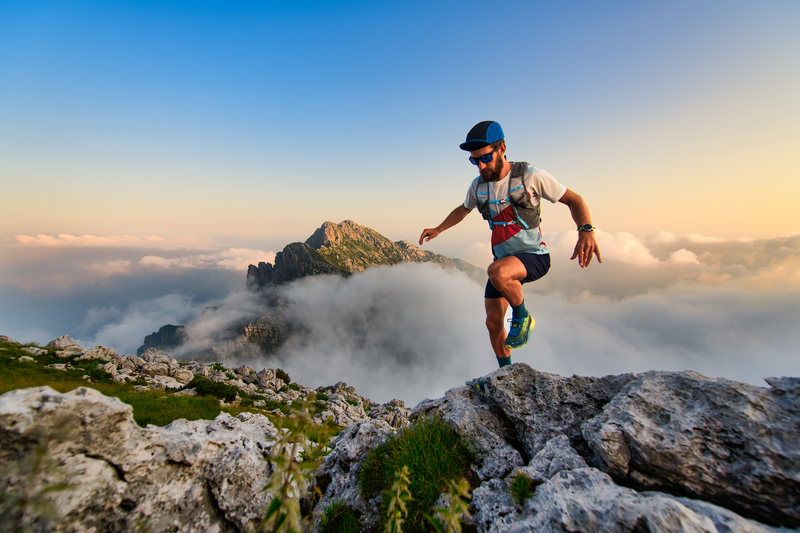
95% of researchers rate our articles as excellent or good
Learn more about the work of our research integrity team to safeguard the quality of each article we publish.
Find out more
ORIGINAL RESEARCH article
Front. Ecol. Evol. , 11 December 2023
Sec. Interdisciplinary Climate Studies
Volume 11 - 2023 | https://doi.org/10.3389/fevo.2023.1307756
Introduction: Studies have shown that green spaces and water bodies can alleviate heat island effects. However, uncertainty remains regarding the characteristics and influence of Green Stormwater Infrastructures (GSIs) on the cooling effects under different weather conditions. To address this issue, a comparative study was conducted between the green spaces in a wetland park with GSIs and a general green space without GSIs.
Methods: In this study, atmospheric temperatures were collected from both green spaces using mobile measurements to compare the cold island effect. In addition, the precise characteristics of the surface temperatures of the underlying surfaces in the wetland park were explored using an Unmanned Aerial Vehicle (UAV).
Results: The results revealed that green spaces with GSIs had a stronger cooling effect on the surrounding thermal environment than green spaces without GSIs, in most cases. The heat fluxes of different types of underlying surfaces in green spaces with different GSIs varied at different time periods. During the daytime, permeable pavement and some grasslands had a warming effect. The cooling effect of the other underlying surfaces was in the order of water bodies>arbors>shrubs>grasslands. At night, the changes in heat flux were lower, and only the arbors showed cooling due to evapotranspiration.
Discussion: These findings may provide innovative ideas and methods for planning GSIs to mitigate the urban heat island effects.
Extreme urbanization has had a dramatic influence on urban surfaces (Ucal and Xydis, 2020), and changes in surface materials have led to changes in the urban thermal environment and disrupted existing hydrological processes (Chew et al., 2021; Yang et al., 2022). Superimposed on extreme rainfall events and heatwaves caused by climate change, cities have suffered serious ecological consequences such as urban flooding (Feng et al., 2021) and the heat island effect (Chapman et al., 2017; Yan Q. et al., 2020), which have significantly affected the well-being of inhabitants. Therefore, it is crucial to develop a rational plan for an urban green stormwater infrastructure to alleviate urban ecological problems.
Previous studies have shown that urban blue-green spaces have favorable ecological benefits (Shi et al., 2020; Yu et al., 2020). Because it is a permeable underlying surface, it can effectively mitigate urban stormwater problems (Nguyen et al., 2019). Simultaneously, plants in green spaces can effectively mitigate urban heat through shading and transpiration (Yan C. et al., 2020; Tan et al., 2021). Numerous studies have shown that green stormwater infrastructure (GSI), as a composite of green spaces and water bodies, can play an important role in urban stormwater infiltration, delaying flood peaks, and replenishing groundwater (Eckart et al., 2017; Steis Thorsby et al., 2020; Yang et al., 2021). Owing to the coupled characteristics of vegetation and water bodies, their internal and external energy exchanges are more frequent and stronger than those of a single green space, and they exhibit stronger ecological edge effects (Du et al., 2019). However, it is unclear whether GSIs have a stronger cooling effect than individual green spaces. Most existing studies have focused on the ideal conditions of sunny days (Wu and Chen, 2017; Spahr et al., 2020; Li et al., 2023). With global climate change and the frequent occurrence of extreme rainfall events (Ohba and Sugimoto, 2019; Moustakis et al., 2021), there is an urgent need to conduct studies on the characteristics of the cooling island effect of GSIs under different weather conditions, such as rainy and cloudy days, by comparing them with general green spaces without GSIs, thus providing new insights into improving the problems of urban waterlogging and thermal environments.
Evapotranspiration, is one of determining factors in process of hydrologic cycle in regional watersheds, and is the factor that has the greatest impact on surface runoff other than rainfall (Zhao et al., 2020). In the context of energy balance, the strong evapotranspiration of the GSI affects urban hydrological and ecological processes, causing a decrease in sensible heat fluxes and an increase in latent heat fluxes to improve the urban thermal environment (Singh et al., 2020; Angelini et al., 2021). However, existing research on the comprehensive ecological effects of GSIs focuses on the study of their stormwater control capacity (Eckart et al., 2018; Taghizadeh and Khani, 2021). Research on the mitigation of the heat island effect remains scarce, and most of them are qualitative studies that lack accurate and detailed quantitative studies (He et al., 2019). In quantitative studies, on-site monitoring or numerical simulations of individual facilities, such as green roofs (Sanchez and Reames, 2019; Iaria and Susca, 2022), permeable pavements (Wang et al., 2019), and rain gardens (Zhao et al., 2022), have been conducted. There is a lack of research on the overall cooling effect of GSIs.
