- 1College of Resources and Environment, Xinjiang Agricultural University, Urumqi, China
- 2Xinjiang Key Laboratory of Soil and Plant Ecological Processes, Xinjiang Agricultural University, Urumqi, China
- 3Henan Normal University, Henan, China
- 4Key Laboratory of Coastal Science and Integrated Management, First Institute of Oceanography, Ministry of Natural Resources, Qingdao, China
- 5State Key Laboratory for Soil Erosion and Dryland Farming on the Loess Plateau, Institute of Soil and Water Conservation, Northwest A&F University, Yangling Shaanxi, China
- 6College of Plant Protection, Jilin Agricultural University, Changchun, China
Climate anomalies and human disturbances exert complex effects on regional carbon sequestration (CS), causing ecosystem CS to either increase or decrease due to factors such as vegetation greening, climate extremes, land use/land cover (LULC) changes, and farming systems. This study employs the patch-level land use simulation model to forecast changes in various land-use types in the Xinjiang Uyghur Autonomous Region, China, between 2020 and 2060 under different climatic conditions. The Intergovernmental Panel on Climate Change has proposed shared socioeconomic pathways (SSPs) as potential socioeconomic and environmental trajectories that might influence the region’s future land-use patterns. Three salient findings emerged: (1) LULC displayed considerable variation across future climate scenarios, with a notable rise in forest and grassland cover and a marked decrease in cropland areas under the SSP126 scenario. Conversely, the SSP585 scenario witnessed a substantial cropland expansion, paralleled by a decrease in forest and grassland areas; (2) CS exhibited significant disparities across different future climate scenarios. Xinjiang achieved a peak CS of 9.81 Pg between 2020 and 2060 under the SSP126 scenario, marking an increase of 335.22 Tg in 2060 compared to that in 2020. The SSP585 scenario registered the lowest CS at 9.41 Pg, indicating a decline of 50.07 Tg in 2060 relative to 2020; (3) The impacts of longitude and latitude on CS in Xinjiang predominantly arose from shifts in forest area and vegetation cover based on latitude, which positively influenced CS. In contrast, a decrease in vegetation cover and a subsequent decline in CS were evident with increasing longitude, particularly in the Altai, Tianshan, and Kunlun Mountains. These findings hold significant implications for formulating CS management strategies for terrestrial ecosystems and enhancing ecological preservation in Xinjiang.
1 Introduction
Land ecosystems play a pivotal role in ensuring the long-term sustainability, viability, and growth of human societies by absorbing carbon dioxide and mitigating the influence of climate change (Reich et al., 2020). Thus, safeguarding the health of land ecosystems is a shared responsibility of the international community. Given its crucial status among the world’s major developing nations, China has a critical role in mitigating the effects of climate change by adopting proactive measures for carbon emission reduction. In 2019, the Chinese government committed to reaching a carbon peak by 2030 and achieving carbon neutrality by 2060, emphasizing its determination to address climate change adaptation on a global scale. China’s commitment to reducing carbon emissions mitigates global climate change and promotes a more sustainable and environmentally friendly approach to economic development by pursuing carbon peak and carbon neutrality. Sustainable development is intrinsically linked to the responsible management of natural resources, especially land use/land cover (LULC) types. Different LULC types profoundly impact carbon sequestration (CS) within land ecosystems, making them an essential component of efforts to achieve carbon neutrality and protect ecological integrity (Turner, 1997).
Rapid socioeconomic development in China has heightened the demand for food and living space, prompting humans to undertake various activities such as deforestation and fossil-fuel emissions. However, these actions have caused significant damage and adversely affected ecosystems, undermining their health and resilience (Malek et al., 2018). Furthermore, the emission of greenhouse gases resulting from global climate change substantially affects ecosystem processes and patterns (Li et al., 2018; Rodríguez-Echeverry et al., 2018; Udara Willhelm Abeydeera et al., 2019). These impacts can be unpredictable and far-reaching, posing considerable risks to global ecosystems, human survival, and economic development (Cramer et al., 2018). Land use/land cover is a key factor influenced by human activities that directly affect the integration and status of ecosystems and their ability to provide vital ecosystem services (ES) (Nelson et al., 2009; Huang et al., 2020). This factor is so critical that it has been emphasized in various studies (Lambin and Meyfroidt, 2011). To address the significant challenges and threats facing different social-ecological systems, the United Nations established 17 sustainable development goals (SDGs) (Barnaud et al., 2018). Evaluating ES and valuing natural capital are subject to the extreme impacts of LULC changes. As industrialization accelerates, the primary catalyst for economic growth is transitioning from agriculture to industry. This trend is accompanied by a continuous rise in urbanization levels. Swift changes in LULC stemming from these trends significantly impact the functions of terrestrial ecosystems (TE) and the capacity of these ecosystems to sequester carbon (Li et al., 2019; Liao et al., 2020).
As China’s socioeconomic development advances, the country must contend with numerous challenges, such as ecological degradation and energy crises, in its pursuit of sustainable development. Recent research has shown a strong correlation between environmental pollution and atmospheric CO2 concentration (Molotoks et al., 2018; Tian et al., 2022; Yi et al., 2022). Enhancing CS in TE is considered one of the most effective and environmentally sustainable options for mitigating climate change. The Xinjiang Uygur Autonomous Region, situated in a semi-arid and arid region, has seen temperature and precipitation exhibit a fluctuating upward trend in recent years (He et al., 2022; Yao et al., 2022). The region may experience continued increases in temperature and precipitation in the future due to global climate change. Given its location in a typical arid and semi-arid region, the Xinjiang area has a fragile ecosystem because of the vegetation’s high sensitivity and the influence of environmental changes on soil. Descriptions of future scenarios can facilitate establishing effective connections between future LULC simulation and land-use regulation optimization (Zhu et al., 2020; Huang et al., 2023). Previous studies investigating land-use change have often focused on historical driving factors and overlooked the potential impacts of future climate change and socioeconomic transformations. This focus can negatively affect the accuracy of land-use simulation results, hindering the formulation of effective strategies for land-use management and recommendations.
The Coupled Model Intercomparison Project (CMIP) allows global climate scientists to share, analyze, and compare the latest simulation results of global climate models. This model data will contribute to future global climate research, and analyses based on these data will serve as the foundation for future climate assessments and negotiations. CMIP6 is an extensive project with a record number of participating models, advanced scientific experiments, and an unprecedented amount of simulated data. Recent advances in CMIP6 have shown that integrating the SSPs with the representative concentration pathways (RCPs) can provide reliable scenarios for predicting future global climate and socioeconomic shifts (Su et al., 2021). The SSP scenarios include SSP1, a people-centered pathway based on sustainable development with strict land-use restrictions (Van Vuuren et al., 2017); SSP2, a pathway based on historical technological, economic, and social development trends emphasizing economic prosperity and social progress but lacking urgent action for climate change and environmental protection (Fricko et al., 2017); and SSP5, a fossil-fuel development route characterized by rapid global economic expansion (Kriegler et al., 2017). Under SSP5, rapid global economic growth and significant challenges from fossil-fuel development occur. While tropical rainforest deforestation is expected to slow down, LULC changes under this pathway are not adequately controlled. Crop yields are high, but there’s widespread use of unhealthy animal feed and waste (Popp et al., 2017). Coupling prospective climate and socioeconomic parameters with the SSP-RCP scenarios to simulate land-use configurations is vital for determining the impact of LULC changes on CS in Xinjiang, highlighting the importance of considering land-use patterns when predicting future CS.
In this study, the CMIP6-patch level land-use simulation (PLUS) model is employed to simulate land-use scenarios, while a random forest (RF) model is used to estimate the CS potential of Xinjiang and predict its future changes. The research findings offer crucial insights into the future trends of CS, promoting coordinated development, achieving carbon neutrality, and advancing socioeconomic development and ecological restoration. There are three main objectives: first, to analyze changes in Xinjiang land use under three distinct future scenarios (SSP126, SSP245, and SSP585); second, to evaluate and map the spatial distribution of CS services provided by ecosystems under these scenarios from 2020 to 2060; and third, to examine the effect of changes in longitude and latitude on the CS of Xinjiang’s TE. The findings provide valuable insights for future land-use planning, achieving carbon neutrality, socioeconomic development, and land- use.
2 Materials and methods
2.1 Study area
Located in inland China, Xinjiang is in the northwest of the country, with geographic coordinates of 73°40′−96°18′ E, 34°25′−48°10′ N. It stretches 2,000 km in the east-west direction and has a maximum width of 1,650 km. Approximately one-sixth of China’s total land area is in Xinjiang, which covers 1.66 million km2 (Figure 1). Xinjiang has a temperate continental climate characterized by significant daily temperature fluctuations, cold winters, and hot summers. The region generally experiences an average annual precipitation of less than 100 mm. Xinjiang has abundant sunshine, with an annual average of 2,400−3,200 hours of sunshine. Over the past two decades, Xinjiang has emerged as a significant center for political, economic, and cultural interactions within the “Belt and Road” region. The population growth rate is 1.5% annually, increasing from 18.49 million in 2000 to 25.23 million in 2020. The average annual growth rate of Xinjiang’s domestic gross product (GDP) is 12.2%. CS in Xinjiang has been impacted by ongoing urbanization and LULC changes (Shi et al., 2021). Therefore, studying the CS potential in Xinjiang is extremely important.
