- 1Zoolab, Department of Life Science and Systems Biology, University of Turin, Turin, Italy
- 2Applied Conservation Ecology Lab, Department of Renewable Resources, University of Alberta, Edmonton, AB, Canada
- 3Department of Environmental Geography, Institute for Environmental Studies, Vrije Universiteit Amsterdam, Amsterdam, Netherlands
The number of studies in functional ecology grew exponentially in the last decades. Whether and how ecologists changed how they conduct these studies, however, remains poorly understood. Using butterflies as a model taxon, we assessed forty years of research asking whether and how functional analyses have changed. We found that how authors contextualize their work corresponds to divergent sub-fields in functional ecology. Articles explicitly referring to “functional traits” have become increasingly common in the last decade, focus on many species, and typically address the relationship between biodiversity and environmental gradients. Meanwhile, articles that do not refer to “functional traits” usually account for variation within species and among sexes, and are based on direct measures of the trait of interest. These differences have increased over time, highlighting a schism. As functional ecology continues to grow, authors and syntheses will benefit from awareness of these different schools of thought.
Introduction
Functional ecology is steadily becoming more popular (Palacio et al., 2022), and the number of studies referring to “functional traits” (Box 1) has grown exponentially in the past two decades (Naeem and Bunker, 2009). Meanwhile, the research landscape also changed profoundly, shaping how science is perceived and conducted (Wu et al., 2019). Theoretical advancements clarified the benefits of seeing natural systems through a functional lens (McGill et al., 2006; Violle et al., 2007; Malaterre et al., 2019), and we are experiencing a technological revolution that has provided us with new methods, increased computational power, and thousands of datasets open to the public (Mammola et al., 2021; Palacio et al., 2022). Because the scientific landscape evolved so profoundly, one might wonder whether and how “functional analyses” (Box 1) also changed.
The idea of functional diversity has become central in ecology (Tilman, 2001), and numerous authors are now interested in following a “trait-based approach” (Box 1) when assessing changes in biodiversity and ecosystem services (Pimm et al., 1995; Chapin et al., 2000; Díaz et al., 2016; Carmona et al., 2021). Landmark papers published in the early 2000s popularized the idea of trait-based analyses, and from these papers stemmed hundreds of contributions (see, e.g., McGill et al., 2006 and Violle et al., 2007). Given this rapid expansion, evaluating whether different subfields exist in functional ecology is of broad interest. For instance, the link between traits and environment has been traditionally explored in the context of species’ biology and life history (Keddy, 1992), but as the concept of “biodiversity” (Wilson, 1988) gained popularity, new emphasis has been placed on the relationships between diversity and ecosystem functioning and services (Bolnick et al., 2011; Violle et al., 2012). This suggests that the focus of functional analyses might have shifted through time to assess more often biodiversity variables. Some work explored similar trends for plants (Duarte et al., 1995), but other taxa, especially invertebrates (Schleuning et al., 2023), remain poorly studied. Indeed, previous research shows that approximately five times fewer publications have been published on functional diversity for animals than for plants (Blaum et al., 2011; Villéger et al., 2017).
Because functional ecology plays a central role for understanding species responses to environmental change [e.g., responses to changes in climate or land use (Hällfors et al., 2021; Riva et al., 2023)], it is paramount to understand if seminal, past analyses are detached from terms that authors are currently more likely to use. Referring to “functional traits” was less common in the past (McGill et al., 2006; Violle et al., 2007), but functional analyses in a broader sense were not rare (Calow, 1987). Additionally, several authors have highlighted that functional ecology needs to integrate different datasets and theories across spatial scales to address novel questions [e.g., on the evolution of plasticity (Usui et al., 2023) or intraspecific variability (Violle et al., 2012) across environmental gradients]. Nevertheless, if different terminology is associated with distinct schools of thought, gaps between sub-fields might be widening, not closing, hindering integration. Last, if a particular subset of literature is easier to retrieve because of terminology, perspectives and syntheses will be inevitably biased in favor of the results of these studies.
In this context, assessments of the literature in functional ecology – particularly for organisms that are typically neglected, such as insects (Chowdhury et al., 2022; Mammola et al., 2023) – can provide insights on the status of the discipline. For instance, if authors tend to focus increasingly often on multiple species and coarser data, functional analyses would become more prone to ignore intraspecific variability through time (Sparrow et al., 2020). Modern technological advances could help addressing similar issues [e.g., providing crowd-sourced observation of multi-species classification through community science, or extracting high-resolution and multidimensional trait data with machine learning and image analysis (Besson et al., 2022; Wolf et al., 2022)], but to be properly addressed, any knowledge gap must first be identified. Awareness on the existence of diverging subfield is therefore an important first step towards better understanding functional ecology. Efforts in this direction have the potential to facilitate answering many exciting questions, e.g., on evolutionary and ecological determinants of functional diversity, or on how trait variation interacts with local environmental processes to shape ecosystem functioning and services across large spatial scales (Duarte et al., 1995; Petchey and Gaston, 2006; Violle et al., 2014).
Here, we assessed how functional studies changed in the last forty years. Specifically, we asked how the field of functional ecology evolved for butterflies, a charismatic insect taxon. We evaluated whether butterflies’ functional analyses that did or did not refer explicitly to functional traits – here defined as “explicit” and “implicit” functional analyses respectively (see Methods) – differ and changed over time. We chose to focus on butterflies because (i) these taxa are a traditional model group, e.g., often used as indicators of environmental change (Thomas, 2005), and (ii) information on functional analyses in invertebrates remains exceedingly rare (Chowdhury et al., 2022; Palacio et al., 2022). We used wing size as a focal trait because of its broad application in functional studies of this taxa (e.g., representing dispersal or body size; Sekar, 2012).
