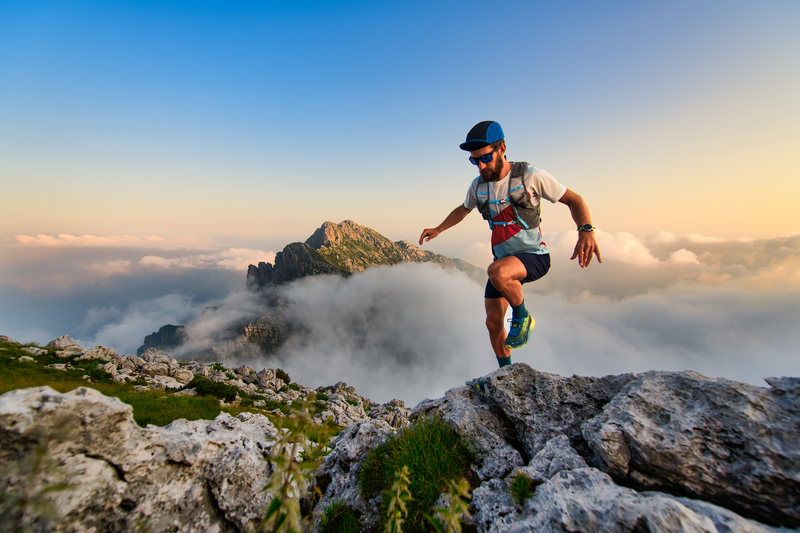
95% of researchers rate our articles as excellent or good
Learn more about the work of our research integrity team to safeguard the quality of each article we publish.
Find out more
ORIGINAL RESEARCH article
Front. Ecol. Evol. , 06 April 2023
Sec. Urban Ecology
Volume 11 - 2023 | https://doi.org/10.3389/fevo.2023.983567
Exploring how water bodies work on the thermal environment in a real and complex urban context is of great significance to develop urban blue infrastructure (BI) system for climate adaption. Previous studies mainly focused on the water cool island (WCI) effect in the microenvironment, which cannot provide enough evidence for systematic application. This study explored the cooling effect of water bodies on the urban environment in Wuhan, China based on three scenarios in which water bodies was regarded as individual samples, water network system and one landscape category of urban ecosystem, respectively. Results indicate that all detected water bodies expressed WCI effect with the mean WCI intensity of 5.5°C and the range of 1.1°C to 13.3°C. The main factors influencing the cooling effect of urban water body system were Percent of landscape (PLAND_W), Landscape shape index (LSI_W) and Contrast-weighted edge density (CWED_W) of water bodies, which could explain 69.0% of urban LST variation. An urban water body system with relatively larger water area, more regular boundary and simpler surroundings might be more efficient in cooling the urban environment. Nevertheless, when considering the interaction of water bodies with other land covers on thermal environment, the contribution of water bodies to the cooling benefit was depressed. The main factors were Similarity index_mean of Construction land (SIMI_MN_C), Class area of Construction land (CA_C), Total edge contrast index of Water body (TECI_W), Landscape shape index of Water body (LSI_W) and Percent of landscape of Vegetated area (PLAND_V). They combined to explain 71.9% of LST variation in urban areas. Developing an urban BI system by connecting the dispersed water bodies and fragmenting the contiguous construction land in rapidly urbanized areas can be more realistic for mitigating UHI effect and adapting to climate change.
With the rapid urbanization, a lot of natural resources have been replaced by artificial surface areas (Pickett et al., 2011; Eckart et al., 2017). The increase of impervious surfaces and decrease of blue-green spaces drastically alter the landscape structure and pattern in urban areas (Estoque et al., 2017; Yu et al., 2019), which leads to the significant modification in physical characteristics of urban surfaces such as thermal capacity and heat conductivity (Mohan and Kandya, 2015; Ward et al., 2016). Due to the higher solar radiation absorption and increased anthropogenic heat induced by intense human activities (Deilamia et al., 2018; Doan et al., 2019), higher temperatures are usually detected in urban areas than in rural surroundings (Ward et al., 2016; Yao et al., 2017; Giridharana and Emmanuel, 2018). The urban heat island (UHI) effect and extreme high temperature events (EHTE) can significantly affect local climate situation, raise energy consumption, increase air pollutants, lead to thermal discomfort and even increase heat-related mortality (Ward et al., 2016; Morris et al., 2017; Li et al., 2018; Abbassi et al., 2019; Ingole et al., 2020). Thus, it is urgent for urban planners and climatologists to take appropriate measures to mitigate UHI effect (Chang et al., 2007; Ward et al., 2016; Morris et al., 2017; Deilamia et al., 2018).
As a nature-based solution, developing urban blue-green infrastructures (BGIs) has been widely accepted to alleviate UHI effect due to the environmental friendliness and high cost-effectiveness (Ward et al., 2016; Carvalho et al., 2017; Taleghani, 2018; Yu et al., 2020b). For containing both green areas and water bodies, urban parks are usually cooler than the surrounding developed area, known as the park cooing island (PCI) effect (Chang et al., 2007; Hwang et al., 2015; Aflaki et al., 2017; Yu et al., 2020b). The PCI intensity in previous studies varied with the location, size and shape of the sample parks, as well as the components and configurations in and around the urban parks (Bowler et al., 2010; Chen et al., 2012; Deilamia et al., 2018; Cheung and Jim, 2019; Su et al., 2021). Large parks with higher canopy coverage and leaf area index (LAI) value were generally considered to be more efficient in cooling the environment (Gill et al., 2007; Bowler et al., 2010; Zhou et al., 2017). However, in recent years, more and more researchers have reported that the cooling efficiency of urban parks depended on the area proportion of water body within the parks to some extent (Xu et al., 2019; Sun et al., 2020; Xie and Sun, 2021).
Further studies focused on the comparison of cooling effect between urban green spaces and water bodies (Liang et al., 2015; Gunawardena et al., 2017; Xu et al., 2019; Sun et al., 2020; Yu et al., 2020b). One investigation showed that water bodies were more sensitive and influential to the surrounding thermal environment than green spaces based on a comparison study on the cooling effect between 16 sample parks and 16 water bodies in Guilin, China. Another study conducted in Nanjing, China by Sun et al. (2020) recorded that the cooling range of water bodies (with the average value of 840 m) was longer than that of green spaces (with the average value of 540 m). Similar findings were reported in many other studies (Chen et al., 2012; Li et al., 2015; Wu et al., 2018; Xueru et al., 2018), which indicated that water bodies seemed more efficient in cooling the environment than green spaces due to the “oasis effects” and “thermostat effects” of water features (Oke, 1987; Spronken-smith et al., 2000). Generally, green spaces have been proved to effectively cool the surrounding area with about half width of the parks (Shashua-Bar and Hoffman, 2000), while water bodies can cool the environment with one width of themselves (Murakawa et al., 1991; Hathway and Sharples, 2012). Even, the average normalized cooling capability index (NCCI) of wetlands can be 42.3 times higher than that of green spaces (Xue et al., 2019).