2.2 Data and processing
This study utilized research data collected from various sources, including basic geographic, land use, soil, digital elevation, socioeconomic, and future climate data (Table 1). The data sources used were as follows: (1) Data were collected from the Chinese Academy of Sciences Resource and Environmental Science Data Center (www.resdc.cn) (Chen et al., 2020a; Yao et al., 2021). The land-use data were classified into six categories: cropland, forest, grassland, water bodies, built-up land, and unused land (Jiyuan et al., 2002); (2) The digital elevation model (DEM) data were sourced from the Geographic Spatial Data Cloud and subsequently employed to extract slope data. The soil type data were obtained from the Food and Agriculture Organization of the United Nations; (3) The study used population spatial distribution and GDP data collected according to previous studies (Chen et al., 2020b); (4) The vector datasets depicting the distances to major and secondary roads were obtained from Open Street Map (https://www.openstreetmap.org), while the vector dataset for river systems was obtained from the National Geospatial Information Service System; (5) Urban night light data for China were obtained through the China Research Data Services Platform; (6) The climate data from future projections utilized in the present study were sourced from the CMIP6 dataset; and (7) This study analyzed the density of vegetation and soil organic carbon in China, with carbon density data collected from studies published between 2004 and 2014 through relevant experiments (Xu et al., 2019a; Xu et al., 2019b).
To enhance the robustness of the model and the consistency and accuracy of spatial data, the researchers used the Weather Research and Forecasting Model (WRF) method and ArcGIS 10.7’s clipping and resampling tools to process the model driving factors, land-use data, and CMIP6 data. These data were then converted into raster files with a unified coordinate system and a resolution of 250 × 250 m (Shi et al., 2022).
2.3 The CMIP6-PLUS model
2.3.1 The CMIP6
The CMIP is an important international multi-model climate research activity that has undergone five development stages. The project has ushered in a new era of research in the field of climate science and advanced assessments of global climate change. In contrast to CMIP5, CMIP6 incorporates various SSP-RCP scenarios to emphasize the influence of diverse socioeconomic development patterns on climate change.
In the Shared Socioeconomic Pathway, which considers different combinations of population growth, economic development, and climate mitigation efforts, three scenarios (SSP126, SSP245, and SSP585) were selected. These scenarios are relatively representative: SSP126 emphasizes sustainability and climate mitigation, SSP245 represents a middle-of-the-road approach, and SSP585 reflects a future with high emissions and limited climate action, leading to varying degrees of climate change and environmental impact. These scenarios are crucial for assessing the potential outcomes and challenges of different socioeconomic and environmental trajectories.
This study aims to evaluate the potential impact of climate change scenarios on future LULC changes in Xinjiang. Four major driving factors were considered: population, GDP, temperature, and precipitation. In order to achieve this, the CNRM-CM6-1 model was used to provide both temperature and precipitation data for the three scenarios (Hurtt et al., 2020).
2.3.2 The WRF model for CMIP6 downscaling
The WRF model is a commonly used atmospheric model for simulating regional and mesoscale climate patterns (Powers et al., 2017). In the context of CMIP6, WRF serves as a dynamic downscaling tool for high-resolution regional climate simulations. It employs non-hydrostatic compressible equations to simulate the movement of the atmosphere on a horizontal grid. In CMIP6, WRF is utilized for regional climate simulation in two ways: spectral nudging and dynamic downscaling techniques. The former constrains regional simulations to follow the large-scale atmospheric circulation of global models, ensuring consistency between high-resolution models and global models, while the latter allows regional models to simulate fine-scale processes freely, using large-scale data from global models as initial and boundary conditions. Both methods have been applied in CMIP6 for simulating regional climate in various areas. The results indicate that WRF can offer high-resolution regional climate simulations and capture the fine-scale features of the climate system, such as temperature gradients, precipitation patterns, and extreme events (Yu et al., 2023). The application of WRF in CMIP6 introduces new tools and methods for climate research and provides scientific support for addressing climate change. This study utilized the dynamic downscaling method to downscale the CMIP6 data.
2.3.3 The PLUS model
Cellular automata (CA) models are commonly used to model complex LULC systems. However, previous CA models have primarily focused on enhancing technical modeling software, which has led to many models being unable to accurately simulate different land-use types. The current study employed a novel modeling approach named the PLUS model that integrates the land-use expansion analysis strategy (LEAS) model with a CA model to accurately simulate land use across various types of randomly distributed patches. By examining the complex relationships among diverse land-use types, the PLUS model can dynamically predict future land-use changes, enhancing the model’s prediction accuracy (Liang et al., 2021). The PLUS model requires two fundamental LULC maps as inputs, and the settings of the transition matrix and neighborhood weights vary based on different LULC scenarios. In order to comprehend the spatial pattern of land-use change, it is imperative to consider multiple driving factors, as highlighted in previous research literature (Shi et al., 2021).
In this study, 15 different factors (DEM, slope, minimum temperature, gross primary productivity [GPP], population [POP], gross domestic product [GDP], soil type, precipitation, waterways, railways, NPP, second road, main road, maximum temperature, and temperature) were collected for use in the LULC simulation. The LEAS model utilizes a two-stage iteration process for land-use data, which incorporates state change image features from subsequent LULC data to reflect the variations of different LULC scenarios. The RF algorithm was applied to analyze the correlations among land-use scenarios and the different driving factors, enabling the researchers to determine the transformation patterns of different land-use types and evaluate the development potential of different land types.
2.3.4 The CMIP6-PLUS model
This study incorporated historical land-use data from Xinjiang at five-year intervals from 2010 to 2020 (Li et al., 2021) and applied the Markov chain method (Nor et al., 2017) to estimate the demand for LULC under various climate change scenarios. Subsequently, the PLUS model used these demands as input parameters to simulate future LULC. In order to evaluate the accuracy of simulating land-use demand, historical data from 2020 were used. The RF model was employed to assess the significant development prospects for different land-use types, using 13 predictive variables that influence LULC. Assuming the predicted results were sufficiently precise, the driving factors and land-use demands for different scenarios (at five-year intervals) from 2020 to 2060 in Xinjiang were input into the PLUS model. Based on the 2020 LULC data as a baseline, predictions of future spatiotemporal changes in land-use distribution were made. Integrating CMIP6 data with the PLUS model allowed for a comprehensive and meticulous simulation of the spatial distributions of various land uses, providing a more thorough prediction of land-use changes compared to previous studies.
2.4 Scenario setup and land-use demands
This study simulated LULC data using the PLUS model and estimated ecosystem CS using the RF model (Figure 2). First, the PLUS model, along with Markov chains, was used to simulate the effects of various factors, such as population, GDP, temperature, and precipitation, on the spatial distribution of LULC under various models in Xinjiang from 2020 to 2060. Markov models were employed to forecast the area of various land types. The RF model was also used to evaluate the spatiotemporal impacts of LULC alterations on CS in ecosystems.
2.5 Estimation of CS by the RF model
This study adopted the RF algorithm that was proposed by Breiman (Rigatti, 2017), which combined a set of weak learners into a strong one through random variable selection (Breiman, 2001). Each tree in the RF model was constructed using bootstrap sampling, and the classification was performed using the Gini index, while regression was executed using a mean-squared error. The RF model is a special type of bagging method that uses decision trees to construct a model and can prevent overfitting. An RF model was employed in this study to assess the CS variations in Xinjiang over time, with a focus on achieving spatially accurate and interpretable results (Zhang et al., 2020).
In this study, the RF model was trained using historical data, including information about LULC, carbon density, and various collected factors. Once the model was trained, it was used to predict future changes in CS sinks based on different scenarios. These scenarios include changes in LULC, climate, and socioeconomic factors as specified in the SSP-RCP scenario. The RF model was implemented in the R 4.1.2 software package.
2.5.1 Carbon sequestration
This study quantified permanent CS and the dynamics of carbon fixation and loss by determining the carbon content in four key carbon density pools: aboveground biomass carbon (AGBC), belowground biomass carbon (BGBC), soil organic carbon (SOC), and dead organic matter carbon (DOMC). Given the complexity and challenges in obtaining DOMC data, the CS in TE was partitioned into three reservoirs: AGBC, BGBC, and SOC (0–20 cm), and the RF model was applied to model and spatially overlay these carbon pools.
Carbon density data for aboveground biomass, belowground biomass, and soil were obtained from a publicly available database compiled by the Chinese Academy of Sciences (Xu et al., 2018; Xu et al., 2019b). The database included data from 3,026 soil samples collected from different regions in China in 2014, sourced from 1,036 published papers and field surveys. The database included 231 sampling points in the Xinjiang region, representing six primary land-use categories. The study also utilized this database to estimate the potential for CS in Xinjiang and develop the model. The equation is as follows:
where is the carbon sequestration supply; Ci-above is AGBC, kg/m2 ; is the BGBC, kg/m2 ; is DOMC, kg/m2 ; Ci-soil is the SOC, kg/m2 .
3 Results and analysis
3.1 Verification of LULC accuracy
The PLUS model was used to simulate the LULC for 2020, and the results were validated against actual data. An accuracy assessment was conducted, and the Kappa coefficient for simulating the 2020 LULC using the PLUS model was 0.905, with an overall accuracy of 0.949. The Kappa experimental findings indicated that the simulation accuracy in this study met the required standards (Huang et al., 2019b).
