- 1US Geological Survey, New Mexico Cooperative Fish and Wildlife Research Unit, Department of Fish, Wildlife, and Conservation Ecology, New Mexico State University, Las Cruces, NM, United States
- 2Department of Fish, Wildlife, and Conservation Ecology, New Mexico State University, Las Cruces, NM, United States
- 3New Mexico Department of Game and Fish, Santa Fe, NM, United States
Ungulates commonly select habitat with higher forage biomass and or nutritional quality to improve body condition and fitness. However, predation risk can alter ungulate habitat selection and foraging behavior and may affect their nutritional condition. Ungulates often choose areas with lower predation risk, sometimes sacrificing higher quality forage. This forage–predation risk trade-off can be important for life history strategies and influences individual nutritional condition and population vital rates. We used GPS collar data from adult female mule deer (Odocoileus hemionus) and mountain lions (Puma concolor) to model mule deer habitat selection in relation to forage conditions, stalking cover and predation risk from mountain lions to determine if a forage-predation risk trade-off existed for mule deer in central New Mexico. We also examined mountain lion kill sites and mule deer foraging locations to assess trade-offs at a finer scale. Forage biomass and protein content were inversely correlated with horizontal visibility, hence associated with higher stalking cover for mountain lions, suggesting a forage-predation risk trade-off for mule deer. Mule deer habitat selection was influenced by forage biomass and protein content at the landscape and within home range spatial scales, with forage protein being related to habitat selection during spring and summer and forage biomass during winter. However, mule deer selection for areas with better foraging conditions was constrained by landscape-scale encounter risk for mountain lions, such that increasing encounter risk was associated with diminished selection for areas with better foraging conditions. Mule deer also selected for areas with higher visibility when mountain lion predation risk was higher. Mountain lion kill sites were best explained by decreasing horizontal visibility and available forage protein, suggesting that deer may be selecting for forage quality at the cost of predation risk. A site was 1.5 times more likely to be a kill site with each 1-meter decrease in visibility (i.e., increased stalking cover). Mule deer selection of foraging sites was related to increased forage biomass, further supporting the potential for a trade-off scenario. Mule deer utilized spatio-temporal strategies and risk-conditional behavior to reduce predation risk, and at times selected suboptimal foraging areas with lower predation risk.
Introduction
When forage abundance or nutritional quality is limiting, ungulate resource selection should be focused towards areas with forage conditions that allow individuals to maximize nutritional condition. However, accessibility of forage can be moderated by environmental constraints, physiological tolerances, and interspecific interactions (Kie, 1999; Johnson et al., 2000; Dupke et al., 2016; Gedir et al., 2020). Predation risk can alter habitat selection and foraging behavior of prey species, and consequently affect their nutritional condition (Barten et al., 2001; Creel et al., 2005; Panzacchi et al., 2010; Dellinger et al., 2019). The effects of predation risk on prey behavior have been demonstrated in numerous species (e.g., Bleich et al., 1997; Altendorf et al., 2001; Creel and Christianson, 2008; Hay et al., 2008).
The effectiveness of proactive responses (e.g., habitat selection, shifting activity periods, and vigilance) used by prey to reduce risk of predation depends on the predictability of risk (Creel, 2018). If risk is predictable, prey can select habitat to avoid risky areas or adjust activity patterns so that use of riskier areas occurs at times when their primary predators are less active (Gehr et al., 2018; Kohl et al., 2018, 2019; Smith et al., 2019a). Coursing predators may be less predictable than stalking or ambush predators because they tend to be wide-ranging, and do not depend strongly on concealment cover or other habitat features to enhance hunting success (Peterson et al., 2021). In contrast, hunting by stalking predators is often associated with fine scale landscape features which may contribute to susceptibility of prey and predictability of risk (Laundré and Hernández, 2003; Schmidt and Kuijper, 2015). Predation risk for ungulates can be influenced by topographic features, vegetative characteristics and horizontal cover, distance to edge, season, time of day, and anthropogenic features (Atwood et al., 2009; DeCesare, 2012; Knopff et al., 2014). For stalking predators, these elements can influence where and when to search for prey, and alter hunting efficiency (Coon et al., 2020).
Under ideal conditions, ungulates would have access to high quality foraging areas with low risk of predation. For example, Pierce et al. (2004) reported that the highest quality forage for mule deer (Odocoileus hemionus) was in areas with lower predation risk from mountain lions (Puma concolor), thus mule deer were able to select higher quality foraging areas without an increased risk of predation. However, ungulates often choose areas with lower predation risk, sacrificing access to higher quality forage (Lima and Dill, 1990; Basille et al., 2015; Chitwood et al., 2022). For example, a mule deer population in Colorado selected better foraging areas but with higher risk of mountain lion predation, resulting in increased vigilance and less efficient foraging, thus there was a trade-off between efficient foraging and predation risk (Altendorf et al., 2001). Similar trade-offs between predation risk and forage have been documented in other ungulate species (Bleich et al., 1997; Hamel and Côté, 2007; Fortin and Fortin, 2009; Rignos, 2015). When forage-predation risk trade-offs occur, animals in better nutritional condition are more likely to avoid riskier areas than those in poor body condition (McNamara and Houston, 1986; Sinclair and Arcese, 1995; Rignos, 2015). Thus, ungulate nutritional condition, often related to proximity of the population to nutritional carrying capacity, can moderate ungulate responses to variable forage conditions and predation risk. At times and places where ungulates are forced to make a trade-off between higher quality foraging conditions and predation risk, it can have important implications for life history strategies with major effects on body condition of individuals, and subsequently population vital rates (Laundré et al., 2001; Hernández and Laundré, 2005; Christianson and Creel, 2010; Creel, 2018). For example, Monteith et al. (2014) reported that mule deer that migrated to summer ranges with superior forage conditions incurred significantly higher neonatal mortality from black bear (Ursus americanus) predation, resulting in lower overall productivity.
The influence of nutritional resources, environmental constraints, and interspecific interactions on habitat selection can vary with the behavioral state of animals. Habitat selection of foraging ungulates should be directed toward finding high quality forage, while simultaneously mitigating predation risk or thermoregulatory constraints. Conversely, habitat selection by satiated ungulates should be primarily driven by the need to find safe areas for resting and ruminating. The forage–rest/rumination cycle of many ungulate species typically has a distinct diel pattern with foraging bouts concentrated during crepuscular periods and resting–ruminating during midday (Sargeant et al., 1994; Roberts et al., 2016; Morano et al., 2019; Patten et al., 2019; Khan et al., 2023). Similarly, predators often have regular diel activity rhythms, with active hunting periods generally related to the time of day most favorable to their hunting style (e.g., stalking vs. coursing; Kohl et al., 2019). Thus, drivers of and constraints on resource selection of ungulates can vary across the diel cycle with selection patterns focused towards meeting behavior-specific resource needs and mitigating constraints imposed by the environment or interspecific interactions.
Heterogeneity in habitat conditions is linked to spatial and temporal variation in forage resources and predation risk (Brown and Litvaitis, 1995; Lowrey et al., 2019; Davidson et al., 2012; Gulsby et al., 2017; Smith et al., 2019b). Natural and anthropogenic disturbances contribute to habitat heterogeneity, influencing forage availability and nutritional quality for herbivores as well as habitat use by predators. Wildfire is one such disturbance, with the magnitude of the changes to habitat and forage conditions for herbivores varying with fire severity and post-fire recovery time (Proffitt et al., 2019; Roerick et al., 2019; Bristow et al., 2020; Hayes et al., 2022; Lewis et al., 2022). Predators also respond to fire-induced changes in habitat heterogeneity, particularly those that increase availability of prey that are attracted to increased forage in burned areas or accessibility of prey due to increased stalking cover resulting from post-fire recovery of woody shrubs (Smith et al., 2019b; Doherty et al., 2022; Gigliotti et al., 2022; Lewis et al., 2022; Suraci et al., 2022). Thus, wildfires or other landscape disturbances resulting in resource heterogeneity can cause favorable changes in forage conditions for herbivores, while simultaneously creating habitat conditions that increase hunting success of predators, which can contribute to the forage–predation risk trade-off for herbivores (Ganz et al., 2022; Lewis et al., 2022).
In this study, we collected field data on forage availability and nutritional quality, predation risk, risk-sensitive foraging site selection, and predation site characteristics, and we developed resource selection functions using GPS collar data from mule deer and mountain lions to examine the relative roles of predation risk and forage conditions on habitat selection of adult female mule deer. Our first objective was to determine if mule deer in our study area faced a trade-off between forage conditions and predation risk from mountain lions, the primary predator of adult deer in our study area. If this trade-off existed, we sought to examine how spatio-temporal variation of predation risk, biomass and nutritional quality of forage, and other habitat characteristics might affect mule deer resource selection. We expected mule deer to deal with the forage–predation risk trade-off in a context specific manner related to seasonal changes in forage conditions and variation in risk. We also expected that mule deer responses would vary by diel period, with responses to forage and predation risk most pronounced during the crepuscular periods when mule deer typically forage and by predation risk during crepuscular and nighttime periods when mountain lions are most active. We expected that during winter, mule deer would adjust habitat selection to prioritize acquisition of nutritional resources even at the cost of incurring higher predation risk from mountain lions. We expected that during the summer fawning season, female mule deer would prioritize reducing exposure to predation risk even at the expense of foraging opportunities in spite of the higher energy demands due to lactation. We also predicted that mule deer would increase selection for higher forage quality/quantity in areas of lower predation risk, and select for poorer forage conditions when predation risk is high. Finally, we expected that wildfire-burned areas would be a source of high quality forage and a risky area given that the post-fire recovery of woody browse that composes the bulk of mule deer diets can be used as stalking cover by mountain lions.
Materials and methods
Study area
We studied a non-migratory deer herd in the Gallinas Mountains area of the Cibola National Forest (Figure 1), near Corona, New Mexico. Elevation ranges from 1,912 to 2,631 m. Mean annual precipitation is 42.5 cm (± SD = 11 cm), with approximately 46% falling during the monsoon season from July to September (Climate data from Corona, NM, ca. 17 km east of the study area; elev. 2,054 m; WRCC, 2014). Average annual snowfall is 69.4 cm (± SD 47 cm). Average daily high and low temperatures are 7.6°C and −5.2°C, and 27.6°C and 11.6°C in January and June, respectively. Based on long-term precipitation and temperature data, seasons were designated as spring (April–June), summer (July–September), and fall–winter (October–March), which approximately corresponded with pre-parturition, parturition/lactation, and post weaning/reproduction, respectively.
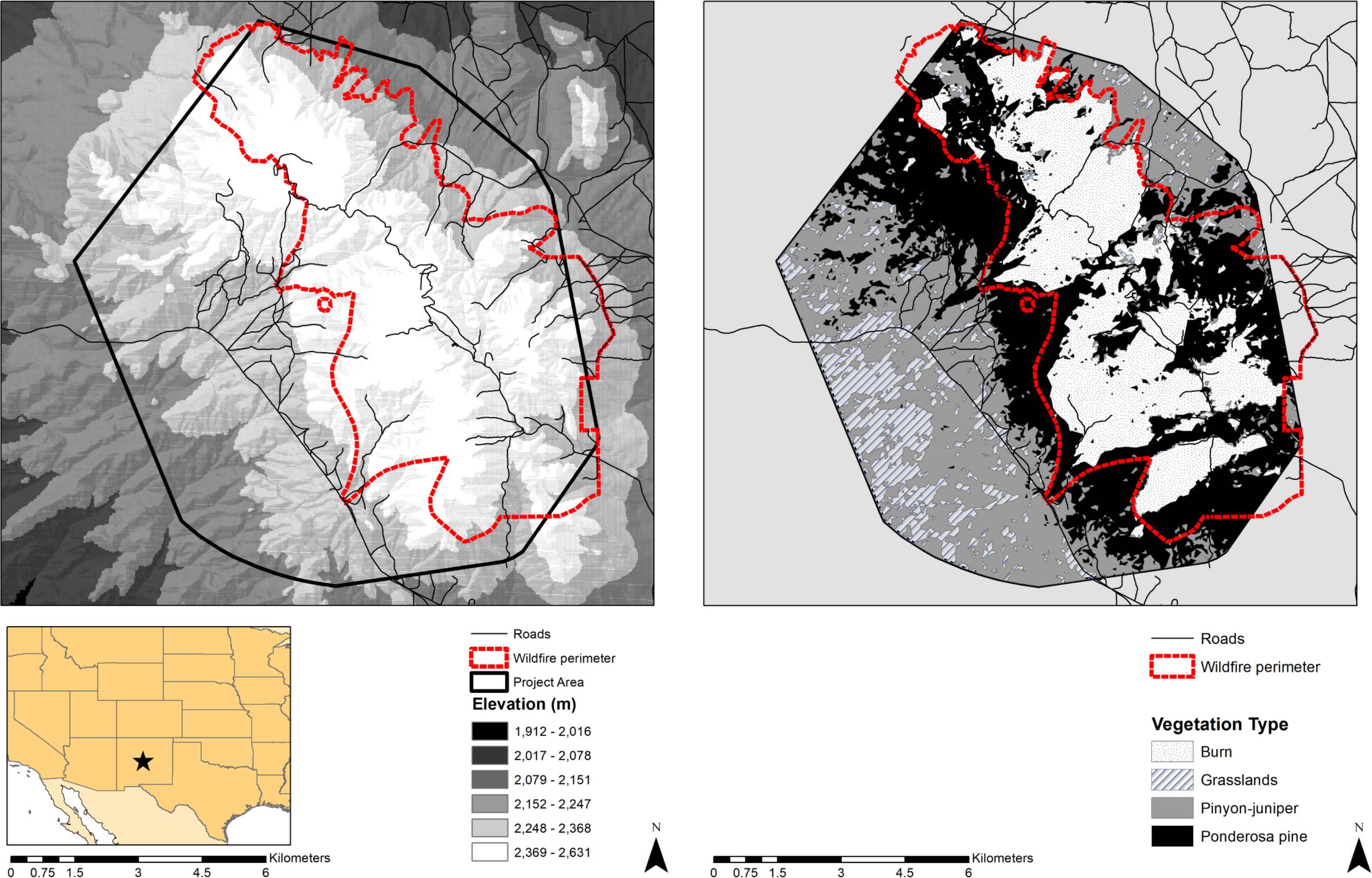
Figure 1 Mule deer and mountain lion study area in the Gallinas Mountains, central New Mexico USA. Left panel depicts the elevation gradient in the study area, while the right panel shows the vegetation types. Project area boundary, roads, and wildfire perimeter are depicted on both panels.
