- 1Program in Ecology, Evolution and Conservation Biology, Department of Geography, University of Nevada, Reno, NV, United States
- 2U.S. Geological Survey, Western Ecological Research Center, Boulder City, NV, United States
- 3U.S. Geological Survey, Western Ecological Research Center, San Diego, CA, United States
- 4U.S. Fish and Wildlife Service, Desert Tortoise Recovery Office, Las Vegas, NV, United States
Introduction: Animal movements are influenced by landscape features; disturbances to the landscape can alter movements, dispersal, and ultimately connectivity among populations. Faster or longer movements adjacent to a localized disturbance or within disturbed areas could indicate reduced habitat quality whereas slower or shorter movements and reduced movements may indicate greater availability of resources. The Mojave desert tortoise (Gopherus agassizii) is a threatened species that is challenged by anthropogenic disturbances.
Methods: We studied tortoise movements using Global Positioning System (GPS) loggers at multiple sites in the Mojave Desert of Nevada and California. Tortoises at our sites encountered localized, linear human infrastructure, including paved roads, dirt roads, and fences, as well as landscape-scale disturbances [wildfire, off highway vehicle use (OHV), livestock grazing area]. We fit two-state (moving and encamped) Hidden Markov models to GPS logger data to infer how tortoise movement behavior relates to anthropogenic and natural features.
Results: We found that temporal covariates, individual-level random effects (intercepts), and sex best explained state transition probability in all sites. We compared relationships between tortoise movement and linear disturbances, which varied depending on site and context. Tortoises made longer movements within the OHV recreation area, near most dirt roads, and near a low-traffic paved road, indicating that tortoises avoid these habitat disturbances. Conversely, tortoises made shorter movements in areas of higher slope and near highways, suggesting that these features may restrict movement or provide resources that result in prolonged use (e.g., forage or drinking locations). Tortoises that encountered fences around utility-scale solar installations were more active and made longer movements near fences, indicative of pacing behavior.
Discussion: These results provide insight into how different disturbances alter tortoise movement behavior and modify tortoise habitat use, providing information that can be used to manage tortoise habitat.
1. Introduction
Animal movement is a key component of behavioral and ecological processes such as foraging, social interactions, and dispersal (Hooten et al., 2017). Describing changes in movement behavior in response to environmental conditions can provide insight into the impacts of habitat disturbance for a given species. Technological advances in tracking devices provide detailed insight into animal movements, enabling a deeper understanding of how individual behaviors shape the ecology and conservation of species (Kays et al., 2015). The use of tracking devices provides the opportunity to evaluate conservation efforts such as translocation, delineation of movement corridors, as well the effects of anthropogenic disturbances on movement patterns (e.g., Zeller et al., 2012; Nickel et al., 2021; Picardi et al., 2022). Wildlife habitat continues to be transformed by anthropogenic disturbance and understanding the influence on animal movement can inform management strategies to ensure the persistence of at-risk populations. This can be especially true for wide ranging species that coexist with different disturbances in different contexts, where understanding how context interacts with a disturbance is important to developing management strategies.
The Mojave desert tortoise (Gopherus agassizii) is a medium-sized (generally < 380 mm midline carapace length), terrestrial tortoise species native to deserts of the southwestern United States (Berry and Murph, 2019). The species is listed as threatened under the United States Endangered Species Act, as critically endangered by the International Union for Conservation of Nature, and has continued to decline since listing (U. S. Fish and Wildlife Service, 2011; Allison and McLuckie, 2018; Vaughn et al., 2020). Tortoise habitat has been altered by various anthropogenic disturbances, which range from local to landscape scales. Understanding how these disturbances alter the quality of tortoise habitat and restrict population connectivity are key recovery actions outlined in the recovery plan (U. S. Fish and Wildlife Service, 2011). Disturbance is generally thought to decrease habitat quality, but evidence used to infer how disturbance alters habitat quality and use is primarily limited to abundance estimates or counts of tortoise sign (Boarman and Sazaki, 2006; Tuma et al., 2016; Berry et al., 2020a,c), and not correlates of fitness or mechanisms that may be driving population declines. Prior research has indicated that anthropogenic disturbances alter tortoise movement, especially at local scales (Peaden et al., 2017; Gray et al., 2019; Hromada et al., 2020), but has left managers with a limited understanding of how both localized and landscape-scale disturbances may alter individual movement behavior. Describing the behavioral variation associated with disturbances can improve effective mitigation practices to protect populations from anthropogenic uses.
Transportation infrastructure, such as roads and railroads, is commonly located within tortoise habitat and can be a source of tortoise mortality as well as a barrier to tortoise movement (Boarman and Sazaki, 2006; Nafus et al., 2013; Rautsaw et al., 2018). Many areas contain networks of dirt roads, which can cause soil compaction and erosion, alter the physiology of native plants, allow for the incursion of predators, and restrict tortoise movement (Sharifi et al., 1997; Brooks and Lair, 2005; Gray et al., 2019; Hromada et al., 2020). Paved roads may attract tortoises by providing areas where water pools for drinking (Medica et al., 1980), and increased runoff can increase plant production in the surrounding margins (Lightfoot and Whitford, 1991). However, paved roads pose a higher mortality risk for tortoises and result in locally reduced tortoise densities (von Seckendorff Hoff and Marlow, 2002; Boarman and Sazaki, 2006; Nafus et al., 2013). To mitigate for tortoise mortality, many of the highways in tortoise habitat have been fenced to exclude tortoises. However, these fences can have unintended consequences, as tortoises will often pace alongside them attempting to access formerly used habitats and can consequently experience potentially detrimental thermal environments (Peaden et al., 2017; Nowakowski et al., 2020).
Other disturbances in remaining desert tortoise habitat include wildfire, grazing and off-highway vehicle (OHV) use. Wildfire, promoted by the spread of non-native Mediterranean grasses, has burned large areas of the desert southwest, often replacing perennial shrubland communities with annual grasslands (Esque et al., 2010; Brooks, 2012). Desert tortoises continue to move within these converted, post-fire landscapes, though loss of shrub cover may reduce thermoregulatory opportunities and consumption of non-native grasses may degrade tortoise health (Drake et al., 2015, 2016; Snyder et al., 2019). Sheep and cattle grazing continue to be important uses of public lands in the Mojave Desert, altering the vegetation community, increasing competition for forage, and damaging soils (Webb and Stielstra, 1979; Nicholson and Humphreys, 1980; Medica et al., 1982; Abella et al., 2019). Off-highway vehicle recreation has proliferated on public lands in the desert southwest, further spreading invasive plant species, and causing soil compaction/erosion, vegetation damage, and disturbance/mortality of animals (Ouren et al., 2007; Switalski, 2018). Prior research suggests that desert tortoise density is reduced or eliminated in areas of high OHV use, and that the closing of areas to OHV use benefits tortoise populations (Bury and Luckenbach, 2002; Custer et al., 2017; Berry et al., 2020b).
In this paper, we examine fine-scale tortoise movement data and relate movement behavior to environmental features. We studied tortoises that live alongside anthropogenic disturbances as well as in different habitat contexts (e.g., mountainous versus flat sandy terrain). Using a generalized Hidden Markov Model (HMM) framework, we demonstrate how disturbances alter both movement state and movement step length parameters. We expected to find strong relationships between tortoise movement behavior and natural features as well as anthropogenic disturbances.
Specifically, we hypothesized the following relationships between the environmental/disturbance covariates and tortoise movement behavior:
• Tortoises are more likely to be moving during the daylight hours and during spring, as this reflects their diurnal habits and known seasonal activity patterns.
• Male tortoises are more likely to be moving and make longer steps than female tortoises. This is expected due to known behavioral differences between tortoise sexes relative to space use and activity.
• When tortoises are near roads, they will be less likely to be moving, and if moving, will make longer step lengths when closer to roads. We expect this response as tortoises select for movement away from roads potentially due to low vegetative cover in roads.
• When tortoises are near linear barriers (fences and railways), they will be more likely to be moving state and will also make longer steps than when away from such features. We anticipate this as a reflection of pacing behavior that tortoises often exhibit alongside barriers.
• When tortoises are moving through landscape-scale disturbances believed to degrade tortoise habitat (open OHV activity, grazing, wildfire), they will be more likely to be moving and make longer steps than in areas without these large-scale disturbances. We expect this due to the reduction of available resources and cover via degradation of tortoise habitat.
• When tortoises are in areas that have natural features that restrict movement and/or provide uncommon resources (areas of high slope and shrub cover), they will be more likely to be not moving and make shorter steps while moving than in areas that do not have these features.
2. Methods
2.1. Study site descriptions
We studied movements of adult tortoises in seven different locations throughout the Mojave Desert of Nevada and California (Figure 1) between 2011 and 2021. Sites primarily consisted of Mojave desert scrub dominated by creosote-bursage (Larrea tridentata-Ambrosia dumosa) associations, and other important shrub components (e.g., Yucca spp., Psorothamnus spp., Cylindropuntia spp.; Turner, 1994). Sites varied topographically from rugged mountain terrain in McCullough Pass, NV (max slope 38°) to flat sandy terrain at the Nipton site.
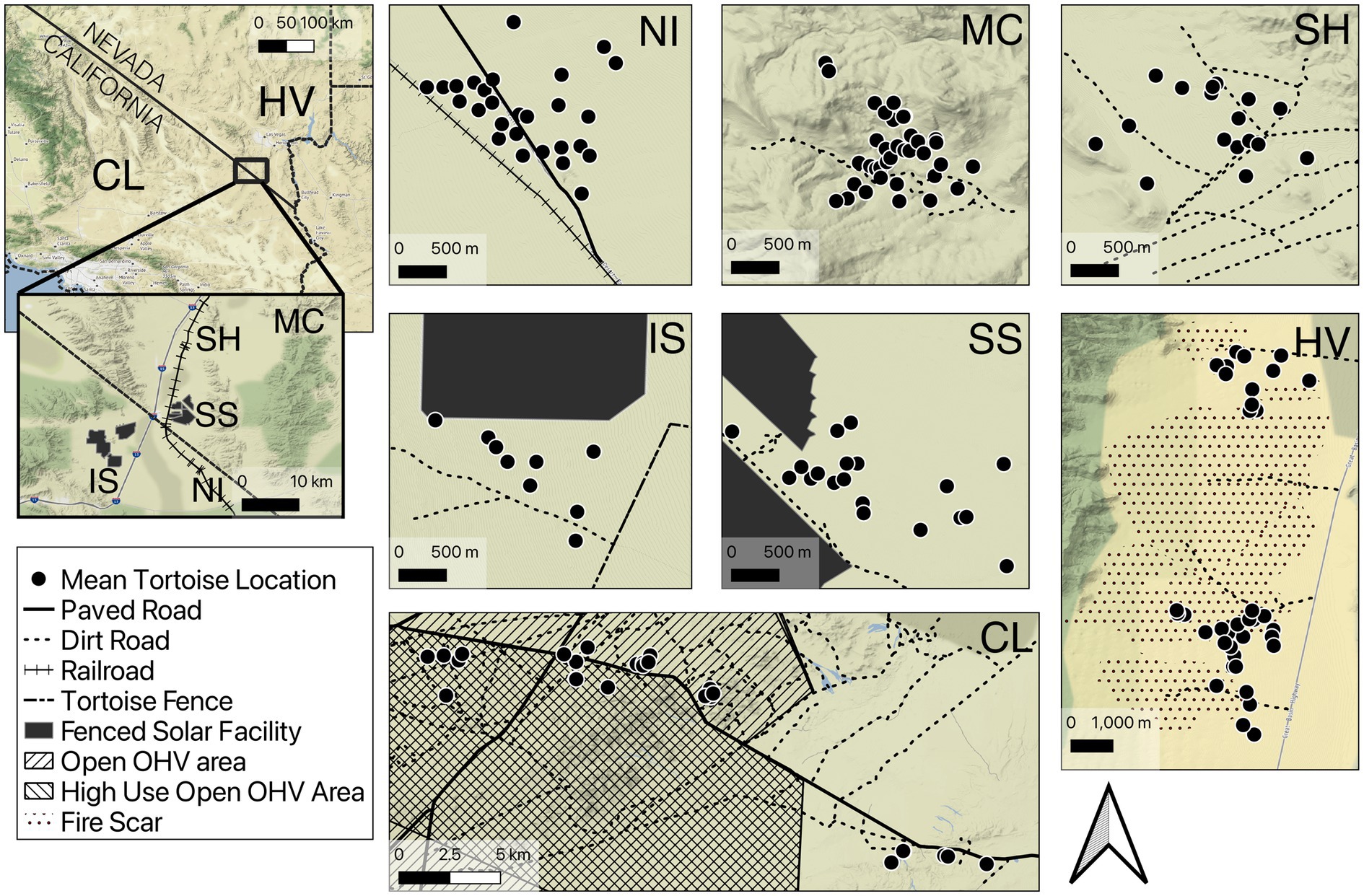
Figure 1. Overview of site locations and features within sites used to study tortoise movements in the Mojave Desert, CA and NV. Black dots represent the mean location for each tortoise with GPS logger data. Site codes: Nipton (NI), McCullough (MC), Sheep Mountain (SH), ISEGS South (IS), Silver State (SS), China Lake (CL), Hidden Valley (HV). Disturbance features are indicated in the legend. The edges of solar installations are fenced.
