of evolutionary forces (Salanti et al., 2005; Rohlfs and Weir, 2008); the Wright–Fisher Model, used to measure frequency changes in discrete, non-overlapping populations due to genetic drift and random processes (Tataru et al., 2016; Hofrichter et al., 2017; González Casanova et al., 2020); and mutation models, which focus on understanding the rates, patterns and effects of mutations on allele frequencies and phenotypic traits (Zeng and Cockerham, 1993; Estoup et al., 2002; Sainudiin et al., 2004). These models can be applied to various evolutionary questions and have previously been had applications from plant and animal breeding (Karlin and O’Donald, 1978; Cooper et al., 2014; Hill, 2014) to conservation genetics (Ouborg, 2010; Hohenlohe et al., 2021; Willi et al., 2022), however many of these models include simplifications and assumptions that natural populations may not fit perfectly.
Phylogenetic comparisons analyse historical relationships between organisms and use them to forecast evolutionary trends (Cornwell and Nakagawa, 2017). Through the examination of genetic sequences, morphological traits or behaviour patterns, phylogenetic trees are constructed to illustrate branching points and divergence times in evolutionary history. These trees, constructed using statistical methods such as maximum likelihood (Steel and Rodrigo, 2008; Amiroch et al., 2018) or Bayesian interference (Makarenkov et al., 2006; Boussau et al., 2013), enable the estimation of ancestral states and the anticipation of future evolutionary trajectories. Phylogenetic analysis has been successfully used to predict growth rates of soil bacteria (Walkup et al., 2023), plant bioprospecting (Saslis-Lagoudakis et al., 2012) and identifying evolutionary drivers of mammalian brain development (Barton and Harvey, 2000).
Coupling in silico modelling with in vitro laboratory work will provide the best approach for forming evolutionary predictions. By integrating computational models with laboratory evolution experiments, researchers can iteratively refine and validate their models, leading to a deeper understanding of evolutionary processes. Computational models can be used to generate predictions about how organisms might adapt in a model environment, which can then be tested experimentally in the laboratory. Conversely, data from laboratory evolution experiments can be used to train and validate computational models improving their accuracy.
It is clear that evolution can be repeatable. Documented cases of parallel and convergent evolution demonstrate repeatability in individuals that are both highly and moderately genetically related (Di Bella et al., 2013; Qiang-long et al., 2014; Reuter et al., 2015). A comprehensive understanding of repeated evolution in the form of convergence and parallelism is likely to increase our ability to accurately predict evolution. Of great importance is the determination that repeatability is a non-binary process that is more accurately placed on a spectrum, with convergence and parallelism reflecting one extreme end (Bolnick et al., 2018). However, knowledge in what determines the placement of repeatability along this spectrum is severely lacking. Are stochastic forces the main driver for evolutionary variation? Does the strength of selection predominantly determine repeatability? In order to accurately make predictions about the trajectories and outcomes of evolution, we need to be able to determine where this repeatability breaks down. At what level of genetic divergence does repeatability end? We also need to clearly define at what level of repeatability we can make such predictions – is it at the broad level of fitness or as specific as a particular nucleotide change?
Currently, literature on the extent to which repeatability is dependent on genetic background is limited. If we can quantify how distance from the shared common ancestor affects similarities in evolutionary trajectories, information about similar or distantly related organisms can be used to determine how a particular individual will react to an evolutionary pressure. For example – predicting the evolution of antibiotic resistance in bacteria. We could use comparisons of whole genome sequencing data to anticipate the mechanisms with which a particular strain might evolve resistance based on how other, related, strains have evolved. This knowledge could prevent resistance evolving in the first place by influencing the design of drug plans to treat infections successfully and completely.
Studies of evolution that involve genetically diverse strains are typically performed using phylogenetic and computational analysis of natural populations (Grant and Grant, 2002; Wood et al., 2005; Langerhans, 2008, Langerhans, 2010; Mertens et al., 2021). Under these conditions, there is no control over environmental changes, selection pressure or recombination. Access to genetic data at all evolutionary steps is also unlikely, reducing the depth and volume of data generated.
Because predictability is difficult to study in the wild, the most promising approach for study is experimental evolution (Szendro et al., 2013a; D’Souza and Kost, 2016). The control and access to a “frozen fossil record” (Lenski et al., 1991) provides in-depth insight into evolutionary processes at all levels of evolution – detail that would not be possible with historical, comparative studies in nature. Typically these experiments are performed on a small scale that involves only a few replicate populations and often using the same ancestral starting strain (D’Souza and Kost, 2016; Scribner et al., 2020) limiting our understanding of how evolution is related to genetic background at the molecular level. Performing large scale evolution experiments with a diverse range of microbial strains will provide a starting point to begin to determine at what level we can predict evolution. Can we predict evolution at the community level? The phenotypic level? The genotypic level?
SP: Writing – original draft, Writing – review & editing. NF: Funding acquisition, Supervision, Conceptualization, Writing – review & editing.
The author(s) declare financial support was received for the research, authorship, and/or publication of this article. This work was supported by the Marsden Fund (20-MAU-054).
We thank Dr. Olin Silander (Massey University) for his work as an associate investigator on our Marsden Fund Grant.
The authors declare that the research was conducted in the absence of any commercial or financial relationships that could be construed as a potential conflict of interest.
All claims expressed in this article are solely those of the authors and do not necessarily represent those of their affiliated organizations, or those of the publisher, the editors and the reviewers. Any product that may be evaluated in this article, or claim that may be made by its manufacturer, is not guaranteed or endorsed by the publisher.
Adams J. (2004). Microbial evolution in laboratory environments. Res. Microbiol. 155, 311–318. doi: 10.1016/j.resmic.2004.01.013
Agor J. K., Özaltın O. Y. (2018). Models for predicting the evolution of influenza to inform vaccine strain selection. Hum. Vaccines immunotherapeutics 14, 678–683. doi: 10.1080/21645515.2017.1423152
Akashi H. (1997). Codon bias evolution in Drosophila. Population genetics of mutation-selection drift. Gene 205, 269–278. doi: 10.1016/S0378-1119(97)00400-9
Åkesson A., Curtsdotter A., Eklöf A., Ebenman B., Norberg J., Barabás G. (2021). The importance of species interactions in eco-evolutionary community dynamics under climate change. Nat. Commun. 12, 4759. doi: 10.1038/s41467-021-24977-x
Allendorf F. W., Berry O., Ryman N. (2014). So long to genetic diversity, and thanks for all the fish. Mol. Ecol. 23, 23–25. doi: 10.1111/mec.12574
Amiroch S., Pradana M. S., Irawan M. I., Mukhlash I. (2018). “Maximum likelihood method on the construction of phylogenetic tree for identification the spreading of SARS epidemic,” in 2018 International Symposium on Advanced Intelligent Informatics (SAIN), August 2018, IEEE, 137–141. doi: 10.1109/sain.2018.8673334
Anand M., Gonzalez A., Guichard F., Kolasa J., Parrott L. (2010). Ecological systems as complex systems: Challenges for an emerging science. Diversity 2, 395–410. doi: 10.3390/d2030395
Arber W. (2000). Genetic variation: molecular mechanisms and impact on microbial evolution. FEMS Microbiol. Rev. 24, 1–7. doi: 10.1111/j.1574-6976.2000.tb00529.x
Arnold F. H. (2018). Directed evolution: Bringing new chemistry to life. Angewandte Chemie 57, 4143–4148. doi: 10.1002/anie.201708408
Baeissa H., Benstead-Hume G., Richardson C. J., Pearl F. M. G. (2017). Identification and analysis of mutational hotspots in oncogenes and tumour suppressors. Oncotarget 8, 21290–21304. doi: 10.18632/oncotarget.v8i13
Bailey S. F., Bataillon T. (2016). Can the experimental evolution programme help us elucidate the genetic basis of adaptation in nature? Mol. Ecol. 25, 203–218. doi: 10.1111/mec.13378
Bailey S. F., Blanquart F., Bataillon T., Kassen R. (2017). What drives parallel evolution?: How population size and mutational variation contribute to repeated evolution. BioEssays: News Rev. molecular Cell. Dev. Biol. 39, 1–9. doi: 10.1002/bies.201700126
Bailey S. F., Guo Q., Bataillon T. (2018). Identifying drivers of parallel evolution: A regression model approach. Genome Biol. Evol. 10, 2801–2812. doi: 10.1093/gbe/evy210
Bailey S. F., Rodrigue N., Kassen R. (2015). The effect of selection environment on the probability of parallel evolution. Mol. Biol. Evol. 32, 1436–1448. doi: 10.1093/molbev/msv033
Bank C., Matuszewski S., Hietpas R. T., Jensen J. D. (2016). On the (un)predictability of a large intragenic fitness landscape. Proc. Natl. Acad. Sci. U. S. A. 113, 14085–14090. doi: 10.1073/pnas.1612677113
Barghi N., Hermisson J., Schlötterer C. (2020). Polygenic adaptation: a unifying framework to understand positive selection. Nat. Rev. Genet. 21, 769–781. doi: 10.1038/s41576-020-0250-z
Barrick J. E., Lenski R. E. (2013). Genome dynamics during experimental evolution. Nat. Rev. Genet. 14, 827–839. doi: 10.1038/nrg3564
Barroso G. V., Dutheil J. Y. (2023). The landscape of nucleotide diversity in Drosophila melanogaster is shaped by mutation rate variation. Peer Community J. 3. doi: 10.24072/pcjournal.267
Barton N. H. (2010). Mutation and the evolution of recombination. Philos. Trans. R. Soc. London. Ser. B Biol. Sci. 365, 1281–1294. doi: 10.1098/rstb.2009.0320
Barton R. A., Harvey P. H. (2000). Mosaic evolution of brain structure in mammals. Nature 405, 1055–1058. doi: 10.1038/35016580
Batut B., Parsons D. P., Fischer S., Beslon G., Knibbe C. (2013). In silico experimental evolution: a tool to test evolutionary scenarios. BMC Bioinf. 14 Suppl 15, S11. doi: 10.1186/1471-2105-14-S15-S11
Baym M., Lieberman T. D., Kelsic E. D., Chait R., Gross R., Yelin I., et al. (2016). Spatiotemporal microbial evolution on antibiotic landscapes. Science 353, 1147–1151. doi: 10.1126/science.aag0822
Beaumont H. J. E., Gallie J., Kost C., Ferguson G. C., Rainey P. B. (2009). Experimental evolution of bet hedging. Nature 462, 90–93. doi: 10.1038/nature08504
Beckerman A. P., Childs D. Z., Bergland A. O. (2016). Eco-evolutionary biology: Feeding and feedback loops. Curr. biology: CB 26, R161–R164. doi: 10.1016/j.cub.2016.01.013
Bell G. (2013). Evolutionary rescue and the limits of adaptation. Philos. Trans. R. Soc. London. Ser. B Biol. Sci. 368, 20120080. doi: 10.1098/rstb.2012.0080
Bell G., Gonzalez A. (2011). Adaptation and evolutionary rescue in metapopulations experiencing environmental deterioration. Science 332, 1327–1330. doi: 10.1126/science.1203105
Bennett A. F., Hughes B. S. (2009). Microbial experimental evolution. Am. J. Physiol. Regulatory Integr. Comp. Physiol. 297, R17–R25. doi: 10.1152/ajpregu.90562.2008
Blair J. M. A., Webber M. A., Baylay A. J., Ogbolu D. O., Piddock L. J. V. (2015). Molecular mechanisms of antibiotic resistance. Nat. Rev. Microbiol. 13, 42–51. doi: 10.1038/nrmicro3380
Blount Z. D., Borland C. Z., Lenski R. E. (2008). Historical contingency and the evolution of a key innovation in an experimental population of Escherichia coli. Proc. Natl. Acad. Sci. U. S. A. 105 (23), 7899–7906. doi: 10.1073/pnas.0803151105
Blount Z. D., Lenski R. E., Losos J. B. (2018). Contingency and determinism in evolution: Replaying life’s tape. Science 362. doi: 10.1126/science.aam5979
Bohutínská M., Vlček J., Yair S., Laenen B., Konečná V., Fracassetti M., et al. (2021). Genomic basis of parallel adaptation varies with divergence in Arabidopsis and its relatives. Proc. Natl. Acad. Sci. U. S. A. 118. doi: 10.1073/pnas.2022713118
Bolnick D. I., Barrett R. D. H., Oke K. B., Rennison D. J., Stuart Y. E. (2018). (Non)Parallel evolution. Annu. Rev. ecology evolution systematics 49, 303–330. doi: 10.1146/annurev-ecolsys-110617-062240
Bons E., Leemann C., Metzner K. J., Regoes R. R. (2020). Long-term experimental evolution of HIV-1 reveals effects of environment and mutational history. PLoS Biol. 18, e3001010. doi: 10.1371/journal.pbio.3001010
Bourguignon T., Kinjo Y., Villa-Martín P., Coleman N. V., Tang Q., Arab D. A., et al. (2020). Increased mutation rate is linked to genome reduction in prokaryotes. Curr. biology: CB 30, 3848–3855.e4. doi: 10.1016/j.cub.2020.07.034
Boussau B., Szöllősi G. J., Duret L., Gouy M., Tannier E., Daubin V. (2013). Genome-scale coestimation of species and gene trees. Genome Res. 23, 323–330. doi: 10.1101/gr.141978.112
Bradwell K., Combe M., Domingo-Calap P., Sanjuán R. (2013). Correlation between mutation rate and genome size in riboviruses: mutation rate of bacteriophage Qβ. Genetics 195, 243–251. doi: 10.1534/genetics.113.154963
Brash D. E., Haseltine W. A. (1982). UV-induced mutation hotspots occur at DNA damage hotspots. Nature 298, 189–192. doi: 10.1038/298189a0
Brigham C. A., Schwartz M. W. (2013). Population viability in Plants: Conservation, Management, and Modeling of Rare Plants. (Springer Science & Business Media).