Only a few natural perennial water sources exist in the form of small springs surrounded by thick vegetation and rough terrain. The majority of perennial water sources are developed water catchments and dirt livestock tanks. Vegetation communities range from grasslands (10% of study area) at lower elevations, transitioning to pinyon-juniper (Pinus monophylla [P. edulis]–Juniperus spp.) woodland (30% of study area) at mid-elevations and ponderosa pine (Pinus ponderosa) forests at upper elevations (34% of study area) with scattered patches of Douglas fir (Pseudotsuga menziesii) and Engelman spruce (Picea engelmannii) at the highest elevations and on north facing slopes. Shrublands composed of Gambel oak (Quercus gambelii), wavyleaf oak (Quercus undulata), buckbrush (Ceanothus fendleri) and mountain mahogany (Cercocarpus montanus) were interspersed within the pinyon-juniper woodlands and ponderosa pine forests. Approximately 25% (29 km2) of the core study area was burned by stand-replacing wildfires within 17 years of our study (15 km2 in 2001, and 14 km2 in 2004). These burned areas remained in an earlier successional stage (i.e., shrubland) than the rest of the study area and were dominated by woody deciduous shrubs (i.e., buckbrush, oak, and mountain mahogany) that typically compose most of mule deer diets in the region.
Cattle ranching occurred on both private and public lands year-round. Big game and wild turkey (Meleagris gallopavo merriami) hunting was common, as was occasional use of the area by other recreationists; legal deer harvest was restricted to adult males. In the Gallinas Mountains, the primary predator of adult mule deer were mountain lions. Annual survival rates of adult female mule deer in the Gallinas Mountains varied between 0.73 and 0.86 with 39% (n = 7) of mortalities of collared mule deer attributed to mountain lion predation, 28% (n = 5) from malnutrition, 5% (n = 1) due to other causes; cause of death for 27% (n = 5) of collared deer mortalities was undetermined (Kay, 2018). Mountain lion kill rates averaged 0.85 deer/week with 67% of mountain lion kills being mule deer (Kay, 2018). Additional predator species of neonatal deer included black bear, bobcat (lynx rufus) and coyote (Canis latrans). A resident elk (Cervus canadensis) herd was also present.
Animal capture and handling
In January–February 2015, we captured 43 adult female mule deer (> 2 years old) via netgun or dart gun fired from a helicopter. We physically restrained deer captured via net gun and immobilized darted deer with 0.03 mg/kg carfentanil + 0.7 mg/kg xylazine, reversed with 3 mg/kg naltrexone + 0.125 mg/kg yohimbine or 0.15 mg/kg thiafentanil + 1 mg/kg xylazine, antagonized with 2 mg/kg naltrexone + 0.125 mg/kg yohimbine. From April 2015 through June 2016, we also darted an additional 14 adult female mule deer from the ground to re-deploy collars from deer that died during the study. These deer were primarily immobilized with 1.5 cc BAM (butorphanol 27.3 mg/ml, azaperone 9.1 mg/ml and medetomidine 10.9 mg/ml), and reversed with 3 cc atipamezole (25 mg/ml) and 0.5 cc of naltrexone (50 mg/ml), or occasionally with thiafentanil (0.15 mg/kg) + xylazine (1 mg/kg), and antagonized with naltrexone (2 mg/kg) and yohimbine (0.125 mg/kg). We fitted 31 deer with GPS store-on-board collars, while 26 were fitted with VHF collars. We programmed GPS collars with a 5-hr fix interval to assess habitat selection patterns in relation to forage conditions and predation risk from mountain lions. We used VHF-collared deer to locate foraging sites of mule deer and for survival and cause-specific mortality (CSM) monitoring (see Kay, 2018 for details of survival and CSM results).
We placed GPS-Iridium collars on mountain lions to investigate prey composition, kill site characteristics, and to model landscape-scale predation risk. We utilized a combination of foot snares and hounds to capture and collar 5 adult mountain lions (3 female, 2 male). We immobilized lions using ketamine (3.0 mg/kg) and medetomidine (0.07 mg/kg) reversed with atipamezole (0.375 mg/kg). We programmed GPS collars on mountain lions with a 3-hr fix interval and transmitted the data every 1–3 days through the Iridium satellite system. All capture and handling procedures were approved by the New Mexico State University Institutional Animal Care and Use Committee (Protocol #2014-041).
Habitat selection covariates, forage conditions and predation risk
Human disturbance, water, and topography
We mapped all open roads, human developments and perennial water sources using a combination of existing GIS data, satellite imagery, and ground data. We checked perennial water sources monthly throughout the study to ensure they consistently had water available. Ephemeral sources were not included in our analyses. We calculated distance from roads, developments, and perennial water in ArcGIS Desktop version 10.6 (Esri, Redlands, CA). We expected that mule deer would select areas closer to perennial water during summer, but that water would have less or no influence on habitat selection during winter and spring.
We used USGS digital elevation models with 30 x 30 m resolution to determine slope (degrees), aspect, and elevation (m). We converted aspect to eastness and northness using sine and cosine transformations, respectively resulting in values between −1 and 1, where −1 is west or south and 1 is east or north. We estimated vector ruggedness measure (i.e., terrain ruggedness; VRM) with the digital elevation model and a 19 x 19 pixel moving window in ArcGIS (Sappington et al., 2007). We created a topographic position index (TPI) using the digital elevation model with a 15 x 15 pixel moving window to categorize the study area as drainage, hill slope, or ridgetop (Jenness, 2006). A 15 x 15 moving window for TPI better represented the major landforms (i.e., drainages/valleys, hillsides, and ridges) in the study area than the 19 x 19 window used for VRM. Rather than bin the TPI values into a categorical covariate, we left the data as a continuous variable where positive values corresponded with ridgetops, values near 0 were slopes, and negative values corresponded to valleys or drainages. Based on previous research, we expected that mule deer and mountain lions would select for areas with intermediate terrain ruggedness, and that mule deer would select north facing slopes in summer due to higher forage abundance, but would avoid north aspects in winter due to deeper snow. We obtained percent tree canopy cover from the National Land Cover Database (Homer et al., 2004).
Vegetation cover type, forage characteristics and stalking cover
We classified vegetation type using existing data from the US Geological Survey (USGS) Gap Analysis Program (Lowry et al., 2005). Vegetation maps were condensed and reclassified into 4 vegetation types: pinyon-juniper woodland, ponderosa/mixed conifer forest, grassland, and burned areas. We confirmed vegetation map accuracy on the ground by randomly locating 200 points within the study area. We visited each point to ensure the correct classification rate for the vegetative communities was > 90%. We classified fire history as burned or unburned within 17 years prior to data collection, with the two largest stand-replacing wildfires occurring in 2001 and 2004 (MTBS Data Access: Fire Level Geospatial Data, 2017). We expected mule deer to select for vegetation types (i.e., pinyon-juniper woodland and burned areas) providing a combination of security cover and high forage abundance (Pierce et al., 2004; Watkins et al., 2007; Roerick et al., 2019; Johnston and Anderson, 2023). However, areas with security cover utilized by mule deer can also be used as stalking cover by mountain lions, so we expected that there would be a trade-off between access to high quality foraging areas and using risky areas with high concealment cover for mountain lions or low visibility for mule deer. We also expected that mule deer access to forage in areas with low visibility would be moderated by landscape-scale predation risk from mountain lions (see below).
We sampled 100 m transects throughout the study area to measure horizontal visibility as an index for stalking cover and to estimate edible forage biomass (i.e., grasses, forbs, and current year’s growth, leaves and twigs <5 mm in diameter for browse), digestible energy and digestible protein content. Our forage sampling targeted plant species that were known to contribute >2% of seasonal mule deer diets and were confirmed with microhistological analysis (see Supplementary Material 1 for details; Kay, 2018). Stalking cover is the inverse of horizontal visibility (m) at a given point based on concealment cover (e.g., terrain or vegetation features) where a higher value indicates increased visibility, hence low stalking cover.
We developed generalized linear models in R (R Core Team, 2023) to estimate forage biomass, digestible energy and digestible protein (g/m2) and horizontal visibility across the study area based on spatial and temporal attributes from the vegetation transects. Covariates included vegetation type, elevation, canopy cover, ruggedness, topographic position, slope and aspect. We also compiled 250 x 250 m Moderate-Resolution Imaging Spectroradiometer raw reflectance data for each 8-day period during the study and calculated the Normalized Difference Vegetation Index (NDVI) for each 8-day period (NASA LP DAAC, 2015). We then calculated the mean NDVI values and NDVI rate of change (ΔNDVI) for each season and transect location (Pettorelli et al., 2011).
We pooled the data on horizontal visibility (i.e., inverse of stalking cover) across the two years and divided it into seasons (i.e., spring, summer, winter), whereas we ran separate models for each season in each year for edible forage biomass, digestible energy and digestible protein (See Supplementary Material 1 for details). We examined pair-wise correlations between covariates and did not include correlated variables (i.e., r ≥ |0.65|) in the same model. We also assessed multicollinearity of the most supported models by calculating variance inflation factors (VIF). We then created GIS surfaces for horizontal visibility, forage biomass, and biomass-specific estimates of digestible energy and protein utilizing raster calculator in ArcGIS based on topographical and vegetative geospatial data and their respective coefficients included in top models (Supplementary Material 1 Tables 1.1–1.6). These raster layers were created at 30 x 30 m resolution and used as predictor variables for mule deer habitat selection.
Mountain lion predation risk
We created a predation risk index for mountain lions that incorporated both a resource selection function (RSF) and probability density functions using kernel estimators (Hebblewhite and Merrill, 2007); separate predation risk indices were estimated for each season and diel period. To estimate the RSF, we censored the first 5 days of GPS collar data post-capture to avoid potential behavioral anomalies resulting from capture. We then generated 5 random points for each GPS location within a 99% kernel home range created from all mountain lion locations using the adehabitat package (Calenge, 2006) in program R (R Core Team, 2023). We used civil twilight times obtained from the US Naval Observatory (2014) to classify diel periods as night, day, and crepuscular, with the crepuscular periods being three hours after civil dawn and three hours before civil dusk; we coded data by season and diel period. Based on previous research on mountain lion habitat selection, we included canopy cover, horizontal visibility, TPI, slope, northness, VRM, distance to human structures and roads, and elevation as predictor variables in a suite of a priori models for our mountain lion RSFs (Robinson et al., 2015; Blake and Gese, 2016; Dellinger et al., 2020; Peterson et al., 2021; Supplementary Material 2 Table 2.1). We expected that mountain lions would select habitat characteristics that would enhance hunting success, including areas with high vegetation concealment cover and low horizontal visibility (i.e., high canopy cover, low visibility, north facing slopes and higher elevations with higher vegetation biomass) and topographic features that would facilitate hunting (i.e., steep slopes, terrain ruggedness) and movements (e.g., ridgelines and drainages). We also expected that mountain lions would avoid areas near roads and other human developments. We expected that some habitat characteristics could have non-linear relationships with resource selection of mountain lions, and that this may vary by season or diel period. Therefore, we evaluated preliminary models using Akaike’s Information Criterion corrected for small sample size (AICc) and AICc weights to compare structures with both linear and quadratic terms for slope, canopy cover, VRM, elevation, and horizontal visibility (Supplementary Material 2 Table 2.1). Preliminary analyses indicated that canopy cover, specifically intermediate levels of canopy cover, was highly predictive of landscape-scale mountain lion habitat selection so a quadratic term for canopy cover was included in all mountain lion RSF models. For a couple of diel/seasonal periods, the model with only linear terms for elevation, VRM, and visibility performed as well as or better than the model with the quadratic terms and we incorporated these into the model structures we evaluated. We scaled continuous covariates by subtracting their mean and dividing by two standard deviations (Gelman, 2008) and only included covariates in the same model that were not collinear (e.g., r < |0.65| and VIF <4.0). We estimated RSF coefficients for lions using mixed-effects logistic regression including a random intercept for individual lions (Breslow and Clayton, 1993; Gillies et al., 2006) with lme4 (Bates et al., 2015) in R (R Core Team, 2023). We used AICc to evaluate model support for each season and diel period model set, and used multi-model averaging for parameter estimates when there was model uncertainty (i.e., > 1 model had ΔAICc ≤ 2; Burnham and Anderson, 2002). We evaluated competitive models for uninformative parameters and models with uninformative parameters were discarded (Arnold, 2010). We used five-fold cross-validation (Boyce et al., 2002) to evaluate predictive performance of the most supported models.
We created a 99% kernel density estimate for mountain lions using Home Range Tools in ArcGIS (Rodgers et al., 2007; Supplementary Material 2 Figure 2.1). Total predation risk was calculated as the product of the season by diel period RSF and kernel density functions (Hebblewhite and Merrill, 2007). This GIS surface was then scaled to values ranging from 0 to 1 using a linear stretch equation (Lillesand and Kiefer, 1994) and utilized as a predictor variable that represented landscape-scale mountain lion predation risk, or the relative probability of mule deer encountering a mountain lion for the mule deer habitat selection analysis (Supplementary Material 2 Figure 2.2). We set multiple camera traps and snares across the study area in travel corridors and at locations where we observed lion sign. Additionally, we conducted foot surveys for mountain lion sign 2–6 days/week (during spring, summer and fall), set cameras on mountain lion kills, and traveled the road network in winter to look for tracks in the snow. Collared lions frequently showed up on cameras, and we detected their tracks often during surveys (verified by matching up GPS collar locations to tracks). All mountain lion sign observed at cameras and during track surveys were associated with collared lions; we did not detect any uncollared lions during our study period.
Mule deer resource selection
We used GPS collar data to develop RSFs for mule deer at the landscape and within home range scales (2nd and 3rd order; Johnson, 1980). We omitted the GPS locations for the first 5 days post-capture. We then determined habitat availability at the landscape scale by amalgamating the GPS locations from all mule deer over the entire study period and estimated 99% seasonal kernel home ranges with the adehabitat package (Calenge, 2006) in program R (R Core Team, 2023). For the within home range scale, we similarly estimated 99% seasonal kernel home ranges for each mule deer. Within the landscape-scale and individual kernel home ranges, we generated 5 random locations for each GPS location from collared mule deer. We selected predictor variables for RSF models based on previous research and mule deer life-history traits (Ager et al., 2003; Pierce et al., 2004; Bishop et al., 2009; Monteith et al., 2014) and used a combination of remote sensing and on-the-ground methods to gather data on habitat characteristics.