Much attention has been gradually paid on the estimation of cooling effect of water bodies (Steeneveld et al., 2014; Cai et al., 2018; Wu et al., 2019; Su et al., 2021). The cooling distance of water bodies on their surroundings ranged from 100 m to 1,000 m in different studies (Sun and Chen, 2012; Tominaga et al., 2015; Cai et al., 2018; Gupta et al., 2018; Wu et al., 2018; Sun et al., 2020). The broad range of influencing distance indicated that the cooling efficiency of water bodies varied with the location, size, shape and their spatial pattern (Eckart et al., 2017; Gunawardena et al., 2017; Taleghani, 2018; Su et al., 2021). These studies were mainly conducted in different microenvironments based on the investigations of one or several individual water bodies. However, land surface temperature (LST) value is context sensitive and significantly influenced by the immediate microclimate (Deilamia et al., 2018; Cheung and Jim, 2019; Xie and Sun, 2021). The composition and configuration of land use/land cover (LULC) in the buffers around the sample water bodies differed from each other among previous studies, which might underestimate or overestimate the cooling benefits of water bodies (Hathway and Sharples, 2012; Cai et al., 2018; Gupta et al., 2018; Yu et al., 2020b).
Additionally, these studies discussed above well illustrated the heat exchange between individual water bodies and their surroundings, which provide evidence for understanding how water bodies affect microclimate (Kim et al., 2008; Du H. et al., 2016; Du S. et al., 2016; Moyer and Hawkins, 2017; Sun et al., 2020; Yu et al., 2020a). Nevertheless, they cannot provide universal information for urban planners or decision-makers to know how water resources balance or regulate urban thermal environment and what are the major factors in influencing the cooling effectiveness from a systematic perspective (Sun and Chen, 2012; Aflaki et al., 2017; Deilamia et al., 2018). The fact is that it is difficult to estimate the mitigating efficiency of water bodies on the environment in real urban context, due to the internal heterogeneity and complexities of LST values and surface characteristics in urban areas (Syafii et al., 2016; Yu et al., 2020a). It needs to be further explored that how water bodies work on the thermal environment in a real and complex urban area with the interaction effects of LULC features on LST into consideration (Hathway and Sharples, 2012; Cai et al., 2018; Xu et al., 2019; Wu et al., 2020).
This study, therefore, aims to explore the mitigating benefit of water resources on UHI effect in urban areas, taking urban water bodies as separate elements, water network system, as well as one landscape category of urban ecosystem. It was conducted in Wuhan, a typical city in China with rapid urbanization these years. There are abundant urban water resources within this city, which wins the title of River City. Nevertheless, it is also known as one of the Four Furnaces of China due to the hot and humid summer. Thus, Wuhan is an ideal case city to investigate the cooling effect of water bodies on the environment. The main objectives of this study are to: (1) illustrate the water cool island (WCI) effect and identify the WCI intensity in Wuhan urbanized area; (2) monitor the cooling effect of water bodies on the environment in a heterogeneous context; (3) detect the major factors that influence the cooling benefit of water bodies from a systemic perspective.
Wuhan (113°41′ ~ 115°05′E, 29°58′ ~ 31°22’N) is the capital of Hubei Province in China (Figure 1). Due to the north subtropical monsoon (humid) climate, Wuhan is characterized by well-defined seasons, abundant rainfall, and sufficient heat. As the Wuhan Statistical Yearbook recorded, the average annual temperature ranges from 15.8°C to 17.5°C and the extreme peak one is 41.3°C. The average annual precipitation is 1,269 mm, ranging from 1,150 mm to 1,450 mm. The precipitation mainly occurs from June to August, which accounts for about 40% of the total annual amount. Wuhan covers a total area of 8569.2 km2, with the built-up area of 812.4 km2. The average altitude is below 50 m, with the range of 19.2 m to 873.7 m. The urbanized areas are mainly covered by flatlands, with the rolling hills and mountains surrounded in the suburb. It is located at the intersection of the Yangtze River and its largest branch, the Han River. Hundreds of water bodies such as lakes, rivers and reservoirs are interweaved throughout the city. The Tangxun Lake in Wuhan is the largest urban lake in China, with an area of 47.6 km2. In recent years, rapid urbanization has led to a drastic decline in water area and serious fragmentation of water network, which significantly degrades the urban ecological function and decreases the ecological service value (Xie and Liu, 2020). The investigation was mainly concentrated in the urbanized area in Wuhan.
A Landsat 8 image acquired on August 3, 2020 (with the cloud volume of 1.9%) was downloaded from the website.1 It was geometrically corrected and registered according to a 1: 100000 map of Wuhan. After preprocessed with georeference, and clipping via boundary in Erdas 9.2, it was performed in ENVI to calculate the radiometric calibration value for each band (Chander and Markham, 2003; Xu, 2015). Then, a COST model was used for atmospheric correction to the image (Chavez, 1996; Xu, 2015).
A supervised classification method was performed in ENVI5.3 by a maximum likelihood algorithm to obtain LULC classification. Because LST is closely related to the surface features such as water content and surface radiative (Ward et al., 2016; Deilamia et al., 2018; Berihun et al., 2019), the study area was classified into three main categories: (1) construction land: area covered by impervious surfaces such as buildings, roads, squares and parking lots, (2) vegetated area: area covered by vegetation mainly including green spaces and a few cultivated land in urban areas, and (3) water body: area covered by reservoirs, lakes, rivers, ponds and wetlands. For each LULC category, more than 300 points were randomly selected as samples for accuracy verification. The overall classification accuracy and kappa coefficient are 92.94% and 0.89, respectively.
There are two thermal infrared bands (TIRS10 and TIRS11) for Landsat 8 image. A single-channel Algorithm was used to retrieve LST value only based on TIRS10 of the preprocessed image, for the calibration parameter of TIRS11 is not stable (Jiménez-Muñoz et al., 2014). It is important to correct the land surface emissivity (ɛ) according to LULC features when calculating LST values (Weng et al., 2004). In this study, construction land and water body were assigned a ɛ value of 0.9212 and 0.9908, respectively (Jiménez-Muñoz et al., 2014). For vegetated areas, ɛ value was corrected based on the modeling relationship with the NDVI values (Griend and Owe, 1993).