Our goal was to assess how functional studies changed in the last 40 years depending on whether authors explicitly refer to functional traits in their article, to evaluate if different use of lexicon corresponds to different conceptual frameworks. We focused on both the questions asked by authors interested in functional analyses, and on the methods employed to answer those questions. Due to theoretical advancements and the need to understand how species respond to environmental change amidst a biodiversity crisis, we expected a shift in functional ecology from a higher focus on the morphology of species, to studies relating traits to ecological phenomena and/or the environment. Furthermore, due to an increasing availability of datasets and methodological approaches, we expected a transition to analyses assessing multiple species, instead of more traditional studies focusing on single species.
Materials and methods
Box 1: Functional ecology, traits, and analyses
Following Calow (1987); McGill et al. (2006) and Bellwood et al. (2019), we broadly consider “functional ecology” as the field that attempts to understand the mechanisms underlying ecological patterns and processes based on their relationship with species’ “functional traits”. We follow Violle et al. (2007) in conceptualizing “functional traits” as any morphological, physiological, or phenological feature measurable at the individual level, from the cell to the whole-organism level, without reference to the environment or any other level of organization. Following on these definitions, we define any attempt to tease apart relationships between properties of taxa and the axes of their niche (Hutchinson, 1978), including species-environment relationship or biotic interactions, as a “functional analysis” (Calow, 1987; Violle et al., 2007).
Data acquisition
We conducted a literature review searching for papers where measures of butterfly wing size were used as functional traits (Box 1). We used wing size as a focal trait because of its broad application in the ecology of these taxa, such as dispersal, body size and allometric rules, or species-environment relationships (Barbaro and van Halder, 2009; Sekar, 2012). For instance, recent work showed that wingspan predicts which species of butterflies are more likely to respond negatively to land use across Italy (Riva et al., 2023) and that cooler temperature selects for larger and darker butterflies in Australia (Xing et al., 2016). We reviewed 172 studies published in the last 40 years and analyzed all functional analyses that either targeted butterflies and used a measure of wing size (defined here as “implicit functional analyses”), or targeted butterflies and used a measure of wing size while explicitly referring to “functional traits” (defined here as “explicit functional analyses”). One of our key objectives was to evaluate whether a different use of language corresponded to different ways to conduct functional analyses (Box 1).
We searched in the ISI Web of Science Database, in the period between 1980 and 2019, for explicit and implicit functional analyses through two search strings. The string for implicit functional analyses was “TOPIC: (butterfl*) AND TOPIC (wingspan$ OR wing$ span$ OR Forewing$ OR wing$ length OR wing$ dimension$)”, whereas the string for explicit functional analyses was “TOPIC: (butterfl*) AND TOPIC: (functional$ traits$)”. Using the same search string for explicit and implicit functional analyses, i.e., “TOPIC: (butterfl*) AND TOPIC (wingspan$ OR wing$ span$ OR Forewing$ OR wing$ length OR wing$ dimension$) AND TOPIC: (functional$ traits$)” was not possible because the number of hits in explicit functional analyses was too limited. We therefore expanded the search for explicit studies to all studies on butterflies referring to “functional traits”, i.e., TOPIC: (butterfl*) AND TOPIC: (functional$ traits$)”. After reading all the 196 articles retrieved, we excluded from the analysis 24 studies where wing size metrics were only mentioned, and where wing size was not used as a functional trait (e.g., studies describing the morphology of a species).
Classification of functional analyses
To assess how functional studies focusing on butterflies changed in the last 40 years, we classified papers based on six different variables related to two main aspects: (i) a “biological” aspect, representing biological characteristics of the system studied that are selected during study design, but are inherent to the system under consideration (variables a and b) and (ii) a “methodological” aspect, representing different methods that are employed by authors to study the same system, and thus can vary even assessing the same system of interest (variables c to f).
For the biological aspect, we assessed whether wing size was linked to extrinsic vs. intrinsic phenomena (a), and whether a single vs. multiple species were considered by authors in their analyses (b). We classified relationships with extrinsic phenomena when wing size was related with factors external to the species/individual (e.g., studies linking environmental variation and functional traits), and relationships with intrinsic phenomena when wing size was measured as proxy of another characteristic of the species/individual (e.g., studies where wing size was used as a proxy of movement ability, reproduction, phenology, or body size). See Supporting Information S1.2 for further information about different type of extrinsic and intrinsic factors related to wing dimensions.
The methodological aspect included four variables that represent methodological approaches in functional analyses (c-f). We asked whether wing dimension was measured as wingspan (WS) or forewing length (FW). We considered FW as the most accurate measure of wing dimension, as WS is often calculating doubling FW and is sensitive to a larger measurement error [Supporting Information S1.1 (Tiple et al., 2009; Burke et al., 2011; Gentile et al., 2021)]. We then asked whether the wing dimension used in the study was directly measured by the authors or extracted from a secondary source (Supporting Information S1.1) (d). Last, variables (e) and (f) were respectively whether variation between sexes and variation across individuals were considered in the analyses and relate to the precision of the analysis. Since variables e, d, and f could have been correlated, we performed a correlation test. The test did not show strong collinearity between the three variables (r always < 0.7; Supplementary Information S1.3).