3.2 The LULC simulation and analysis
The PLUS model was used for simulating LULC datasets in Xinjiang under various future scenarios (from 2025 to 2060, at five-year intervals). Changes in LULC were calculated in three distinct situations by determining the differences in the distribution of various LULC categories (Figure 3). Under the SSP126 scenario, the LULC trends in 2060 displayed a gradual expansion of water bodies, grassland, built-up land, and forest, with increases of 64.95%, 3.02%, 40.31%, and 6.50%, respectively, compared to those in 2020. Trends in LULC for cropland and unutilized land showed a downward trend with decreases of −8.26% and −3.96%, respectively. Under the SSP245 scenario, relative to 2020, the LULC trend in 2060 displayed a gradual increase in cropland, forest, and built-up land, with increases of 9.53%, 8.65%, and 22.08%, respectively. Trends for grasslands, water bodies, and unutilized land showed a downward trend, with decreases of −0.58%, −2.65%, and −0.91%, respectively. Under the SSP585 scenario, there was significant growth in cropland (30.92%) and built-up land (50.65%) compared to 2020. Forests, grasslands, watersheds, and unutilized land declining, with decreases of −4.88%, −2.06%, −4.43%, and −1.94%, respectively.
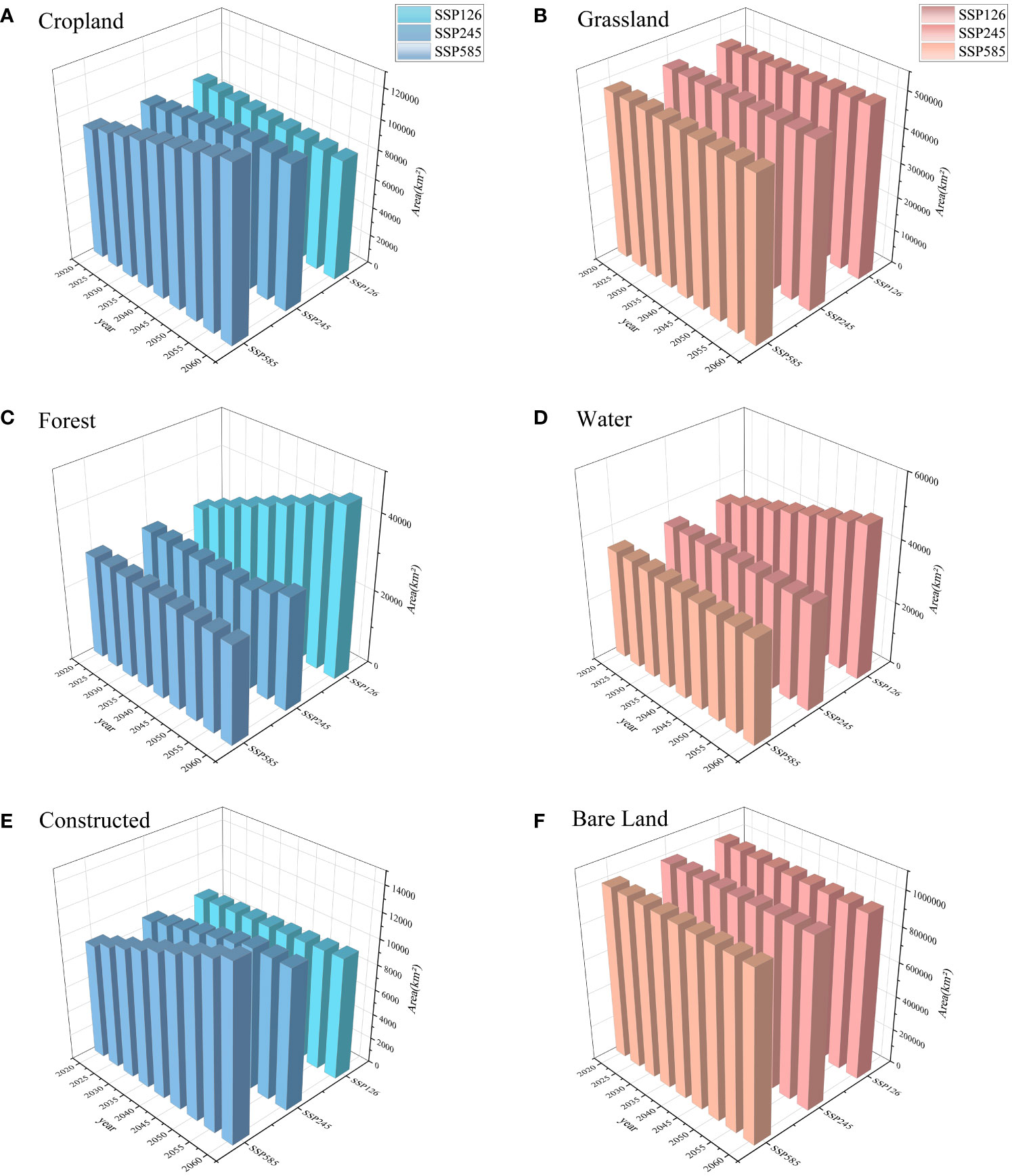
Figure 3 LULC simulation results under different future scenarios: (A) simulation results of cropland under different scenarios from 2020 to 2060; (B) simulation results of grassland under different scenarios from 2020 to 2060; (C) simulation results of forest under different scenarios from 2020 to 2060; (D) simulation results of water under different scenarios from 2020 to 2060; (E) simulation results of constructed under different scenarios from 2020 to 2060; (F) simulation results of arable bare land under different scenarios from 2020 to 2060.
This study aims to analyze the spatiotemporal changes in landscape patterns in Xinjiang under three different land-use scenarios. The LULC area was determined for the period from 2020 to 2060, and maps were produced to show the spatiotemporal pattern of variations in the different LULC types in Xinjiang for three scenarios. The results showed that from 2020 to 2060, arable land significantly increased in both the SSP245 and SSP585 scenarios. This newly added cropland was mainly located around the Tianshan North Slope urban agglomeration, the Ili River Valley, the Aksu region, and the surrounding cropland areas in the Hetian region. According to the calculations in this study, the cropland area increased by 8,602.94 km² and 27,919.38 km² under the SSP245 and SSP585 scenarios, respectively, during this period. Under the SSP126 and SSP245 scenarios, the forest area in Xinjiang displayed a small but steady growth trend by 2060, increasing by 17,878.38 km² and 2,381.31 km², respectively. However, the increase in forest area was mainly concentrated in the river valley buffer zones near the Ili River Valley, the Kunlun new built-up area of aboutMountains, and the Altai Mountains. With the rapid growth of Xinjiang’s economy, an extension of the built-up area was projected from 2020 to 2060, particularly under the SSP585 scenario. A new built-up area of about 4,594.25 km² was projected in an urban cluster on the northern slopes of the Tianshan Mountains, including Kashgar (Figure 4).
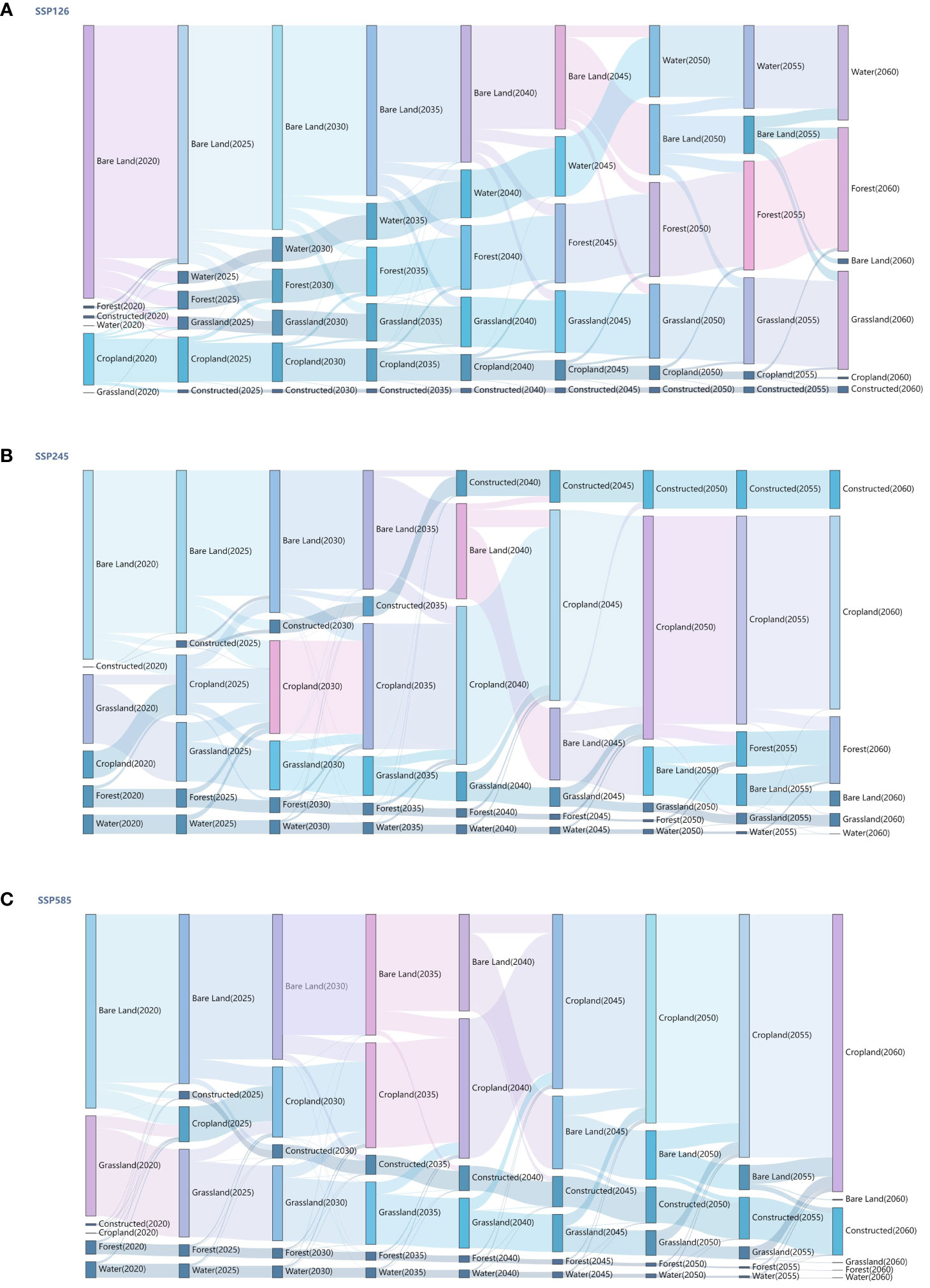
Figure 4 Simulation results of the land transfer matrix under three scenarios: (A) alluvial plot of the land use–transfer matrix for the SSP126 scenario from 2020 to 2060; (B) alluvial plot of the land use–transfer matrix for the SSP245 scenario from 2020 to 2060; (C) alluvial plot of the land use–transfer matrix for the SSP585 scenario from 2020 to 2060.
