- 1Hunan Provincial Institute of Land and Resources Planning, Changsha, China
- 2College of Tourism, Hunan Normal University, Changsha, China
The urgent need to maintain ecosystem provisioning services and achieve urban sustainable development goals has led to a long-standing focus on the driving effects of land urbanization (LU) in the Middle Reaches of the Yangtze River Urban Agglomeration (MRYRU) on vegetation cover system productivity. Previous studies have lacked consideration for the long-term correlation between land urbanization (LU) and the Gross Primary Productivity (GPP) of vegetation, as well as the spatial non-stationarity of LU’s impact in the region. This study is based on land cover remote sensing data and GPP imagery data to monitor the long-term evolution trends of LU and GPP in MRYRU from the perspectives of townships and grids. It further investigates the spatial correlation and clustering characteristics between the two using bivariate spatial autocorrelation method. Additionally, a Geographically and Temporally Weighted Regression (GTWR) model was employed to analyze the spatial effects of LU on GPP. The results indicate that the GPP of MRYRU grows to 1572.88gCm-2a-1 with an average annual growth rate of 0.848% from 2000 to 2020. High-value areas are distributed in the central and eastern mountainous of the urban agglomeration, while low-value areas gradually formed an “n”-shaped clustered distribution pattern. The negative spatial autocorrelation between LU and GPP shows a general upward trend, and Low-High is the dominant agglomeration type, concentrated in Hengyang and some mountainous cities in Jiangxi Province. The overall negative impact of land urbanization on GPP in MRYRU is maintained in the spatially nonstationary pattern of driving coefficients, with negative impact areas mostly concentrated in the city’s main city zone and positive impact areas concentrated in the urban agglomeration’s mountainous townships. This study provides support for MRYRU to adopt more resilient land optimization management strategies and vegetation ecological restoration plans.
1 Introduction
Gross Primary Productivity (GPP) is the amount of organic matter produced from carbon dioxide fixation by photosynthesis in green plants per unit area per unit time. It determines the initial organic matter and energy that enters terrestrial ecosystems and directly or indirectly determines the ecosystem’s provision of product services such as food, fiber, and fuel needed for human survival (Kim et al., 2017; Jia et al., 2022; Wang et al., 2022). GPP is also commonly used as a key element in measuring terrestrial ecosystem health and land-atmosphere CO2 exchange. GPP has an irreplaceable contribution to the regulation of the global bio-carbon balance, the maintenance of global climate stability and the sustainability of the material cycle, and is the ecological basis for the survival and development of human society (Guido et al., 2017; Dyvavani et al., 2023; Yang et al., 2023). Regional GPP variations due to global changes in environmental and biological factors have been recognized in previous studies, such as temperature, humidity and solar radiation, and changes in vegetation phenology (Zhong et al., 2021; Zhao et al., 2022; Guo et al., 2023; Tan et al., 2023). However, with the rise of the human footprint and urban construction activities, it has changed urban land use functions, scale, and product services of vegetation ecosystems, and as a result, posed risks to soil fertility, vegetation growing space, and bio-carbon cycling processes (Hu et al., 2022; Wei et al., 2023b). For example, a study by Liu et al. (2019) confirms that global urban land expansion encroached on terrestrial vegetation net primary productivity (NPP) at a rate of 22.4 Tg·C per year in the first decade of the 21st century and offset 30% of climate change-driven NPP growth. In particular, the encroachment of urban production-living space on ecological space during land urbanization has placed a heavy burden on the ecological pattern of urban landscapes and the global vegetation cover system and its productivity, as well as posing a challenge to the maintenance of the carbon cycle in urban ecosystems and the construction of sustainable cities (Tan et al., 2023; Xu et al., 2023). How to measure the change in the intensity of intrusion of land urbanization process on vegetation ecosystems and the response pattern of GPP to urban land transformation is of great significance for urban ecological environment governance and sustainable land management.
During the past 20 years of the 21st century, the global urban land area grew from 239,900 km2 to 519,800 km2, expanding by 117.49%, especially in Asia, where the urban land area grew by 133,700 km2 (Liu et al., 2019; Wei et al., 2023b). Governments have developed a series of binding strategies based on macro-consensus strategies such as the Global Assessment Report on Biodiversity and Ecosystem Services and the International Geosphere-Biosphere Program (IGBP) (Guido et al., 2017; Xie et al., 2022). Aiming to mitigate the encroachment of urban sprawl on the productive capacity of vegetated ecosystems in order to better serve the urban sustainable development goals of sustainable urbanization (Goal11, SDG), maintenance of the ecosystem carbon cycle (Goal13, SDG) and ecosystem conservation (Goal15, SDG). For example, China has recently issued policies such as the Opinions on Comprehensively Implementing the Forest Chief System and the Natural Forest Protection and Restoration System Program to mitigate the negative ecological impacts of urban construction activities, to increase natural ecosystem and environmental protection, and to promote the protection and ecological restoration of pristine forests (Zhao et al., 2022). Similar policies have been implemented in the United States in terms of financial disbursement, where a national ecological compensation program, the Land Fallow Conservation Program (CRP), has been implemented to achieve the objectives of returning ecologically fragile agricultural lands to forests, restoring vegetation cover, improving forest land quality, and enhancing the carbon storage capacity of forests. Scholars have mainly developed case studies on the long-term trends of land urbanization and vegetation cover systems and the prospects for sustainability, the driving effects and correlations of urbanization on vegetation production capacity under climate change, and the externalities and indirect effects of the impacts of land urban expansion (Chen et al., 2019; Gui et al., 2021; Wang et al., 2021). For example, Zhou et al. (2023) constructed an “urbanization-vegetation cover coordination index” to analyze the coordinated relationship between urbanization and vegetation cover systems and their productivity in China, and found that the expansion of urban land use has caused a significant decline in the greenness of urban areas in China, with the degradation of new urban areas being the most significant. Zhuang et al. (2022) investigated the joint mechanism of “climate-urbanization-soil” driving spatio-temporal changes in NPP, and found that soil salinization has a stronger impact on the productive capacity of vegetation cover systems than urbanization. Zafar et al. (2023) predicted the future trends in the productive capacity of urban and rural vegetation in a typical Pakistani city and analyzed the long-term impact of urbanization on urban and rural vegetation and environmental vulnerability. Mu et al. (2023) estimated the trend of NPP in the Central Plains Urban Agglomeration over the past 40 years, and found that NPP showed a continuous downward trend under rapid urbanization, and that the indirect impact of urbanization on the high-speed developing cities was higher than that of the medium- and low-speed cities. These studies provide support for building sustainable and resilient urban agglomerations and green and sustainable urban planning policies. However, whether land urbanization contributes to or coerces total vegetation productivity? As well as the potential correlation and the estimation of the spatial effects of land urbanization on GPP are still insufficiently integrated. This is clearly detrimental to the development of site-specific regional vegetation ecosystem restoration strategies and green urban planning policies.
The Middle Reaches of the Yangtze River Urban Agglomeration (MRYRU) is another strategic urban cluster in China after the Beijing-Tianjin-Hebei Urban Agglomeration, the Yangtze River Delta Urban Agglomeration, the Guangdong-Hong Kong-Macao Greater Bay Area, and the Chengdu-Chongqing Urban Agglomeration, and the region brings together Wuhan, Changsha, and Nanchang, which are important supportive cities in China (Yang et al., 2022; Zeng et al., 2023). According to data released by China’s Bureau of Statistics, the total GDP and population of the city cluster in the middle reaches of the Yangtze River in 2020 will be 9.3 trillion and 120 million people, accounting for 9.175% and 9.207% of China’s total, respectively. Rapid clustering of population, knowledge, information and technology factors has contributed to the rapid development of the socio-economic level of MRYRU, but also generated mutant expansion of urban land to meet the demand for the construction of transportation, housing, factories and power generation sites in the urban agglomeration (Su et al., 2013). A large number of ecosystem protection and restoration projects have been constructed in the region since the implementation of the “Development Plan for the Urban Agglomeration in the Middle Reaches of the Yangtze River” to achieve a green and low-carbon transition and ecological civilization. However, the long-term evolution of land urbanization and GPP and the impact of land urbanization on the vegetation ecology of MRYRU in the context of the human-land conflict of “development and conservation” still need to be further explored (Zhu and He, 2021; Ma et al., 2022). This is an urgent issue for understanding the eco-carbon cycle process and ecologically sustainable development pathways of MRYRU. MRYRU contains three key regions, that is, Wuhan City Circle, Changsha-Zhuzhou-Xiangtan Urban Agglomeration and Poyang Lake Urban Agglomeration, and the existing studies focus more on the evolutionary trends of land urbanization and vegetation ecosystems in these subregions. The quantification of the overall evolutionary pattern and spatial correlation characteristics of land urbanization and GPP in MRYRU is still insufficient (Luo et al., 2020). In particular, there is a lack of impact analysis experiments based on township or grid perspectives, and few studies have focused on the regional heterogeneity of impacts exerted by land urbanization (Chen and Chi, 2022). This is not conducive to providing targeted green urban planning strategies, vegetation ecological resilience development and ecological conservation programs for the MRYRU.