Collectively the sites were impacted by an array of disturbances including roads, railroads, solar development, OHV use, and recent wildfire (Table 1). Most sites had dirt roads used by recreational OHVs as well as power line maintenance traffic. The Silver State, NV and ISEGS South, CA sites had tortoise exclusion fencing surrounding utility-scale solar plants that were installed prior to data collection (2014 and 2011; respectively). The Nipton, CA site had a low-traffic volume paved road with no posted speed limit that served as access for an active railroad maintenance site that also intersected the study site. The China Lake, CA site had two unfenced, fairly high-traffic highways (speed limit = 88.5 km/h) that resulted in several tortoise mortalities in the years prior to and during our study (J. Hendrix, Naval Air Weapons Center—China Lake—pers. comm.). Portions of the surrounding public lands were designated for unrestricted “open” travel of OHVs; two areas were designated as seasonal grazing allotments for sheep in years of good forage production (Spangler Hills and Cantil Common; grazed 2019 and 2020 during our study period). The eastern portion of the site was within the off-limits boundaries of the China Lake Naval Weapons Station, which does not allow public access. The remaining areas in the China Lake site and all other sites were located on land managed by the United States Bureau of Land Management (BLM). The Hidden Valley, NV site experienced lightning caused wildfires during 2005 which led to large areas being burned (Drake et al., 2015). Site characteristics are summarized (Table 1) and maps of sites are provided (Figure 1).
Tortoises were found during surveys of one-km2 plots at Nipton, McCullough Pass, Sheep Mountain, ISEGS South, and Silver State as part of another study (Mitchell et al., 2021). Surveys were also conducted in the area within 1 km of Randsburg Wash Road (China Lake) and on 400 m2 vegetation study plots in Hidden Valley (Drake et al., 2015).
2.2. Tortoise telemetry protocol
All tortoises involved in the study were outfitted with Very High Frequency (VHF; Model RI-2B; Holohil Systems Ltd.) radio-transmitters. Tortoises at all sites except Hidden Valley were equipped with GPS loggers (i-gotU GT-120; Mobile Action Technology). Tortoises at these sites were physically relocated once per month using VHF telemetry equipment. During these visits the GPS loggers were swapped for battery recharge and data downloading. Tortoises at the Hidden Valley site were outfitted with custom-made Advanced Telemetry Systems GPS/VHF combination loggers. Tortoises at the Hidden Valley site were relocated at approximately one-week intervals, and GPS loggers were replaced approximately every 3 months for data downloading and charging. All tortoises were greater than 200 mm midline carapace length; tortoises smaller than this size could not carry both the radio and logger without excessively modifying the vertical profile of the tortoise. Most GPS loggers were programmed to collect a point every hour, but some of the Hidden Valley and McCullough Pass data were collected every half hour during initial deployment trials and later filtered to provide an hourly dataset. The number of tortoises monitored, and GPS locations recorded per site are provided in Table 1. Thirteen of the twenty tortoises at the Silver State site were translocated from the footprint of the nearby utility-scale solar installation in 2014, and one of the nine tortoises at the ISEGS South site was translocated from the nearby utility-scale solar installation in 2011. Tortoises were translocated to areas just outside the footprint of the utility-scale solar installations. All but one of the tortoises that interacted with the fences were translocated. Therefore, we were not able to separate movement behavior differences due to the fences and translocation; translocation is known to alter movement behavior in tortoises (Nussear et al., 2012). All tortoises were handled in accordance with a USFWS Permit (permit TE-030659-10), Nevada Department of Wildlife Scientific Collection Permit 317351, University of Nevada, Reno Animal Care and Use Committee protocol (IACUP 00671), and a Memorandum of Understanding with the California Department of Fish and Wildlife (all to T. Esque).
2.3. Environmental predictors
We chose environmental predictors that we hypothesized were important to tortoise movement behavior and used them as covariates in our model construction. We used the TIGER road datasets for dirt and paved roads in our study areas and modified them to better match satellite imagery (United States Census Bureau, 2021). We log-transformed distance to linear features (roads, railroad, and fences) to reflect the spatial decay around the impact of these features as we expected the effect of these covariates to be localized near the disturbance. We used the National Land Cover Dataset (NLCD) shrub coverage layer to determine if differences in shrub cover may act as a cue for tortoises to recognize disturbed areas (Homer and Fry, 2012; Rigge et al., 2021). To represent areas burned in wildfire, we digitized a polygon that corresponded with the burned areas seen on Google satellite imagery as available layers from the BLM did not accurately reflect the wildfire boundaries visible on satellite imagery and from data collected while tracking tortoises (Drake et al., 2015). Additionally, we considered slope as it has been shown to alter tortoise movement (Hromada et al., 2020); slope was derived from the USGS Digital Elevation Model using the terrain function in package raster (U.S. Geological Survey, 2017; Hijmans et al., 2022).
Open OHV areas on BLM lands typically have both established routes designated by the BLM and user-created routes that are often missing from BLM road and trail inventories. BLM designated open routes are intended for travel by street legal vehicles, while user-created routes are tracks created by OHVs driving outside of established routes. We found that established routes in the Spangler Hills OHV Area at the China Lake site were best represented by data sourced from Owlshead GPS, a service intended for OHV recreationists (Friends of Jawbone, 2022). We also used a polygon from the same source to represent the entire open OHV area. However, we found that although the entire area is designated for off-trail OHV use, certain areas within the boundaries used by our tortoises saw little to no off-trail use, likely due to their greater distance from major staging areas, or rougher terrain. These included the areas north of Randsburg Wash Road and areas that were rocky hills with large boulders. We created another polygon that excluded these areas and tested both as categorical predictors in our modeling efforts. Sheep grazing occurred during years of higher productivity across the area contained within the entire OHV polygon; thus, the two polygons represent a combination of grazing pressure and relatively high OHV use versus mostly just grazing pressure with relatively low OHV use.
2.4. Discrete-time hidden Markov models
Hidden Markov models (HMMs) are a popular approach for analyzing temporally regular animal location data for inference on movement behavior (McClintock et al., 2012; McClintock and Michelot, 2018). HMMs are a form of state-space models that assume that observed data arise from a number of “hidden” (i.e., latent) states. In the case of telemetry data these are movement behavior states that can represent activities such as exploration, foraging or rest (Patterson et al., 2009). The state changes can be identified from differences in the distribution of the step lengths and turning angles of the relocation dataset (Morales et al., 2004; McClintock et al., 2012). Many HMMs fit to animal movement data can only identify two biologically meaningful states; one that corresponds to a moving state and one that corresponds to a resting or encamped state (Beyer et al., 2013; McClintock et al., 2014). An extension of the HMM framework (known as a generalized HMM) allows for inference of changes in behavioral states and movement parameters using environmental and individual level covariates (McClintock et al., 2017; Carter et al., 2020).
We fit generalized discrete-time hidden Markov movement models (HMMs) to tortoise GPS location data using the package momentuHMM (version 1.5.4) in program R (version 4.0.4; McClintock and Michelot, 2018; R Core Team, 2018). Hidden Markov movement models require bursts of continuous GPS data; however, tortoise behavior (i.e., burrowing and cave dwelling) often results in missed fixes. As we are primarily interested in above-ground activity in this study, we were not concerned about missed fixes during burrow use. We partitioned our GPS telemetry data first to contain temporally continuous segments that had no more than 2 h of missing data and greater than 36 consecutive hours. To filter out inaccurate fixes from the GPS loggers, we used the uere.fit function from package ctmm to estimate error for the GPS loggers based on locations taken with stationary loggers placed at study sites (Calabrese et al., 2016). We placed GPS loggers within unoccupied tortoise burrows to determine how this positioning would alter fix accuracy. For the custom made ATS GPS loggers (used in the Hidden Valley site), we fit an error model using the HDOP (Horizontal Dilution of Precision) data column as a predictor of horizontal error to the stationary logger data. For the i-gotU GPS loggers, we fit an error model without using the EHPE (Expected Horizontal Position Error) column as much of the data were retrieved from the loggers without the column. We filtered extreme outlier points for the i-gotU GPS loggers by removing points from both the stationary logger and tortoise telemetry datasets that had greater than 25 m of elevation error recorded altitude—elevation from a digital elevation model (Laver et al., 2015), and then fit an error model on the stationary logger data. We then used the outlie function in ctmm to detect and remove telemetry points that had a movement rate greater than that expected for a tortoise (300 m/h; Nussear and Esque, unpublished data) after accounting for locational error.
As HMMs assume exact temporal consistency, GPS data had to be imputed by fitting a continuous-time correlated random walk to the data using the crawlWrap function from the momentuHMM package as a wrapper around the crwlMLE function from package crwl (version 2.2.1; Johnson and London, 2018). We fit 2-state HMM models with the two states representing a “moving” state and an “encamped” state. The “moving” state was described by longer hourly step lengths and low turning angles while the “encamped” state was described by short hourly step lengths and high turning angles. We fit a two-state model as we anticipated that our data would not allow for differentiation between different tortoise activities when movement lengths were below 10 m (e.g., foraging vs. thermoregulating) and different movement purposes (e.g., nesting vs. socializing; Pohle et al., 2017). We used a gamma distribution for the step length and a von Mises distribution for turning angles as well as a zero-mass parameter to account for time steps with no movement.
