- 1Yangtze River Basin Ecological Environment Monitoring and Scientific Research Center, Yangtze River Basin Ecological Environment Supervision and Administration Bureau, Ministry of Ecology and Environment, Wuhan, China
- 2State Key Laboratory of Freshwater Ecology and Biotechnology, Institute of Hydrobiology, Chinese Academy of Sciences, Wuhan, China
- 3College of Advanced Agricultural Sciences, University of Chinese Academy of Sciences, Beijing, China
- 4Institute of Environment and Ecology, Shandong Normal University, Jinan, China
In the face of rapid environmental changes, understanding and monitoring biological traits and functional diversity are crucial for effective biomonitoring. However, when it comes to freshwater macroinvertebrates, a significant dearth of biological trait data poses a major challenge. In this opinion article, we put forward a machine-learning framework that incorporates phylogenetic conservatism and trait collinearity, aiming to provide a better vision for predicting macroinvertebrate traits in freshwater ecosystems. By adopting this proposed framework, we can advance biomonitoring efforts in freshwater ecosystems. Accurate predictions of macroinvertebrate traits enable us to assess functional diversity, identify environmental stressors, and monitor ecosystem health more effectively. This information is vital for making informed decisions regarding conservation and management strategies, especially in the context of rapidly changing environments.
1 Introduction
1.1 Freshwater biodiversity and sustainable development
Freshwater biodiversity provides vital natural resources for humans in economic, cultural, aesthetic, scientific, and educational terms. Its conservation and management are of paramount importance to the interests and well-being of all humans, nations and governments. However, despite conservation efforts, freshwater biodiversity is experiencing rapid declines at regional or global scales, due to increasing intensity of disturbances from human activity, biotic pressure, and environmental changes (Mouillot et al., 2013). As a consequence, biodiversity loss poses a threat to the sustainability of ecological processes and the provision of ecosystem services (Renault et al., 2022). Thus, biodiversity challenges become one of the most important issues in the Sustainable Development Goals (SDGs). Among the SDGs, the sustainable use of marine and aquatic resources and terrestrial ecosystems are most closely related with biodiversity. Biodiversity is an important indicator to evaluate the sustainable development of human society since it provides the essential resource for human surviving. The balance between biodiversity conservation and utilization is crucial for sustainable development.
Documenting losses of biodiversity, diagnosing their causes, and finding solutions are key issues in freshwater ecology (Strayer and Dudgeon, 2010). Traditional freshwater biodiversity studies mainly focused on taxonomic diversity. Although some kinds of new technologies were implemented, such as meta-barcoding (Serrana et al., 2018) and single-molecule real-time (SMRT) sequencing (Zhang et al., 2020), which obtain species taxonomic annotation by aligning environmental sequences to reference databases, analyses of these new methods still primarily focused on species diversity. It is argued that taxonomic diversity alone cannot explain the observed patterns that respond to environmental disturbances (Gagic et al., 2015).
1.2 Biological trait, functional diversity, macroinvertebrates, and global change
Despite a range of conservation measures being implemented, biodiversity loss continues to occur widely in the context of habitat destruction (Visconti et al., 2011) and global changes (Maclean and Wilson, 2011). So, it cannot wait to develop a method for quantifying and predicting the impact of disturbance on biodiversity patterns (Mouillot et al., 2013).
It has been confirmed that the response to disturbances and other environmental conditions depends on the traits (including life history, behavior, physiology, morphology, ecology, environmental preferences, and tolerances or sensitivities) of the species (Mouillot et al., 2013; Gagic et al., 2015). Functional traits have been shown to serve as important characterizations of community or ecosystem function in response to various disturbances (Tilman et al., 1997; Petchey et al., 2004; Verberk et al., 2013). Furthermore, researches based on species traits can provide more information than studies merely on classical taxonomy (Barnett et al., 2007; Luo Q. et al., 2022). So, the focus of related research has also shifted from taxonomic diversity to functional diversity (Renault et al., 2022).