Overall, the existing studies provide a rich practical basis for revealing the effects of land urbanization on vegetation GPP from a doctrinal perspective, but further discussion is needed on the spatial effects of land urbanization on the vegetation cover systems in MRYRU: (1) Insufficient attention has been paid to the spatial correlation between land urbanization and vegetation GPP; (2) the study scale usually focuses on the individual analysis of subdivided urban agglomerations, and seldom concerns the comprehensive impacts of land urbanization in the urban agglomeration areas in the MRYRU from the townships perspective; (3) the estimation of the spatial effects of land urbanization on vegetation GPP needs to be improved, in order to accommodate the spatial heterogeneity of the driving impacts brought about by regional differences in topography, meteorology, urbanization processes and socio-economic levels. Based on multivariate remote sensing image data, this study utilizes GIS spatial analysis techniques, land use transfer matrix and bivariate spatial autocorrelation to monitor the long-term trends and spatially correlated clustering features of land urbanization process and GPP in the MRYRU, both from the grid and the township perspectives. Further integrating anthropogenic and natural control variables such as climate, topography, spatial distance and socio-economic factors, a Geographically and Temporally Weighted Regression (GTWR) model was used to investigate the spatially differentiated impacts of land urbanization on GPP under the township perspective. The purpose is to provide a decision-making reference and scientific guidance for MRYRU to adopt more resilient land optimization management strategies and vegetation ecological restoration programs.
2 Study area, methods and data sources
2.1 Study area
MRYRU is a Chinese strategic urban agglomeration with Wuhan City Circle, Changsha-Zhuzhou-Xiangtan Urban Agglomeration and the Urban Agglomeration around Poyang Lake as the main components, and is also an important part of the Yangtze River Economic Belt, which involves the three provincial-level central cities of Wuhan, Changsha, and Nanchang as well as 28 prefectural-level municipalities, and contains 4,037 townships units in total. Our study takes 4037 townships as the study sample to explore the spatio-temporal evolution and spatial correlation between land urbanization and GPP, and to reveal the spatio-temporal impact effect of land urbanization on the GPP in the MRYRU region. MRYRU is an important part of the Yangtze River Basin, which belongs to the subtropical monsoon climate region and the evergreen broad-leaved forest belt. With its warm climate, abundant rainfall, well-developed water system, lush vegetation and rich species resources, the region is a concentrated area for a large number of rare and endangered wild animals and plants in China. However, owing to the high population density and long history of urbanization, the coercion on the vegetation cover system by population movement, land use expansion and economic factor agglomeration under the land urbanization process still need to be mitigated. In particular, under the regional differences in the “natural-social-economic” complex ecosystems of urban agglomerations, the complexity of vegetation ecosystem restoration and resilience building projects and the coordination of “urban construction-ecological protection” need more attention. Therefore, studying the impact of land urbanization on GPP in MRYRU has great practical and doctrinal value for building ecological resilience and sustainable development in the region (Figure 1).
2.2 Methods
2.2.1 Accounting for land urbanization levels
Land urbanization is generally regarded as the proportion of built-up land area to all land area in a city, reflecting the process of urbanization in which urban built-up land is increasing and rural land is contracting. It is a core indicator for evaluating the level of urbanization, along with population urbanization and economic urbanization. Drawing on the studies of Peng et al. (2020) and Wei et al. (2023a), the spatio-temporal dynamic evolution of the land urbanization level in the MRYRU is measured using the proportion of urban land size to the overall land size. The calculation formula is as follows:
where LUi is the land urbanization level of unit i; Uurban is the urban land area; and Utotal is the total urban area.
2.2.2 Land-use transfer matrix
Land urbanization is intuitively reflected in the variation and scale change of urban land use types. We use the land use transfer matrix to quantify the area conversion process of each land use type in the urban agglomeration in the MRYRU from 2000 to 2020 for showing the encroachment of urban land on other types of land use and the dynamic change of scale (Yang and Liu, 2022; Tan et al., 2023). The land use transfer matrix is calculated in the ArcGIS platform with the following formula:
Where S is the area of a certain land use type in the city; n is the number of land use types; and Sij is the area of conversion from land use type i to land use type j in a certain period of time.
2.2.3 Bivariate spatial autocorrelation
Bivariate spatial autocorrelation models are used to analyze the spatial correlation between land urbanization and GPP. The method can test the global and local correlation features that exist between the two systems in geospatial terms. Among them, the local bivariate spatial autocorrelation is calculated as:
where Wij is the spatial weight matrix; LUi and GPPj are the land urbanization level and total vegetation productivity of units i and j, respectively; σLU and σGPP are the variances of LU and GPPl, respectively (Dong et al., 2023).
2.2.4 Geographically and temporally weighted regression
The GWR model focuses on the effect of spatial heterogeneity in local areas on the overall regression fit, and can be used to test for individual spatial variation in regression coefficients (Tian et al., 2022). The GTWR model takes into account the expansion of geographically weighted regression models due to the non-stationarity of time, and incorporates both temporal and spatial effects into the model, which allows the model to deal with spatial-temporal heteroskedasticity at the same time. In this study, the local variation effect of the impact of land urbanization on the GPP of MRYRU was measured based on the GTWR model for discussing the spatio-temporal characteristics of the impacts generated by land urbanization (Tian et al., 2023). The model calculation formula is as follows:
Where β0(ui,vi,ti) is the regression constant; ϵi is the residuals of the model; the Xik table is the observed values of the drivers, including the key explanatory variable LU and the other control variables; and βk(ui,vi,ti) is the regression coefficients of the variables, i.e., the weighting coefficients of the variables at the spatio-temporal location (ui,vi,ti).
To measure βk(ui,vi,ti) for each variable k in all spatio-temporal positions i, βk(ui,vi,ti) can be transformed as follows:
Where W(ui,vi,ti) is the spatio-temporal weight matrix taking into account spatio-temporal effects.
2.3 Data sources
2.3.1 Land cover remote sensing data
The land urbanization level measurement was based on land cover remote sensing data, which was obtained from the Environment and Resources Data Center of the Chinese Academy of Sciences (http://www.resdc.cn) with a spatial resolution of 30m (CNLUCC). The dataset is a national-scale multi-period land-use thematic database of China constructed through manual visual interpretation, using Landsat TM/ETM and Landsat 8 remote sensing images as the main information source. The dataset involves 25 land-use types, which can be grouped into six primary classifications: cropland, forest land, grassland, watershed, urban land and bare land, and has been widely used in many research fields, such as land urbanization, expansion of urban land, carbon storage and ecological spatial dynamics evolution (Table 1).
2.3.2 Gross primary productivity
Gross primary productivity (GPP) of vegetation is an important basis for food production and the ecological-carbon cycle in terrestrial ecosystems. Internationally recognized methods for estimating GPP include continuous observation at flux stations, estimation by terrestrial ecological process models, and other methods. In this study, we used the GPP dataset provided by Fan et al. (2023) in China Scientific Data Platform. This dataset is based on the long-term networked observation data and open datasets of ChinaFLUX, combining biological, climatic and soil factors, and using the random forest regression tree model to simulate GPP per unit of leaf area to construct the GPP dataset of China from 2000 to 2020, with a spatial resolution of 30 arcsec (Dataset doi: 10.57760/sciencedb.o00119.00077).