We used a similar approach to model mule deer habitat selection as described above for mountain lions. We used mixed-effects logistic regression and included a random intercept for individual deer (Breslow and Clayton, 1993; Gillies et al., 2006) with lme4 (Bates et al., 2015) in R (R Core Team, 2023). Prior to analyses, we tested predictor variables for collinearity and standardized all continuous predictor variables. Due to temporally changing habitat conditions, we conducted all habitat selection analyses on a seasonal and diel basis. We developed a priori model sets to assess resource selection of mule deer relative to predation risk, forage conditions, and habitat characteristics (Supplementary Material 3 Table 3.1). Forage availability and nutritional quality are two common currencies used to assess nutritional constraints on herbivore foraging and habitat selection. Therefore, we developed model structures that included covariates related to predation risk and other non-forage-related habitat characteristics. We then added either edible forage biomass or forage protein content to these base models for seasonal mule deer habitat selection to determine which forage metric (biomass vs. protein content) was most related to seasonal habitat selection (Supplementary Material 3 Table 3.1). We did not use digestible energy content of forage in our mule deer RSF models because forage biomass and digestible energy content were highly correlated (all r > 0.9). Additionally, our models predicting digestible energy content of forage using transect and geospatial covariates performed worse (all r2 = 0.45) than our models for edible forage biomass (r2 = 0.70) and digestible forage protein (r2 = 0.73; Supplementary Material 1 Table 1.1). We therefore compared seasonal models of mule deer habitat selection with forage protein content to those with edible forage biomass (along with other predation risk and habitat covariates). We selected top models utilizing AICc to determine which predictor variables best explained mule deer habitat selection. We then used five-fold cross-validation to evaluate the performance of top-ranking models (Boyce et al., 2002).
Vegetation type appeared to be an important factor for deer in our study area due to the quantity and quality of forage. However, continuous nutritional covariates (i.e., edible biomass and digestible protein; Supplementary Material 1 Figures 1.1–1.2) were not independent of vegetation type, and therefore we used continuous forage metrics in the resource selection analysis to reduce the number of parameters estimated and simplify interpretation. Horizontal visibility was also related to vegetation type with the lowest visibility in burned areas, intermediate in pinyon-juniper and ponderosa stands and highest in grasslands (Supplementary Material 1 Figure 1.3). To assess broad selection patterns by mule deer with respect to vegetation type, including wildfire-burned areas, we analyzed mule deer selection for specific vegetation types using wi function (design II data) in the adehabitatHS package in R (Calenge, 2006) to calculate selection ratios of vegetation types (Manly et al., 2002).
Characteristics of mountain lion kill sites and mule deer foraging sites
To further examine forage–predation risk trade-offs, we collected data at mountain lion kill sites to determine the habitat characteristics that best predicted the likelihood of an area being a kill site (e.g., higher predation risk, lower visibility). To identify potential kill sites for field visitation, we evaluated GPS clusters (Knopff et al., 2009; Ruth and Murphy, 2010) using a cluster detection code and mountain lion GPS collar data (Kindschuh et al., 2016) in R (R Core Team, 2023). Potential clusters or predation events were defined as ≥ 2 GPS points within 200 m of each other that were recorded within a 72-h period (Anderson and Lindzey, 2003). We also used radio-collared deer killed by lions during our study in this analysis. We determined cause of death by field necropsy (Kay, 2018). We skinned the head and neck of each deer to examine potential subcutaneous hemorrhaging (hemorrhaging distinguished predation from scavenging) and we palpated all extremities for physical trauma and signs of predation. Additionally, we searched the mortality site for other indicators of predation such as predator tracks, feces, drag marks, or cache piles.
At each kill site where we confirmed the location of a carcass, we backtracked from the cached carcass until we found a point where we felt confident the prey had first fallen in the predation sequence. These points were located by observing tracks of both mountain lions and prey, as well as other signs found in the surrounding area (e.g., broken vegetation, hair and blood). We defined this as the actual kill site and measured micro-habitat characteristics at this location. While this point may not represent exactly where the deer was when the attack initiated, it is probably closer to the attack site than where the cached carcass was located during field visits. Laundré and Hernández (2003) reported that mountain lions typically made contact with a deer within 10 m of its initial pursuit location, and that mule deer traveled from 10–15 m after initial contact. If we did not have high confidence that our estimated attack location was where the animal first fell, we did not survey the site. From the determined site, we then generated a random location between 100 and 550 m away at a random azimuth to use as a matched-paired random point. We selected the minimum of 100 m to avoid potential overlap of sampling between kill and random sites and the maximum 550 m distance was based on vegetation heterogeneity in the study area. At both kill sites and random sites, we classified the dominant vegetation type within a 50-m radius, measured horizontal visibility and estimated edible biomass of forage species using the modified comparative yield method and dry weight rank multipliers using two quadrats (1 m3) in each cardinal direction (8 total) at 10 and 20 m from the kill or random site (t’Mannetje and Haydock, 1963; Haydock and Shaw, 1975; Mazaika and Krausman, 1991; Marshal et al., 2005). We then estimated biomass-specific digestible protein and digestible energy based on nutritional content analyses of forage samples (See Supplementary Material 1 for details on visibility and forage sampling).
To evaluate habitat characteristics associated with mule deer foraging sites, we used telemetry to track collared deer during crepuscular hours. When we located undisturbed foraging deer, we recorded the location and returned within a week to record micro-site characteristics. We never used the same deer twice when locating foraging sites. Similar to lion kill sites, we also generated a paired random point within 100–550 m from the foraging site. Data collected at foraging sites were the same as for mountain lion kill sites.
We then used conditional logistic regression with the clogit function in the survival package in R (Therneau and Lumley, 2015) to evaluate a suite of a priori models (Supplementary Material 4 Table 4.1) to determine which habitat characteristics best predicted mountain lion kill sites and mule deer foraging sites. Biomass and digestible energy content estimates of forage were highly correlated at both kill sites (r = 0.88) and foraging sites (r = 0.84), so we only included biomass and forage protein content in modeling mountain lion kill sites and mule deer foraging sites. We used AICc to evaluate model support and used multi-model averaging across all models for parameter estimates when there was model uncertainty (i.e., > 1 model had ΔAICc ≤ 2; Burnham and Anderson, 2002).
To determine if mule deer were forced to make a trade-off between forage and predation risk, we tested for correlation between forage biomass, protein, digestible energy content and horizontal visibility estimates from our transect locations where we sampled forage conditions and horizontal visibility. We extracted our mountain lion predation risk index to transect locations. If a correlation between forage and predation risk metrics existed, we utilized parameter estimates from our top deer habitat selection models to evaluate how mule deer in our study area approached this trade-off.
Results
Mountain lion habitat selection
In 8 of the 9 model sets, there was a clear top model. Mountain lion habitat selection was broadly similar across seasons and diel periods (Supplementary Material 2 Table 2.2–2.3). Five-fold cross-validation analyses indicated that the most supported model for each model set performed well (ρ > 0.80 for top models).
Mule deer habitat selection
Landscape scale
The relative probability of use for mule deer at the landscape scale was best explained by terrain ruggedness, distance to water, northness, mountain lion predation risk, horizontal visibility and interactions between predation risk and horizontal visibility. There was relatively strong support for the highest-ranking seasonal RSF models in each diel period (wi ≥ 0.7). When there were competitive models (e.g., AICc ≤ 2.0) in the model sets, the two highest ranking models had similar structures with differences generally related to the presence or absence of interaction terms between mountain lion predation risk and horizontal visibility or between mountain lion predation risk and either forage protein content or edible forage biomass (Supplementary Material 3 Table 3.2). Five-fold cross-validation indicated that the most supported model for each season and diel period performed well (ρ > 0.91 for the most supported models). Mule deer disproportionately selected areas previously burned by wildfires over every other vegetation type across all seasons and diel periods (Supplementary Material 3 Figure 3.1); wildfire burned areas had higher edible biomass and protein content for woody browse, but some of the lowest horizontal visibility (Supplementary Material 1 Figures 1.1-1.3).
At the landscape scale, mule deer consistently selected for areas with lower visibility (i.e., increased stalking cover), intermediate ruggedness, areas near perennial water, and south-facing aspects (Supplementary Material 3 Table 3.3; Figure 2). The main effect of predation risk was in the most supported models in all 9 model sets (Supplementary Material 3 Table 3.2), although for some seasons and diel periods, the confidence intervals for main effect of predation risk included 0 (Figure 2). More importantly, interaction terms between predation risk and forage protein content and predation risk and horizontal visibility in these same models, indicated that spatial and temporal heterogeneity in mountain lion predation risk across the landscape (Supplementary Material 2 Figure 2.2) influenced mule deer habitat selection by moderating the influence of forage protein content and horizontal visibility. We also observed significant interactions between terrain ruggedness and horizontal visibility (Supplementary Material 3 Table 3.3).
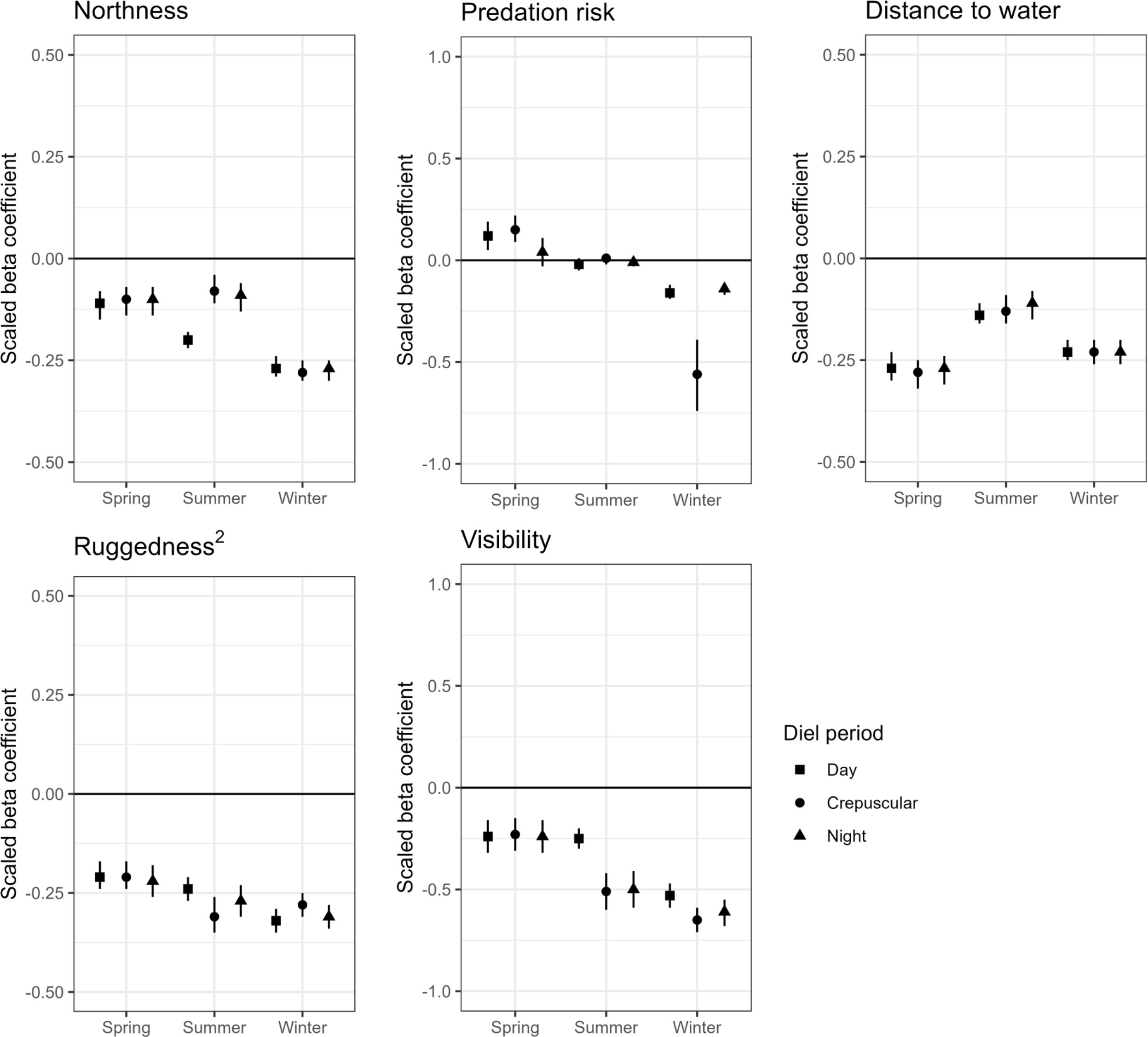
Figure 2 Standardized beta coefficient estimates and 95% confidence intervals for resource selection functions at the landscape scale for adult female mule deer in the Gallinas Mountains, New Mexico, 2015–2016. Covariates include northness, mountain lion predation risk, distance to perennial water, quadratic terrain ruggedness, and horizontal visibility (inverse of stalking cover). Note that the axes for the scaled beta coefficients depicted on each panel may be on different scales.
During spring and summer, RSF models including forage protein content were more supported than those with edible forage biomass. However, during winter, models with edible forage biomass were the most supported models during the day and nighttime, with the model with forage protein content ranked highest during the crepuscular periods (Supplementary Material 3 Table 3.2). During all seasons at the landscape scale, the relative probability of selection for mule deer increased with increasing forage protein content (g/m2) but mountain lion predation risk had a dampening effect on this response with greater increases in the relative probability of selection by mule deer as predation risk decreased (Figure 3; Supplementary Material 3 Figure 3.2).
