- 1School of Agriculture and Natural Resources Sciences, Namibia University of Science and Technology, Windhoek, Namibia
- 2Cheetah Conservation Fund, Otjiwarongo, Namibia
- 3Evolutionary Ecology Group, Department of Biology, University of Antwerp, Antwerp, Belgium
- 4Zambeze Delta Conservation, Dallas, TX, United States
- 5Department of Conservation Ecology and Entomology, Stellenbosch University, Matieland, South Africa
Mesocarnivores fill important roles in ecological communities globally, but their distribution and abundance are often understudied. Many species have historically been regarded as vermin and subject to lethal control due to their role in livestock predation. Identifying the factors influencing mesocarnivore populations can help disentangle their relationship within ecological communities and inform conflict mitigation and conservation priorities. To help identify these factors, we used camera traps to study the community of medium and large mammals in four communal conservancies of northeastern Namibia covering the wet and dry seasons using 99 and 97 camera trap stations, respectively. We modelled black-backed jackal (Lupulella mesomelas) abundance using the robust Royle-Nichols model. Black-backed jackal were widespread, with a mean per site abundance of 2.01 (SD=0.66) in the wet season and 2.41 (SD=0.49) in the dry season. Black-backed jackal showed seasonally contrasting covariate associations, with lower abundance in areas with medium and large-sized wild prey during the wet season, and higher abundance in areas with more villages and close to African wild dog (Lycaon pictus) dens in the dry season. We identified localized hotspots of black-backed jackal abundance during the dry season, which may indicate that when resources are scarce, black-backed jackals rely on anthropogenic food sources despite an elevated risk for conflict, and on carcass remains from African wild dog kills. These findings highlight potential drivers of mesocarnivore abundance that would be obscured in a conventional occurrence modelling framework, and illustrate how local abundance may be influenced by seasonal variability, wild and anthropogenic food sources, and a likely facilitative relationship with a large carnivore. Further investigations in areas with more complex carnivore guilds and higher density of dominant predators are needed to understand black-backed jackal-African wild dog interactions and impacts on population dynamics.
1 Introduction
Mesocarnivores are a diverse group of small to midsized carnivores with body weight <15kg which inhabit a variety of biomes with variable extent of human footprint, from wilderness to urban and other human-dominated environments (Roemer et al., 2009; Ćirović et al., 2016). Many mesocarnivores have been subject to negative perceptions, viewed as vermin or pests and targeted by lethal control programs due to their propensity of conflicting with human interests (Berger, 2006; Roemer et al., 2009; Ćirović et al., 2016). However, mesocarnivores fill an important role in communities and perform ecosystem services such as predation on rodents that could be disease vectors and affect crops, animal waste removal, and seed dispersal (Ćirović et al., 2016; Nakashima and Do Linh San, 2022). Where large carnivores are functionally absent, mesocarnivores may partially replace the role of apex predators by controlling prey populations and inhibiting competitors (Prugh et al., 2009). Due to anthropogenic extinction and its disproportionate effects on large carnivores, many mesocarnivores experience reduced competitive pressures and subsequent increases in their abundance often inflict ecological, economic, and social costs globally (Roemer et al., 2009). Mesocarnivores have recently been identified as ideal sentinel species for monitoring the effects of ecosystem and global change, as the smallest carnivore in a system is proposed to be the most responsive to anthropogenic and environmental changes. The “middle-out ecology” paradigm emphasizes refocusing research efforts on smaller carnivores within systems (Jachowski et al., 2023; Marneweck et al., 2022; Do Linh San, 2024).
Black-backed jackal (Lupulella mesomelas; hereafter jackal) are a widespread mesocarnivore in Africa and categorized by the IUCN as a species of least concern (Hoffmann, 2014). They are opportunistic and thrive in a wide range of habitats including human-dominated environments (Klare et al., 2010; Hoffmann, 2014). Jackals are responsible for significant smallstock depredation across southern Africa (Kamler et al., 2012; Humphries et al., 2016; Drouilly et al., 2018; Verschueren et al., 2020). The cost of smallstock depredation in South Africa, which is primarily attributed to jackal and caracal (Caracal caracal), is estimated to amount to approximately US$126 million per year (Kerley et al., 2018). In a survey of communal conservancies and resettled farms of Namibia, where jackal were implicated for the majority of sheep and goat depredation, the cost of smallstock loss was calculated at US$1,800 per person per year (Rust and Marker, 2013). Consequently, jackal are highly persecuted, but predator control efforts are largely ineffective and may only decrease local numbers temporarily due to the species’ generalist and adaptable characteristics (Hoffmann, 2014). Determining drivers of jackal distribution and abundance in human-use areas is a crucial priority due to their significant financial impact on livestock farmers.
Previous studies in southern Africa have found opposing effects of season on jackal distribution, with their probability of occupying a site (i.e. occupancy) either higher in the dry season (Rich et al., 2017) or the wet season (Verschueren et al., 2021a). Similarly, while Masseloux et al. found a positive relationship between woody vegetation and occupancy in east Africa (2018), recently published studies from southern Africa have found higher jackal occupancy to be associated with open grassland or reduced cover (Verschueren et al., 2021a; Pardo et al., 2022). Occupancy of mesocarnivores including jackal was found to decrease in the presence of large predators in South Africa (Curveira-Santos et al., 2021). Jackal occupancy was found to be higher in agricultural areas compared to riparian and mountainous areas in Namibia (Fink et al., 2020) and has been positively associated with livestock abundance in Botswana (Van der Weyde et al., 2018) and Kenya (Masseloux et al., 2018), suggesting conflict potential (Schuette et al., 2013; Masseloux et al., 2018). Although present in human-dominated landscapes, jackal have been shown to avoid anthropogenic disturbance in both eastern and southern Africa, with low occupancy found near human settlements (Schuette et al., 2013; Masseloux et al., 2018) and far from protected areas (Van der Weyde et al., 2018).
While research on the factors influencing jackal occupancy has been comprehensive and shows regional variations, little information exists on the factors influencing abundance of jackals (Hernández-Sánchez et al., 2017). This is however key to developing effective conservation strategies, in particular in systems where large carnivores are depleted. In such systems it is predicted that mesocarnivores may be released from top-down regulatory forces, thereby shifting and/or exacerbating human-wildlife conflicts (Prugh et al., 2009). In much of southern Africa, jackals are reportedly the major carnivore responsible for livestock loss (Lines, 2008; Verschueren et al., 2020; Gusset et al., 2009; Avenant and Du Plessis, 2008). Our objective was to understand jackal distribution and abundance in a landscape with a simplified carnivore guild, in which jackals are the primary livestock predator, to provide insights into the ecology of human-impacted ecosystems and assist conflict mitigation priorities. Based on a hierarchical modeling approach using camera trapping data, we hypothesized that jackal abundance would be higher at sites with high visibility (a proxy for low horizontal cover) and reduced canopy cover (Verschueren et al., 2021a; Pardo et al., 2022) and at high occupancy of wild prey (Verschueren et al., 2021a) and livestock (Masseloux et al., 2018; Van der Weyde et al., 2018). We also hypothesized that jackal abundance would be negatively influenced by anthropogenic factors such as proximity to villages (Schuette et al., 2013; Masseloux et al., 2018), and where dominant predator presence was high (Curveira-Santos et al., 2019), as indexed by locations of African wild dog (Lycaon pictus; hereafter wild dog) dens. We expected these associations to be stronger in the dry season compared to the wet season due to resource scarcity in the dry season.
2 Methods
2.1 Study area
We conducted this study within northeastern Namibia in the Okakarara District Communal Area (ODCA), which forms part of the Greater Waterberg Landscape. The ODCA consists of four communal conservancies (Okakmatapati, Otjituo, Ozonahi, African wild dog) that span a total area of 18,951 km2. The study area for this research covered 6,720 km2 (Figure 1).
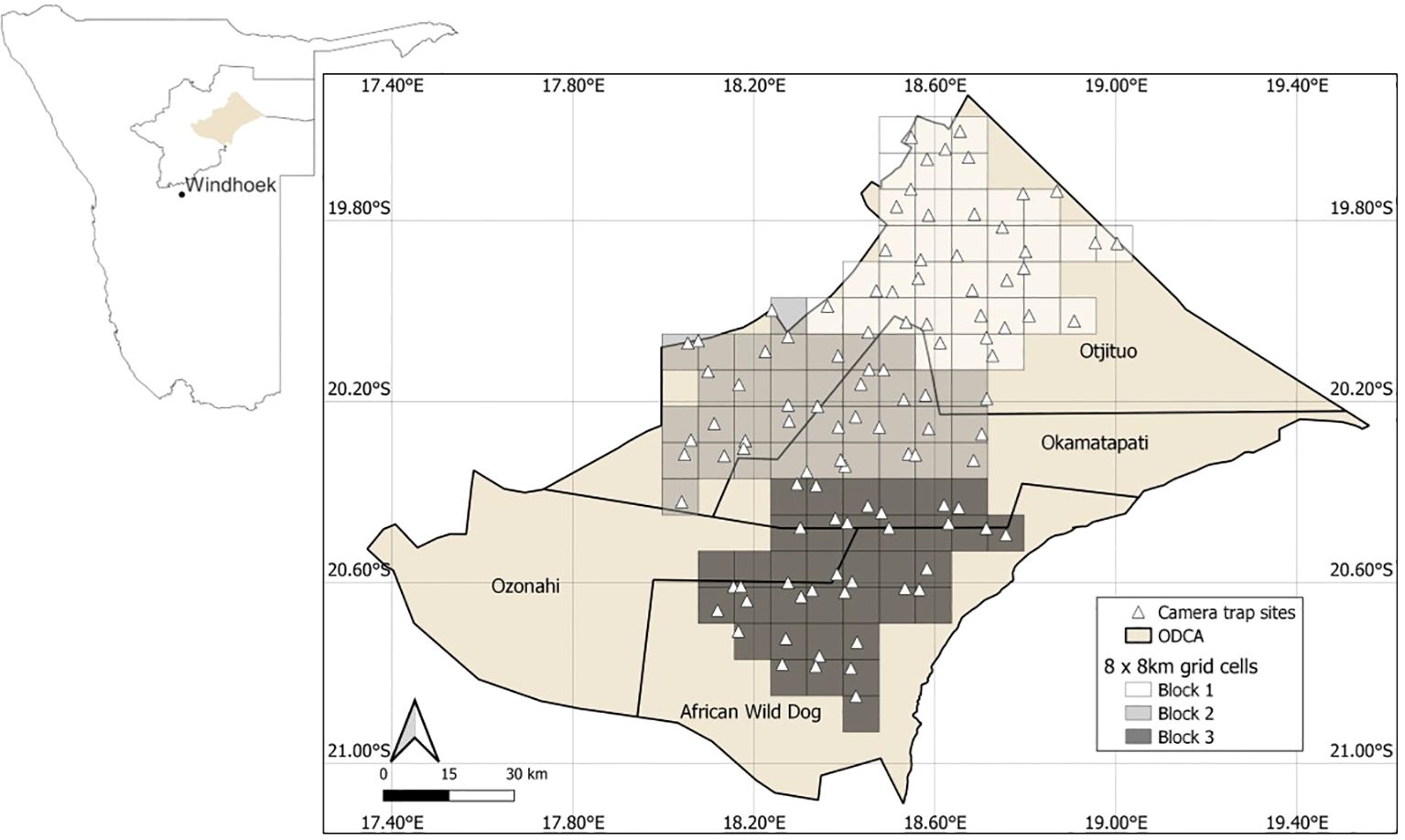
Figure 1. Grid and camera trap locations in north-eastern Namibia. The study area location is depicted within the Otjozondjupa region of Namibia (inset). One camera was deployed in each 8 km x 8 km grid cell.