2.3.3 Natural and socio-economic control variables
In addition to land urbanization, the natural geographic context and socio-economic conditions are also considered to have important effects on terrestrial vegetation ecosystems. Referring to the study of Li et al. (2022), we selected natural indicators such as temperature, wind speed, humidity, and distance to rivers, and anthropogenic indicators such as GDP density, population density, distance to county administrative centers, and distance to railroads and highways as control variables to be introduced into the regression model in order to improve the degree of model fit. The data for the natural control variables are mainly derived from relevant data products provided by the Geographic Data Cloud Platform (http://www.gscloud.cn/sources/index) and the Earth System Science Data Center (http://www.geodata.cn/) of the Chinese Academy of Sciences. The GDP density among the anthropogenic control variables is mainly derived from the grid dataset of China’s regional GDP density with a spatial resolution of 1km constructed by the Resource and Environmental Science Data Center of the Chinese Academy of Sciences (https://www.resdc.cn/). Population densities are expressed as Landscan Global Population Statistics Analysis data, developed by the U.S. Department of Energy’s Oak Ridge National Laboratory (ORNL), with a spatial resolution of nearly 1km x 1km (https://www.satpalda.com/product/landscan/).
3 Result
3.1 Evolution of the spatio-temporal dynamics of the GPP
From the grid perspective, the overall average GPP of MYRYU increased from 1328.40 gCm-2a-1 to 1572.88 gCm-2a-1 at an average annual growth rate of 0.848% from 2000 to 2020, which is an increase of 18.404% (Figures 2A–C). This indicates that ecosystem vegetation cover and productivity in MYRYU showed a rapid increasing trend in the first two decades of the 21st century. From the township perspective, the overall GPP also showed an increasing trend from 2000 to 2020, with the mean value of GPP increasing from 1166.649 gCm-2a-1 to 1368.795 gCm-2a-1, an increase of 17.733% (Figures 2E–G). The spatial distribution pattern of GPP is relatively stable, with high values distributed in the Luoxiao Mountains in the central part of the urban agglomeration, the Mofu Mountains and the Wuyi Mountains in the east. These areas have sparse human footprints, lush vegetation, and vegetation ecosystems that maintain good carbon sequestration and production services. Low-value areas are concentrated in the Wuhan, Changsha and Nanchang metropolitan areas and the surrounding densely populated urban areas, and gradually forming an “n” shaped cluster distribution pattern. However, the overall upward trend in GPP does not mean that the level of vegetation coverage levels and productivity are increasing across MYRYU. In terms of changing trends, despite significant GPP growth in the southern and eastern regions of the urban agglomeration from 2000 to 2020, the GPP in parts of the main city zone of the cities of Wuhan, Changsha, Nanchang, Xiangyang and Yichang showed a significant downward trend. This may be due to the gradual degradation of the natural habitat and vegetation cover of the ecosystem as a result of the crowding out of a large amount of ecological space by productive-living space in the main city zone during the study period (Figures 2D, H).
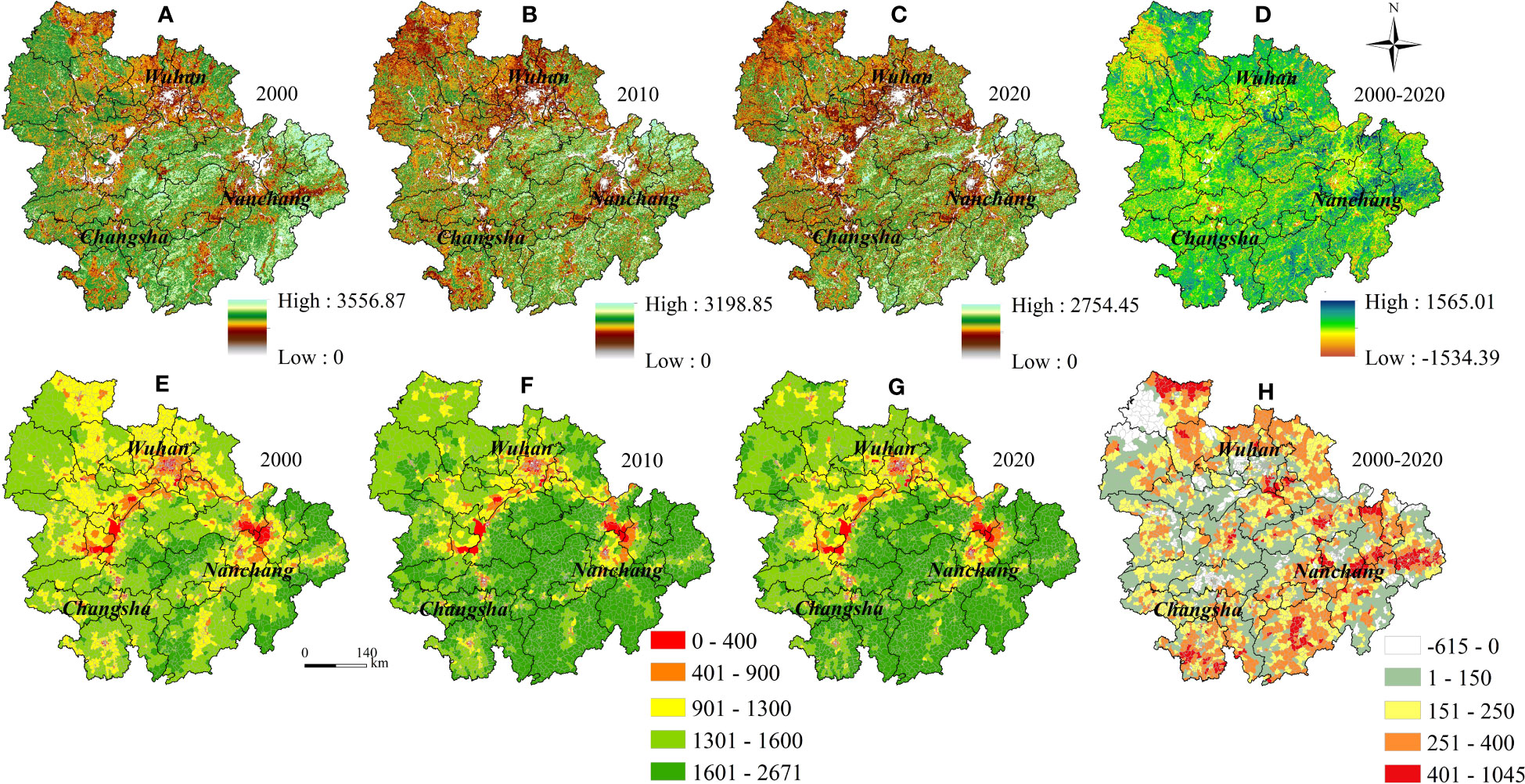
Figure 2 2000-2020 GPP distribution (A–C) and trends (D) of MRYRU at grid scale; GPP distribution (E–G) and trends (H) at township scales.
3.2 Evolution of the spatio-temporal dynamics of land urbanization
The land use types of MYRYU are dominated by two categories, farmland and forest land, which are distributed in the plain areas (Jianghan Plain, Dongting Lake Plain and Poyang Lake Plain) and the mountainous areas (Luoxiao Mountains, Shufu Mountains and Wuyi Mountains, etc.) of the urban agglomeration, respectively. The average share of them was 37.091% and 49.588% in 2000 and 2020, respectively, but decreased by 4,798.480km2 and 2,063.764km2 during the study period (Figures 3A–C). The urban land in MYRYU increased from 9,098.238km2 to 15,394.108km2 at an average annual growth rate of 2.664% from 2000 to 2020 (Figure 3E). According to the land use transfer matrix information, the growth of urban land in MYRYU from 2000 to 2020 is mainly realized by encroachment on farmland and forest land. Especially in 2000-2010, the scale of conversion of farmland and forest land to urban land was 2882.403km2 and 1048.1643km2, respectively, and in 2010-2020, the scale of conversion was 1487.278km2 and 293.614km2, respectively (Figure 3E). These new urban land uses occurred mainly in the areas surrounding the Wuhan, Changsha and Nanchang metropolitan areas (Figure 3D). The LU level of MRYRU increased from 2.657% in 2000 to 4.496% in 2020. And the high value area were clustered in point-shaped distribution centered on the main city zone of the provincial capital city in 2000, and by 2020 are gradually shifting to a face-shaped cluster centered on the metropolitan area of the provincial capital cities, such as Wuhan metropolitan (Wuhan-Xiaogan-Xiantao-Jingzhou) and the Changsha metropolitan (Changsha-Zhuzhou-Xiangtan). Geographically, the distribution of high values has formed a “ring-shaped” due to the socio-economic disparity of the region and the mountain range barrier (Figure 4).