Macroinvertebrates, characterized by their rich diversity, wide distribution, and environmental sensitivity, have been long utilized to study ecological responses, such as hydrological disturbances, based on their functional traits (Townsend et al., 1997). Until now, studies on macroinvertebrates’ functional diversity have kept exploding for more than 20 years. Recently, research has delved into the typical number of traits considered for estimating functional diversity and the specific traits commonly used for index calculation (Ao et al., 2023). Some studies summarized the macroinvertebrate trait databases that have already been produced (Kefford et al., 2020). These research foundations offered the possibility of constructing a relatively well-developed framework based on macroinvertebrate traits to quantitatively predict the impact of disturbance on biodiversity patterns. However, acquiring comprehensive trait datasets for organisms demands substantial sampling efforts, consequently leading to frequent occurrence of missing data.
1.3 Limitation of current applications and our intention
We propose a conceptual framework that integrates machine learning, phylogenetic conservatism, and trait collinearity to address the paucity of biological trait data for freshwater macroinvertebrates (Kefford et al., 2020). This lack hinders our understanding of functional diversity and the effects of global change. To predict missing traits, we propose a machine-learning model with predictors of phylogenetic information and trait collinearity. By incorporating these predictors, we can improve predictions by accounting for 1) phylogenetic distances among species and 2) associations among traits within species or taxonomic groups. This advance contributes to understanding functional diversity, assessing the impacts of global change, and guiding conservation and management efforts for freshwater ecosystems. In the position paper, we then discuss the current state and our proposed framework for addressing missing trait data.
2 Current state to address the lack of trait database
2.1 Current frameworks
By addressing the lack of biological trait data, the current frameworks rely on the selection of traits available in freshwater macroinvertebrates (Ao et al., 2023). This limitation can be overcome by predicting biological traits based on phylogenetic conservatism and using mathematical tools such as machine learning (Debastiani et al., 2021). Phylogenetic conservatism suggests that closely related species share similar trait values due to their shared evolutionary history. Machine learning algorithms analyze large datasets and extract patterns from complex biological data. By integrating machine learning into the framework, researchers can estimate missing trait values based on known trait values of closely related species. By leveraging machine learning’s ability to identify patterns and correlations among traits, this approach can enable researchers to gain insights into the ecological implications of these traits and their impact on ecosystem dynamics.
2.2 Phylogenetic conservatism
As species traits are integrated as a part of a hierarchically structured phylogeny (Felsenstein, 1985), and given the propensity for greater trait similarity among closely related species compared to those more distantly related (Pagel, 1999), coupled with the conservatism of the phylogeny, it gives the theoretical basis for linking phylogenetic information and traits. So, some data imputation methods encompassed or based on phylogenetic information had been developed, such as Phylopars, which utilizes phylogeny and allometric relationships among traits (Bruggeman et al., 2009), and Phylogenetic Eigenvector Maps (PEM) (Guenard et al., 2013).
The methods that take phylogenetic information of species into account have been considered to be potentially powerful ways to complete trait data imputation (Swenson, 2014). Debastiani et al. (2021) proposed a framework that integrates phylogenetic information with imputation methods employing missForest and assessed the performance of the missForest algorithm for imputing species trait values by incorporating phylogenetic information. The results showed that the inclusion of phylogenetic vectors into the missForest algorithm leads to a substantial improvement in the imputation of missing values under some certain conditions. It demonstrates the promising application of incorporating phylogenetic conservatism into machine learning frameworks to predict macroinvertebrate traits.