The ODCA is situated on the western fringe of the Kalahari basin (Strohbach, 2014). The surface soils are dominated by sandy plains that are flat to rolling with 6-9° slopes (Strohbach, 2014). Vegetation type is primarily Terminalia sericea-Combretum collinum shrub and bushlands (Figure 2, Hüttich et al., 2009; Strohbach, 2014). The ODCA is characterized by two seasons: wet (November–April) and dry (May–October). The average temperature ranges from 6°C in the cool-dry season to 31°C in the hot-dry season (Mendelsohn and El Obeid, 2002). Annual rainfall averages from 400–450 mm, with droughts common (Mendelsohn and El Obeid, 2002; Strohbach, 2014).
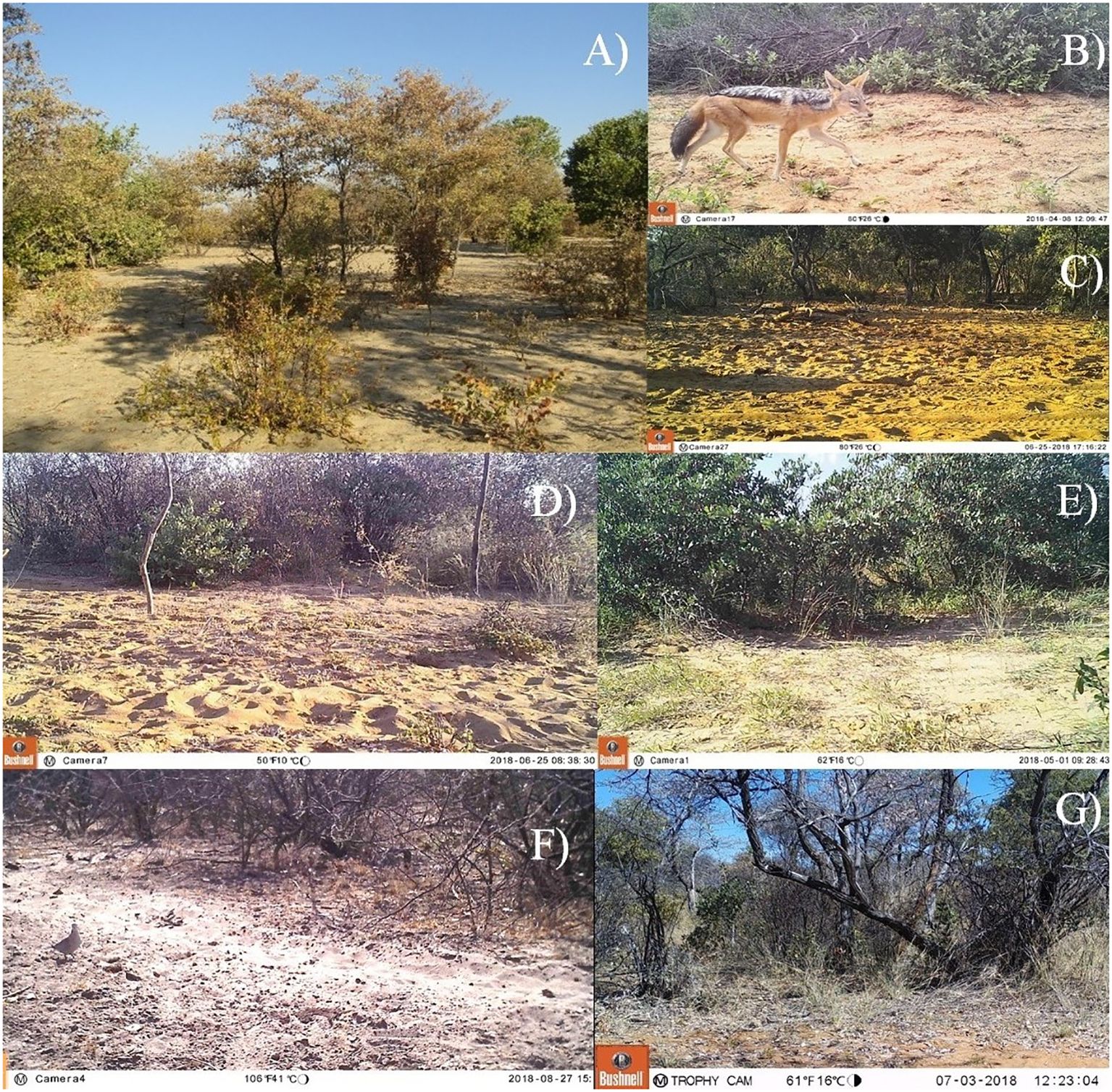
Figure 2. Typical landscape and vegetation of north-eastern Namibia (A), black-backed jackal (Lupullela mesomelas) camera trap image from the north-eastern Namibia study (B), camera trap image depicting the proximity feature road (C), cutline (D), fenceline (E), trail (F), and bush (G).
The ODCA is wildlife-depleted compared to other communal conservancies in Namibia and the surrounding freehold farmland, likely as a result of unsustainable and illegal hunting (Lines, 2008; Verschueren et al., 2020). Small antelope such as common duiker (Sylvicapra grimmia) and steenbok (Raphicerus campestris) persist, while greater kudu (Tragelaphus strepsiceros) and warthog (Phacochoerus africanusare) are the only large ungulates found in the ODCA and presumed to be rare (Lines, 2008). Little was known on the carnivore community prior to this study, but we assumed mesocarnivores were widespread, whereas large carnivores were likely sparsely distributed (Lines, 2008). The region is inhabited by a small population of critically endangered wild dog (Lycaon pictus; Hanssen et al., 2022). Through ongoing human-wildlife conflict work, the wild dog population in the ODCA has previously been estimated at approximately 15–20 individuals (CCF, unpublished data).
The human population in the ODCA is composed primarily of the Herero people, whose principal livelihood is livestock farming of cattle and smallstock (sheep and goats), with live cattle sales representing the primary form of income (Lines, 2008). Human settlements are concentrated primarily in the western and central regions of the ODCA, with less human impact to the East.
2.2 Data collection
In January 2018, we began an extensive camera trapping survey of the ODCA. We overlaid the study area using an 8 × 8 km square grid. From this grid, 105 grid cells were selected to sample across a broad range of habitats and human densities across the conservancies. We divided the selected 105 cells into three blocks, each block containing 35 cells, and blocks were sampled sequentially. The sequential sampling was implemented due to a limited number of staff and available camera traps, an approach commonly employed when resources are limited (Verschueren et al., 2021a; Phumanee et al., 2020; Rovero et al., 2020).
We deployed single camera traps (Bushnell Trophy Cam model 119676, Overland Park, Kansas, USA) in each cell of a given block which were active for 30 days in the wet season (November–April 2018-2019) and 30 days in the dry season (May–October 2018). The location of deployment within each grid cell was selected based on local knowledge with rural community involvement to increase the probability of wildlife detection (mean camera trap spacing 5.06 ± 2.17 km). We asked farmers or farm workers to identify areas of high wildlife movement, frequently targeting linear features such as roads or trails where wildlife were known to travel. The cameras were deployed at a height of approximately 60 cm. Additional information was collected at each camera trap site including canopy (vertical) cover, habitat visibility (horizontal cover), and proximity to linear feature. While our aim was to target the grid cell center for camera trap placement, this was rarely possibly due to limited accessibility and availability of linear features.
We measured canopy cover using a Bitterlich gauge (Friedel and Chewings, 1988) based on the cover estimation method of Zimmermann (2009) modified to Mberirua (2018). We set the Bitterlich gauge to a half angle of 12°55’ and pointed at all trees and shrubs over 0.5 m tall 360° around the camera at each site. We recorded when the canopy of a tree extended beyond the two sighting pins of the Bitterlich gauge. When canopies from multiple overlapping trees extended beyond the sighting pins, we recorded these as one canopy (Zimmermann, 2009). To obtain a relative indication of canopy cover, the number of recorded canopies was divided by the total number of trees and shrubs over 0.5 m at the site.
Habitat visibility was recorded using a range finder. The observer crouched at a height of 70cm, assumed to be eye level of larger carnivores. The distance at which an object was last visible was recorded for 4 directions (0°; 90°; 180°, and 270°) around the camera. These 4 values were averaged to obtain an average habitat visibility value for each camera trap site.
The distance from the camera trap to the nearest linear feature was recorded in meters. Site proximity features were classified into 4 categories: dirt road (n=43), cutline (an area cleared of vegetation typically for the purpose of creating a road; n=15), clear wildlife trail (n=12), and fenceline (n=12). Cameras with none of these features present were classified as bush (n=23). We classified cameras into one of the above feature categories when the feature was within the camera’s detection range of 20 m. When a camera was located at two of these features within the camera’s detection range (e.g. at the intersection of a fence and road), the camera was categorized based on the feature assumed to be most influential on wildlife movement. Roads were assumed to be most influential, followed by cutlines, wildlife trails, fencelines, and bush.
We manually classified images to species level following camera retrieval. Temporal independence was defined as when photos of the same species were separated by more than 30 minutes (Rich et al., 2017; Verschueren et al., 2021a). We calculated camera trapping nights by multiplying the number of nights a camera was active by the number of camera sites. While 105 cameras were deployed in both seasons, due to camera malfunctions 97 cameras were active throughout the dry season and 99 cameras were active in the wet season. The wet (November–April) and dry (May–October) season surveys were divided into three 10-day survey periods to construct detection histories for jackal.
2.3 Data analysis
2.3.1 Covariates
We tested three detection terms: canopy cover, average habitat visibility, and nearest linear feature (e.g. dirt road, fenceline). We also tested 10 abundance covariates: canopy cover, average habitat visibility, longitude, distance to human settlement, village density, common duiker intensity of use, steenbok intensity of use, ungulate intensity of use (which pools common duiker and steenbok data), scrub hare (Lepus saxatilis) intensity of use, and proximity to wild dog den (Table 1). It is common practice to include some covariates in both the abundance and detection components of a model, provided that there is biological reasoning to justify inclusion in both components (Denes et al., 2015; Sólymos et al., 2012). Prior to constructing seasonal candidate models, covariates were tested for correlation. Where two covariates were strongly correlated (|r| >0.7), we included in the candidate models for abundance the covariate with better goodness-of-fit and ΔAICc < ΔAICc of the null model when tested univariately.