Analysis
We propose two analyses. We first assessed how temporal trends in the number of manuscripts produced differed based on whether a functional analysis was explicit or implicit (Figure 1). We initially fit a linear model assuming a Gaussian distribution and identity link function, where the response (number of papers published per year) was modelled as a function of year and research type (implicit vs. explicit). After fitting this model, we observed that explicit functional studies started to appear only after 2005, a pattern suggesting the presence of a “breaking point”. Therefore, we used the segmented package to fit a piecewise regression, where the number of explicit functional studies was modelled as a function of year and type of research.
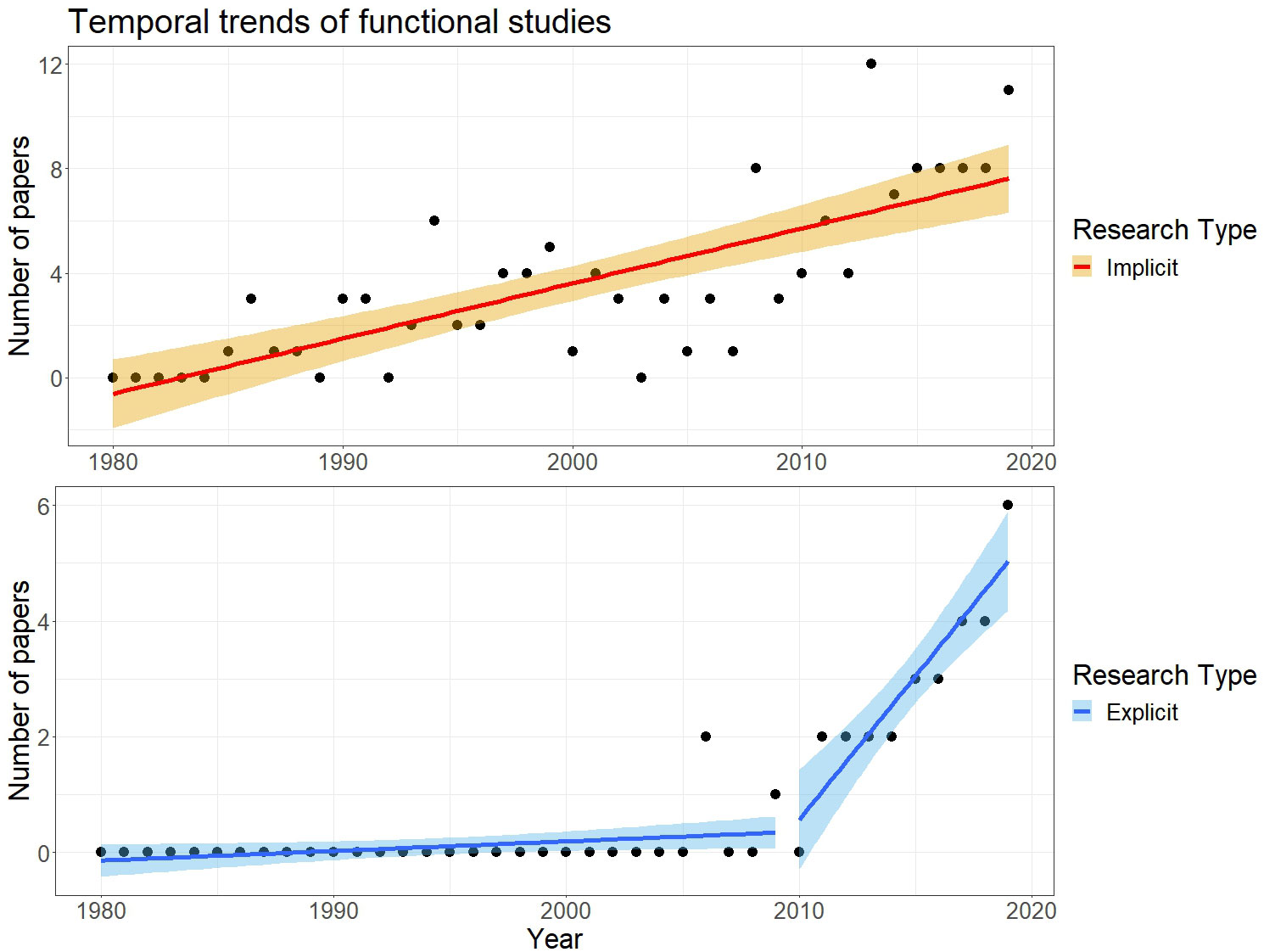
Figure 1 Temporal trends in functional studies of butterflies that use wing measures as functional traits between 1980 and 2020. The top row, in orange, illustrates studies that did not explicitly mention “functional traits” in the title, abstract and key words (“implicit functional studies”). The bottom row, in blue, illustrates the studies that did mention “functional traits” in the title, abstract and key words (“explicit functional studies”). For explicit functional studies, we found support for a breakpoint corresponding approximately to the year 2009.