3.3 Spatial-temporal variability of carbon storage under various future climate scenarios
This study integrated the RF model and environmental factors to quantitatively evaluate the potential CS of Xinjiang’s future TE. In 2020, Xinjiang’s TE was estimated to sequester approximately 9.47 Pg of carbon. According to the SSP245 scenario prediction, by 2040, the CS in the region was expected to significantly increase by approximately 180.67 Tg compared to 2020. Compared to SSP245, the CS in 2040 under SSP126 increased by 130.12 Tg. The outcomes showed that the SSP126 scenario emphasized ecological development, resulting in an expansion of the area of forest and grassland and an overall increase in CS. Conversely, under the SSP585 scenario, CS in 2040 decreased by 190.25 Tg compared to the SSP245 scenario. This reduction is primarily attributed to the extensive expansion of industrial and agricultural activities, leading to a significant decline in the area covered by forest and grassland and a subsequent reduction in CS. Specifically, in comparison to the SSP245 scenario, for the SSP126 scenario, there was an increase of 162.16 Tg by 2060, while for the SSP585 scenario, there was a decrease of 230.12 Tg. Under the SSP126 scenario, significant improvements in CS were observed due to the transition of large agricultural and idle land areas to forest and grassland. Conversely, the SSP585 scenario resulted in a substantial reduction in CS following the transformation of unused land, grassland, and forest to cropland and built-up land (Figure 5).
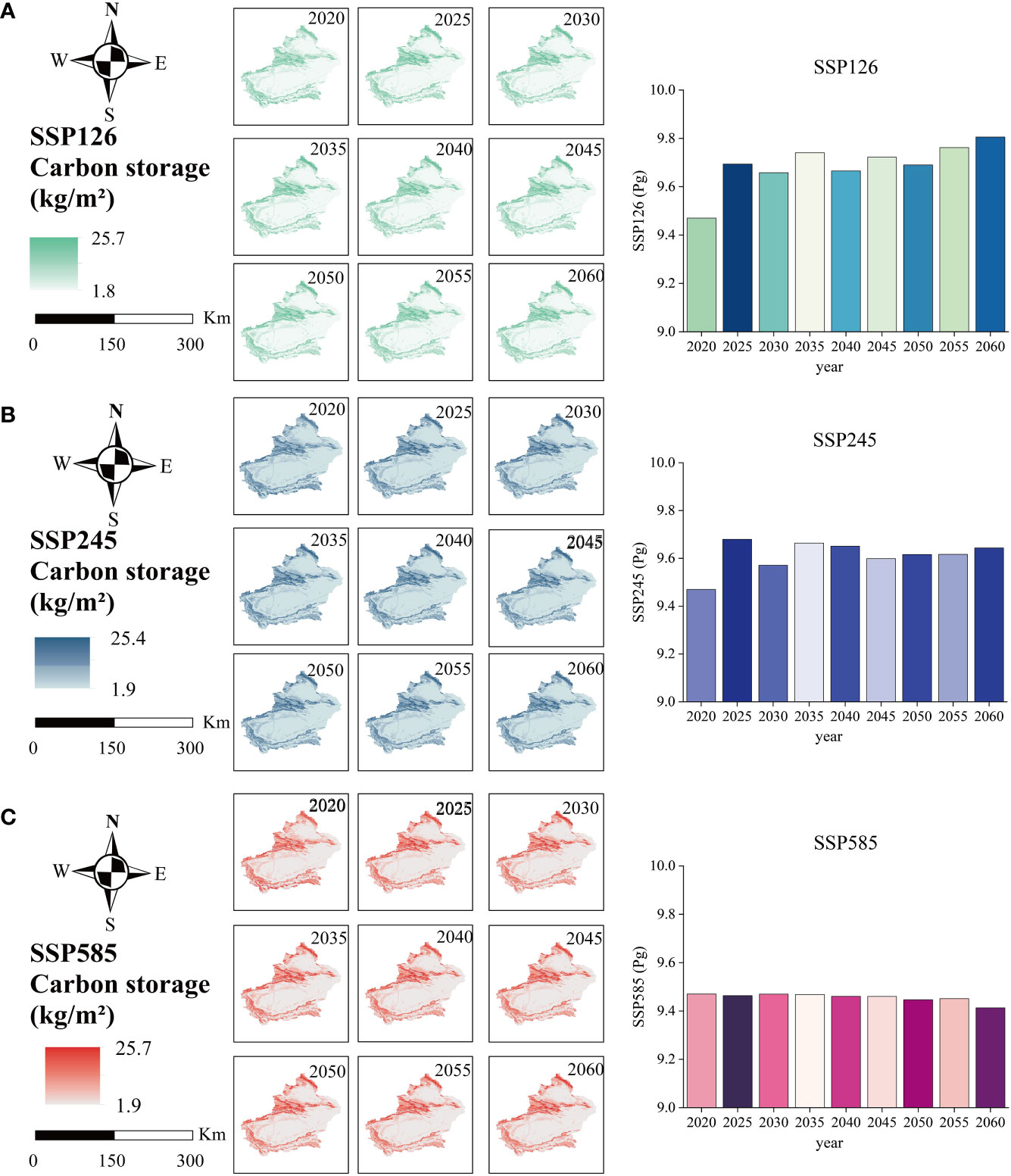
Figure 5 Spatial and temporal CS changes in Xinjiang during 2020−2060: (A) spatial and temporal variation of CS for the SSP126 scenario from 2020 to 2060; (B) spatial and temporal variation of CS for the SSP245 scenario from 2020 to 2060; (C) spatial and temporal variation of CS for the SSP585 scenario from 2020 to 2060.
3.4 Regional and latitudinal analysis of CS under various future scenarios.
A spatial analysis of CS in Xinjiang across various scenarios from 2020 to 2060 was conducted. Three typical zones were selected for analysis: the Yili Valley, Altay, and the Hotan region in southern Xinjiang (Figure 6). In the Altay region, forests and grasslands contributed significantly to CS. Under the SSP126 scenario, the preservation and restoration of forests and grasslands in the Altay region led to a significant increase in CS. In the Ili River Valley region, vegetation and soil were the main contributors to CS. Under the SSP245 scenario, there was a slow but steady increase in cropland and forest area, while the grassland area slightly decreased due to climate warming and human activities. Consequently, there was a slight increase in CS. The Hotan region in southern Xinjiang, characterized by an arid climate and dominated by desert and grassland, experienced a decrease in CS under the SSP585 scenario. The primary reason for this is the massive conversion of forest and grassland to agricultural land, which resulted in reduced land availability and the destruction of CS due to the removal of vegetation and land transformation.
The longitudinal and latitudinal spatial allocation of CS in Xinjiang under various climate scenarios was analyzed. The findings revealed that the distribution of CS differed significantly across the region, with unique patterns along both the latitude and longitude axes. Specifically, the latitude bands of 35°, 40°, and 47.5° exhibited significant fluctuations in CS due to the existence of extensive forested land in the Kunlun, Tianshan, and Altai Mountains, respectively. In contrast, CS gradually decreased with increasing longitude away from the mountain ranges due to the decrease in vegetation cover (Figure 7).