Generally, UHI intensity is defined as the temperature difference between urban areas and their surroundings (Oke, 1987; Voogt and Oke, 2003). Following this definition, WCI intensity was defined as the LST difference between water body and its surroundings (Wu et al., 2020). In this study, the WCI intensity can be calculated based on the following equation:
where WCI is the value of WCI intensity for a water body, T0 is the average LST value of the study area, and Tw is the LST value of the water body.
Previous studies reported that the influencing factors of water bodies on the cooling effect involved both spatial composition and configuration based on class and landscape level such as the indexes related to area, density, shape, edge feature, core patch, proximity, concentration or dispersion, and landscape diversity (Deilamia et al., 2018; Xie et al., 2018). However, researchers usually selected several potential factors related to the spatial characteristics of water bodies in advance (Moyer and Hawkins, 2017; Cai et al., 2018; Wu et al., 2020; Yu et al., 2020a). As a result, some more important variables might be omitted, which could affect the interpretation of WCI effect (Xie et al., 2018). Additionally, researchers might have significantly different findings from each other due to the varied selection of variables, resulting in the lack of comparability and universality of the conclusions (Yu et al., 2020b). To avoid these issues, 28 variables covering almost all the universal landscape metrics were involved to indicate the spatial arrangements of water bodies and the urban context (Wu, 2000). The calculation was conducted in Fragstats 4.2.
It is confirmed that the influencing factors and their impact on LST variation are scale-dependent (Li et al., 2013; Su et al., 2021). Thus, it is significant to determine an appropriate spatial scale (grid size in this study) when illustrating WCI effect in urban areas. Previous studies found that the influencing distance of water bodies on the environment was mostly about 300 m (Gupta et al., 2018; Wu et al., 2018). Considering the pixel size of the image (30 m × 30 m) and referring the previous relevant researches (Masson et al., 2014; Nassar et al., 2016; Cai et al., 2018), we set the grid size at 300 m × 300 m in this study. After eliminating those grids close to the boundary of the study area and with incomplete internal area, a total of 7,604 grids were generated in ArcGIS 10.2. For each grid, the average values of LST and all selected variables were calculated. A Pearson correlation analysis between LST and each variable was performed in SPSS 19.0 to indicate the effect of single factor on LST. A stepwise regression method was used to identify the main factors and to quantify their contribution to the LST variation in an urban context.
As Figure 2A drafted, the construction land spreads throughout the study area, covering an area of 409.2 km2 (Table 1). It became the dominant LULC type due to the high area proportion (59.7%) and spatial extension. Although the vegetated area covered 160.9 km2 (with the area proportion of 23.5%), they were spatially fragmented by the construction land. The area of water bodies was 115.4 km2, with the smallest area proportion of 16.8%.
Figure 2B demonstrates the spatial distribution map of LST values on August 3, 2020 in the urbanized area in Wuhan. The average LST value was 41.24°C with a wide range of 23.94°C to 72.98°C in the study area. The LST distribution well spatially corresponded to the LULC map (Figure 2A). The higher LST values usually distributed in construction land, with the medium ones covering greening area and lower ones concentrating in water bodies. Significant difference in LST values were detected among different LULC categories (Table 2). Construction land had the highest maximum and mean LST values (72.98°C and 44.61°C, respectively), much higher than those of Water body (46.45°C and 31.42°C, respectively). The difference values in maximum and mean LST between Construction land and Water body can reach 26.53°C and 13.19°C, respectively. Additionally, obvious difference in the minimum, maximum and mean LST values could also be observed between Vegetated area and Water body with the values of 2.40°C, 17.05°C and 8.24°C, respectively.
In this study, a total of 36 water bodies were involved. All of them showed a WCI effect, with the LST values within the water bodies lower than that in study area. The WCI intensity ranged from 1.1°C to 13.3°C, with the mean WCI value of 5.5°C (Table 3). The high standard deviation (SD) value (2.5°C) indicated that the WCI values significantly varied among different water bodies. It could be further supported by Figure 3. Of all 36 involved water bodies, 29 (80.5%) had the WCI intensity from 2°C to 8°C. Specifically, there were 13 water bodies with the WCI value of 6°C to 8°C, accounting for 36.1% of the water number. Three water bodies (about 8.3%) were detected to have the WCI intensity less than 2°C. Two water bodies (5.6%) had significant lower LST values than their environment, with the LST difference of more than 10°C.
To further explore the WCI variation, the relationships of WCI intensity with water area and shape were modeled. As Figure 4 shown, there existed significant correlations between the WCI intensity and both water area and shape index (SI), though the correlations were nonlinear. The positive correlations indicated that the WCI value tended to increase with the increase of water area and SI value. Expanding water area and complicating water shoreline helped to strengthen the WCI effect. In comparison, water size (Figure 4A) could provide better explanation (62.29%) in WCI variation than water shape (45.23%) (Figure 4B).
Figure 4. Scatterplots and fitting curves between the WCI intensity and (A) the area of water body at the significance level of 0.01 and (B) the shape index of water body at the significance level of 0.05 (n = 36).
To better understand how water bodies influence the thermal environment in urbanized area, 28 variables related to Water body (in Fragstats 4.2) were selected and statistically modeled with LST values based on 7,604 gridded samples. Table 4 lists the correlations coefficients between LST and the common water body-related variables. Except for density-related indexes, all variables were significantly correlated with LST values at the significance level of 0.01. In general, the variables indicate area features such as Class area (CA), Percent of landscape (PLAND), Core area percent of landscape (CPLAND) and Core area_mean (CORE_MN) had the closest correlation with LST, with the coefficients of −0.827, −0.829, −0.829 and − 0.796, respectively. Increasing the areas of water bodies and core patches in a grid can effectively reduce the internal LST value. Besides, the spatial relationship among individual water bodies could also influence the thermal environment. The variables such as Contiguity Index _mean (CONTIG_MN) and Patch cohesion index (COHESION) were negatively, while Similarity index_mean (SIMI_MN) and Landscape division index (DIVISION) positively correlated to LST values. Connecting different individuals as a whole might help to efficiently cool the urban environment.
Table 4. Correlation coefficients between LST and the main water-related variables with the grid of 300 × 300 m (n = 7,604).
Table 5 displays the multiple stepwise regression equations between LST values and 28 water body-related variables. Both the standardized regression equation and general one were modeled in this study. In the process of stepwise regression, only three water body-related variables, namely PLAND_W, Landscape shape index (LSI_W) and Contrast-weighted edge density (CWED_W), remained as the main influencing factors. These three variables combined to explain 69.0% of the LST variation among grids. The standardized regression model provided a clue that the index of PLAND_W was the major contributor of water bodies to cool urban environment, contributing much more than LSI_W and CWED_W. The LST value could be decreased by 1.03°C for each 10% increase in area proportion of water bodies, when the other variables keep unchanged.