2.3 Machine learning in ecology and environmental sciences
Machine learning methods possess a robust nonlinear modeling capability, making them particularly effective in detecting and describing structural patterns within large datasets, as well as providing relative importance values among independent variables (Biau and Scornet, 2016). They had been widely applied for species distribution pattern prediction and constrained environmental factors detection in community-level studies in ecology and environment sciences (Smith and Carstens, 2020). The prediction function made machine learning methods being used in extremely wide areas such as satellite data processing (Kim et al., 2014), weather and climate prediction (Watson-Parris, 2021), air quality forecasting (Fu et al., 2023), and monitoring of snow, ice, and forests (Luo J. et al., 2022). The nonlinear modeling capability of machine learning methods made them powerful in genomic prediction for non-linear traits. Song et al. (2023) had performed Bayesian threshold model and machine learning methods to improve the accuracy of genomic prediction for ordered categorical traits in fish. Fish egg color was predicted with both methods. Machine learning methods showed higher prediction accuracies than Bayesian methods. In wheat leaf traits monitoring studies, machine learning methods could provide comparatively precise and robust prediction of leaf parameters based on high-resolution satellite imagery data (Jamali et al., 2023). Li et al. (2020) extended those methods to population genetic studies. The relative importance was used to determine environmental factors that drive adaptive divergence.
3 Proposed framework with phylogenetic conservatism, trait collinearity, and machine learning
3.1 The proposed framework
We propose an innovative framework that combines phylogenetic conservatism, trait collinearity, and machine learning to revolutionize the prediction and understanding of biological traits (Figure 1). The analysis of an incomplete macroinvertebrate trait set using this framework consists of the following steps: (1) View the data set and clarify missing data. (2) Get the phylogenetic distances between related species based on phylogenetic data. (3) Deduce the value of the missing trait by phylogenetic distances through machine learning. (4) Get the co-occurrence relationships between related traits and add it to machine learning to estimate the deduced value. By incorporating the construct of trait collinearity along with known features such as phylogenetic conservatism and machine learning, this integrated framework enhances our ability to