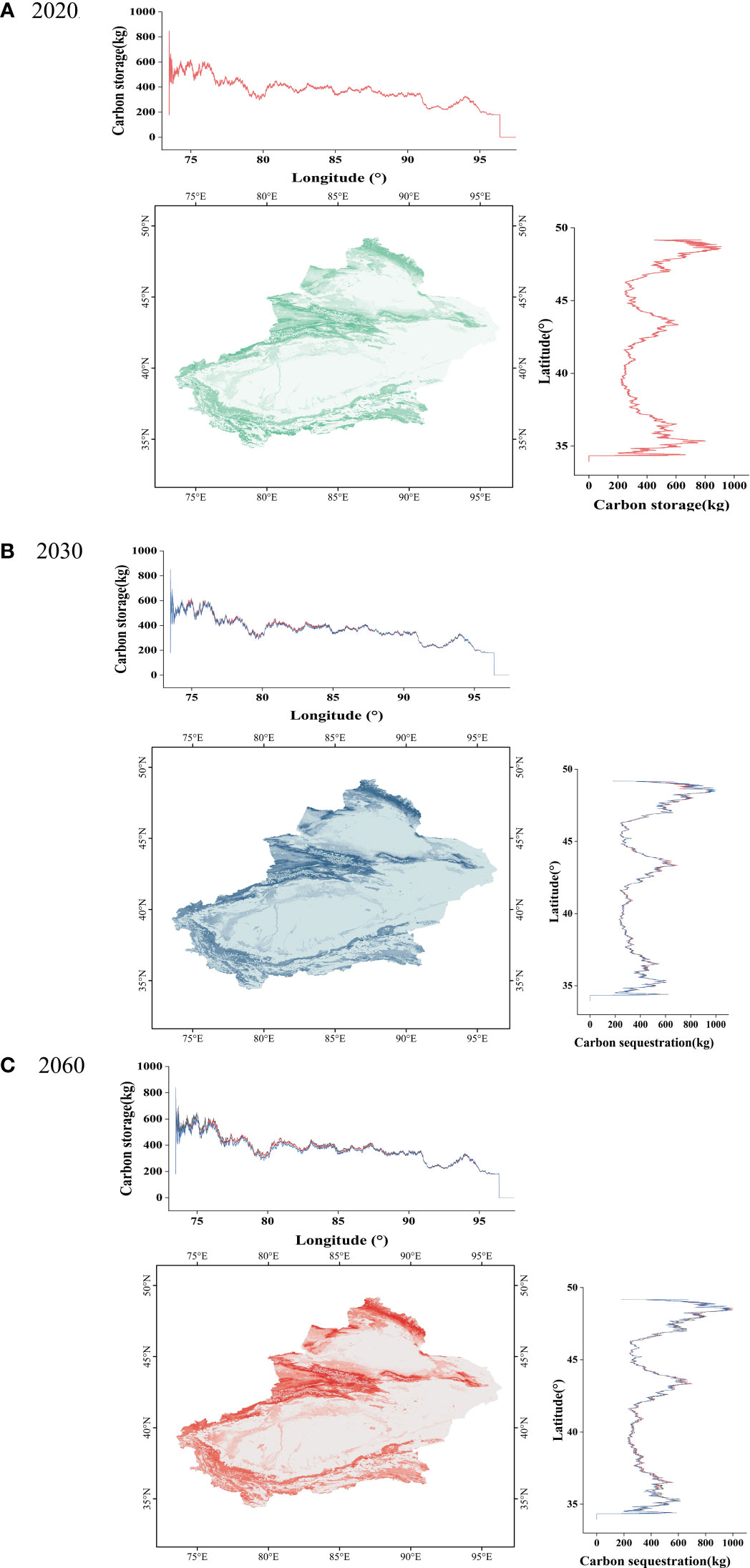
Figure 7 Spatial distribution (latitude and longitude) of CS in Xinjiang under future climate scenarios: (A) spatial distribution (latitude and longitude) of CS in Xinjiang under the SSP126 scenario; (B) spatial distribution (latitude and longitude) of CS in Xinjiang under the SSP245 scenario; (C) spatial distribution (latitude and longitude) of CS in Xinjiang under the SSP585 scenario.
4 Discussion
4.1 Analysis of LULC changes under different future scenarios
This study investigated how the SDGs can be utilized at the local level, aiming to achieve global sustainable development, effectively facilitating the development of provincial regions, offering decision-making guidance, and incorporating regional development into the globalization process. At the same time, it was recognized that the United Nations Environment Programme believes TE services will have a crucial impact on the SDGs. Thus, this study specifically examined changes in land use aligned with SDG 15 (Peng et al., 2021) to contribute to sustainable development targets and provide a foundation for decision-making at the local level (Huang et al., 2019a). The aim is to achieve the target of SDG 15.3, namely, establishing a world in which land degradation does not occur by implementing land-use scenario planning. Additionally, the study envisaged the incorporation of ES into national and local development processes, aligning with the core objective of SDG 15.9 (Wu et al., 2022).
To ensure the accuracy of the research, the PLUS model was used to simulate LULC data for the year 2020. Additionally, the PLUS model simulated the spatiotemporal distribution of LULC changes in Xinjiang under three distinct scenarios (SSP126, SSP245, and SSP585) from 2020 to 2060. Significant differences were observed in cropland, forest, grassland, and built-up land under the three scenarios. Moreover, the PLUS model diagnosed the drivers of LULC change. Figure 8 ranks the growth drivers for each of the four LULC types. The drivers of cropland, forest, grassland, and built-up land were essentially the same in all three scenarios (Zhao et al., 2021a).
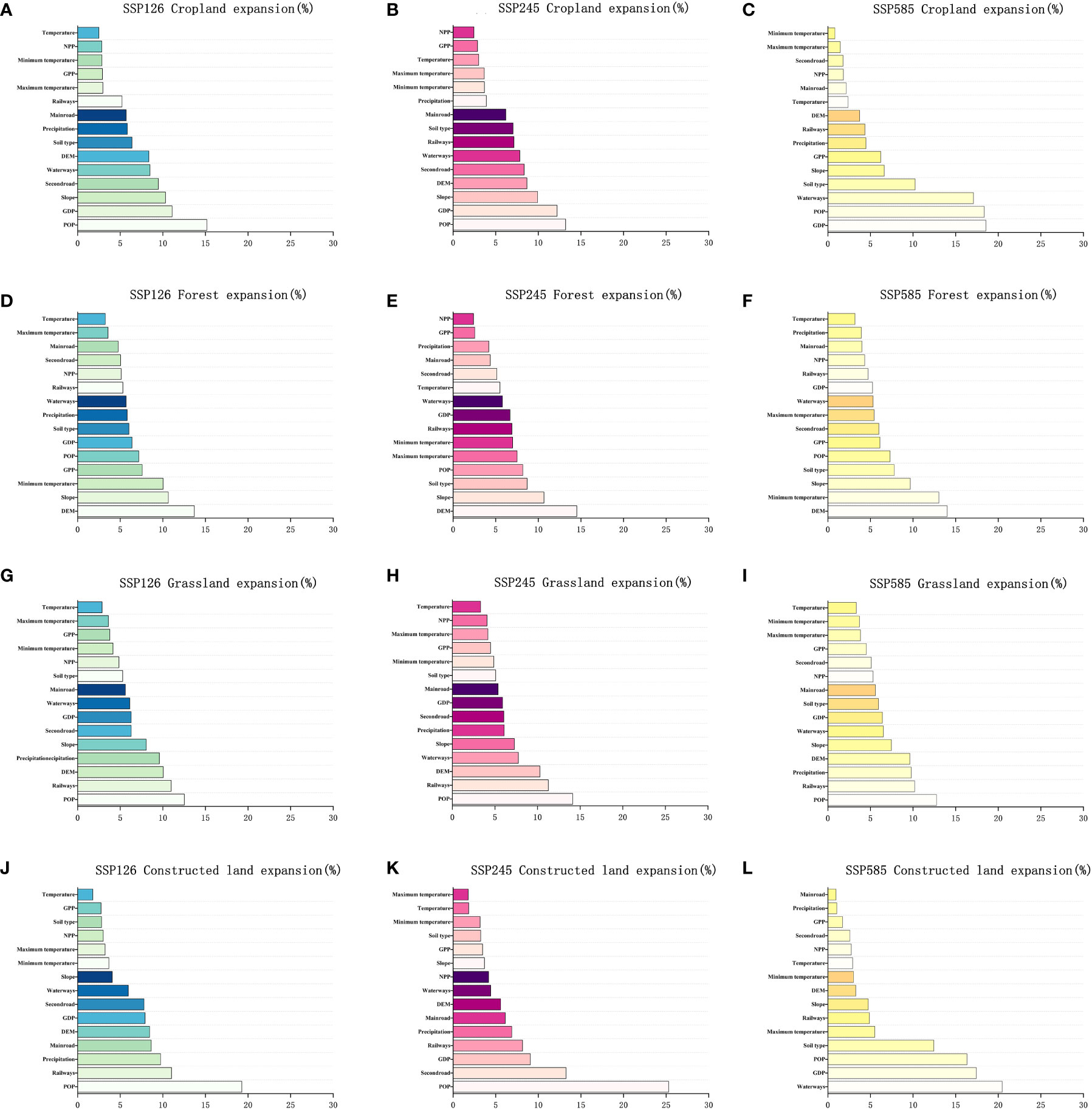
Figure 8 Importance of the response of each factor to the contribution of the growth in four LULC types: (A, D, G, J) significance of each factor’s response to the growth contribution in the four LULC types under the SSP126 scenario; (B, E, H, K) significance of each factor’s response to the growth contribution in the four LULC types under the SSP245 scenario; (C, F, I, L) significance of each factor’s response to the growth contribution in the four LULC types under the SSP585 scenario.
The growth of cropland was primarily influenced by population density. As the population increased, it resulted in a higher demand for food. In order to meet the increasing food demand, more land needs to be cultivated when food production reaches a certain level. Population growth and economic development also play significant and decisive roles in the development of future agricultural systems (Popp et al., 2017). The development of other socioeconomic factors, such as crop and livestock technology advances, investment in agricultural technology, and agricultural trade, is also important. Population growth significantly impacted the resulting changes in the cropland area (Zhang and Xie, 2019).
Altitude and population density were the main factors affecting changes in forest cover in Xinjiang. The majority of the forest area in Xinjiang is located in high-altitude regions, particularly in the Tianshan and Altai Mountains. Population density significantly impacts forest area because population growth increases demand for products derived from forests and the ecological services forests provide (Wang et al., 2021; Huang et al., 2022b). Xinjiang, situated in northwestern China, is characterized by alternating plateaus and mountains. It exhibits various climatic types and significant temperature variations. Xinjiang’s forests are primarily in mountainous areas with high altitudes and low temperatures, which are ideal for the growth of coniferous trees such as spruce and red pine. Some forests are also in low-altitude areas of Xinjiang. The tree species suitable for growth under different altitudes and terrain conditions differ. For instance, in the Junggar Basin area of Xinjiang, desert plants and shrubs are more common. These factors jointly affect the complex changes in the forest area in Xinjiang. In general, the annual average minimum temperature is considered one of the primary factors driving forest growth (Liu et al., 2022).
