- 1Laboratório de Fauna e Unidades de Conservação, Departamento de Engenharia Florestal, Faculdade de Tecnologia, Universidade de Brasília, Brasília, DF, Brazil
- 2Pyron Lab, Columbian College of Arts & Science, Department of Biological Sciences, The George Washington University, Washington, DC, United States
- 3Coleção Herpetológica da Universidade de Brasília, Departamento de Zoologia, Instituto de Biologia, Universidade de Brasília, Brasília, DF, Brazil
- 4Department of Biology, University of Richmond, Richmond, VA, United States
Contrasting environmental conditions across geographic space might promote divergent selection, making adaptation to local biotic and abiotic conditions necessary for populations to survive. In order to understand how populations adapt to different environmental conditions, studies of local adaptation have been largely used as an interface to address ecological and evolutionary questions. Here, we studied populations of Gymnodactylus amarali (Phyllodactylidae) isolated in rapidly created artificial islands. We combined a genotyping-by-sequence (GBS) survey and redundancy analyses (RDA) to investigate genotype–environment associations (GEA), while DAPC, Fst, and Admixture analyses were used to determine genetic structure. Our hypothesis is that G. amarali populations on the islands are going through a local adaptation process and consequently becoming genetically different from the populations on the mainland. Our results indicate that geographic and environmental differences are related to genetic variation, as we detected the presence of two or three distinct genetic lineages in Serra da Mesa, Minaçu, and Colinas do Sul. Fst analysis shows moderate isolation between Serra da Mesa and Minaçu (0.082). The RDA pointed out potential local genetic signal correlated with temperature and precipitation. We identified 230 candidate loci associated with the environment and at least two locally structured subpopulations (Serra da Mesa and Minaçu) show significant association with environmental variation.
1 Introduction
Spatial environmental variation is ubiquitous and has been increased by anthropogenic forces (Albert et al., 2023). Contrasting conditions across geographic space can promote divergent selection (Wadgymar et al., 2022), making adaptation to local biotic and abiotic conditions necessary for populations to survive (Savolainen et al., 2013). In order to understand how populations adapt to different environmental conditions, studies of local adaptation have been largely used as an interface to address ecological and evolutionary questions (Hofmeister et al., 2019). Ecological selection in local populations in regions with environmental constraints such as divergent selection might quickly promote populations to move to distinct optimum trait values (Pyron et al., 2015). As a result of this process, populations would show weak genetic differentiation and strong morphological changes if lineage maintains gene flow, but phenotypic plasticity is common (Hu et al., 2019). Alternatively, populations under strong divergent landscape selection could present high genetic differences but low morphological disparity, due to reduce gene flow but high conservatism in trait optimum (Pyron et al., 2015). Nonetheless, selection would sort genotypes in each population that has a higher relative fitness for other genotypes comprising the region pool of interest (Kawecki and Ebert, 2004).
Ecological specialization resulting from local adaptation may culminate in speciation when local adaptation is a significant component of responses to environments going through changes (Savolainen et al., 2013). For example, climatic and geographic change caused by human activities is ruthlessly impacting biodiversity on our planet (Scheffers et al., 2016). Organisms carrying genetic variation for climate-related traits are expected to possess the capacity of adapting rapidly to changing environments (Bay et al., 2018). Commonly, these traits which allow local adaptations are polygenic quantitative traits, and it is a highly demanding task to identify the loci that control variation in such traits (Savolainen et al., 2013). A very important first step aiming at establishing evidence of local adaptation is the measurement of genome-wide patterns of variation using, for instance, large-scale single nucleotide polymorphism (SNP) genotyping arrays (Schweizer et al., 2016).
Savannas, deserts, semi-arid regions, and other open habitats are known as high-diversity areas for lizard faunas. The Brazilian Cerrado is a unique savanna hotspot (Nogueira et al., 2010; Azevedo et al., 2016) with both daily and seasonal shifts in temperature and moisture (Vitt et al., 2007). The Maranhão Naked-toed Gecko Gymnodactylus amarali Barbour (1925) (Phyllodactylidae) is a termitaria specialist, living in termite mounds – a microhabitat that buffers the strong variation in temperature and its damaging effects on organisms’ time of activity. However, populations from areas where termite mounds are absent were reported using rocky habitats as refuge (Vitt et al., 2007), which exposes them to higher temperature and moisture variations. Furthermore, most Cerrado lizards reproduce during the rainy season and this reproductive seasonality is determined by fluctuations in arthropod abundance, which are related to rainfall fluctuations (Colli et al., 2002). Most reproductive individuals of G. amarali, however, were observed during the dry season (Colli et al., 2003). In this study, we investigate populations of the gecko G. amarali isolated in rapidly created artificial islands resulting from the flooding of the Serra da Mesa Hydroelectric Plant reservoir, in Central Brazil.
The Serra da Mesa reservoir flooding started in 1996: 170,000 ha of area was flooded, valleys were submerged, and hilltops became ~ 290 islands (Amorim et al., 2017). Local adaptations may appear rapidly according to biogeography and studies on contemporary adaptation (Savolainen et al., 2013). High degrees and rapid onset of local adaptation are usually expected in isolated populations, which are generally free from the homogenizing effects of high gene flow (Hofmeister et al., 2021). However, most studies in this field focused on rapid adaptation to local conditions in response to new selection regimes that some taxa encountered in the process of range expansion (e.g., cane toads, sticklebacks, honeybees, steelhead trout, deer mice; see Hofmeister et al., 2021). Amorim et al. (2017) demonstrated in a study analyzing morphology and diet parameters that only 15 years of isolation were enough for G. amarali to present a significant head size increase and consequent ecological niche breadth expansion. Lizards living on the islands have disproportionally larger heads compared to adjacent mainland populations. Furthermore, all five island populations presented this morphology shift independently (Amorim et al., 2017). Due to ecological release, after the extinction of larger lizards on the islands, all five populations went through a process of parallel local adaptation (Amorim et al., 2017).
Herein, we focused on testing for correlations between SNPs and environmental predictors and genetic divergence between island and mainland populations in this termite specialist gecko. We combined a genotyping-by-sequence (GBS) survey and redundancy analyses to investigate genotype–environment associations (GEA), while Discriminant Analysis of Principal Components (DAPC), total genetic variance (Fst), and Admixture analyses were used to determine genetic structure. Our hypothesis is that Gymnodactylus amarali populations on the islands are experiencing local adaptative pressures from natural selection, and consequently becoming genetically different from the populations on the mainland. We found support for both spatial genetic structure (i.e., geographic lineages) and candidate loci association with climatic factors among sites, including significant variation for Serra da Mesa populations. These results offer a direction for future research in this system to identify the genomic basis and functional differences in selected alleles between populations.