To exhibit the interaction effect of water bodies with other elements on the urban thermal environment, 123 variables related to both water bodies and the other LULC types were involved. The Pearson correlation analysis was performed between LST and all landscape metrics (on both class and landscape levels). Due to the length limitation, the correlation coefficients were not fully displayed in this paper. Table 6 shows the multiple stepwise regression equations between LST values and all landscape metrics. Five variables, namely SIMI_MN_C (Similarity index_mean of Construction land), CA_C (Class area of Construction land), TECI_W (Total edge contrast index of Water body), LSI_W (Landscape shape index of Water body) and PLAND_V (Percent of landscape of Vegetated area), were entered into the ultimate principal component analysis. They contributed 71.9% to the LST variation.
Table 6. Principal component regression model between LST and all selected indexes with the grid of 300 × 300 m (n = 7,604).
The indexes of SIMI_MN_C and CA_C were the most dominant contributors to LST variation, which were both related to construction land. Although their contributions to LST variation were almost equal, one was positive and the other was negative. When considering the interaction of water bodies with other factors, TECI_W were detected to positively and LSI_W negatively affect LST increase. In urbanized area, to some extent, high TECI_W value meant a relatively higher area proportion of Construction land around the water body. The complex shape of water bodies can provide more opportunities for heat exchange between water body and its surroundings, which reduced the ambient LST. Unexpectedly, the vegetated area exhibited a very slight impact on LST variation, with the standardized regression coefficient of PLAND_V (0.036) much lower than those of the other land covers. Moreover, the positive coefficient indicated that the cooling benefit of Vegetated area in developed areas was offset by other factors.
It has been well demonstrated that the area of a water body is a significant variable affecting its cooling effect (Yu et al., 2020b; Su et al., 2021). Similar findings were detected in this study. A wide range of WCI intensity (1.1 ~ 13.3°C) existed in different water bodies (Table 3 and Figure 3). About 62.29% of the WCI variation could be explained by the size of water bodies (Figure 4A). Usually, a large-sized water body is less affected by the warmer surroundings due to the relatively stable microclimate (Xie et al., 2018; Cheung and Jim, 2019), which intensifies the WCI effect. In addition to keeping themselves cool, water bodies can also reduce the LST values of the surrounding environment (Tominaga et al., 2015; Gupta et al., 2018). This study found that the area of water bodies was negatively correlated to the LST values in grids sized at 30 m × 30 m, with the correlation coefficients of −0.827 (Table 4). It meant that the larger the water area, the lower the average LST of a grid. Water area could significantly influence the environment temperatures in urban areas, which was consistent with the previous studies (Sun and Chen, 2012; Sun et al., 2020; Wu et al., 2020).
In terms of the cooling mechanism of water bodies, PLAND_W was one of the main influencing factors and the most important contributor to LST variation among grids, with the standardized regression coefficient (−0.828) much higher than the other two (0.229 and 0.184) (Table 5). In this study, the index of PLAND_W had the same ecological meaning as CA_W base on a grid statistics, for the size of grids was given. Of all the water body-related variables, water area and its percentage contributed the most to the cooling benefit of water bodies on the environment. However, water area and the relevant variables no longer played a leading role in regulating the thermal environment when considering the interaction between water bodies and other land covers (Table 6). They were eliminated and the index of CA_C was introduced during the stepwise regression analysis. It can be partly attributed to the matrix characteristics of urban areas (Doan et al., 2019; Su et al., 2021). In the highly urbanized area, Construction land is the dominant LULC type (Figure 2A), which affects and even controls the ecological process (Ward et al., 2016; Deilami et al., 2018). The contribution of water area to the cooling benefit was depressed by the area of construction land in an urbanized context.
Previous studies confirmed that water shape was another important factor in influencing the WCI effect (Yu et al., 2020b; Su et al., 2021). In this study, when the 36 water bodies were involved into analysis as individual samples, the WCI intensity was found to be positively correlated to water shape (Figure 4B). This was inconsistent with the previous study (Du H. et al., 2016; Du S. et al., 2016), which found that water shape was negatively correlated to the WCI intensity and efficiency. This inconsistency could be attributed to the differences in sample water-body characteristics and WCI definition. The sample water bodies selected by Du et al. were all larger than 1 ha with small difference in area among different individuals. However, the samples in our study had a wide area range from 2.3 ha to 4787.3 ha (Figure 4A). Thus, the circumference and shape index of a water body might be closely related to its area (Xie et al., 2018), which had a great and positive impact on WCI intensity. In addition, they defined WCI intensity as the LST difference between a water body and the immediate surroundings, while we defined it as the difference between a water body and study area (Wu et al., 2020). This might affect the correlation between the two databases.
Two variables related to water shape, namely LSI and PARA_MN were both significantly correlated to the LST values in grids (Table 4). Water shape could obviously affect the cooling benefit of water bodies on the environment. The positive correlations meant that regularly shaped water bodies had better effect in cooling the environment. To some extent, complex water shape means an increased integration of water bodies with the surrounding land covers and frequent heat exchange with the warmer environment (Sun and Chen, 2012; Theeuwes et al., 2013). Moreover, the index of LSI_W was remained as one of the main influencing factors during the coupling analysis with multiple factors (Table 5). Water shape played a negative role in affecting the cooling benefit of water bodies when the selected water bodies were involved into the study as an infrastructure system. Water shape reflects not only its own features and the connection between water body and the surrounding world. The index of LSI_W was still the main contributor to LST variation when considering the interaction of water bodies and the surrounding context (Table 6). When the water bodies were integrated into the urban ecosystem as a landscape category, water shape provided a positive contribution to reduce the LST of the environment. The role transformation of water shape highlighted the significance of factor selection in explaining LST variation and water cooling benefits (Xie et al., 2018).
Both landscape composition and configuration were proved to have great influence on LST variation in urban areas (Aflaki et al., 2017; Deilamia et al., 2018; Su et al., 2021). This study confirmed that besides the composition metrics, the variables indicating landscape configuration also had significant impact on urban thermal environment. As shown in Table 4, the variables reflecting the spatial location, proximity and connection such as CONTIG_MN, SIMI_MN, DIVISION and COHESION for water bodies showed relatively strong correlation with LST values. The negative correlations between LST and CONTIG_MN and COHESION meant that enhancing the connectivity among different water bodies could effectively cool the urban environment. This finding was consistent with the previous studies (Sun et al., 2013; Du H. et al., 2016; Du S. et al., 2016; Peng et al., 2016). Theeuwes et al. (2013) reported that compared with a large water body, several smaller lakes equally distributed within the urban area expressed a weaker WCI intensity but a better capacity in cooling the environment. When the area is given, multiple small-sized water bodies distributed discretely can influence a larger area of the city. In most metropolises, urbanization leads to the serious fragmentation of water bodies (Yao et al., 2017; Xie and Liu, 2020). It is unrealistic to enlarge water bodies in urban areas (Deilamia et al., 2018; Yu et al., 2019). Connecting the dispersed water bodies to form an internally connected water network may be a good alternative.