Next, we evaluated whether the type of research (i.e., explicit vs. implicit functional analyses) affects the probability of observing different biological and methodological variables through time. We hypothesized that different terminological choices could correspond to different approaches to functional analyses. Each of the six variables assessed was coded as binary, representing the incidence of a given aspect. We fit generalized linear models assuming a binomial distribution and with a logit link function to predicted probabilities of each variable occurrence as a function of year and research type. We created for each response four models representing competing hypotheses: (i) null model, (ii) only effects of year of publication, (iii) additive effects of year of publication and research type and (iv) interactive effects of year of publication and research type. For each variable, we selected the most supported model (pattern in temporal trends) using the Akaike Information Criteria (AIC) (Burnham and Anderson, 2004). Following Burnham and Anderson (2004), models with the lowest AIC value were considered as the best models in their set, and when differences between AIC values were less than 2, we selected the less complex model (i.e., those with a lower number of parameters) for parsimony. We provide in supporting information parameter estimates, their standard errors, Wald statistics, and p-values of fitted models for all models (Supporting Information S1.3; Supplementary Tables S2, S3).
All models and plots were generated in software R version 4.2.0 (R Core Team, 2022). Data and R scripts are available in the supplementary information (Supplementary Data sheet 1, 2, and R code).
Results
We found 172 papers published between 1980 and 2019 that used metrics of wing size in butterflies to conduct functional analyses (Box 1; Supporting Information S2.1). More than 80% of these papers were implicit functional studies that did not refer to “functional traits” (n = 140) with the first article published in 1985. For the remaining explicit functional studies that did refer to “functional traits” (n = 32), the first article was published in 2006. Both explicit and implicit functional studies increased through time (Supporting Information S1.3, Supplementary Table S2), but the former displayed an evident breakpoint in 2009, after which such analyses increased drastically (Figure 1).
In addition to different temporal patterns in publication numbers, implicit and explicit studies differed for both biological and methodological aspects. These differences have also typically increased through time (Figure 2). Studies that referred directly to functional traits were more likely to relate functional traits to extrinsic factors (e.g., environmental conditions) (Figure 2A), and to consider multiple species (Figure 2B), outlining a focus on different biological variables. Both implicit and explicit studies are increasingly assessing extrinsic factors through time (Figure 2A), but only explicit studies are assessing increasingly frequently multiple species (Figure 2B). When considering methodological aspects, explicit and implicit studies present substantial differences, i.e., explicit studies were more likely to use wingspan, a less accurate metric of body size (Figure 2C) (Supporting Material S1.1), and less likely to use a trait measured directly (Figure 2D). At the same time, explicit studies were less likely to incorporate variability between sexes (Figure 2E) and among individuals (Figure 2F). Based on AIC scores, the models including an effect of time, and the division between explicit and implicit studies, were always more supported than simpler models (Supplementary Information). Overall, these results suggest that differences in ideas and approaches within functional ecology are intertwined with the terminology that authors use to contextualize their functional analyses.
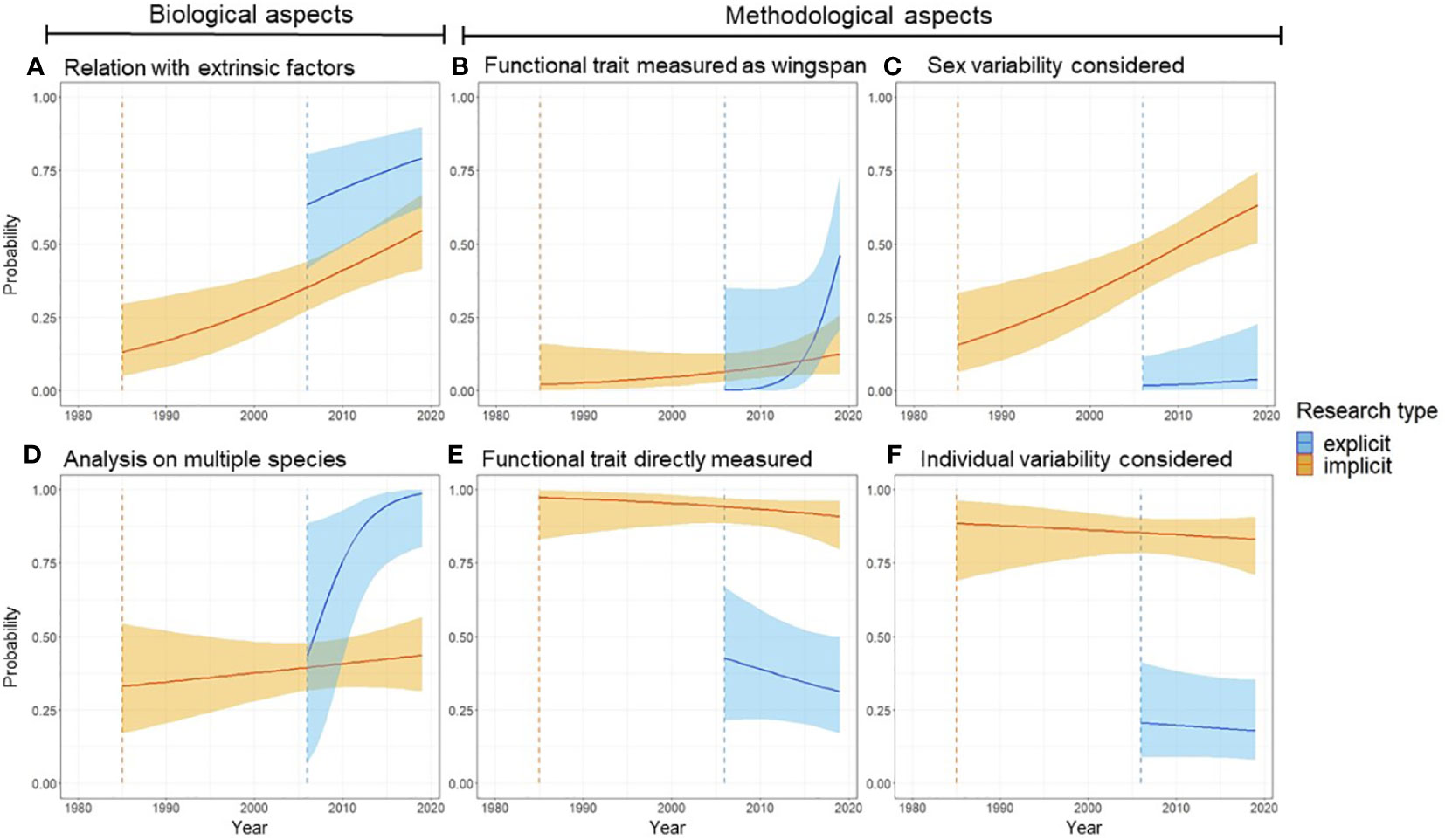
Figure 2 Functional studies using butterfly wing metrics changed between 1980 and 2020, and change differed between implicit (orange lines) and explicit (blue lines) functional studies. Panels (A, B) show temporal trends for biological variables, whereas panels (C–F) show temporal trends of methodological variables. Orange and blue vertical dashed lines show when implicit and explicit functional studies first appeared in the literature based on our review (respectively 1985 and 2006).