We first fit models to determine if environmental covariates altered the probability of state transition between the moving and stationary states. Tortoises are largely diurnal, and their activity is largely determined by environmental conditions such as temperature and food availability which change throughout the year (Woodbury and Hardy, 1948; Ruby et al., 1994b; Rautenstrauch et al., 2002; Ennen et al., 2012). We fit models incorporating hour and Julian date and their interaction to find the best combination of the two variables to explain transition probability of tortoises changing from encamped to moving states. We used the cosinor function from momentuHMM on the hour variable to allow state transition probability to reflect the typical diurnal activity patterns of desert tortoise (Zimmerman et al., 1994). We also tested models that looked for relationships between sex and transition probability, as male tortoises are often more active than females (Agha et al., 2015a). We then used the top-ranked ranked model (based on AIC), representing circadian and circannual movement patterns, to test additional environmental predictors that we hypothesized to alter the transition probabilities of movement states.
We fit generalized HMMs to the best performing state transition model that related lengths of hourly time steps to environmental covariates and the sex covariate. We restricted our modeling of step related covariates to only consider effects of environmental covariates on steps made while in the moving state, as the mean step length in the encamped state was below that of the positional error (~ 10 m) of our datasets. We fit models relating both the mean and standard deviation of step length and the mean and concentration of turning angles to the environmental covariates. We compared models with additive combinations of step covariates using AIC to find the most supported combination of covariates. For the Hidden Valley site, we examined the potential for an interaction term between the categorical burn predictor and distance to dirt roads. We fit and compared models for each site separately as not all anthropogenic features were present at each site, and we wanted to determine if covariates had similar effects among sites. We also attempted to fit random intercepts for each tortoise as a covariate in both the transition and step models to allow for the potential of individual-level differences in parameters.
3. Results
Tracking of 155 different tortoises across seven sites resulted in 790922 GPS locations after filtering (Table 1). After filtering data from both logger types, the resulting dataset had hourly relocations with an estimated location error of 10 meters. Stationary GPS loggers within tortoise burrows failed to record any fixes during a month of deployment, indicating that tortoises located in burrows would be unlikely to have GPS locations recorded. We successfully fit 2-state HMMs to our data from each site. The moving state was characterized by longer hourly step lengths and more narrow turning angles, while the encamped state was characterized by short hourly step lengths and wider turning angles (Figure 2). Mean hourly step lengths for the moving state differed moderately amongst sites.
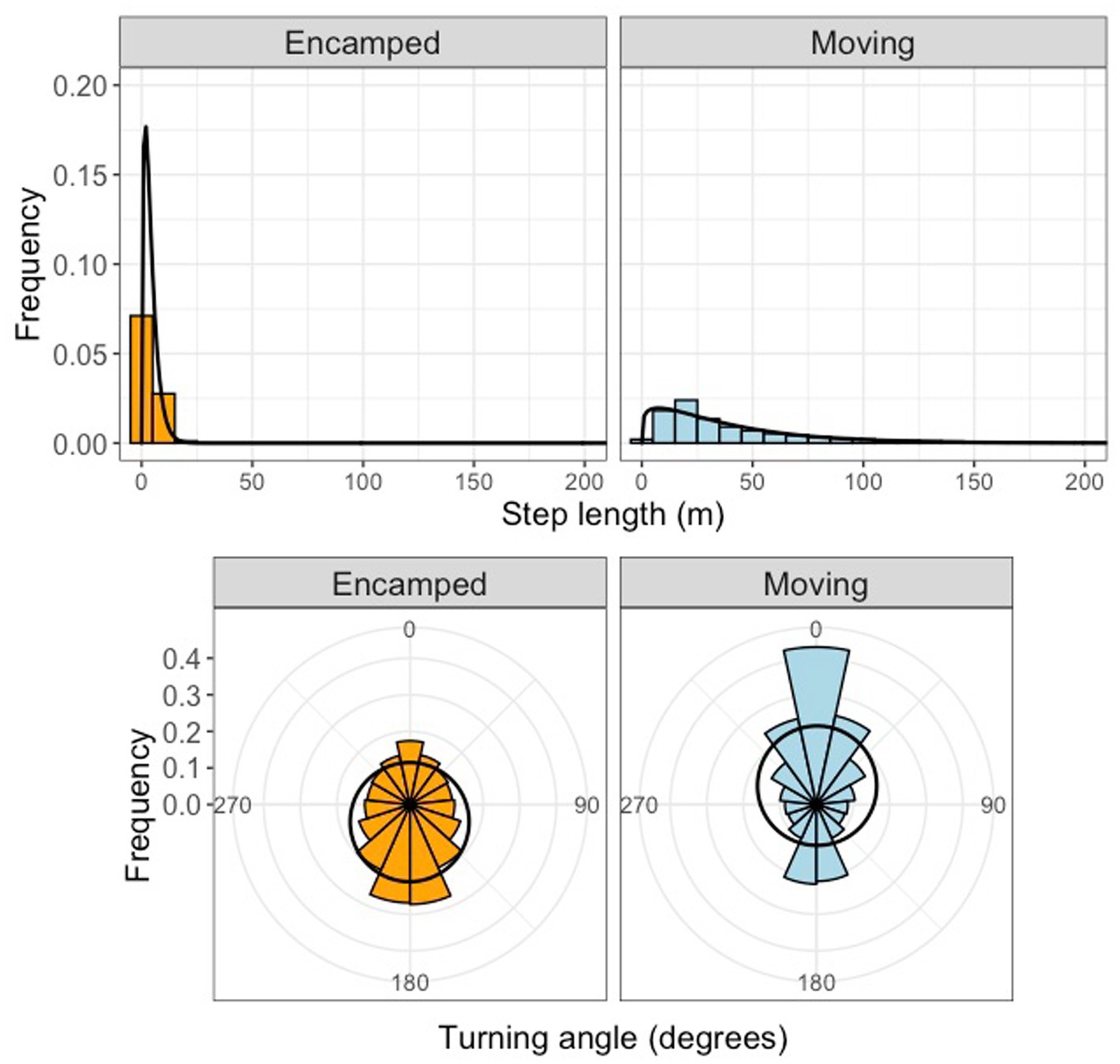
Figure 2. Step length and turning angle distributions for different movement behavior states for models fit to Mojave desert tortoise movement data. The encamped state (orange) was characterized by shorter mean step lengths and high turning angles while the moving state (blue) was characterized by longer mean step lengths and low turning angles.
3.1. State transition models
The top-ranked behavioral state probability models were similar across our seven study sites, although the best models for some sites did not include all covariates tested, as presented in Table 2. AIC tables for models run at each site are given in the Supplementary Materials. All top models performed better than null models with no covariates. We found that an interaction between the continuous day-of-year variable with the cyclical hour variable best accounted for state, where tortoises were more likely to be moving in the earlier days of the active season (spring) and more likely to be moving during the daylight hours (Figure 3). The best model for every site included sex as a predictor, with males more likely to be in the moving state and more likely to remain in the moving state once moving (Figure 3).
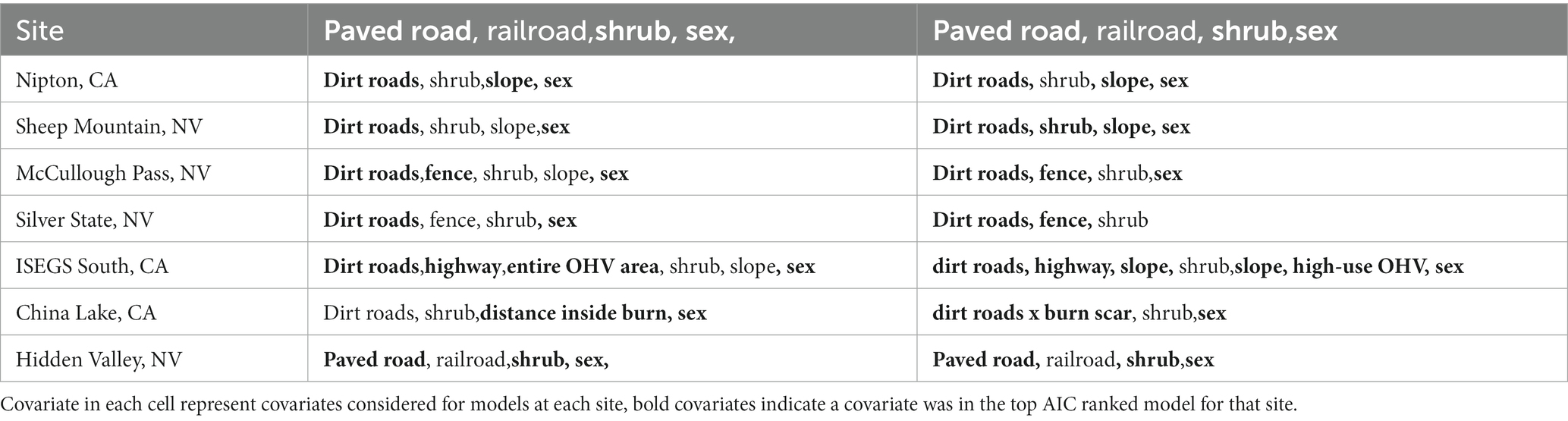
Table 2. Covariates that were in the tested in hidden Markov movement models for tortoise movement data.
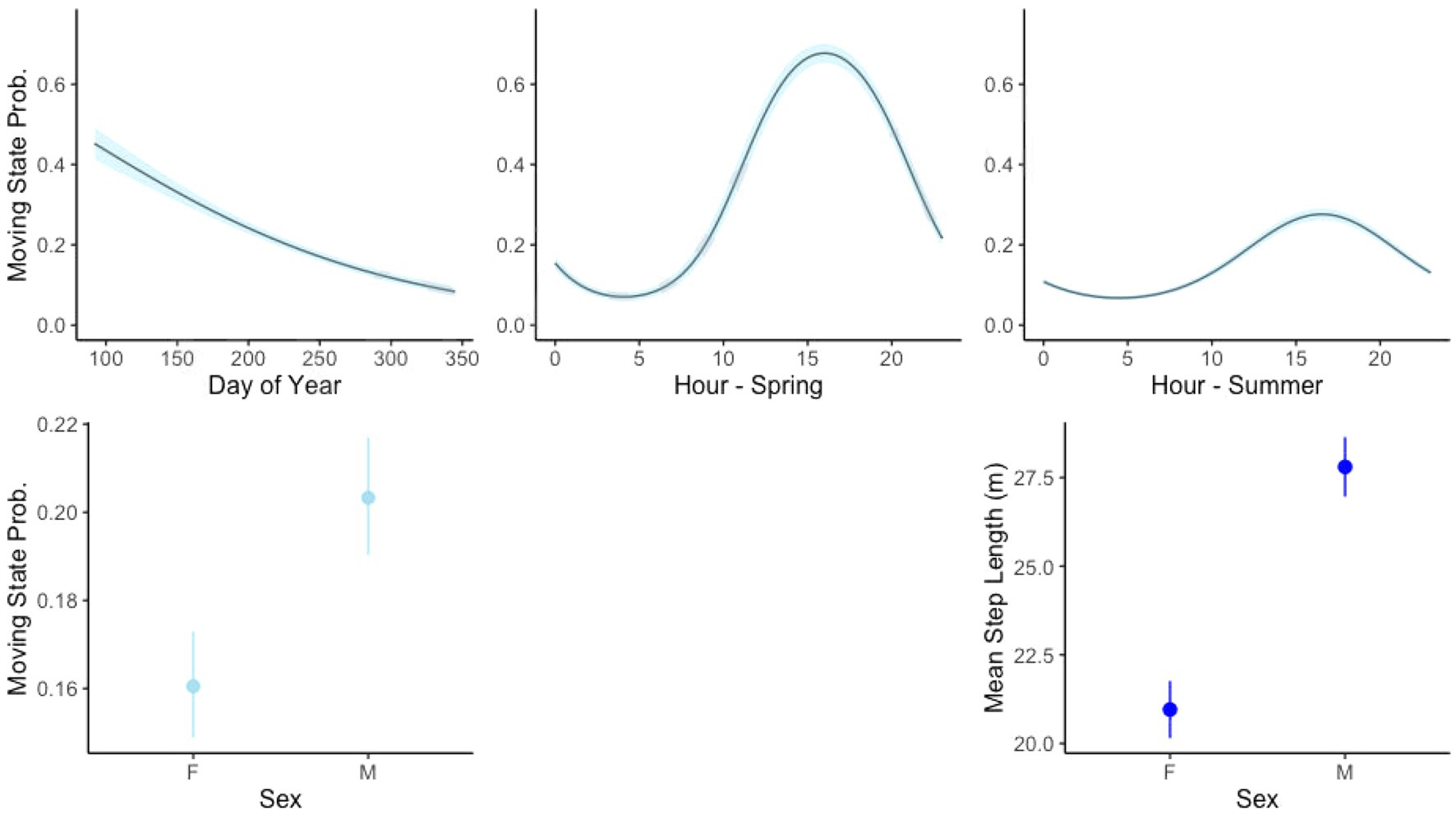
Figure 3. Relationship between covariates between stationary state probability (movement behavior state with other covariates held constant) and mean step length in the moving state for variables included in all models of tortoise movement.