Though urban water bodies could significantly influence the LST variation (Table 4), their contribution to thermal environment was weakened when the non-water related factors were considered (Table 6). As discussed in Section 4.1, the urbanized matrix characterizing by close connection and large amount of construction land influenced the regulation benefit of urban water bodies on thermal environment (Cai et al., 2018; Wu et al., 2020). When all variables were comprehensively considered, the biggest contributor to thermal environment was SIMI_MN_C (with the standardized regression coefficient of −0.745) (Table 6). High SIMI_MN_C value implies relatively long average distribution distance between construction lands. Fragmenting the contiguous construction land with water bodies and green spaces and developing a BGI system can be more efficient for climate adaption (Sun and Chen, 2012; Sun et al., 2020; Wu et al., 2020; Su et al., 2021).
Most previous studies illustrated the cooling effect of water bodies based on the correlation analysis with several landscape metrics (Deilamia et al., 2018; Taleghani, 2018; Yu et al., 2020b; Su et al., 2021). However, the selected variables varied with the study location, object, goal, scale and sample size (Sun and Chen, 2012; Tominaga et al., 2015; Sun et al., 2020; Wu et al., 2020), which leads to lack of comparability and universality between results. The main influencing factors could only be generated from the potential variables, which characterize by subjectivity and randomness (Xie et al., 2018; Su et al., 2021). This study involved all the landscape metrics in Fragstats 4.2, which avoided missing some important factors. For a given indicator, the interpretation for the regulation benefit of water bodies on thermal environment varied in different scenarios. For example, shape index alone could explain 45.23% of the WCI intensity variation (Figure 4B), while it was not the biggest contributor to cooling the environment (Table 5 and Table 6) due to different contexts and spatial scales. Selecting appropriate potential variables is an important prerequisite for representing the cooling mechanism of water bodies. An in-depth understanding of the ecological significance of indicators is the basis of implementation of the research findings.
Our results indicated that both landscape composition and configuration might significantly influence the cooling effect of water bodies (Table 4). It provided evidence that optimizing the landscape configurations of water bodies and land covers in urban areas was of great significance for improving the thermal environment (Du H. et al., 2016; Du S. et al., 2016). As expected, increasing urban water area or connecting the dispersed small water bodies could significantly improve the urban thermal environment (Sun et al., 2020; Su et al., 2021). Governing the expansion of construction land could slow down the thermal deterioration to some extent (Deilamia et al., 2018; Xie and Sun, 2021). However, it is difficult to control construction land area, as well as enlarge water bodies without limit in urban areas due to development needs (Giridharana and Emmanuel, 2018). This study found that SIMI_MN_C was the biggest contributor to LST variation in urban areas with multiple factors into consideration (Table 6). For urban planners and meteorologists, it provides a systemic perspective to understand the cooling effect of water bodies and develop a comprehensive water body network to combat the deteriorating thermal environment by considering the interactive effect of water body and other land covers.
This study was conducted at a grid of 300 m × 300 m to reveal the cooling capacity of water bodies, which referred the previous studies. The main findings in this study were from the statistical analysis based on the 7,604 samples. The uneven distribution of water bodies lead to a consequence that many grids have no water elements, which might partially affect the statistical results (Cao et al., 2010). Furthermore, the cooling effect of BGIs and its influencing factors are scale-dependent (Li et al., 2013; Su et al., 2021). This study was involved at only one single scale, which could not provide enough interpretation for the regulating benefit of water bodies on thermal environment. It needs to be further investigated at different scales in the next step. In addition, previous studies revealed that the WCI intensity was affected by the surrounding environment (Cai et al., 2018; Yu et al., 2020b). It is necessary to explore the cooling effect of urban water bodies in a varied context. This study focused on the spatial variation of context features, but did not pay attention to its temporal change. The dynamic regulation mechanism of urban water bodies on thermal environment should be discussed in future.
This study explored the cooling effect of urban water bodies in Wuhan based on three scenarios in which water bodies was regarded as individual samples, water network system and one landscape category of urban ecosystem, respectively. Our results revealed that urban water bodies could not only express WCI effect, but also cool the urban environment. All detected water bodies showed WCI effect with the mean WCI intensity of 5.5°C and range of 1.1°C to 13.3°C. Water area and shape index of individuals alone could explain 62.29 and 45.23% of the WCI variation, respectively. Both the features and the spatial relationship of water bodies could significantly affect the cooling benefit of water bodies on thermal environment. The main influencing factors were PLAND_W, LSI_W and CWED_W. An urban water body system with relatively larger water area, more regular boundary and simpler surroundings might be more efficient in cooling the urban environment. When considering the interaction of water bodies with other land covers on thermal environment, the contribution of water bodies to the cooling benefit was depressed. The most dominant contributors to LST variation in urban areas were CA_C and SIMI_MN_C, which were both related to construction land. It meant that in the real urban context, the interactive effect of other land covers must be taken into consideration when exploring the cooling benefit of urban water bodies. For urban planners and meteorologists, it is necessary to develop a comprehensive water body network by integrating the water bodies and other land covers to combat the deteriorating thermal environment. Developing an urban BGI system by connecting the dispersed water bodies and fragmenting the contiguous construction land in rapidly urbanized areas can be more realistic for mitigating UHI effect and adapting to climate change.
The original contributions presented in the study are included in the article/supplementary material, further inquiries can be directed to the corresponding author.
QX contributed to the conception and design of the research. LR and CY performed the investigation and data analysis. QX wrote the manuscript and and revised the manuscript. All authors contributed to the article and approved the submitted version.
This work was supported by MOE (Ministry of Education in China) Project of Humanities and Social Sciences (19YJCZH195) and the National Natural Science Foundation of China (41401186).
The authors declare that the research was conducted in the absence of any commercial or financial relationships that could be construed as a potential conflict of interest.
All claims expressed in this article are solely those of the authors and do not necessarily represent those of their affiliated organizations, or those of the publisher, the editors and the reviewers. Any product that may be evaluated in this article, or claim that may be made by its manufacturer, is not guaranteed or endorsed by the publisher.