accurately estimate missing trait values. Its applications span across ecology, evolution, and conservation biology, deepening our understanding of trait evolution, functional diversity, and the impacts of global change. Furthermore, the framework informs conservation and management strategies by highlighting traits crucial for species resilience. Through this integration, valuable insights into trait variation and its ecological and evolutionary significance are unlocked.
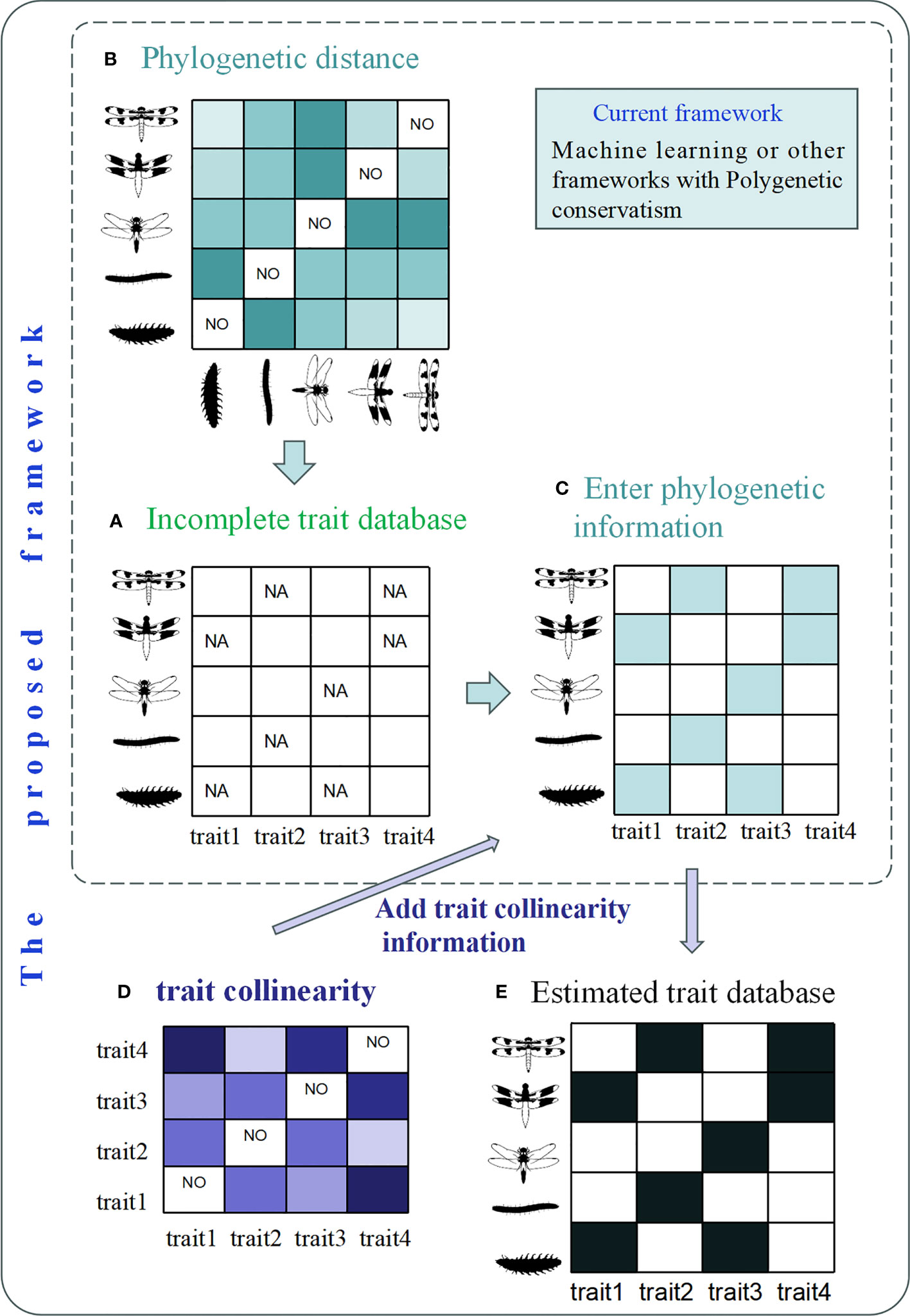
Figure 1 Current framework (with phylogenetic data) and prospect framework (with addition of trait collinearity information): (A) analysis of incomplete trait sets in macroinvertebrate, NA representing missing trait information. (B) Phylogenetic distance between species. (C) The value of the missing trait is deduced by adding phylogenetic data through machine learning (light blue data box). (D) Co-occurrence relationships between traits. (E) The inclusion of information on trait collinearity makes the derived values (Black data box) of missing traits more reliable. Silhouettes were obtained from phylopic.org under the public domain licenses.
3.2 Trait collinearity
The diversity in functional traits within species is shaped by both genetic differentiation and phenotypic plasticity (Albert et al., 2010). Additionally, it mirrors the evolutionary past and the species’ adjustments to environmental conditions (Diaz and Cabido, 2001). Given the intricate nature of the origins of functional diversity, employing a multivariate framework that combines the phylogenetic aspects of biodiversity with trait-based methodologies becomes crucial (Felsenstein, 1985). To complete trait datasets, the use of data imputation methods with phylogenetic information of species is considered a potentially effective approach until more accurate trait information is obtained (Swenson, 2014). The foundation for linking evolutionary traits to traits lies in the recognition that species traits are interrelated rather than independent and they are embedded within a hierarchical phylogenetic tree (Felsenstein, 1985). Studies of functional variation are based on the idea of functional trait covariation(Grime et al., 1997), a model of covariation that can define general ecological strategies (Reich et al., 2003). The study of functional variation has been more widely explored in the field of botany than in aquatic organisms. For example, covariation patterns among leaf traits (such as the leaf economic spectrum) have been linked to strategies for efficient resource acquisition and resource conservation (Wright et al., 2004).