In addition, studies have found that the areas of green spaces and water bodies are not linearly related to their cooling effect; there is a certain threshold beyond which the cooling effect dramatically weakens (Yan C. et al., 2020). When the area of the blue-green space was too large, it was difficult for heat from the surrounding environment to reach the center of the site. Therefore, small- and medium-scale green infrastructures can fully perform the functions of vegetation and water to alleviate the thermal environment (Oliveira et al., 2011). Nevertheless, most existing studies based on remote sensing images have been conducted at medium or large scales to study the cooling effect of the entire urban green space system, which is of macroscopic guidance significance (Peng et al., 2021; Qian and Li, 2023; Yu et al., 2020), and there are still few studies on the cooling effect of small-scale green spaces or water bodies at neighboring scales (Wang et al., 2021).
To address the research gap on the cold island effect of small-scale GSI under different weather conditions, a green space in Nanjing Yinchun Road Wetland Park and a general green space without GSIs in its vicinity were selected for a comparative study under different weather conditions. By obtaining atmospheric temperatures from field measurements and thermal infrared images from UAVs, this study quantified the different cooling effects and characteristics of the GSI in summer. The objectives of this study were: (1) to analyze the characteristics of daily changes in atmospheric temperatures of green spaces with GSI compared to general green spaces under different weather conditions; (2) to analyze the effect of GSI application on temperature; and (3) to determine the characteristics of heat fluxes and cooling effects of the underlying surfaces of green spaces with GSIs. The findings of this study provide relevant guidelines for local GSI planning regarding the benefits of GSIs in mitigating the urban heat island effects.
Nanjing (31°14’–32°36’N, 118°22’–119°14’E), the capital of Jiangsu Province, is one of the large metropolitan areas in eastern China. As of 2022, the permanent urban population of Nanjing has reached 8.258 million, with an urbanization rate of 87.1% and a population density of 1,430 people per square kilometer. Nanjing has a northern subtropical monsoon climate with abundant rainfall, four distinct seasons, and a significant temperature difference between the day and night. With the expanding scale of urban construction and intensive socioeconomic activities in Nanjing’s main districts, the urban thermal environment problem is becoming increasingly significant. The implementation of blue-green infrastructure is crucial to alleviate the urban heat island effect and improve the urban environment. For this study, the Yinchun Road Wetland Park and the green space adjacent to the Qixia Bridge in Nanjing’s central district were selected as research areas.
As shown in Figure 1, the Yinchun Road Wetland Park is a provincial sponge city construction pilot project that was completed in November 2018. As an initial exploratory project for sponge city construction in Nanjing, it serves as an important demonstration and guide and is an instrumental part of the city’s adaptive planning. The park covers a total area of approximately 10.5 hm2, of which around 4.1 hectares dedicated to transformation. The park includes water-wet ponds, grass-planting ditches, and rain gardens. Through numerous model validations and years of field monitoring, the park demonstrated effective stormwater management capabilities. Furthermore, the construction of GSIs significantly improved the cooling effect of the park’s surrounding environment. The green space attached to the Qixia Bridge is separated from the wetland park by Yinchun Road, and the area has a certain slope, which has high potential for constructing GSIs. The green space is separated into two sections by the Qixia Bridge, north and south, each with an area of approximately 1 hm2. The eastern side of the green space near Yinchun Road had a higher amount of three dimensional greening, whereas the western side was dominated by grasslands.
Wireless temperature sensors were used to conduct dynamic measurements of green spaces throughout the day. These sensors rely on cell phone signals to transmit data suitable for field monitoring. Multiple sensors were placed at fixed points, atmospheric temperature measurements were obtained every 10 minutes, and the data were uploaded to the server platform immediately after the measurements.
Onsite atmospheric temperature measurements were conducted between June 12 and July 16, 2021. Considering the significant impact of the overpass on the surrounding thermal environment, the measurement site was divided into two sections, with Qixia Avenue serving as the boundary for comparison and analysis. Figure 2 illustrates that the water bodies on the south side of the Yinchun Road Wetland Park (sequence A) primarily consist of blocky wet ponds, whereas the water body on the north side (sequence B) is an east–west-oriented river. The disparity between the northern and southern sides makes this comparison meaningful. Starting from the boundary of the green space, six sensors were placed at equal intervals of 30 m on the waterside of the path, maintaining a distance of 2 m from the path. This arrangement weakens the influence of different environments on atmospheric temperature. The greenspace attached to the Qixia Bridge was divided into several separate greenspaces using ramps. On both the north and south sides of Qixia Avenue (south side: C sequence; north side: D sequence), sensors were positioned every 30 m towards the west, ensuring that at least one sensor was present in each green space, separated by a ramp. In addition, a sensor was installed at the bottom of the viaduct (sequence E) to obtain the temperature in the region without direct sunlight for comparative analysis.
Data measured under different weather conditions were used to compare the atmospheric temperatures of the two green spaces. Considering the influence of rain on temperature, the average values for June 24 and July 14, 2021, which were sunny for three consecutive days, were selected for sampling on sunny days. The averages of June 16 and July 8, 2021, which had the longest rainfall periods, were selected for the rainy day sample. The atmospheric temperature was collected at two-hour intervals starting from 00:00 on the measurement date.
Using Landsat8 and other satellite images to obtain surface temperatures has some problems, such as low image resolution and short shooting times. Currently, the thermal infrared technology of unmanned aerial vehicles (UAVs) has been widely used in all types of measurement research, and the accuracy of surface temperature measurement has been affirmed by all circles (Sagan et al., 2019).