The expansion of the grassland area is primarily influenced by altitude, population size, and precipitation. This highlights the sensitivity of grassland to temperature changes (Li et al., 2020), as well as the potential impact of human activity on its expansion (Liang et al., 2021). The increase in urban land is primarily driven by the distance from water bodies, population density, and anthropogenic factors, mainly the proximity to railways and secondary roads. Water bodies are obstacles to urban expansion, and therefore cities often encroach on cropland, grassland, or other land-use types to bypass or surround water bodies (Chen and Huang, 2021; Zhai et al., 2021). As population density increases, urban areas require further expansion to accommodate the increasing number of inhabitants. Urban expansion in Xinjiang has largely been influenced by anthropogenic factors, with terrain factors significantly impacting the challenges and costs associated with constructing urban infrastructure (Liu et al., 2021b). Urban areas are expanded along the periphery of artificial roads to avoid the larger terrain fluctuations in mountainous areas (Zhai et al., 2021; Zhao et al., 2021b).
The results suggest that human activity is the primary factor driving LULC change. Continued expansion and development of human activities have caused alterations in land utilization patterns and the depletion of land resources. This, in turn, has affected the stability and sustainable development of ecosystems, making human activity the most significant factor driving land-use change.
4.2 The analysis of changes in CS across different future climate scenarios
This study adopted a novel approach that combined the CMIP6-PLUS and RF models to simulate the spatial CS changes of TE in Xinjiang for the next 40 years. The resulting spatial distribution maps revealed significant spatial differences in CS changes, which were affected by various factors. The scheme considered the impact of various climate scenarios, and the results suggest that CS in the region is likely to be affected by a range of complex and interrelated factors. The results enabled an understanding of future CS changes, especially those caused by LULC changes. The spatial distribution map also considered both natural and human needs, showing the possibility of achieving a balance between protecting natural resources and promoting human development (Chaplin-Kramer et al., 2019; Huang et al., 2022c). These findings suggest that it is possible to balance conserving ecosystem integrity and facilitating human development. The study identified significant anthropogenic driving factors in the form of LULC changes that profoundly impact the functioning of regional ES.
Although few simulations of the CS of TE under future climate change scenarios exist, this study used measured data sets, including AGBC, BGBC, and SOC, and combined the data with an RF model to spatially map CS under various future climate scenarios (Zhang et al., 2020). To ensure accuracy, the parameters of the CS output results obtained through the RF model were validated, and the results were compared with the findings of other studies. This study considered the CS of soil, AGBC, and BGBC and estimated that CS in the TE of the Xinjiang region was about 9.47 Pg (Shi et al., 2022). There were significant differences in LULC in different environments, which led to spatial variability in CS changes in Xinjiang. The decline in CS in Xinjiang can be attributed to the expansion of urban areas and cropland and a decrease in forest and grassland areas (Liu et al., 2021a).
The SSP585 scenario predicted a decline of 57.07 Tg in CS in Xinjiang by 2060 compared to that in 2020. The expansion of urban and agricultural land caused the decline. The long-term consequences of urban expansion may include reducing regional ecosystem CS despite its short-term employment and economic growth benefits. It is, therefore, crucial to balance urban expansion and ecological protection to maintain sustainable development. Accelerated economic expansion and urban growth have severely impacted ecosystem quality, causing the ongoing degradation of forests, grassland, and shrubland, ultimately reducing CS in TE. The findings aligned with previous studies, which showed that rapid economic development will gradually destroy ecosystems, leading to a decline in CS in TE (Li et al., 2020; Huang et al., 2022a).
In order to enhance ES and CS, it is crucial to prioritize high-quality economic development over rapid growth. Moreover, dynamic LULC changes might have significant impacts on the CS of TE given the rate of climate change and socioeconomic uncertainties in the future (Popp et al., 2017). In order to achieve a balance in the CS of TE, it is essential to reduce reliance on fossil fuels and promote the utilization of renewable energy sources, such as solar and wind power. This approach will help mitigate the impacts of global warming and prevent further degradation of forests, grassland, and shrubland.
4.3 The impact of latitude and longitude on CS in Xinjiang
The distribution of CS in Xinjiang is significantly affected by latitude and longitude. As a unique land area in northwest China, Xinjiang’s geographical features and climatic conditions differ from other regions of China. The study revealed significant spatial variation in CS across Xinjiang, with the most significant values identified in the northern, central, and southern areas. The Kunlun Mountains lie to the south of Xinjiang, with the Tianshan Mountains situated in the central region and the Altai Mountains in the north. These mountainous areas were found to have the highest CS, while the central-south and central-north regions exhibited the lowest values. Notably, the Taklimakan Desert in the central-south region and the Gurbantunggut Desert in the central-north region were identified as areas with low CS. The high CS in northern, central, and southern Xinjiang is attributed to the rich forest and grassland areas in these regions, which possess great potential for CS. In contrast, the central-south and central-north regions have limited vegetation cover and, as a result, a lower CS potential (Sha et al., 2022).
The results also showed that the contribution of LULC types to CS varies depending on the latitude and longitude of the region. Forests and grasslands in the northern, central, and southern regions had the highest CS due to their thriving vegetation and high biomass. Conversely, the central and northern desert and arid regions have very low levels of CS due to the lack of vegetation cover. Additionally, cropland and urban land contributed to CS in Xinjiang, although the contribution was relatively small. The geographical location of the different regions in Xinjiang impacted the distribution of its CS. Latitude and longitude also influenced the climate conditions in different regions of Xinjiang, which in turn affected vegetation growth and carbon cycling (Chen et al., 2019). This influence occurred because the northern, central, and southern regions are relatively humid, with conditions more suitable for vegetation growth. In contrast, the central-south and central-north regions lack water resources and suitable climatic conditions, resulting in relatively slow vegetation growth and low CS. The suitability of vegetation growth in different regions of Xinjiang varies based on the prevailing climate. The southern region of the study area, characterized by a temperate continental climate, was found to be more conducive to vegetation growth than the northern region, which possesses a cold and arid climate. Therefore, CS in southern Xinjiang was relatively high, while CS in northern Xinjiang was relatively low.
Furthermore, latitude and longitude influenced the distribution of the population and economic development in Xinjiang, thus affecting land use and CS (Xiang et al., 2022). For instance, areas with plains and well-developed transportation networks exhibited relatively high population densities, high levels of economic development, and more intensive land use. In contrast, areas with mountains and limited transportation networks had lower population densities, lower levels of economic development, and more scattered land-use types. Therefore, CS in plains and easily accessible areas was relatively low, while CS in mountainous and less accessible areas was higher. In conclusion, while latitude and longitude were significant factors influencing the distribution of CS in Xinjiang, they were not the only determinants. Climate conditions, land use, and population distribution also significantly influenced the distribution of CS.
4.4 Limitations of the study and future research prospects
This study utilized a novel approach, integrating the PLUS and RF models to forecast and assess spatial patterns and the potential for CS in forthcoming LULC changes. The method applied key drivers, such as population, GDP, climate variables, and LULC demand, across various SSP-RCP scenarios. The PLUS model was employed to create a spatial distribution of LULC changes, considering input parameters such as GDP, population, temperature, precipitation, and future land-use demand from the SSP-RCP scenarios. It should be noted that the PLUS model relies on invariant rules of transition when simulating LULC changes for each LULC type, which might not reflect the actual LULC changes that could occur in the future (Liang et al., 2021). Additionally, this study examined only three climate change scenarios produced by the CNRM-CM6-1 model. In future research, variations in climate projections generated by different global circulation models will be examined (Kim et al., 2020).
Given the rapid progress in developing low-carbon technologies and the government’s focus on its dual-carbon goals, it remains unclear whether the current carbon density trend will continue in the future. Therefore, future research should formulate LULC scenarios more rationally, considering the changes in carbon intensity over an extended time series. This approach will provide more science-based information for future regional policy-making and sustainability planning.
5 Conclusion
This study employed the CMIP6-PLUS-RF coupled model for the first time and predicted the spatiotemporal dynamics of LULC changes and the CS potential of TE in a typical arid region of China over 40 years. The study investigated three scenarios, SSP126, SSP245, and SSP585, and showed significant variations in LULC and terrestrial ecosystem CS under each scenario. In the SSP126 scenario, areagrasslands, forests, and built-up land areas are expected to increase, while the cultivated land area is expected to will decrease. Forests and grasslands will be effectively protected. Under the SSP245 scenario, the areas of grasslands and water bodies are expected to decrease, while the areas of cultivated land and forests are expected to increase. Under the SSP585 scenario, grassland and forest will no longer be effectively protected, and the cropland and built-up land areas will expand rapidly, leading to the occupation of existing grassland and forest. The main factors influencing the increase in cropland, forest, grassland, and built-up land are population, elevation, precipitation, and distance to water. Forest and grassland ecosystems play a pivotal role in CS, serving as the primary resource for increasing CS. These ecosystems effectively counteract the decrease in CS resulting from the reduced vegetation land cover area caused by the expansion of built-up land and cropland. Therefore, increasing the forest area through afforestation, which involves planting trees on previously non-forested land and converting agricultural land back to natural forest ecosystems, can enhance the CS capacity of forest ecosystems. This enhancement occurs as trees absorb and store more atmospheric carbon dioxideatmosphere, contributing to climate change mitigation. In summary, the findings of this study will assist in determining the optimal allocation of land resources and economic development plans in the area, providing essential data support for China’s aspirations to achieve carbon neutrality.