Abbassi, Y., Ahmadikia, H., and Baniasadi, E. (2019). Prediction of pollution dispersion under urban heat island circulation for different atmospheric stratification. Build. Environ. 168:106374. doi: 10.1016/j.buildenv.2019.106374
Aflaki, A., Mirnezhad, M., Ghaffarianhoseini, A., Ghaffarianhoseini, A., Omrany, H., Wang, Z.-H., et al. (2017). Urban heat island mitigation strategies: a state-of-the-art review on Kuala Lumpur, Singapore and Hong Kong. Cities 62, 131–145. doi: 10.1016/j.cities.2016.09.003
Berihun, M. L., Tsunekawa, A., Haregeweyn, N., Meshesha, D. T., Adgo, E., Tsubo, M., et al. (2019). Exploring land use/land cover changes, drivers and their implications in contrasting agro-ecological environments of Ethiopia. Land Use Pol. 87:104052. doi: 10.1016/j.landusepol.2019.104052
Bowler, D. E., Buyung-Ali, L., Knight, T. M., and Pullin, A. S. (2010). Urban greening to cool towns and cities: a systematic review of the empirical evidence. Landsc. Urban Plan. 97, 147–155. doi: 10.1016/j.landurbplan.2010.05.006
Cai, Z., Han, G., and Chen, M. (2018). Do water bodies play an important role in the relationship between urban form and land surface temperature? Sust. Cities Soc. 39, 487–498. doi: 10.1016/j.scs.2018.02.033
Cao, X., Onishi, A., Chen, J., and Imura, H. (2010). Quantifying the cool island intensity of urban parks using ASTER and IKONOS data. Landsc. Urban Plan. 96, 224–231. doi: 10.1016/j.landurbplan.2010.03.008
Carvalho, D., Martins, H., Marta-Almeida, M., Rocha,, and Borrego, C. J. U. C. (2017). Urban resilience to future urban heat waves under a climate change scenario: a case study for Porto urban area (Portugal). Urban Clim. 19, 1–27. doi: 10.1016/j.uclim.2016.11.005
Chander, G., and Markham, B. (2003). Revised Landsat-5 TM radiometric calibration procedures and postcalibration dynamic ranges. IEEE Trans. Geosci. Remote Sens. 41, 2674–2677. doi: 10.1109/TGRS.2003.818464
Chang, C. R., Li, M. H., and Chang, S. D. (2007). A preliminary study on the local cool-island amplitude of Taipei city parks. Landsc. Urban Plan. 80, 386–395. doi: 10.1016/j.landurbplan.2006.09.005
Chavez, P. S. (1996). Image-based atmospheric corrections-revisited and improved. Photogramm. Eng. Remote. Sens. 62, 1025–1036. doi: 10.1016/0031-0182(96)00019-3
Chen, X., Su, Y., Li, D., Huang, G., Chen, W., and Chen, S. (2012). Study on the cooling effects of urban parks on surrounding environments using Landsat TM data: a case study in Guangzhou, Southern China. Int. J. Remote Sens. 33, 5889–5914. doi: 10.1080/01431161.2012.676743
Cheung, P. K., and Jim, C. Y. (2019). Differential cooling effects of landscape parameters in humid-subtropical urban parks. Landsc. Urban Plan. 192:103651. doi: 10.1016/j.landurbplan.2019.103651
Deilamia, K., Kamruzzamanb, M., and Liu, Y. (2018). Urban heat island effect: a systematic review of spatio-temporal factors, data, methods, and mitigation measures. Int. J. Appl. Earth Obs. Geoinf. 67, 30–42. doi: 10.1016/j.jag.2017.12.009
Doan, V. Q., Kusakaa, H., and Nguyen, T. M. (2019). Roles of past, present, and future land use and anthropogenic heat release changes on urban heat island effects in Hanoi, Vietnam: numerical experiments with a regional climate model. Sust. Cities Soc. 47:101479. doi: 10.1016/j.scs.2019.101479
Du, H., Song, X., Jiang, H., Kan, Z., Wang, Z., and Cai, Y. (2016). Research on the cooling island effects of water body: a case study of Shanghai, China. Ecol. Indic. 67, 31–38. doi: 10.1016/j.ecolind.2016.02.040
Du, S., Xiong, Z., Wang, Y. C., and Guo, L. (2016). Quantifying the multilevel effects of landscape composition and configuration on land surface temperature. Remote Sens. Environ. 178, 84–92. doi: 10.1016/j.rse.2016.02.063
Eckart, K., McPhee, Z., and Bolisetti, T. (2017). Performance and implementation of low impact development-a review. Sci. Total Environ. 607-608, 413–432. doi: 10.1016/j.scitotenv.2017.06.254
Estoque, R. C., Murayama, Y., and Myint, S. W. (2017). Effects of landscape composition and pattern on land surface temperature: an urban heat island study in the megacities of Southeast Asia. Sci. Total Environ. 577, 349–359. doi: 10.1016/j.scitotenv.2016.10.195
Gill, S., Handley, J., Ennos, R., and Pauleit, S. (2007). Adapting cities for climate change: the role of the green infrastructure. Built Environ. 33, 115–133. doi: 10.2148/benv.33.1.115
Giridharana, R., and Emmanuel, R. (2018). The impact of urban compactness, comfort strategies and energy consumption on tropical urban heat island intensity: a review. Sust. Cities Soc. 40, 677–687. doi: 10.1016/j.scs.2018.01.024
Griend, A. A. V. D., and Owe, M. (1993). On the relationship between thermal emissivity and the normalized difference vegetation index for natural surfaces. Int. J. Remote Sens. 14, 1119–1131. doi: 10.1080/01431169308904400
Gunawardena, K., Wells, M., and Kershaw, T. (2017). Utilising green and blue space to mitigate urban heat island intensity. Sci. Total Environ. 584-585, 1040–1055. doi: 10.1016/j.scitotenv.2017.01.158
Gupta, N., Mathew, A., and Khandelwal, S. (2018). Analysis of cooling effect of water bodies on land surface temperature in nearby region: a case study of Ahmedabad and Chandigarh cities in India. Egypt. J. Remote Sens. Space Sci. 22, 81–93. doi: 10.1016/j.ejrs.2018.03.007
Hathway, E. A., and Sharples, S. (2012). The interaction of rivers and urban from in mitigating the urban heat island effect: a UK case study. Build. Environ. 58, 14–22. doi: 10.1016/j.buildenv.2012.06.013
Hwang, Y. H., Lum, Q. J. G., and Chan, Y. K. D. (2015). Micro-scale thermal performance of tropical urban parks in Singapore. Build. Environ. 94, 467–476. doi: 10.1016/j.buildenv.2015.10.003
Ingole, V., Marí-Dell’Olmo, M., Deluca, A., Quijal, M., Borrell, C., Rodríguez-Sanz, M., et al. (2020). Spatial variability of heat-related mortality in Barcelona from 1992–2015: a case crossover study design. Int. J. Environ. Res. Public Health 17:2553. doi: 10.3390/ijerph17072553
Jiménez-Muñoz, J. C., Sobrino, J. A., Skoković, D., Mattar, C., and Cristobal, J. (2014). Land surface temperature retrieval methods from Landsat-8 thermal infrared sensor data. IEEE Geosci. Remote Sens. Lett. 11, 1840–1843. doi: 10.1109/LGRS.2014.2312032