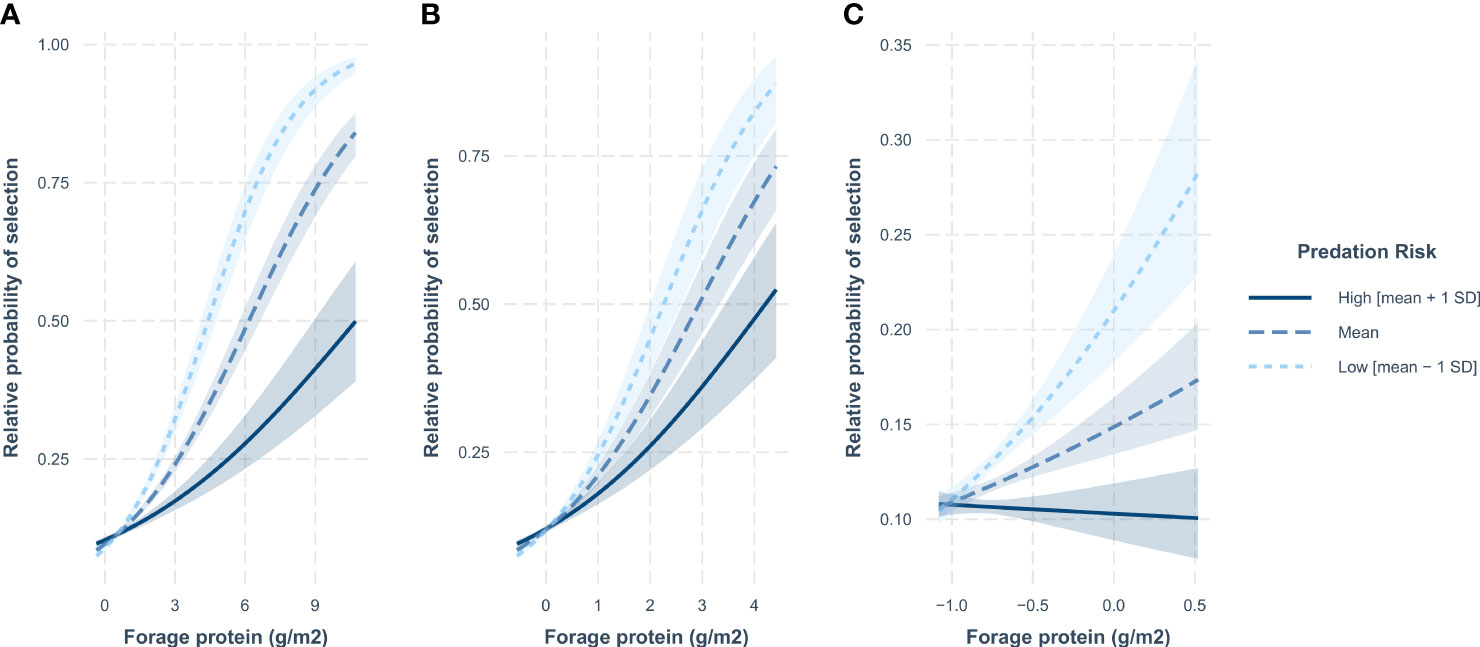
Figure 3 Predicted relative probability of selection by mule deer at the landscape scale in relation to forage protein content (g/m2) as a function of mountain lion predation risk during (A) spring, (B) summer, and (C) winter crepuscular diel periods in the Gallinas Mountains, New Mexico, 2015–2016. Confidence bands are 90% confidence intervals. The relationship between predicted relative probability of selection and forage protein content are plotted for three levels of mountain lion predation risk (mean predation risk [mean], low predation risk [mean − 1 standard deviation SD] and high predation risk [mean + 1 SD]). Note that the axes for the relative probability of selection depicted on each panel may be on different scales.
We also found an interaction between horizontal visibility and mountain lion predation risk, but when present in the top models, the nature of this relationship varied across seasons. During the daytime in spring, the relative probability of selection decreased with increasing mountain lion predation risk, but less so when visibility was lower; however, confidence intervals were wide (Figure 4A). Whereas during summer, the relative probability of selection increased with mountain lion predation risk when visibility was high, but decreased when visibility was low (Figure 4B). During day and night time in winter, relative probability of selection increased with increasing predation risk when visibility was high, but declined when visibility was average to low (Figures 4C, D; Supplementary Material 3 Figure 3.3D, E).
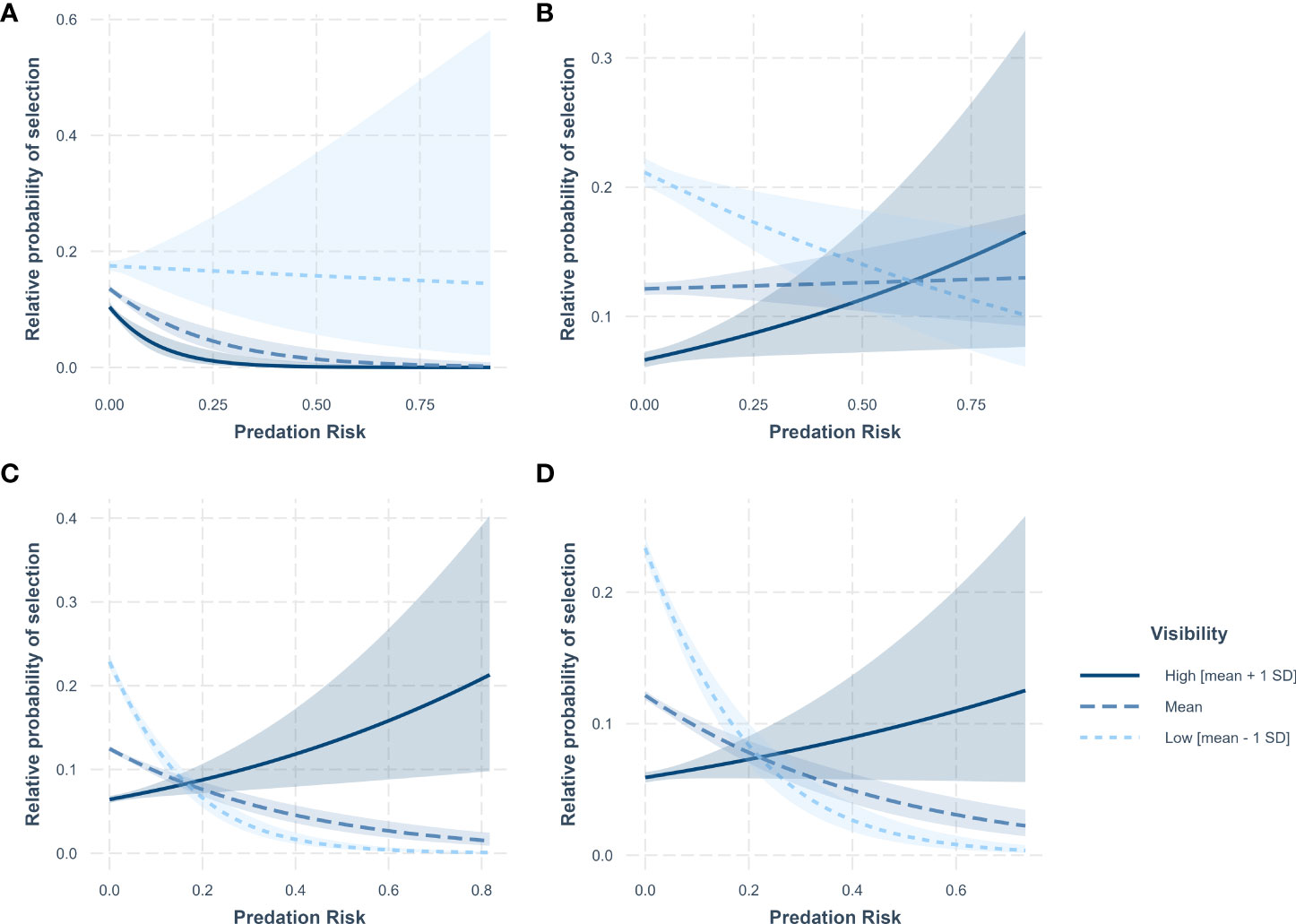
Figure 4 Predicted relative probability of selection by mule deer in relation to mountain lion predation risk as a function of horizontal visibility at the landscape scale during (A) spring – day, (B) summer – crepuscular, (C) winter – day, and (D) winter – night diel periods in the Gallinas Mountains, New Mexico, 2015–2016. Confidence bands are 90% confidence intervals. The relationship between predicted relative probability of selection and mountain lion predation risk is plotted for three levels of horizontal visibility (mean visibility [mean], low visibility [mean − 1 standard deviation SD] and high visibility [mean + 1 SD]). Note that the axes for the relative probability of selection depicted on each panel may be on different scales.
Relative probability of selection for mule deer generally decreased with increasing horizontal visibility, but the influence of horizontal visibility depended on terrain ruggedness (visibility × VRM interaction) in all diel periods. During spring, selection was highest at low visibility when terrain ruggedness was highest (Supplementary Material 3 Figure 3.4A–C). Similarly, in summer and winter, terrain ruggedness moderated the effects of horizontal visibility on the relative probability of selection (Supplementary Material 3 Figure 3.4D–I).
Within home range scale
The most supported models for mule deer habitat selection at the within home range scale had similar model structures as the most supported models at the landscape scale (Supplementary Material 3 Tables 3.2, 3.4). Five-fold cross-validation analyses indicated good model performance for the highest-ranking model for each season and diel period (ρ > 0.78 for the most supported models). At the within home range scale, mule deer habitat selection was related to terrain ruggedness, distance to water, northness, forage conditions and predation risk (Figure 5; Supplementary Material 3 Table 3.5). Similar to landscape scale, there were significant interactions between predation risk and forage protein content, predation risk and edible forage biomass, and terrain ruggedness and horizontal visibility. Some interactions between predation risk and horizontal visibility had confidence intervals that included 0 (Figure 5; Supplementary Material 3 Table 3.5). During spring and summer, models with forage protein content had more support than those with edible forage biomass. In winter, models with edible forage biomass were more supported compared to similar models with forage protein content (Supplementary Material 3 Table 3.4). The relative magnitude of parameter estimates within model sets was lower at the within home range scale than the landscape scale for VRM and VRM2 during summer and winter, and for distance to perennial water and north-facing aspects for all seasons and diel periods (Supplementary Material 3 Table 3.5).
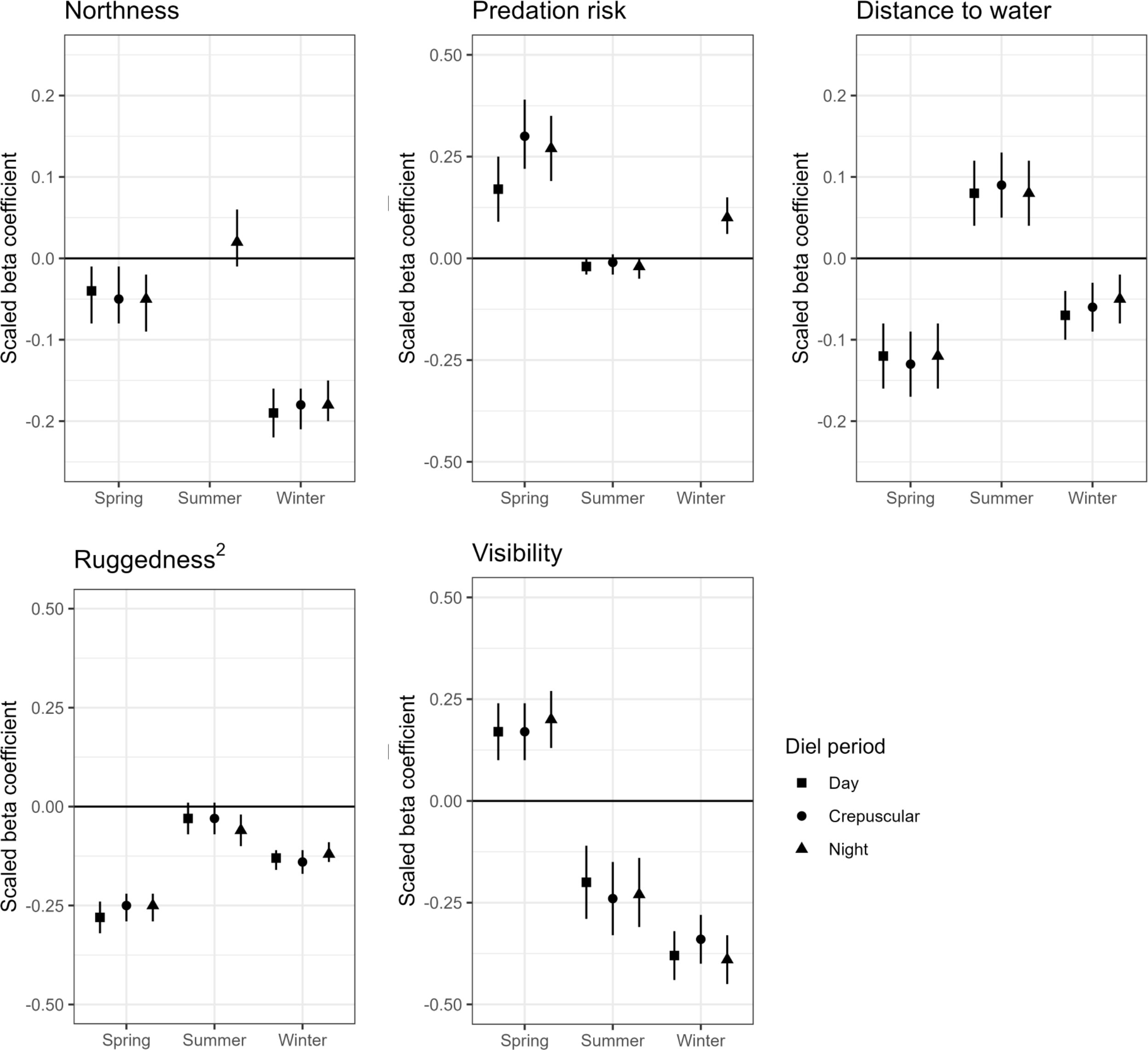
Figure 5 Standardized beta coefficient estimates and 95% confidence intervals for resource selection functions at the within home range scale for adult female mule deer in the Gallinas Mountains, New Mexico, 2015–2016. Covariates include northness, mountain lion predation risk, distance to perennial water, quadratic terrain ruggedness, and horizontal visibility (inverse of stalking cover). Some panels are missing coefficient estimates for some predictor variables because those variables were not in the most supported model for that season. Note that the axes for the scaled beta coefficients depicted on each panel may be on different scales.
At the within home range scale, relative probability of selection was highest at intermediate ruggedness (Figure 5). During spring and winter, mule deer selected areas closer to perennial water and avoided north-facing slopes. During summer, selection increased slightly as distance to water increased (Figure 5; Supplementary Material 3 Table 3.5). At the landscape scale, relative probability of selection increased with decreasing visibility, whereas at the within home range scale, selection increased in spring with increasing visibility (Figure 5; Supplementary Material Table 3.5).
The effect of forage protein content on relative probability of use showed a similar pattern of being moderated by mountain lion predation risk, although there was substantial overlap in the 90% confidence intervals (Supplementary Material 3 Figure 3.5A–C). During spring, relative probability of selection increased with forage protein content. However, during summer, the relative probability of selection for mule deer decreased with increasing forage protein content, but with greater declines in the relative probability of use in areas with high predation risk (Supplementary Material 3 Figure 3.5D–E); however, 90% confidence intervals overlapped. During winter, the relative probability of selection was related to edible forage biomass rather than forage protein content, with mule deer selecting areas with higher forage biomass (Supplementary Material 3 Table 3.5). During nighttime in winter, the influence of forage biomass on relative probability of selection depended on predation risk, with mule deer selecting areas of higher forage biomass at higher levels of mountain lion predation risk (Figure 6).