Brisson D. (2018). Negative frequency-dependent selection is frequently confounding. Front. Ecol. Evol. 6. doi: 10.3389/fevo.2018.00010
Bromig L., Weuster-Botz D. (2023). Accelerated adaptive laboratory evolution by automated repeated batch processes in parallelized bioreactors. Microorganisms 11, 275. doi: 10.3390/microorganisms11020275
Brown S. M., Harrisson K. A., Clarke R. H., Bennett A. F., Sunnucks P. (2013). Limited population structure, genetic drift and bottlenecks characterise an endangered bird species in a dynamic, fire-prone ecosystem. PLoS One 8, e59732. doi: 10.1371/journal.pone.0059732
Bruger E. L., Marx C. J. (2018). A decade of genome sequencing has revolutionized studies of experimental evolution. Curr. Opin. Microbiol. 45, 149–155. doi: 10.1016/j.mib.2018.03.002
Bzymek M., Lovett S. T. (2001). Instability of repetitive DNA sequences: the role of replication in multiple mechanisms. Proc. Natl. Acad. Sci. U. S. A. 98, 8319–8325. doi: 10.1073/pnas.111008398
Cabrerizo M. J., Marañón E. (2021). Temperature fluctuations in a warmer environment: impacts on microbial plankton. Faculty Rev. 10, 9. doi: 10.12703/r
Cano A. V., Gitschlag B. L., Rozhoňová H., Stoltzfus A., McCandlish D. M., Payne J. L. (2023). Mutation bias and the predictability of evolution. Philos. Trans. R. Soc. London. Ser. B Biol. Sci. 378, 20220055. doi: 10.1098/rstb.2022.0055
Cano A. V., Payne J. L. (2020). Mutation bias interacts with composition bias to influence adaptive evolution. PLoS Comput. Biol. 16, e1008296. doi: 10.1371/journal.pcbi.1008296
Caporale L. H. (2003). Natural selection and the emergence of a mutation phenotype: an update of the evolutionary synthesis considering mechanisms that affect genome variation. Annu. Rev. Microbiol. 57, 467–485. doi: 10.1146/annurev.micro.57.030502.090855
Chebib J., Guillaume F. (2017). What affects the predictability of evolutionary constraints using a G-matrix? The relative effects of modular pleiotropy and mutational correlation. Evolution; Int. J. organic Evol. 71, 2298–2312. doi: 10.1111/evo.13320
Chen K., Arnold F. H. (1993). Tuning the activity of an enzyme for unusual environments: sequential random mutagenesis of subtilisin E for catalysis in dimethylformamide. Proc. Natl. Acad. Sci. U. S. A. 90, 5618–5622. doi: 10.1073/pnas.90.12.5618
Chen Y., Shenkar N., Ni P., Lin Y., Li S., Zhan A. (2018). Rapid microevolution during recent range expansion to harsh environments. BMC evolutionary Biol. 18, 187. doi: 10.1186/s12862-018-1311-1
Chevin L.-M., Gompert Z., Nosil P. (2022). Frequency dependence and the predictability of evolution in a changing environment. Evol. Lett. 6, 21–33. doi: 10.1002/evl3.266
Colosimo P. F., Hosemann K. E., Balabhadra S., Villarreal G. Jr., Dickson M., Grimwood J., et al. (2005). Widespread parallel evolution in sticklebacks by repeated fixation of Ectodysplasin alleles. Sci. (New York N.Y.) 307, 1928–1933. doi: 10.1126/science.1107239
Conant G. C., Wagner A. (2003). Convergent evolution of gene circuits. Nat. Genet. 34, 264–266. doi: 10.1038/ng1181
Cooper M., Messina C. D., Podlich D., Radu Totir L., Baumgarten A., Hausmann N. J., et al. (2014). Predicting the future of plant breeding: complementing empirical evaluation with genetic prediction. Crop Pasture Sci. 65, 311–336. doi: 10.1071/CP14007
Cornwell W., Nakagawa S. (2017). Phylogenetic comparative methods. Curr. biology: CB 27, R333–R336. doi: 10.1016/j.cub.2017.03.049
Cortez M. H. (2018). Genetic variation determines which feedbacks drive and alter predator-prey eco-evolutionary cycles. Ecol. Monogr. 88, 353–371. doi: 10.1002/ecm.1304
Couce A., Tenaillon O. A. (2015). The rule of declining adaptability in microbial evolution experiments. Front. Genet. 6, 99. doi: 10.3389/fgene.2015.00099
Crooks A. T., Heppenstall A. J. (2012). “Introduction to agent-based modelling,” in Agent-Based Models of Geographical Systems. (Springer Netherlands, Dordrecht), 85–105.
Csilléry K., Rodríguez-Verdugo A., Rellstab C., Guillaume F. (2018). Detecting the genomic signal of polygenic adaptation and the role of epistasis in evolution. Mol. Ecol. 27, 606–612. doi: 10.1111/mec.14499
Dadgostar P. (2019). Antimicrobial resistance: Implications and costs. Infection Drug resistance 12, 3903–3910. doi: 10.2147/IDR
Dallinger W. H. (1878). I. On the life-history of a minute septic organisms with an account of experiments made to determine its thermal death point. Proc. R. Soc. London 27, 332–350. doi: 10.1098/rspl.1878.0055
Darwin C. R. (1859). The Origin of Species by Means of Natural Selection, or the Preservation of Favoured Races in the Struggle for Life. (London, United Kingdom: Gale and the British Library). doi: 10.5962/bhl.title.61216
DeAngelis D. L., Diaz S. G. (2019). Decision-making in agent-based modeling: A current review and future prospectus. Front. Ecol. Evol. 6. doi: 10.3389/fevo.2018.00237
Denamur E., Matic I. (2006). Evolution of mutation rates in bacteria. Mol. Microbiol. 60, 820–827. doi: 10.1111/j.1365-2958.2006.05150.x
Desai M. M., Weissman D., Feldman M. W. (2007). Evolution can favor antagonistic epistasis. Genetics 177, 1001–1010. doi: 10.1534/genetics.107.075812
Dettman J. R., Rodrigue N., Aaron S. D., Kassen R. (2013). Evolutionary genomics of epidemic and nonepidemic strains of Pseudomonas aeruginosa. Proc. Natl. Acad. Sci. U. S. A. 110, 21065–21070. doi: 10.1073/pnas.1307862110
de Visser J. A. G. M., Cooper T. F., Elena S. F. (2011). The causes of epistasis. Proc. Biol. Sci. / R. Soc. 278, 3617–3624. doi: 10.1098/rspb.2011.1537
de Visser J. A. G. M., Elena S. F., Fragata I., Matuszewski S. (2018). The utility of fitness landscapes and big data for predicting evolution. Heredity 121, 401–405. doi: 10.1038/s41437-018-0128-4
de Visser J. A. G. M., Krug J. (2014). Empirical fitness landscapes and the predictability of evolution. Nat. Rev. Genet. 15, 480–490. doi: 10.1038/nrg3744
Diaz Caballero J., Clark S. T., Coburn B., Zhang Y., Wang P. W., Donaldson S. L., et al. (2015). Selective sweeps and parallel pathoadaptation drive Pseudomonas aeruginosa evolution in the cystic fibrosis lung. mBio 6, e00981–e00915. doi: 10.1128/mBio.00981-15
Di Bella J. M., Bao Y., Gloor G. B., Burton J. P., Reid G. (2013). High throughput sequencing methods and analysis for microbiome research. J. microbiological Methods 95, 401–414. doi: 10.1016/j.mimet.2013.08.011
Dickinson B. C., Leconte A. M., Allen B., Esvelt K. M., Liu D. R. (2013). Experimental interrogation of the path dependence and stochasticity of protein evolution using phage-assisted continuous evolution. Proc. Natl. Acad. Sci. U. S. A. 110, 9007–9012. doi: 10.1073/pnas.1301301110
Doak D. F., Morris W. F. (2010). Demographic compensation and tipping points in climate-induced range shifts. Nature 467, 959–962. doi: 10.1038/nature09439
Doebeli M., Ispolatov I. (2014). Chaos and unpredictability in evolution. Evolution; Int. J. organic Evol. 68, 1365–1373. doi: 10.1111/evo.2014.68.issue-5
Dragosits M., Mattanovich D. (2013). Adaptive laboratory evolution – principles and applications for biotechnology. Microbial Cell factories 12, 64. doi: 10.1186/1475-2859-12-64
Drake J. W., Charlesworth B., Charlesworth D., Crow J. F. (1998). Rates of spontaneous mutation. Genetics 148, 1667–1686. doi: 10.1093/genetics/148.4.1667
Drummond D. A., Bloom J. D., Adami C., Wilke C. O., Arnold F. H. (2005). Why highly expressed proteins evolve slowly. Proceedings of the National Academy of Sciences. 102 (40), 14338–14343. doi: 10.1073/pnas.0504070102
D’Souza G., Kost C. (2016). Experimental evolution of metabolic dependency in bacteria. PLoS Genet. 12, e1006364. doi: 10.1371/journal.pgen.1006364
Duan J., Lupyan D., Wang L. (2020). Improving the accuracy of protein thermostability predictions for single point mutations. Biophys. J. 119, 115–127. doi: 10.1016/j.bpj.2020.05.020
Ebert D. (1998). Experimental evolution of parasites. Science 282, 1432–1435. doi: 10.1126/science.282.5393.1432
Eckert C. G., Samis K. E., Lougheed S. C. (2008). Genetic variation across species’ geographical ranges: the central-marginal hypothesis and beyond. Mol. Ecol. 17, 1170–1188. doi: 10.1111/j.1365-294X.2007.03659.x
Endler J. A., Rojas B. (2009). The spatial pattern of natural selection when selection depends on experience. Am. Nat. 173, E62–E78. doi: 10.1086/596528
Estoup A., Jarne P., Cornuet J.-M. (2002). Homoplasy and mutation model at microsatellite loci and their consequences for population genetics analysis. Mol. Ecol. 11, 1591–1604. doi: 10.1046/j.1365-294X.2002.01576.x
Eyre-Walker A., Keightley P. D. (2007). The distribution of fitness effects of new mutations. Nat. Rev. Genet. 8, 610–618. doi: 10.1038/nrg2146
Fisher K. J., Lang G. I. (2016). Experimental evolution in fungi: An untapped resource. Fungal Genet. biology: FG B 94, 88–94. doi: 10.1016/j.fgb.2016.06.007