Discussion
Functional ecology is undeniably a growing field (Palacio et al., 2022). Nevertheless, how the field has evolved throughout its growth is less clear. Here, we review the literature related to butterfly wing metrics to assess how functional studies changed in the last 40 years, and if this change is related to whether authors refer to functional traits in their work. Our results suggest that (i) functional analyses have not only increased, but also changed significantly, with more scientists interested in understanding how species are related to the environment (Figures 1 and 2); (ii) a combination of technological developments and influential papers since the early 2000s have fostered the development of a new trend within functional ecology, with an explicit reference to functional traits (Figure 1); (iii) this new trend is tied with differences in how functional studies are conducted methodologically. Such differences are increasing through time, suggesting the existence of diverging sub-fields in functional ecology (Figure 2). Similar trends have been described for plants (see, e.g., trade-offs between different types of traits measurements, or advantages and disadvantages of considering multiple species; Duckworth et al., 2000; Albert et al., 2011). Here we provide new evidence for butterflies, a charismatic group among largely neglected insect taxa, adding to a general understanding of trends in the discipline of functional ecology.
Surprisingly, we found that the term “functional traits” has not been used in our sample before 2006 (Figure 1). We speculate that the adoption of this terminology was likely fostered by a few influential papers (e.g., McGill et al., 2006; Violle et al., 2007) that catalyzed interest on trait-based functional analyses. It is impossible to resolve whether an increase in studies that explicitly refer to functional traits was a consequence of the publication of such seminal manuscripts, or whether these increased in number because they crystallized mounting interest in functional analyses (Loreau et al., 2001). Most likely, both processes have been at play. But more broadly, our analysis shows how functional ecology has evolved in the last 40 years – at least for butterflies. For instance, we found a general increase in the studies that attempt to relate butterfly wing size with environmental factors (Figure 2), a pattern consistent with the hypothesis that ecologists have been increasingly concerned about how functional traits mediate species-environment relationships (Díaz et al., 2016; Carmona et al., 2021). The positive trend between time and interest in such extrinsic factors, such as environmental gradients (Figure 2A), is the strongest trend shared between explicit and implicit analyses across all the variables we measured and is opposite to the more common pattern of divergence (e.g., Figures 2B–E).
Our results hint at the existence of two distinct sub-fields in butterfly functional ecology. Specifically, depending on whether authors explicitly refer to functional traits in their article or not, they tended to follow different approaches in their analyses (Figure 2). While the classification in “implicit” or “explicit” studies is an outcome of our literature search method, the differences we documented between the two types of studies were only hypothesized as we approached the study and emerged from our analysis. Model selection based on information theory supports the hypothesis that two different sub-fields, or schools of thought, exist in functional ecology. Importantly, the gap between these sub-fields has been widening, not closing, even if only 15 years of overlapping data are available (Figure 1).
Authors choosing to characterize their work referring to functional traits are capitalizing on increasing availability of larger datasets and new methodological approaches, as demonstrated by a higher likelihood to study many species (Figure 2B), but often neglecting intraspecific diversity (Figures 2D, F). Several programs have been designed to share databases of functional traits in the last two decades (Middleton-Welling et al., 2020; Palacio et al., 2022; Shirey et al., 2022), allowing authors to easily access information on multiple species. With this increasing availability of data, new avenues of research have also been proposed [e.g., functional biogeography (Violle et al., 2014)]. Explicit functional analyses seem therefore to have emerged as the result of positive feedback among conceptual advancements (e.g., McGill et al., 2006; Violle et al., 2014), increasing availability of data (Shirey et al., 2022), and methodological and technological advancements (Palacio et al., 2022). Conversely, implicit studies are typically characterized by information on only one species, and this information tends to be more comprehensive. Such studies might reveal important effects, e.g., of variation in traits among individuals, between sexes, and trait plasticity that are increasingly recognized as important eco-evolutionary forces (Wong et al., 2019; Gentile et al., 2021; Wong and Carmona, 2021; Luiz et al., 2022; Usui et al., 2023).