2 Materials and methods
2.1 Sequencing and bioinformatics
We obtained 46 tissues (liver and muscle) of Gymnodactylus amarali from the CHUNB and LAFUC collections at the Universidade de Brasília, from the municipalities of Colinas do Sul (one individual), Minaçu (20 individuals), and from 10 sites in and around the Serra da Mesa reservoir (25 individuals), Goiás state, Brazil (Figure 1; see Appendix S1 for sample numbers, localities, and associated data). We extracted genomic DNA using Qiagen DNEasy kits and visualized the results on a 2% agarose gel. We sent ~30mg to the UWBC Biotechnology Center for genotype-by-sequencing (GBS) analysis (Elshire et al., 2011). The samples were optimized using the ApeKI enzyme for digestion, bead cleanup for size selection, amplification using 18 PCR cycles, and a post-PCR normalization step prior to sequencing. We sequenced each sample for an average of ~6.5 million 150bp paired-end reads using an Illumina NovaSeq6000. We processed the raw reads to extract SNP calls using ipyrad 0.9.59 (Eaton and Overcast, 2020) using the denovo pairgbs pipeline on the GW HPCC Pegasus cluster (MacLachlan et al., 2020). We used ‘C[W]GC’ to identify the ApeKI restriction overhang, and employed strict filtering and trimming for adapter sequence and quality of reads. We generally used the default parameters for SNP calling (e.g., a depth of 6 for majority rule and statistical base-calling), with a 90% clustering threshold as a stringent compromise between over-splitting and over-lumping of loci as recommended by the authors. We retained loci called for ~80% of individuals (i.e., 37 of 46), resulting in 2,068 loci and 2,808 SNPs, for a total alignment length of up to 113,439bp per individual.
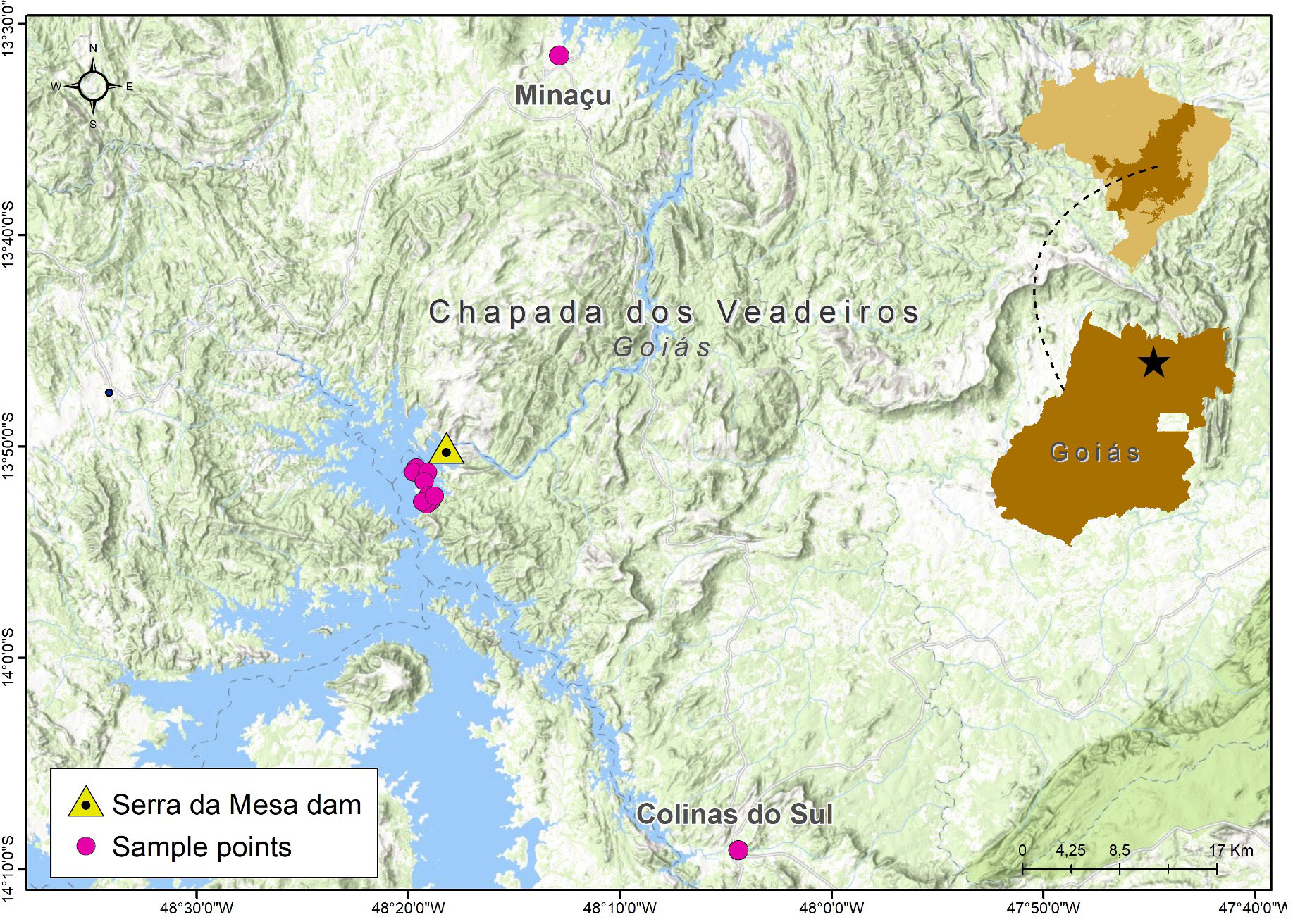
Figure 1 Map showing the three detected clusters: two “mainland” clusters, Minaçu and Colinas do Sul, which are genetically distinct from each other and from Serra da Mesa.
2.2 Population structure and multilocus adaptation
The full SNP matrix corresponds to 46 individuals and 2,808 SNPs, which after filtering out non-polymorphic sites and loci with more than 20% missing data, resulted in the final dataset of 41 individuals and 2,648 loci. We used this dataset to estimate heterozygosity, inbreeding coefficient (Fis), and total genetic variance (Fst). We tested for population structure using the DAPC algorithm in the R package ‘adegenet’ (Jombart and Collins, 2015), which produces a principal-component reduction of the SNP matrix for which discriminant functions can be used to estimate membership probability in an optimally-determined number of clusters. The DAPC is a powerful tool to summarize the genetic divergence found between groups while disconsidering within-group variation (Jombart et al., 2010). We determined the optimal number of clusters by cross-validation and retained the number of PCs achieving the lowest mean squared error. These were then compared to the sites (Colinas do Sul, Minaçu, and the 10 sites within Serra da Mesa, five islands and five locations on the reservoir margin) to determine the extent of local population structure within Gymnodactylus amarali in the study region (see Domingos et al., 2014). In addition, we ran the summary genetic statistics and DAPC without the Colinas do Sul individual (Supplementary Material). We further tested population structure using Admixture (Alexander et al., 2009) using cross-validation to test the best-fit K value to the data.