This study used the DJI Mavic 2 Advanced Unmanned Aerial Vehicle (UAV) to conduct regular flights every two hours from June 20 to July 20, 2021, to obtain thermal infrared images. Subsequently, the Pix4Dmapper was used to concatenate the images, as shown in Figure 3. The surface temperatures at the sites were obtained from these images. In this study, when measuring the surface temperature of the surrounding environment of the GSIs, five sample areas that were equidistant from the site were selected using the box selection method, and the average temperature in the sample area was taken as the surface temperature of the underlying surface during the measurement to reduce the error caused by a few samples.
The intensity of the cold island effect is generally expressed by its magnitude, distance, and cooling rate. This study compared the intensity of the cold island effect using the cooling magnitude, which refers to the temperature difference between different green spaces at the same time and is a relative value that is generally calculated by selecting the higher temperature measurement point as the reference value (Huang et al., 2008). The research selected the average value of points A1 and B1 adjacent to the road in the two green spaces as the reference value and took the difference between it and other atmospheric temperatures as the cooling magnitude, which was calculated by Equation 1:
where Tu represents the average of the atmospheric temperature at point A1 and B1, and Tp is the atmospheric temperature measured by each of the other atmospheric thermometers. A positive value of ΔT indicates that the surrounding environment represented by this point produces a cold island effect, the larger the value, the stronger the cold island effect; conversely, it produces a heat island effect, the larger the value, the stronger the heat island effect.
In this study, the surface temperature obtained from the UAV thermal infrared image and the atmospheric temperature and wind speed obtained by the wireless sensor were used to calculate the heat flux of each underlying surface, and the impact of various underlying surface types on the thermal environment was analyzed qualitatively and quantitatively according to the heat flux value.
Heat flux is the heat energy through a unit area per unit time, which reflects the effect of the wind environment on the temperature of matter. In the process of heating or cooling, the heat flux absorbed or released by substances whose temperature increases or decreases without changing their original state is the sensible heat flux, and the heat flux absorbed or released by substances whose temperature and state do not change is the latent heat flux. Generally, the larger the sensible heat flux, the higher is the atmospheric temperature; the larger the latent heat flux, the lower is the atmospheric temperature. The stronger the atmospheric turbulence, the more heat flux was exchanged. The heat flux was calculated by Equation 2 (Qin and Hiller, 2014):
where H is the sensible heat release, Ts is the surface temperature, Ta is the air temperature, and hc is the air turbulence coefficient, which can be obtained using Equation 3:
where v is the wind speed at a height of 9 m above ground level. The height of the wind speed sensor used in this study was 1.5 m. To obtain the wind speed at 9 m, the wind speed profile was used to convert wind speed as shown in Equation 4:
where u is the average wind speed at a known height z1, u1 is the average wind speed at the calculated height z, and m is the stability parameter, which refers to the degree of atmospheric stability. In the actual measurements, the atmospheric stability was neutral and the value of m under neutral conditions was 0.25.
Figure 4A illustrates the daily variation of atmospheric temperature in the two green spaces under different weather conditions. The absolute values of temperature changes followed the pattern of sunny day>cloudy day>rainy day. Both green spaces showed a positive correlation between atmospheric temperature and solar radiation intensity. However, the time required to reach the highest temperature differed between the two groups. The general green space without GSIs reached its peak at approximately 14:00, whereas the green space with GSIs, which had water bodies with a high heat capacity, continued to rise until approximately 16:00. Throughout the day, both green spaces experienced a sharp drop in temperature after reaching a peak, which gradually slowed until approximately 20:00. Overall, the green space with GSIs exhibited better cooling effects, except for a brief period from 08:00 to 10:00, when it heated faster than the general green space.
Figure 4 Daily variation of atmospheric temperature under three weather conditions: (A) sunny day; (B) cloudy day; (C) rainy day.
Under cloudy conditions, as shown in Figure 4B, the atmospheric temperature variation was similar to that on sunny days, but with a slower temperature increase from 10:00 to 15:00 owing to weak solar radiation. The temperature range on cloudy days (20.9°C–37.6°C) was much lower compared to sunny days (26.7°C–42.5°C). General green spaces without GSIs had higher temperatures than green spaces with GSIs on cloudy days, and wind speed had a greater influence on temperature. In the morning and evening, regions C and D of the general green space had similar temperatures, but region D on the north side had slightly lower temperatures, owing to the north wind. Region E, located at the bottom of the overpass, consistently had lower temperatures during the daytime, indicating a significant influence of solar radiation.
On representative rainy days, as shown in Figure 4C, heavy rainfall between 09:00 and 12:00 reduced the maximum temperature compared with sunny and cloudy days. As shown in Table 1, the minimum temperature on rainy days was higher than that on sunny and cloudy days, likely because of the absorption of surface energy by rainfall and increased surface runoff. Overall, the atmospheric temperature in ordinary green spaces was higher than that in green spaces with GSIs. However, the temperature differences between the regions within the green spaces decreased after rainfall. Region E consistently had similar temperature patterns to cloudy days and became the warmest region after 08:00 due to the influence of rain.
Considering the impact of the Qixia Bridge on the thermal environment, when comparing the green space with GSI and the general green space, regions A and C on the south side of the bridge and regions B and D on the north side were compared. This study used the maximum daytime and average nighttime temperatures to calculate the intensity of the cold island effect under different weather conditions.
Figure 5A shows that green spaces with GSIs had a better cooling effect than general green spaces on sunny days. During the daytime, the cooling range of Sequence A was 0.20–0.90°C, higher than that of sequence C, 0.07–0.30°C. At night, there was a heat island effect in both sequences. The warming amplitude of sequence A was 0.23–0.92°C, which was lower than 0.68–1.15°C of sequence C, indicating that the warming effect of general green space was higher than that of green space with GSIs on sunny nights.
Figure 5 Cooling range of cold island effect in each sensor area: (A) sunny conditions; (B) cloudy conditions; (C) rainy conditions.