Fisher D. O., Owens I. P. F. (2004). The comparative method in conservation biology. Trends Ecol. Evol. 19, 391–398. doi: 10.1016/j.tree.2004.05.004
Fisher D. N., Pruitt J. N. (2020). Insights from the study of complex systems for the ecology and evolution of animal populations. Curr. zoology 66, 1–14. doi: 10.1093/cz/zoz016
Fogle C. A., Nagle J. L., Desai M. M. (2008). Clonal interference, multiple mutations and adaptation in large asexual populations. Genetics 180, 2163–2173. doi: 10.1534/genetics.108.090019
Friedberg E. C., McDaniel L. D., Schultz R. A. (2004). The role of endogenous and exogenous DNA damage and mutagenesis. Curr. Opin. Genet. Dev. 14, 5–10. doi: 10.1016/j.gde.2003.11.001
Fryxell D. C., Wood Z. T., Robinson R., Kinnison M. T., Palkovacs E. P. (2019). Eco-evolutionary feedbacks link prey adaptation to predator performance. Biol. Lett. 15, 20190626. doi: 10.1098/rsbl.2019.0626
Furusawa C., Horinouchi T., Maeda T. (2018). Toward prediction and control of antibiotic-resistance evolution. Curr. Opin. Biotechnol. 54, 45–49. doi: 10.1016/j.copbio.2018.01.026
Futuyma D. J. (2015). “Can modern evolutionary theory explain macroevolution?,” in Macroevolution: Explanation, Interpretation and Evidence. Eds. Serrelli E., Gontier N. (Springer International Publishing, Cham), 29–85.
Futuyma D. J. (2017). Evolutionary biology today and the call for an extended synthesis. Interface Focus 7, 20160145. doi: 10.1098/rsfs.2016.0145
Galen S. C., Natarajan C., Moriyama H., Weber R. E., Fago A., Benham P. M., et al. (2015). Contribution of a mutational hot spot to hemoglobin adaptation in high-altitude Andean house wrens. Proc. Natl. Acad. Sci. U. S. A. 112, 13958–13963. doi: 10.1073/pnas.1507300112
Gallagher A. J., Hammerschlag N., Cooke S. J., Costa D. P., Irschick D. J. (2015). Evolutionary theory as a tool for predicting extinction risk. Trends Ecol. Evol. 30, 61–65. doi: 10.1016/j.tree.2014.12.001
Gerrish P. J., Lenski R. E. (1998). The fate of competing beneficial mutations in an asexual population. Genetica 102–103, 127–144. doi: 10.1023/A:1017067816551
Ghalambor C. K., Walker J. A., Reznick D. N. (2003). Multi-trait selection, adaptation, and constraints on the evolution of burst swimming performance. Integr. Comp. Biol. 43, 431–438. doi: 10.1093/icb/43.3.431
Giraud A., Matic I., Tenaillon O., Clara A., Radman M., Fons M., et al. (2001). Costs and benefits of high mutation rates: adaptive evolution of bacteria in the mouse gut. Science 291, 2606–2608. doi: 10.1126/science.1056421
Gomulkiewicz R., Holt R. D. (1995). When does evolution by natural selection prevent extinction? Evolution; Int. J. organic Evol. 49, 201–207. doi: 10.2307/2410305
Gong L. I., Suchard M. A., Bloom J. D. (2013). Stability-mediated epistasis constrains the evolution of an influenza protein. eLife 2, e00631. doi: 10.7554/eLife.00631.041
Gonzalez A., Ronce O., Ferriere R., Hochberg M. E. (2013). Evolutionary rescue: an emerging focus at the intersection between ecology and evolution. Philos. Trans. R. Soc. London. Ser. B Biol. Sci. 368, 20120404. doi: 10.1098/rstb.2012.0404
González Casanova A., Miró Pina V., Pardo J. C. (2020). The Wright-Fisher model with efficiency. Theor. population Biol. 132, 33–46. doi: doi 10.1016/j.tpb.2020.02.003
Good B. H., McDonald M. J., Barrick J. E., Lenski R. E., Desai M. M. (2017). The dynamics of molecular evolution over 60,000 generations. Nature 551, 45–50. doi: 10.1038/nature24287
Gossmann T. I., Keightley P. D., Eyre-Walker A. (2012). The effect of variation in the effective population size on the rate of adaptive molecular evolution in eukaryotes. Genome Biol. Evol. 4, 658–667. doi: 10.1093/gbe/evs027
Gould S. J. (1989). Wonderful Life: The Burgess Shale and the Nature of History. (New York City, USA: WW Norton & Company).
Govaert L., Fronhofer E. A., Lion S., Eizaguirre C., Bonte D., Egas M., et al. (2019). Eco-evolutionary feedbacks—Theoretical models and perspectives. Funct. Ecol. 33, 13–30. doi: 10.1111/1365-2435.13241
Graham J. K., Smith M. L., Simons A. M. (2014). Experimental evolution of bet hedging under manipulated environmental uncertainty in Neurospora crassa. Proc. Biol. Sci. / R. Soc. 281(1787), 20140706. doi: 10.1098/rspb.2014.0706
Grant P. R., Grant B. R. (2002). Unpredictable evolution in a 30-year study of Darwin’s finches. Science 296, 707–711. doi: 10.1126/science.1070315
Grant P. R., Grant B. R., Huey R. B., Johnson M. T.J., Knoll A. H., Schmitt J. (2017). Evolution caused by extreme events. Philos. Trans. R. Soc. London. Ser. B Biol. Sci. 372. doi: 10.1098/rstb.2016.0146
Gravel S. (2016). When is selection effective? Genetics 203, 451–462. doi: 10.1534/genetics.115.184630
Gregory T. R. (2009). Understanding natural selection: Essential concepts and common misconceptions. Evolution: Educ. Outreach 2, 156–175. doi: 10.1007/s12052-009-0128-1
Gresham D., Dunham M. J. (2014). The enduring utility of continuous culturing in experimental evolution. Genomics 104, 399–405. doi: 10.1016/j.ygeno.2014.09.015
Gupta A., Gupta R., Singh R. L. (2017). “Microbes and environment,” in Principles and Applications of Environmental Biotechnology for a Sustainable Future. (Springer Singapore, Singapore), 43–84.
Gupta A., LaBar T., Miyagi M., Adami C. (2016). Evolution of genome size in asexual digital organisms. Sci. Rep. 6, 25786. doi: 10.1038/srep25786
Gutierrez B., Escalera-Zamudio M., Pybus O. G. (2019). Parallel molecular evolution and adaptation in viruses. Curr. Opin. Virol. 34, 90–96. doi: 10.1016/j.coviro.2018.12.006
Hairston N. G. Jr, Ellner S. P., Geber M. A., Yoshida T., Fox J. A. (2005). Rapid evolution and the convergence of ecological and evolutionary time. Ecol. Lett. 8, 1114–1127. doi: 10.1111/j.1461-0248.2005.00812.x
Hancock Z. B., Lehmberg E. S., Bradburd G. S. (2021). Neo-darwinism still haunts evolutionary theory: A modern perspective on Charlesworth, Lande, and Slatkin, (1982). Evolution; Int. J. organic Evol. 75, 1244–1255. doi: 10.1111/evo.14268
Handel A., Rozen D. E. (2009). The impact of population size on the evolution of asexual microbes on smooth versus rugged fitness landscapes. BMC evolutionary Biol. 9, 236. doi: 10.1186/1471-2148-9-236
Hartl D. L. (2014). What can we learn from fitness landscapes? Curr. Opin. Microbiol. 21, 51–57. doi: 10.1016/j.mib.2014.08.001
Harvey P. H., Pagel M. D. (1991) The comparative method in evolutionary biology. Available online at: http://www.sbs.utexas.edu/bio386/Readings/Text/Harvey.Ch.1.pdf (Accessed 18 June 2023). doi: 10.1093/oso/9780198546412.001.0001
Hawkins N. J., Fraaije B. A. (2021). Contrasting levels of genetic predictability in the evolution of resistance to major classes of fungicides. Mol. Ecol. 30, 5318–5327. doi: 10.1111/mec.15877
Hayden E. J., Bratulic S., Koenig I., Ferrada E., Wagner A. (2014). The effects of stabilizing and directional selection on phenotypic and genotypic variation in a population of RNA enzymes. J. Mol. Evol. 78, 101–108. doi: 10.1007/s00239-013-9604-x
Hector A., Hautier Y., Saner P., Wacker L., Bagchi R., Joshi J., et al. (2010). General stabilizing effects of plant diversity on grassland productivity through population asynchrony and overyielding. Ecology 91, 2213–2220. doi: 10.1890/09-1162.1
Heffernan J. M., Wahl L. M. (2002). The effects of genetic drift in experimental evolution. Theor. population Biol. 62, 349–356. doi: 10.1016/S0040-5809(02)00002-3
Hendry A. P., Kinnison M. T. (1999). Perspective: The pace of modern life: Measuring rates of contemporary microevolution. Evolution; Int. J. organic Evol. 53, 1637–1653. doi: 10.2307/2640428
Her H.-L., Wu Y.-W. (2018). A pan-genome-based machine learning approach for predicting antimicrobial resistance activities of the Escherichia coli strains. Bioinformatics 34, i89–i95. doi: 10.1093/bioinformatics/bty276
Heyduk K., Moreno-Villena J. J., Gilman I. S., Christin P.-A., Edwards E. J. (2019). The genetics of convergent evolution: insights from plant photosynthesis. Nat. Rev. Genet. 20, 485–493. doi: 10.1038/s41576-019-0107-5
Hill W. G. (2014). Applications of population genetics to animal breeding, from Wright, Fisher and Lush to genomic prediction. Genetics 196, 1–16. doi: 10.1534/genetics.112.147850
Hindré T., Knibbe C., Beslon G., Schneider D. (2012). New insights into bacterial adaptation through in vivo and in silico experimental evolution. Nat. Rev. Microbiol. 10, 352–365. doi: 10.1038/nrmicro2750
Hoang K. L., Morran L. T., Gerardo N. M. (2016). Experimental evolution as an underutilized tool for studying beneficial animal-microbe interactions. Front. Microbiol. 7, 1444. doi: 10.3389/fmicb.2016.01444
Hoffmann A. A., Hercus M. J. (2000). Environmental stress as an evolutionary force. Bioscience 50, 217–226. doi: 10.1641/0006-3568(2000)050[0217:ESAAEF]2.3.CO;2
Hofrichter J., Jost J., Tran T. D. (2017). “The wright–fisher model,” in Understanding Complex Systems. (Springer International Publishing, Cham), 17–43.