Our results also hint at important trade-offs in functional ecology, and such trade-offs could affect our perception of the discipline. Because explicit and implicit functional analyses focus on different aspects, perspectives and syntheses based on studies from only one of the two subfields will provide an incomplete picture of the literature (Duarte et al., 1995). When the objective is finding results relevant to functional ecology, studies that refer explicitly to “functional traits” are easier to retrieve because this term is unequivocally related to functional ecology (Box 1), whereas words representing the trait themselves can be the object of studies in other contexts, such as taxonomy or evolution of species. This implies that the information reached by the readers that try to synthesize knowledge in functional ecology could be biased in favor of specific approaches and methodologies (i.e., multi-species analyses that neglect intraspecific variation and sexual dimorphism), potentially leading to an incomplete picture of the state of the field. Focusing on big databases including hundreds of species allows studying large communities of species and spatial scales (Violle et al., 2014; Palacio et al., 2022; Riva et al., 2023), but these opportunities often neglect variation in traits within and across populations (Gentile et al., 2021; Wong and Carmona, 2021). Note that our analysis is not based on large data repositories, nor on traits we measured ourselves – we instead analyzed papers which used similar datasets.
In conclusion, functional ecology is evolving thanks to new concepts, methods, and theories. This evolution points to the emergence of two related, but different ways to conceptualize functional analyses. We documented these patterns for butterflies, one of the most well-studied groups among the neglected invertebrates (Chowdhury et al., 2022; Mammola et al., 2023), but anecdotally we have observed similar trends in other taxa. Awareness of the diverging patterns we documented (Figure 2) is a first step to close the gap between different sub-fields. Decades of work outline crucial hypotheses, mechanisms, and patterns in functional ecology, and we hope that this scientific treasure will not be neglected as ecologists transition increasingly often to the analysis of big, open data. This will require capitalizing not only on big data, but also on new technologies that will allow incorporating more subtle, but important, variation in traits within species. Community science initiatives and machine learning might represent the means to this end, e.g., by automatic extraction of traits from photos. To date, community science has already been used successfully to conduct global analyses of trait distributions (e.g., Wolf et al., 2022), but the technology to automatically extract variation in traits from multiple data sources is still in development (Besson et al., 2022). More broadly, it is important to appreciate trade-offs among different approaches within functional ecology. Our hope is that our study will raise awareness in this direction.
Data availability statement
The original contributions presented in the study are included in the article/Supplementary Material. Further inquiries can be directed to the corresponding author.
Author contributions
LV: Conceptualization, Data curation, Formal analysis, Methodology, Software, Writing – original draft, Writing – review & editing. SB: Conceptualization, Resources, Supervision, Writing – review & editing. GG: Data curation, Writing – review & editing. EP: Data curation, Writing – review & editing. FR: Conceptualization, Data curation, Supervision, Validation, Visualization, Writing – review & editing.
Funding
The author(s) declare that no financial support was received for the research, authorship, and/or publication of this article.
Conflict of interest
The authors declare that the research was conducted in the absence of any commercial or financial relationships that could be construed as a potential conflict of interest.
Publisher’s note
All claims expressed in this article are solely those of the authors and do not necessarily represent those of their affiliated organizations, or those of the publisher, the editors and the reviewers. Any product that may be evaluated in this article, or claim that may be made by its manufacturer, is not guaranteed or endorsed by the publisher.
Supplementary material
The Supplementary Material for this article can be found online at: https://www.frontiersin.org/articles/10.3389/fevo.2024.1267605/full#supplementary-material
References
Albert C. H., Grassein F., Schurr F. M., Vieilledent G., Violle C. (2011). When and how should intraspecific variability be considered in trait-based plant ecology? Perspect. Plant Ecol. Evol. Systematics 13 (3), 217–225. doi: 10.1016/j.ppees.2011.04.003
Barbaro L., van Halder I. (2009). Linking bird, carabid beetle and butterfly life-history traits to habitat fragmentation in mosaic landscapes. Ecography 32 (2), 321–333. doi: 10.1111/j.1600-0587.2008.05546.x
Bellwood D. R., Streit R. P., Brandl S. J., Tebbett S. B. (2019). The meaning of the term ‘function’in ecology: A coral reef perspective. Funct. Ecol. 336, 948–961. doi: 10.1111/1365-2435.13265
Besson M., Alison J., Bjerge K., Gorochowski T. E., Høye T. T., Jucker T., et al. (2022). Towards the fully automated monitoring of ecological communities. Ecol. Lett. 25 (12), 2753–2775. doi: 10.1111/ele.14123
Blaum N., Mosner E., Schwager M., Jeltsch F. (2011). How functional is functional? Ecological groupings in terrestrial animal ecology: towards an animal functional type approach. Biodiversity Conserv. 20, 2333–2345. doi: 10.1007/s10531-011-9995-1
Bolnick D. I., Amarasekare P., Araújo M. S., Bürger R., Levine J. M., Novak M., et al. (2011). Why intraspecific trait variation matters in community ecology. Trends Ecol. Evol. 26 (4), 183–192. doi: 10.1016/j.tree.2011.01.009
Burke R. J., Fitzsimmons J. M., Kerr J. T. (2011). A mobility index for Canadian butterfly species based on naturalists’ knowledge. Biodiversity Conserv. 20 (10), 2273–2295. doi: 10.1007/s10531-011-0088-y
Burnham K. P., Anderson D. R. (2004). Multimodel inference: understanding AIC and BIC in model selection. Sociological Methods Res. 33 (2), 261–304. doi: 10.1177/0049124104268644
Calow P. (1987). Towards a definition of functional ecology. Funct. Ecol. 1 (1), 57–61. doi: 10.2307/2389358
Carmona C. P., Tamme R., Pärtel M., de Bello F., Brosse S., Capdevila P., et al. (2021). Erosion of global functional diversity across the tree of life. Sci. Adv. 7 (13), eabf2675. doi: 10.1126/sciadv.abf2675
Chapin F. S. III, Zavaleta E. S., Eviner V. T., Naylor R. L., Vitousek P. M., Reynolds H. L., et al. (2000). Consequences of changing biodiversity. Nature 405 (6783), 234–242.