4 Summary
Here, we propose integrating machine learning, phylogenetic conservatism, and trait collinearity into a conceptual framework. The lack of biological trait data for freshwater macroinvertebrates impedes our exploration of functional diversity and understanding of global change impacts (Kefford et al., 2020). Thus, there is a global effort to develop methods for predicting missing traits. To bridge this gap, we recommend incorporating trait collinearity into the existing framework that already considers machine learning and phylogenetic conservatism. Trait collinearity involves the correlation or association of certain traits within a species or taxonomic group. By integrating this concept, we can enhance our ability to predict missing traits. Machine learning algorithms are effective at extracting patterns from complex biological data, making them valuable tools. By training these algorithms with existing trait data and incorporating phylogenetic information, predictive models can estimate missing traits based on known trait correlations. This approach leverages the similarity of trait values among closely related species due to phylogenetic conservatism. Including trait collinearity in the framework would improve predictions by considering interrelationships among traits within species or taxonomic groups. Accounting for these associations enhances the accuracy of trait predictions and provides a comprehensive understanding of functional diversity. By integrating trait collinearity and phylogenetic conservatism into machine learning models, we can leverage available data to predict missing biological traits in freshwater macroinvertebrates. This advancement significantly contributes to our understanding of functional diversity, enables better assessments of global change impacts, guides conservation efforts, and informs effective management strategies for freshwater ecosystems, promoting their long-term sustainability.
Author contributions
SL: Conceptualization, Funding acquisition, Software, Writing – original draft, Writing – review & editing. QL: Conceptualization, Software, Writing – original draft. RL: Conceptualization, Writing – original draft, Writing – review & editing. BL: Conceptualization, Writing – original draft, Writing – review & editing.
Funding
This research was funded by the National Key R&D Program of China (No. 2021YFC3200105). The author(s) declare financial support was received for the research, authorship, and/or publication of this article.
Acknowledgments
We thank the reviewers for their many insightful comments and suggestions.
Conflict of interest
The authors declare that the research was conducted in the absence of any commercial or financial relationships that could be construed as a potential conflict of interest.
Publisher’s note
All claims expressed in this article are solely those of the authors and do not necessarily represent those of their affiliated organizations, or those of the publisher, the editors and the reviewers. Any product that may be evaluated in this article, or claim that may be made by its manufacturer, is not guaranteed or endorsed by the publisher.
References
Albert C. H., Thuiller W., Yoccoz N. G., Soudant A., Boucher F., Saccone P., et al. (2010). Intraspecific functional variability: extent, structure and sources of variation. J. Ecol. 98, 604–613. doi: 10.1111/j.1365-2745.2010.01651.x
Ao S., Chiu M.-C., Lin X., Cai Q. (2023). Trait selection strategy for functional diversity in freshwater systems: A case framework of macroinvertebrates. Ecol. Indic. 153, 110450. doi: 10.1016/j.ecolind.2023.110450
Barnett A. J., Finlay K., Beisner B. E. (2007). Functional diversity of crustacean zooplankton communities: towards a trait-based classification. Freshw. Biol. 52, 796–813. doi: 10.1111/j.1365-2427.2007.01733.x