State transition probabilities were affected by distance to dirt roads at every site except Hidden Valley, though the AIC difference was low between the best model at that site and the model including the dirt road covariate (Δ2.4 AIC). In all sites with distance to dirt road in the transition model, tortoises were more likely to be in the moving state when close to a dirt road, except for China Lake and Silver State which showed the opposite relationship (Figure 4). Distance to paved road was included in the top transition model for the Nipton site, but was not in the top model for China Lake, although the difference in AIC was relatively low between the best model and the model that included the paved road covariate for this site (Δ1.1 AIC). Tortoises at the Nipton site were more likely to be moving when closer to the paved road. The distance to railroad covariate was not included in the top model for the Nipton site; likely because tortoises were seldom located close to the railroad. Distance to fence was included in the top model for both sites where fences were present and indicated that tortoises were more likely to be moving when close to the fence (Figure 4).
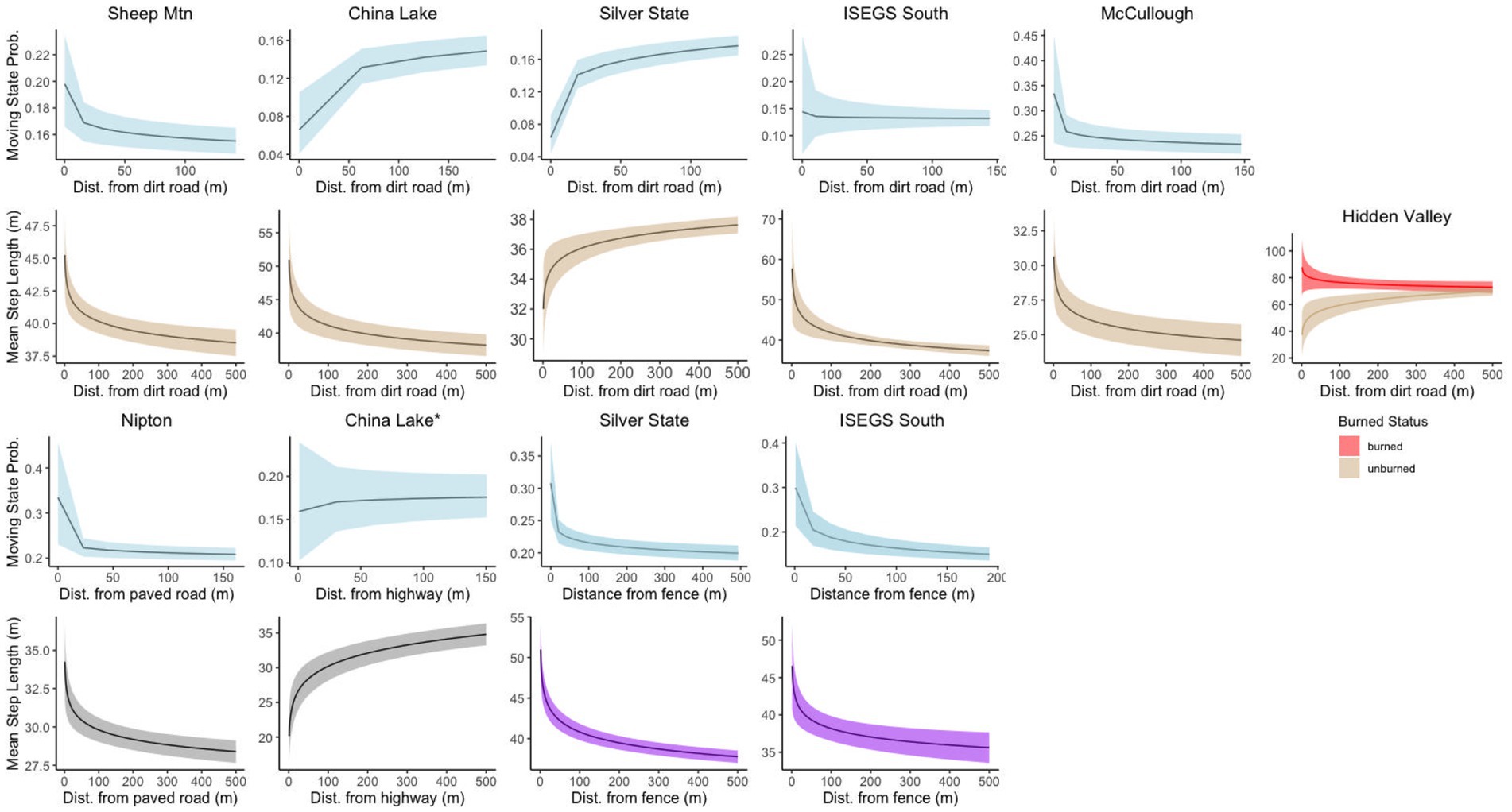
Figure 4. Stationary state transition probabilities and movement step lengths for covariates related to linear disturbances at different sites for tortoise movement. Tortoises responded to disturbances differently in different contexts. *The relationship between movement state and distance to highway was not in the top model for China Lake but is displayed for informational purposes.
The categorical covariate representing the OHV use area was in the top model for China Lake; tortoises were more likely to be moving in areas with relatively low OHV use and grazing than in areas without these disturbances. Distance inside the burn scar was in the top transition model for the Hidden Valley site, suggesting tortoises were more likely to be moving in areas closer to the edge of the burned area (Figure 5). Slope was included only in the best transition model for the Sheep Mountain site, suggesting that tortoises are more likely to be in the moving state in areas of higher slope. The only site that contained the shrub covariate in the top model was Nipton, which suggests that tortoises were more likely to be moving in areas of higher shrub cover. For all sites, a random intercept model for each individual was found to improve the AIC as there was considerable variation amongst individuals (Supplemental Figure 1). However, we chose not to include this in subsequent model fits as it added considerably to computational times and did not provide additional information on the covariates of interest.
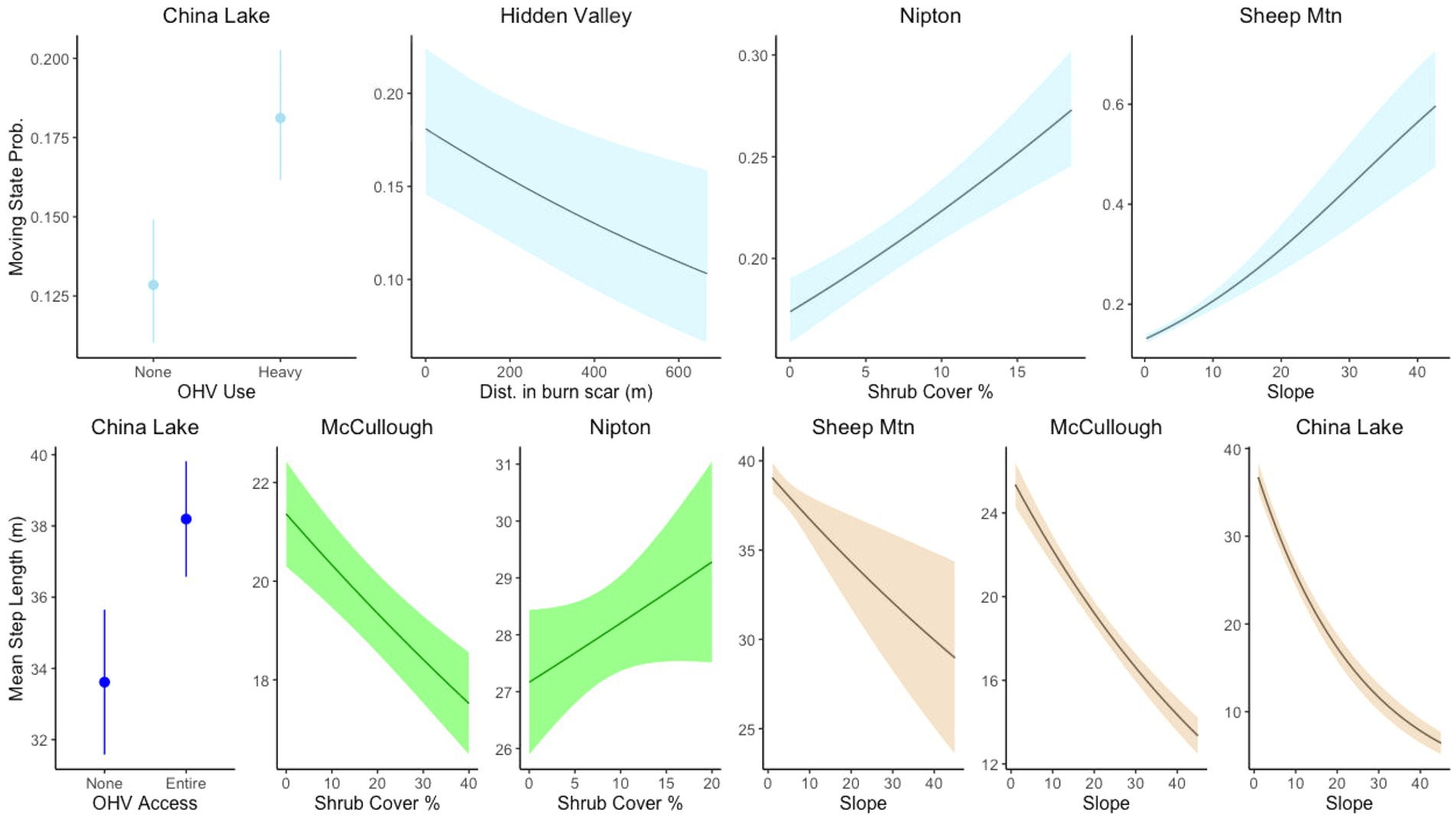
Figure 5. Stationary state transition probabilities and movement step lengths for covariates related to natural habitat features and landscape scale disturbances at different sites for tortoise movement. Tortoises responded to disturbances differently in different contexts.