Chowdhury S., Jennions M. D., Zalucki M. P., Maron M., Watson J. E., Fuller R. A. (2022). Protected areas and the future of insect conservation. Trends Ecol. Evol 38 (1), 85–95. doi: 10.1016/j.tree.2022.09.004
Díaz S., Kattge J., Cornelissen J. H., Wright I. J., Lavorel S., Dray S., et al. (2016). The global spectrum of plant form and function. Nature 529 (7585), 167–171.
Duarte C. M., Sand-Jensen K., Nielsen S. L., Enríquez S., Agustí S. (1995). Comparative functional plant ecology: rationale and potentials. Trends Ecol. Evol. 10 (10), 418–421. doi: 10.1016/S0169-5347(00)89163-6
Duckworth J. C., Kent M., Ramsay P. M. (2000). Plant functional types: an alternative to taxonomic plant community description in biogeography? Prog. Phys. Geogr. 24 (4), 515–542. doi: 10.1177/030913330002400403
Gentile G., Bonelli S., Riva F. (2021). Evaluating intraspecific variation in insect trait analysis. Ecol. Entomology 46 (1), 11–18. doi: 10.1111/een.12984
Hällfors M. H., Pöyry J., Heliölä J., Kohonen I., Kuussaari M., Leinonen R., et al. (2021). Combining range and phenology shifts offers a winning strategy for boreal Lepidoptera. Ecol. Lett. 24 (8), 1619–1632. doi: 10.1111/ele.13774
Hutchinson G. E. (1978). Introduction to population ecology (New Haven and London: Yale University Press).
Keddy P. A. (1992). A pragmatic approach to functional ecology. Funct. Ecol. 6 (6), 621–626. doi: 10.2307/2389954
Loreau M., Naeem S., Inchausti P., Bengtsson J., Grime J. P., Hector A., et al. (2001). Biodiversity and ecosystem functioning: current knowledge and future challenges. Science 294 (5543), 804–808. doi: 10.1126/science.1064088
Luiz O. J., Olden J. D., Kennard M. J., Crook D. A., Douglas M. M., Saunders T. M., et al. (2022). Substantial intraspecific trait variation across a hydrological gradient in northern Australian fishes. Ecosphere 13 (7). doi: 10.1002/ecs2.4169
Malaterre C., Dussault A. C., Rousseau-Mermans S., Barker G., Beisner B. E., Bouchard F., et al. (2019). Functional diversity: an epistemic roadmap. BioScience 69 (10), 800–811. doi: 10.1093/biosci/biz089
Mammola S., Adamo M., Antić D., Calevo J., Cancellario T., Cardoso P., Correia R. A. (2023). Drivers of species knowledge across the Tree of Life. Elife 12, RP88251. doi: 10.7554/eLife.88251.3
Mammola S., Carmona C. P., Guillerme T., Cardoso P. (2021). Concepts and applications in functional diversity. Funct. Ecol. 35 (9), 1869–1885. doi: 10.1111/1365-2435.13882
McGill B. J., Enquist B. J., Weiher E., Westoby M. (2006). Rebuilding community ecology from functional traits. Trends Ecol. Evol. 21 (4), 178–185. doi: 10.1016/j.tree.2006.02.002
Middleton-Welling J., Dapporto L., García-Barros E., Wiemers M., Nowicki P., Plazio E., et al. (2020). A new comprehensive trait database of European and Maghreb butterflies, Papilionoidea. Sci. Data 7 (1), 351. doi: 10.1038/s41597-020-00697-7
Naeem S., Bunker D. E. (2009). “TraitNet: furthering biodiversity research through the curation, discovery, and sharing of species trait data,” in Biodiversity, ecosystem functioning, and human wellbeing: An ecological and economic perspective Oxford University Press: Oxford, United Kingdom, 281–289.
Palacio F. X., Callaghan C. T., Cardoso P., Hudgins E. J., Jarzyna M. A., Ottaviani G., et al. (2022). A protocol for reproducible functional diversity analyses. Ecography 11, e06287. doi: 10.1111/ecog.06287
Petchey O. L., Gaston K. J. (2006). Functional diversity: back to basics and looking forward. Ecol. Lett. 9 (6), 741–758. doi: 10.1111/j.1461-0248.2006.00924.x
Pimm S. L., Russell G. J., Gittleman J. L., Brooks T. M. (1995). The future of biodiversity. Science 269 (5222), 347–350. doi: 10.1126/science.269.5222.347
R Core Team (2022). R: A language and environment for statistical computing (Vienna, Austria: R Foundation for Statistical Computing).