We then tested for multilocus adaptation using Redundancy Analysis (RDA) to detect loci under potential selection in a genotype–environment association framework (Rellstab et al., 2015; Forester et al., 2018). Recent studies showed that RDA-based methods are efficient for detection of local adaptation signatures (Forester et al., 2016). This analysis presented a combination of low false-positive and high true-positive rates throughout weak, moderate, and strong multilocus selection when compared to other methods (Forester et al., 2016), whereas RDA genome scans presented greater statistical power than scans based on PCA, by allowing individual-based simulations (Capblancq et al., 2018).
We predicted that temporal, spatial, and climatic axes in concert may all have resulted in weakly covarying signatures in the molecular data, for which we tested the response of the SNP data to the multivariate environment for detection of candidate genes (Schweizer et al., 2016). We employed this multivariate ordination technique in the R package ‘vegan’ (Oksanen et al., 2008). The response variable was the 46 individuals by 2,808 SNP matrix, with the 50,087 missing values imputed as the most common genotype at each SNP across individuals, since RDA requires complete entries for all values; for a full description of this method, see Forester et al. (2018).
2.3 Environmental predictors
Our data set was designed to reflect the relationship between genetic divergence and the local climate experienced by G. amarali. For predictor variables, we included a set of weakly-correlated climatic variables from the 30-second BIOCLIM dataset (Fick and Hijmans, 2017). Unfortunately, we did not have data collected locally in the sites at the time specimens were collected. We identified environmental variation at each sampling location using the R package ‘raster’ (Hijmans and van Etten, 2012), then we extracted values for all 19 variables at the 10 sites and estimated collinearity with r < 0.7 as a cutoff (Dormann et al., 2013). This yielded a reduced set of four weakly correlated climatic variables consisting of annual mean temperature (BIO1), isothermality (BIO3), annual precipitation (BIO12), and precipitation seasonality (BIO15). These variables presented relatively low variance inflation factors (<10): BIO1 (VIF = 8.03), BIO3 (2.85), BIO12 (8.28), and BIO15 (6.43). Annual mean temperature and isothermality are parameters associated with the diurnal and annual temperature variation that lizards endure in that region, whereas annual precipitation and precipitation seasonality inform about the humidity available in the environment across periods.
3 Results
3.1 Genetic diversity structure of Gymnodactylus amarali
Heterozygosity levels vary slightly across sites, Minaçu (Ho = 0.12, He = 0.14) and Colinas do Sul (Ho = 0.12, He = NA) have slightly higher observed and expected diversity (Minaçu only) of alleles relative to Serra da Mesa (Ho = 0.11, He = 0.12). It was not possible to estimate the expected heterozygosity and inbreeding coefficient (Fis) for Colinas do Sul given that our sampling includes solely one individual from this locality.
Using the function ‘xvalDapc,’ we detected the optimal number of PCs achieving the lowest mean squared error as equal to 25. Then, we used this number of clusters to run the DAPC (Figure 2). The histogram shows that this division in three subpopulations explains a large proportion of the genetic variance in G. amarali (Figure 2). Moreover, the DAPC for these subpopulations show strong genetic structure and no shared haplotypes (Figure 3), whereas Admixture identified two subpopulations (Figure 4). The second-best fit K with lowest error rate was K = 3 (Supplementary Material), showing alleles of Colinas do Sul within Minaçu. Individual island and reservoir margin populations are differentiated from each other. However, the Fst analysis shows moderate isolation between Minaçu and Serra da Mesa (0.082). Values of the inbreeding coefficient are higher for Minaçu (Fis = 0.103) than for Serra da Mesa (Fis = 0.064).
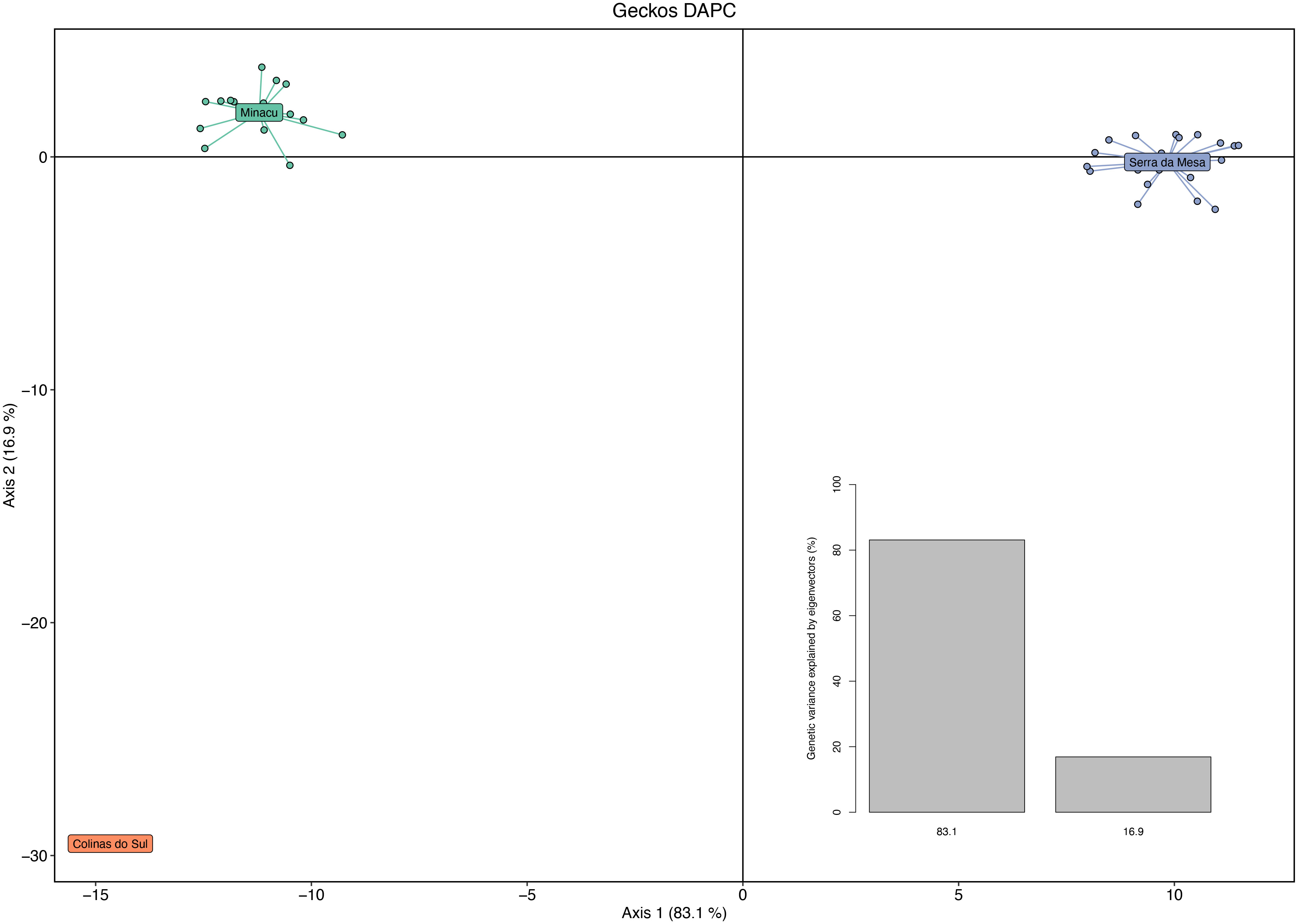
Figure 2 Axes 1 and 2 of Discriminant Analysis of Principal Components (DAPC) of 41 individuals from Serra da Mesa and the other two localities: Colinas do Sul and Minaçu. Analysis eigenvalues are shown inset.