Kim, Y. H., Ryoo, S. B., Baik, J. J., Park, I. S., Koo, H. J., and Nam, J. C. (2008). Does the restoration of an inner-city stream in Seoul affect local thermal environment? Theor. Appl. Climatol. 92, 239–248. doi: 10.1007/s00704-007-0319-z
Li, H. F., Li, Y. S., Lu, Z., Guo, K., and Peng, W. F. (2015). Analysis on the thermal environment effect of river corridor landscape. Geogr. Info. Sci. 31, 51–54. doi: 10.3969/j.issn.1672-0504.2015.04.011
Li, H., Meier, F., Lee, X. H., Chakraborty, T., Liu, J. F., Schaap, M., et al. (2018). Interaction between urban heat island and urban pollution island during summer in Berlin. Sci. Total Environ. 636, 818–828. doi: 10.1016/j.scitotenv.2018.04.254
Li, X. M., Zhou, W. Q., and Ouyang, Z. Y. (2013). Relationship between land surface temperature and spatial pattern of green space: what are the effects of spatial resolution. Landsc. Urban Plan. 114, 1–8. doi: 10.1016/j.landurbplan.2013.02.005
Liang, B., Ma, Y., and Li, H. (2015). esearch on cooling effect of the landscape green space and urban water in Guilin city. Ecology and Environmental Sciences 24, 278–285. doi: 10.16258/j.cnki.1674-5906.2015.02.015
Masson, V., Marchadier, C., Adolphe, L., Aguejdad, R., Avner, P., Bonhomme, M., et al. (2014). Adapting cities to climate change: a systemic modelling approach. Urban Clim. 10, 407–429. doi: 10.1016/j.uclim.2014.03.004
Mohan, M., and Kandya, A. (2015). Impact of urbanization and land-use/land-cover change on diurnal temperature range: a case study of tropical urban airshed of India using remote sensing data. Sci. Total Environ. 506-507, 453–465. doi: 10.1016/j.scitotenv.2014.11.006
Morris, K. I., Chan, A., Morris, K. J. K., Ooi, M. C. G., Oozeer, M. Y., Abakr, Y. A., et al. (2017). Impact of urbanization level on the interactions of urban area, the urban climate, and human thermal comfort. Appl. Geogr. 79, 50–72. doi: 10.1016/j.apgeog.2016.12.007
Moyer, A. N., and Hawkins, T. W. (2017). River effects on the heat island of a small urban area. Urban Clim. 21, 262–277. doi: 10.1016/j.uclim.2017.07.004
Murakawa, S., Sekine, T., Narita, K. I., and Nishina, D. (1991). Study of the effects of a river on the thermal environment in an urban area. Energ. Buildings 16, 993–1001. doi: 10.1016/0378-7788(91)90094-J
Nassar, A. K., Blackburn, G. A., and Whyatt, J. D. (2016). Dynamics and controls of urban heat sink and island phenomena in a desert city: development of a local climate zone scheme using remotely-sensed inputs. Int. J. Appl. Earth Obs. Geoinf. 51, 76–90. doi: 10.1016/j.jag.2016.05.004
Peng, J., Xie, P., Liu, Y. X., and Ma, J. (2016). Urban thermal environment dynamics and associated landscape pattern factors: a case study in the Beijing metropolitan region. Remote Sens. Environment 173, 145–155. doi: 10.1016/j.rse.2015.11.027
Pickett, S. T. A., Cadenasso, M. L., Grove, J. M., Boone, C. G., Groffman, P. M., Irwin, E., et al. (2011). Urban ecological systems: scientific foundations and a decade of progress. J. Environ. Manag. 92, 331–362. doi: 10.1016/j.jenvman.2010.08.022
Shashua-Bar, L., and Hoffman, M. E. (2000). Vegetation as a climatic component in the design of an urban street: an empirical model for predicting the cooling effect of urban green areas with trees. Energ. Buildings 31, 221–235. doi: 10.1016/S0378-7788(99)00018-3
Spronken-smith, R. A., Oke, T. R., and Lowry, W. P. (2000). Advection and the surface energy balance across an irrigated urban park. Int. J. Climatol. 20, 1033–1047. doi: 10.1002/1097-0088(200007)20:9<1033::AID-JOC508>3.0.CO;2-U
Steeneveld, G., Koopmans, S. A., Heusinkveld, B. G., and Theeuwes, N. (2014). Refreshing the role of open water surfaces on mitigating the maximum urban heat island effect. Landsc. Urban Plan. 121, 92–96. doi: 10.1016/j.landurbplan.2013.09.001
Su, W. X., Chang, Q., Liu, X., and Zhang, L. K. (2021). Cooling effect of urban green and blue infrastructure: a systematic review of empirical evidence. Acta Ecol. Sin. 41, 2902–2917 (in Chinese). doi: 10.5846/stxb201903290607
Sun, R., and Chen, L. (2012). How can urban water bodies be designed for climate adaptation? Landsc. Urban Plan. 105, 27–33. doi: 10.1016/j.landurbplan.2011.11.018
Sun, R. H., Lü, Y. H., Chen, L. D., Yang, L., and Chen, A. (2013). Assessing the stability of annual temperatures for different urban functional zones. Build. Environ. 65, 90–98. doi: 10.1016/j.buildenv.2013.04.001
Sun, X., Tan, X. Y., Chen, K. L., Song, S., Zhu, X. D., and Hou, D. L. (2020). Quantifying landscape-metrics impacts on urban green-spaces and water-bodies cooling effect: the study of Nanjing, China. Urban For. Urban Green. 55:126838. doi: 10.1016/j.ufug.2020.126838
Syafii, N. I., Ichinose, M., Wong, N. H., Kumakura, E., Jusuf, S. K., and Chigusa, K. (2016). Experimental study on the influence of urban water body on thermal environment at outdoor scale model. Procedia Eng. 169, 191–198. doi: 10.1016/j.proeng.2016.10.023
Taleghani, M. (2018). Outdoor thermal comfort by different heat mitigation strategies-a review. Renew. Sust. Energ. Rev. 81, 2011–2018. doi: 10.1016/j.rser.2017.06.010
Theeuwes, N., Solcerová, A., and Steeneveld, G. (2013). Modeling the influence of open water surfaces on the summertime temperature and thermal comfort in the city. J. Geophys. Res. Atmos. 118, 8881–8896. doi: 10.1002/jgrd.50704
Tominaga, Y., Sato, Y., and Sadohara, S. (2015). CFD simulations of the effect of evaporative cooling from water bodies in a micro-scale urban environment: validation and application studies. Sust. Cities Soc. 19, 259–270. doi: 10.1016/j.scs.2015.03.011
Voogt, J. A., and Oke, T. R. (2003). Thermal remote sensing of urban climates. Remote Sens. Environ. 86, 370–384. doi: 10.1016/S0034-4257(03)00079-8
Ward, K., Lauf, S., and Kleinschmit, B. (2016). Heat waves and urban heat islands in Europe: a review of relevant drivers. Sci. Total Environ. 569-570, 527–539. doi: 10.1016/j.scitotenv.2016.06.119
Weng, Q., Lu, D. S., and Schubring, J. (2004). Estimation of land surface temperature-vegetation abundance relationship for urban heat island studies. Remote Sens. Environ. 89, 467–483. doi: 10.1016/j.rse.2003.11.005
Wu, J., (2000). Landscape Ecology: Pattern, Process, Scale and Hierarchy. Beijing: Higher Education Press.