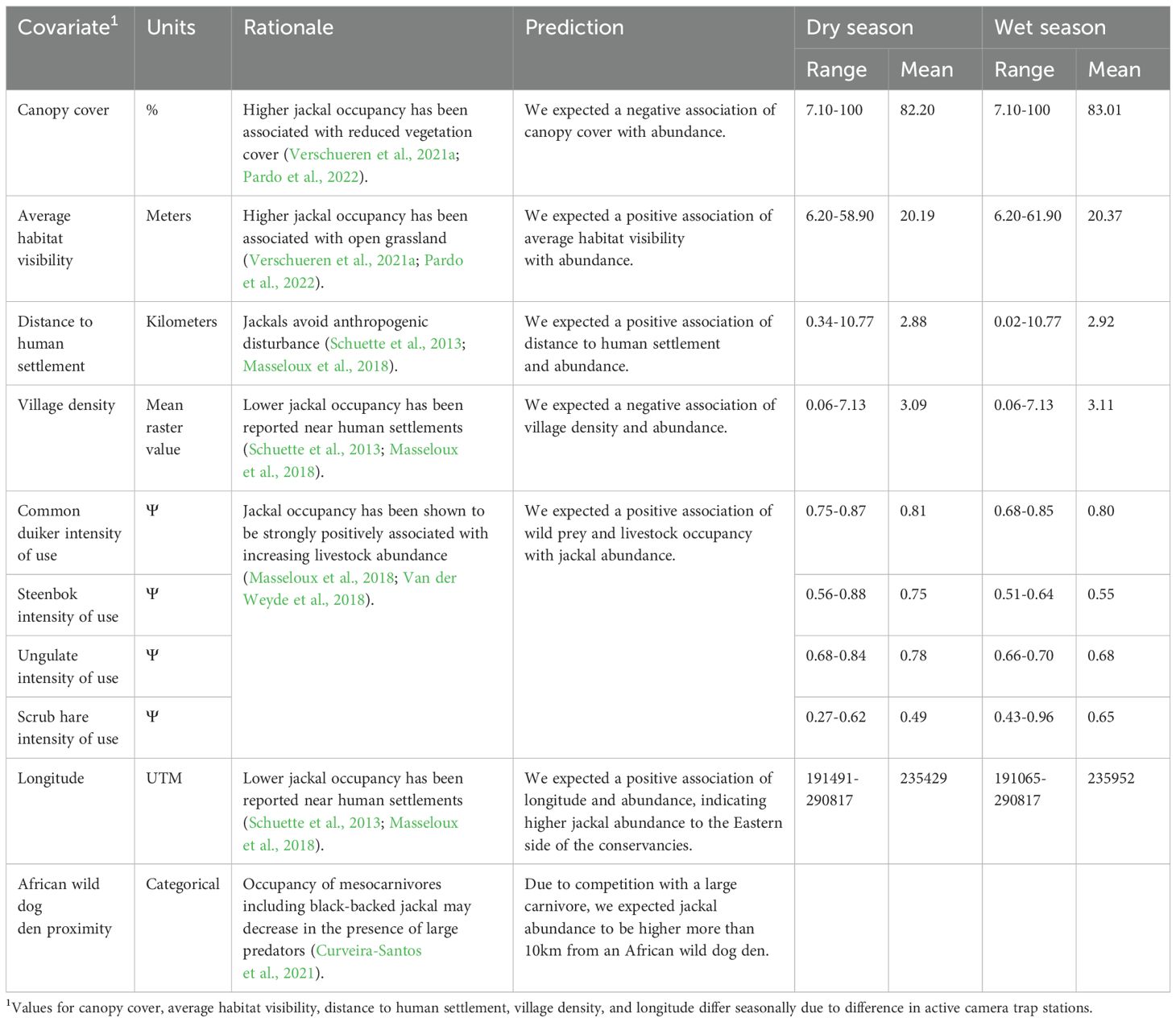
Table 1. Covariates included in the abundance models for black-backed jackal (Lupulella mesomelas) for dry and wet seasons.
While the study area is a semi-arid landscape and water is likely to influence jackal distribution, it was not possible to account for water sources due to the regular shifting of sources based on farmer and livestock needs as well as frequently bursting or leaking water pipes. Prey intensity of use values were calculated by occupancy modelling using function occu in R package unmarked for the medium and large-sized mammalian prey species which were determined to be within the prey range of jackal and which were detected at >10% of the camera trap sites (see section 2.3.4 Prey intensity of use modeling for further description). We opted to model intensity of use because the spacing of camera trap stations was larger than the presumed home ranges of prey species, thereby violating occupancy assumptions (MacKenzie et al., 2006). The prey intensity of use model outputs can therefore be interpreted as “site use” or the frequency or intensity with which a site is used rather than true occupancy (Suraci et al., 2021). We acknowledge that co-occurrence derived from observational data may be a poor proxy for ecological interactions among predators and prey due to various issues (e.g., insufficient information or sample size, incorrect sample scale, or habitat filtering and joint habitat preferences; Blanchet et al., 2020). Additionally, it is crucial to address the need to propagate uncertainty in ungulate occupancy estimates in jackal abundance models to avoid potential skewing of results and interpretation errors. Ultimately these complexities in predator-prey relationships make interpretations challenging, and while alternative methods exist to get closer to measuring these ecological interactions (Amir et al., 2022), such additional analysis is beyond the scope of this study.
We included the longitude covariate to account for potential greater human activity in the western part of the study area than in the East. Distance to village and village density were also included to represent human influence. The distance between each camera trap and the nearest human settlement was calculated in QGIS 2.24.1 using a point shapefile for the ODCA villages. Village density was also calculated using the village point shapefile. We used the shapefile to create a raster of village density with a kernel bandwidth of 10 km. We created buffers around the camera trap locations to represent the average home range size of jackal and used the zonal statistics tool in QGIS to calculate the mean raster value within each buffer. Jackal home range has been reported to vary from 1–34 km2 in southern Africa (Kamler et al., 2019) and was not available for the study area. We plotted scatterplots to compare the buffer sizes of the min, max, and mean within this 1–34 km2 range. The village density raster values were very similar between the tested buffer sizes. Therefore, we used the buffer size representing the mean of home range, 17.5 km2, as a covariate in the jackal abundance models.
We planned to incorporate wild dog occurrence as a covariate to explore the apex carnivore’s influence on jackal abundance, however only five wild dog detections were recorded, precluding occupancy or abundance modeling. Instead, we used locations of known wild dog dens to estimate den proximity as a categorical variable indicating whether a camera trap was within 10 km of an identified wild dog den. Wild dogs tend to den at sites located close to dens from previous years (Mbizah et al., 2014) and we obtained knowledge of dens identified during our ongoing human-wildlife conflict work in the ODCA (2017–2022). Although the home range of the wild dogs in the ODCA is unknown, the 10 km buffer size reflects approximate home range contraction during the denning season, when movement is restricted to proximity of the den (Darnell et al., 2014; Pomilia et al., 2015).
The continuous covariates (canopy cover, average habitat visibility, distance to village, and longitude, and all prey intensity of use values) were standardized to z-scores. We included quadratic terms for canopy cover and average habitat visibility to account for potential non-linear relationships.
2.3.2 Abundance modeling
Royle and Nichols (2003) developed a model within the occupancy modelling framework to estimate local abundance (λ), or the number of individuals of a target species using a given sampling site. The Royle- and Nichols’ abundance model is ideal for modelling detection data on widespread species with high naïve occupancy or when there is heterogeneity uncaptured by the model’s covariates (Dorazio, 2007; Royle, 2006; Royle and Nichols, 2003). In the Royle-Nichols formulation, detection probability is modeled as a function of encountering individuals rather than a species, hence detection probability is assumed to correlate positively with abundance (Tobler et al., 2015). In addition, the Royle-Nichols model is found to be more accurate in estimating relative population abundance than Poisson binomial N-mixture models that correct for imperfect detection using count data (Nakashima, 2019).
We modeled relative abundance of jackal with the function occuRN in R package unmarked (Royle and Nichols, 2003; Royle, 2006; Dorazio, 2007). The model considers that the population level probability of detection is a function of individual detection probability and the local abundance in the grid cell based on the following equation:
where p is the population level detection probability in cell i on occasion j and r is the individual-level probability of detection in cell i. According to this equation, cell-level detection probability increases with local abundance (Ni) (Royle and Nichols, 2003). The observed detection non-detection data (y) in cell i on occasion j are modelled as a Bernoulli trial:
where pij is the population level detection probability. In the local abundance (λ) model, the number of individuals exposed to sampling in a cell, Ni, are modelled as being drawn from a Poisson distribution:
where log(λi) is modelled as a function of site covariates.
Local abundance values are a function of the effective trapping area for target species and are not directly comparable between species (Efford and Dawson, 2012; Wearn et al., 2017). Therefore, it is important to note that local abundance is distinct from true abundance or density (individuals per unit area). Relative abundance is a more accurate term to describe this model’s output. Relative abundance values are spatially comparable and function as a measure of relative density changes across an area (Wearn et al., 2017). We acknowledge that as a result of the camera trap placement, our estimates of local abundance and detection probability rather reflect the area surrounding the camera trap site than the entire grid cell considered to guide camera trap placement.
2.3.3 Model selection
We modeled relative abundance of jackal in a two-step abundance-detection framework (MacKenzie et al., 2006; Paolino et al., 2018). Detection covariates were tested in the detection function first while holding abundance constant. The covariates from the best fitting detection function model were retained in the candidate models which tested covariates for abundance.
To assess model fit, we applied McKenzie and Bailey’s goodness-of-fit test to all supported models (ΔAICc <2) for each season using 1,000 simulations where a non-significant p-value >0.05 indicates sufficient model fit. We also estimated overdispersion using a variance inflation parameter (ĉ; Burnham and Anderson, 2002) as an indicator of lack-of-fit where ĉ >1 indicates overdispersion (O’Brien et al., 2020).
Supported abundance models were averaged (Oberosler et al., 2017; Zeller et al., 2017; Wevers et al., 2021) using function modavg from R package AICcmodavg to provide averaged parameter estimates and to plot relationships between jackal abundance and covariates with confidence intervals that did not overlap zero (Burnham and Anderson, 2002). To obtain abundance estimates for each camera trap site, we averaged models with ΔAICc <2 using function modavgPred (Burnham and Anderson, 2002).
2.3.4 Prey intensity of use modeling
Using R packages unmarked and AICcmodavg and function occu, we modelled intensity of use for the medium and large-sized mammalian prey species which were determined to be within the prey range of jackal and which were detected at >10% of the camera trap sites. We used a two-step modeling approach to generate detection histories and create models for common duiker, steenbok, scrub hare, and smallstock (i.e. sheep and goats).