The different vegetation structures in the four regions had varying effects on the thermal environment. During the daytime, region B showed a cold island effect except for point B1, with a cooling range of 0.09–2.7°C, which was significantly higher than region A. This is because of the presence of more water bodies and trees in region B that can transpire to cool temperatures. Unlike region C, region D exhibits a cooling effect at points D1 and D2, whereas points D3 and D4 exhibit warming effects. Point D1 had a sparse forest grassland with fewer trees and only a 0.97°C cooling range, while the structural integrity of trees, shrubs and grasses at point D2, resulted in a cooling range of 1.85°C. Points D3 and D4, which were closer to the residential area, experienced temperature increases of 0.59°C and 1.17°C respectively compared to B1, likely influenced by the thermal environment of the nearby residential area. At night, a heat island effect was observed in all four regions. The heating effect range in region B was the lowest, ranging from 0.15 to 0.25°C.
Under cloudy conditions, as shown in Figure 5B, with weak solar radiation and less temperature fluctuation than sunny days, the green space with GSIs still exhibited a cooling effect during the daytime, with a cooling range of 0.2–1.3°C in region A. In contrast, the ordinary green spaces showed a warming effect at each point in Region C, ranging from 0.10–0.75°C. At night, region A had a slight warming effect range of 0.05–0.18°C, while region C experienced a cooling effect range of 0.10–0.48°C. Green spaces with GSIs maintained the cold island effect, whereas ordinary green spaces showed the opposite trend, warming during the day and cooling at night, which is consistent with the findings of Doick et al. (2014), Lu et al. (2017) and Pan et al. (2023). This can be attributed to the strong influence of weaker solar radiation, strong winds, and atmospheric turbulence under cloudy conditions.
The absolute value of the heat flux was small on cloudy days, and the surrounding environment had a stronger correlation with atmospheric temperature. Region B showed similar patterns as region A, both of which experienced cooling effects. However, the cooling range in region B (0.3–1.6°C) was lower than the maximum cooling range under sunny conditions (2.7°C), indicating that the intensity of the cold island effect in the green space with GSIs was directly proportional to solar radiation intensity. The night-time temperature fluctuation between the measurement points in Region B was smaller. Region D generally exhibited higher temperatures, with heat island effects at points D3 and D4, showing warming amplitudes of 1.65°C and 1.8°C respectively, stronger than the warming amplitudes under sunny conditions. Points D1 and D2 had cooling effects, with the cooling range at D1 point being higher than that at point D2, contrary to that under sunny conditions. This may be because region D was affected by its proximity to a residential area with a severe thermal environment.
Figure 5C shows that the intensity of the cold island effect on rainy days was significantly different from those on sunny and cloudy days. Green spaces with GSIs have a warming effect, whereas ordinary green spaces still have a cooling effect. In the daytime, region C had a cooling range of 0.2–1.9°C, while region A had a warming amplitude of 0.9–1.5°C. This may be because green spaces with GSIs intercept more surface runoff during rainfall, collecting heat from the high-temperature surface, which increases vegetation and soil temperature, resulting in a heat island effect. However, rapid infiltration and convergence of rainwater in ordinary green spaces reduces the temperature of the surrounding environment. Point C1, surrounded by sparse forests and grasslands, had a smaller cooling range of 0.2°C compared to points C2–C4 with more trees. The presence of many roads around C1 also contributes to its smaller cooling range. Both green spaces experienced a heat island effect in most areas at night, similar to sunny and cloudy days, but the increased nighttime temperature due to rainfall was small, below 0.5°C, with a maximum of only 0.7°C. Point C4 had a temperature drop of 0.1°C, but the range was small, possibly due to atmospheric turbulence caused by strong winds on rainy days.
The temperature trend in Sequence B was similar to that in Sequence A. During the day, sequence B had a warming amplitude of 0.5-1.6°C, and at night, it had a heat island effect of about 0.5°C. The smaller difference may be attributed to differences in the water volume and living vegetation volume in each region. During the daytime, region D showed the opposite trend to region C, with higher atmospheric temperatures at points D2, D3, and D4, which had higher living vegetation volumes than point D1. This phenomenon may still be influenced by the proximity of Region D to residential areas. The temperature trend in sequence D at night was similar to that of sequence C, but irregular owing to the influence of gale weather.
Based on the current situation, the site is divided into five types of underlying surfaces: arbors, shrubs, grasslands, water bodies, and permeable pavements. Combined with the surface temperature obtained from the UAV thermal infrared image and the atmospheric temperature and wind speed obtained by the wireless sensors, the heat fluxes of each underlying surface were calculated. Based on the nature and magnitude of the heat flux, the influence of various types of underlying surfaces on the surrounding environment was analyzed qualitatively and quantitatively.
According to the results presented in Section 3.1, the heat and cold island effects of both green spaces under sunny conditions were stronger than those on cloudy days, and the green space with GSIs showed the highest temperature at 16:00. As shown in Figure 6, the data measured at 16:00 were selected as representative for calculating and analyzing the daytime heat flux.
Permeable pavements have sensible heat flux in both regions, resulting in an increase in temperature. This effect varies, depending on the pavement material used. Marble had a lower sensible heat flux (115.69 W/m³) compared to permeable plastic (524.82–424.99 W/m³). This suggests that the marble has a weaker heating effect under sunny conditions. In region B, where the pavements were made of permeable plastic, the sensible heat flux ranged from 360.22–417.02 W/m³, contributing to warming. The lack of a linear relationship in region B may be due to differences in the permeability efficiency and the surrounding environment.
Grasslands in region A had a small absolute value of the sensible heat flux (12.10–47.33 W/m³), while grasslands in region B had a cooling effect with the latent heat flux of 10.47–21.90 W/m³. According to the investigation, the survival condition of the grasslands in Region B was better; therefore, they could absorb heat more effectively. This suggests that the cooling effect of grasslands is significantly influenced by their growth status.
Water bodies were the most effective in cooling both regions, with a strong correlation between cooling effect and water volume. The latent heat flux of water bodies in region A ranged from 283.97–199.83 W/m³, decreasing with decreasing water area. In region B, the latent heat flux ranged from 339.14–457.51 W/m³ and did not show a linear correlation. This could be attributed to the differences in river width and flow rate between the two regions, with flowing water bodies having a stronger cooling effect than static water bodies.