3.2. Step length models
All top-ranked step length models performed better than null models; selection tables of all models fit examined are available in the Supplemental Materials. Dirt roads were included in the top model for every site where present. Hourly step lengths were both longer and more variable when close to dirt roads at every site except Silver State (Figure 4). The covariate for distance to paved road was in the top step parameter model for both the Nipton and China Lake sites, though they had the opposite effects. When moving closer to the paved road in Nipton, tortoises had longer hourly steps. In contrast, tortoises at China Lake had shorter hourly steps when closer to the paved road (Figure 4). Distance to fence was included as a covariate in the step length models for both the Silver State and ISEGS South sites, which were the only sites with fences. Hourly steps were longer when tortoises were moving near the fences (Figure 4). The OHV covariate representing the relatively heavy use area was included in the step model for China Lake, indicating that tortoises took longer hourly steps when moving in the high OHV use area (Figure 5). The fire scar covariate was included in the best model for the Hidden Valley Site with an interaction with the road covariate that indicated that tortoises took longer hourly steps near roads in burned areas but shorter hourly steps near roads in unburned areas (Figure 4). All the sites that had varied terrain (McCullough Pass, China Lake, Sheep Mountain) included slope in the top step model, indicating that tortoises took shorter steps in areas of higher slope (Figure 5). The shrub covariate was in the best model for McCullough pass and Nipton and indicated that hourly steps were shorter in areas that had higher shrub cover for McCullough pass, but hourly steps were longer in areas of high shrub cover for Nipton (Figure 5).
4. Discussion
Describing changes in animal movement behavior in relation to environmental conditions is an important step toward understanding how disturbances and habitat features may shape ecological processes for a species. Our results describe fine-scale tortoise movement behavior that corroborates prior knowledge, and importantly, provide insight on movement responses to anthropogenic disturbances. As hypothesized, known relationships between temporal period and tortoise sex were recovered by our models. Tortoise behavior is known to change from day to night and throughout the year, and our models captured the diurnal habits of the species, as well as reduced movement propensity in hot summer months (Rautenstrauch et al., 2002; Inman et al., 2009). Our models also found that male tortoises are more likely to be moving and make longer movements than female tortoises, similar to the findings of other telemetry-based studies (Agha et al., 2015a; Sadoti et al., 2017). Tortoises typically move less in years of lower forage production, although they make longer movements when doing so (U. S. Fish and Wildlife Service, 1994; Duda et al., 1999; Sadoti et al., 2017). Increased movements likely occur in areas with reduced resources due to disturbance, especially as tortoises seek out rare forage items on the landscape (Jennings and Berry, 2015). In this study, our models consistently recovered relevant parameters confirming preexisting knowledge of tortoise ecology, and we demonstrated that HMMs provide a suitable method to analyze movement behavior in the presence of variable conditions. In addition, we found that tortoises typically responded to disturbances as we hypothesized, at both linear and habitat scales, though that these responses can depend on different contexts.
4.1. Natural habitat features
We found that tortoise movement behavior is influenced by natural features such as slope and shrub cover. Tortoise movement is limited by high slope (Hromada et al., 2020), and we found that tortoises made shorter hourly movements in higher slope areas at all three sites with varied terrain. This finding could be due to the higher cost of movement through rougher terrain, or potentially higher availability of resources in rugged areas. Sloped areas in tortoise habitat often feature rock outcrops that can provide areas where water pools during rain, shelter sites, forage cover, and areas of higher thermal inertia that may buffer extreme temperatures (Nowicki et al., 2019). The only site where we recovered a relationship between movement state and slope is also the only site on which tortoises moved between creosote flats and hills. Some studies have suggested that Mojave desert tortoise populations in areas with varied terrain may be more resilient to declines than those in flat areas (Allison and McLuckie, 2018; Berry et al., 2020c). Future research should focus on the causes and potential importance of these areas in preserving the species. We found that shrub cover was an important predictor of tortoise movement at only two of the seven sites in our study (Nipton and McCullough Pass). At Nipton, we found that tortoises were more likely to move, and take longer hourly steps, in areas of higher shrub cover, while tortoises at McCullough Pass were more likely to take shorter hourly steps in areas of higher shrub cover. The Nipton area is flat, sandy, and has relatively low shrub cover; areas of higher shrub cover generally occur along washes where tortoises prefer to move, though foraging opportunities may be lower (Jennings and Berry, 2015; Gray et al., 2019; Hromada et al., 2020). In contrast, McCullough pass is rugged and rocky with much higher overall shrub cover; tortoises may move more slowly in areas of higher shrub cover here due to higher availability of desirable resources (e.g., shade, forage underneath shrubs), and higher slopes often associated with that cover. While previous studies have shown that tortoises prefer moving in areas of higher perennial vegetation cover (Sadoti et al., 2017; Hromada et al., 2020), our results illustrate the importance of site-specific context in how tortoises are responding to environmental conditions.
4.2. Responses To linear and habitat disturbances
4.2.1. Paved roads
Our results indicate that tortoises respond differently to roads in different environmental contexts. Heavily trafficked paved roads have been associated with localized tortoise decline and shown to fragment tortoise populations (Nafus et al., 2013; Dutcher et al., 2020). Tortoises at the China Lake site took shorter steps when near the high-traffic highways, and distance to the highways did not appreciably affect the probability of being in a moving behavioral state. One possible reason for this could be that these tortoises perceive the danger of the highway and make shorter movements in an attempt to cross at a safe time, or they may become startled by traffic and withdraw into their shells as an attempted defense mechanism. Turtles, and many other species of reptiles, are considered ‘pausers’ in their response to traffic (Jacobson et al., 2016). Desert tortoises are known to respond by pausing to perceived threats, which may lead to shorter hourly step lengths near roads with high traffic levels (Ruby and Niblick, 1994). Some of the paths tortoises took near the highway ran parallel to the highway and may represent foraging trips taking advantage of increased plant growth from the road run off, or just a reluctance to cross the highway (Brooks and Lair, 2005). Although all tortoises monitored at China Lake (n = 28) were found within 1 km of the highway, only four (including one dispersing juvenile too small for a GPS logger) ever crossed the highway. While this could be due to road avoidance, it is also possible that the established home ranges of these animals did not include the highways. Tortoise mortalities were noted on the China Lake highway in years prior to and during our study period; thus, the remaining tortoises that continue to cross may be the few individuals that have so far managed to survive crossing. Our findings at China Lake may also be related to how the few crossings we observed. The tortoises that crossed the highway spent little time near the road when crossing, so paths of other tortoises along the road would likely have overwhelmed the small sample size of crossing events captured by our data. The one male tortoise that made multiple crossings of the highways at China Lake lost its GPS logger after two crossings, so data for those events were not available for our analysis.
We found the opposite relationship with the low-traffic paved road in the Nipton site, which may represent less perceived risk. When tortoises choose to make a movement near it, they may make the movement faster than they would otherwise, as the road typically provides no resources outside of rain events that pool water on the road surface (Medica et al., 1980). One additional difference between the highway at China Lake and the paved road at Nipton is that the soft shoulders of the China Lake highways are actively maintained (graded) while the Nipton road shoulder has infrequent maintenance. This additional disturbance of the soil around the highway may cause tortoises to alter their behavior, as the soil is less compacted (potentially more difficult to move through), but also may provide forage plants (e.g., Astragalus sp.) that are not commonly available elsewhere and may attract tortoise activity (Jennings and Berry, 2015).
4.2.2. Dirt roads
Our results indicate that dirt roads typically influence tortoise movement behavior in the way we hypothesized, but not always in the same manner. Prior research has demonstrated that tortoises are less likely to make movements in the vicinity of dirt roads (Sadoti et al., 2017; Gray et al., 2019; Hromada et al., 2020), yet tortoises still encounter and cross these disturbances regularly. Our results suggested that tortoises were more likely to be in a moving state closer to roads in some sites (McCullough Pass and Sheep Mountain), while they were more likely to be in the encamped state closer to dirt roads in others (China Lake and Silver State). This may be due to the different context of the roads at these different sites; the areas where the roads exist in China Lake and Silver State are more disturbed by anthropogenic activities. Many of the dirt roads at China Lake are located within sandy washes where OHV traffic has resulted in loose soil that may discourage tortoise movement. The main road at Silver State falls within a rocky wash in the area and is located ~ 40 m from the fenced solar facility; and many of the translocated tortoises use burrows and shelter sites directly adjacent to the road. The pattern recovered from our other sites, where tortoises are more likely to be moving when near a road, suggests that where dirt roads are in more undisturbed contexts, tortoises are more likely to be moving when near them. We found that when tortoises are moving near roads, at all sites aside from Silver State, they were more likely to make longer hourly movements. Tortoises have been noted to make movements along gravel roads (Grandmaison et al., 2010; Agha et al., 2015b), and it might be the case that roads allow for faster movement through some areas. However, as tortoises are known to avoid moving near roads, this behavior may be indicative of avoidance behavior, in that tortoises could be moving faster to minimize the time they spend in an exposed areas that offer few resources and increased exposure to threats. Movements of tortoises that interact with roads at Silver State are restricted by fences and they are forced to spend more time closer to roads. The primary road at this location is in a wash and used as part of an annual OHV race. As tortoises cannot as easily move away from the area directly affected by the roads, they may move slower to use resources associated with the wash, and to avoid perceived danger from road activity. These differences in tortoise movement behavior are influenced by different contexts of roads and may play a role in connectivity of tortoise populations.
4.2.3. Fencing
Fences have been built to prevent desert tortoises from accessing high-traffic highways throughout its range, and fenced utility scale solar energy development has been constructed on previously suitable habitat. As these fences often intersect areas that were previously available to tortoises, individuals will often pace the fence attempting to return to previously known resources (Ruby et al., 1994a; Peaden et al., 2017; Sadoti et al., 2017). Tortoises are more likely to be moving in and took longer hourly steps in areas closer to the fences supporting observations of these prior studies, and as we had hypothesized. Vegetation was removed from areas immediately adjacent to fences at our study sites; thus, tortoises moving along the fences are likely exposed to dangerous thermal environments that may influence movement behavior (Peaden et al., 2017; Nowakowski et al., 2020). Tortoises that interacted with fences were primarily translocated tortoises, with only one exception. This non-translocated tortoise, originally found outside the solar footprint in the ISEGS South site, continued to pace along the fence 10 years after its construction, and the translocated tortoises at the Silver State site continued to pace the fence 7 years post construction. This suggests that these linear disturbances can have a long-lasting impact on the space use and movement behavior when placed into formerly occupied tortoise habitat and used to exclude former resident tortoises. Translocated tortoises are known to move more than resident animals when translocated into unfamiliar habitat (Nussear et al., 2012); the increased movement behavior and movement length near the fence are similar to these prior findings. There may be a different behavioral response to fences by tortoises that were not subject to fence construction within their established home range area (e.g., offspring of tortoises translocated from solar installations or tortoises that disperse into an area with a fence). Studying animals that encountered fences throughout their lives would help us to better understand the potential long-term effects that fences may have on tortoise populations.