Biau G., Scornet E. (2016). A random forest guided tour. Test 25, 197–227. doi: 10.1007/s11749-016-0481-7
Bruggeman J., Heringa J., Brandt B. W. (2009). PhyloPars: estimation of missing parameter values using phylogeny. Nucleic Acids Res. 37, W179–W184. doi: 10.1093/nar/gkp370
Debastiani V. J., Bastazini V. A. G., Pillar V. D. (2021). Using phylogenetic information to impute missing functional trait values in ecological databases. Ecol. Inf. 63, 101315. doi: 10.1016/j.ecoinf.2021.101315
Diaz S., Cabido M. (2001). Vive la difference: plant functional diversity matters to ecosystem processes. Trends Ecol. Evol. 16, 646–655. doi: 10.1016/s0169-5347(01)02283-2
Felsenstein J. (1985). Phylogenies and the comparative method. Am. Nat. 125, 1–15. doi: 10.1086/284325
Fu L., Li J., Chen Y. (2023). An innovative decision making method for air quality monitoring based on big data-assisted artificial intelligence technique. J. Innov. Knowl. 8. doi: 10.1016/j.jik.2022.100294
Gagic V., Bartomeus I., Jonsson T., Taylor A., Winqvist C., Fischer C., et al. (2015). Functional identity and diversity of animals predict ecosystem functioning better than species-based indices. Proc. R. Soc. B Biol. Sci. 282. doi: 10.1098/rspb.2014.2620
Grime J. P., Thompson K., Hunt R., Hodgson J. G., Cornelissen J. H. C., Rorison I. H., et al. (1997). Integrated screening validates primary axes of specialisation in plants. Oikos 79, 259–281. doi: 10.2307/3546011
Guenard G., Legendre P., Peres-Neto P. (2013). Phylogenetic eigenvector maps: a framework to model and predict species traits. Methods Ecol. Evol. 4, 1120–1131. doi: 10.1111/2041-210x.12111
Jamali M., Soufizadeh S., Yeganeh B., Emam Y. (2023). Wheat leaf traits monitoring based on machine learning algorithms and high-resolution satellite imagery. Ecol. Inf. 74, 101967. doi: 10.1016/j.ecoinf.2022.101967
Kefford B. J., Botwe P. K., Brooks A. J., Kunz S., Marchant R., Maxwell S., et al. (2020). An integrated database of stream macroinvertebrate traits for Australia: Concept and application. Ecol. Indic. 114, 106280. doi: 10.1016/j.ecolind.2020.106280
Kim Y. H., Im J., Ha H. K., Choi J.-K., Ha S. (2014). Machine learning approaches to coastal water quality monitoring using GOCI satellite data. GIsci. Remote Sens. 51, 158–174. doi: 10.1080/15481603.2014.900983
Li B., Yaegashi S., Carvajal T. M., Gamboa M., Chiu M.-C., Ren Z., et al. (2020). Machine-learning-based detection of adaptive divergence of the stream mayflyEphemera strigatapopulations. Ecol. Evol. 10, 6677–6687. doi: 10.1002/ece3.6398
Luo Q., Chiu M.-C., Tan L., Cai Q. (2022). Hydrological season can have unexpectedly insignificant influences on the elevational patterns of functional diversity of riverine macroinvertebrates. Biology 11. doi: 10.3390/biology11020208
Luo J., Dong C., Lin K., Chen X., Zhao L., Menzel L. (2022). Mapping snow cover in forests using optical remote sensing, machine learning and time-lapse photography. Remote Sens. Environ. 275. doi: 10.1016/j.rse.2022.113017
Maclean I. M. D., Wilson R. J. (2011). Recent ecological responses to climate change support predictions of high extinction risk. Proc. Natl. Acad. Sci. U. S. A. 108, 12337–12342. doi: 10.1073/pnas.1017352108
Mouillot D., Graham N. A. J., Villeger S., Mason N. W. H., Bellwood D. R. (2013). A functional approach reveals community responses to disturbances. Trends Ecol. Evol. 28, 167–177. doi: 10.1016/j.tree.2012.10.004
Pagel M. (1999). Inferring the historical patterns of biological evolution. Nature 401, 877–884. doi: 10.1038/44766
Petchey O. L., Hector A., Gaston K. J. (2004). How do different measures of functional diversity perform? Ecology 85, 847–857. doi: 10.1890/03-0226