Data availability statement
The raw data supporting the conclusions of this article will be made available by the authors, without undue reservation.
Author contributions
HW and HG were responsible for the research design, analysis, and the manuscript’s design and its review. KZ drafted the manuscript and was responsible for data preparation, experiments, and analyses. MS is responsible for preparing the data and guiding the experiments. PJ, PH, and TD contributed to manuscript editing. ZL, XW, NZ, and RW reviewed and polished the manuscript. All authors have read and agreed to the published version of the manuscript. The authors declare no conflicts of interest. All authors contributed to the article and approved the submitted version.
Funding
The author(s) declare financial support was received for the research, authorship, and/or publication of this article. This research was financially supported by Major Science and Technology Special Pro-jects in Xinjiang Uygur Autonomous Region, China (No. 2020A01002 and No. 2022A02011), in part by the 2023 Xinjiang Uygur Autonomous Region Graduate Innovation Project.
Acknowledgments
We also like to thank the reviewers for their constructive comments on the manuscript.
Conflict of interest
The authors declare that the research was conducted in the absence of any commercial or financial relationships that could be construed as a potential conflict of interest.
Publisher’s note
All claims expressed in this article are solely those of the authors and do not necessarily represent those of their affiliated organizations, or those of the publisher, the editors and the reviewers. Any product that may be evaluated in this article, or claim that may be made by its manufacturer, is not guaranteed or endorsed by the publisher.
References
Barnaud C., Corbera E., Muradian R., Salliou N., Sirami C., Vialatte A., et al. (2018). Ecosystem services, social interdependencies, and collective action. Ecol. Soc. 23, 14. doi: 10.5751/ES-09848-230115
Chaplin-Kramer R., Sharp R. P., Weil C., Bennett E. M., Pascual U., Arkema K. K., et al. (2019). Global modeling of nature's contributions to people. Science 366, 255–258. doi: 10.1126/science.aaw3372
Chen J. M., Ju W., Ciais P., Viovy N., Liu R., Liu Y., et al. (2019). Vegetation structural change since 1981 significantly enhanced the terrestrial carbon sink. Nat. Commun. 10, 4259. doi: 10.1038/s41467-019-12257-8
Chen R., Huang C. (2021). Landscape evolution and it’s impact of ecosystem service value of the Wuhan city, China. Int. J. Environ. Res. Public Health 18, 13015. doi: 10.3390/ijerph182413015
Chen W., Chi G., Li J. (2020a). Ecosystem services and their driving forces in the middle reaches of the Yangtze river urban agglomerations, China. Int. J. Environ. Res. Public Health 17, 3717. doi: 10.3390/ijerph17103717
Chen Y., Guo F., Wang J., Cai W., Wang C., Wang K. (2020b). Provincial and gridded population projection for China under shared socioeconomic pathways from 2010 to 2100. Sci. Data 7, 83. doi: 10.1038/s41597-020-0421-y
Cramer W., Guiot J., Fader M., Garrabou J., Gattuso J-P., Iglesias A., et al. (2018). Climate change and interconnected risks to sustainable development in the mediterranean. Nat. Climate Change 8, 972–980. doi: 10.1038/s41558-018-0299-2
Fricko O., Havlik P., Rogelj J., Klimont Z., Gusti M., Johnson N., et al. (2017). The marker quantification of the shared socioeconomic pathway 2: A middle-of-the-road scenario for the 21st century. Global Environ. Change 42, 251–267. doi: 10.1016/j.gloenvcha.2016.06.004
He P., Ma J., Ye H., Han Z., Ma X., Alitengbieke H., et al. (2022). Satellite greenness and solar-induced chlorophyll fluorescence reveal reverse desertification in Gurbantunggut desert. Ecol. Appl., e2757. doi: 10.1002/eap.2757
Huang D., Huang J., Liu T. (2019b). Delimiting urban growth boundaries using the clue-s model with village administrative boundaries. Land Use Policy 82, 422–435. doi: 10.1016/j.landusepol.2018.12.028
Huang C., Huang X., Peng C., Zhou Z., Teng M., Wang P. (2019a). Land use/cover change in the three gorges reservoir area, China: Reconciling the land use conflicts between development and protection. Catena 175, 388–399. doi: 10.1016/j.catena.2019.01.002
Huang C., Qin Y., Du X., He J., Fan X. (2022a). Ecosystem health and environmental geography in the belt and road regions. Int. J. Environ. Res. Public Health 19, 5843. doi: 10.3390/ijerph19105843
Huang C., Zhao D., Deng L. (2022b). Landscape pattern simulation for ecosystem service value regulation of three gorges reservoir area, China. Environ. Impact Assess. Rev. 95, 106798. doi: 10.1016/j.eiar.2022.106798
Huang C., Zhao D., Fan X., Liu C., Zhao G. (2022c). Landscape dynamics facilitated non-point source pollution control and regional water security of the three gorges reservoir area, China. Environ. Impact Assess. Rev. 92, 106696. doi: 10.1016/j.eiar.2021.106696
Huang C., Zhao D., Liao Q., Xiao M. (2023). Linking landscape dynamics to the relationship between water purification and soil retention. Ecosys. Serv. 59, 101498. doi: 10.1016/j.ecoser.2022.101498
Huang C., Zhou Z., Teng M., Wang P. (2020). Effects of climate, land use and land cover changes on soil loss in the three gorges reservoir area, China. Geogr. Sustain. 1, 200–208. doi: 10.1016/j.geosus.2020.08.001
Hurtt G. C., Chini L., Sahajpal R., Frolking S., Bodirsky B. L., Calvin K., et al. (2020). Harmonization of global land use change and management for the period 850–2100 (luh2) for cmip6. Geosci. Model. Dev. 13, 5425–5464. doi: 10.5194/gmd-13-5425-2020
Jiyuan L., Mingliang L., Xiangzheng D., Dafang Z., Zengxiang Z., Di L. (2002). The land use and land cover change database and its relative studies in China. J. Geograph. Sci. 12, 275–282. doi: 10.1007/BF02837545
Kim Y.-H., Min S.-K., Zhang X., Sillmann J., Sandstad M. (2020). Evaluation of the cmip6 multi-model ensemble for climate extreme indices. Weather Climate Extremes 29, 100269. doi: 10.1016/j.wace.2020.100269
Kriegler E., Bauer N., Popp A., Humpenöder F., Leimbach M., Strefler J., et al. (2017). Fossil-fueled development (ssp5): An energy and resource intensive scenario for the 21st century. Global Environ. Change 42, 297–315. doi: 10.1016/j.gloenvcha.2016.05.015
Lambin E. F., Meyfroidt P. (2011). Global land use change, economic globalization, and the looming land scarcity. Proc. Natl. Acad. Sci. 108, 3465–3472. doi: 10.1073/pnas.1100480108
Li S., Bing Z., Jin G. (2019). Spatially explicit mapping of soil conservation service in monetary units due to land use/cover change for the three gorges reservoir area, China. Remote Sens. 11, 468. doi: 10.3390/rs11040468
Li J., Chen X., Kurban A., Van de Voorde T., De Maeyer P., Zhang C. (2021). Coupled ssps-rcps scenarios to project the future dynamic variations of water-soil-carbon-biodiversity services in central Asia. Ecol. Indic. 129, 107936. doi: 10.1016/j.ecolind.2021.107936
Li J., Gong J., Guldmann J.-M., Li S., Zhu J. (2020). Carbon dynamics in the Northeastern Qinghai–Tibetan plateau from 1990 to 2030 using landsat land use/cover change data. Remote Sens. 12, 528. doi: 10.3390/rs12030528
Li S., Zhang Y., Wang Z., Li L. (2018). Mapping human influence intensity in the Tibetan plateau for conservation of ecological service functions. Ecosys. Serv. 30, 276–286. doi: 10.1016/j.ecoser.2017.10.003
Liang X., Guan Q., Clarke K. C., Liu S., Wang B., Yao Y. (2021). Understanding the drivers of sustainable land expansion using a patch-generating land use simulation (plus) model: A case study in Wuhan, China. Computers Environ. Urban Syst. 85, 101569. doi: 10.1016/j.compenvurbsys.2020.101569
Liao Q., Wang Z., Huang C. (2020). Green infrastructure offset the negative ecological effects of urbanization and storing water in the three gorges reservoir area, China. Int. J. Environ. Res. Public Health 17, 8077. doi: 10.3390/ijerph17218077
Liu C., Liang Y., Zhao Y., Liu S., Huang C. (2021a). Simulation and analysis of the effects of land use and climate change on carbon dynamics in the Wuhan city circle area. Int. J. Environ. Res. Public Health 18, 11617. doi: 10.3390/ijerph182111617
Liu S., Liao Q., Liang Y., Li Z., Huang C. (2021b). Spatio–temporal heterogeneity of urban expansion and population growth in China. Int. J. Environ. Res. Public Health 18, 13031. doi: 10.3390/ijerph182413031
Liu S., Liao Q., Xiao M., Zhao D., Huang C. (2022). Spatial and temporal variations of habitat quality and its response of landscape dynamic in the three gorges reservoir area, China. Int. J. Environ. Res. Public Health 19, 3594. doi: 10.3390/ijerph19063594