Both arbors and shrubs had a cooling effect, which was determined by the volume of the living vegetation. The latent heat flux of arbors in region A ranged from 68.36–220.87 W/m³, while shrubs had a latent heat flux of 82.14–120.95 W/m³. However, the heat flux of the shrubs at points A4 and A5 was higher, possibly because only sparse trees were planted near the points in their vicinity, which were greatly affected by the surrounding environment. In region B, owing to the limited planting of shrubs for slope protection, arbors were planted between the river and the path, and there were few shrubs for slope protection. Therefore, the latent heat flux of arbors in region B was always higher than that of shrubs, at 291.86 W/m³–210.88 W/m³. The intensity of the cooling effect at other points gradually increased.
During the nighttime, the average values at 18:00, 20:00, and 22:00 were selected to calculate the heat flux of each underlying surface. As shown in Figure 7, there were certain differences in heat flux between different underlying surfaces, but the maximum absolute value of heat flux at night was only 150 W/m³, with a gentler change compared to daytime.
Permeable pavements had a warming effect at night but with a significantly lower amplitude. The sensible heat flux ranged from 36.46–95.71 W/m³, much lower than daytime values. The different permeable pavement materials exhibited similar heat fluxes, possibly owing to equipment limitations or the lower impact of permeable pavements at night.
Grasslands had a warming effect at night, with a lower sensible heat flux compared to daytime. In Region A, the sensible heat flux ranged from 2.08–7.56 W/m³. In region B, the grassland changes into a heat island effect due to the influence of the surrounding environment, with a sensible heat flux of 2.32–7.14 W/m³.
Water bodies, which had the strongest cooling effect during the day, exhibited warming effects at night. The sensible heat flux ranged from 36.46–82.04 W/m³ in region A and 77.48–95.71 W/m³ in region B. The latent heat flux in Region A showed an increasing trend with decreasing water area, in contrast to the daytime trends. The heat flux in region B is not linearly related to the distance from the boundary, possibly because of the influence of the surrounding environment.
Arbors continue to absorb heat from the surrounding environment through evaporation at night, resulting in a unique cooling effect. The latent heat flux ranged from 18.23–54.69 W/m³ in region A and 36.16–123.05 W/m³ in region B, lower than daytime values. The reduction in latent heat flux in region A was related to the decrease vegetation volume, while the increasing latent heat flux in region B indicated compared with the boundary of green space, the center of green space is more likely to form a center of cold island or a heat island.
In shrubs with lower vegetation volume and evapotranspiration at night, the stomata of plants were mostly closed and had the strongest warming effect. The sensible heat flux ranged from 118.50–164.07 W/m³ in A and 132.17–154.96 W/m³ in region B. The sensible heat flux increased with the number of shrubs and distance from the boundary in both regions.
Previous studies have primarily focused on the cold island effect in water bodies or green spaces (Liu et al., 2021), neglecting the investigation of blue-green composite spaces, such as GSIs. Furthermore, studies on GSIs have mainly concentrated on stormwater management and rainwater purification (Baek et al., 2020; Zhao et al., 2023), with limited attention given to the mitigation of the urban heat island effect. However, this study confirms that green spaces with GSIs generally exhibit a stronger cooling effect than ordinary green spaces, supporting the notion that a combination of blue and green spaces is more likely to create the center of a cold island (Le Phuc et al., 2022). This phenomenon, known as the “synergistic cold island effect,” has been observed in previous studies comparing the cooling effects of urban blue-green spaces in various locations. For example, a comparative assessment of Chongqing (Li and Chuck Wah, 2014) found that the comprehensive effect of blue-green spaces surpassed that of lakes and regular parks without water bodies. Similarly, Aboelata et al. (2021); Nasir et al. (2021) and Kridakorn Na Ayutthaya et al. (2023) found that GSIs, such as green roofs, bioretention facilities, and permeable pavements, can significantly alleviate the urban heat island effect while ensuring urban flood management.
In contrast to previous research that relied on a limited number of temperature-measuring instruments to capture temperatures at a few points at different sites, this research employed multiple wireless temperature sensors to continuously monitor atmospheric temperatures at various nodes within the site over a 24-hour period. This approach can be used to effectively investigate the effect of the distance to the boundary of a green space on the thermal environment of the site. Furthermore, this study employed thermal infrared images captured by a UAV to obtain surface temperatures. This method offers several advantages over current research on the cold island effect in blue-green spaces, which primarily relies on remote sensing images for temperature inversion (Sun et al., 2020) and microclimate software for temperature simulation (Lin and Lin, 2016). The UAV thermal infrared imaging provides high accuracy, flexibility in shooting time, and applicability under different weather conditions (Feng et al., 2022). Moreover, this method allows the collection of surface temperatures from various types of underlying surfaces, providing a more detailed exploration of the cold island effect characteristics of GSIs compared to the limited accuracy of subsurface temperatures obtained from satellite images at a resolution of 100 m×100 m.
The presence of Green Stormwater Infrastructure (GSIs) enhances the cooling effect of green spaces compared to general green spaces without GSIs. By optimizing the elements and spatial layout of general green spaces, the thermal environments of green spaces and their surroundings can be significantly improved. Additionally, this study highlighted the specific characteristics of the cold island effect in green spaces with GSIs, which can help maximize their ecological service functions and overall benefits.
However, this study had some limitations. The equipment and experimental conditions were limited, resulting in the comparison and analysis of only two types of green spaces. The selected samples were relatively narrow in scope with fewer types of underlying surfaces in green spaces with GSIs, and the coverage of seasons and weather types was not sufficiently comprehensive. Therefore, the findings of this study cannot guide the construction of large-scale green spaces using GSIs.