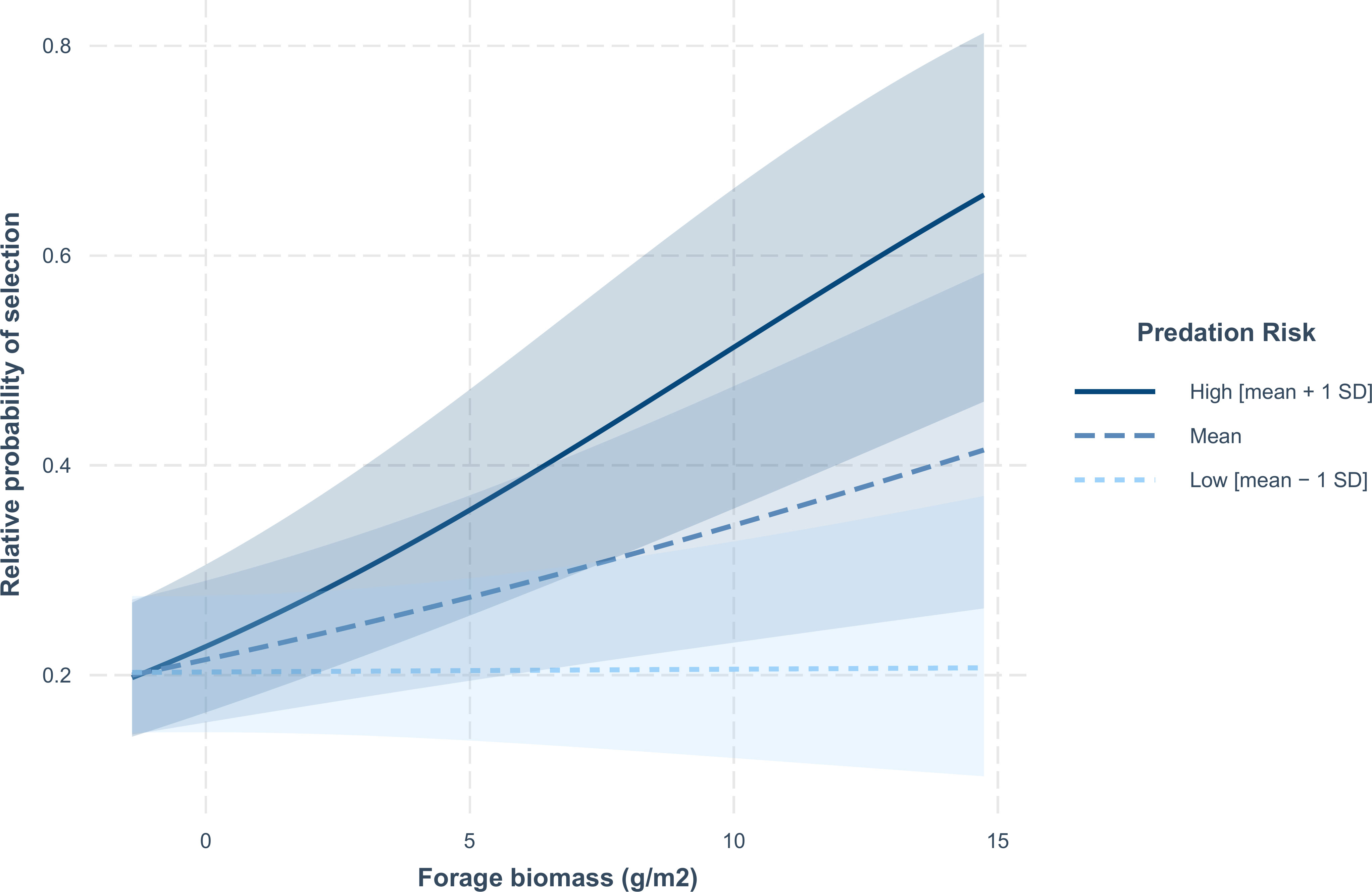
Figure 6 Predicted relative probability of selection by mule deer in relation to forage biomass at the within home range scale during the winter crepuscular period in the Gallinas Mountains, New Mexico, 2015–2016. Confidence bands are 90% confidence intervals. The relationship between predicted relative probability of selection and forage biomass is plotted for three levels of mountain lion predation risk (mean predation risk [mean], low predation risk [mean − 1 standard deviation SD] and high predation risk [mean + 1 SD]).
The influence of horizontal visibility on relative probability of selection also depended on terrain ruggedness in all diel periods at the within home range scale. During spring, the relative probability of selection decreased with increasing visibility in areas with low terrain ruggedness and increased in areas with high ruggedness (Supplementary Material 3 Figure 3.6A–C). During summer, the relative probability of selection decreased with increasing visibility for average and high terrain ruggedness (Supplementary Material 3 Figure 3.6D–F). In winter, the relative probability of selection was highest in areas with low visibility when ruggedness was high, but the relative probability of selection decreased with increasing visibility for all levels of terrain ruggedness (Supplementary Material 3 Figure 3.6G–I).
Kill and foraging site microhabitat characteristics
We were able to identify 33 kill sites and 22 foraging sites. The top model predicting kill sites included horizontal visibility and digestible protein of forage (g/m2; Supplementary Material 4 Table 4.2). Although there was some model selection uncertainty, horizontal visibility was in all models that carried any model weight (Supplementary Material 4 Table 4.2). Sites were less likely to be a kill site as horizontal visibility increased (i.e., stalking cover declined); there was more horizontal cover in areas where deer were killed by lions (Supplementary Material 4 Table 4.3). A site was 34% less likely to be a kill site with each 1-meter increase in visibility. Mean [95% CI] horizontal visibility at kill sites was 10.47 m [9.53–11.41 m] compared to 14.43 m [12.8–16.06 m) at random points. Digestible protein (g/m2) was also positively associated with kill sites, but parameter estimates overlapped zero. Mean forage protein was almost 5 times higher (9.60 g/m2 ± 1.87 SE; range 0.45 to 49.1 g/m2) at kill sites compared to random sites (1.93 g/m2 ± 0.282 SE; range 0.08 to 8.16 g/m2).
There was model uncertainty for mule deer foraging site models (Supplementary Material 4 Table 4.4); the highest-ranking models included edible forage biomass (g/m2), horizontal visibility, and digestible protein (g/m2). However, the parameter estimates for digestible protein and horizontal visibility included 0. The probability of a location being a foraging site was best explained by available forage biomass (Supplementary Material 4 Table 4.5). As edible forage biomass increases by 1 g/m2, the odds of a site being a foraging site increases by 2.2%. On average, foraging locations contained 229% (250.08 g/m2 ± 15.7 SE; range 128.7 to 433.6 g/m2) more edible forage biomass compared to matched paired sites (75.91 g/m2 ± 10.02 SE; range 9.03 to 202.64 g/m2). Available forage protein at foraging sites was 7.6 times (11.99 g/m2 ± 1.97 SE; range 0.46 to 39.02 g/m2) higher than random paired sites (1.39 g/m2 ± 0.28 SE; range 0.03 to 4.55 g/m2). Mean horizontal visibility at foraging sites was 12.6 m [9.26–11.23 m]) compared to 10.2 m [10.98–14.18 m]) at random sites.
Trade-off correlations
There was an inverse relationship between edible forage biomass and horizontal visibility at our forage and horizontal visibility transects (n = 112) during all seasons (winter: r = −0.521, P <0.001; spring: r = −0.573, P <0.001; summer: r = −0.627, P <0.01). Digestible energy (winter: r = −0.485, P <0.001; spring: r = −0.544, P <0.001; summer: r = −0.609, P <0.001) and protein (spring: r = −0.473, P <0.001; summer: r = −0.522, P <0.001) were also negatively correlated with horizontal visibility with the exception of forage protein during winter (winter: r = −0.045, P = 0.636). Diel landscape-scale mountain predation risk indices had a moderate negative association with horizontal visibility during spring crepuscular (r = −0.202, P = 0.037), spring night (r = −0.252, P = 0.009), and summer night (r = −0.327, P < 0.001); there was no relationship for all other seasonal-diel periods (r < −0.076, P > 0.436).
Edible forage biomass during summer was positively associated with the nighttime mountain lion predation risk (r = 0.462, P <0.001), and negatively correlated during winter for all diel periods (r range −0.208 to −0.268, all P <0.031); forage biomass and mountain lion predation risk were not associated during spring. Digestible energy at sampling points was positively correlated with our nighttime mountain lion predation risk index during summer (r = 0.329, P <0.001), but digestible energy was not correlated with diel predation risk indices during spring (all r ≤ 0.172, all P ≥ 0.076). There were moderate negative correlations with diel predation risk during winter (day: r = −0.203, P = 0.035; crepuscular: r = −0.194, P = 0.044; night: r = −0.268, P = 0.005). Digestible protein content of forage was positively correlated with diel mountain lion predation risk during nighttime in spring (r = 0.367, P <0.001) and summer (r = 0.462, P <0.001), but was negatively correlated with diel predation risk indices in winter (day: r = −0.209, P = 0.030; crepuscular: r = −0.223, P = 0.020; night: r = −0.203, P = 0.035).
Discussion
Ungulates must acquire sufficient nutritional resources for survival and reproduction while also mitigating the risk of predation, thus habitat selection is influenced by spatial and temporal variation in predation risk and foraging conditions (Schmidt and Kuijper, 2015; Kohl et al., 2018, 2019). These demands can lead to a trade-off when high quality foraging areas correspond with areas of increased predation risk (Lima and Dill, 1990; Ganz et al., 2022). We determined that this trade-off existed for mule deer in our study. Habitat selection by mule deer was influenced by forage availability and nutritional content at both the landscape and within home range spatial scales. However, the influence of forage conditions on habitat selection was moderated by landscape-scale encounter risk for mountain lions, the primary predator of adult mule deer in our study area. The influence of forage conditions on habitat selection by mule deer also depended on horizontal visibility as it related to stalking cover for mountain lions. Mule deer consistently selected wildfire-burned areas, which contained the highest biomass and forage protein content for browse species that composed the majority of their seasonal diets in our study area, as well as high biomass and protein content of herbaceous forages during spring (Kay, 2018). Wildfire-burned areas also had the lowest horizontal visibility, hence highest stalking cover for mountain lions.
One potential limitation of our study was that we were only able to capture and collar 5 mountain lions. However, given the size of our study area and typical densities of mountain lions in the western US, we believe that we had all resident mountain lions with home ranges overlapping our study area fitted with collars. We maintained camera traps in areas with conditions frequently used as travel corridors by lions and we conducted sign surveys (track and snow) a couple times a week across our study area. All sign that we detected matched up with GPS collar data from our sample of mountain lions and we did not detect any collared deer mortalities attributable to mountain lion predation that were not associated with mountain lions we had fitted with collars.
Changes in forage biomass and nutritional content interact with herbivore dietary demands including previous and current year’s nutritional reserves (or deficiencies therein) to determine the influence of forage conditions on habitat selection. Forage conditions influenced mule deer habitat selection at both spatial scales in our study, but whether forage biomass or protein content were in the most supported RSF models varied seasonally. At the landscape scale, mule deer strongly selected for areas with higher forage protein content in spring and summer, with forage biomass replacing forage protein content in the winter during day and night. At the within home range scale, forage protein content was positively related to mule deer habitat selection in spring. Seasonal climatic patterns drive inter-annual changes in forage availability and nutritional content, with nutritional quality of forages declining during winter. In our study, winter RSF models at the within home range scale suggested that forage biomass, rather than protein content of forage was more important. During winter, forage protein content was substantially lower with little spatial variation, while biomass declines were far less pronounced but with more spatial variation in biomass across the vegetation types in our study area. Consistent with our predictions on the influence of diel period, when we detected diel differences in the magnitude of the parameter estimates for forage metrics, the relative magnitude of those parameter estimates was reduced (i.e., had less influence on habitat selection) during the day when mule deer would typically be resting or ruminating. However, as predicted, our metrics of forage conditions had a larger influence on habitat selection during crepuscular periods when deer typically feed (Beier and McCullough, 1990; Ager et al., 2003; Morano et al., 2019).
Merems et al. (2020) reported that forage conditions had a strong effect on landscape-scale habitat selection by mule deer in Oregon, but forage had a reduced influence at smaller scales because of the availability of high quality forage within the home range resulting from selection at broader spatial scales. However, we observed that the relative magnitude for the forage parameter estimates were similar across spatial scales in spring and summer, indicating that forage conditions were a key driver of habitat selection at both scales. During the growing season, forage protein content would be expected to have a strong effect on habitat selection at the larger spatial scale given the importance of forage quality on enhancing nutritional condition, supporting the energetic demands of milk production, and recovery of nutritional reserves following winter and gestation (Monteith et al., 2013, 2014; Merems et al., 2020). During summer, forage protein content had a strong, positive influence on mule deer habitat selection at the landscape scale; however, at the within home range scale, mule deer avoided areas with the highest protein content, likely because these areas were associated with higher predation risk. Previous research has demonstrated that predation risk for vulnerable neonates may result in maternal habitat selection focused more towards neonate survival rather than enhancing maternal nutritional condition. For example, female bighorn sheep (O. canadensis) select areas with poorer foraging conditions and reduced predation risk compared to areas used by males, thus females enhance fitness via offspring survival (Festa-Bianchet, 1988; Bleich et al., 1997). Similarly, white-tailed deer (O. virginianus) in Florida that selected areas with higher quality forage during fawning were more likely to be killed by Florida panthers (P.c. coryi), whereas those that selected recently burned areas with both lower predation risk and good foraging conditions had higher survival during fawning (Abernathy et al., 2022). Deer in the Gallinas Mountains appeared to employ risk-mitigation strategies at times when the influence of forage protein content on habitat selection was reduced in areas with increased predation risk, particularly during the summer when adult female deer would be expected to be supporting neonates. Although we were unable to capture and collar fawns to determine fawn survival or fawn at heel status for our collared adult deer, pregnancy rates during our study were ≥89% indicating that most of our collared adult female deer likely had fawns each year (Kay, 2018).