4.2.4. Off-highway vehicle activity and grazing
Off-highway vehicle recreation continues to grow in popularity within the deserts of the southwestern United States, as desert urban growth continues to sprawl, and off-highway vehicles become more capable and accessible. We were unable to independently estimate the effects of livestock grazing and OHV use on tortoise movement at China Lake, due to multiple simultaneous land uses. However, we found that the covariates representing different levels of this OHV disturbance, indicated tortoise movement behavior was expressed in different ways. The probability of being in a moving state was higher in areas that had both OHV and grazing activity, suggesting that tortoises may need to move more often and more quickly due to scarcity of resources, and potentially in recognition of dangerous conditions. Research on movement in other species has shown context dependent relationships between movement behavior and human land use. African wild dogs moved more often and faster in areas of lower resources but slowed when human activity was present (Creel et al., 2020). Indeed, movements by a wide variety of species are influenced by anthropogenic habitat disturbance and human presence (Doherty et al., 2021). Both OHV activity and livestock grazing reduce plant cover, damage tortoise burrows, and cause tortoise mortalities (Lovich, 1999; Switalski, 2018). The best model supported longer hourly step lengths in the high use OHV area designated area. This could be due to a variety of factors. Tortoises may be influenced by high OHV use areas in a similar manner as they are with dirt roads, making faster movements to avoid areas with low resources and unsuitable environmental conditions. Many of the routes created by OHV activity in the high-use area are in sandy washes, creating potentially difficult moving conditions for tortoises and destroying vegetation important for both thermoregulation and forage. Prior work has shown that tortoise sign is reduced in areas with active OHV recreation in washes (Custer et al., 2017). As we noted in the results for the Nipton site, tortoises may be using washes as movement areas, and some of the signal that we found may be from the use of the washes by both tortoises and OHV recreationalists for movement. Livestock grazing in the Mojave Desert may have some effects on plant cover and soil conditions. Grazing has been shown to cause soil compaction as well as a reduction in cover of native annual and perennial plants, increasing the potential of colonization by invasive annual plants (Webb and Stielstra, 1979; Nicholson and Humphreys, 1980; Brooks et al., 2006). Sheep grazing occurred throughout the OHV area. The BLM is tasked with assessing rangeland health of areas leased for grazing, yet the two allotments in our China Lake study area (Spangler Hills and Cantil Common) have never been assessed (Public Employees for Environmental Responsibility, 2022). The adjacent allotment (Rudnick Common) did not meet the BLM’s standard of rangeland health when last assessed in 2004 (Public Employees for Environmental Responsibility, 2022). The alteration of tortoise movement caused by the compounded effects of both landscape scale disturbances (i.e., OHV use and grazing) provides further evidence that tortoise habitat is degraded in these situations. More research would help us better understand how these separate land uses effect tortoise movement and populations.
4.2.5. Burned habitat
Wildfire is a relatively new disturbance on desert ecosystems. Historically, fire was limited in the Mojave Desert and rarely spread to landscape scales; however, the establishment of non-native, annual grasses has allowed for fire to rapidly spread between shrubs and effect large areas of suitable tortoise habitat (Brooks and Esque, 2002; Brooks and Matchett, 2006). Desert tortoises continue to inhabit burned areas, and health and reproductive output does not seem to be affected (Lovich et al., 2011, 2018; Drake et al., 2015). However the invasive annual grasses that typically colonize burned areas have been shown to have negative impacts on health and survivorship in juvenile tortoises (Drake et al., 2016). Our data came from an area that burned six years prior to GPS telemetry collection. VHF telemetry data from these animals suggested that tortoise use of the burned areas had declined by this time, concurrent with the decline of Sphaeralcea ambigua, an important cover and forage plant, in the burned area (Drake et al., 2015). Our results suggested that the tortoises were less likely to be moving in areas deeper in the fire scar, though lack of GPS logger data from the years immediately after the fire precluded any interpretation of how tortoise movement changed as time since fire increased. We also found that tortoises interacted with dirt roads differently depending on whether the roads were inside the fire scar or not; suggesting that tortoises make longer steps near roads in the fire scar and shorter steps near roads when not inside the fire scar.
4.3. Limitations
Although our analysis leveraged a large GPS dataset, we were limited in scope to only making inference on tortoise surface activity, as our GPS units could not record data while the tortoises were underground. Thus, our inference is on the surface activity of tortoises as data on time spent inactive in burrows was not available for this analysis. Although tortoises spend most of their time in burrows (Rautenstrauch et al., 2002), we were mostly concerned with how tortoises are moving. However, understanding where tortoises use burrows in relation to disturbances is also important in describing how disturbances alter tortoise space use. The use of an additional data loggers, such as a light-sensitive loggers, would enable researchers to capture the times that a tortoise was inactive and in a burrow. This could allow additional inference on surface activity and on how different disturbances and environmental settings may influence this. However, we have demonstrated the utility of these methods to describe the movement behavior of a reptile and how it is influenced by disturbed habitat from different sources. Though outside the scope of this study, the addition of site and year specific weather covariates that may also influence tortoise movement (Duda et al., 1999) would allow for a more comprehensive model of tortoise movement behavior.
Individual-level variation in behavior can be an important aspect to consider in movement ecology (Merrick and Koprowski, 2017; Spiegel et al., 2017). Tortoises have distinct personalities that can play an important role in determining movement patterns and responses to stressors (Currylow et al., 2017; Germano et al., 2017; Le Balle et al., 2021). Animal personality potentially determines how an individual responds to an environmental disturbance or features (Michelangeli et al., 2022), and it is likely that tortoises with different personalities have different propensities to cross roads or pace fences. If so, tortoises that are more likely to cross a road are more susceptible to mortality. As a result, the distribution of the variation in personality syndromes in a population may be altered, which could have emergent effects on population function (Spiegel et al., 2017). Although our results indicated that there was considerable individual-level variability around movement state probability, these models took considerable computational time and models with random slope terms typically failed to converge. Further exploration of individual-level movement variation and personality is warranted to better understand how personality in this species may influence spatial distribution of tortoises and the implications for genetic connectivity.
4.4. Management implications
Our results indicate that tortoise movement behavior is altered by both local and landscape scale disturbances. Both the initial, and the revised recovery plan for the Mojave desert tortoise recommends non-essential or redundant routes to be closed (U. S. Fish and Wildlife Service, 1994, 2011), and our results suggest that this action could aid in restoring historic connectivity of tortoises populations. Although fences alter tortoise movement behavior, they continue to be important tools in restricting tortoise access to dangerous areas; further work could better describe how mitigation (e.g., shade structures) may alter how tortoises interact with fences. OHV activity and grazing continue to be prevalent uses of public lands in the southwestern US; our results suggest that these uses may be degrading tortoise habitat. Further study of how these disturbances affect tortoise populations is warranted to better understand how areas designated for these purposes influence landscape scale connectivity and viability of tortoise populations.
Data availability statement
The datasets presented in this article are not readily available due to the sensitivity of the species and easily accessible study sites. Requests to access the datasets should be directed to: c3RldmVocm9tYWRhQGdtYWlsLmNvbQ==.
Ethics statement
The animal study was reviewed and approved by University of Nevada, Reno Animal Care and Use Committee protocol.
Author contributions
SH assisted in data collection, analyzed data, and wrote the manuscript. KN, TE, AV, and KD designed the overall projects, conducted and supervised data collection, and provided substantial comments on the manuscript. FC, BG, and JS managed field logistics and database upkeep and consistency as well as provided substantial comments on the manuscript. All authors contributed to the article and approved the submitted version.
Funding
This work was supported by the U.S. Bureau of Land Management, the U.S. Fish and Wildlife Service, the National Fish and Wildlife Foundation (8006.15.058835 and 8006.20.070838), Clark County Desert Conservation Program, USGS Priority Ecosystem Science Program, Coyote Springs Investment Corporation, the U.S. Geological Survey Ecosystems Mission Area Energy and Wildlife Program, and the China Lake Naval Air Weapons Station Environmental Management Division. The funder was not involved in the study design, collection, analysis, interpretation of data, the writing of this article or the decision to submit it for publication.
Acknowledgments
We thank the many people who collected tortoise telemetry and other associated data, especially R. Inman, P. Medica, A. Modlin, A. Macdonald, B. Cunningham, S. Murray, G. Berry, E. Gaylord, G. Gantz, R. Van Gaalen, S. Lewicki, S. Dahl, B. Smith, S. Thomson, C. Silva, C. Quick, A. Poage, M. Walden, K. Vittum, C. Phillips, S. Snyder, B. Jacobs, M. Walden, G. Olson, V. Van Zerr, R. Kunicki, A. Terry, J. Willand, E. Sinnott, A. Chaney, D. Essary, T. Carmona, B. Reynolds, J. Jay, R. Lamkin, A. Berger, M. Kang, P. Cosmann, P. Emblidge, C. Aiello, R. Saulino, R. Lamkin, K. Bauer, A. Smith, S.Steel, P. Van Linn, K. Huxster, M. Poelman, B. Waitman and others. We are particularly grateful to Amy Fesnock (BLM—California), Mark Slaughter (BLM—Nevada), and Julie Hendrix (China Lake NAWS) for their support of our desert tortoise research program. We also thank K. Dutcher, A. Parandhaman, C. Carroll, and W. Plafcan for helpful comments on an earlier version of the manuscript. We thank B. Prochazka and two peer reviewers for their constructive reviews of this manuscript. Any use of trade, firm, or product names is for descriptive purposes only and does not imply endorsement by the U.S. Government.
Conflict of interest
The authors declare that the research was conducted in the absence of any commercial or financial relationships that could be construed as a potential conflict of interest.
Publisher’s note
All claims expressed in this article are solely those of the authors and do not necessarily represent those of their affiliated organizations, or those of the publisher, the editors and the reviewers. Any product that may be evaluated in this article, or claim that may be made by its manufacturer, is not guaranteed or endorsed by the publisher.
Supplementary material
The Supplementary material for this article can be found online at: https://www.frontiersin.org/articles/10.3389/fevo.2023.971337/full#supplementary-material
References
Abella, S. R., Guida, R. J., Roberts, C. L., Norman, C. M., and Holland, J. S. (2019). Persistence and turnover in desert plant communities during a 37-yr period of land use and climate change. Ecol. Monogr. 89:e01390. doi: 10.1002/ecm.1390
Agha, M., Augustine, B., Lovich, J. E., Delaney, D., Sinervo, B., Murphy, M. O., et al. (2015a). Using motion-sensor camera technology to infer seasonal activity and thermal niche of the desert tortoise (Gopherus agassizii). J. Therm. Biol. 49-50, 119–126. doi: 10/ggcv2k
Agha, M., Lovich, J. E., Ennen, J. R., Augustine, B., Arundel, T. R., Murphy, M. O., et al. (2015b). Turbines and terrestrial vertebrates: variation in tortoise survivorship between a wind energy facility and an adjacent undisturbed wildland area in the desert southwest (USA). Environ. Manag. 56, 332–341. doi: 10/f7jq76
Allison, L. J., and McLuckie, A. M. (2018). Population trends in Mojave Desert tortoises (Gopherus agassizii). Herpetol. Conserv. Biol. 13, 433–452.