Future field research should focus on diversifying research objects and scales (Du et al., 2017). For example, measurements should be conducted in green spaces with GSIs of different scales and types. The number and duration of measurements should be increased, including more measurements in spring, autumn, and winter, and monitoring before and after different rainfall scenarios. Considering the various factors that influence the cold island effect, microclimate simulation software can be utilized to compare and analyze different planning and design schemes, with the goal of maximizing the cold island effect per unit area.
This study compared the cold island effect of green spaces with GSIs and general green spaces without GSIs under different weather conditions and found that the impact of green spaces with GSIs on the surrounding thermal environment was always higher. The atmospheric temperatures of the two green spaces varied greatly depending on the weather conditions. Green spaces with GSIs had a stronger cold island effect than ordinary green spaces on both sunny and cloudy days. At night, both green spaces exhibited a heat island effect. On rainy days, ordinary green spaces exhibit the cold island effect in the daytime, whereas green spaces with GSIs exhibit the heat island effect due to the warming effect of surface runoff.
A study on the characteristics of the cold island effect in green spaces with GSI showed that different types of underlying surfaces have different heat fluxes and mitigation effects on the heat island effect. During the daytime, permeable pavements and parts of grasslands have a warming effect on the surrounding environment, whereas the other types have a cooling effect. The water body is the type of underlying surface with the best cooling effect, and the cooling effect of the other types of underlying surfaces is arbors>shrubs>grasslands. At night, the absolute value of the heat flux change was smaller than that during the daytime. Except for arbors with strong evapotranspiration, which still have a cooling effect, other types of underlying surfaces have a warming effect. The overall warming effect followed the order shrubs>water bodies>permeable pavement>grasslands.
The raw data supporting the conclusions of this article will be made available by the authors, without undue reservation.
HX: Conceptualization, Funding acquisition, Supervision, Writing – review & editing. KS: Formal analysis, Validation, Visualization, Writing – original draft, Writing – review & editing. JG: Conceptualization, Data curation, Formal analysis, Software, Writing – original draft.
The author(s) declare financial support was received for the research, authorship, and/or publication of this article. This work was supported by Humanities and Social Science Fund of Ministry of Education of the People’s Republic of China (No. 20YJCZH190),China Scholarship Council (No. 201808320046) and the Priority Academic Program Development of Jiangsu Higher Education Institutions (PAPD).
The authors declare that the research was conducted in the absence of any commercial or financial relationships that could be construed as a potential conflict of interest.
All claims expressed in this article are solely those of the authors and do not necessarily represent those of their affiliated organizations, or those of the publisher, the editors and the reviewers. Any product that may be evaluated in this article, or claim that may be made by its manufacturer, is not guaranteed or endorsed by the publisher.
Aboelata A., Lund H., Kaiser M. J. (2021). Assessment of green roof benefits on buildings’ energy-saving by cooling outdoor spaces in different urban densities in arid cities. Energy 219, S0360544220326219. doi: 10.1016/j.energy.2020.119514
Angelini L. P., Sacardi Biudes M., Gomes MaChado N., M. E. Geli H., Louis Vourlitis G., Ruhoff A., et al. (2021). Surface albedo and temperature models for surface energy balance fluxes and evapotranspiration using SEBAL and landsat 8 over cerrado-pantanal, Brazil. SENSORS 21 (21), 7196. doi: 10.3390/s21217196
Baek S. S., Ligaray M., Pachepsky Y., Chun J. A., Yoon K.-S., Park Y., et al. (2020). Assessment of a green roof practice using the coupled SWMM and HYDRUS models. J. Environ. Manage. 261, 109920. doi: 10.1016/j.jenvman.2019.109920
Chapman S., Watson J. E. M., Salazar A., Thatcher M., McAlpine C. A. (2017). The impact of urbanization and climate change on urban temperatures: A systematic review. Landsc. Ecol. 32, 1921–1935. doi: 10.1007/s10980-017-0561-4
Chew L. W., Liu X., Li X. X., Norford L. K. (2021). Interaction between heat wave and urban heat island: A case study in a tropical coastal city, Singapore. Atmos. Res. 247, 105134.1–105134.13. doi: 10.1016/j.atmosres.2020.105134ck
Doick K. J., Peace A., Hutchings T. R. (2014). The role of one large greenspace in mitigating London’s nocturnal urban heat island. Sci. Total Environ. 493, 662–671. doi: 10.1016/j.scitotenv.2014.06.048
Du H. Y., Ai J. Q., Cai Y. L., Jiang H., Liu P. D. (2019). Combined effects of the surface urban heat island with landscape composition and configuration based on remote sensing: A case study of Shanghai, China. Sustainability. 11, 1–13. doi: 10.3390/su11102890
Du H., Cai W., Xu Y., Wang Z., Wang Y., Cai Y. (2017). Quantifying the cool island effects of urban green spaces using remote sensing Data. Urban For. Urban Greening. 27, 24–31. doi: 10.1016/j.ufug.2017.06.008
Eckart K., McPhee Z., Bolisetti T. (2017). Performance and implementation of low impact development - A review. Sci. Total Environ. 607–608, 413–432. doi: 10.1016/j.scitotenv.2017.06.254