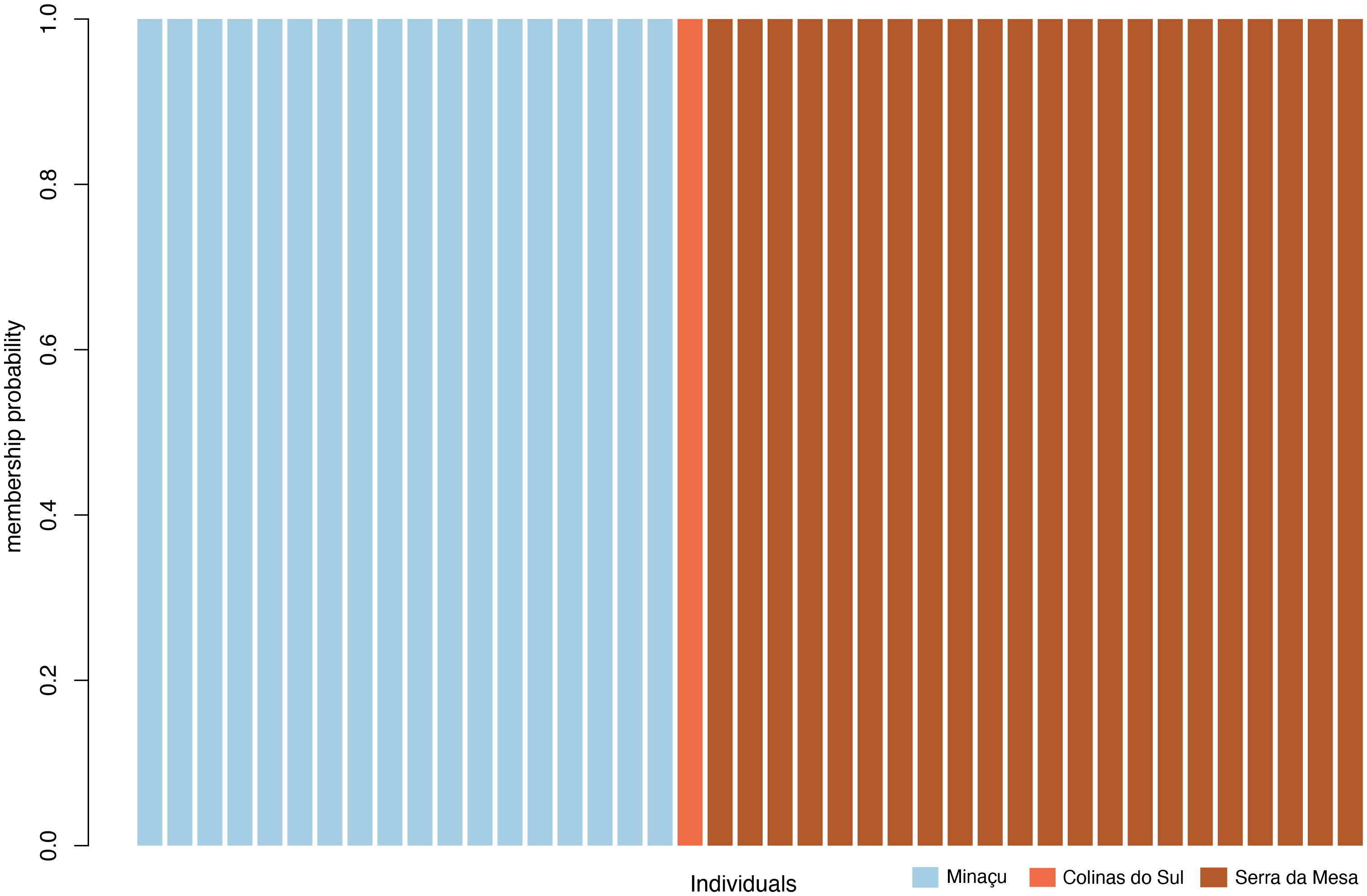
Figure 3 Genetic membership plot showing the separation of Gymnodactylus amarali in three subpopulations.
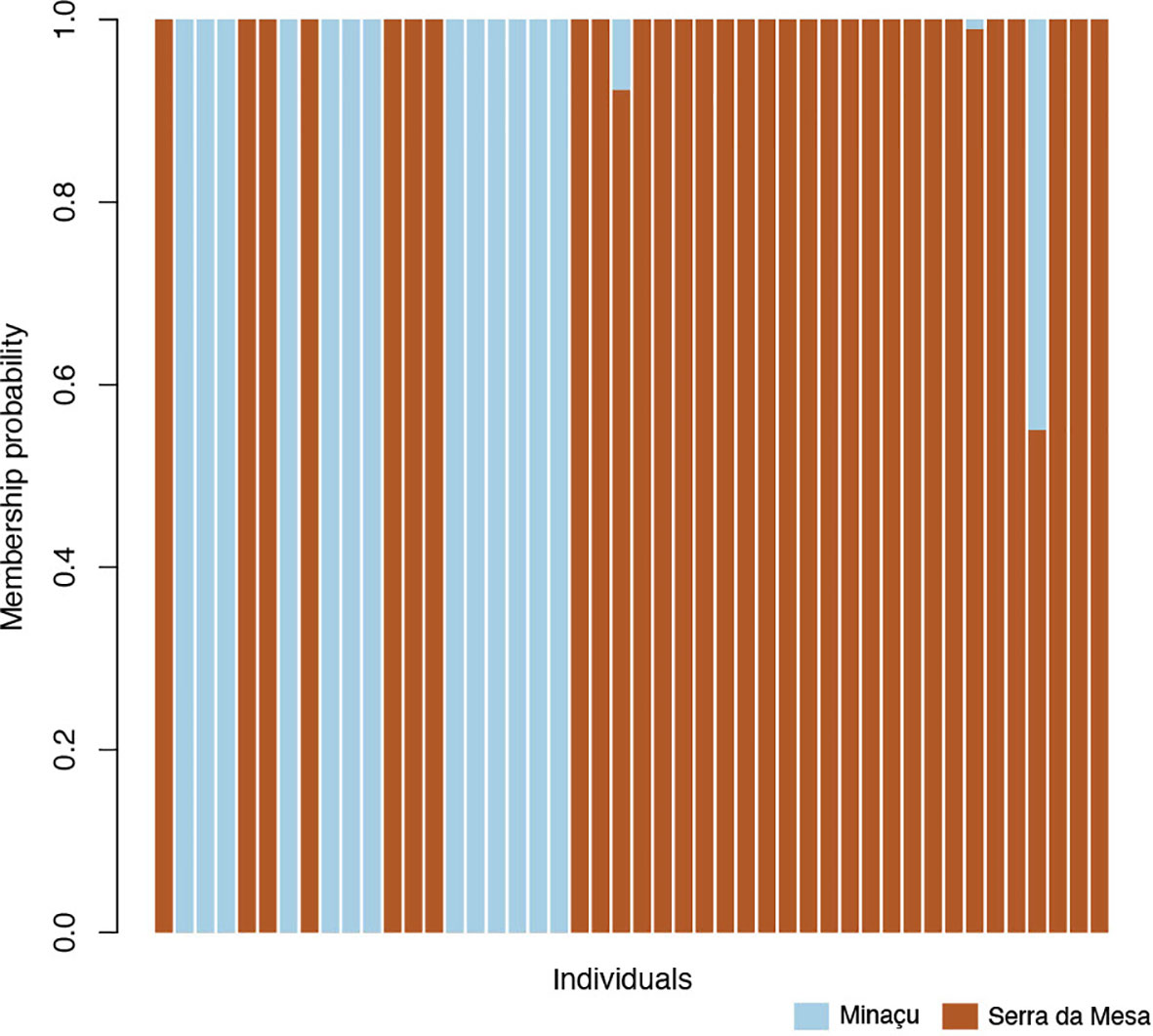
Figure 4 Admixture plot showing the results for K = 2. In brown are individuals from Serra da Mesa and in blue are individuals from Minaçu. Note that seven individuals from Serra da Mesa were assigned to Minaçu subpopulation. In Serra da Mesa we observed a hybrid with almost 50% representation in the genome from each subpopulation.