Mule deer utilized spatio-temporal strategies and risk-conditional behavior to reduce predation risk, and at times selected suboptimal foraging areas with lower predation risk. Mule deer selection of areas with higher forage protein content was constrained at the landscape scale by encounter risk for mountain lions, such that increasing encounter risk was associated with diminished selection for areas containing high forge protein content. Similar interactions were reported for elk in Montana (Paterson et al., 2022). The moderating effect of mountain lion encounter risk on selection for areas with high forage protein content was particularly pronounced during the spring and summer at the landscape scale when mule deer would be expected to select for forages with high protein content (Anton et al., 2022). We found that influence of the main effect of landscape-scale encounter risk for mountain lions on mule deer habitat selection was inconsistent across seasons. During winter, mule deer avoided areas with high encounter risk for mountain lions at the landscape scale, but selected for areas with higher encounter risk during spring at the within home range scale. However, we also documented an interaction between landscape-scale encounter risk for mountain lions and horizontal visibility in some seasons with mule deer selecting for areas with reduced encounter risk for mountain lions when horizontal visibility was lower. During spring when mule deer selected for areas with higher encounter risk at the within home range scale, they also selected for areas with higher visibility. Many other large herbivores have been observed to detect when predators are in the area and alter their habitat selection in order to reduce predation risk (Creel et al., 2005; Winnie and Creel, 2007; Valeix et al., 2009; Padié et al., 2015). Because they rely on habitat attributes that increase kill success, predation risk from stalking predators may be more predictable than coursing predators (Preisser et al., 2007). Thus, mule deer may be responding to both direct cues related to previous encounters with mountain lions as well as indirect cues related to variability in vegetation cover and other terrain features used by mountain lions as stalking cover. When high quality foraging areas correspond with spatial variation in encounter risk for mountain lions, but also have conditions that provide stalking cover that could increase risk of kill success, prey responses may be more nuanced than simply avoiding areas of increased predator activity or shifting active periods to times when their primary predators are less active.
Mule deer generally selected for areas with reduced horizontal visibility, likely because those areas contained the highest biomass and protein content for woody browse. Mountain lions also selected for areas with reduced visibility. However, the effect of horizontal visibility on mule deer selection depended on terrain ruggedness. At the landscape scale, the highest relative probability of selection for mule deer was for areas of low horizontal visibility when terrain ruggedness was highest. However at the within home range scale, the nature of the visibility−terrain ruggedness interaction on mule deer habitat selection differed during spring when mule deer selection increased with increasing visibility when terrain ruggedness was high. Incidentally, spring was the only season when mule deer strongly selected for areas with increased encounter risk for lions at the within home range scale. Mule deer commonly select steeper and more rugged slopes than other ungulates to enhance predator avoidance or detection (Dellinger et al., 2019; Saudenmaier et al., 2021; Ganz et al., 2022). Our landscape-scale results are consistent with mule deer exploiting terrain features as a predator avoidance mechanism by selecting steeper or more rugged slopes when stalking cover was lower (i.e., visibility was higher). Selection of areas with steeper slopes has been related to increased fawn survival for mule deer (Bonar et al., 2016). However, mule deer use of more rugged areas would be a better predator avoidance strategy for coursing rather than stalking predators (Bonar et al., 2016; Dellinger et al., 2019). Mountain lions commonly select for areas with topographic complexity that also provide stalking cover (Robinson et al., 2015; Blake and Gese, 2016; Peterson et al., 2021), which may enhance kill success (Elbroch et al., 2013).
Our evaluation of lion kill sites and mule deer foraging sites were consistent with our RSF results. Forage biomass proved to be the most important variable for predicting foraging site selection by mule deer, while stalking cover and forage protein content best distinguished mountain lion kill sites. Areas with more forage biomass and higher forage protein content also had the highest stalking cover, increasing the risk of being killed by a mountain lion following an encounter because stalking cover can increase the efficacy of ambush predators (Beier et al., 1995; Blake and Gese, 2016). These results further suggest that deer in our study area are making habitat selection decisions based on forage quality, but habitat selection was constrained by the increased cost of predation risk. This trade-off appears to affect mule deer in the Gallinas in that they may choose to forgo areas with superior foraging conditions thereby compromising nutritional benefit, or increase their risk of being preyed upon. Our kill-site analysis indicated that micro-habitat features are important predictors of predation risk, and that when combined with our RSF results indicates that deer may approach the forage−predation risk trade-off at small as well as large spatial scales. Past research has also shown that mountain lion hunting strategies generally operate on smaller spatial scales than coursing predators and mountain lions often select hunting areas based on the availability of stalking cover and prey (Murphy, 1998; Husseman et al., 2003; Blake and Gese, 2016). Due to limited time and resources, we were only able to visit a small number of kill and foraging sites, yet we were still able to detect biologically and statistically meaningful effects. Increasing the sample size of foraging sites and kill sites as well as increasing the number of paired random points may provide additional insight into drivers of predation risk and foraging behavior by ungulates.
Decades of fire suppression, logging, and overgrazing have altered the conditions of Southwestern forests resulting in increased densities of small diameter trees and fuel loads (Covington and Moore, 1994a). These changes have been associated with limited understory development, reducing forage for ungulates and other wildlife, and more frequent and severe wildfires (Covington and Moore, 1994b). Wildfire-burned areas can benefit ungulate nutritional condition and subsequent productivity (e.g., Proffitt et al., 2016). Changes in herbaceous forage for ungulates often occur immediately after prescribed or low-severity fires, but these changes are often short-lived, lasting only a few years (Hobbs and Spowart, 1984). The effects of high-severity fires can persist for longer periods (Bartos et al., 1994; Bataineh et al., 2006; Wan et al., 2014; Proffitt et al., 2016; Roerick et al., 2019; Fredriksson et al., 2023). High-severity, stand-replacing wildfires can result in dramatic changes in vegetation structure and species composition. Given sufficient post-fire recovery time, stand conversion from high-density conifer patches with little to no understory to open grasslands or shrublands can occur following high severity wildfires (Coop et al., 2020; Falk et al., 2022; Guiterman et al., 2022). This was the case in our study area where browse species that comprise a substantial portion of mule deer diets in the Southwest (including Gambel oak, buckbrush, and mountain mahogany; Boeker et al., 1972; Austin and Urness, 1985; Sandoval et al., 2005), responded favorably following two stand-replacing wildfires after the removal of conifer forest canopy. Deer in our study strongly selected for these burned areas, which contained the highest quantity and quality of forage. However, burned areas also had low visibility and higher stalking cover for lions. Ganz et al. (2022) similarly reported strong selection of moderate and high severity burns by mule deer in wildfire-burned areas in north-central Washington; however, deer in their study avoided areas with high burn severity and the oldest burns as predicted use by mountain lions increased. Thus, predation risk by lions had a moderating effect on mule deer use of wildfire-burned areas (Ganz et al., 2022).
How ungulates exploit a seasonally dynamic nutritional landscape underpins seasonal changes in body condition, ultimately influencing survival and reproduction (Keech et al., 2000; Bishop et al., 2009; Parker et al., 2009; Lamb et al., 2023). Merems et al. (2020) reported that female mule deer that used areas with the highest forage biomass and nutritional content entered winter in better nutritional condition. However, exploitation of the nutritional landscape can interact with varying predator composition and levels of predation risk (Monteith et al., 2014; Duquette et al., 2015). Ungulates incorporate the risks and rewards of the forage–predation risk trade-off into their behavioral decisions, which consequently affects their ability to survive and reproduce. Given the seasonal changes in forage availability and nutritional quality, and subsequent density-dependent effects when forage conditions decline, it is important to assess individual changes in nutritional status in relation to use of risk-prone versus risk-averse behavioral strategies. Deer that are in a poorer nutritional state in general or during periods when the quality of the nutritional landscape declines should be more likely to engage in risk-prone foraging and suffer from this trade-off (McNamara and Houston, 1986; Brown, 1999; Bastille-Rousseau et al., 2015; Rignos, 2015). More informative insights could be gained from future research that incorporates data on individual body condition and reproduction, and forage conditions when examining the indirect effects of predation, thus linking ungulate behavioral decisions to individual health and productivity as well as population vital rates. It is also essential to study resource selection at multiple spatial and temporal scales in order to fully explore variability in potential trade-offs and corresponding behavioral decisions made by ungulates to balance nutritional demands and risk of predation.
Habitat selection by ungulates, including mule deer, is often driven by the abundance and nutritional quality of forage because of the effects on fitness (Kie, 1999; Monteith et al., 2014). However, risk of predation influences not only where ungulates can safely forage, but also foraging efficiency, which is reduced when ungulates increase vigilance to mitigate risk of predation (Lima and Dill, 1990; Brown, 1999; Altendorf et al., 2001; Childress and Lung, 2003; Creel et al., 2014). Prey species sometimes sacrifice forage quality to alleviate predation risk, but may find ways to at least partially mitigate risk by selecting areas that still contain sufficient food or by foraging more efficiently (Lima and Dill, 1990; Hebblewhite and Merrill, 2009). The variable risk-dependent behavior we report show that mule deer behavioral decisions operated at and were influenced by factors at multiple temporal and spatial scales. These behavioral decisions can correspond to dynamic levels of individual body condition, predation risk, the presence of offspring, and climate-related changes in forage conditions (Kie, 1999; Lima and Bednekoff, 1999; Creel and Christianson, 2008; Basille et al., 2015; Rignos, 2015; Dupke et al., 2016). Deer in our study appeared to utilize a mix of strategies to balance nutritional requirements and predation risk at the landscape, within home range, and foraging areas scales.
Data availability statement
The raw data supporting the conclusions of this article will be made available by the authors, without undue reservation.
Ethics statement
The animal study was approved by Animal Care and Use Committee, New Mexico State University. The study was conducted in accordance with the local legislation and institutional requirements.
Author contributions
JC, JK, and SL contributed to conception and design of the study. JK and JC collected the data. JK and JC performed the statistical analysis. JK and JC wrote the initial draft of the manuscript. All authors contributed to manuscript revision, read, and approved the submitted version.
Funding
The author(s) declare financial support was received for the research, authorship, and/or publication of this article. Funding was provided by the New Mexico Department of Game and Fish (NMDGF), New Mexico Agricultural Experiment Station, and the Mule Deer Foundation.
Acknowledgments
We thank NMDGF assistance capturing and monitoring mule deer and mountain lions. D. Wilckens assisted with mountain lion captures. N. Moore, J. Buskirk and T. Turnbull assisted with fieldwork. We thank the Sanchez Family, Huey Family, D. Frost, D. McCall, and J. Davidson for access to their lands and W. Garfield for always keeping the roads plowed. We thank R. Dunlap, S. Cox and the NMSU Corona Range and Livestock Center for logistical support. C. R. Anderson, Jr. and 4 reviewers provided helpful comments on an earlier draft of this manuscript. Any use of trade, firm or product names is for descriptive purposes only and does not imply endorsement by the US Government.
Conflict of interest
The authors declare that the research was conducted in the absence of any commercial or financial relationships that could be construed as a potential conflict of interest.
Publisher’s note
All claims expressed in this article are solely those of the authors and do not necessarily represent those of their affiliated organizations, or those of the publisher, the editors and the reviewers. Any product that may be evaluated in this article, or claim that may be made by its manufacturer, is not guaranteed or endorsed by the publisher.
Supplementary material
The Supplementary Material for this article can be found online at: https://www.frontiersin.org/articles/10.3389/fevo.2024.1121439/full#supplementary-material
References
Abernathy H. N., Chandler R. B., Crawford D. A., Garrison E. P., Conner L. M., Miller K. V., et al. (2022). Behavioral responses to ecological disturbances influence predation risk for a capital breeder. Landsc. Ecol. 37, 233–248. doi: 10.1007/s10980-021-01345-0
Ager A. A., Johnson B. K., Kern J. W., Kie J. G. (2003). Daily and seasonal movements and habitat use by female Rocky Mountain elk and mule deer. J. Mammal. 84, 1076–1088. doi: 10.1644/BBa-020
Altendorf K. B., Laundré J. W., Gonzalez C. A. L., Brown J. S. (2001). Assessing effects of predation risk on foraging behavior of mule deer. J. Mammal. 82, 430–439. doi: 10.1644/1545-1542(2001)082.0430:AEOPRO.2.0.CO;2
Anderson C.R., Lindzey F. G. (2003). Estimating cougar predation rates from GPS location clusters. J. Wildl. Manage. 67, 307–316. doi: 10.2307/3802772
Anton C. B., DeCesare N. J., Peterson C., Hayes T. A., Bishop C. J. (2022). Climate, habitat interactions and mule deer resource selection on winter landscapes. J. Wildl Manage. 86, e22299. doi: 10.1002/jwmg.22299
Arnold T. W. (2010). Uninformative parameters and model selection using Akaike’s Information Criterion. J. Wildl. Manage. 74, 1175–1178. doi: 10.1111/j.1937-2817.2010.tb01236.x
Atwood T. C., Gese E. M., Kunkel K. E. (2009). Spatial partitioning of predation risk in a multiple predator-multiple prey system. J. Wildl. Manage 73, 876–884. doi: 10.2193/2008-325
Austin D. D., Urness P. J. (1985). Values of four communities for mule deer on ranges with limited summer habitat. J. Range Manage. 38, 167–171. doi: 10.2307/3899263
Barten N. L., Bowyer R. T., Jenkins K. J. (2001). Habitat use by female caribou: tradeoffs associated with parturition. J. Wildl. Manage 65, 77–92. doi: 10.2307/3803279
Bartos D. L., Brown J. K., Booth G. D. (1994). Twelve years biomass response in aspen communities following fire. Range. Ecol. Manage. 47, 79–83. doi: 10.2307/4002846
Basille M., Fortin D., Dussault C., Bastille-Rousseau G., Ouellet J., Courtois R. (2015). Plastic response of fearful prey to the spatiotemporal dynamics of predator distribution. Ecology 96, 2622–2631. doi: 10.1890/14-1706.1
Bastille-Rousseau G., Potts J. R., Schaefer J. A., Lewis M. A., Ellington E. H., Rayl N. D., et al. (2015). Unveiling trade-offs in resource selection of migratory caribou using a mechanistic movement model of availability. Ecography 38, 1049–1059. doi: 10.1111/ecog.01305
Bataineh A. L., Oswald B. P., Bataineh M. M., Williams H. M., Coble D. W. (2006). Changes in understory vegetation of a ponderosa pine forest in northern Arizona 30 years after a wildfire. For. Ecol. Manage. 235, 283–294. doi: 10.1016/j.foreco.2006.09.003
Bates D., Mächler M., Bolker B., Walker S. (2015). Fitting linear mixed-effects models using lme4. J. Stat. Softw. 67, 1–48. doi: 10.18637/jss.v067.i01
Beier P., Choate D., Barrett R. H. (1995). Movement patterns of mountain lions during different behaviors. J. Mammal. 76, 1056–1070. doi: 10.2307/1382599
Beier P., McCullough D. R. (1990). Factors influencing white-tailed deer activity patterns and habitat use. Wildl. Monogr. 109, 3–51.