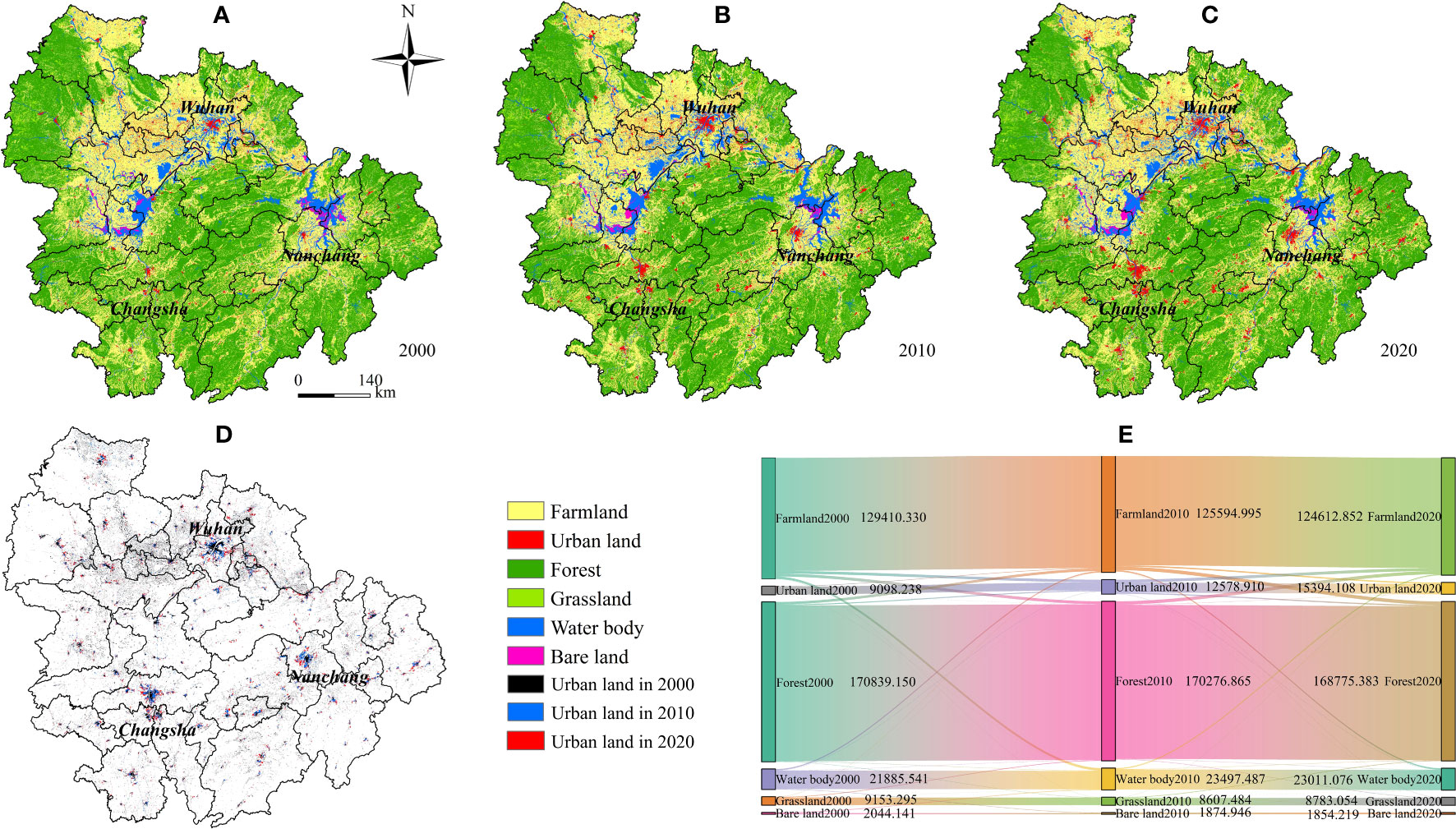
Figure 3 Trends in land-use transformation (A-C), urban expansion process (D) and the land-use transfer matrix (E) in MRYRU, 2000-2020.
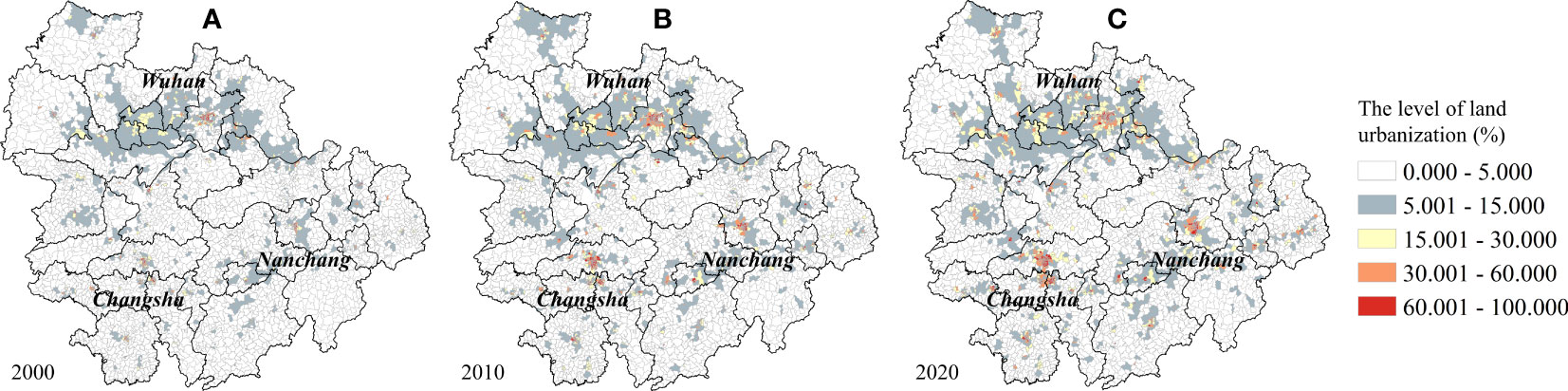
Figure 4 Trends in spatio-temporal distribution of land urbanization levels in MRYRU, 2000 (A), 2010 (B) and 2020 (C).
3.3 Spatio-temporal correlation between land urbanization and GPP
The bivariate global spatial autocorrelation indices of LU and GPP for MYRYU are -0.499, -0.510 and -0.504 in 2000, 2010 and 2020, respectively (Figure 5). This showed that the negative correlation between land urbanization and vegetation GPP in MYRYU is on a general upward trend in geospatial terms, and an urgent need to regulate the correlation among them. Negative correlation clusters (including High-Low and Low-High) are the main type of local spatial autocorrelation of the LU and GPP bivariate at the township scale, with the share increasing from 27.467% in 2000 to 29.607% in 2020. In particular, Low-High clusters accounted for more than 18% of all townships in each of the study periods. This suggests that land urbanization is an important geographic factor associated with the productivity of vegetated ecosystems, and that there is an urgent need for MYRYU to regulate land expansion strategies to reduce the negative impacts on the stability of vegetated ecosystems and carbon stock functions. In terms of spatial distribution, High-Low cluster townships are stably distributed around the main city zone of Wuhan, Nanchang, and Changsha-Zhuzhou-Xiangtan, while Low-High cluster townships are mainly distributed in Hengyang and some mountainous cities in the Urban Agglomeration around Poyang Lake (Yichun, Ji’an, Fuzhou, Yingtan and Shangrao). Low-Low cluster townships are distributed in the mountainous areas at the junction of Hunan, Hubei and Jiangxi provinces, and High-High type townships are in a fragmented layout.