3.2 Redundancy analysis
The proportion of the variance explained by the environmental predictors in the Redundancy Analysis is 37.5% for RDA1, 30.7% for RDA2, and 16.4% for RDA3. Isothermality loads positively whereas annual precipitation, annual mean precipitation, and precipitation seasonality show negative loadings on axis 1. On axis 2, annual precipitation loads negatively whereas annual mean temperature, precipitation seasonality, and isothermality have positive loadings (Figure 5). The main contrast was related to precipitation and temperature, which is in accordance with the Cerrado seasonality. A similar pattern is observed for the comparisons with RDA1 vs RDA3.
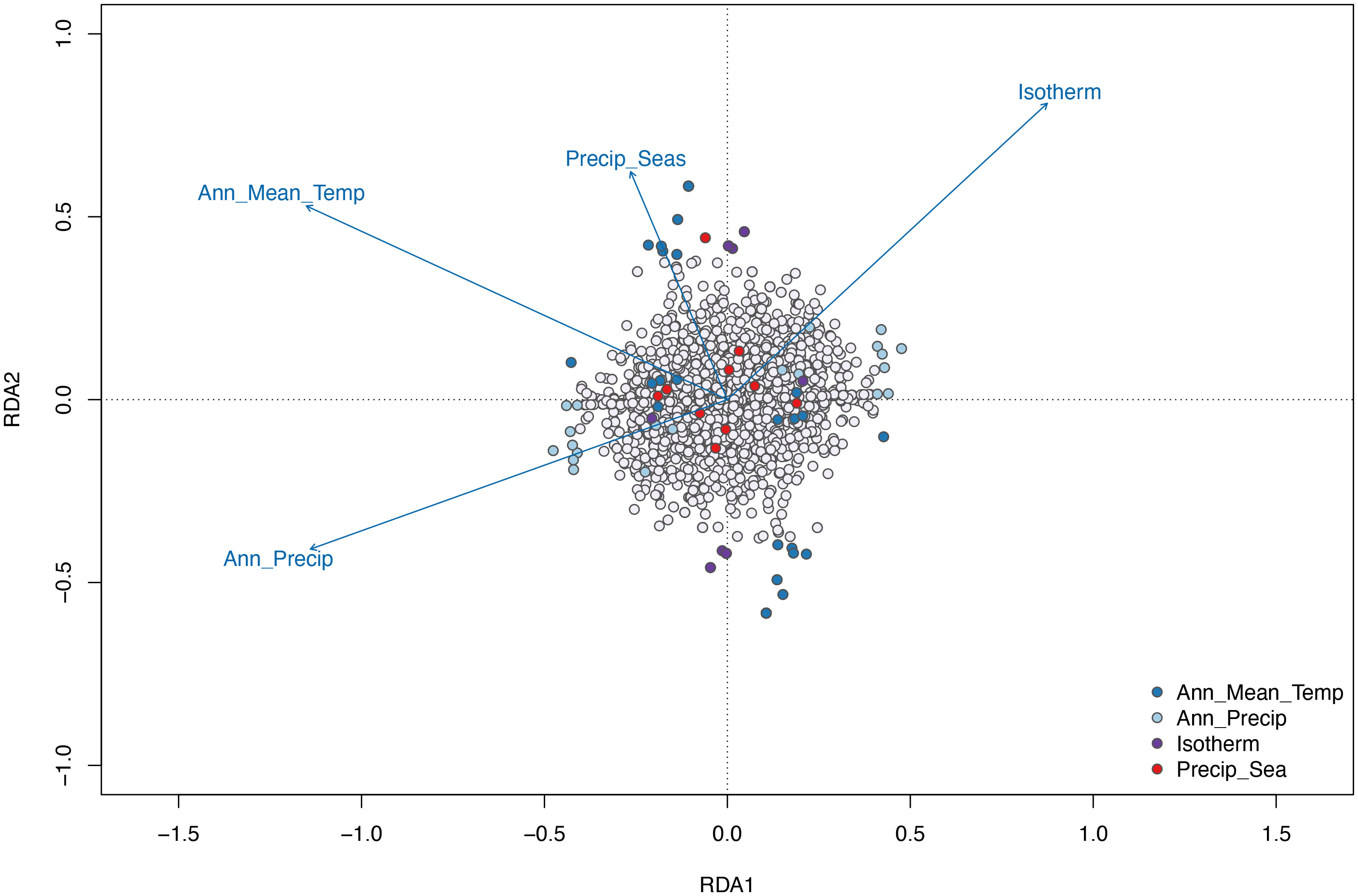
Figure 5 RDA Triplot of specimens, SNPs (small light grey points), and variables (vectors) on axes 1 and 2, by environmental factors.