Bishop C. J., White G. C., Freddy D. J., Watkins B. E., Stephenson T. R. (2009). Effect of enhanced nutrition on mule deer population rate of change. Wildl. Monogr. 172, 1–28. doi: 10.2193/2008-107
Blake L. W., Gese E. M. (2016). Resource selection by cougars: Influence of behavioral state and season. J. Wildl. Manage. 80, 1205–1217. doi: 10.1002/jwmg.21123
Bleich V. C., Bowyer R. T., Wehausen J. D. (1997). Sexual segregation in mountain sheep: Resources or predation? Wild. Mongr. 134, 1–50.
Boeker E. L., Scott V. E., Reynolds H. G., Donaldson B. A. (1972). Seasonal food habits of mule deer in southwestern New Mexico. J. Wildl. Manage. 36, 56–63. doi: 10.2307/3799188
Bonar M., Manseau M., Geisheimer J., Bannatyne T., Lingle S. (2016). The effect of terrain and female density on survival of neonatal white-tailed deer and mule deer fawns. Ecol. Evol. 6, 4387–4402. doi: 10.1002/ece3.2178
Boyce M. S., Vernier P. R., Nielsen S. E., Schmiegelow F. K. A. (2002). Evaluating resource selection functions. Ecol. Modell. 157, 281–300. doi: 10.1016/S0304-3800(02)00200-4
Breslow N. E., Clayton D. G. (1993). Approximate inference in generalized linear mixed models. J. Am. Stat. Assoc. 88, 9–21. doi: 10.1080/01621459.1993.10594284
Bristow K. D., Harding L. E., Lucas R. W., McCall T. C. (2020). Influence of fire severity and vegetation treatments on mule deer (Odocoileus hemionus) winter habitat use on the Kaibab Plateau, Arizona. Anim. Prod. Sci. 60, 1292–1302. doi: 10.1071/AN19373
Brown J. S. (1999). Vigilance, patch use, and habitat selection: foraging under predation risk. Evo. Eco. Res. 1, 49–71.
Brown A. L., Litvaitis J. A. (1995). Habitat features associated with predation of New England cottontails: what scale is appropriate? Can. J. Zool. 73, 1005–1011. doi: 10.1139/z95-120
Burnham K. P., Anderson D. R. (2002). Model selection and multimodal inference: a practice information-theoretic approach. 2nd edition (New York: Springer).
Calenge C. (2006). The package adehabitat for the R software: tool for the analysis of space and habitat use by animals. Ecol. Model. 197, 1035. doi: 10.1016/j.ecolmodel.2006.03.017
Childress M. J., Lung M. A. (2003). Predation risk, gender and the group size effect: Does elk vigilance depend on the behaviour of conspecifics? Anim. Behav. 66, 389–398. doi: 10.1006/anbe.2003.2217
Chitwood M. C., Baruzzi C., Lashley M. A. (2022). Ecology of fear” in ungulates: Opportunities for improving conservation. Ecol. Evol. 12, e8657. doi: 10.1002/ece3.8657
Christianson D., Creel S. (2010). A nutritionally mediated risk effect of wolves on elk. Ecology 91, 1184–1191. doi: 10.1890/09-0221.1
Coon C. A. C., Mahoney P. J., Edelblutte E., McDonald Z., Stoner D. C. (2020). Predictors of puma occupancy indicate prey vulnerability is more important than prey availability in a highly fragmented landscape. Wildlife Biol. 2020, wlb.00540. doi: 10.2981/wlb.00540
Coop J. D., Parks S. A., Stevens-Rumann C. S., Crausbay S. D., Higuera P. E., Hurteau M. D., et al. (2020). Wildfire-driven forest conversion in western north american landscapes. Bioscience 70, 659–673. doi: 10.1093/biosci/biaa061
Covington W. W., Moore M. M. (1994a). Southwestern ponderosa forest structure: changes since Euro-American settlement. J. For. 92, 39–47. doi: 10.1093/jof/92.1.39
Covington W. W., Moore M. M. (1994b). Post-settlement changes in natural fire regimes and forest structure: ecological restoration of old growth ponderosa pine forests. J. Sustain. For. 2, 153–181. doi: 10.1300/J091v02n01_07
Creel S. (2018). The control of risk hypothesis: Reactive vs. proactive antipredator responses and stress-mediated vs. food-mediated costs of response. Ecol. Lett. 21, 947–956. doi: 10.1111/ele.12975
Creel S., Christianson D. (2008). Relationships between direct predation and risk effects. TREE 23, 194–201. doi: 10.1016/j.tree.2007.12.004
Creel S., Schuette P., Christianson D. (2014). Effects of predation risk on group size, vigilance, and foraging behavior in an African ungulate community. Behav. Ecol. 25, 773–784. doi: 10.1093/beheco/aru050
Creel S., Winnie J. Jr., Maxwell B., Hamlin K., Creel M. (2005). Elk alter habitat selection as an antipredator response to wolves. Ecology 86, 3387–3397. doi: 10.1890/05-0032
Davidson Z., Valeix M., Loveridge A., Hunt J., Johnson P., Madzikanda H., et al. (2012). Environmental determinants of habitat and kill site selection in a large carnivore: scale matters. J. Mammal. 95, 677–685. doi: 10.1644/10-MAMM-A-424.1
DeCesare N. J. (2012). Separating spatial search and efficiency rates as components of predation risk. Proc. R. Soc B 279, 4246–4633. doi: 10.1098/rspb.2012.1698
Dellinger J. A., Cristescu B., Ewanyk J., Gammons D. J., Garcelon D., Johnston P. (2020). Using mountain lion habitat selection in management. J. Wildl Manage. 84, 359–371. doi: 10.1002/jwmg.21798
Dellinger J. A., Shores C. R., Craig A., Heithaus M. R., Ripple W. J., Wirsing A. J. (2019). Habitat use of sympatric prey suggests divergent anti-predator responses to recolonizing gray wolves. Oecologia 189, 487–500. doi: 10.1007/s00442-018-4323-z
Doherty T. S., Geary W. L., Jolly C. J., Macdonald K. J., Miritis V., Watchorn D. J., et al. (2022). Fire as a driver and mediator of predator-prey interactions. Biol. Rev. 94, 1539–1558. doi: 10.1111/brv.12853
Dupke C., Bonenfant C., Reineking B., Hable R., Zeppenfeld T., Ewald M., et al. (2016). Habitat selection by a large herbivore at multiple spatial and temporal scales is primarily governed by food resources. Ecography 39, 1–14. doi: 10.1111/ecog.02152
Duquette J., Belant J., Svoboda N. J., Beyer D. E. Jr., Lederle P. E. (2015). Scale dependence of female ungulate reproductive success in relation to nutritional condition, resource selection and multi-predator avoidance. PloS One 10, e0140433. doi: 10.1371/journal.pone.0140433
Elbroch L. M., Lendrum P. E., Newby J., Quigley H., Craighead D. (2013). Seasonal foraging ecology of non-migratory cougars in a system with migrating prey. PlosOne 8, e83375. doi: 10.1371/journal.pone.0083375
Falk D. A., van Mantgem P. J., Keeley J. E., Gregg R. M., Guiterman C. H., Tepley A. J., et al. (2022). Mechanisms of forest resilience. For. Ecol. Manag 512, 120129. doi: 10.1016/j.foreco.2022.120129
Festa-Bianchet M. (1988). Seasonal range selection in bighorn sheep: conflicts between forage quality, forage quantity, and predator avoidance. Oecologia 75, 580–586. doi: 10.1007/BF00776423
Fortin D., Fortin M. E. (2009). Group-size-dependent association between food profitability, predation risk and distribution of free-ranging bison. Anim. Behav. 78, 887–892. doi: 10.1016/j.anbehav.2009.06.026
Fredriksson E., Wallgren M., Löfroth T. (2023). Wildfire and prescribed burning impact moose forage availability and browsing levels in the northern boreal forest. Scand. J. For. Res. 38, 58–69. doi: 10.1080/02827581.2023.2184489
Ganz T. R., DeVivo M. T., Kertson B. N., Roussin T., Satterfield L., Wirsing A. J., et al. (2022). Interactive effects of wildfires, season and predator activity shape mule deer movements. J. Anim. Ecol. 91, 2273–2288. doi: 10.1111/1365-2656.13810
Gedir J. V., Cain J. W. III, Swetnam T. L., Krausman P. R., Morgart J. R. (2020). Extreme drought and adaptive resource selection by a desert ungulate. Ecosphere 11, e03175. doi: 10.1002/ecs2.3175
Gehr B., Hofer E. J., Pewsner M., Ryser A., Vimercati E., Vogt K., et al. (2018). Hunting-mediated predator facilitation and superadditive mortality in a European ungulate. Ecol. Evol. 8, 109–119. doi: 10.1002/ece3.3642
Gelman A. (2008). Scaling regression inputs by dividing by two standard deviations. Stat. Med. 27, 2865–2873. doi: 10.1002/sim.3107
Gigliotti L. C., Curveira-Santos C., Slotow R., Sholto-Douglas C., Swanepoel L. H., Jachowski D. S. (2022). Community-level responses of African carnivores to prescribed burning. J. Appl. Ecol. 59, 251–262. doi: 10.1111/1365-2664.14050
Gillies C. S., Hebblewhite M., Nielsen S. E., Krawchuk M. A., Aldridge C. L., Frair J. L., et al. (2006). Application of random effects to the study of resource selection by animals. J. Anim. Ecol. 75, 887–898. doi: 10.1111/j.1365-2656.2006.01106.x
Guiterman C. H., Gregg R. M., Marshall L. A., Beckmann J. J., van Mantgem P. J., Falk D. A., et al. (2022). Vegetation type conversion in the US Southwest: frontline observations and management responses. Fire Ecol. 18, 1–16. doi: 10.1186/s42408-022-00131-w
Gulsby W. D., Kilgo J. C., Vukovich M., Martin J. A. (2017). Landscape heterogeneity reduces coyote predation on white-tailed deer fawns. J. Wild. Manage. 81, 601–609. doi: 10.1002/jwmg.21240
Hamel S., Côté S. D. (2007). Habitat use patterns in relation to escape terrain: are alpine ungulate females trading off better foraging sites for safety? Can. J. Zool. 85, 933–943. doi: 10.1139/Z07-080
Hay C., Cross P., Funston P. (2008). Trade-offs of predation and foraging explain sexual segregation in African buffalo. J. Anim. Ecol. 77, 850–858. doi: 10.1111/j.1365-2656.2008.01409.x
Haydock K., Shaw N. (1975). The comparative yield method for estimating dry matter yield of pasture. Aust. J. Exp. Agric. 15, 663–670. doi: 10.1071/EA9750663
Hayes T. A., DeCesare N. J., Peterson C. J., Bishop C. J., Mitchell M. S. (2022). Trade-offs in forest disturbance management for plant communities and ungulates. For. Ecol. Manage. 506, 119972. doi: 10.1016/j.foreco.2021.119972
Hebblewhite M., Merrill E. H. (2007). Multiscale wolf predation risk for elk: does migration reduce risk? Oecologia 152, 377–387. doi: 10.1007/s00442-007-0661-y
Hebblewhite M., Merrill E. H. (2009). Trade-offs between predation risk and forage differ between migrant strategies in a migratory ungulate. Ecology 90, 3445–3454. doi: 10.1890/08-2090.1
Hernández L., Laundré J. W. (2005). Foraging in the "landscape of fear" and its implications for habitat use and diet quality of elk cervus elaphus and bison bison bison. Wildl. Biol. 11, 215–220. doi: 10.2981/0909-6396(2005)11[215:FITLOF]2.0.CO;2
Hobbs N. T., Spowart R. A. (1984). Effects of prescribed fire on nutrition of mountain sheep and mule deer during winter and spring. J. Wildl. Manage 48, 551–560. doi: 10.2307/3801188
Homer C., Huang C., Yang L., Wylie B., Coan M. (2004). Development of a 2001 national landcover database for the United States. Photogramm. Eng. Remote Sens. 70, 829–840. doi: 10.14358/PERS.70.7.829
Husseman J. S., Murray D. L., Power G., Mack C., Wenger C. R., Quigley H. (2003). Assessing differential prey selection patterns between two sympatric large carnivores. Oikos 101, 591–601. doi: 10.1034/j.1600-0706.2003.12230.x
Jenness J. (2006)Topographic Position Index (tpi_jen.avx) extension for ArcView 3.x, v. 1.3a. Available online at: http://www.jennessent.com/arcview/tpi.Htm.
Johnson D. H. (1980). The comparison of usage and availability measurements for evaluating resource preference. Ecology 61, 65–71. doi: 10.2307/1937156
Johnson B. K., Kern J. W., Wisdom M. J., Findholt S. L., Kie J. G. (2000). Resource selection and spatial separation of mule deer and elk during spring. J. Wildl. Manage. 64, 685–697. doi: 10.2307/3802738
Johnston D. B., Anderson C. R. Jr (2023). Plant and mule deer response to pinyon-juniper removal by three mechanical methods. Wildl. Soc Bull. 47, e1421. doi: 10.1002/wsb.1421
Kay J. H. (2018). Top-down and bottom up influences on mule deer in central New Mexico (Las Cruces (NM: New Mexico State University).
Keech M. A., Bowyer R. T., Ver Hoef J. M., Boertje R. D., Dale B. W., Stephenson T. R. (2000). Life-history consequences of maternal condition in Alaskan moose. J. Wildl. Manage. 64, 450–462. doi: 10.2307/3803243
Khan P., Eliuk L., Frey S., Bone C., Fisher J. T. (2023). Shifts in diel activity of Rocky Mountain mammal communities in response to anthropogenic disturbance and sympatric invasive white-tailed deer. Glob. Ecol. Conserv. 41, e02355. doi: 10.1016/j.gecco.2022.e02355
Kie J. G. (1999). Optimal foraging and risk of predation: Effects on behavior and social structure in ungulates. J. Mammal. 80, 1114–1129. doi: 10.2307/1383163
Kindschuh S. R., Cain J. W. III, Daniel D., Peyton M. A. (2016). Efficacy of GPS cluster analysis for predicting carnivory sites of a wide-ranging omnivore: the American black bear. Ecosphere 7, e01513. doi: 10.1002/ecs2.1513
Knopff A. A., Knopff K. H., Boyce M. S., St. Clair C. C. (2014). Flexible habitat selection by cougars in response to anthropogenic development. Biol. Conserv. 178 136–145. doi: 10.1016/j.biocon.2014.07.017
Knopff K. H., Knopff A. A., Warren M. B., Boyce M. S. (2009). Evaluating global positioning system telemetry techniques for estimating cougar predation parameters. J. Wildl. Manage. 73, 586–597. doi: 10.2193/2008-294
Kohl M. T., Ruth T. K., Metz M. C., Stahler D. R., Smith D. W., White P. J., et al. (2019). Do prey select for vacant hunting domains to minimize a multi-predator threat? Ecol. Lett. 22, 1724–1733. doi: 10.1111/ele.13319
Kohl M. T., Stahler D. R., Metz M. C., Forester J. D., Kauffman M. J., Varley N., et al. (2018). Diel predator activity drives a dynamic landscape of fear. Ecol. Monogr. 88, 638–652. doi: 10.1002/ecm.1313
Lamb S., McMillan B. R., van de Kerk M., Frandsen P. B., Hersey K. R., Larsen R. T. (2023). From conception to recruitment: nutritional condition of the dam dictates the likelihood of success in a temperate ungulate. Front. Ecol. Evol. 11, 1090116. doi: 10.3389/fevo.2023.1090116
Laundré J. W., Hernández L. (2003). Winter hunting habitat of pumas Puma concolor in northwestern Utah and southern Idaho, USA. Wildl. Biol. 9, 123–129. doi: 10.2981/wlb.2003.034
Laundré J. W., Hernández L., Altendorf K. B. (2001). Wolves, elk, and bison: reestablishing the “landscape of fear” in Yellowstone National Park, U.S.A. Can. J. Zool. 79, 1401–1409. doi: 10.1139/cjz-79-8-1401
Lewis J. S., LeSueur L., Oakleaf J., Rubin E. S. (2022). Mixed severity wildfire shapes habitat use of large herbivores and carnivores. For. Ecol. Manage. 506, 119933. doi: 10.1016/j.foreco.2021.119933
Lillesand T. M., Kiefer R. W. (1994). Remote sensing and interpretation (New York, NY: John Wiley & Sons).