Hohenlohe P. A., Funk W. C., Rajora O. P. (2021). Population genomics for wildlife conservation and management. Mol. Ecol. 30, 62–82. doi: 10.1111/mec.15720
Holmes E. C. (2013). What can we predict about viral evolution and emergence? Curr. Opin. Virol. 3, 180–184. doi: 10.1016/j.coviro.2012.12.003
Horst J.-P., Wu T.-H., Marinus M. G. (1999). Escherichia coli mutator genes. Trends Microbiol. 7, 29–36. doi: 10.1016/S0966-842X(98)01424-3
Horton J. S., Taylor T. B. (2023). Mutation bias and adaptation in bacteria. Microbiol. 169 (11), 001404. doi: 10.1099/mic.0.001404
Hosseini S.-R., Diaz-Uriarte R., Markowetz F., et al. (2019). Estimating the predictability of cancer evolution. Bioinformatics 35, i389–i397. doi: 10.1093/bioinformatics/btz332
Hua B., Springer M. (2018). Widespread cumulative influence of small effect size mutations on yeast quantitative traits. Cell Syst. 7, 590–600.e6. doi: 10.1016/j.cels.2018.11.004
Hughes A. L. (2008). Near neutrality: leading edge of the neutral theory of molecular evolution. Ann. New York Acad. Sci. 1133, 162–179. doi: 10.1196/annals.1438.001
Huxley J. (1942). Evolution: The Modern Synthesis. (London, United Kingdom: Harper & Brothers). Avaialble at: https://www.cabidigitallibrary.org/doi/full/10.5555/19432202794.
Imhof M., Schlotterer C. (2001). Fitness effects of advantageous mutations in evolving Escherichia coli populations. Proc. Natl. Acad. Sci. U. S. A. 98, 1113–1117. doi: 10.1073/pnas.98.3.1113
James M. E., Wilkinson M. J., Bernal D. M., Liu H., North H. L., Engelstädter J., et al. (2021). Phenotypic and genotypic parallel evolution in parapatric ecotypes of Senecio. Evolution; Int. J. organic Evol. 75, 3115–3131. doi: 10.1111/evo.14387
Jatsenko T., Tover A., Tegova R., Kivisaar M. (2010). Molecular characterization of Rifr mutations in Pseudomonas aeruginosa and Pseudomonas putida. Mutat. Res. 683, 106–114. doi: 10.1016/j.mrfmmm.2009.10.015
Jensen J. D., Payseur B. A., Stephan W., Aquadro C. F., Lynch M., Charlesworth D., et al. (2019). The importance of the Neutral Theory in 1968 and 50 years on: A response to Kern and Hahn 2018. Evolution; Int. J. organic Evol. 73, 111–114. doi: 10.1111/evo.2019.73.issue-1
Jeong H., Lee S. J., Kim P. (2016). Procedure for adaptive laboratory evolution of microorganisms using a chemostat. J. visualized experiments: JoVE 115), 2016. doi: 10.3791/54446
Jiang X., Mu B., Huang Z., Zhang M., Wang X., Tao S. (2010). Impacts of mutation effects and population size on mutation rate in asexual populations: a simulation study. BMC evolutionary Biol. 10, 298. doi: 10.1186/1471-2148-10-298
Joyce P., Rokyta D. R., Beisel C. J., Orr H. A. (2008). A general extreme value theory model for the adaptation of DNA sequences under strong selection and weak mutation. Genetics 180, 1627–1643. doi: 10.1534/genetics.108.088716
Kaltenbach M., Tokuriki N. (2014). Dynamics and constraints of enzyme evolution. J. Exp. zoology. Part B Mol. Dev. Evol. 322, 468–487. doi: 10.1002/jez.b.22562
Karlin S., O’Donald P. (1978). Some population genetic models combining sexual selection with assortative mating. Heredity 41, 165–174. doi: 10.1038/hdy.1978.85
Kassen R. (2009). Toward a general theory of adaptive radiation: insights from microbial experimental evolution. Ann. New York Acad. Sci. 1168, 3–22. doi: 10.1111/j.1749-6632.2009.04574.x
Katju V., Bergthorsson U. (2019). Old trade, new tricks: Insights into the spontaneous mutation process from the partnering of classical mutation accumulation experiments with high-throughput genomic approaches. Genome Biol. Evol. 11, 136–165. doi: 10.1093/gbe/evy252
Kawecki T. J., Lenski R. E., Ebert D., Hollis B., Olivieri I., Whitlock M. C. (2012). Experimental evolution. Trends Ecol. Evol. 27, 547–560. doi: 10.1016/j.tree.2012.06.001
Kazahari N., Inoue E., Nakagawa N., Kawamoto Y., Uno T., Inoue-Murayama M. (2023). Genetic effects of demographic bottleneck and recovery in Kinkazan Island and mainland populations of Japanese macaques (Macaca fuscata). Primates; J. primatology 64, 239–246. doi: 10.1007/s10329-023-01050-3
Kelly M. W. (2022). Experimental evolution reveals complex responses to environmental change. Proc. Natl. Acad. Sci. U. S. A. 119(43), p.e2214263119. doi: 10.1073/pnas.2214263119
Kern A. D., Hahn M. W. (2018). The neutral theory in light of natural selection. Mol. Biol. Evol. 35, 1366–1371. doi: 10.1093/molbev/msy092
Kimura M. (1967). On the evolutionary adjustment of spontaneous mutation rates. Genet. Res. 9, 23–34. doi: 10.1017/S0016672300010284
Kimura M. (1977). Preponderance of synonymous changes as evidence for the neutral theory of molecular evolution. Nature 267, 275–276. doi: 10.1038/267275a0
Kimura M. (1987). Molecular evolutionary clock and the neutral theory. J. Mol. Evol. 26, 24–33. doi: 10.1007/BF02111279
Kimura M. (2020). “The neutral theory and molecular evolution,” in My Thoughts on Biological Evolution. Ed. Kimura M. (Springer Singapore, Singapore) 119–138.
Knoll A. H. (1992). The early evolution of eukaryotes: a geological perspective. Science 256, 622–627. doi: 10.1126/science.1585174
Kondrashov D. A., Kondrashov F. A. (2015). Topological features of rugged fitness landscapes in sequence space. Trends genetics: TIG 31, 24–33. doi: 10.1016/j.tig.2014.09.009
Kousathanas A., Keightley P. D. (2013). A comparison of models to infer the distribution of fitness effects of new mutations. Genetics 193, 1197–1208. doi: 10.1534/genetics.112.148023
Kuo C.-H., Moran N. A., Ochman H. (2009). The consequences of genetic drift for bacterial genome complexity. Genome Res. 19, 1450–1454. doi: 10.1101/gr.091785.109
Kustin T., Stern A. (2021). Biased mutation and selection in RNA viruses. Mol. Biol. Evol. 38, 575–588. doi: 10.1093/molbev/msaa247
Kvitek D. J., Sherlock G. (2011). Reciprocal sign epistasis between frequently experimentally evolved adaptive mutations causes a rugged fitness landscape. PLoS Genet. 7, e1002056. doi: 10.1371/journal.pgen.1002056
LaBar T., Adami C. (2016). Different evolutionary paths to complexity for small and large populations of digital organisms. PLoS Comput. Biol. 12, e1005066. doi: 10.1371/journal.pcbi.1005066
Lalić J., Elena S. F. (2013). Epistasis between mutations is host-dependent for an RNA virus. Biol. Lett. 9, 20120396. doi: 10.1098/rsbl.2012.0396
Lang G. I., Desai M. M. (2014). The spectrum of adaptive mutations in experimental evolution. Genomics 104, 412–416. doi: 10.1016/j.ygeno.2014.09.011
Langerhans R. B. (2008). Predictability of phenotypic differentiation across flow regimes in fishes. Integr. Comp. Biol. 48, 750–768. doi: 10.1093/icb/icn092
Langerhans R. B. (2010). Predicting evolution with generalized models of divergent selection: a case study with poeciliid fish. Integr. Comp. Biol. 50, 1167–1184. doi: 10.1093/icb/icq117
Lankau R. A., Strauss S. Y. (2011). Newly rare or newly common: evolutionary feedbacks through changes in population density and relative species abundance, and their management implications. Evolutionary Appl. 4, 338–353. doi: 10.1111/j.1752-4571.2010.00173.x
Lässig M., Mustonen V., Walczak A. M. (2017). Predicting evolution. Nat. Ecol. Evol. 1, 77. doi: 10.1038/s41559-017-0077
LeClerc J. E., Li B., Payne W. L., Cebula T. A. (1996). High mutation frequencies among Escherichia coli and Salmonella pathogens. Science 274, 1208–1211. doi: 10.1126/science.274.5290.1208
Lee H., Popodi E., Tang H., Foster P. L. (2012). Rate and molecular spectrum of spontaneous mutations in the bacterium Escherichia coli as determined by whole-genome sequencing. Proc. Natl. Acad. Sci. U. S. A. 109, E2774–E2783. doi: 10.1073/pnas.1210309109
Lehtonen J. (2020). Longevity and the drift barrier: Bridging the gap between Medawar and Hamilton. Evol. Lett. 4, 382–393. doi: 10.1002/evl3.173
Leigh E. G. Jr (2007). Neutral theory: a historical perspective. J. evolutionary Biol. 20, 2075–2091. doi: 10.1111/j.1420-9101.2007.01410.x
Lenormand T. (2002). Gene flow and the limits to natural selection. Trends Ecol. Evol. 17, 183–189. doi: 10.1016/S0169-5347(02)02497-7
Lenormand T., Roze D., Rousset F. (2009). Stochasticity in evolution. Trends Ecol. Evol. 24, 157–165. doi: 10.1016/j.tree.2008.09.014
Lenski R. E. (2017). Experimental evolution and the dynamics of adaptation and genome evolution in microbial populations. ISME J. 11, 2181–2194. doi: 10.1038/ismej.2017.69
Lenski R. E. (2023). Revisiting the design of the long-term evolution experiment with escherichia coli. J. Mol. Evol. 91, 241–253. doi: 10.1007/s00239-023-10095-3
Lenski R. E., Rose M. R., Simpson S. C., Tadler S. C. (1991). Long-term experimental evolution in escherichia coli. I. Adaptation and divergence during 2,000 generations. Am. Nat. 138, 1315–1341. doi: 10.1086/285289
Lenski R. E., Travisano M. (1994). Dynamics of adaptation and diversification: a 10,000-generation experiment with bacterial populations. Proc. Natl. Acad. Sci. U. S. A. 91, 6808–6814. doi: 10.1073/pnas.91.15.6808
Li H., Roossinck M. J. (2004). Genetic bottlenecks reduce population variation in an experimental RNA virus population. J. Virol. 78, 10582–10587. doi: 10.1128/JVI.78.19.10582-10587.2004
Lieberman T. D., Michel J.-B., Aingaran M., Potter-Bynoe G., Roux D., Davis M. R. Jr., et al. (2011). Parallel bacterial evolution within multiple patients identifies candidate pathogenicity genes. Nat. Genet. 43, 1275–1280. doi: 10.1038/ng.997