Berry, K. H., and Murphy, R. W. (2019). Gopherus agassizii (Cooper 1861)–Mojave Desert tortoise, Agassiz’s desert tortoise. Chelonian Res. Monogr. 5, 1–45. doi: 10.3854/crm.5.109.agassizii.v1.2019
Berry, K. H., Yee, J. L., and Lyren, L. M. (2020a). Feral burros and other influences on desert tortoise presence in the western Sonoran desert. Herpetologica 76, 403–413. doi: 10/ghzxs9
Berry, K. H., Yee, J., Lyren, L., and Mack, J. S. (2020c). An uncertain future for a population of desert tortoises experiencing human impacts. Herpetologica 76:1. doi: 10/ggrnpv
Berry, K. H., Yee, J. L., Shields, T. A., and Stockton, L. (2020b). The catastrophic decline of tortoises at a fenced natural area. Wild. Mon. 205, 1–53. doi: 10/gg7ftc
Beyer, H. L., Morales, J. M., Murray, D., and Fortin, M.-J. (2013). The effectiveness of Bayesian state-space models for estimating behavioural states from movement paths. Methods Ecol. Evol. 4, 433–441. doi: 10/f4xqwz
Boarman, W. I., and Sazaki, M. (2006). A highway’s road-effect zone for desert tortoises (Gopherus agassizii). J. Arid Environ. 65, 94–101. doi: 10/fgdn5j
Brooks, M. L. (2012). Effects of high fire frequency in creosote bush scrub vegetation of the Mojave desert. Int. J. Wildland Fire 21:61. doi: 10/d8wmnq
Brooks, M. L., and Esque, T. C. (2002). Alien plants and fire in desert tortoise (Gopherus agassizii) habitat in the Mojave and Colorado deserts. Chelonian Conserv. Biol. 4, 330–340.
Brooks, M. L., and Lair, B. (2005). Ecological Effects of Vehicular Routes in a Desert Ecosystem. Las Vegas, NV: United States Geological Survey, Western Ecological Center.
Brooks, M. L., and Matchett, J. R. (2006). Spatial and temporal patterns of wildfires in the Mojave Desert, 1980–2004. J. Arid Environ. 67, 148–164. doi: 10/cwmqv9
Brooks, M. L., Matchett, J. R., and Berry, K. H. (2006). Effects of livestock watering sites on alien and native plants in the Mojave Desert, USA. J. Arid Environ. 67, 125–147. doi: 10.1016/j.jaridenv.2006.09.022
Bury, R. B., and Luckenbach, R. A. (2002). Comparison of desert tortoise (Gopherus agassizii) populations in an unused and off-road vehicle area in the Mojave Desert. Chelonian Conserv. Biol. 4, 457–463.
Calabrese, J. M., Fleming, C. H., and Gurarie, E. (2016). Ctmm: an R package for analyzing animal relocation data as a continuous-time stochastic process. Methods Ecol. Evol. 7, 1124–1132. doi: 10/f86qch
Carter, M. I. D., McClintock, B. T., Embling, C. B., Bennett, K. A., Thompson, D., and Russell, D. J. F. (2020). From pup to predator: generalized hidden Markov models reveal rapid development of movement strategies in a naïve long-lived vertebrate. Oikos 129, 630–642. doi: 10/gn7shx
Creel, S., Merkle, J., Mweetwa, T., Becker, M. S., Mwape, H., Simpamba, T., et al. (2020). Hidden Markov models reveal a clear human footprint on the movements of highly mobile African wild dogs. Sci. Rep. 10:17908. doi: 10.1038/s41598-020-74329-w
Currylow, A. F. T., Louis, E. E., and Crocker, D. E. (2017). Stress response to handling is short lived but may reflect personalities in a wild, critically endangered tortoise species. Conserv. Physiol. 5:cox0008. doi: 10.1093/conphys/cox0008
Custer, N. A., DeFalco, L. A., Nussear, K. E., and Esque, T. C. (2017). Drawing a line in the sand: effectiveness of off-highway vehicle management in California’s Sonoran desert. J. Environ. Manag. 193, 448–457. doi: 10/gg23g3
Doherty, T. S., Hays, G. C., and Driscoll, D. A. (2021). Human disturbance causes widespread disruption of animal movement. Nat. Ecol. Evol. 5, 513–519. doi: 10.1038/s41559-020-01380-1
Drake, K. K., Bowen, L., Nussear, K. E., Esque, T. C., Berger, A. J., Custer, N. A., et al. (2016). Negative impacts of invasive plants on conservation of sensitive desert wildlife. Ecosphere 7, 1–20. doi: 10.1002/ecs2.1531
Drake, K. K., Esque, T. C., Nussear, K. E., Defalco, L. A., Scoles-Sciulla, S. J., Modlin, A. T., et al. (2015). Desert tortoise use of burned habitat in the eastern Mojave desert. J. Wild. Mgmt. 79, 618–629. doi: 10/gf8x6x
Duda, J. J., Krzysik, A. J., and Freilich, J. E. (1999). Effects of drought on desert tortoise movement and activity. J. Wildl. Manag. 63:1181. doi: 10/dqvxv6
Dutcher, K. E., Vandergast, A. G., Esque, T. C., Mitelberg, A., Matocq, M. D., Heaton, J. S., et al. (2020). Genes in space: what Mojave desert tortoise genetics can tell us about landscape connectivity. Conserv. Genet. 21, 289–303. doi: 10/ggkqzg
Ennen, J. R., Meyer, K., and Lovich, J. (2012). Female Agassiz’s desert tortoise activity at a wind energy facility in southern California: the influence of an El Niño event. Nat. Sci. 4, 30–37. doi: 10/fz2xqw
Esque, T. C., Kaye, J. P., Eckert, S. E., DeFalco, L. A., and Tracy, C. R. (2010). Short-term soil inorganic N pulse after experimental fire alters invasive and native annual plant production in a Mojave desert shrubland. Oecologia 164, 253–263. doi: 10/ckrrm9
Friends of Jawbone (2022). Spangler Hills OHV open riding area. Available at: http://www.owlsheadgps.com/owlshead/index.php/ohv-open-areas-2/79-spangler-hills-ohv-open-riding-area. Accessed January 6, 2022.
Germano, J. M., Nafus, M. G., Perry, J. A., Hall, D. B., and Swaisgood, R. R. (2017). Predicting translocation outcomes with personality for desert tortoises. Behav. Ecol. 28, 1075–1084. doi: 10/gbswk2
Grandmaison, D. D., Ingraldi, M. F., and Peck, F. R. (2010). Desert tortoise microhabitat selection on the Florence military reservation, south-Central Arizona. J. Herpetol. 44, 581–590. doi: 10/ftms78
Gray, M. E., Dickson, B. G., Nussear, K. E., Esque, T. C., and Chang, T. (2019). A range-wide model of contemporary, omnidirectional connectivity for the threatened Mojave desert tortoise. Ecosphere 10, 1–16. doi: 10/gf7vs6
Hijmans, R. J., Etten, J.Van, Sumner, M., Cheng, J., Baston, D., Bevan, A., et al. (2022). Raster: Geographic data analysis and modeling. Available at: https://CRAN.R-project.org/package=raster
Homer, C., and Fry, J. (2012). The National Land Cover Database United States Geological Survey. Fact Sheet 2012–3020.
Hooten, M. B., Johnson, D. S., McClintock, B. T., and Morales, J. M. (2017). Animal Movement: Statistical Models for Telemetry Data. 1st. Boca Raton, FL: CRC Press/Taylor & Francis Group.
Hromada, S. J., Esque, T. C., Vandergast, A. G., Dutcher, K. E., Mitchell, C. I., Gray, M. E., et al. (2020). Using movement to inform conservation corridor design for Mojave desert tortoise. Mov. Ecol. 8, 1–18. doi: 10/ghg88t
Inman, R. D., Nussear, K. E., and Tracy, C. R. (2009). Detecting trends in desert tortoise population growth: elusive behavior inflates variance in estimates of population density. Endang. Species. Res. 10, 295–304. doi: 10/dx8n2c
Jacobson, S. L., Bliss-Ketchum, L. L., Rivera, C. E., and Smith, W. P. (2016). A behavior-based framework for assessing barrier effects to wildlife from vehicle traffic volume. Ecosphere 7:e01345. doi: 10.1002/ecs2.1345
Jennings, W. B., and Berry, K. H. (2015). Desert tortoises (Gopherus agassizii) are selective herbivores that track the flowering phenology of their preferred food plants. PLoS One 10:e0116716. doi: 10.1371/journal.pone.0116716
Johnson, D. S., and London, J. M. (2018). Crawl: An R package for fitting continuous-cime correlated random walk models to animal movement data. doi: 10.5281/zenodo.596464,
Kays, R., Crofoot, M. C., Jetz, W., and Wikelski, M. (2015). Terrestrial animal tracking as an eye on life and planet. Science 348:aaa2478. doi: 10/ggb9mj
Laver, P. N., Powell, R. A., and Alexander, K. A. (2015). Screening GPS telemetry data for locations having unacceptable error. Eco. Inform. 27, 11–20. doi: 10/gd2xnt
Le Balle, R., Cote, J., and Fernandez, F. A. S. (2021). Evidence for animal personalities in two Brazilian tortoises (Chelonoidis denticulatus and Chelonoidis carbonarius) and insights for their conservation. Appl. Anim. Behav. Sci. 241:105400. doi: 10/gm4f3n
Lightfoot, D. C., and Whitford, W. G. (1991). Productivity of creosotebush foliage and associated canopy arthropods along a desert roadside. Am. Midl. Nat. 125:310. doi: 10/dfz5mc
Lovich, J. E. (1999). Anthropogenic degradation of the southern California desert ecosystem and prospects for natural recovery and restoration. Environ. Manag. 24, 309–326. doi: 10.1007/s002679900235
Lovich, J. E., Agha, M., Ennen, J. R., Arundel, T. R., and Austin, M. (2018). Agassiz’s desert tortoise (Gopherus agassizii) activity areas are little changed after wind turbine induced fires in California. Int. J. Wildland Fire 27:851. doi: 10.1071/WF18147
Lovich, J. E., Ennen, J. R., Madrak, S. V., Loughran, C. L., Meyer, K. P., Arundel, T. R., et al. (2011). Long-term post-fire effects on spatial ecology and reproductive output of female Agassiz’s desert tortoises (Gopherus agassizii) at a wind energy facility near Palm Springs, California, USA. Fire Ecol. 7, 75–87. doi: 10/fzv658
McClintock, B. T., Johnson, D. S., Hooten, M. B., Hoef, J. M. V., and Morales, J. M. (2014). When to be discrete: the importance of time formulation in understanding animal movement. Mov. Ecol. 2, 1–14.
McClintock, B. T., King, R., Thomas, L., Matthiopoulos, J., McConnell, B. J., and Morales, J. M. (2012). A general discrete-time modeling framework for animal movement using multistate random walks. Ecol. Monogr. 82, 335–349. doi: 10/f398sj
McClintock, B. T., London, J. M., Cameron, M. F., and Boveng, P. L. (2017). Bridging the gaps in animal movement: hidden behaviors and ecological relationships revealed by integrated data streams. Ecosphere 8:e01751. doi: 10/f9zs6t
McClintock, B. T., and Michelot, T. (2018). momentuHMM: R package for generalized hidden Markov models of animal movement. Methods Ecol. Evol. 9, 1518–1530. doi: 10/gc7qx6
Medica, P. A., Bury, R. B., and Luckenbach, R. A. (1980). Drinking and construction of water catchments by the desert tortoise, Gopherus agassizii, in the Mojave Desert. Herpetologica 36, 301–304.