Canopy cover, average habitat visibility, and proximity feature were included as detection covariates for detection model selection. Continuous detection covariates (canopy cover and average habitat visibility) were standardized to z-scores to have a mean of 0. Intensity of use covariates included were canopy cover, average habitat visibility, longitude, distance to village, and village density. Distance to village and village density were calculated using the same process as described for jackal abundance models. Prey species home range sizes were defined as follows: scrub hare (0.0825 km2, Wessels, 1978), common duiker (0.03–0.17 km2, Furstenburg, 2018), and steenbok (0.12–0.3 km2, Furstenburg, 2008). We followed the same buffer selection methodology used for jackal for each prey species (scrub hare = 0.0825 km2, common duiker = 0.1 km2, steenbok = 0.21 km2). For the smallstock covariate, we considered their “home range” to be the average distance from the camera trap locations to the nearest village (2.88 km), scaled to a hypothetical circular home range of an area of 26 km2.
All models with ΔAICc <2 were averaged (Oberosler et al., 2017; Zeller et al., 2017; Wevers et al., 2021) using function modavg from R package AICcmodavg to interpret relationships between intensity of use and covariates with confidence intervals that did not overlap zero (Burnham and Anderson, 2002). To obtain intensity of use estimates for each camera trap site, we averaged models with ΔAICc <2 using function modavgPred (Burnham and Anderson, 2002). For the ungulate intensity of use values per site, we averaged the per-site use values of common duiker and steenbok. To assess model fit, we computed McKenzie and Bailey’s goodness-of-fit tests and variance inflation parameters (č; Burnham and Anderson, 2002) for all supported models (see Supplementary Table 1).
The smallstock intensity of use models for the wet and dry seasons had evidence of lack of fit and overdispersion (see Supplementary Table 1). Cameras were deployed to maximize wildlife detections and not near villages, where smallstock are likely more abundant and potentially preyed upon more intensely (Verschueren et al., 2021b). Unlike cattle, which free-range day and night and were highly detected by camera traps, smallstock in the ODCA graze on rangelands during the day and are moved to protective enclosures in the villages at night (Verschueren et al., 2020). Their probability of detection was therefore lower than cattle and wildlife species, which may have influenced the low intensity of use estimate. We are cognizant of the limitations mentioned above and therefore dropped smallstock intensity of use as a covariate from the jackal abundance modeling.
3 Results
3.1 Dry season
We obtained 1,003 independent records of jackal over 2,910 camera trapping nights in the dry season. Naïve occupancy for jackal in the dry season was high (0.74) with a 0.35 probability of detection. There were six supported (ΔAICc <2) detection models (Supplementary Table 2). The covariates canopy cover, average habitat visibility, and proximity feature were included among these top-ranked models (Table 2). There were no significant covariates for detection, and the null model was highly ranked (ΔAICc <2) and only 0.2 units away from the top ranked model, which included average habitat visibility as a detection covariate. Therefore, no detection covariates were included in abundance model candidates.
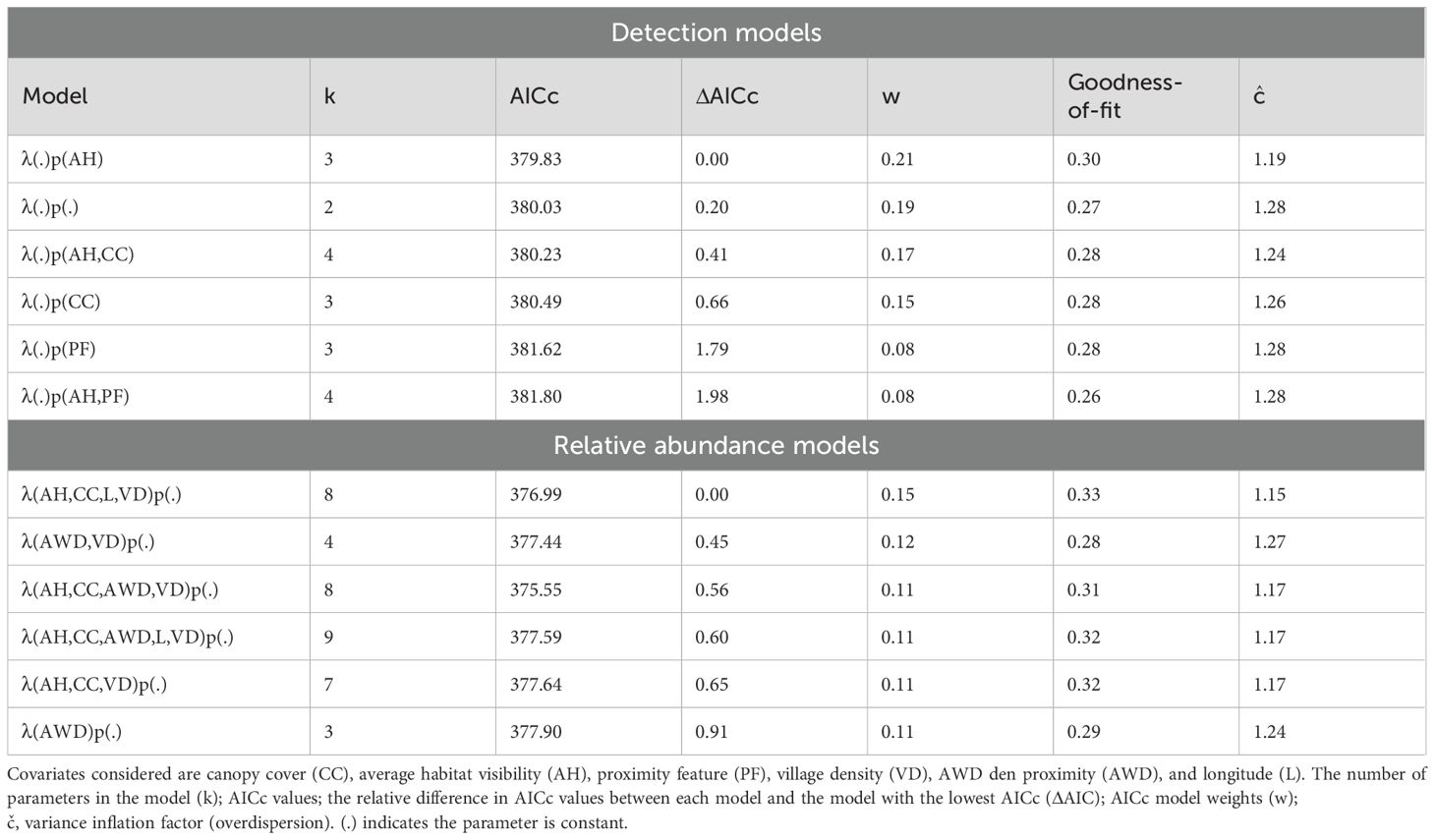
Table 2. Top-ranked (ΔAICc <2) models for factors influencing black-backed jackal (Lupulella mesomelas) site detectability (p) and relative abundance (λ) in the dry season.
There were six supported (ΔAICc <2) abundance models. The covariates average habitat visibility, canopy cover, wild dog den proximity, longitude, and village density were included among these top-ranked models (Table 2; Supplementary Table 4). Per-site relative abundance estimates ranged from 1.07–4.06 (x̄= 2.01, SD=0.66). Goodness-of-fit for the top abundance models ranged from 0.28–0.33, indicating no evidence of lack of fit. Variance inflation parameters (ĉ) ranged from 1.15–1.27 indicating slight overdispersion.
The averaged results from all supported abundance models showed that jackal abundance was significantly higher in areas of higher village density (β̂ = 0.23, SE = 0.12, Figure 3). Abundance was also significantly higher at camera traps within 10 km of a wild dog den (β̂ = 0.40, SE = 0.23, Figure 3).
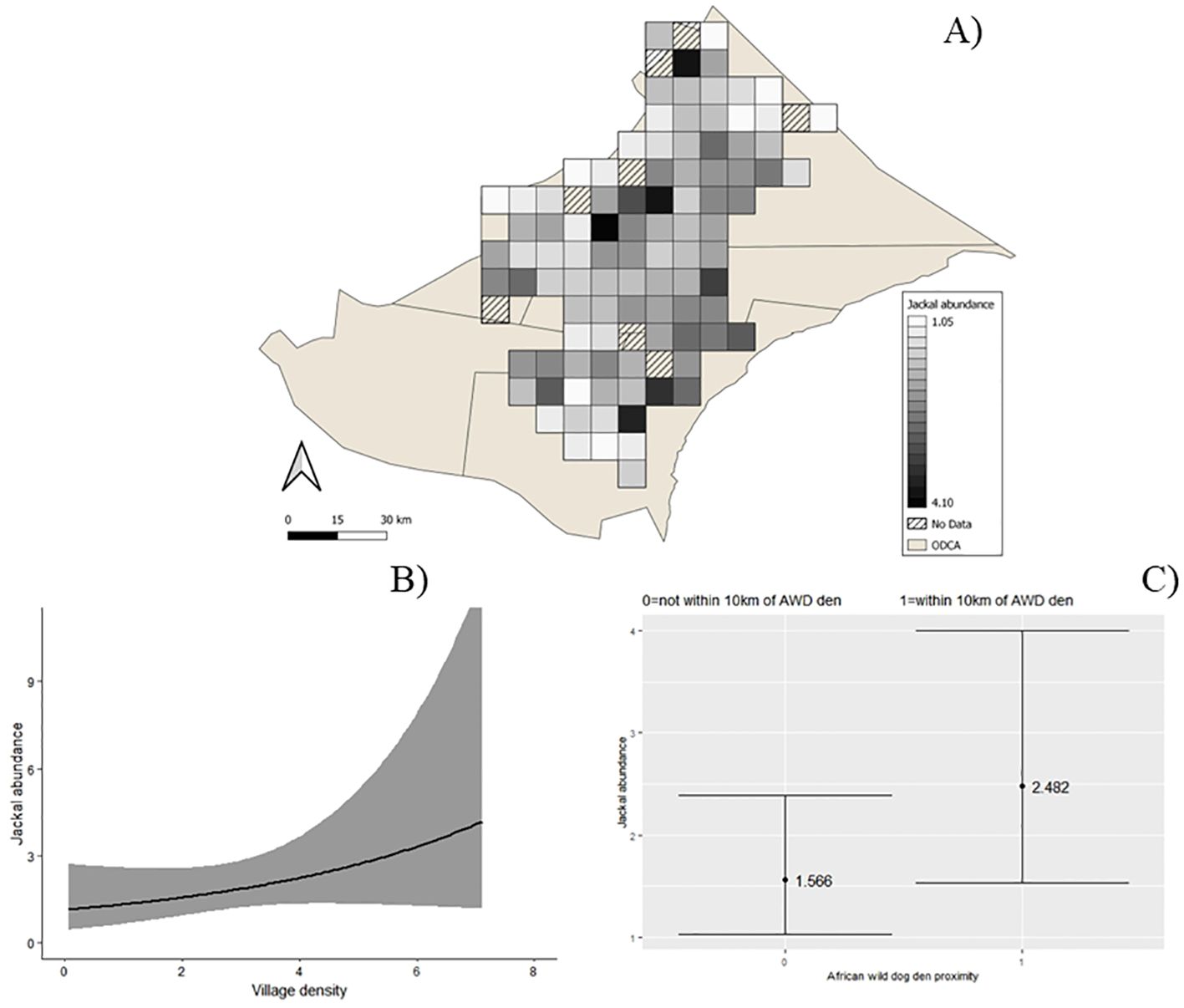
Figure 3. Black-backed jackal (Lupulella mesomelas) predicted dry season abundance in north-eastern Namibia (A), jackal abundance and village density in the dry season (B), jackal predicted abundance for cameras traps within 10 km of African wild dog (Lycaon pictus) dens in the dry season (C). Ribbons and error bars represent 95% confidence intervals. Supported models ΔAICc <2 averaged to plot relationships between covariates and abundance.