Lind P. A., Farr A. D., Rainey P. B. (2015). Experimental evolution reveals hidden diversity in evolutionary pathways. eLife 4. doi: 10.7554/eLife.07074.019
Lind P. A., Libby E., Herzog J., Rainey P. B. (2019). Predicting mutational routes to new adaptive phenotypes. eLife 8. doi: 10.7554/eLife.38822
Lindsey H. A., Gallie J., Taylor S., Kerr B. (2013). Evolutionary rescue from extinction is contingent on a lower rate of environmental change. Nature 494, 463–467. doi: 10.1038/nature11879
Linnen C. R. (2018). Predicting evolutionary predictability. 27 (12), 2647–2650. doi: 10.1111/mec.14444
Lipinski K. A., Barber L. J., Davies M. N., Ashenden M., Sottoriva A., Gerlinger M. (2016). Cancer evolution and the limits of predictability in precision cancer medicine. Trends Cancer Res. 2, 49–63. doi: 10.1016/j.trecan.2015.11.0031
Liu Y. (2018). “Chapter five - natural selection and pangenesis: the darwinian synthesis of evolution and genetics,” in Advances in Genetics. Ed. Kumar D. (Academic Press), 121–142. doi: 10.1016/bs.adgen.2018.05.010
Liu J., Champer J., Langmüller A. M., Liu C., Chung J., Reeves R., et al. (2019). Maximum likelihood estimation of fitness components in experimental evolution. Genetics 211, 1005–1017. doi: 10.1016/j.trecan.2015.11.003
Liu H., Zhang J. (2022). Is the mutation rate lower in genomic regions of stronger selective constraints? Mol. Biol. Evol. 39 (8). doi: 10.1093/molbev/msac169
Lobkovsky A. E., Koonin E. V. (2012). Replaying the tape of life: quantification of the predictability of evolution. Front. Genet. 3, 246. doi: 10.3389/fgene.2012.00246
Lohr J. N., David P., Haag C. R. (2014). Reduced lifespan and increased ageing driven by genetic drift in small populations. Evolution; Int. J. organic Evol. 68, 2494–2508. doi: 10.1111/evo.2014.68.issue-9
Long A., Liti G., Luptak A., Tenaillon O. (2015). Elucidating the molecular architecture of adaptation via evolve and resequence experiments. Nat. Rev. Genet. 16, 567–582. doi: 10.1038/nrg3937
Losos J. B. (2011). Convergence, adaptation, and constraint. Evolution; Int. J. organic Evol. 65, 1827–1840. doi: 10.1111/evo.2011.65.issue-7
Lynch M. (2010). Evolution of the mutation rate. Trends genetics: TIG 26, 345–352. doi: 10.1016/j.tig.2010.05.003
Lynch M., Ackerman M. S., Gout J.-F., Long H., Sung W., Thomas W. K., et al. (2016). Genetic drift, selection and the evolution of the mutation rate. Nat. Rev. Genet. 17, 704–714. doi: 10.1038/nrg.2016.104
Mabee P. M., Ashburner M., Cronk Q., Gkoutos G. V., Haendel M., Segerdell E., et al. (2007). Phenotype ontologies: the bridge between genomics and evolution. Trends Ecol. Evol. 22, 345–350. doi: 10.1016/j.tree.2007.03.013
MacColl A. D. C. (2011). The ecological causes of evolution. Trends Ecol. Evol. 26, 514–522. doi: 10.1016/j.tree.2011.06.009
MacLean R. C., Hall A. R., Perron G. G., Buckling A. (2010). The population genetics of antibiotic resistance: integrating molecular mechanisms and treatment contexts. Nat. Rev. Genet. 11, 405–414. doi: 10.1038/nrg2778
Maddamsetti R., Lenski R. E., Barrick J. E. (2015). Adaptation, clonal interference, and frequency-dependent interactions in a long-term evolution experiment with escherichia coli. Genetics 200, 619–631. doi: 10.1534/genetics.115.176677
Mahfouz N., Ferreira I., Beisken S., von Haeseler A., Posch A. E. (2020). Large-scale assessment of antimicrobial resistance marker databases for genetic phenotype prediction: a systematic review. J. antimicrobial chemotherapy 75, 3099–3108. doi: 10.1093/jac/dkaa257
Makarenkov V., Kevorkov D., Legendre P. (2006). “Phylogenetic network construction approaches,” in Applied Mycology and Biotechnology. (Elsevier), 61–97.
Marin-Bejar O., Rogiers A., Dewaele M., Femel J., Karras P., Pozniak J., et al. (2021). Evolutionary predictability of genetic versus nongenetic resistance to anticancer drugs in melanoma. Cancer Cell 39, 1135–1149.e8. doi: 10.1016/j.ccell.2021.05.015
Martincorena I., Seshasayee A. S. N., Luscombe N. M. (2012). Evidence of non-random mutation rates suggests an evolutionary risk management strategy. Nature 485, 95–98. doi: 10.1038/nature10995
Martínez J. L., Baquero F., Andersson D. I. (2007). Predicting antibiotic resistance. Nat. Rev. Microbiol. 5, 958–965. doi: 10.1038/nrmicro1796
Marvig R. L., Sommer L. M., Molin S., Johansen H. K. (2015). Convergent evolution and adaptation of Pseudomonas aeruginosa within patients with cystic fibrosis. Nat. Genet. 47, 57–64. doi: 10.1038/ng.3148
Mas A., Lagadeuc Y., Vandenkoornhuyse P. (2020). Reflections on the predictability of evolution: Toward a Conceptual Framework. iScience 23, 101736. doi: 10.1016/j.isci.2020.101736
Mathieson I. (2021). The omnigenic model and polygenic prediction of complex traits. Am. J. Hum. Genet. 108, 1558–1563. doi: 10.1016/j.ajhg.2021.07.003
McDonald M. J. (2019). Microbial Experimental Evolution - a proving ground for evolutionary theory and a tool for discovery. EMBO Rep. 20, e46992. doi: 10.15252/embr.201846992
McDonald M. J., Gehrig S. M., Meintjes P. L., Zhang X.-X., Rainey P. B. (2009). Adaptive divergence in experimental populations of Pseudomonas fluorescens. IV. Genetic constraints guide evolutionary trajectories in a parallel adaptive radiation. Genetics 183, 1041–1053. doi: 10.1534/genetics.109.107110
McDonough Y., Connallon T. (2023). Effects of population size change on the genetics of adaptation following an abrupt change in environment. Evolution; Int. J. organic evolution. forthcoming June 4, 2023. doi: 10.1093/evolut/qpad103
McKeown A. N., Bridgham J. T., Anderson D. W., Murphy M. N., Ortlund E. A., Thornton J. W. (2014). Evolution of DNA specificity in a transcription factor family produced a new gene regulatory module. Cell 159, 58–68. doi: 10.1016/j.cell.2014.09.003
McVean G. (2010). What drives recombination hotspots to repeat DNA in humans? Philos. Trans. R. Soc. London. Ser. B Biol. Sci. 365, 1213–1218. doi: 10.1098/rstb.2009.0299
Melbinger A., Vergassola M. (2015). The Impact of Environmental Fluctuations on Evolutionary Fitness Functions. Sci. Rep. 5, 15211. doi: 10.1038/srep15211
Merlo L. M. F., Sprouffske K., Howard T. C., Gardiner K. L., Caulin A. F., Blum S. M., et al. (2020). Application of simultaneous selective pressures slows adaptation. Evolutionary Appl. 13, 1615–1625. doi: 10.1111/eva.13062
Mertens D., Boege K., Kessler A., Koricheva J., Thaler J. S., Whiteman N. K., et al. (2021). Predictability of biotic stress structures plant defence evolution. Trends Ecol. Evol. 36, 444–456. doi: 10.1016/j.tree.2020.12.009
Metzker M. L. (2010). Sequencing technologies - the next generation. Nat. Rev. Genet. 11, 31–46. doi: 10.1038/nrg2626
Miller J. H. (1996). SPONTANEOUS MUTATORS IN BACTERIA: insights into pathways of mutagenesis and repair. Annu. Rev. Microbiol. 50, 625–643. doi: 10.1146/annurev.micro.50.1.625
Miller M., Gauthier N., Reznik E., Sander C. (2016). Pan-cancer analysis of mutation hotspots in protein domains. Eur. J. Cancer (Oxford England: 1990) 61, S20–S21. doi: 10.1016/j.cels.2015.08.014
Miton C. M., Tokuriki N. (2016). How mutational epistasis impairs predictability in protein evolution and design. Protein science: Publ. Protein Soc. 25, 1260–1272. doi: 10.1002/pro.2876
Monroe J. G., Srikant T., Carbonell-Bejerano P., Becker C., Lensink M., Exposito-Alonso M., et al. (2022). Mutation bias reflects natural selection in Arabidopsis thaliana. Nature 602, 101–105. doi: 10.1038/s41586-021-04269-6
Morris D. H., Gostic K. M., Pompei S., Bedford T., Łuksza M., Neher R. A., et al. (2018). Predictive modeling of influenza shows the promise of applied evolutionary biology. Trends Microbiol. 26, 102–118. doi: 10.1016/j.tim.2017.09.004
Morrison L., Zembower T. R. (2020). Antimicrobial resistance. Gastrointestinal endoscopy Clinics North America 30, 619–635. doi: 10.1016/j.giec.2020.06.004
Morton B. R. (1995). Neighboring base composition and transversion/transition bias in a comparison of rice and maize chloroplast noncoding regions. Proc. Natl. Acad. Sci. U. S. A. 92, 9717–9721. doi: 10.1073/pnas.92.21.9717
Mouquet N., Lagadeuc Y., Devictor V., Doyen L., Duputié A., Eveillard D., et al. (2015). REVIEW: Predictive ecology in a changing world. J. Appl. Ecol. 52, 1293–1310. doi: 10.1111/1365-2664.12482
Moustakas A., Evans M. R. (2013). Integrating evolution into ecological modelling: accommodating phenotypic changes in agent based models. PLoS One 8, e71125. doi: 10.1371/journal.pone.0071125
Nachman M. W., Crowell S. L. (2000). Estimate of the mutation rate per nucleotide in humans. Genetics 156, 297–304. doi: 10.1093/genetics/156.1.297
Nei M., Suzuki Y., Nozawa M. (2010). The neutral theory of molecular evolution in the genomic era. Annu. Rev. Genomics Hum. Genet. 11, 265–289. doi: 10.1146/annurev-genom-082908-150129
Nemiche M., M’Hamdi A., Chakraoui M., Cavero V., Pla Lopez R. (2013). A theoretical agent-based model to simulate an artificial social evolution. Syst. Res. Behav. Sci. 30, 693–702. doi: 10.1002/sres.2238
Nesta A. V., Tafur D., Beck C. R. (2021). Hotspots of human mutation. Trends genetics: TIG 37, 717–729. doi: 10.1016/j.tig.2020.10.003
Nghe P., Kogenaru M., Tans S. J. (2018). Sign epistasis caused by hierarchy within signalling cascades. Nat. Commun. 9, 1451. doi: 10.1038/s41467-018-03644-8
Niida A., Iwasaki W. M. (2022). “Agent-based modeling and analysis of cancer evolution,” in Simulation Modeling (IntechOpen).