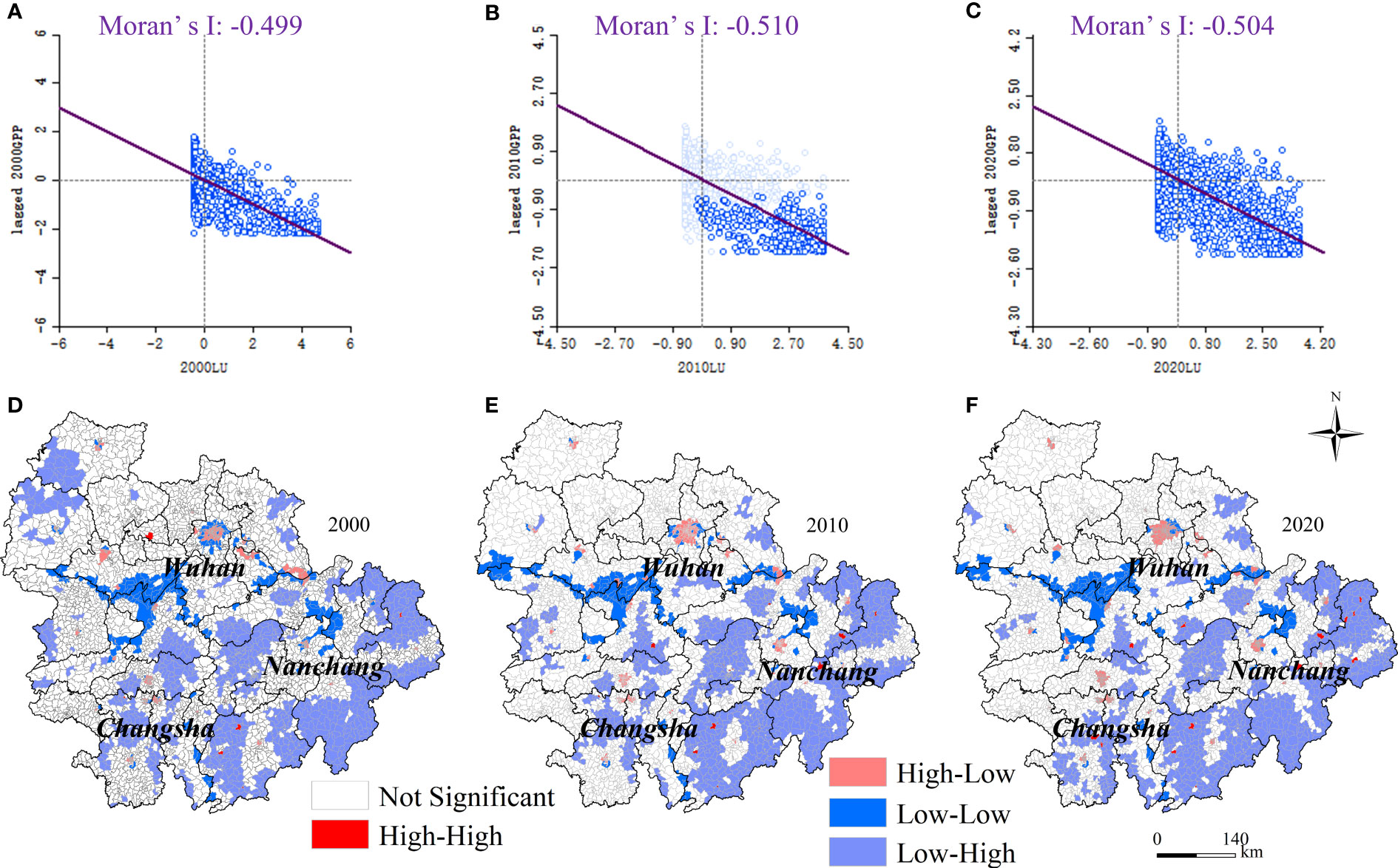
Figure 5 Evolution of global (A–C) and local bivariate spatial correlation pattern (D–F) between land urbanization and GPP in MRYRU, 2000-2020.
3.4 Spatio-temporal impacts of land urbanization on GPP based on the GTWR model
The control variables were selected to input into the GTWR model (spatial distribution of control variables shown in Figure 6), and the Adjusted R2 of the model was 0.619, 0.632, and 0.756 for 2000, 2010, and 2020, respectively (Figure 7). Our study confirms that the regression coefficients of land urbanization in MYRYU have significant spatial non-stationary properties in 2000, 2010 and 2020, validating the importance of investigating the impact of LU on GPP from a spatially localized perspective. Overall, the LUs of more than 2/3 of the MYRYU townships exert a negative depressing effect on the GPP in the study period, especially in the 2020 when 77.241% of the townships are in a negative impact. Geospatially, the spatial effect of LU on GPP shows obvious steadily characteristics, and the negatively affected areas are concentrated in the main city zone of the cities, especially in Changsha-Zhuzhou-Xiangtan area, Wuhan, JingZhou and Nanchang. The reason for this phenomenon may lie in the long history of disturbance of the vegetation cover system in the main city zone by urban construction activities, with a large amount of vegetated ecological space being crowded out by houses, factories and transportation routes. However, with the implementation of the concepts of urban ecological resilience and sustainable development, the three major urban agglomerations with the centers in Wuhan, Changsha and Nanchang, respectively, have proposed urban land optimization management plans and the concepts of forest city and park city. This will provide more possibilities for regulating the relationship between the demand for urban landuse and the total primary productivity of the ecosystem. The townships where LU has a positive impact on GPP account for about 22% of the whole number of townships in MYRYU, and are mainly located in Xiangyang, Yichang, and the southern counties of Zhuzhou (Youxian, Chaling, and Yanling counties). A possible reason is that these areas are covered with high-quality vegetation cover, and the terrain is mainly mountainous and hillyhave. For example, Xiangyang and Yichang are located in the mountains of western Hubei, and the southern counties of Zhuzhou are located in the hills of central Hunan, which are more resilient to land urbanization and human remodeling activities, and have a certain degree of resistance to ecological risks and disruptive capacity. Furthermore, a series of ecological restoration measures taken by the national and local governments in the Yangtze River Basin Ecological Conservation Area optimized the environmental conditions for the vegetation growth and the ecological suitability of urban expansion activities, and may have contributed to the increase in vegetation cover and productivity.
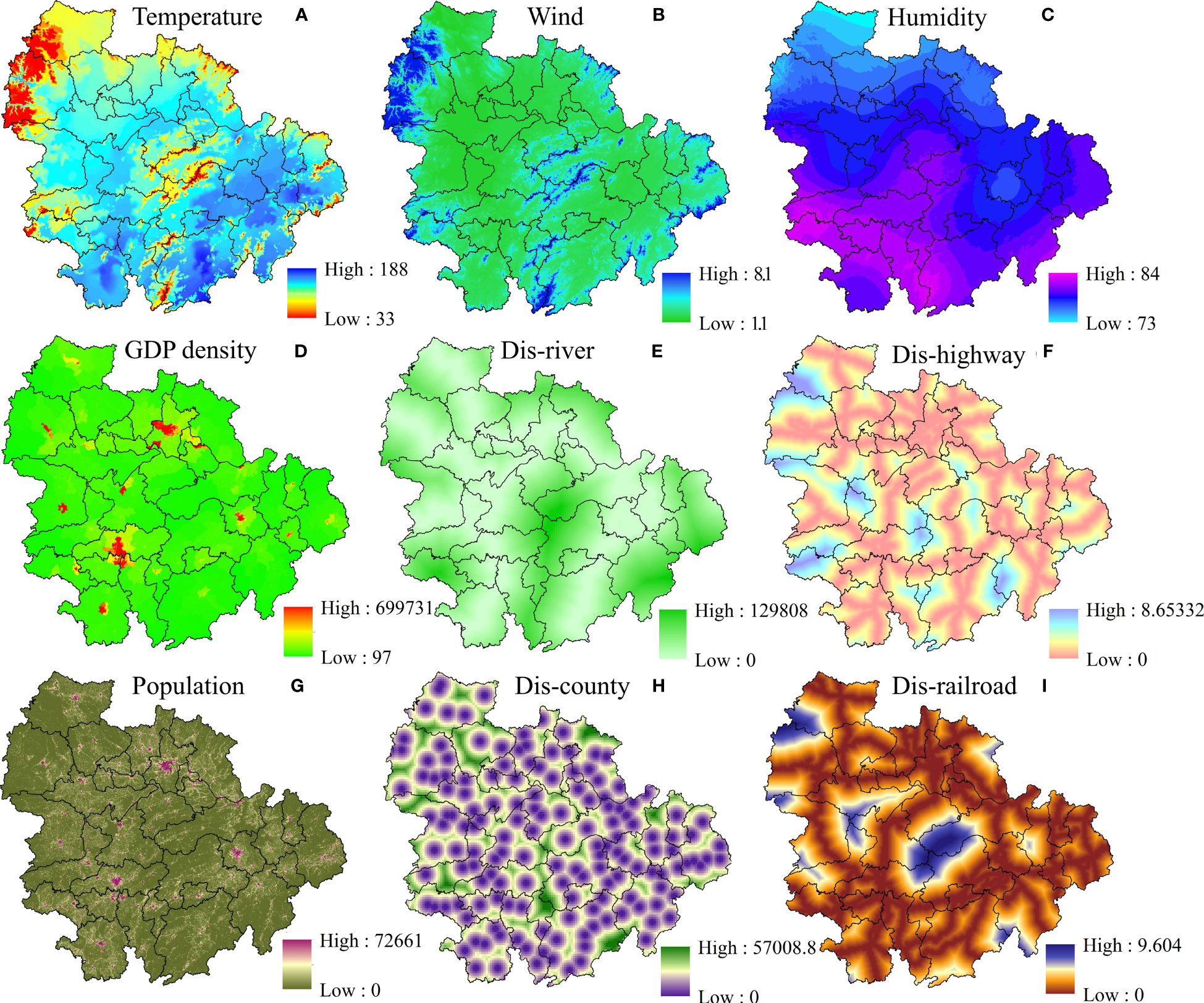
Figure 6 Spatial distribution of control variables: temperature (A); wind (B); humidity (C); GDP density (D); distance to river (E); distance to highway (F); population density (G); distance to county (H); distance to railroad (I).