Reich P. B., Wright I. J., Cavender-Bares J., Craine J. M., Oleksyn J., Westoby M., et al. (2003). The evolution of plant functional variation: Traits, spectra, and strategies. Int. J. Plant Sci. 164, S143–S164. doi: 10.1086/374368
Renault D., Hess M. C. M., Braschi J., Cuthbert R. N., Sperandii M. G., Bazzichetto M., et al. (2022). Advancing biological invasion hypothesis testing using functional diversity indices. Sci. Total Environ. 834. doi: 10.1016/j.scitotenv.2022.155102
Serrana J. M., Yaegashi S., Kondoh S., Li B., Robinson C. T., Watanabe K. (2018). Ecological influence of sediment bypass tunnels on macroinvertebrates in dam-fragmented rivers by DNA metabarcoding. Sci. Rep. 8, 10185. doi: 10.1038/s41598-018-28624-2
Smith M. L., Carstens B. C. (2020). Process-based species delimitation leads to identification of more biologically relevant species. Evolution 74, 216–229. doi: 10.1111/evo.13878
Song H., Dong T., Yan X., Wang W., Tian Z., Hu H. (2023). Using Bayesian threshold model and machine learning method to improve the accuracy of genomic prediction for ordered categorical traits in fish. Agric. Commun. 1, 100005. doi: 10.1016/j.agrcom.2023.100005
Strayer D. L., Dudgeon D. (2010). Freshwater biodiversity conservation: recent progress and future challenges. J. North Am. Benthol. Soc. 29, 344–358. doi: 10.1899/08-171.1
Swenson N. G. (2014). Phylogenetic imputation of plant functional trait databases. Ecography 37, 105–110. doi: 10.1111/j.1600-0587.2013.00528.x
Tilman D., Naeem S., Knops J., Reich P., Siemann E., Wedin D., et al. (1997). Biodiversity and ecosystem properties. Science 278, 1866–1867. Retrieved from <Go to ISI>://WOS:A1997YL00200004. doi: 10.1126/science.278.5345.1865c
Townsend C. R., Scarsbrook M. R., Doledec S. (1997). Quantifying disturbance in streams: alternative measures of disturbance in relation to macroinvertebrate species traits and species richness. J. North Am. Benthol. Soc. 16, 531–544. doi: 10.2307/1468142
Verberk W. C. E. P., van Noordwijk C. G. E., Hildrew A. G. (2013). Delivering on a promise: integrating species traits to transform descriptive community ecology into a predictive science. Freshw. Sci. 32, 531–547. doi: 10.1899/12-092.1
Visconti P., Pressey R. L., Giorgini D., Maiorano L., Bakkenes M., Boitani L., et al. (2011). Future hotspots of terrestrial mammal loss. Philos. Trans. R. Soc. B Biol. Sci. 366, 2693–2702. doi: 10.1098/rstb.2011.0105
Watson-Parris D. (2021). Machine learning for weather and climate are worlds apart. Philos. Trans. R. Soc. A Math. Phys. Eng. Sci. 379. doi: 10.1098/rsta.2020.0098
Wright I. J., Reich P. B., Westoby M., Ackerly D. D., Baruch Z., Bongers F., et al. (2004). The worldwide leaf economics spectrum. Nature 428, 821–827. doi: 10.1038/nature02403
Keywords: biodiversity, global change, sustainable development, phylogenetic tree, trait
Citation: Li S, Luo Q, Li R and Li B (2023) Incorporating phylogenetic conservatism and trait collinearity into machine learning frameworks can better predict macroinvertebrate traits. Front. Ecol. Evol. 11:1260173. doi: 10.3389/fevo.2023.1260173
Received: 17 July 2023; Accepted: 11 August 2023;
Published: 29 August 2023.
Edited by:
Jhih-Rong Liao, Tokyo Metropolitan University, JapanCopyright © 2023 Li, Luo, Li and Li. This is an open-access article distributed under the terms of the Creative Commons Attribution License (CC BY). The use, distribution or reproduction in other forums is permitted, provided the original author(s) and the copyright owner(s) are credited and that the original publication in this journal is cited, in accordance with accepted academic practice. No use, distribution or reproduction is permitted which does not comply with these terms.
*Correspondence: Ruiwen Li, lrw1986@126.com; Bin Li, binglee527@sdnu.edu.cn