Norton H. L., Kittles R. A., Parra E., McKeigue P., Mao X., Cheng K., et al. (2007). Genetic evidence for the convergent evolution of light skin in Europeans and East Asians. Mol. Biol. Evol. 24, 710–722. doi: 10.1093/molbev/msl203
Nosil P., Flaxman S. M., Feder J. L., Gompert Z. (2020). Increasing our ability to predict contemporary evolution. Nat. Commun. 11, 5592. doi: 10.1038/s41467-020-19437-x
Nosil P., Villoutreix R., de Carvalho C. F., Farkas T. E., Soria-Carrasco V., Feder J. L., et al. (2018). Natural selection and the predictability of evolution in Timema stick insects. Science 359, 765–770. doi: 10.1126/science.aap9125
Nowell P. C. (1976). The clonal evolution of tumor cell populations. Science 194, 23–28. doi: 10.1126/science.959840
Ohta T. (1992). The nearly neutral theory of molecular evolution. Annu. Rev. Ecol. systematics 23, 263–286. doi: 10.1146/annurev.es.23.110192.001403
Oliver A., Cantón R., Campo P., Baquero F., Blázquez J. (2000). High frequency of hypermutable Pseudomonas aeruginosa in cystic fibrosis lung infection. Science. 288(5469), 1251–1253. doi: 10.1126/science.288.5469.1251
Oostermeijer J. G. B. (2003). Threats to rare plant persistence. In Population viability in plants: Conservation, management, and modeling of rare plants. Berlin, Heidelberg: Springer Berlin Heidelberg, 17–58. doi: 10.1007/978-3-662-09389-4
Ord T. J., Summers T. C. (2015). Repeated evolution and the impact of evolutionary history on adaptation. BMC evolutionary Biol. 15, 137. doi: 10.1186/s12862-015-0424-z
Orgogozo V. (2015). Replaying the tape of life in the twenty-first century. Interface Focus 5, 20150057. doi: 10.1098/rsfs.2015.0057
Orr H. A. (2000). The rate of adaptation in asexuals. Genetics 155, 961–968. doi: 10.1093/genetics/155.2.961
Orr H. A. (2005). The probability of parallel evolution. Evolution; Int. J. organic Evol. 59, 216–220. doi: 10.1111/j.0014-3820.2005.tb00907.x
Ossowski S., Schneeberger K., Lucas-Lledó J. I., Warthmann N., Clark R. M., Shaw R. G., et al. (2010). The rate and molecular spectrum of spontaneous mutations in Arabidopsis thaliana. Science 327, 92–94. doi: 10.1126/science.1180677
Ostrowski E. A., Woods R. J., Lenski R. E. (2008). The genetic basis of parallel and divergent phenotypic responses in evolving populations of Escherichia coli. Proc. Biol. Sci. / R. Soc. 275, 277–284. doi: 10.1098/rspb.2007.1244
Ouborg N. J. (2010). Integrating population genetics and conservation biology in the era of genomics. Biol. Lett. 6, 3–6. doi: 10.1098/rsbl.2009.0590
Packer M. S., Liu D. R. (2015). Methods for the directed evolution of proteins. Nat. Rev. Genet. 16, 379–394. doi: 10.1038/nrg3927
Palmer A. C., Kishony R. (2013). Understanding, predicting and manipulating the genotypic evolution of antibiotic resistance. Nat. Rev. Genet. 14, 243–248. doi: 10.1038/nrg3351
Palmer A. C., Toprak E., Baym M., et al. (2015). Delayed commitment to evolutionary fate in antibiotic resistance fitness landscapes. Nat. Commun. 6, 7385. doi: 10.1038/ncomms8385
Payne J. L., Menardo F., Trauner A., et al. (2019). Transition bias influences the evolution of antibiotic resistance in Mycobacterium tuberculosis. PLoS Biol. 17, e3000265. doi: 10.1371/journal.pbio.3000265
Peck K. M., Lauring A. S. (2018). Complexities of viral mutation rates. J. Virol. 92. doi: 10.1128/JVI.01031-17
Perera D., Poulos R. C., Shah A., Beck D., Pimanda J. E., Wong J. W. H. (2016). Differential DNA repair underlies mutation hotspots at active promoters in cancer genomes. Nature 532, 259–263. doi: 10.1038/nature17437
Pflughoeft K. J., Versalovic J. (2012). Human microbiome in health and disease. Annu. Rev. Pathol. 7, 99–122. doi: 10.1146/annurev-pathol-011811-132421
Phillips P. C. (2008). Epistasis–the essential role of gene interactions in the structure and evolution of genetic systems. Nat. Rev. Genet. 9, 855–867. doi: 10.1038/nrg2452
Pilakouta N., Humble J. L., Hill I. D. C., Arthur J., Costa A. P. B., Smith B. A., et al. (2023). Testing the predictability of morphological evolution in contrasting thermal environments. Evolution; Int. J. organic Evol. 77, 239–253. doi: 10.1093/evolut/qpac018
Poelwijk F. J., Tănase-Nicola S., Kiviet D. J., Tans S. J. (2011). Reciprocal sign epistasis is a necessary condition for multi-peaked fitness landscapes. J. Theor. Biol. 272, 141–144. doi: 10.1016/j.jtbi.2010.12.015
Press M. O., Hall A. N., Morton E. A., Queitsch C. (2019). Substitutions are boring: Some arguments about parallel mutations and high mutation rates. Trends genetics: TIG 35, 253–264. doi: 10.1016/j.tig.2019.01.002
Pritchard J. K., Di Rienzo A. (2010). Adaptation - not by sweeps alone. Nat. Rev. Genet. 11, 665–667. doi: 10.1038/nrg2880
Pritchard J. K., Pickrell J. K., Coop G. (2010). The genetics of human adaptation: hard sweeps, soft sweeps, and polygenic adaptation. Curr. biology: CB 20, R208–R215. doi: 10.1016/j.cub.2009.11.055
Qiang-long Z., Shi L., Peng G., Fei-shi L. (2014). High-throughput sequencing technology and its application. J. Northeast Agric. Univ. 21, 84–96. doi: 10.1016/S1006-8104(14)60073-8
Qin D. (2019). Next-generation sequencing and its clinical application. Cancer Biol. Med. 16, 4–10. doi: 10.20892/j.issn.2095-3941.2018.0055
Radchuk V., Reed T., Teplitsky C., van de Pol M., Charmantier A., Hassall C., et al. (2019). Adaptive responses of animals to climate change are most likely insufficient. Nat. Commun. 10, 3109. doi: 10.1038/s41467-019-10924-4
Ramiro R. S., Durão P., Bank C., Gordo I. (2020). Low mutational load and high mutation rate variation in gut commensal bacteria. PLoS Biol. 18, e3000617. doi: 10.1371/journal.pbio.3000617
Ratcliff W. C., Denison R. F., Borrello M., Travisano M. (2012). Experimental evolution of multicellularity. Proc. Natl. Acad. Sci. U. S. A. 109, 1595–1600. doi: 10.1073/pnas.1115323109
Raynes Y., Wylie C. S., Sniegowski P. D., Weinreich D. M. (2018). Sign of selection on mutation rate modifiers depends on population size. Proc. Natl. Acad. Sci. U. S. A. 115, 3422–3427. doi: 10.1073/pnas.1713338115
Razeto-Barry P., Díaz J., Vásquez R. A. (2012). The nearly neutral and selection theories of molecular evolution under the fisher geometrical framework: substitution rate, population size, and complexity. Genetics 191, 523–534. doi: 10.1534/genetics.112.138628
Rego-Costa A., Débarre F., Chevin L.-M. (2018). Chaos and the (un)predictability of evolution in a changing environment. Evolution. 72(2), 375–385. doi: 10.1101/222471
Rellstab C., Dauphin B., Exposito-Alonso M. (2021). Prospects and limitations of genomic offset in conservation management. Evolutionary Appl. 14, 1202–1212. doi: 10.1111/eva.13205
Remigi P., Masson-Boivin C., Rocha E. P. C. (2019). Experimental evolution as a tool to investigate natural processes and molecular functions. Trends Microbiol. 27, 623–634. doi: 10.1016/j.tim.2019.02.003
Reuter J. A., Spacek D. V., Snyder M. P. (2015). High-throughput sequencing technologies. Mol. Cell 58, 586–597. doi: 10.1016/j.molcel.2015.05.004
Rieseberg L. H., Widmer A., Arntz A. M., Burke J. M. (2002). Directional selection is the primary cause of phenotypic diversification. Proc. Natl. Acad. Sci. U. S. A. 99, 12242–12245. doi: 10.1073/pnas.242579899
Rockman M. V. (2012). The QTN program and the alleles that matter for evolution: all that’s gold does not glitter. Evolution; Int. J. organic Evol. 66, 1–17. doi: 10.1111/evo.2012.66.issue-1
Roff D. A., Fairbairn D. J. (2012). The evolution of trade-offs under directional and correlational selection. Evolution; Int. J. organic Evol. 66, 2461–2474. doi: 10.1111/evo.2012.66.issue-8
Rogozin I. B., Pavlov Y. I. (2003). Theoretical analysis of mutation hotspots and their DNA sequence context specificity. Mutat. Res. 544, 65–85. doi: 10.1016/S1383-5742(03)00032-2
Rohlfs R. V., Weir B. S. (2008). Distributions of Hardy–Weinberg equilibrium test statistics. Genetics 180, 1609–1616. doi: 10.1534/genetics.108.088005
Rokyta D. R., Joyce P., Caudle S. B., Wichman H. A. (2005). An empirical test of the mutational landscape model of adaptation using a single-stranded DNA virus. Nat. Genet. 37, 441–444. doi: 10.1038/ng1535
Rouzine I. M., Rodrigo A., Coffin J. M. (2001). Transition between stochastic evolution and deterministic evolution in the presence of selection: general theory and application to virology. Microbiol. Mol. Biol. reviews: MMBR 65, 151–185. doi: 10.1128/MMBR.65.1.151-185.2001
Sackman A. M., McGee L. W., Morrison A. J., Pierce J., Anisman J., Hamilton H., et al. (2017). Mutation-driven parallel evolution during viral adaptation. Mol. Biol. Evol. 34, 3243–3253. doi: 10.1093/molbev/msx257
Sainudiin R., Durrett R. T., Aquadro C. F., Nielsen R. (2004). Microsatellite mutation models. Genetics 168, 383–395. doi: 10.1534/genetics.103.022665
Salanti G., Amountza G., Ntzani E. E., Ioannidis J. P. A. (2005). Hardy–Weinberg equilibrium in genetic association studies: an empirical evaluation of reporting, deviations, and power. Eur. J. Hum. genetics: EJHG 13, 840–848. doi: 10.1038/sj.ejhg.5201410
Salverda M. L. M., Dellus E., Gorter F. A., Debets A. J. M., van der Oost J., Hoekstra R. F., et al. (2011). Initial mutations direct alternative pathways of protein evolution. PLoS Genet. 7, e1001321. doi: 10.1371/journal.pgen.1001321