3.2 Wet season
We collected 592 independent records of jackal over 2,970 camera trapping nights in the wet season. Naïve occupancy for jackal in the wet season was also high (0.79). Detection probability was 0.31, but detectability was significantly higher with increasing average habitat visibility (β̂ = 0.32, SE = 0.16) and this model was >2 ΔAICc units away from the null model (Table 3; Supplementary Figure 1). Therefore, average habitat visibility was maintained as a covariate for the abundance model candidates.
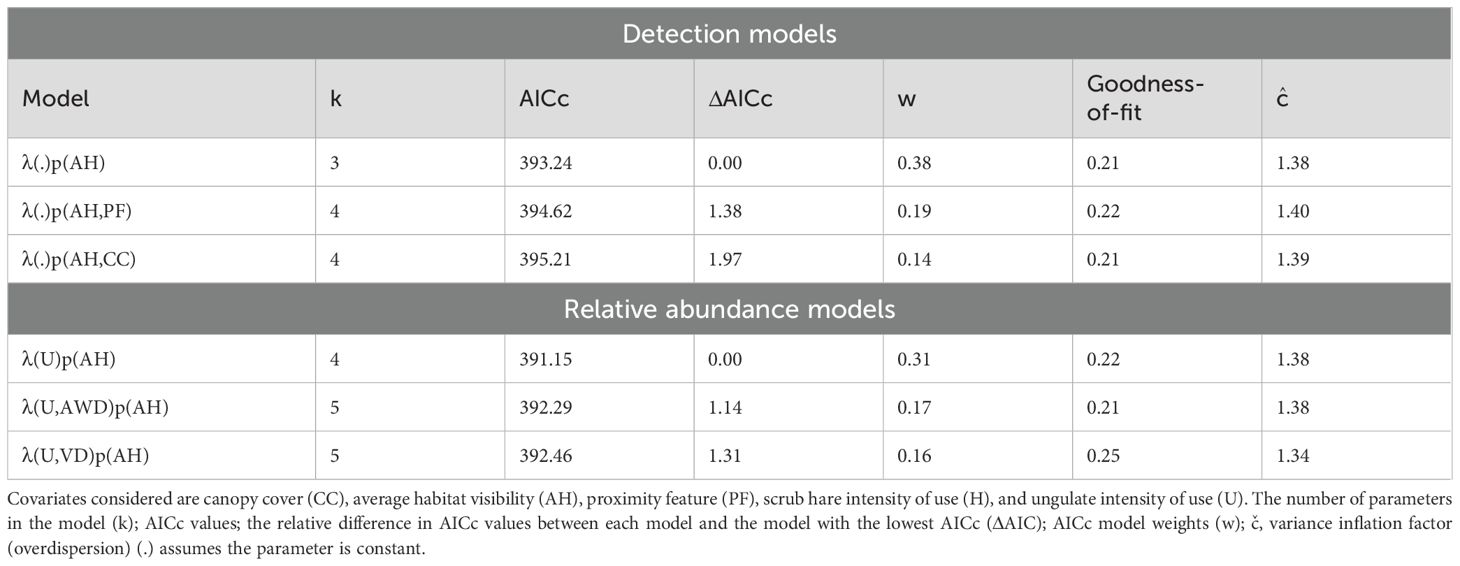
Table 3. Top-ranked (ΔAICc <2) models for factors influencing black-backed jackal (Lupulella mesomelas) site detectability (p) and relative abundance (λ) in the wet season.
There were three supported (ΔAICc <2) detection models (Supplementary Table 3). The covariates canopy cover, average habitat visibility, and proximity feature were included among these top-ranked models. There were three supported abundance models, which included the covariates wild dog den proximity, ungulate intensity of use, and village density (Table 3; Supplementary Table 5). Per-site abundance estimates ranged from 1.62–3.70 (x̄= 2.41, SD=0.49). Goodness-of-fit for the top abundance models ranged from 0.21 to 0.25, indicating no evidence of lack of fit. The variance inflation parameters (ĉ) ranged from 1.34 to 1.38, indicating slight overdispersion. The averaged results from the supported abundance models showed that jackal abundance was significantly higher with decreasing ungulate intensity of use (β̂ = -19.59, SE = 9.67, Figure 4).
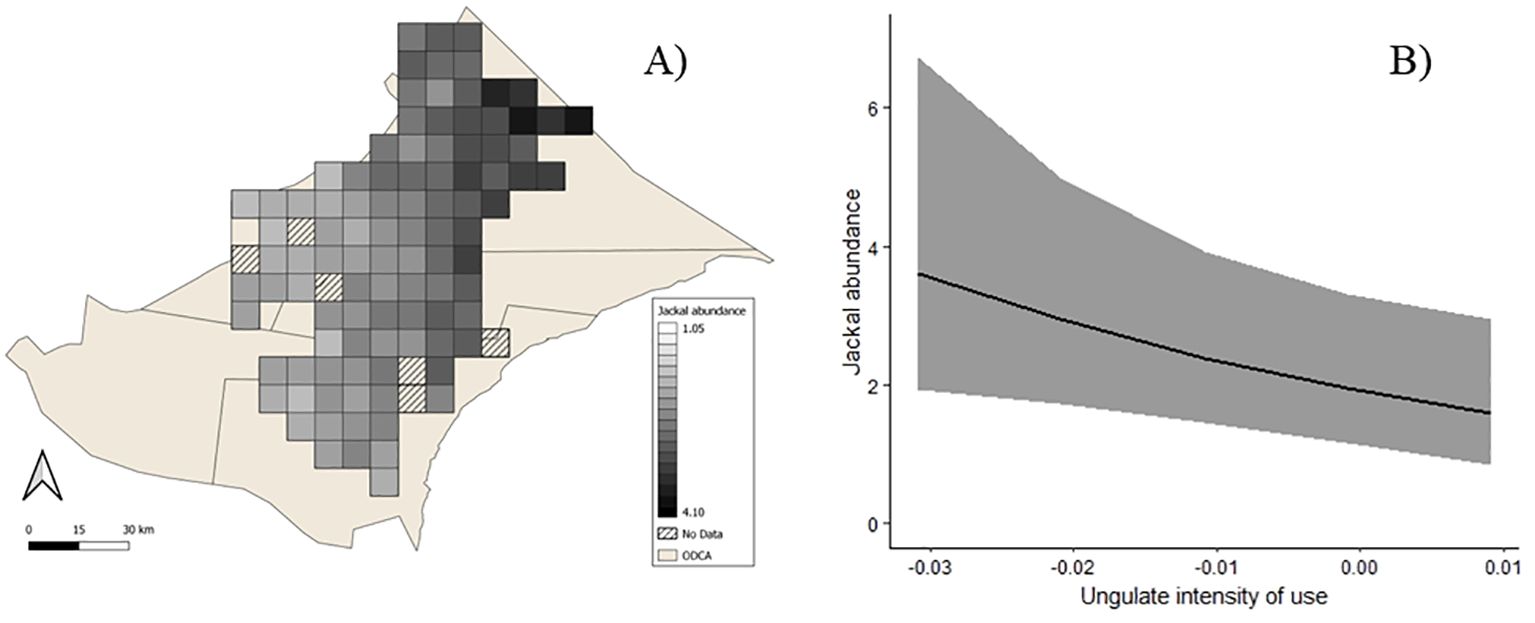
Figure 4. Black-backed jackal (Lupulella mesomelas) predicted wet season abundance in north-eastern Namibia (A), jackal abundance and ungulate intensity of use in the wet season (B). Ribbon envelops 95% confidence intervals. Supported models ΔAICc <2 averaged to plot relationship between ungulate intensity of use and abundance.
4 Discussion
Jackals are a wide-ranging mesocarnivore in the ODCA, as indicated by the high naïve occupancy. Detection of jackal increased with higher habitat visibility in the wet season, likely due to vegetation growth which reduced visibility at camera sites in the wet season (Nghikembua et al., 2016). Despite seasonal differences in capture frequencies, estimates of jackal relative abundance and occurrence were similar between seasons. This demonstrates the robustness of our modeling approach and emphasizes that capture frequencies may not reflect true abundance. This finding also underscores the importance of using appropriate modeling techniques to gain a more accurate understanding of wildlife populations, especially in environments where seasonal variations can affect detection rates.
Despite the widespread occurrence of jackals within the ODCA, we identified seasonally contrasting covariate associations, with localized hotspots of jackal relative abundance during the dry season. Jackal abundance was higher in the dry season in areas of higher village density. While typically avoiding human settlements, some studies have found jackal occupancy to be higher in human-modified areas (Fink et al., 2020) and positively associated with livestock capture frequency (Van der Weyde et al., 2018). Areas closer to villages may represent increased food resources for jackal in the form of livestock and potentially human refuse (Kaunda and Skinner, 2003; Loveridge and Macdonald, 2003), which may be exploited when other resources are low (Lewis et al., 1993; Jaeger et al., 2007). In a diet study of jackal we completed in the same study area, one jackal scat sample collected in the dry season contained plastic, potential evidence of human refuse consumption (Reasoner, 2023). Although carnivores often avoid large human settlements (Nellemann et al., 2007; Schuette et al., 2013; Masseloux et al., 2018), homesteads in the ODCA are relatively small and may bring foraging opportunities for jackal, reflecting in increased jackal abundance in village-dense areas during the dry season.