About 8% of the sampled SNPs are associated with environmental variation. There are SNPs heavily associated with all four variables, revealing that the estimated genotypes are correlated with local temperature and precipitation. Among the 230 candidate SNPs detected, the majority were correlated with temperature variables: 170 SNPs correlated with annual mean temperature (BIO1) and 27 SNPs correlated with isothermality (BIO3). The remaining SNPs were associated with precipitation: 23 SNPs associated with annual precipitation (BIO12) and 10 with precipitation seasonality (BIO15). The Serra da Mesa population is clustered in the right quadrant showing a strong positive correlation with temperature (isothermality), whereas the Minaçu population is clustered in the left quadrant, presenting a positive correlation with both annual mean temperature and annual precipitation. Although clustered together with Minaçu population in the DAPC, the single individual from Colinas do Sul appears isolated. The distance from the other two populations demonstrates a magnitude of divergence and a strong correlation with the environmental predictors as well (Figure 6).
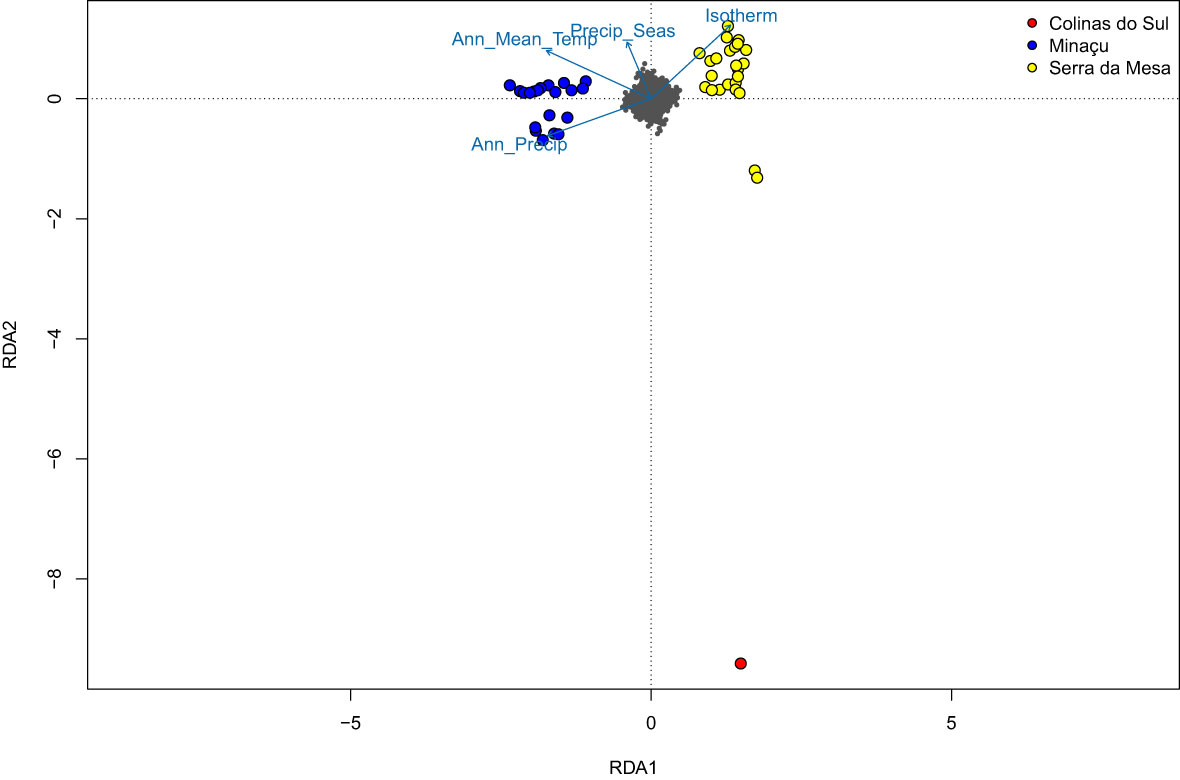
Figure 6 RDA Triplot of specimens, SNPs (small grey points), and variables (vectors) on axes 1 and 2, by site.
4 Discussion
Cases of heritable phenotypic differentiation between local populations have always been a subject that attracts the attention of evolutionary biologists and naturalists (Kawecki and Ebert, 2004). Local adaptation plays a crucial role in initiating the divergence of incipient species (Kawecki and Ebert, 2004). Several examples of adaptation have been reported, such as the genomic mapping of the color polymorphism in the peppered moth Biston betularia, coat color variation in different mouse species in response to the variation of the environment background color, and trichome variation to improve herbivory resistance in Arabidopsis lyrate (Savolainen et al., 2013). Recent studies with Anolis lizards also reported rapid directional changes, where after experiencing extreme cold temperatures, they presented a greater resistance to the cold due to a shift in gene expression (Campbell-Staton et al., 2017). Another interesting case involving Anolis describes their improved capacity of surviving hurricanes in the Caribbean region because of their larger toepads (Dufour et al., 2019). All these examples show visibly rapid local adaptation on human timescales, often in response to human pressures.
Recently published studies on local adaptation by genotype–environment associations (GEA) were focused on birds (Bay et al., 2018; Hofmeister et al., 2021), wolves (Schweizer et al., 2016), and plants (Vangestel et al., 2018). All these papers investigated genomic variations of metapopulations across the range of distribution of different species. Although it is not possible to claim that human interference was responsible for the genetic differentiation observed, we present herein results with anthropogenically isolated organisms, where gene flow was reduced, and rapid genetic morphological and ecological differentiation has previously been detected (Amorim et al., 2017).
Admixture, DAPC, and Fst detected the presence of distinct genetic clusters of G. amarali in Serra da Mesa, which is differentiated from nearby “mainland” populations (i.e., Colinas do Sul and Minaçu). Our results showed separation among at least two subpopulations: Serra da Mesa and Minaçu, according to Admixture, or three subpopulations, recovering Colinas do Sul as separate from Serra da Mesa and Minaçu. This fine-scale population structure was not detected in previous range-wide phylogeographic analyses of the species (see Domingos et al., 2014). We found potential evidence of genotype association with environmental conditions for all subpopulations. RDA pointed out possible local genetic adaptations correlated with temperature and precipitation, suggesting that lizards might adapt to the climate at each site, highlighting the effect of Cerrado seasonality on lizard populations (Françoso et al., 2020). Previous studies with G. amarali in Serra da Mesa reported head size increase (Amorim et al., 2017) and negative allometry in the hemipenis lobe in the “island” populations (Coelho, 2020). Future goals of this project include sequencing whole-genome data of these populations and testing genome-wide associations with the environment in a more robust framework.
Adaptive phenotypic plasticity may lead to adaptive phenotypic differentiation, even without genetic divergence (Kawecki and Ebert, 2004). Thus, although the geckos living on the islands and the ones from the margins were clustered together, ecological release detected on the islands was hypothesized to result from the local competition of large body-size competitors yielding the reported morphological differences (Amorim et al., 2017). This was not reflected in our genetic analyses, which suggested a cluster of locally restricted genotypes at Serra da Mesa, including sampling sites on the “islands” and across the reservoir margins as part of the same subpopulation. It is possible that ongoing gene flow to and from the islands overrides any hyper-local adaptations. Additional geographic sampling is needed to robustly test how widespread the signal of presumable locally adapted genotypes at Serra da Mesa occurring elsewhere other than Minaçu as shown in Admixture, but not DAPC, and how frequently and geographically distributed such locally climate-adapted population structure occurs.