Eckart K., McPhee Z., Bolisetti T. (2018). Multiobjective optimization of low impact development stormwater controls. J. Hydrol. 562, 564–576. doi: 10.1016/j.jhydrol.2018.04.068
Feng L., Liu Y., Zhou Y., Yang S. (2022). A UAV-derived thermal infrared remote sensing three-temperature model and estimation of various vegetation evapotranspiration in urban micro-environments. Urban For. Urban Greening. 69, 127495. doi: 10.1016/j.ufug.2022.127495
Feng B. Y., Zhang Y., Bourke R. (2021). Urbanization impacts on flood risks based on urban growth data and coupled flood models. Nat. Hazards. 106, 613–627. doi: 10.1007/s11069-020-04480-0
He B.-J., Zhu J., Zhao D.-X., Gou Z. H., Qi J.-D., Wang J. (2019). Co-benefits approach: Opportunities for implementing sponge city and urban heat island mitigation. Land Use Policy. 86, 147–157. doi: 10.1016/j.landusepol.2019.05.003
Huang L., Li J., Zhao D., Zhu J. (2008). A fieldwork study on the diurnal changes of urban microclimate in four types of ground cover and urban heat island of Nanjing, China. Build. Environ. 43, 7–17. doi: 10.1016/j.buildenv.2006.11.025
Iaria J., Susca T. (2022). Analytic Hierarchy Processes (AHP) evaluation of green roof- and green wall- based UHI mitigation strategies via ENVI-met simulations. Urban Clim. 46, 101293. doi: 10.1016/j.uclim.2022.101293
Kridakorn Na Ayutthaya T., Suropan P., Sundaranaga C., Phichetkunbodee N., Anambutr R., Suppakittpaisarn P., et al. (2023). The influence of bioretention assets on outdoor thermal comfort in the urban area. Energy Rep. 9, 287–294. doi: 10.1016/j.egyr.2023.05.257
Le Phuc C. L., Nguyen H. S., Dao Dinh C. D., Tran N. B., Pham Q. B., Nguyen X. C. (2022). Cooling island effect of urban lakes in hot waves under foehn and climate change. Theor. Appl. Climatol. 149, 817–830. doi: 10.1007/s00704-022-04085-6
Li C., Chuck Wah Y. (2014). Mitigation of urban heat development by cool island effect of green space and water body (Berlin Heidelberg: Springer). doi: 10.1007/978-3-642-39584-0_62
Li Y., Lin D., Zhang Y., Song Z., Sha X., Zhou S., et al. (2023). Quantifying tree canopy coverage threshold of typical residential quarters considering human thermal comfort and heat dynamics under extreme heat. Build. Environ. 233, 110100. doi: 10.1016/j.buildenv.2023.110100
Lin B. S., Lin C. T. (2016). Preliminary study of the influence of the spatial arrangement of urban parks on local temperature reduction. Urban For. Urban Greening. 20, 348–357. doi: 10.1016/j.ufug.2016.10.003
Liu Z., Cheng W., Jim C. Y., Morakinyo T. E., Shi Y., Ng E. (2021). Heat mitigation benefits of urban green and blue infrastructures: A systematic review of modeling techniques, validation and scenario simulation in ENVI-met V4. Build. Environ. 200, 107939.1–107939.15. doi: 10.1016/j.buildenv.2021.107939
Lu J., Li Q. S., Zeng L. Y., Chen J., Liu G. X., Li Y. C., et al. (2017). A micro-climatic study on cooling effect of an urban park in a hot and humid climate. Sustain. Cities Soc 32, 513–522. doi: 10.1016/j.scs.2017.04.017
Moustakis Y., Papalexiou S. M., Onof C. J., Paschalis A. (2021). Seasonality, intensity, and duration of rainfall extremes change in a warmer climate. Earths Future. 9, 1–15. doi: 10.1029/2020EF001824
Nasir D. S., Pantua C. A. J., Zhou B., Vital B., Calautit J., Hughes B. (2021). Numerical analysis of an urban road pavement solar collector (U-RPSC) for heat island mitigation: Impact on the urban environment. Renew. Energy. 164, 618–641. doi: 10.1016/j.renene.2020.07.107
Nguyen T. T., Ngo H. H., Guo W. S., Wang X. C. C., Ren N. Q., Li G. B., et al. (2019). Implementation of a specific urban water management - Sponge City. Sci. Total Environ. 652, 147–162. doi: 10.1016/j.scitotenv.2018.10.168
Ohba M., Sugimoto S. (2019). Differences in climate change impacts between weather patterns: Possible effects on spatial heterogeneous changes in future extreme rainfall. Clim. Dyn. 52, 4177–4191. doi: 10.1007/s00382-018-4374-1
Oliveira S., Andrade H., Vaz T. (2011). The cooling effect of green spaces as a contribution to the mitigation of urban heat: A case study in Lisbon. Build. Environ. 46, 2186–2194. doi: 10.1016/j.buildenv.2011.04.034
Pan H. A., Luo Y. H., Zeng L. Y., Shi Y. R., Hang J., Zhang X. L., et al. (2023). Outdoor thermal environment regulation of urban green and blue infrastructure on various types of pedestrian walkways. Atmosphere. 14, 1037. doi: 10.3390/atmos14061037
Peng J., Dan Y., Qiao R., Liu Y., Dong J., Wu J. (2021). How to quantify the cooling effect of urban parks? Linking maximum and accumulation perspectives. Remote Sens. Environ. 252, 1–12. doi: 10.1016/j.rse.2020.112135
Qian W., Li X. (2023). A cold island connectivity and network perspective to mitigate the urban heat island effect. Sustain. Cities Soc 94, 104525. doi: 10.1016/j.scs.2023.104525
Qin Y., Hiller J. E. (2014). Understanding pavement-surface energy balance and its implications on cool pavement development. Energy Build. 85, 389–399. doi: 10.1016/j.enbuild.2014.09.076
Sagan V., Maimaitijiang M., Sidike P., Eblimit K., Peterson K., Hartling S., et al. (2019). UAV-based high resolution thermal imaging for vegetation monitoring, and plant phenotyping using ICI 8640 P, FLIR Vue Pro R 640, and thermoMap Cameras. Remote Sens. 11, 62. doi: 10.3390/rs11030330
Sanchez L., Reames T. G. (2019). Cooling Detroit: A socio-spatial analysis of equity in green roofs as an urban heat island mitigation strategy. Urban For. Urban Greening. 44, 126331. doi: 10.1016/j.ufug.2019.04.014