Mesocarnivores can interact with apex predators through competition, where the apex predator prevents access to food resources, or facilitation, where mesocarnivores benefit from scavenging on prey sourced from the larger carnivore (Codron et al., 2018). In North America, grey wolves are the dominant canid over coyotes, suppressing the coyote population and frequently killing them (Berger and Gese, 2007; Merkle et al., 2009). However, grey wolves also act as facilitators to coyotes by providing scavenging opportunities (Wilmers et al., 2003). In the ODCA system, jackal were more abundant closer to wild dog dens (and significantly more so during the dry season) which is possibly facilitated by wild dogs hunting near their dens and thus providing increased scavenging opportunities for jackal, particularly during periods of resource scarcity (Verschueren et al., 2021a). However, alternative theories could likewise explain this response, for example, jackal may be more abundant within this buffer because den sites are selected by wild dogs based on risk avoidance factors (Jackson et al., 2014) including dense vegetation (Davies-Mostert et al., 2013; Alting et al., 2021), rugged terrain (Davies-Mostert et al., 2013; O’Neill et al., 2020; Alting et al., 2021), and concealment (Van der Meer et al., 2014). Nonetheless, we recommend further investigation to elucidate the relationship between jackal abundance and wild dog den proximity.
Reduced food availability in the dry season may cause jackals to rely more on livestock and other food resources of human origin in more village-dense areas, as well as possibly scavenging from wild dog kills (Verschueren et al., 2021a). Localized hotspots of jackal abundance may intensify conflict with humans, while during the wet season, jackal abundance was more homogeneously distributed and not significantly constrained by village density or proximity to wild dog dens. Jackal diet is known to vary seasonally depending on resource availability (Kaunda and Skinner, 2003; Klare et al., 2010; Kamler et al., 2012; Van de Ven et al., 2013). In the wet season, jackals in the ODCA may capitalize on alternative food sources such as small mammals and berries (Moehlman, 1987; Kamler et al., 2012). Due to the abundance of these resources in the wet season, other food items such as livestock, human refuse, and scavenging from wild dog kills may become less important food sources during this time of year.
Jackal abundance increased with decreased ungulate intensity of use in the wet season. However, the common duiker intensity of use model had evidence of lack of fit and overdispersion, and was combined with the steenbok model to obtain the ungulate intensity of use estimates. Duiker are widespread across the study area (Lines, 2008) and were detected more frequently and at a higher number of camera traps than other prey species, which may have influenced the model fit issues. To yield more accurate intensity of use estimates, we thus suggest future studies employ alternative approaches for modeling duiker in this region which consider their widespread nature.
As we were unable to include smallstock intensity of use as a covariate due to significant model fit issues, we suggest future modeling approaches should better represent the higher abundance of smallstock near villages. Village density had a significant positive association with jackal abundance in the dry season, and may be a better indicator of smallstock availability and its influence on jackal abundance. Previous research in the study system found that jackals were perceived responsible for 73% of smallstock losses (Verschueren et al., 2020), financially devastating to communal farmers in the ODCA, who are less able than freehold farmers to withstand economic losses (Rust and Marker, 2013; Shilongo et al., 2018). The authors’ recent diet study completed in the same study area, which analyzed the contents of genetically confirmed jackal scats, found that smallstock species contributed substantially to the total biomass in the jackals’ diet (Reasoner, 2023). Although scat analysis does not allow for determination of if prey was hunted and killed or scavenged, these findings nonetheless suggest the pervasiveness of smallstock depredation in this system. Livestock-carnivore conflict may be widespread in part due to the absence of wild prey (Rasmussen, 1999; Woodroffe et al., 2005; Gusset et al., 2009). Restoring wild prey populations is a priority for the ODCA and areas with similar socio-ecological context. This will be a significant challenge due to the depletion and degradation of such landscapes, often worsened by climate change. Wild prey such as large ungulates, which once existed in large numbers, have been continually hunted by the community, leading in part to the unbalanced ecosystem that exists today (Lines, 2008; Verschueren et al., 2020). Not only does the region now lack browsing herbivores, including megaherbivores, which may reduce bush encroachment, it is also further degraded by overgrazing of livestock (O'Connor et al., 2014; Strohbach, 2014) and increasingly common droughts (Schwieger, 2022).
This study suggests that jackal and wild dog may have a limited competitive relationship in the ODCA. Since wild dogs exist at low densities in this system, it is plausible that reduced top-down suppression could positively influence jackal populations and exploitation of food resources, including livestock (Reasoner, 2023). This ecosystem imbalance may have severe consequences on vulnerable prey species and increase human-wildlife conflict due to mesocarnivores’ tendency to thrive in human-dense environments (Ritchie and Johnson, 2009; Krofel et al., 2017; Prugh et al., 2009), while large carnivores face disproportionate challenges in human-altered landscapes due to their large space requirements, low tolerance by humans, and sensitivity to persecution (Prugh et al., 2009). Jackal abundance in the ODCA is likely also facilitated by access to trophic subsidies in the form of food resources of human origin (Manlick and Pauli, 2020; Petroelje et al., 2019). Similar to those observed in generalist omnivores in other systems (Luskin et al., 2017), this may create a landscape-scale cross-boundary cascade as populations of small mammals in areas distant from villages could increase as a result of jackals’ adaptability to food sources near villages.
In financially disadvantaged communities like the ODCA, addressing livestock losses, predator persecution, and cascading ecosystem effects of the latter requires a dual approach involving both short-term and long-term strategies. Improving livestock management and implementing measures to mitigate human-carnivore conflict are essential in the short-term. This could be achieved through integrated workshops within the farmer communities, while providing tangible benefits of wildlife co-occurrence such as through wildlife credit schemes (Espinosa and Jacobson, 2011; Dinerstein et al., 2013). As human refuse appears to be an attractant for jackal in this community, improved waste management may also reduce conflict (Poessel et al., 2017). In the long-term, restoring natural prey populations and re-establishing large carnivore populations are critical. Ensuring the availability of wild prey, coupled with intraguild competitive interactions, may help maintain balanced wildlife populations (Ripple et al., 2014; Khorozyan et al., 2015). Comprehensive land management strategies and livelihood-focused interventions can further alleviate pressures on local ecosystems (Wright et al., 2016). These combined efforts will promote ecological stability while supporting the coexistence of wildlife and human communities, and encouraging community-led initiatives will be key to achieving sustainable outcomes.
Data availability statement
The raw data supporting the conclusions of this article will be made available by the authors, without undue reservation.
Ethics statement
Ethical approval was not required for the study involving animals in accordance with the local legislation and institutional requirements because the study was non-invasive (camera trapping) and did not require ethical approval.
Author contributions
ER: Conceptualization, Formal analysis, Investigation, Writing – original draft. LM: Project administration, Supervision, Writing – review & editing. SV: Investigation, Methodology, Writing – review & editing. WB: Investigation, Writing – review & editing. MM: Supervision, Writing – review & editing. BC: Conceptualization, Methodology, Supervision, Writing – review & editing.
Funding
The author(s) declare financial support was received for the research, authorship, and/or publication of this article. Cheetah Conservation Fund (CCF) received funding for this research through the Go Green Fund, initiated by Nedbank Namibia and Namibia Nature Foundation. ER received a grant-in-aid of research from Sigma Xi Scientific Honor Society (G2022315-2283.
Acknowledgments
We thank the communal conservancies and farmers of the ODCA for working with us to collect data on their farmlands. We are grateful to all Cheetah Conservation Fund staff and interns who contributed to the data collection efforts which made this research possible.
Conflict of interest
The authors declare that the research was conducted in the absence of any commercial or financial relationships that could be construed as a potential conflict of interest.
Publisher’s note
All claims expressed in this article are solely those of the authors and do not necessarily represent those of their affiliated organizations, or those of the publisher, the editors and the reviewers. Any product that may be evaluated in this article, or claim that may be made by its manufacturer, is not guaranteed or endorsed by the publisher.
Supplementary material
The Supplementary Material for this article can be found online at: https://www.frontiersin.org/articles/10.3389/fevo.2024.1333162/full#supplementary-material
References
Alting B. F., Bennitt E., Golabek K. A., Pitcher B. J., McNutt J. W., Wilson A. M., et al. (2021). The characteristics and consequences of African wild dog (Lycaon pictus) den site selection. Behav. Ecol. Sociobiology. 75, 1–14. doi: 10.1007/s00265-021-03047-8
Amir Z., Sovie A., Luskin M. S. (2022). Inferring predator-prey interactions from camera traps: A Bayesian co-abundance modeling approach. Ecol. Evolution. 12, e9627. doi: 10.1002/ece3.9627
Avenant N. L., Du Plessis J. J. (2008). Sustainable small stock farming and ecosystem conservation in southern Africa: A role for small mammals? Mammalia 72, 258–263. doi: 10.1515/MAMM.2008.041
Berger K. M. (2006). Carnivore-livestock conflicts: effects of subsidized predator control and economic correlates on the sheep industry. Conserv. Biol. 20, 751–761. doi: 10.1111/j.1523-1739.2006.00336.x
Berger K. M., Gese E. M. (2007). Does interference competition with wolves limit the distribution and abundance of coyotes? J. Anim. Ecology. 76, 1075–1085. doi: 10.1111/j.1365-2656.2007.01287.x
Blanchet F. G., Cazelles K., Gravel D. (2020). Co-occurrence is not evidence of ecological interactions. Ecol. Letters. 23, 1050–1063. doi: 10.1111/ele.13525
Burnham K. P., Anderson D. R. (2002). Model selection and multimodal inference: a practical information theoretic approach (New York: Springer).