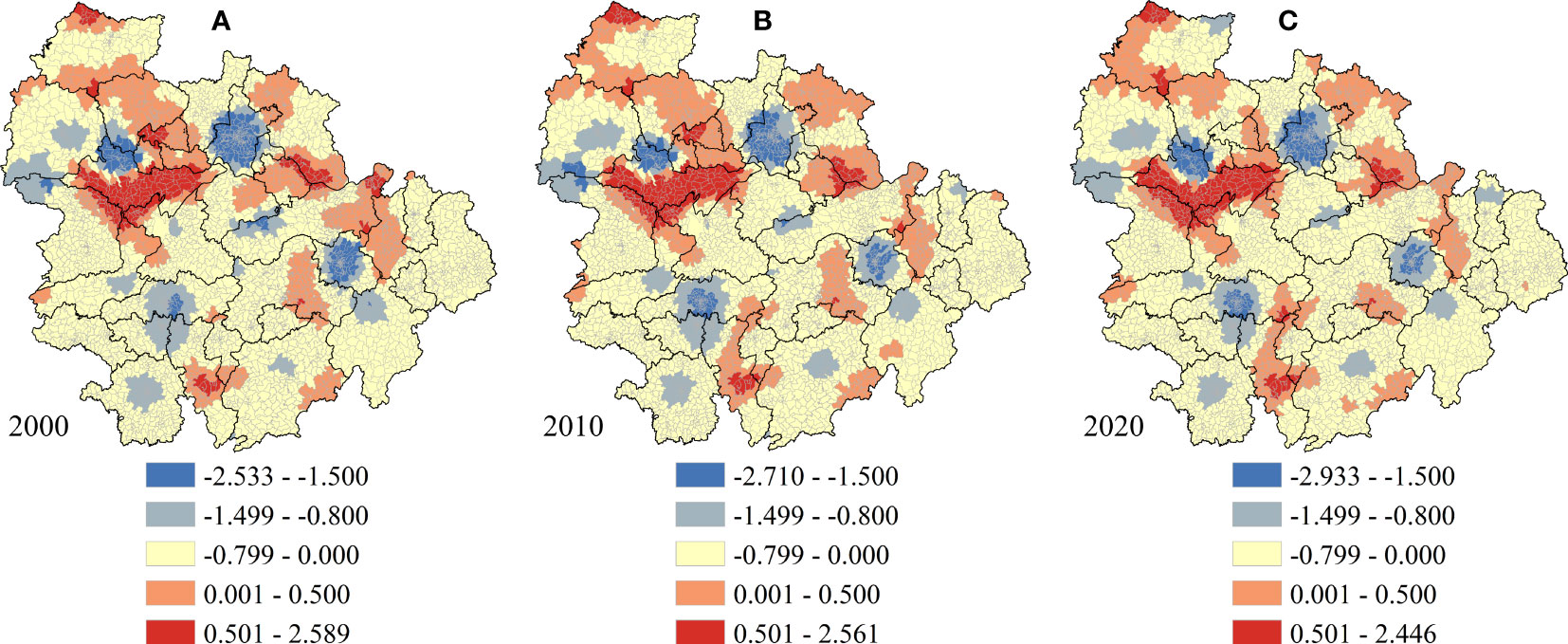
Figure 7 Distribution of regression coefficients for land urbanization under the GTWR model in 2000 (A), 2010 (B) and 2020 (C).
4 Discussion
4.1 Driving mechanisms and spatial heterogeneity effect of LU on GPP
Natural variations in temperature, precipitation, and photosynthesis of vegetation can shape the long-term steady-state evolution of vegetation cover and productivity, while anthropogenic programs such as land urbanization, farmland expansion, and predatory exploitation of forest resources can shift this stable evolution process, resulting in abrupt changes in the vegetation GPP (Qin and Sha, 2023). However, green urban planning, environmental regulation and eco-engineering are considered to be an optimization measure that can achieve the gradual harmonization of “development and conservation” objectives at the land urbanization level. The pathway is to control the pattern of land urbanization at the front end of ecological restoration, to modulate the input technology, capital and knowledge of ecological restoration at the middle end, and to strengthen comprehensive ecological management at the end (Guan et al., 2019; Zafar et al., 2023; Zhang et al., 2023). Our study confirms the joint growth trend of LU and GPP at the township level in the MYRYU region, however, the encroachment of vegetation by urbanization is still occurring, especially in the major urban areas where the significant decline in GPP and the rapid growth of LU are forming an opposite. Although the high quality ecological substrate of MYRYU itself is trying hard to whitewash the overall increase in vegetation GPP in the area, investigations based on the GWTR model confirm that the negative impacts of the LU are in control of the bigger picture in the long term, and that the rapid growth of the main city zone in particular is exacerbating this productivity deprivation. This has been mentioned in previous studies that the effects of deep modification of human settlements on the productivity of vegetation cover systems are significant and sustained over time (Zhong et al., 2021; Muntasir et al., 2022; Mu et al., 2023). The study also further observed some success in the conservation of mountain vegetation. In contrast to the declining trend of GPP in the main city zone and the metropolitan area of the provincial capital city, the mountainous cities around MYRYU have a significant trend of GPP growth and land urbanization has a positive impact on GPP in some mountainous areas. This is similar to the findings of several studies (Asif and Almagul, 2022; Hu et al., 2023; Liu et al., 2023). We suggest that this is related to the implementation of tightened and sustainable environmental regulations, urban planning and ecological engineering policies in the Yangtze River Basin in China in recent years. For example, the Action Plan for the Protection and Restoration of the Yangtze River, the 13th Five-Year Plan for Environmental Protection in Hubei Province, and Hunan Provincial Government’s “No. 1 Key Project”. These policies focus on building ecological corridors along the river, strengthening the conservation and ecological restoration of forests, wetlands and other ecosystems, and helping to enhance the service functions and productivity of various types of ecosystems. These policies pay attention to the construction of ecological corridors along the rivers, strengthening the conservation and ecological restoration of forests, wetlands and other ecosystems in mountainous areas, and helping to enhance the service functions and productivity of various ecosystems.
4.2 Policy implications and shortcomings
The long-term spatial correlation between land urbanization and GPP was confirmed in this study, and a macro-optimization strategy of the vegetation cover system and its productivity based on the land urbanization perspective should be given first priority. First of all, the development strategy of the Yangtze River Basin of “grasping great protection, not engaging in great development” should be further practiced. The development of a resource-saving and ecologically optimized industrial structure and production and living style should be promoted, as these factors largely determine the process of land urbanization and the demand for urban land. Secondly, strict curbing of disorderly development activities and nature modification activities in urban agglomerations should be emphasized. A range of ecological protection measures should be mentioned, such as the construction of urban green corridors, park cities and forest cities. More over, the spatial non-smoothness of the impact of land urbanization on GPP is confirmed, and it is time for differentiated urbanization management strategies and ecological restoration policies based on the mountainous areas around urban agglomerations and the main city zone to be further mentioned. In the case of main city zone, vegetation system productivity enhancement projects with more resilient and ecologically resilient should be implemented. These areas should take into account the ecological value, landscape integrity, and connectivity of the city in urban planning practices, improve the urban ecological environment management system, and emphasize the economic, residential, and ecological functions of main city zone. In the case of mountainous cities with high ecological quality and a relative lack of urbanization activities, further monitoring of risks and challenges is needed, despite the relatively minor impacts of land urbanization and the positive increase in regional GPP. These areas should continue to implement projects such as returning farmland to forest and planting artificial forests and grasses, strengthening ecological protection and management of vegetation in mountainous areas, and constructing green barriers for soil and water conservation, water protection and other vegetation ecological security. Adequate and sustainable financial investment is also important. The establishment of ecological compensation mechanisms, such as resource development compensation and watershed compensation, should be explored, and comprehensive forest and grassland ecological improvement and monitoring management systems should be strengthened. The key is to address the coordinated relationship between regional socio-economic development and ecological protection, increase the rate of intensive urban land use and the rate of integrated resource development, and support more effective and flexible policies for environmental restoration of vegetation growth.