Lima S. L., Bednekoff P. A. (1999). Temporal variation in danger drives antipredator behavior: the predation risk allocation hypothesis. Biol. Conserv. 153, 649–659. doi: 10.1086/303202
Lima S. L., Dill L. M. (1990). Behavioral decisions made under the risk of predation: a review and prospectus. Can. J. Zool. 68, 619–640. doi: 10.1139/z90-092
Lowry J. H. Jr., Ramsey R. D., Boykin K., Bradford D., Comer P., Falzarano S., et al. (2005). Southwest regional gap analysis project: final report on land cover mapping methods, RS/GIS laboratory (Utah: Utah State University).
Lowrey C., Longshore K. M., Choate D. M., Nagol J. R., Sexton J., Thompson D. (2019). Ecological effects of fear: how spatiotemporal heterogeneity in predation risk influences mule deer access to forage in a sky-island system. Ecol. Evol. 9, 7213–7226. doi: 10.1002/ece3.5291
Manly B. F. J., McDonald L. L., Thomas D. L., McDonald T. L., Erickson W. P. (2002). Resource Selection by Animals: Statistical design and analysis for field studies (Dordrecht: Kluwer Academic Publishers).
Marshal J. P., Krausman P. R., Bleich V. C. (2005). Dynamics of mule deer forage in the Sonoran Desert. J. Arid Environ. 60, 593–609. doi: 10.1016/j.jaridenv.2004.07.002
Mazaika R., Krausman P. R. (1991). Use of dry-weight rank multipliers for desert vegetation. J. Range Manage. 44, 409–411. doi: 10.2307/4002411
McNamara J. M., Houston A. I. (1986). The common currency for behavioral decisions. Am. Nat. 127, 358–378. doi: 10.1086/284489
Merems J. L., Shipley L. A., Levi T., Ruprecht J., Clark D. A., Wisdom M. J., et al. (2020). Nutritional-landscape models link habitat use to condition of mule deer (Odocoileus hemionus). Front. Ecol. Evol. 8, 98. doi: 10.3389/fevo.2020.00098
Monteith K. L., Bleich V. C., Stephenson T. R., Pierce B. M., Conner M. M., Kie J. G., et al. (2014). Life-history characteristics of mule deer: Effects of nutrition in a variable environment. Wildl. Monogr. 186, 1–62. doi: 10.1002/wmon.1011
Monteith K. L., Stephenson T. R., Bleich V. C., Conner M. M., Pierce B. M., Bowyer R. T. (2013). Risk-sensitive allocation in seasonal dynamics of fat and protein reserves in a long-lived mammal. J. Anim. Ecol. 82, 377–388. doi: 10.1111/1365-2656.12016
Morano S., Stewart K. M., Dilts T., Ellsworth A., Bleich V. C. (2019). Resource selection of mule deer in a shrub-steppe ecosystem: influence of woodland distribution and animal behavior. Ecosphere 10, e02811. doi: 10.1002/ecs2.2811
MTBS Data Access: Fire Level Geospatial Data. (2017). MTBS project (USDA forest service/US Geological survey). Available online at: http://mtbs.gov/direct-download.
Murphy K. M. (1998). The ecology of the cougar (Puma concolor) in the Northern Yellowstone ecosystem: interactions with prey, bears, and humans (Dissertation. Moscow (ID: University of Idaho).
NASA LP DAAC (2015) MOD13Q1: MODIS/Terra Vegetation Indices 16-Day L3 Global 250 m SIN Grid V006 (South Dakota: NASA EOSDIS Land Processes DAAC, USGS Earth Resources Observation and Science (EROS) Center, Sioux Falls). Available online at: https://lpdaac.usgs.gov (Accessed March, 2017).
Padié S., Morellet N., Hewison A. J., Martin J. L., Bonnot N., Cargnelutti B., et al. (2015). Roe deer at risk: teasing apart habitat selection and landscape constraints in risk exposure at multiple scales. Oikos 124, 1536–1546. doi: 10.1111/oik.02115
Panzacchi M., Herfindal I., Linnell J. D. C., Odden M., Odden J., Andersen R. (2010). Trade-offs between maternal foraging and fawn predation risk in an income breeder. Behav. Ecol. Sociobio. 64, 1267–1278. doi: 10.1007/s00265-010-0941-0
Parker K. L., Barboza P. S., Gillingham M. P. (2009). Nutrition integrates environmental responses of ungulates. Funct. Ecol. 23, 57–69. doi: 10.1111/j.1365-2435.2009.01528.x
Paterson J. T., Proffitt K. M., DeCesare N. J., Gude J. A., Hebblewhite M. (2022). Evaluating the summer landscapes of predation risk and forage quality for elk (Cervus canadensis). Ecol. Evo. 12, e9201. doi: 10.1002/ece3.9201
Patten M. A., Burger J. C., Mitrovich M. (2019). The intersection of human disturbance and diel activity, with potential consequences on trophic interactions. PloS One 14, e0226418. doi: 10.1371/journal.pone.0226418
Peterson C. J., Mitchell M. S., DeCesare N. J., Bishop C. J., Sells S. S. (2021). Habitat selection by wolves and mountain lions during summer in western Montana. PloS One 16, e0254827. doi: 10.1371/journal.pone.0254827
Pettorelli N., Ryan S., Mueller T., Bunnefeld N., Jedrzejewska B., Lima M., et al. (2011). The Normalized Difference Vegetation Index (NDVI): unforeseen successes in animal ecology. Clim. Res. 46, 15–27. doi: 10.3354/cr00936
Pierce B. M., Bowyer R. T., Bleich V. C. (2004). Habitat selection by mule deer: Forage benefits or risk of predation? J. Wildl. Manage. 68, 533–541. doi: 10.2193/0022-541X(2004)068[0533:HSBMDF]2.0.CO;2
Preisser E. L., Orrock J. L., Schmitz O. J. (2007). Predator hunting mode and habitat domain alter nonconsumptive effects in predator-prey interactions. Ecology 88, 2744–2751. doi: 10.1890/07-0260.1
Proffitt K. M., DeVoe J., Barker K., Durham R., Hayes T., Hebblewhite M., et al. (2019). A century of changing fire management alters ungulate forage in a wildfire-dominated landscape. Forestry 92, 523–537. doi: 10.1093/forestry/cpz017
Proffitt K. M., Hebblewhite M., Peters W., Hupp N., Shamhart J. (2016). Linking landscape-scale differences in forage to ungulate nutritional ecology. Ecol. Appl. 26, 2156–2174. doi: 10.1002/eap.1370
R Core Team (2023). R: a language and environment for statistical computing (Vienna, Austria: R Foundation for Statistical Computing).
Rignos C. (2015). Climate and the landscape of fear in an African savanna. J. Anim. Ecol. 84, 124–133. doi: 10.1111/1365-2656.12262
Roberts C. P., Cain J. W. III, Cox R. D. (2016). Application of activity sensors for estimating behavioral patterns. Wildl. Soc Bull. 40, 764–771. doi: 10.1002/wsb.717
Robinson H. S., Ruth T., Gude J. A., Choate D., DeSimone R., Hebblewhite M., et al. (2015). Linking resource selection and mortality modeling for population estimation of mountain lions in Montana. Ecol. Modell 312, 11–25. doi: 10.1016/j.ecolmodel.2015.05.013
Rodgers A. R., Carr A. P., Beyer H. L., Smith L., Kie J. G. (2007). HRT: home range tools for arcGIS. Version 1.1 (Thunder Bay, Ontario, Canada: Ontario Ministry of Natural Resources, Centre for Northern Forest Ecosystem Research).
Roerick T. M., Cain J. W. III, Gedir J. V. (2019). Forest restoration, wildfire and habitat selection by female mule deer. For. Ecol. Manage. 447, 169–179. doi: 10.1016/j.foreco.2019.05.067
Ruth T., Murphy K. (2010). “Competition with other carnivores for prey,” in Cougar: ecology and conservation. Eds. Hornocker M., Negri S. (University of Chicago Press, Chicago), 163–174.
Sandoval L., Holechek J., Biggs J., Valdez R., VanLeeuwen D. (2005). Elk and mule deer diets in north-central New Mexico. Range. Ecol. Manage. 58, 366–372. doi: 10.2111/1551-5028(2005)058[0366:EAMDDI]2.0.CO;2
Sappington J. M., Longshore K. M., Thomson B. D. (2007). Quantifying landscape ruggedness for animal habitat analysis: A case study using bighorn sheep in the Mojave Desert. J. Wildl. Manage. 711419–1426. doi: 10.2193/2005-723
Sargeant G. A., Eberhardt L. E., Peek J. M. (1994). Thermoregulation by mule deer (Odocoileus hemionus) in arid rangelands of southcentral Washington. J. Mammal. 75, 536–544. doi: 10.2307/1382578
Saudenmaier A. R., Shipley L. A., Bibelnieks A. J., Camp M. J., Thomposon D. H. (2021). Habitat use and spatio-temporal interactions of mule deer and white-tailed deer in an area of sympatry in NE Washington. Ecosphere 12, e03813. doi: 10.1002/ecs2.3813
Schmidt K., Kuijper D. P. J. (2015). A “death trap” in the landscape of fear. Mammal Res. 60, 275–284. doi: 10.1007/s13364-015-0229-x
Sinclair A. R. E., Arcese P. (1995). Population consequences of predation-sensitive foraging: the Serengeti wildebeest. Ecology 76, 882–891. doi: 10.2307/1939353
Smith J. A., Donadio E., Pauli J. N., Sheriff M. J., Bidder O. R., Middleton A. D. (2019b). Habitat complexity mediates the predator–prey space race. Ecology 100, e02724. doi: 10.1002/ecy.2724
Smith J. A., Donadio E., Pauli J. N., Sheriff M. J., Middleton A. D. (2019a). Integrating temporal refugia into landscapes of fear: prey exploit predator downtimes to forage in risky places. Oecologia 189, 883–890. doi: 10.1007/s00442-019-04381-5
Suraci J. P., Smith J. A., Chamaillé-Jammes S., Gaynor K. M., Jones M., Luttbeg B., et al. (2022). Beyond spatial overlap: harnessing new technologies to resolve the complexities of predator–prey interactions. Oikos 2022, e09004. doi: 10.1111/oik.09004
Therneau T. M., Lumley T. (2015). Package ‘survival’. R Top Doc, Vol. 128. 28–33. Available at: https://vps.fmvz.usp.br/CRAN/web/packages/survival/survival.pdf.
t’Mannetje L., Haydock K. P. (1963). The Dry-Weight-Rank method for the botanical analysis of pasture. Grass Forage Sci. 18, 268–275. doi: 10.1111/j.1365-2494.1963.tb00362.x
US Naval Observatory (2014) Sun or moon rise/set table for one year. Available online at: http://aa.usno.navy.mil (Accessed May 2015).
Valeix M., Loveridge A. J., Chamaille-Jammes S., Davidson Z., Murindagomo F., Fritz H., et al. (2009). Behavioral adjustments of African herbivores to predation risk by lions: spatiotemporal variations influence habitat use. Ecology 90, 23–30. doi: 10.1890/08-0606.1
Wan H. Y., Olson A. C., Muncey K. D., St. Clair S. B. (2014). Legacy effects of fire size and severity on forest regeneration, recruitment and wildlife activity in aspen forests. For. Ecol. Manage. 329, 59–68. doi: 10.1016/j.foreco.2014.06.006
Watkins B. E., Bishop C. J., Bergman E. J., Bronson A., Hale B., Wakeling B. F., et al. (2007). Habitat guidelines for mule deer: Colorado plateau shrubland and forest ecoregion (Boise, ID: Mule Deer Working Group, Western Association of Fish and Wildlife Agencies).
Keywords: forage, mountain lion predation, mule deer, Odocoileus hemionus, Puma concolor, predation, resource selection, trade-off
Citation: Cain JW III, Kay JH, Liley SG and Gedir JV (2024) Mule deer (Odocoileus hemionus) resource selection: trade-offs between forage and predation risk. Front. Ecol. Evol. 12:1121439. doi: 10.3389/fevo.2024.1121439
Received: 11 December 2022; Accepted: 21 February 2024;
Published: 04 April 2024.
Edited by:
Mark C. Drever, Environment and Climate Change Canada (ECCC), CanadaReviewed by:
Daniel Fortin, Laval University, CanadaShawn Crimmins, University of Alaska Fairbanks, United States
Copyright At least a portion of this work is authored by James W. Cain III as part of his official duties on behalf of the U.S. Government and as regards Dr. Cain and the U.S. Government, that portion of the work constitutes a "work of the U.S. government" and is not subject to copyright protection in the United States. Foreign jurisdiction copyright laws may apply. This is an open-access article distributed under the terms of the Creative Commons Attribution License (CC BY). The use, distribution or reproduction in other forums is permitted, provided the original author(s) and the copyright owner(s) are credited and that the original publication in this journal is cited, in accordance with accepted academic practice. No use, distribution or reproduction is permitted which does not comply with these terms.
*Correspondence: James W. Cain III, andjYWluQG5tc3UuZWR1
†Present address: Jacob H. Kay, Kasilof, AK, United States