Ćirović D., Penezić A., Krofel M. (2016). Jackals as cleaners: Ecosystem services provided by a mesocarnivore in human-dominated landscapes. Biol. Conserv. 199, 51–55. doi: 10.1016/j.biocon.2016.04.027
Codron D., Radloff F. G., Codron J., Kerley G. I., Tambling C. J. (2018). Meso-carnivore niche expansion in response to an apex predator’s reintroduction–a stable isotope approach. Afr. J. Wildlife Res. 48, 1–16. doi: 10.3957/056.048.013004
Curveira-Santos G., Sutherland C., Tenan S., Fernández-Chacón A., Mann G. K., Pitman R. T., et al. (2021). Mesocarnivore community structuring in the presence of Africa's apex predator. Proc. Roy. Soc. London, Ser. B, Biol. Sci. 288, 20202379. doi: 10.1098/rspb.2020.2379
Curveira-Santos G., Pedroso N. M., Barros A. L., Santos-Reis M. (2019). Mesocarnivore community structure under predator control: Unintended patterns in a conservation context. PloS One 14 (1), e0210661. doi: 10.1371/journal.pone.0210661
Darnell A. M., Graf J. A., Somers M. J., Slotow R., Szykman Gunther M. (2014). Space use of African wild dogs in relation to other large carnivores. PLoS One 9, e98846. doi: 10.1371/journal.pone.0098846
Davies-Mostert H. T., Mills M. G. L., Macdonald D. W. (2013). Hard boundaries influence African wild dogs’ diet and prey selection. J. Appl. Ecology. 50, 1358–1366. doi: 10.1111/1365-2664.12129
Denes F. V., Silveira L. F., Beissinger S. R. (2015). Estimating abundance of unmarked animal populations: accounting for imperfect detection and other sources of zero inflation. Methods Ecol. Evol. 6, 543–556. doi: 10.1111/2041-210X.12333
Dinerstein E., Varma K., Wikramanayake E., Powell G., Lumpkin S., Naidoo R., et al. (2013). Enhancing conservation, ecosystem services, and local livelihoods through a wildlife premium mechanism. Conserv. Biol. 27, 14–23. doi: 10.1111/j.1523-1739.2012.01959.x
Do Linh San E. (2024). Time for a paradigm shift? Small carnivores’ sensitivity highlights the importance of monitoring mid-rank predators in future global change studies. J. Anim. Ecol. 93, 126–13. doi: 10.1111/1365-2656.14047
Dorazio R. M. (2007). On the choice of statistical models for estimating occurance and extinction from animal surveys. Ecology 88, 2773–2782. doi: 10.1890/07-0006.1
Drouilly M., Nattrass N., O'riain M. J. (2018). Dietary niche relationships among predators on farmland and a protected area. J. Wildl. Manag. 82 (3), 507–518. doi: 10.1002/jwmg.21407
Efford M. G., Dawson D. K. (2012). Occupancy in continuous habitat. Ecosphere 3, 1–15. doi: 10.1890/ES11-00308.1
Espinosa S., Jacobson S. K. (2011). Human-wildlife conflict and environmental education: Evaluating a community program to protect the Andean bear in Ecuador. J. Environ. Educ. 43, 55–65. doi: 10.1080/00958964.2011.579642
Fink S., Chandler R., Chamberlain M., Castleberry S., Glosenger-Thrasher S. (2020). Distribution and activity patterns of large carnivores and their implications for human–carnivore conflict management in Namibia. Human–Wildlife Interactions. 14, 287–295. doi: 10.26077/658e-e8a8
Friedel M. H., Chewings V. H. (1988). Comparison of crown cover estimates for woody vegetation in arid rangelands. Aust. J. Ecology. 13, 463–468. doi: 10.1111/j.1442-9993.1988.tb00994.x
Gusset M., Swarner M. J., Mponwane L., Keletile K., McNutt J. W. (2009). Human–wildlife conflict in northern Botswana: livestock predation by endangered African wild dog Lycaon pictus and other carnivores. Oryx 43, 67–72. doi: 10.1017/S0030605308990475
Hanssen L., Lines R., Thomson G., Marker L., LeRoux N., Pfeiffer L. (2022). “A conservation assessment of African Wild Dog Lycaon pictus,” in Conservation Status and Red List of the Terrestrial Carnivores of Namibia (MEFT, LCMAN & NCE, Windhoek, Namibia), 83–91. eds. NCE, LCMAN, MEFT.
Hernández-Sánchez A., Santos-Moreno A., Pérez-Irineo G. (2017). Abundance of mesocarnivores in two vegetation types in the southeastern region of Mexico. Southwestern Naturalist. 62, 101–108. doi: 10.1894/0038-4909-62.2.101
Hoffmann M. (2014). Canis mesomelas. IUCN Red List Threatened Species 2014, e.T3755A46122476. doi: 10.2305/IUCN.UK.2014-1.RLTS.T3755A46122476.en
Humphries B. D., Ramesh T., Downs C. T. (2016). Diet of black-backed jackals (Canis mesomelas) on farmlands in the KwaZulu-Natal Midlands, South Africa. Mammalia 80, 405–412. doi: 10.1515/mammalia-2014-0103
Hüttich C., Gessner U., Herold M., Strohbach B. J., Schmidt M., Keil M., et al. (2009). On the suitability of MODIS time series metrics to map vegetation types in dry savanna ecosystems: A case study in the Kalahari of NE Namibia. Remote Sensing. 1, 620–643. doi: 10.3390/rs1040620
Jachowski D. S., Marneweck C. J., Olfenbuttel C., Harris S. N. (2023). Support for the size-mediated sensitivity hypothesis within a diverse carnivore community. J. Anim. Ecology. 93, 109–122. doi: 10.1111/1365-2656.13916
Jackson C. R., Power R. J., Groom R. J., Masenga E. H., Mjingo E. E., Fyumagwa R. D., et al. (2014). Heading for the hills: risk avoidance drives den site selection in African wild dogs. PloS One 9, e99686. doi: 10.1371/journal.pone.0099686
Jaeger M. M., Haque E., Sultana P., Bruggers R. L. (2007). Daytime cover, diet, and space-use of golden jackals (Canis aureus) in agro-ecosystems of Bangladesh. Mammalia 71, 1–10. doi: 10.1515/MAMM.2007.016
Kamler J. F., Klare U., Macdonald D. W. (2012). Seasonal diet and prey selection of black-backed jackals on a small-livestock farm in South Africa. Afr. J. Ecology. 50, 299–307. doi: 10.1111/j.1365-2028.2012.01324.x
Kamler J. F., Stenkewitz U., Gharajehdaghipour T., Macdonald D. W. (2019). Social organization, home ranges, and extraterritorial forays of black-backed jackals. J. Wildlife Management. 83, 1800–1808. doi: 10.1002/jwmg.21748
Kaunda S. K. K., Skinner J. D. (2003). Black-backed jackal diet at Mokolodi Nature Reserve, Botswana. Afr. J. Ecology. 41, 39–46. doi: 10.1046/j.1365-2028.2003.00405.x
Kerley G. I. H., Wilson S. L., Balfour D. (2018). Livestock predation and its management in South Africa: A scientific assessment (Port Elizabeth: Centre for African Conservation Ecology, Nelson Mandela University).
Khorozyan I., Ghoddousi A., Soofi M., Waltert M. (2015). Big cats kill more livestock when wild prey reaches a minimum threshold. Biol. Conserv. 192, 268–275. doi: 10.1016/j.biocon.2015.09.031
Klare U. N. N., Kamler J. F., Stenkewitz U. T. E., Macdonald D. W. (2010). Diet, prey selection, and predation impact of black-backed jackals in South Africa. J. Wildlife Management. 74, 1030–1041. doi: 10.2193/2009-211
Krofel M., Giannatos G., Ćirovič D., Stoyanov S., Newsome T. M. (2017). Golden jackal expansion in Europe: a case of mesopredator release triggered by continent-wide wolf persecution? Hystrix 28, 9–15. doi: 10.4404/hystrix-28.1-11819
Lewis J. C., Sallee K. L., Golightly R. T. (1993). Introduced red fox in California (Sacramento, California, USA: State of California, the Resources Agency, Department of Fish and Game, Wildlife Management Division).
Loveridge A. J., Macdonald D. W. (2003). Niche separation in sympatric jackals (Canis mesomelas and Canis adustus). J. Zoology. 259, 143–153. doi: 10.1017/S0952836902003114
Luskin M. S., Brashares J. S., Ickes K., Sun I. F., Fletcher C., Wright S. J., et al. (2017). Cross-boundary subsidy cascades from oil palm degrade distant tropical forests. Nat. Commun. 8, 2231. doi: 10.1038/s41467-017-01920-7
MacKenzie D. I., Nichols J. D., Royle J. A., Pollock K. H., Bailey L. L., Hines J. E. (2006). Occupancy estimation and modeling: inferring patterns and dynamics of species occurrence (San Diego, USA: Elsevier).
Manlick P. J., Pauli J. N. (2020). Human disturbance increases trophic niche overlap in terrestrial carnivore communities. Proc. Natl. Acad. Sci. 117, 26842–26848.1. doi: 10.1073/pnas.2012774117
Moehlman P. D. (1987). Social organization in jackals: the complex social system of jackals allows the successful rearing of very dependent young. Sci. Am. 75 (4), 366–375.
Marneweck C. J., Allen B. L., Butler A. R., Do Linh San E., Harris S. N., Jensen A. J., et al. (2022). Middle-out ecology: small carnivores as sentinels of global change. Mammal Rev. 52, 471–479. doi: 10.1111/mam.12300
Masseloux J., Epps C. W., Duarte A., Schwalm D., Wykstra M. (2018). Using detection/non-detection surveys and interviews to assess carnivore site use in Kenya. Afr. J. Wildlife Res. 48, 1–24. doi: 10.3957/056.048.013006
Mberirua P. (2018). Rapid habitat suitability assessment for carnivores in three communal conservancies (African wild dogs, Otjituuo & Okamatapati) in the Greater Waterberg Landscape, Namibia (Windhoek (Namibia: Namibia University of Science and Technology).
Mbizah M. M., Joubert C. J., Joubert L., Groom R. J. (2014). Implications of African wild dog (Lycaon pictus) denning on the density and distribution of a key prey species: addressing myths and misperceptions. Biodiversity Conserv. 23, 1441–1451. doi: 10.1007/s10531-014-0675-9
Mendelsohn J. M., El Obeid S. (2002). The Communal Lands in Eastern Namibia (Windhoek, Namibia: RAISON and Namibian Nature Foundation).