This study has several shortcomings. First, the mediating mechanisms through which land urbanization affects GPP are insufficiently quantified. In the future, mediating variables such as ecosystem organization, structure, and provisioning service functions can be included, and the theoretical thinking of economics and geography can be combined to further explore the profound mechanism of the impact of LU on GPP. Second, GWTR well illustrates the long-term comprehensive impact of LU on GPP. However, in the future, we can focus on the comparative differences in the impacts of land urbanization patterns on vegetation cover systems and their productivity, and the paths that can be taken for land ecological management between MYRYU and other urban agglomerations in the Yangtze River Economic Belt, as well as in China and globally, from a broader perspective. This is important to further enrich the global value and macro-reference of the study. Third, population and economic urbanization as important areas of urbanization were not considered in this study. In the future, population, land and economic urbanization can be integrated to monitor the mechanisms of urbanization impacts on vegetation ecological resources, structure and function.
5 Conclusion
Previous studies have paid little attention to the associative relationship between land urbanization and vegetation GPP in MYRYU, and the spatially localized effects of land urbanization on GPP have been less comprehensively investigated. This study explores the spatio-temporal evolution of land urbanization and GPP in MYRYU from a township perspective based on multivariate remote sensing datasets. The spatial correlation properties of the two were further investigated, as well as the spatial effects of LU to produce driving impacts were analyzed based on the GTWR model. This study has drawn widespread attention to ecological engineering in mountainous areas and green urban planning in the main city zone of the city, and more importantly the findings provide evidence for a subject area and regionally differentiated management strategies for ecological restoration of vegetation in MRYRU. This leads to some interesting conclusions: (1) The average GPP of MYRYU grows overall to 1572.88 gCm-2a-1 from 2000 to 2020. High-value areas are distributed in the central and eastern mountainous areas, while low-value areas gradually form an “n”-shaped cluster distribution pattern, and the GPP of some areas in the main city zone has decreased significantly. (2) The negative spatial autocorrelation between LU and GPP shows a general upward trend, and Low-High is the dominant cluster type for the bivariate spatial correlation, followed by High-Low. (3) The impact of land urbanization on GPP has obvious spatial non-stationary distribution characteristics, and is dominated by negative impact. More importantly, based on the theory of “population-land-nature” complex ecosystem, our study explores the coordinated and optimized strategies and sustainable development paths of land urbanization and vegetation ecosystem productivity in MYRYU from the perspectives of land policy, green urban planning, and ecological engineering.
Data availability statement
The original contributions presented in the study are included in the article/supplementary files. Further inquiries can be directed to the corresponding author.
Author contributions
DP: Conceptualization, Data curation, Formal Analysis, Investigation, Methodology, Software, Writing – original draft. YC: Methodology, Project administration, Resources, Writing – review & editing. WW: Data curation, Funding acquisition, Investigation, Methodology, Project administration, Resources, Validation, Writing – review & editing. All authors contributed to the article and approved the submitted version.
Conflict of interest
The authors declare that the research was conducted in the absence of any commercial or financial relationships that could be construed as a potential conflict of interest.
Publisher’s note
All claims expressed in this article are solely those of the authors and do not necessarily represent those of their affiliated organizations, or those of the publisher, the editors and the reviewers. Any product that may be evaluated in this article, or claim that may be made by its manufacturer, is not guaranteed or endorsed by the publisher.
References
Asif R., Almagul T. (2022). Dynamic impacts of economic growth, energy use, urbanization, agricultural productivity, and forested area on carbon emissions: New insights from Kazakhstan. World Dev. Sustain. 1.
Chen W., Chi G. (2022). Urbanization and ecosystem services: The multi-scale spatial spillover effects and spatial variations. Land Use Policy 114, 105964. doi: 10.1016/j.landusepol.2021.105964
Chen W., Zhu D., Huang C., Ciais P., Yao Y., Friedlingstein P., et al. (2019). Negative extreme events in gross primary productivity and their drivers in China during the past three decades. Agric. For. Meteorol. 275, 47–58.
Dong Y., Peng F., Li H., Men Y. (2023). Spatial autocorrelation and spatial heterogeneity of underground parking space development in chinese megacities based on multisource open data. Appl. Geogr. 153, 102897. doi: 10.1016/j.apgeog.2023.102897
Dyvavani K K., Taibanganba W., Hitendra P., Ritika S., Subrata N. (2023). Improved gross primary productivity estimation using semi-empirical (PRELES) model for moist Indian sal forest. Ecol. Model. 475, 110175.
Fan R. X., Zhu X., Chen Z., Yu G., Zhang W., Han L., et al. (2023). A dataset of annual gross primary productivity over Chinese terrestrial ecosystems during 2000-2020. Sci. Data Bank V3, 1–20. doi: 10.57760/sciencedb.o00119.0007
Guan X., Shen H., Li X., Gan X., Zhang L. (2019). A long-term and comprehensive assessment of the urbanization-induced impacts on vegetation net primary productivity. Sci. Total Environ. 669, 342–352. doi: 10.1016/j.scitotenv.2019.02.361
Gui X., Wang L., Su X., Yi X., Chen X., Yao R., et al. (2021). Environmental factors modulate the diffuse fertilization effect on gross primary productivity across Chinese ecosystems. Sci. total Environ. 793, 148443. doi: 10.1016/J.SCITOTENV.2021.148443
Guido S., Christian K., Katerina T., David D., Jeffrey D. S. (2017). National baselines for the Sustainable Development Goals assessed in the SDG Index and Dashboards. Nat. Geosci. 10 (8), 547–555. doi: 10.1038/ngeo2985
Guo H., Zhou X., Dong Y., Wang Y., Li S. (2023). On the use of machine learning methods to improve the estimation of gross primary productivity of maize field with drip irrigation. Ecol. Model. 476, 110250. doi: 10.1016/j.ecolmodel.2022.110250
Hu Z., Piao S., Knapp A. K., Wang X., Peng S., Yuan W., et al. (2022). Decoupling of greenness and gross primary productivity as aridity decreases. Remote Sens. Environ. 279, 113120. doi: 10.1016/j.rse.2022.113120
Hu Q., Shen W., Zhang Z. (2023). How does urbanization affect the evolution of territorial space composite function? Appl. Geogr. 155, 102976. doi: 10.1016/J.APGEOG.2023.102976
Jia J., Gao Y., Qin B., Dungait J., Liu Y., Lu Y., et al. (2022). Evolving geographical gross primary productivity patterns in global lake systems and controlling mechanisms of associated phytoplankton communities since the 1950s. Earth-Science Rev. 234, 104221. doi: 10.1016/j.earscirev.2022.104221
Kim J., Kug J., Jeong S., Deborah N.H., Anna M., Christopher R. S., et al. (2017). Reduced North American terrestrial primary productivity linked to anomalous Arctic warming. Nat. Geosci. 10 (8), 572–576. doi: 10.1038/ngeo2986
Li W., Zhang Y., Yang C., Gong W., Wang C., Zhang R. (2022). Does producer services agglomeration improve urban green development performance of the yangtze river economic belt in China? Ecol. Indic. 145, 109581. doi: 10.1016/j.ecolind.2022.109581
Liu R., Dong X., Wang X., Zhang P., Liu M., Zhang Y. (2023). Relationship and driving factors between urbanization and natural ecosystem health in China. Ecol. Indic. 147, 109972. doi: 10.1016/j.ecolind.2023.109972
Liu X., Pei F., Wen Y., Li X., Wang S., Wu C., et al. (2019). Global urban expansion offsets climate-driven increases in terrestrial net primary productivity. Nat. Commun. 10 (1), 18866. doi: 10.1038/s41467-019-13462-1
Luo Q., Zhou J., Li Z., Yu B. (2020). Spatial differences of ecosystem services and their driving factors: A comparation analysis among three urban agglomerations in China’s Yangtze River Economic Belt. Sci. Total Environ. 725, 138452. doi: 10.1016/j.scitotenv.2020.138452
Ma L., Xiang L., Wang C., Chen N., Wang W. (2022). Spatiotemporal evolution of urban carbon balance and its response to new-type urbanization: A case of the middle reaches of the Yangtze River Urban Agglomerations, China. J. Clean. Prod. 380, 135122. doi: 10.1016/j.jclepro.2022.135122
Mu W., Zhu X., Ma W., Han Y., Huang H., Huang X. (2023). Impact assessment of urbanization on vegetation net primary productivity: A case study of the core development area in central plains urban agglomeration, China. Environ. Res. 229, 115995. doi: 10.1016/j.envres.2023.115995
Muntasir M., Nicholas A., Shabbir M. A., Khan U., Mahmud S. (2022). The impacts of renewable energy, financial inclusivity, globalization, economic growth, and urbanization on carbon productivity: Evidence from the net moderation and mediation effects of energy efficiency gains. Renewable Energy 196, 824–838. doi: 10.1016/J.RENENE.2022.07.012
Peng J., Wang X. Y., Liu Y. X., Zhao Y., Xu Z., Zhao M., et al. (2020). Urbanization impact on the supply-demand budget of ecosystem services: Decoupling analysis. Ecosystem Serv. 44, 101139.