Shi D. C., Song J. Y., Huang J. X., Zhuang C. Q., Guo R., Gao Y. F. (2020). Synergistic cooling effects (SCEs) of urban green-blue spaces on local thermal environment: A case study in Chongqing, China. Sustain. Cities Soc 55, 102065. doi: 10.1016/j.scs.2020.102065
Singh R. P., Paramanik S., Bhattacharya B. K., Behera M. D. (2020). Modelling of evapotranspiration using land surface energy balance and thermal infrared remote sensing. Trop. Ecol. 61, 42–50. doi: 10.1007/s42965-020-00076-8
Spahr K. M., Bell C. D., McCray J. E., Hogue T. S. (2020). Greening up stormwater infrastructure: Measuring vegetation to establish context and promote cobenefits in a diverse set of US cities. Urban For. Urban Greening. 48, 126548. doi: 10.1016/j.ufug.2019.126548
Steis Thorsby J. S., Miller C. J., Treemore-Spears L. (2020). The role of green stormwater infrastructure in flood mitigation (Detroit, MI USA) - Case study. Urban Water J. 17, 838–846. doi: 10.1080/1573062X.2020.1823429
Sun X., Tan X., Chen K., Song S., Zhu X., Hou D. (2020). Quantifying landscape-metrics impacts on urban green-spaces and water-bodies cooling effect: The study of Nanjing, China. Urban For. Urban Greening. 55, 126838. doi: 10.1016/j.ufug.2020.126838
Taghizadeh S., Khani S. (2021). Hybrid SWMM and particle swarm optimization model for urban runoff water quality control by using green infrastructures (LID-BMPs). Urban For. Urban Greening. 60, 127032. doi: 10.1016/j.ufug.2021.127032
Tan X., Sun X., Huang C., Yuan Y., Hou D. (2021). Comparison of cooling effect between green space and water body. Sustain. Cities Soc 67, 102711. doi: 10.1016/j.scs.2021.102711
Ucal M., Xydis G. (2020). Multidirectional relationship between energy resources, climate changes and sustainable development: Technoeconomic analysis. Sustain. Cities Soc 60, 102210. doi: 10.1016/j.scs.2020.102210
Wang J., Meng Q., Zhang L., Zhang Y., He B. J., Zheng S., et al. (2019). Impacts of the water absorption capability on the evaporative cooling effect of pervious paving materials. Build. Environ. 151, 187–197. doi: 10.1016/j.buildenv.2019.01.033
Wang Y., Sheng S., Xiao H. (2021). The cooling effect of hybrid land-use patterns and their marginal effects at the neighborhood scale. Urban For. Urban Greening. 59, 100781. doi: 10.1016/j.ufug.2021.127015
Wu Z., Chen L. (2017). Optimizing the spatial arrangement of trees in residential neighborhoods for better cooling effects: Integrating modeling with in-situ measurements. Landsc. Urban Plan. 167, 463–472. doi: 10.1016/j.landurbplan.2017.07.015
Yan Q., Hu Y. H., Ye H. B. (2020). Time to update China's panda loan terms. Science 367 (6476), 373–373. doi: 10.1126/science.aba3948
Yan C., Guo Q., Li H., Li L., Qiu G. Y. (2020). Quantifying the cooling effect of urban vegetation by mobile traverse method: A local-scale urban heat island study in a subtropical megacity. Build. Environ. 169, 1–12. doi: 10.1016/j.buildenv.2019.106541
Yang Y., Li J., Huang Q., Xia J., Li J., Liu D., et al. (2021). Performance assessment of sponge city infrastructure on stormwater outflows using isochrone and SWMM models. J. Hydrol. 597, 126151. doi: 10.1016/j.jhydrol.2021.126151
Yang F., Zhao C. C., Wang J. Y., Liu C. S., Sun Y., Soomro S. E. H., et al. (2022). Grid-quantification study on the effect of rapid urbanization on hydrological processes. Water Supply. 22, 5853–5872. doi: 10.2166/ws.2022.202
Yu Z., Yang G., Zuo S., Jørgensen G., Koga M., Vejre H. (2020). Critical review on the cooling effect of urban blue-green space: A threshold-size perspective. Urban For. Urban Greening. 49, 126630. doi: 10.1016/j.ufug.2020.126630
Zhao J., Huang S., Huang Q., Leng G., Wang H., Li P. (2020). Watershed water-energy balance dynamics and their association with diverse influencing factors at multiple time scales. Sci. Total Environ. 711, 135189. doi: 10.1016/j.scitotenv.2019.135189
Zhao X., Luo Y., He J. (2022). Quantitative analysis and evaluation of rainwater absorption and thermal environment for residential design plan with consideration of LID strategies. Urban Clim. 45, 101274. doi: 10.1016/j.uclim.2022.101274
Keywords: green stormwater infrastructures, cold island effect, cooling effect, field measurement, heat flux
Citation: Xu H, Sheng K and Gao J (2023) Mitigation of heat island effect by green stormwater infrastructure: a comparative study between two diverse green spaces in Nanjing. Front. Ecol. Evol. 11:1307756. doi: 10.3389/fevo.2023.1307756
Received: 05 October 2023; Accepted: 30 November 2023;
Published: 11 December 2023.
Edited by:
Chenghao Wang, University of Oklahoma, United StatesReviewed by:
Ying Zhu, Xi’an University of Architecture and Technology, ChinaCopyright © 2023 Xu, Sheng and Gao. This is an open-access article distributed under the terms of the Creative Commons Attribution License (CC BY). The use, distribution or reproduction in other forums is permitted, provided the original author(s) and the copyright owner(s) are credited and that the original publication in this journal is cited, in accordance with accepted academic practice. No use, distribution or reproduction is permitted which does not comply with these terms.
*Correspondence: Haishun Xu, eHVoYWlzaHVuQG5qZnUuZWR1LmNu
Disclaimer: All claims expressed in this article are solely those of the authors and do not necessarily represent those of their affiliated organizations, or those of the publisher, the editors and the reviewers. Any product that may be evaluated in this article or claim that may be made by its manufacturer is not guaranteed or endorsed by the publisher.
Research integrity at Frontiers
Learn more about the work of our research integrity team to safeguard the quality of each article we publish.