Although the emergence of the local adaptation concept is relatively well-studied in evolutionary biology, there is still much room for further investigation. Finding the SNPs associated with Gene Ontology categories, for instance, the morphological categories enriched by temperature and precipitation would be a desirable next step (e.g., Schweizer et al., 2016). We provide here another piece for the puzzle, complementing de Amorim et al. (2017) findings, aiming at a deeper comprehension of what is underlying genetic consequences of the rapid phenotypic evolution of G. amarali in the Serra da Mesa reservoir.
In summary, we show that both island and reservoir margin populations in Serra da Mesa are genetically distinct from nearby “mainland” sites such as Colinas do Sul and Minaçu. We identified 230 candidate loci associated with the environment and at least two locally structured subpopulations (Serra da Mesa and Minaçu) show significant association with environmental variation. Our results add valuable information about ongoing evolutionary processes to the previous reports accounting for severe ecological and morphological changes of this gecko (in preparation; Amorim et al., 2017; Coelho, 2020). Further study is now needed to untangle the genomic basis and developmental causes of short- and long-term genetic and phenotypic variation in these unique populations.
Data availability statement
The data presented in the study are deposited in the NCBI BioProject repository, accession number PRJNA761098.
Ethics statement
Ethical review and approval was not required for the animal study because the study follows the rules of the CONCEA and CRBio for studies involving animals. The material collected for this study is under the SISBIO license number 28190-2.
Author contributions
All authors listed have made a substantial, direct, and intellectual contribution to the work and approved it for publication.
Funding
This work was funded in part by PDSE (CAPES) and PPG-Zoo to RM, Centro UnB-Cerrado to RB and RM, and the CCAS, OVPR, and Pegasus HPC at George Washington University and U.S. National Science Foundation [grant #DEB-1655737] to RP. CNPq [productivity grant #306644/2020-7] to RB. RP was supported by US NSF grant DEB-1441719, and JT acknowledges support from NSF DEB-1949749 to Rafael de Sa.
Acknowledgments
We thank Pedro De Podestà Uchôa de Aquino (CHUNB) for tissue loans. We thank Josué Azevedo (INPA), Brenna Forester (Colorado State University) and Dean Urban (Duke University) for their valuable insights and suggestions on the RDA analysis and on the manuscript, as well. We also thank Maria Eduarda Coelho for the help with the map. The authors thank the University of Wisconsin Biotechnology Center DNA Sequencing Facility for providing GBS and Illumina facilities and services.
Conflict of interest
The authors declare that the research was conducted in the absence of any commercial or financial relationships that could be construed as a potential conflict of interest.
Publisher’s note
All claims expressed in this article are solely those of the authors and do not necessarily represent those of their affiliated organizations, or those of the publisher, the editors and the reviewers. Any product that may be evaluated in this article, or claim that may be made by its manufacturer, is not guaranteed or endorsed by the publisher.
Supplementary material
The Supplementary Material for this article can be found online at: https://www.frontiersin.org/articles/10.3389/fevo.2023.980777/full#supplementary-material
References
Albert J. S., Carnaval A. C., Flantua S. G., Lohmann L. G., Ribas C. C., Riff D., et al. (2023). Human impacts outpace natural processes in the Amazon. Science 379 (6630), eabo5003. doi: 10.1126/science.abo5003
Alexander D. H., Novembre J., Lange K. (2009). Fast model-based estimation of ancestry in unrelated individuals. Genome Res. 19 (9), 1655–1664. doi: 10.1101/gr.094052.109
Amorim M., Schoener T. W., Santoro G. R. C. C., Lins A. C. R., Piovia-Scott J., Brandão R. A. (2017). Lizards on newly created islands independently and rapidly adapt in morphology and diet. Proc. Natl. Acad. Sci. USA 114, 8812–8816. doi: 10.1073/pnas.1709080114
Azevedo J. A. R., Valdujo P. H., de C. Nogueira C. (2016). Biogeography of anurans and squamates in the Cerrado hotspot: coincident endemism patterns in the richest and most impacted savanna on the globe. J. Biogeogr. 43, 2454–2464. doi: 10.1111/jbi.12803
Bay R., Harrigan R., Le Underwood V., Gibbs H. L., Smith T., Ruegg K. (2018). Comment on “Genomic signals of selection predict climate-driven population declines in a migratory bird”. Science 80, 83–86. doi: 10.1126/science.aat7279
Campbell-Staton S. C., Cheviron Z. A., Rochette N., Catchen J., Losos J. B., Edwards S. V. (2017). Winter storms drive rapid phenotypic, regulatory, and genomic shifts in the green anole lizard. Science 80, 495–498. doi: 10.1126/science.aam5512
Capblancq T., Luu K., Blum M. G. B., Bazin E. (2018). Evaluation of redundancy analysis to identify signatures of local adaptation. Mol. Ecol. Resour. 18, 1223–1233. doi: 10.1111/1755-0998.12906
Coelho W. (2020). Variações morfológicas nos hemipenes de Gymnodactylus Spix 1825 (Squamata, Phyllodactylidae (Brasília (DF: Universidade de Brasília). dissertation/master's thesis.