Qin Z., Sha Z. (2023). Modeling the impact of urbanization and climate changes on terrestrial vegetation productivity in China by a neighborhood substitution analysis. Ecol. Model. 482, 110405. doi: 10.1016/j.ecolmodel.2023.110405
Su M., Fath D., Yang Z., Chen B., Liu G. (2013). Ecosystem health pattern analysis of urban clusters based on emergy synthesis: Results and implication for management. Energy Policy 59, 600–613. doi: 10.1016/j.enpol.2013.04.015
Tan X., Jia Y., Yang D., Niu C., Hao C. (2023). Turning points in the impact of earlier green-up on evapotranspiration and gross primary productivity in a semi-arid grassland watershed. J. Hydrol. 616, 128755. doi: 10.1016/j.jhydrol.2022.128755
Tian J., Cai D., Han K., Zhou K. (2022). Understanding peasant household’s land transfer decision-making: A perspective of financial literacy. Land Use Policy 119, 106189. doi: 10.1016/J.LANDUSEPOL.2022.106189
Tian A., Xu T., Gao J., Liu C., Han L. (2023). Multi-scale spatiotemporal wetland loss and its critical influencing factors in china determined using innovative grid-based GWR. Ecol. Indic. 149, 110144. doi: 10.1016/j.ecolind.2023.110144
Wang J., Delang C., Hou G., Gao L., Lu X. (2021). Net primary production increases in the Yangtze River Basin within the latest two decades. Global Ecol. Conserv. 26, e01497. doi: 10.1016/j.gecco.2021.e01497
Wang Y., Yu X., Zhao B., Xiong X., Li Y., Zhang M. (2022). Evaluation of ecological carrying capacity in Yangtze River Economic Belt and analysis of its spatial pattern evolution. Ecol. Indic. 144, 109535. doi: 10.1016/j.ecolind.2022.109535
Wei G., Bi M., Liu X., Zhang Z., He B. (2023a). Investigating the impact of multi-dimensional urbanization and FDI on carbon emissions in the belt and road initiative region: Direct and spillover effects. J. Clean. Prod. 384, 135608. doi: 10.1016/j.jclepro.2022.135608
Wei G., He B., Sun P., Liu Y., Li R., Ouyang X., et al. (2023b). Evolutionary trends of urban expansion and its sustainable development: Evidence from 80 representative cities in the belt and road initiative region. Cities 138, 104353. doi: 10.1016/j.cities.2023.104353
Xie X., Tian J., Wu C., Li A., Jin H., Bian J., et al. (2022). Long-term topographic effect on remotely sensed vegetation index-based gross primary productivity (GPP) estimation at the watershed scale. Int. J. Appl. Earth Observ. Geoinform. 108, 102755. doi: 10.1016/j.jag.2022.102755
Xu H., Zhang Z., Wu X., Wan J. (2023). Light use efficiency models incorporating diffuse radiation impacts for simulating terrestrial ecosystem gross primary productivity: A global comparison. Agric. For. Meteorol. 332, 109376. doi: 10.1016/J.AGRFORMET.2023.109376
Yang Z., Huang Y., Duan Z., Tang J. (2023). Capturing the spatiotemporal variations in the gross primary productivity in coastal wetlands by integrating eddy covariance, Landsat, and MODIS satellite data: A case study in the Yangtze Estuary, China. Ecol. Indic. 149, 110154. doi: 10.1016/j.ecolind.2023.110154
Yang X., Liu X. (2022). Carbon conduction effect and temporal-spatial difference caused by land type transfer in Chang-Zhu-Tan urban agglomeration from 1995 to 2018. Acta Ecol. Sin. 42 (4), 338–347. doi: 10.1016/j.chnaes.2022.02.004
Yang B., Zhang Z., Wu H. (2022). Detection and attribution of changes in agricultural eco-efficiency within rapid urbanized areas: A case study in the Urban agglomeration in the middle Reaches of Yangtze River, China. Ecol. Indic. 144, 109533. doi: 10.1016/j.ecolind.2022.109533
Zafar Z., Mehmood M. S., Zhai S., Zubair M., Sajjad M., Qin Y. (2023). Fostering deep learning approaches to evaluate the impact of urbanization on vegetation and future prospects. Ecol. Indic. 146, 109788. doi: 10.1016/j.ecolind.2022.109788
Zeng X., Ma Y., Ren J., He B. (2023). Assessing the network characteristics and structural effects of eco-efficiency: A case study in the urban agglomerations in the middle reaches of Yangtze River, China. Ecol. Indic. 150, 110169. doi: 10.1016/j.ecolind.2023.110169
Zhang T., Zhou J., Yu P., Li J., Kang Y., Zhang B. (2023). Response of ecosystem gross primary productivity to drought in northern China based on multi-source remote sensing data. J. Hydrol. 616, 128808. doi: 10.1016/j.jhydrol.2022.128808
Zhao Y., Peng J., Ding Z., Qiu S., Liu X., Wu J., et al. (2022). Divergent dynamics between grassland greenness and gross primary productivity across China. Ecol. Indic. 142, 109100. doi: 10.1016/j.ecolind.2022.109100
Zhong J., Liu J., Jiao L., Lian X., Xu Z., Zhou Z. (2021). Assessing the comprehensive impacts of different urbanization processes on vegetation net primary productivity in Wuhan, China, from 1990 to 2020. Sustain. Cities Soc. 75, 103295. doi: 10.1016/J.SCS.2021.103295
Zhou T., Liu H., Gou P., Xu N. (2023). Conflict or Coordination? measuring the relationships between urbanization and vegetation cover in China. Ecol. Indic. 147, 109993. doi: 10.1016/j.ecolind.2023.109993
Zhu Z., He Q. (2021). Spatio-temporal evaluation of the urban agglomeration expansion in the middle reaches of the Yangtze River and its impact on ecological lands. Sci. Total Environ. 790, 148150. doi: 10.1016/J.SCITOTENV.2021.148150
Keywords: land urbanization, vegetation gross primary productivity, GWTR, driving influence, Middle Reaches of the Yangtze River Urban Agglomeration (MRYRU)
Citation: Peng D, Chen Y and Wang W (2023) Spatio-temporal analysis of the impact of land urbanization on the gross primary productivity of vegetation in the Middle Reaches of the Yangtze River Urban Agglomeration: new evidence from the township scale. Front. Ecol. Evol. 11:1260641. doi: 10.3389/fevo.2023.1260641
Received: 18 July 2023; Accepted: 31 July 2023;
Published: 21 August 2023.
Edited by:
Xiao Ouyang, Hunan University of Finance and Economics, ChinaReviewed by:
Lang Yi, Hunan University of Finance and Economics, ChinaWenfang Pu, Huazhong Agricultural University, China
Copyright © 2023 Peng, Chen and Wang. This is an open-access article distributed under the terms of the Creative Commons Attribution License (CC BY). The use, distribution or reproduction in other forums is permitted, provided the original author(s) and the copyright owner(s) are credited and that the original publication in this journal is cited, in accordance with accepted academic practice. No use, distribution or reproduction is permitted which does not comply with these terms.
*Correspondence: Duming Peng, 15111018295@163.com