Riva F., Barbero F., Balletto E., Bonelli S. (2023). Combining environmental niche models, multi-grain analyses, and species traits identifies pervasive effects of land use on butterfly biodiversity across Italy. Global Change Biol. 29 (7), 1715–1728. doi: 10.1111/gcb.16615
Sekar S. (2012). A meta-analysis of the traits affecting dispersal ability in butterflies: Can wingspan be used as a proxy? J. Anim. Ecol. 81 (1), 174–184. doi: 10.1111/j.1365-2656.2011.01909.x
Schleuning M., García D., Tobias J. A. (2023). Animal functional traits: Towards a trait‐based ecology for whole ecosystems. Functional Ecology 37 (1), 4–12.
Shirey V., Larsen E., Doherty A., Kim C. A., Al-Sulaiman F. T., Hinolan J. D., et al. (2022). LepTraits 1.0 A globally comprehensive dataset of butterfly traits. Sci. Data 9 (1), 1–7. doi: 10.1038/s41597-022-01473-5
Sparrow B. D., Edwards W., Munroe S. E., Wardle G. M., Guerin G. R., Bastin J. F., et al. (2020). Effective ecosystem monitoring requires a multi-scaled approach. Biol. Rev. 95 (6), 1706–1719. doi: 10.1111/brv.12636
Thomas J. A. (2005). Monitoring change in the abundance and distribution of insects using butterflies and other indicator groups. Philos. Trans. R. Soc. B. Biol. Sci. 360 (1454), 339–357. doi: 10.1098/rstb.2004.1585
Tilman D. (2001). Functional diversity. Encyclopedia biodiversity 3 (1), 109–120. doi: 10.1016/B0-12-226865-2/00132-2
Tiple A. D., Khurad A. M., Dennis R. L. H. (2009). Adult butterfly feeding-nectar flower associations: Constraints of taxonomic affiliation, butterfly, and nectar flower morphology. J. Natural History 43 (13–14), 855–884. doi: 10.1080/00222930802610568
Usui T., Lerner D., Eckert I., Angert A. L., Garroway C. J., Hargreaves A., et al. (2023). The evolution of plasticity at geographic range edges. Trends Ecol. Evol 38 (9), 831–842. doi: 10.1016/j.tree.2023.04.004
Villéger S., Brosse S., Mouchet M., Mouillot D., Vanni M. J. (2017). Functional ecology of fish: current approaches and future challenges. Aquat. Sci. 79, 783–801. doi: 10.1007/s00027-017-0546-z
Violle C., Enquist B. J., McGill B. J., Jiang L. I. N., Albert C. H., Hulshof C., et al. (2012). The return of the variance: intraspecific variability in community ecology. Trends Ecol. Evol. 27 (4), 244–252. doi: 10.1016/j.tree.2011.11.014
Violle C., Navas M. L., Vile D., Kazakou E., Fortunel C., Hummel I., et al. (2007). Let the concept of trait be functional! Oikos 116 (5), 882–892. doi: 10.1111/j.0030-1299.2007.15559.x
Violle C., Reich P. B., Pacala S. W., Enquist B. J., Kattge J. (2014). “The emergence and promise of functional biogeography,” in Proceedings of the National Academy of Sciences of the United States of America, Vol. 111. 13690–13696.
Wolf S., Mahecha M. D., Sabatini F. M., Wirth C., Bruelheide H., Kattge J., et al. (2022). Citizen science plant observations encode global trait patterns. Nat. Ecol. Evol. 6 (12), 1850–1859. doi: 10.1038/s41559-022-01904-x
Wong M. K. L., Carmona C. P. (2021). Including intraspecific trait variability to avoid distortion of functional diversity and ecological inference: Lessons from natural assemblages. Methods Ecol. Evol. 12 (5), 946–957. doi: 10.1111/2041-210X.13568
Wong M. K., Guénard B., Lewis O. T. (2019). Trait-based ecology of terrestrial arthropods. Biol. Rev. 94 (3), 999–1022. doi: 10.1111/brv.12488
Wu L., Wang D., Evans J. A. (2019). Large teams develop and small teams disrupt science and technology. Nature 566 (7744), 378–382. doi: 10.1038/s41586-019-0941-9
Keywords: functional trait, functional analysis, butterfly, wing size, wingspan, forewing length
Citation: Viliani L, Bonelli S, Gentile G, Parile E and Riva F (2024) Diverging sub-fields in functional ecology. Front. Ecol. Evol. 12:1267605. doi: 10.3389/fevo.2024.1267605
Received: 26 July 2023; Accepted: 26 January 2024;
Published: 07 February 2024.
Edited by:
Pavel Kindlmann, Charles University, CzechiaReviewed by:
Tina Heger, Technical University of Munich, GermanyElise Anne Larsen, Georgetown University, United States
Copyright © 2024 Viliani, Bonelli, Gentile, Parile and Riva. This is an open-access article distributed under the terms of the Creative Commons Attribution License (CC BY). The use, distribution or reproduction in other forums is permitted, provided the original author(s) and the copyright owner(s) are credited and that the original publication in this journal is cited, in accordance with accepted academic practice. No use, distribution or reproduction is permitted which does not comply with these terms.
*Correspondence: Simona Bonelli, c2ltb25hLmJvbmVsbGlAdW5pdG8uaXQ=