Colli G. R., Bastos R. P., Araujo A. F. B., Oliveira P. S., Marquis R. J. (2002). “The character and dynamics of the Cerrado herpetofauna,” in The Cerrados of BRAZIL: Ecology and Natural History of a Neotropical Savanna (New York: Columbia Univ. Press), 223–241. doi: 10.7312/oliv12042
Colli G. R., Mesquita D. O., Rodrigues P. V. V., Kitayama K. (2003). Ecology of the Gecko Gymnodactylus geckoides amarali in a Neotropical Savanna. J. Herpetol. 37, 694–706. doi: 10.1670/180-02A
Domingos F. M. C. B., Bosque R. J., Cassimiro J., Colli G. R., Rodrigues M. T., Santos M. G., et al. (2014). Out of the deep: Cryptic speciation in a Neotropical gecko (Squamata, Phyllodactylidae) revealed by species delimitation methods. Mol. Phylogenet. Evol. 80, 113–124. doi: 10.1016/j.ympev.2014.07.022
Dormann C. F., Elith J., Bacher S., Buchmann C., Carl G., Carré G., et al. (2013). Collinearity: A review of methods to deal with it and a simulation study evaluating their performance. Ecography (Cop.). 36, 27–46. doi: 10.1111/j.1600-0587.2012.07348.x
Dufour C. M. S., Donihue C. M., Losos J. B., Herrel A. (2019). Parallel increases in grip strength in two species of Anolis lizards after a major hurricane on Dominica. J. Zool. 309, 77–83. doi: 10.1111/jzo.12685
Eaton D. A. R., Overcast I. (2020). Ipyrad: Interactive assembly and analysis of RADseq datasets. Bioinformatics 36, 2592–2594. doi: 10.1093/bioinformatics/btz966
Elshire R. J., Glaubitz J. C., Sun Q., Poland J. A., Kawamoto K. (2011). A robust, simple Genotyping-by-Sequencing (GBS) approach for high diversity species. PloS One 6, 19379. doi: 10.1371/journal.pone.0019379
Fick S. E., Hijmans R. J. (2017). WorldClim 2: new 1-km spatial resolution climate surfaces for global land areas. Int. J. Climatol. 37, 4302–4315. doi: 10.1002/joc.5086
Forester B. R., Jones M. R., Joost S., Landguth E. L., Lasky J. R. (2016). Detecting spatial genetic signatures of local adaptation in heterogeneous landscapes. Mol. Ecol. 25, 104–120. doi: 10.1111/mec.13476
Forester B. R., Lasky J. R., Wagner H. H., Urban D. L. (2018). Comparing methods for detecting multilocus adaptation with multivariate genotype–environment associations. Mol. Ecol. 27, 2215–2233. doi: 10.1111/mec.14584
Françoso R. D., Dexter K. G., Machado R. B., Pennington R. T., Pinto J. R. R., Brandão R. A., et al. (2020). Delimiting floristic biogeographic districts in the Cerrado and assessing their conservation status. Biodivers. Conserv. 29, 1477–1500. doi: 10.1007/s10531-019-01819-3
Hijmans R. J., van Etten J. (2012). raster: geographic analysis and modeling with raster data. R package version 2.0-12. Available at: http://cran.r-project.org/web/packages/raster/index.html.
Hofmeister N. R., Werner S. J., Lovette I. J. (2019). Environment but not geography explains genetic variation in the invasive and largely panmictic European starling in North America. bioRxiv. doi: 10.1101/643858
Hofmeister N. R., Werner S. J., Lovette I. J. (2021). Environmental correlates of genetic variation in the invasive European starling in North America. Mol. Ecol. 30, 1251–1263. doi: 10.1111/mec.15806
Hu C. C., Wu Y. Q., Ma L., Chen Y. J., Ji X. (2019). Genetic and morphological divergence among three closely related Phrynocephalus species (Agamidae). BMC Evol. Biol. 19 (1), 1–15. doi: 10.1186/s12862-019-1443-y
Jombart T., Collins C. (2015). A tutorial for tDiscriminant Analysis of Principal Components (DAPC) using adegenet 2.0.0.
Jombart T., Devillard S., Balloux F. (2010). Discriminant analysis of principal components: A new method for the analysis of genetically structured populations. BMC Genet. 11, 94. doi: 10.1186/1471-2156-11-94
Kawecki T. J., Ebert D. (2004). Conceptual issues in local adaptation. Ecol. Lett. 7, 1225–1241. doi: 10.1111/j.1461-0248.2004.00684.x
MacLachlan G., Hurlburt J., Suarez M., Burke W., Lewis T., Gallo A., et al. (2020). Building a Shared Resource HPC Center Across University Schools and Institutes: A Case Study (ArXiv).
Nogueira C., Colli G. R., Costa G., Machado R. B. (2010). “Diversidade de répteis Squamata e evolução do conhecimento faunístico no Cerrado,” in Cerrado Conhecimento Científico Quantitativo Como Subsídio Para Ações de Conservação. Eds. Diniz I. R., Marinho-Filho J.(UnB, Brasília), 331–371.
Oksanen J., Kindt R., Lagendre P., O’Hara B., Simpson G., Solymos P., et al. (2008). “The vegan package,” in Community Ecology Package, 1–190.
Pyron R. A., Costa G. C., Patten M. A., Burbrink F. T. (2015). Phylogenetic niche conservatism and the evolutionary basis of ecological speciation. Biol. Rev. 90 (4), 1248–1262. doi: 10.1111/brv.12154
Rellstab C., Gugerli F., Eckert A. J., Hancock A. M., Holderegger R. (2015). A practical guide to environmental association analysis in landscape genomics. Mol. Ecol. 24, 4348–4370. doi: 10.1111/mec.13322
Savolainen O., Lascoux M., Merilä J. (2013). Ecological genomics of local adaptation. Nat. Rev. | Genet. 14, 807. doi: 10.1038/nrg3522
Scheffers B. R., De Meester L., Bridge T. C. L., Hoffmann A. A., Pandolfi J. M., Corlett R. T., et al. (2016). The broad footprint of climate change from genes to biomes to people. Science 354. doi: 10.1126/science.aaf7671
Schweizer R. M., VonHoldt B. M., Harrigan R., Knowles J. C., Musiani M., Coltman D., et al. (2016). Genetic subdivision and candidate genes under selection in North American grey wolves. Mol. Ecol. 25, 380–402. doi: 10.1111/mec.13364
Vangestel C., Eckert A. J., Wegrzyn J. L., St. Clair J. B., Neale D. B. (2018). Linking phenotype, genotype and environment to unravel genetic components underlying cold hardiness in coastal Douglas-fir (Pseudotsuga menziesii var. menziesii). Tree Genet. Genomes 14, 1–14. doi: 10.1007/s11295-017-1225-x
Vitt L. J., Shepard D. B., Caldwell J. P., Vieira G. H. C., França F. G. R., Colli G. R. (2007). Living with your food: Geckos in termitaria of Cantão. J. Zool. 272, 321–328. doi: 10.1111/j.1469-7998.2006.00273.x
Keywords: redundancy analysis, Central Brazil, reservoir, habitat isolation, local adaptation
Citation: Miranda RB, Brandão RA, O’Connell K, Colli GR, Tonini JFR and Pyron RA (2023) Multilocus environmental adaptation and population structure in the Cerrado gecko Gymnodactylus amarali (Sauria, Phyllodactylidae) from Serra da Mesa Hydroelectric Plant, Central Brazil. Front. Ecol. Evol. 11:980777. doi: 10.3389/fevo.2023.980777
Received: 28 June 2022; Accepted: 21 July 2023;
Published: 16 August 2023.
Edited by:
Hernani Oliveira, Federal University of Paraná, BrazilReviewed by:
Ella Vázquez-Domínguez, National Autonomous University of Mexico, MexicoCarolina Blefari Batista, Centro Universitário de Goiatuba (UniCerrado), Brazil
Copyright © 2023 Miranda, Brandão, O’Connell, Colli, Tonini and Pyron. This is an open-access article distributed under the terms of the Creative Commons Attribution License (CC BY). The use, distribution or reproduction in other forums is permitted, provided the original author(s) and the copyright owner(s) are credited and that the original publication in this journal is cited, in accordance with accepted academic practice. No use, distribution or reproduction is permitted which does not comply with these terms.
*Correspondence: Rogério Benevides Miranda, rogeriobenevides@gmail.com