Sandberg T. E., Long C. P., Gonzalez J. E., Feist A. M., Antoniewicz M. R., Palsson B. O. (2016). Evolution of E. coli on [U-13C]Glucose reveals a negligible isotopic influence on metabolism and physiology. PLoS One 11, e0151130. doi: 10.1371/journal.pone.0151130
Sandberg T. E., Salazar M. J., Weng L. L., Palsson B. O., Feist A. M. (2019). The emergence of adaptive laboratory evolution as an efficient tool for biological discovery and industrial biotechnology. Metab. Eng. 56, 1–16. doi: 10.1016/j.ymben.2019.08.004
Sane M., Diwan G. D., Bhat B. A., Wahl L. M., Agashe D. (2023). Shifts in mutation spectra enhance access to beneficial mutations. Proc. Natl. Acad. Sci. U. S. A. 120, e2207355120. doi: 10.1073/pnas.2207355120
Saslis-Lagoudakis C. H., Savolainen V., Williamson E. M., Forest F., Wagstaff S. J., Baral S. R., et al. (2012). Phylogenies reveal predictive power of traditional medicine in bioprospecting. Proc. Natl. Acad. Sci. U. S. A. 109, 15835–15840. doi: 10.1073/pnas.1202242109
Schaaper R. M., Dunn R. L. (1987). Spectra of spontaneous mutations in Escherichia coli strains defective in mismatch correction: the nature of in vivo DNA replication errors. Proc. Natl. Acad. Sci. U. S. A. 84, 6220–6224. doi: 10.1073/pnas.84.17.6220
Schenk M. F., Zwart M. P., Hwang S., Ruelens P., Severing E., Krug J., et al. (2022). Population size mediates the contribution of high-rate and large-benefit mutations to parallel evolution. Nat. Ecol. Evol. 6, 439–447. doi: 10.1038/s41559-022-01669-3
Scribner M. R., Santos-Lopez A., Marshall C. W., Deitrick C., Cooper V. S. (2020). Parallel evolution of tobramycin resistance across species and environments. mBio 11. doi: 10.1128/mBio.00932-20
Sexton J. P., Hangartner S. B., Hoffmann A. A. (2014). Genetic isolation by environment or distance: which pattern of gene flow is most common? Evolution; Int. J. organic Evol. 68, 1–15. doi: 10.1111/evo.2014.68.issue-1
Shefferson R. P., Mason C. M., Kellett K. M., Goolsby E. W., Coughlin E., Flynn R. W. (2018). The evolutionary impacts of conservation actions. Population Ecol. 60, 49–59. doi: 10.1007/s10144-018-0614-9
Shendure J., Balasubramanian S., Church G. M., Gilbert W., Rogers J., Schloss J. A., et al. (2017). DNA sequencing at 40: past, present and future. Nature 550, 345–353. doi: 10.1038/nature24286
Shepherd M. J., Horton J. S., Taylor T. B. (2022). A near-deterministic mutational hotspot in Pseudomonas fluorescens is constructed by multiple interacting genomic features. Mol. Biol. Evol. 39. doi: 10.1093/molbev/msac132
Shreiner A. B., Kao J. Y., Young V. B. (2015). The gut microbiome in health and in disease. Curr. Opin. Gastroenterol. 31, 69–75. doi: 10.1097/MOG.0000000000000139
Simpson G. G. (2019). Principles of Animal Taxonomy. (New York, NY: Columbia University Press). doi: 10.7312/simp92414
Skinner M. K. (2015). Environmental epigenetics and a unified theory of the molecular aspects of evolution: A neo-lamarckian concept that facilitates neo-darwinian evolution. Genome Biol. Evol. 7, 1296–1302. doi: 10.1093/gbe/evv073
Smadja C. M., Butlin R. K. (2011). A framework for comparing processes of speciation in the presence of gene flow. Mol. Ecol. 20, 5123–5140. doi: 10.1111/j.1365-294X.2011.05350.x
Smith S. D., Rausher M. D. (2011). Gene loss and parallel evolution contribute to species difference in flower color. Mol. Biol. Evol. 28, 2799–2810. doi: 10.1093/molbev/msr109
Sniegowski P. D., Gerrish P. J., Johnson T., Shaver A. (2000). The evolution of mutation rates: separating causes from consequences. BioEssays: News Rev. molecular Cell. Dev. Biol. 22, 1057–1066. doi: 10.1002/1521-1878(200012)22:12<1057::AID-BIES3>3.0.CO;2-W
Spencer C. C., Tyerman J., Bertrand M., Doebeli M. (2008). Adaptation increases the likelihood of diversification in an experimental bacterial lineage. Proc. Natl. Acad. Sci. U. S. A. 105, 1585–1589. doi: 10.1073/pnas.0709632105
Sprouffske K., Aguilar-Rodríguez J., Sniegowski P., Wagner A. (2018). High mutation rates limit evolutionary adaptation in Escherichia coli. PLoS Genet. 14, e1007324. doi: 10.1371/journal.pgen.1007324
Sprouffske K., Merlo L. M. F., Gerrish P. J., Maley C. C., Sniegowski P. D. (2012). Cancer in light of experimental evolution. Curr. Biol. 22, R762–R771. doi: 10.1016/j.cub.2012.06.065
Starr T. N., Thornton J. W. (2016). Epistasis in protein evolution. Protein science: Publ. Protein Soc. 25, 1204–1218. doi: 10.1002/pro.2897
Steel M., Rodrigo A. (2008). Maximum likelihood supertrees. Systematic Biol. 57, 243–250. doi: 10.1080/10635150802033014
Steenackers H. P., Parijs I., Dubey A., Foster K. R., Vanderleyden J. (2016). Experimental evolution in biofilm populations. FEMS Microbiol. Rev. 40, 373–397. doi: 10.1093/femsre/fuw002
Stern D. L. (2013). The genetic causes of convergent evolution. Nat. Rev. Genet. 14, 751–764. doi: 10.1038/nrg3483
Stern D. L., Orgogozo V. (2008). The loci of evolution: how predictable is genetic evolution? Evolution; Int. J. organic Evol. 62, 2155–2177. doi: 10.1111/evo.2008.62.issue-9
Stillman R. A., Goss-Custard J. D. (2010). Individual-based ecology of coastal birds. Biol. Rev. Cambridge Philos. Soc. 85, 413–434. doi: 10.1111/j.1469-185X.2009.00106.x
Stoltzfus A., McCandlish D. M. (2017). Mutational biases influence parallel adaptation. Mol. Biol. Evol. 34, 2163–2172. doi: 10.1093/molbev/msx180
Stoltzfus A., Yampolsky L. Y. (2009). Climbing mount probable: mutation as a cause of nonrandomness in evolution. J. heredity 100, 637–647. doi: 10.1093/jhered/esp048
Storz J. F. (2016). Causes of molecular convergence and parallelism in protein evolution. Nat. Rev. Genet. 17, 239–250. doi: 10.1038/nrg.2016.11
Sung W., Ackerman M. S., Miller S. F., Doak T. G., Lynch M. (2012). Drift-barrier hypothesis and mutation-rate evolution. Proc. Natl. Acad. Sci. U. S. A. 109, 18488–18492. doi: 10.1073/pnas.1213613110
Svensson E. I., Berger D. (2019). The role of mutation bias in adaptive evolution. Trends Ecol. Evol. 34, 422–434. doi: 10.1016/j.tree.2019.01.015
Swallow J. G., Carter P. A., Garland T. Jr (1998). Artificial selection for increased wheel-running behavior in house mice. Behav. Genet. 28, 227–237. doi: 10.1023/A:1021479331779
Szendro I. G., Franke J., de Visser J. A. G. M., Krug J. (2013a). Predictability of evolution depends nonmonotonically on population size. Proc. Natl. Acad. Sci. U. S. A. 110, 571–576. doi: 10.1073/pnas.1213613110
Szendro I. G., Schenk M. F., Franke J., Krug J., de Visser J. A.G. M. (2013b). Quantitative analyses of empirical fitness landscapes. J. Stat. mechanics 2013, P01005. doi: 10.1088/1742-5468/2013/01/P01005
Takahata N. (1996). Neutral theory of molecular evolution. Curr. Opin. Genet. Dev. 6, 767–772. doi: 10.1016/S0959-437X(96)80034-7
Tataru P., Simonsen M., Bataillon T., Hobolth A. (2017). Statistical inference in the wright–fisher model using allele frequency data. Systematic Biol. 66(1), e30-e46. doi: 10.1093/sysbio/syw056
Tenaillon O., Rodríguez-Verdugo A., Gaut R. L., McDonald P., Bennett A. F., Long A. D., et al. (2012). The molecular diversity of adaptive convergence. Sci. (New York N.Y.) 335, 457–461. doi: 10.1126/science.1212986
Tenaillon O., Taddei F., Radmian M., Matic I. (2001). Second-order selection in bacterial evolution: selection acting on mutation and recombination rates in the course of adaptation. Res. Microbiol. 152, 11–16. doi: 10.1016/S0923-2508(00)01163-3
Teotónio H., Chelo I. M., Bradić M., Rose M. R., Long A. D. (2009). Experimental evolution reveals natural selection on standing genetic variation. Nat. Genet. 41, 251–257. doi: 10.1038/ng.289
Tilman D., Reich P. B., Knops J. M. H. (2006). Biodiversity and ecosystem stability in a decade-long grassland experiment. Nature 441, 629–632. doi: 10.1038/nature04742
Travis J., Reznick D., Bassar R. D., López-Sepulcre A., Ferriere R., Coulson T. (2014). “Chapter one - do eco-evo feedbacks help us understand nature?,” in Answers from Studies of the Trinidadian Guppy. Eds. Moya-Laraño J., Rowntree J., Woodward G. (Academic Press), 1–40. doi: 10.1016/B978-0-12-801374-8.00001-3
Tremblay R. L., Ackerman J. D. (2001). Gene flow and effective population size in Lepanthes (Orchidaceae): a case for genetic drift. Biol. J. Linn. Society. Linn. Soc. London 72, 47–62. doi: 10.1111/j.1095-8312.2001.tb01300.x
Tscharntke T., Tylianakis J. M., Rand T. A., Didham R. K., Fahrig L., Batáry P., et al. (2012). Landscape moderation of biodiversity patterns and processes - eight hypotheses. Biol. Rev. Cambridge Philos. Soc. 87, 661–685. doi: 10.1111/j.1469-185X.2011.00216.x
Tufts D. M., Natarajan C., Revsbech I. G., Projecto-Garcia J., Hoffmann F. G., Weber R. E., et al. (2015). Epistasis constrains mutational pathways of hemoglobin adaptation in high-altitude pikas. Mol. Biol. Evol. 32, 287–298. doi: 10.1093/molbev/msu311
Turner C. B., Marshall C. W., Cooper V. S. (2018). Parallel genetic adaptation across environments differing in mode of growth or resource availability. Evol. Lett. 2, 355–367. doi: 10.1002/evl3.75