Medica, P. A., Lyons, C. L., and Turner, F. B. (1982). A comparison of 1981 populations of desert tortoise (Gopherus agassizi) in grazed and ungrazed areas in Ivanpah Valley, California. in Proceedings of the 1982 Symposium. ed. K. Hashagen.
Merrick, M. J., and Koprowski, J. L. (2017). Should we consider individual behavior differences in applied wildlife conservation studies? Biol. Conserv. 209, 34–44. doi: 10/gbm62v
Michelangeli, M., Payne, E., Spiegel, O., Sinn, D., Leu, S. T., and Sih, A. (2022). Personality, spatiotemporal ecological variation, and resident/explorer movement syndromes in the sleepy lizard. J. Anim. Ecol. 91, 210–223.
Mitchell, C. I., Shoemaker, K. T., Esque, T. C., Vandergast, A. G., Hromada, S. J., Dutcher, K. E., et al. (2021). Integrating telemetry data at several scales with spatial capture–recapture to improve density estimates. Ecosphere 12:e03689. doi: 10.1002/ecs2.3689
Morales, J. M., Haydon, D. T., Frair, J., Holsinger, K. E., and Fryxell, J. M. (2004). Extracting more out of relocation data: building movement models as mixtures of random walks. Ecology 85, 2436–2445. doi: 10/bw24dj
Nafus, M. G., Tuberville, T. D., Buhlmann, K. A., and Todd, B. D. (2013). Relative abundance and demographic structure of Agassiz’s desert tortoise (Gopherus agassizii) along roads of varying size and traffic volume. Biol. Conserv. 162, 100–106. doi: 10/gf5kbd
Nicholson, L., and Humphreys, K. (1980). Sheep grazing at the Kramer study plot, San Bernardino County, California. in Proceedings of the 1981 symposium (Long Beach, CA). ed. K. Hashagen. 163–194.
Nickel, B. A., Suraci, J. P., Nisi, A. C., and Wilmers, C. C. (2021). Energetics and fear of humans constrain the spatial ecology of pumas. Proc. Natl. Acad. Sci. U. S. A. 118:e2004592118. doi: 10/ghwdsm
Nowakowski, A. J., Peaden, J. M., Tuberville, T. D., Buhlmann, K. A., and Todd, B. D. (2020). Thermal performance curves based on field movements reveal context-dependence of thermal traits in a desert ectotherm. Landsc. Ecol. 35, 893–906. doi: 10/ggw6cv
Nowicki, S. A., Inman, R. D., Esque, T. C., Nussear, K. E., and Edwards, C. S. (2019). Spatially consistent high-resolution land surface temperature mosaics for thermophysical mapping of the Mojave desert. Sensors 19:2669. doi: 10/gf327x
Nussear, K. E., Tracy, C. R., Medica, P. A., Wilson, D. S., Marlow, R. W., and Corn, P. S. (2012). Translocation as a conservation tool for Agassiz’s desert tortoises: survivorship, reproduction, and movements. J. Wildl. Manag. 76, 1341–1353. doi: 10/f3766q
Ouren, D. S., Haas, C., Melcer, C. P., Stewart, S. C., Ponds, P. D., Sexton, N. R., et al. (2007). Environmental effects of off-highway vehicles on Bureau of Land Management lands: A literature synthesis, annotated bibliographies, extensive bibliographies, and internet resources. U.S. Department of the Interior, U.S. Geological Survey.
Patterson, T. A., Basson, M., Bravington, M. V., and Gunn, J. S. (2009). Classifying movement behaviour in relation to environmental conditions using hidden Markov models. J. Anim. Ecol. 78, 1113–1123. doi: 10/c3244t
Peaden, J. M., Justin Nowakowski, A., Tuberville, T. D., Buhlmann, K. A., and Todd, B. D. (2017). Effects of roads and roadside fencing on movements, space use, and carapace temperatures of a threatened tortoise. Biol. Conserv. 214, 13–22. doi: 10/gcgg4q
Picardi, S., Coates, P., Kolar, J., O’Neil, S., Mathews, S., and Dahlgren, D. (2022). Behavioural state-dependent habitat selection and implications for animal translocations. J. Appl. Ecol. 59, 624–635. doi: 10/gphbvw
Pohle, J., Langrock, R., van Beest, F. M., and Schmidt, N. M. (2017). Selecting the number of states in hidden Markov models: pragmatic solutions illustrated using animal movement. JABES 22, 270–293. doi: 10/gdnd44
Public Employees for Environmental Responsibility (2022). Mapping rangeland health. Mapping rangeland health. Available at: https://peer.org/areas-of-work/public-lands/grazing-reform/mapping-rangeland-health/. Accessed March 17, 2022.
R Core Team (2018). R: A Language and Environment for Statistical Computing. Vienna, Austria: R Foundation for Statistical Computing.
Rautenstrauch, K. R., Brown, G. A., and Lederle, P. E. (2002). Patterns of burrow use by desert tortoises (Gopherus agassizii) in southcentral Nevada. Chelonian Conserv. Biol. 4, 398–405.
Rautsaw, R. M., Martin, S. A., Vincent, B. A., Lanctot, K., Bolt, M. R., Seigel, R. A., et al. (2018). Stopped dead in their tracks: the impact of railways on gopher tortoise (Gopherus polyphemus) movement and behavior. Copeia 106, 135–143. doi: 10.1643/CE-17-635
Rigge, M., Homer, C., Shi, H., Meyer, D., Bunde, B., Granneman, B., et al. (2021). Rangeland fractional components across the western United States from 1985 to 2018. Remote Sens. 13:813. doi: 10.3390/rs13040813
Ruby, D. E., and Niblick, H. A. (1994). A behavioral inventory of the desert tortoise: development of an ethogram. Herpetol. Monogr. 8:88. doi: 10/fd5vcz
Ruby, D. E., Spotila, J. R., Martin, S. K., and Kemp, S. J. (1994a). Behavioral responses to barriers by desert tortoises: implications for wildlife management. Herpetol. Monogr. 8:144. doi: 10/b72xw5
Ruby, D. E., Zimmerman, L. C., Bulova, S. J., Salice, C. J., O’Connor, M. P., and Spotila, J. R. (1994b). Behavioral responses and time allocation differences in desert tortoises exposed to environmental stress in semi-natural enclosures. Herpetol. Monogr. 8:27. doi: 10.2307/1467068
Sadoti, G., Gray, M. E., Farnsworth, M. L., and Dickson, B. G. (2017). Discriminating patterns and drivers of multiscale movement in herpetofauna: the dynamic and changing environment of the Mojave desert tortoise. Ecol. Evol. 7, 7010–7022. doi: 10.1002/ece3.3235
Sharifi, M. R., Gibson, A. C., and Rundel, P. W. (1997). Surface dust impacts on gas exchange in Mojave desert shrubs. J. Appl. Ecol. 34:837. doi: 10.2307/2405275
Snyder, S. J., Tracy, C. R., and Nussear, K. E. (2019). Modeling operative temperature in desert tortoises and other reptiles: effects imposed by habitats that filter incident radiation. J. Therm. Biol. 85:102414. doi: 10.1016/j.jtherbio.2019.102414
Spiegel, O., Leu, S. T., Bull, C. M., and Sih, A. (2017). What’s your move? Movement as a link between personality and spatial dynamics in animal populations. Ecol. Lett. 20, 3–18. doi: 10.1111/ele.12708
Switalski, A. (2018). Off-highway vehicle recreation in drylands: a literature review and recommendations for best management practices. J. Outdoor Recreat. Tour. 21, 87–96. doi: 10.1016/j.jort.2018.01.001
Tuma, M. W., Millington, C., Schumaker, N., and Burnett, P. (2016). Modeling Agassiz’s desert tortoise population response to anthropogenic stressors. J. Wild. Mgmt. 80, 414–429. doi: 10.1002/jwmg.1044
Turner, R. M. (1994). “Mohave desertscrub” in Biotic Communities of the American Southwest-United States and Mexico (Salt Lake City, UT: University of Utah Press), 157–168.
U. S. Fish and Wildlife Service (1994). Desert Tortoise (Mojave population) Recovery Plan. U. S. Fish and Wildlife Service: Oregon.
U. S. Fish and Wildlife Service (2011). Revised Recovery Plan for the Mojave Population of the Desert Tortoise (Gopherus agassizii).
U.S. Geological Survey (2017). 1/3rd Arc-second Digital Elevation Models (DEMs)–USGS National Map 3DEP.
United States Census Bureau (2021). 2021 TIGER/line shapefiles technical documentation. Available at: https://www2.census.gov/geo/pdfs/maps-data/data/tiger/tgrshp2021/TGRSHP2021_TechDoc.pdf
Vaughn, M., Berry, K., McLuckie, A., Allison, L., and Murphy, R. (2020). IUCN red list of threatened species: Gopherus agassizii. Available at: https://www.iucnredlist.org/species/97246272/3150871. Accessed September 27, 2021.
von Seckendorff Hoff, K., and Marlow, R. W. (2002). Impacts of vehicle road traffic on desert tortoise populations with consideration of conservation of tortoise habitat in southern Nevada. Chelonian Conserv. Biol. 4, 449–456.
Webb, R. H., and Stielstra, S. S. (1979). Sheep grazing effects on Mojave desert vegetation and soils. Environ. Manag. 3, 517–529. doi: 10/bgmdcq
Woodbury, A. M., and Hardy, R. (1948). Studies of the desert tortoise, Gopherus agassizii. Ecol. Monogr. 18, 145–200. doi: 10.2307/1948638
Zeller, K. A., McGarigal, K., and Whiteley, A. R. (2012). Estimating landscape resistance to movement: a review. Landsc. Ecol. 27, 777–797. doi: 10/f326fn
Keywords: roads, fencing, hidden Markov movement model, off-highway recreation, livestock grazing, wildfire
Citation: Hromada SJ, Esque TC, Vandergast AG, Drake KK, Chen F, Gottsacker B, Swart J and Nussear KE (2023) Linear and landscape disturbances alter Mojave desert tortoise movement behavior. Front. Ecol. Evol. 11:971337. doi: 10.3389/fevo.2023.971337
Edited by:
Silviu O. Petrovan, University of Cambridge, United KingdomReviewed by:
Christina Aiello, Oregon State University, United StatesInger Suzanne Prange, Appalachian Wildlife Research Institute (United States), United States
Javan Bauder, Arizona Cooperative Fish and Wildlife Research Unit, United States
Giancarlo Sadoti, Independent Researcher, Santa Fe, United States
Copyright © 2023 Hromada, Esque, Vandergast, Drake, Chen, Gottsacker, Swart and Nussear. This is an open-access article distributed under the terms of the Creative Commons Attribution License (CC BY). The use, distribution or reproduction in other forums is permitted, provided the original author(s) and the copyright owner(s) are credited and that the original publication in this journal is cited, in accordance with accepted academic practice. No use, distribution or reproduction is permitted which does not comply with these terms.
*Correspondence: Steven J. Hromada, c3RldmVocm9tYWRhQGdtYWlsLmNvbQ==
†Present address: Steven J. Hromada,Conservation Science Partners, Inc, Truckee, CA, United States