Merkle J. A., Stahler D. R., Smith D. W. (2009). Interference competition between gray wolves and coyotes in Yellowstone National Park. Can. J. Zoology. 87, 56–63. doi: 10.1139/Z08-136
Nakashima Y. (2019). Potentiality and limitations of N-mixture and Royle-Nichols models to estimate animal abundance based on noninstantaneous point surveys. Population Ecol. 62, 151–157. doi: 10.1002/1438-390X.12028
Nakashima Y., Do Linh San E. (2022). Seed dispersal by mesocarnivores: importance and functional uniqueness in a changing world. In Do Linh S. M., San E., Sato J. J., Belant J. L. (Eds.), Small carnivores: Evolution, ecology, behaviour and conservation. (Wiley-Blackwell), 347–391. doi: 10.1002/9781118943274.ch18
Nellemann C., Støen O. G., Kindberg J., Swenson J. E., Vistnes I., Ericsson G., et al. (2007). Terrain use by an expanding brown bear population in relation to age, recreational resorts and human settlements. Biol. Conserv. 138, 157–165. doi: 10.1016/j.biocon.2007.04.011
Nghikembua M., Harris J., Tregenza T., Marker L. (2016). Spatial and temporal habitat use by GPS collared male cheetahs in modified bushland habitat. Open J. Forestry 6, 269–280. doi: 10.4236/ojf.2016.64022
O'Connor T. G., Puttick J. R., Hoffman M. T. (2014). Bush encroachment in southern Africa: changes and causes. Afr. J. Range Forage Science. 31, 67–88. doi: 10.2989/10220119.2014.939996
O’Brien T. G., Ahumada J., Akampurila E., Beaudrot L., Boekee K., Brncic T., et al. (2020). Camera trapping reveals trends in forest duiker populations in African National Parks. Remote Sens. Ecol. Conserv. 6, 168–180. doi: 10.1002/rse2.132
O’Neill H. M. K., Durant S. M., Woodroffe R. (2020). What wild dogs want: Habitat selection differs across life stages and orders of selection in a wide-ranging carnivore. BMC Zoology. 5, 1–11. doi: 10.1186/s40850-019-0050-0
Oberosler V., Groff C., Iemma A., Pedrini P., Rovero F. (2017). The influence of human disturbance on occupancy and activity patterns of mammals in the Italian Alps from systematic camera trapping. Mamm. Biol. 87, 50–61. doi: 10.1016/j.mambio.2017.05.005
Paolino R. M., Royle J. A., Versiani N. F., Rodrigues T. F., Pasqualotto N., Krepschi V. G., et al. (2018). Importance of riparian forest corridors for the ocelot in agricultural landscapes. J. Mammalogy. 99, 874–884. doi: 10.1093/jmammal/gyy075
Pardo L. E., Swanepoel L., Curveira-Santos G., Fritz H., Venter J. A. (2022). Habitat structure, not the anthropogenic context or large predators, shapes occupancy of a generalist mesopredator across protected areas in South Africa. Mammal Res. 67, 265–278. doi: 10.1007/s13364-022-00636-4
Petroelje T. R., Belant J. L., Beyer D. E. Jr., Svoboda N. J. (2019). Subsidies from anthropogenic resources alter diet, activity, and ranging behavior of an apex predator (Canis lupus). Sci. Rep. 9, 13438. doi: 10.1038/s41598-019-49879-3
Phumanee W., Steinmetz R., Phoonjampa R., Bejraburnin T., Grainger M., Savini T. (2020). Occupancy-based monitoring of ungulate prey species in Thailand indicates population stability, but limited recovery. Ecosphere 11, e03208. doi: 10.1002/ecs2.3208
Poessel S. A., Mock E. C., Breck S. W. (2017). Coyote (Canis latrans) diet in an urban environment: variation relative to pet conflicts, housing density, and season. Can. J. Zoology 95, 287–297. doi: 10.1139/cjz-2016-0029
Pomilia M. A., Mcnutt J. W., Jordan N. R. (2015). Ecological predictors of African wild dog ranging patterns in Northern Botswana. J. Mammalogy. 96, 1214–1223. doi: 10.1093/jmammal/gyv130
Prugh L. R., Stoner C. J., Epps C. W., Bean W. T., Ripple W. J., Laliberte A. S., et al. (2009). The rise of the mesopredator. Bioscience 59, 779–791. doi: 10.1525/bio.2009.59.9.9
Rasmussen G. S. A. (1999). Livestock predation by the painted hunting dog Lycaon pictus in a cattle ranching region of Zimbabwe: a case study. Biol. Conserv. 88, 133–139. doi: 10.1016/S0006-3207(98)00006-8
Reasoner E. (2023). Human-wildlife conflict and coexistence of black-backed jackal (Lupullela mesomelas) and African wild dog (Lycaon pictus) in the Okakarara District Communal Area, Namibia (Windhoek (Namibia: Namibia University of Science and Technology).
Rich L. N., Miller D. A. W., Robinson H. S., McNutt J. W., Kelly M. J. (2017). Carnivore distributions in Botswana are shaped by resource availability and intraguild species. J. Zoology. 303, 90–98. doi: 10.1111/jzo.12470
Ripple W. J., Estes J. A., Beschta R. L., Wilmers C. C., Ritchie E. G., Hebblewhite M., et al. (2014). Status and ecological effects of the world’s largest carnivores. Science 343, 1241484. doi: 10.1126/science.1241484
Ritchie E. G., Johnson C. N. (2009). Predator interactions, mesopredator release and biodiversity conservation. Ecol. Letters. 12, 982–998. doi: 10.1111/j.1461-0248.2009.01347.x
Roemer G. W., Gompper M. E., Van Valkenburgh B. (2009). The ecological role of the mammalian mesocarnivore. BioScience 59, 165–173. doi: 10.1525/bio.2009.59.2.9
Rovero F., Ahumada J., Jansen P. A., Sheil D., Alvarez P., Boekee K., et al. (2020). A standardized assessment of forest mammal communities reveals consistent functional composition and vulnerability across the tropics. Ecography 43, pp.75–pp.84. doi: 10.1111/ecog.04773
Royle J. A. (2006). Site occupancy models with heterogeneous detection probabilities. Biometrics 62, 97–102. doi: 10.1111/j.1541-0420.2005.00439.x
Royle J. A., Nichols J. D. (2003). Estimating abundance from repeated presence-absence data or point counts. Ecology 84, 777–790. doi: 10.1890/0012-9658(2003)084[0777:EAFRPA]2.0.CO;2
Rust N. A., Marker L. L. (2013). Cost of carnivore coexistence on communal and resettled land in Namibia. Environ. Conserv. 41, 45–53. doi: 10.1017/S0376892913000180
Schuette P., Wagner A. P., Wagner M. E., Creel S. (2013). Occupancy patterns and niche partitioning within a diverse carnivore community exposed to anthropogenic pressures. Biol. Conserv. 158, 301–312. doi: 10.1016/j.biocon.2012.08.008
Schwieger D. A. M. (2022). Exploring pastoralists’ perceptions of desertification tipping points in Namibia’s communal drylands: An ethnographic case study from Okakarara constituency. Pastoralism 12, 1–15. doi: 10.1186/s13570-022-00231-x
Shilongo S. M., Sam M., Simuela A. (2018). Using incentives as mitigation measure for human wildlife conflict management in Namibia. Int. J. Sci. Res. Publications. 8, 677. doi: 10.29322/IJSRP.8.11.2018.p8374
Sólymos P., Lele S., Bayne E. (2012). Conditional likelihood approach for analyzing single visit abundance survey data in the presence of zero inflation and detection error. Environmetrics 23, 197–205. doi: 10.1002/env.1149
Strohbach B. J. (2014). Vegetation of the eastern communal conservancies in Namibia: I. Phytosociological descriptions. Koedoe: Afr. Protected Area Conserv. Science. 56, 1–18. doi: 10.4102/koedoe.v56i1.1116
Suraci J. P., Gaynor K. M., Allen M. L., Alexander P., Brashares J. S., Cendejas-Zarelli S., et al. (2021). Disturbance type and species life history predict mammal responses to humans. Global Change Biol. 27, 3718–3731. doi: 10.1111/gcb.15650
Tobler M. W., Zúñiga Hartley A., Carrillo-Percastegui S. E., Powell G. V. (2015). Spatiotemporal hierarchical modelling of species richness and occupancy using camera trap data. J. Appl. Ecol. 52, 413–421. doi: 10.1111/1365-2664.12399
Van der Meer E., Fritz H., Blinston P., Rasmussen G. S. (2014). Ecological trap in the buffer zone of a protected area: effects of indirect anthropogenic mortality on the African wild dog Lycaon pictus. Oryx 48, 285–293. doi: 10.1017/S0030605312001366
Van der Weyde L. K., Mbisana C., Klein R. (2018). Multi-species occupancy modelling of a carnivore guild in wildlife management areas in the Kalahari. Biol. Conserv. 220, 21–28. doi: 10.1016/j.biocon.2018.01.033
Van de Ven T. M. F. N., Tambling C. J., Kerley G. I. H. (2013). Seasonal diet of black-backed jackal in the Eastern Karoo, South Africa. J. Arid Environments. 99, 23–27. doi: 10.1016/j.jaridenv.2013.09.003
Verschueren S., Briers-Louw W. D., Monterroso P., Marker L. (2021a). Local-scale variation in land use practice supports a diverse carnivore guild on Namibian multiple-use rangeland. Rangeland Ecol. Management. 79, 64–76. doi: 10.1016/j.rama.2021.07.007
Verschueren S., Briers-Louw W. D., Torres-Uribe C., Siyaya A., Marker L. (2020). Assessing human conflicts with carnivores in Namibia’s eastern communal conservancies. Hum. Dimensions Wildlife. 25, 452–467. doi: 10.1080/10871209.2020.1758253
Verschueren S., Torres-Uribe C., Briers-Louw W. D., Fleury G., Cristescu B., Marker L. (2021b). Flashing lights to deter small stock depredation in communal farmlands of Namibia. Conserv. Evidence J. 18, 50–51. doi: 10.52201/CEJ18VQFL3817
Wearn O. R., Rowcliffe J. M., Carbone C., Pfeifer M., Bernard H., Ewers R. M. (2017). Mammalian species abundance across a gradient of tropical land-use intensity: A hierarchical multi-species modelling approach. Biol. Conserv. 212, 162–171. doi: 10.1016/j.biocon.2017.05.007
Wevers J., Beenaerts N., Casaer J., Zimmermann F., Artois T., Fattebert J. (2021). Modelling species distribution from camera trap by-catch using a scale-optimized occupancy approach. Remote Sens. Ecol. Conserv. 7, 534–549. doi: 10.1002/rse2.207
Wilmers C. C., Crabtree R. L., Smith D. W., Murphy K. M., Getz W. M. (2003). Trophic facilitation by introduced top predators: grey wolf subsidies to scavengers in Yellowstone National Park. J. Anim. Ecology. 72, 909–916. doi: 10.1046/j.1365-2656.2003.00766.x
Woodroffe R., Lindsey P., Romanach S., Stein A., ole Ranah S. M. (2005). Livestock predation by endangered African wild dogs (Lycaon pictus) in northern Kenya. Biol. Conserv. 124, 225–234. doi: 10.1016/j.biocon.2005.01.028
Wright J. H., Hill N. A., Roe D., Rowcliffe J. M., Kümpel N. F., Day M. (2016). Reframing the concept of alternative livelihoods. Conserv. Biol. 30, 7–13. doi: 10.1111/cobi.12607
Zeller K. A., Vickers T. W., Ernest H. B., Boyce W. M. (2017). Multi-level, multi-scale resource selection functions and resistance surfaces for conservation planning: Pumas as a case study. PLoS One 12, e0179570. doi: 10.1371/journal.pone.0179570
Keywords: African wild dog, black-backed jackal, carnivore abundance, Lupulella mesomelas, Lycaon pictus, Namibia, Okakarara District Communal Area
Citation: Reasoner E, Marker L, Verschueren S, Briers-Louw WD, Mbidzo M and Cristescu B (2024) Relative abundance of a mesocarnivore in a human-dominated, semi-arid rangeland in Namibia. Front. Ecol. Evol. 12:1333162. doi: 10.3389/fevo.2024.1333162
Received: 04 November 2023; Accepted: 27 August 2024;
Published: 19 September 2024.
Edited by:
Dennis Murray, Trent University, CanadaReviewed by:
Thomas Göttert, Eberswalde University for Sustainable Development, GermanyMichelle Kissling, Fulbright Association, United States
Copyright © 2024 Reasoner, Marker, Verschueren, Briers-Louw, Mbidzo and Cristescu. This is an open-access article distributed under the terms of the Creative Commons Attribution License (CC BY). The use, distribution or reproduction in other forums is permitted, provided the original author(s) and the copyright owner(s) are credited and that the original publication in this journal is cited, in accordance with accepted academic practice. No use, distribution or reproduction is permitted which does not comply with these terms.
*Correspondence: Bogdan Cristescu, Ym9nZGFuQGNoZWV0YWgub3Jn