Urban M. C., Bocedi G., Hendry A. P., Mihoub J.-B., Pe’er G., Singer A., et al. (2016). Improving the forecast for biodiversity under climate change. Sci. (New York N.Y.) 353. doi: 10.1126/science.aad8466
Vahdati A. R., Sprouffske K., Wagner A. (2017). Effect of population size and mutation rate on the evolution of RNA sequences on an adaptive landscape determined by RNA folding. Int. J. Biol. Sci. 13, 1138–1151. doi: 10.7150/ijbs.19436
Van Cleve J., Weissman D. B. (2015). Measuring ruggedness in fitness landscapes. Proc. Natl. Acad. Sci. U. S. A.. 112(24), 7345–7346. doi: 10.1073/pnas.1507916112
Van den Bergh B., Swings T., Fauvart M., Michiels J. (2018). Experimental design, population dynamics, and diversity in microbial experimental evolution. Microbiol. Mol. Biol. reviews: MMBR 82(3), 10–1128. doi: 10.1128/MMBR.00008-18
Vanderkooi O. G., Low D. E., Green K., Powis J. E., McGeer A., Toronto Invasive Bacterial Disease Network. (2005). Predicting antimicrobial resistance in invasive pneumococcal infections. Clin. Infect. diseases: an Off. Publ. Infect. Dis. Soc. America 40, 1288–1297. doi: 10.1086/429242
van Dijk T., Hwang S., Krug J., de Visser J. A.G., Zwart M. P. (2017). Mutation supply and the repeatability of selection for antibiotic resistance. Phys. Biol. 14, 055005. doi: 10.1088/1478-3975/aa7f36
van Dijk B., Meijer J., Cuypers T. D., Hogeweg P. (2019). Trusting the hand that feeds: microbes evolve to anticipate a serial transfer protocol as individuals or collectives. BMC evolutionary Biol. 19, 201. doi: 10.1186/s12862-019-1512-2
van Velzen E. (2020). Predator coexistence through emergent fitness equalization. Ecology 101, e02995. doi: 10.1002/ecy.2995
Venkataram S., Kryazhimskiy S. (2023). Evolutionary repeatability of emergent properties of ecological communities. Philos. Trans. R. Soc. London. Ser. B Biol. Sci. 378, 20220047. doi: 10.1098/rstb.2022.0047
Vinogradov A. E. (2004). Evolution of genome size: multilevel selection, mutation bias or dynamical chaos? Curr. Opin. Genet. Dev. 14, 620–626. doi: 10.1016/j.gde.2004.09.007
Vogelmann R., Amieva M. R. (2007). The role of bacterial pathogens in cancer. Curr. Opin. Microbiol. 10, 76–81. doi: 10.1016/j.mib.2006.12.004
Vogwill T., Kojadinovic M., Furió V., MacLean R. C. (2014). Testing the role of genetic background in parallel evolution using the comparative experimental evolution of antibiotic resistance. Mol. Biol. Evol. 31, 3314–3323. doi: 10.1093/molbev/msu262
Vogwill T., MacLean R. C. (2015). The genetic basis of the fitness costs of antimicrobial resistance: a meta-analysis approach. Evolutionary Appl. 8, 284–295. doi: 10.1111/eva.12202
Wakeley J. (1996). The excess of transitions among nucleotide substitutions: new methods of estimating transition bias underscore its significance. Trends Ecol. Evol. 11, 158–162. doi: 10.1016/0169-5347(96)10009-4
Walkup J., Dang C., Mau R. L., Hayer M., Schwartz E., Stone B. W., et al. (2023). The predictive power of phylogeny on growth rates in soil bacterial communities. ISME Commun. 3, 73. doi: 10.1038/s43705-023-00281-1
Walters R. J., Blanckenhorn W. U., Berger D. (2012). Forecasting extinction risk of ectotherms under climate warming: an evolutionary perspective. Funct. Ecol. 26, 1324–1338. doi: 10.1111/j.1365-2435.2012.02045.x
Wang Y., Xue P., Cao M., Yu T., Lane S. T., Zhao H. (2021). Directed evolution: Methodologies and applications. Chem. Rev. 121, 12384–12444. doi: 10.1021/acs.chemrev.1c00260
Wein T., Dagan T. (2019). The effect of population bottleneck size and selective regime on genetic diversity and evolvability in bacteria. Genome Biol. Evol. 11, 3283–3290. doi: 10.1093/gbe/evz243
Weinreich D. M. (2005). The rank ordering of genotypic fitness values predicts genetic constraint on natural selection on landscapes lacking sign epistasis. Genetics 171, 1397–1405. doi: 10.1534/genetics.104.036830
Weinreich D. M., Delaney N. F., Depristo M. A., Hartl D. L. (2006). Darwinian evolution can follow only very few mutational paths to fitter proteins. Sci. (New York N.Y.) 312, 111–114. doi: 10.1126/science.1123539
Willi Y., Kristensen T. N., Sgrò C. M., Weeks A. R., Ørsted M., Hoffmann A. A. (2022). Conservation genetics as a management tool: The five best-supported paradigms to assist the management of threatened species. Proc. Natl. Acad. Sci. U. S. A. 119, e2105076119. doi: 10.1073/pnas.2105076119
Witting L. (2003). Major life-history transitions by deterministic directional natural selection. J. Theor. Biol. 225, 389–406. doi: 10.1016/S0022-5193(03)00274-1
Wong A., Rodrigue N., Kassen R. (2012). Genomics of adaptation during experimental evolution of the opportunistic pathogen Pseudomonas aeruginosa. PLoS Genet. 8, e1002928. doi: 10.1371/journal.pgen.1002928
Wood T. E., Burke J. M., Rieseberg L. H. (2005). Parallel genotypic adaptation: when evolution repeats itself. Genetica 123, 157–170. doi: 10.1007/s10709-003-2738-9
Wortel M. T., Agashe D., Bailey S. F., Bank C., Bisschop K., Blankers T., et al. (2023). Towards evolutionary predictions: Current promises and challenges. Evolutionary Appl. 16, 3–21. doi: 10.1111/eva.13513
Wright S. (1963). “The roles of mutation, inbreeding, crossbreeding, and selection in evolution,” in Proceedings of the XI International Congress of Genetics (Pergamon Press, Oxford), 1963–1965.
Xu Q., Yang X., Yan Y., Wang S., Loreau M., Jiang L. (2021). Consistently positive effect of species diversity on ecosystem, but not population, temporal stability. Ecol. Lett. 24, 2256–2266. doi: 10.1111/ele.13777
Xue Z. P., Chindelevitch L., Guichard F. (2023). Supply-driven evolution: Mutation bias and trait-fitness distributions can drive macro-evolutionary dynamics. Front. Ecol. Evol. 10, 1048752. doi: 10.3389/fevo.2022.1048752
Yampolsky L. Y., Stoltzfus A. (2001). Bias in the introduction of variation as an orienting factor in evolution. Evol. Dev. 3, 73–83. doi: 10.1046/j.1525-142x.2001.003002073.x
Yang Q., Fowler M. S., Jackson A. L., Donohue I. (2019). The predictability of ecological stability in a noisy world. Nat. Ecol. Evol. 3, 251–259. doi: 10.1038/s41559-018-0794-x
Yang M.-R., Wu Y.-W. (2022). Enhancing predictions of antimicrobial resistance of pathogens by expanding the potential resistance gene repertoire using a pan-genome-based feature selection approach. BMC Bioinf. 23, 131. doi: 10.1186/s12859-022-04666-2
Yarmarkovich M., Warrington J. M., Farrel A., Maris J. M. (2020). Identification of SARS-CoV-2 vaccine epitopes predicted to induce long-term population-scale immunity. Cell Rep. Med. 1, 100036. doi: 10.1016/j.xcrm.2020.100036
Yeaman S. (2022). Evolution of polygenic traits under global vs local adaptation. Genetics 220. doi: 10.1093/genetics/iyab134
Yuan X., Miller D. J., Zhang J., Herrington D., Wang Y. (2012). An overview of population genetic data simulation. J. Comput. biology: J. Comput. Mol. Cell Biol. 19, 42–54. doi: 10.1089/cmb.2011.0186
Zella D., Gallo R. C. (2021). Viruses and bacteria associated with cancer: An overview. Viruses 13. doi: 10.3390/v13061039
Zeng Z. B., Cockerham C. C. (1993). Mutation models and quantitative genetic variation. Genetics 133, 729–736. doi: 10.1093/genetics/133.3.729
Zhang Z., Gerstein M. (2003). Patterns of nucleotide substitution, insertion and deletion in the human genome inferred from pseudogenes. Nucleic Acids Res. 31, 5338–5348. doi: 10.1093/nar/gkg745
Ziegler A., Leffell D. J., Kunala S., Sharma H. W., Gailani M., Simon J. A., et al. (1993). Mutation hotspots due to sunlight in the p53 gene of nonmelanoma skin cancers. Proc. Natl. Acad. Sci. U. S. A. 90, 4216–4220. doi: 10.1073/pnas.90.9.4216
Keywords: evolution, experimental evolution, repeatability, convergence, parallelism, antimicrobial resistance
Citation: Pearless SM and Freed NE (2024) Unravelling the factors of evolutionary repeatability: insights and perspectives on predictability in evolutionary biology. Front. Ecol. Evol. 12:1335452. doi: 10.3389/fevo.2024.1335452
Received: 08 November 2023; Accepted: 05 April 2024;
Published: 23 April 2024.
Edited by:
Yoh Iwasa, Kyushu University, JapanReviewed by:
James Horton, University of Bath, United KingdomCopyright © 2024 Pearless and Freed. This is an open-access article distributed under the terms of the Creative Commons Attribution License (CC BY). The use, distribution or reproduction in other forums is permitted, provided the original author(s) and the copyright owner(s) are credited and that the original publication in this journal is cited, in accordance with accepted academic practice. No use, distribution or reproduction is permitted which does not comply with these terms.
*Correspondence: Stella M. Pearless, c3BlYTc1N0BhdWNrbGFuZHVuaS5hYy5ueg==
Disclaimer: All claims expressed in this article are solely those of the authors and do not necessarily represent those of their affiliated organizations, or those of the publisher, the editors and the reviewers. Any product that may be evaluated in this article or claim that may be made by its manufacturer is not guaranteed or endorsed by the publisher.
Research integrity at Frontiers
Learn more about the work of our research integrity team to safeguard the quality of each article we publish.