Malek Ž., Verburg P. H., Geijzendorffer I. R., Bondeau A., Cramer W. (2018). Global change effects on land management in the mediterranean region. Global Environ. Change 50, 238–254. doi: 10.1016/j.gloenvcha.2018.04.007
Molotoks A., Stehfest E., Doelman J., Albanito F., Fitton N., Dawson T. P., et al. (2018). Global projections of future cropland expansion to 2050 and direct impacts on biodiversity and carbon storage. Global Change Biol. 24, 5895–5908. doi: 10.1111/gcb.14459
Nelson E., Mendoza G., Regetz J., Polasky S., Tallis H., Cameron D., et al. (2009). Modeling multiple ecosystem services, biodiversity conservation, commodity production, and tradeoffs at landscape scales. Front. Ecol. Environ. 7, 4–11. doi: 10.1890/080023
Nor A. N. M., Corstanje R., Harris J. A., Brewer T. (2017). Impact of rapid urban expansion on green space structure. Ecol. Indic. 81, 274–284. doi: 10.1016/j.ecolind.2017.05.031
Peng K., Jiang W., Ling Z., Hou P., Deng Y. (2021). Evaluating the potential impacts of land use changes on ecosystem service value under multiple scenarios in support of sdg reporting: A case study of the Wuhan urban agglomeration. J. Clean. Product. 307, 127321. doi: 10.1016/j.jclepro.2021.127321
Popp A., Calvin K., Fujimori S., Havlik P., Humpenöder F., Stehfest E., et al. (2017). Land-use futures in the shared socio-economic pathways. Global Environ. Change 42, 331–345. doi: 10.1016/j.gloenvcha.2016.10.002
Powers J. G., Klemp J. B., Skamarock W. C., Davis C. A., Dudhia J., Gill D. O., et al. (2017). The weather research and forecasting model: Overview, system efforts, and future directions. Bull. Am. Meteorol. Soc. 98, 1717–1737. doi: 10.1175/BAMS-D-15-00308.1
Reich P. B., Hobbie S. E., Lee T. D., Rich R., Pastore M. A., Worm K. (2020). Synergistic effects of four climate change drivers on terrestrial carbon cycling. Nat. Geosci. 13, 787–793. doi: 10.1038/s41561-020-00657-1
Rodríguez-Echeverry J., Echeverría C., Oyarzún C., Morales L. (2018). Impact of land-use change on biodiversity and ecosystem services in the Chilean temperate forests. Landscape Ecol. 33, 439–453. doi: 10.1007/s10980-018-0612-5
Sha Z., Bai Y., Li R., Lan H., Zhang X., Li J., et al. (2022). The global carbon sink potential of terrestrial vegetation can be increased substantially by optimal land management. Commun. Earth Environ. 3, 8. doi: 10.1038/s43247-021-00333-1
Shi M., Wu H., Fan X., Jia H., Dong T., He P., et al. (2021). Trade-offs and synergies of multiple ecosystem services for different land use scenarios in the Yili river valley, China. Sustainability 13, 1577. doi: 10.3390/su13031577
Shi M., Wu H., Jiang P., Shi W., Zhang M., Zhang L., et al. (2022). Cropland expansion mitigates the supply and demand deficit for carbon sequestration service under different scenarios in the future—the case of Xinjiang. Agriculture 12, 1182.
Su B., Huang J., Mondal S. K., Zhai J., Wang Y., Wen S., et al. (2021). Insight from cmip6 ssp-rcp scenarios for future drought characteristics in China. Atmosph. Res. 250, 105375. doi: 10.1016/j.atmosres.2020.105375
Tian L., Tao Y., Fu W., Li T., Ren F., Li M. (2022). Dynamic simulation of land use/cover change and assessment of forest ecosystem carbon storage under climate change scenarios in Guangdong province, China. Remote Sens. 14. doi: 10.3390/rs14102330
Turner B. (1997). The sustainability principle in global agendas: Implications for understanding land-use/cover change. Geograph. J., 133–140. doi: 10.2307/3060176
Udara Willhelm Abeydeera L. H., Wadu Mesthrige J., Samarasinghalage T. I. (2019). Global research on carbon emissions: A scientometric review. Sustainability 11, 3972. doi: 10.3390/su11143972
Van Vuuren D. P., Stehfest E., Gernaat D. E., Doelman J. C., Van den Berg M., Harmsen M., et al. (2017). Energy, land-use and greenhouse gas emissions trajectories under a green growth paradigm. Global Environ. Change 42, 237–250. doi: 10.1016/j.gloenvcha.2016.05.008
Wang Z., Miao Y., Xu M., Zhu Z., Qureshi S., Chang Q. (2021). Revealing the differences of urban parks’ services to human wellbeing based upon social media data. Urban Forest. Urban Green. 63, 127233. doi: 10.1016/j.ufug.2021.127233
Wu X., Fu B., Wang S., Song S., Li Y., Xu Z., et al. (2022). Decoupling of sdgs followed by re-coupling as sustainable development progresses. Nat. Sustain., 1–8. doi: 10.1038/s41893-022-00868-x
Xiang M., Wang C., Tan Y., Yang J., Duan L., Fang Y., et al. (2022). Spatio-temporal evolution and driving factors of carbon storage in the western Sichuan plateau. Sci. Rep. 12, 8114. doi: 10.1038/s41598-022-12175-8
Xu L., He N., Yu G. (2019a). A dataset of carbon density in Chinese terrestrial ecosystems, (2010s). China Sci. Data 4, 49–54. doi: 10.1007/s11442-019-1583-4
Xu L., He N., Yu G. (2019b). A dataset of carbon density in Chinese terrestrial ecosystems, (2010s). China Sci. Data 4, 90–96. doi: 10.11922/sciencedb.603.
Xu L., Yu G., He N., Wang Q., Gao Y., Wen D., et al. (2018). Carbon storage in China’s terrestrial ecosystems: A synthesis. Sci. Rep. 8, 1–13.
Yao J., Chen Y., Guan X., Zhao Y., Chen J., Mao W. (2022). Recent climate and hydrological changes in a mountain–basin system in Xinjiang, China. Earth-Science Rev., 226. doi: 10.1016/j.earscirev.2022.103957
Yao J., Mao W., Chen J., Dilinuer T. (2021). Signal and impact of wet-to-dry shift over Xinjiang, China. Acta Geograph. Sin. 76, 57–72.
Yi H., Zhao L., Qian Y., Zhou L., Yang P. (2022). How to achieve synergy between carbon dioxide mitigation and air pollution control? Evidence China Sustain. Cities Soc. 78, 103609. doi: 10.1016/j.scs.2021.103609
Yu E., Liu D., Yang J., Sun J., Yu L., King M. P. (2023). Future climate change for major agricultural zones in China as projected by cordex-ea-ii, cmip5 and cmip6 ensembles. Atmosph. Res., 106731. doi: 10.1016/j.atmosres.2023.106731
Zhai H., Lv C., Liu W., Yang C., Fan D., Wang Z., et al. (2021). Understanding spatio-temporal patterns of land use/land cover change under urbanization in Wuhan, China 2000–2019. Remote Sens. 13, 3331. doi: 10.3390/rs13163331
Zhang M., Shi W., Xu Z. (2020). Systematic comparison of five machine-learning models in classification and interpolation of soil particle size fractions using different transformed data. Hydrol. Earth Sys. Sci. 24, 2505–2526. doi: 10.5194/hess-24-2505-2020
Zhang Y., Xie H. (2019). Interactive relationship among urban expansion, economic development, and population growth since the reform and opening up in China: An analysis based on a vector error correction model. Land 8, 153. doi: 10.3390/land8100153
Zhao D., Xiao M., Huang C., Liang Y., Yang Z. (2021a). Land use scenario simulation and ecosystem service management for different regional development models of the beibu gulf area, China. Remote Sens. 13, 3161. doi: 10.3390/rs13163161
Zhao D., Xiao M., Huang C., Liang Y., An Z. (2021b). Landscape dynamics improved recreation service of the three gorges reservoir area, China. Int. J. Environ. Res. Public Health 18, 8356. doi: 10.3390/ijerph18168356
Keywords: land use/land cover, carbon sequestration, patch level land use simulation, Coupled Model Intercomparison Project 6, Xinjiang
Citation: Zheng K, Shi M, Wu H, Gu H, Jiang P, He P, Dong T, Liu Z, Wang X, Zhang N and Wei R (2023) Estimation and simulation of carbon sequestration in typical dryland areas of China under future climate change scenarios. Front. Ecol. Evol. 11:1250586. doi: 10.3389/fevo.2023.1250586
Received: 30 June 2023; Accepted: 30 October 2023;
Published: 16 November 2023.
Edited by:
Dafeng Hui, Tennessee State University, United StatesReviewed by:
Chunbo Huang, China University of Geosciences Wuhan, ChinaKiyan Sorgog, Jackson State University, United States
Copyright © 2023 Zheng, Shi, Wu, Gu, Jiang, He, Dong, Liu, Wang, Zhang and Wei. This is an open-access article distributed under the terms of the Creative Commons Attribution License (CC BY). The use, distribution or reproduction in other forums is permitted, provided the original author(s) and the copyright owner(s) are credited and that the original publication in this journal is cited, in accordance with accepted academic practice. No use, distribution or reproduction is permitted which does not comply with these terms.
*Correspondence: Hongqi Wu, whq@xjau.edu.cn; Haibin Gu, haibingu@xjau.edu.cn
†These authors have contributed equally to this work