Wu, C., Li, J., Wang, C., Song, C., Chen, Y., Finka, M., et al. (2019). Understanding the relationship between urban blue infrastructure and land surface temperature. Sci. Total Environ. 694:133742. doi: 10.1016/j.scitotenv.2019.133742
Wu, J., Li, C., Zhang, X., Zhao, Y., Liang, J., and Wang, Z. (2020). Seasonal variations and main influencing factors of the water cooling islands effect in Shenzhen. Ecol. Indic. 117:106699. doi: 10.1016/j.ecolind.2020.106699
Wu, D., Wang, Y., Fan, C., and Xia, B. (2018). Thermal environment effects and interactions of reservoirs and forests as urban blue-green infrastructures. Ecol. Indic. 91, 657–663. doi: 10.1016/j.ecolind.2018.04.054
Xie, Q. J., Duan, L. H., and Wang, Z. X. (2018). Impact of urban landscape pattern on spatial distribution of thermal field in summer: a case study of Wuhan. Resour. Environ. Yangtze Basin 27, 1735–1744 (in Chinese). doi: 10.11870/cjlyzyyhj201808009
Xie, Q. J., and Liu, J. H. (2020). Spatio-temporal dynamics of lake distribution and their impact on ecosystem service values in Wuhan urbanized area during 1987-2016. Acta Ecol. Sin. 40, 7840–7850 (in Chinese). doi: 10.5846/stxb201806281432
Xie, Q. J., and Sun, Q. (2021). Monitoring thermal environment deterioration and its dynamic response to urban expansion in Wuhan, China. Urban Clim. 39:100932. doi: 10.1016/j.uclim.2021.100932
Xu, H. Q. (2015). Retrieval of the reflectance and land surface temperature of the newly-launched Landsat 8 satellite. Chin. J. Geophys. 58, 741–747 (in Chinese). doi: 10.6038/cjg20150304
Xu, X., Liu, S., Sun, S., Zhang, W., Liu, Y., Lao, Z., et al. (2019). Evaluation of energy saving potential of an urban green space and its water bodies. Energ. Buildings 188-189, 58–70. doi: 10.1016/j.enbuild.2019.02.003
Xue, Z., Hou, G. L., Zhang, Z. S., Lyu, X., Jiang, M., Zou, Y. C., et al. (2019). Quantifying the cooling-effects of urban and peri-urban wetlands using remote sensing data: case study of cities of Northeast China. Landscape Urban Plan 182, 92–100. doi: 10.1016/j.landurbplan.2018.10.01
Xueru, Z., Xiao, J., and Yue, Q. (2018). “Ecological land cold island effect evaluation based on land surface temperature retrieval” in 2018 7th International Conference on Agro-Geoinformatics, Agro-Geoinformatics, 1–4. doi: 10.1109/agro-geoinformatics.2018.8476105
Yao, R., Wang, L. C., Huang, X., Niu, Z. G., Liu, F. F., and Wang, Q. (2017). Temporal trends of surface urban heat islands and associated determinants in major Chinese cities. Sci. Total Environ. 609, 742–754. doi: 10.1016/j.scitotenv.2017.07.217
Yu, K., Chen, Y., Liang, L., Gong, A., and Li, J. (2020a). Quantitative analysis of the interannual variation in the seasonal water cooling island (WCI) effect for urban areas. Sci. Total Environ. 727:138750. doi: 10.1016/j.scitotenv.2020.138750
Yu, Z. W., Yang, G. Y., Zuo, S. D., Jørgensen, G., Koga, M., and Vejre, H. (2020b). Critical review on the cooling effect of urban blue-green space: a threshold-size perspective. Urban For. Urban Green. 49:126630. doi: 10.1016/j.ufug.2020.126630
Yu, Z., Yao, Y., Yang, G., Wang, X., and Vejre, H. (2019). Spatiotemporal patterns and characteristics of remotely sensed regional heat islands during the rapid urbanization (1995–2015) of southern China. Sci. Total Environ. 674, 242–254. doi: 10.1016/j.scitotenv.2019.04.088
Keywords: urban heat island, blue infrastructure, landscape heterogeneity, interaction effect, climate adaptation
Citation: Xie Q, Ren L and Yang C (2023) Regulation of water bodies to urban thermal environment: Evidence from Wuhan, China. Front. Ecol. Evol. 11:983567. doi: 10.3389/fevo.2023.983567
Received: 01 December 2022; Accepted: 17 March 2023;
Published: 06 April 2023.
Edited by:
Ümüt Halik, Xinjiang University, ChinaReviewed by:
Lei Shi, Shandong Jianzhu University, ChinaCopyright © 2023 Xie, Ren and Yang. This is an open-access article distributed under the terms of the Creative Commons Attribution License (CC BY). The use, distribution or reproduction in other forums is permitted, provided the original author(s) and the copyright owner(s) are credited and that the original publication in this journal is cited, in accordance with accepted academic practice. No use, distribution or reproduction is permitted which does not comply with these terms.
*Correspondence: Qijiao Xie, eGllcWlqaWFvQDEyNi5jb20=
Disclaimer: All claims expressed in this article are solely those of the authors and do not necessarily represent those of their affiliated organizations, or those of the publisher, the editors and the reviewers. Any product that may be evaluated in this article or claim that may be made by its manufacturer is not guaranteed or endorsed by the publisher.
Research integrity at Frontiers
Learn more about the work of our research integrity team to safeguard the quality of each article we publish.