- State Key Laboratory of Protein and Plant Gene Research, School of Life Sciences, Peking University, Beijing, China
The distribution of species changes over time, and the current distribution of different species could result from distinct eco-evolutionary processes. Thus, investigating the spatiotemporal changes in the niche and geographic range of species is fundamental to understanding those processes and mechanisms shaping the current distributions of species. However, many studies only compared the current distribution and niche of the target species, ignoring the fact that the range shift of species is a dynamic process. Here, we reconstructed niche evolution and range dynamics of species to provide more information on related eco-evolutionary processes. We focused on a monophyletic species complex, Chrysanthemum zawadskii species complex, in which species occupy diverse habitats and exhibit different distribution patterns. Specifically, we investigated the niche breadth and overlap between lineages or species of the complex in geographic and environmental spaces. We then tested the phylogenetic signals for different climatic variables and estimated the niche of ancestral nodes on a time-calibrated phylogeny. Next, we used phyloclimatic modeling to reconstruct the dynamics of range shift for this complex. Our results show that this complex contains both specialist and generalist species, and niche diverges greatly among different species and intraspecific lineages of the complex. The moisture gradient may be the primary driver of the niche divergence of species in the complex. The reconstruction of ancestral distribution shows that this complex originated in the Qinling mountains and surrounding areas during the early Pliocene, and then diverged with the range expansion and niche evolution. Species of the complex have different range dynamics. Based on our findings, we propose that niche evolution, range dynamics, and their coupling shape the distribution of species, which provides insight into the eco-evolutionary processes that formed the current distribution of species in the C. zawadskii complex.
1 Introduction
The ranges of species are dynamic owing to expansions, contractions, or shifts over space and time, which result from the evolutionary and ecological processes (Sheth et al., 2020; Bridle and Hoffmann, 2022; Rafajlović et al., 2022). Thus, different species, even those that are closely related, can have varying range sizes. Notably, it is crucial to distinguish between the drivers and consequences of changes in the range of species to understand the mechanisms of speciation and conserve biodiversity (Harnik et al., 2012; Saupe et al., 2015; Rafajlović et al., 2022). The niche is a core concept in ecology and evolution. It represents a set of ecological conditions affecting the survival and reproduction of species and is often used to explain the distribution, abundance, and diversification of species (Carscadden et al., 2020). As a critical component of the niche, niche breadth and its evolution are central to the explanations described above (Morin and Lechowicz, 2013; Slatyer et al., 2013). It is commonly assumed that the range of a species covaries with its niche breadth, but controversy over whether the range of a species is merely the spatial representation of its environmental tolerances remains (Harnik et al., 2012; Lancaster, 2022). Studying the range shifts between sister species is considered to be an ideal way to address these issues (Evans et al., 2009; Sheth et al., 2020). Some studies have measured the current range sizes of sister species and compared their niche breadths to understand the relationship between range changes and niche evolution of species (Kalkvik et al., 2012; Anacker and Strauss, 2014; Grossenbacher et al., 2014). However, the range shift of species is a dynamic process, and the current range of a species reflects the consequences of this process (Bridle and Hoffmann, 2022). Studies on identifying such a macroecological pattern provide little information on the underlying evolutionary and ecological processes (Sheth et al., 2020; Lancaster, 2022), and more knowledge of niche evolution and range dynamics is merited.
In recent decades, many hypotheses have been proposed to deduce possible mechanisms for variations in the range of different species, such as the oscillation hypothesis, climate variability hypothesis, and ecological opportunity hypothesis among others (Stevens, 1989; Hardy and Otto, 2014; Wellborn and Langerhans, 2015; Torres-Martínez et al., 2021; Lancaster, 2022). A key difference between these hypotheses is whether niche evolution or range expansion is the driver or the consequence. From this perspective, the relevant hypotheses can be divided into two categories. First is the niche breadth–range size hypothesis, which posits that increased niche breadth is the cause of range expansion, and second is the range size–niche breadth hypothesis, which suggests that increased niche breadth could just be the consequence of range expansion (Lancaster, 2022). Notably, both those hypotheses could oversimplify the processes that underlie variations in the range of a species. Lancaster (2022) thus proposed the range shift–niche breadth hypothesis, which posits that niche breadth evolves under eco-evolutionary processes during range expansion. Clearly, more study is required to reconstruct the historical dynamics of both the range and niche of species in a phylogenetic framework to understand the processes and mechanisms that shape the current distributions of species and to test the above hypotheses.
Ecological niche modeling (ENM) is one of the commonly used methods to deduce the potential distribution of species (Waltari et al., 2007; Maguire et al., 2015). In recent decades, ENM has developed rapidly, and many modeling methods, such as random forest, MaxEnt, and boosted regression trees, have been proposed (Sillero et al., 2021). Nonetheless, such an approach assumes that the niche of species is conserved, so that it is usually limited to shallow evolutionary timescales, e.g., mid-Holocene, Last Glacial Maximum, and Last Interglacial (Culshaw et al., 2021; Guillory and Brown, 2021). Since the niche of species is often regarded as evolving during speciation, it is necessary to consider the evolution of niche when reconstructing the historical distribution of species over deeper timescales. Fortunately, a solution that combines ENMs and phylogenetic analyses (also known as phyloclimatic modeling) has been proposed to solve this problem (Yesson and Culham, 2006). This method assumes that niches of species are not static over evolutionary time and reconstructs the ancestral niches of the target species based on climate data and phylogenetic information. Those ancestral niches could be converted into ancestral niche models which are projected into paleo-climate layers to estimate the ancestral geographic ranges of species (Yesson and Culham, 2006; Evans et al., 2009; Smith and Donoghue, 2010; Guillory and Brown, 2021). Therefore, we can use the results of both phyloclimatic modeling and ancestral niche reconstruction across evolutionary timescales to deduce the possible agents for changes in the range of species. In addition, we need to consider the effects of historical biogeographic processes on range dynamics of species and niche evolution to better understand the underlying eco-evolutionary processes that shape the distribution of species (Brown and Maurer, 1989; Ruggiero et al., 1998; Reed, 2003; Hoorn et al., 2010; Smith and Donoghue, 2010; Favre et al., 2015; Li et al., 2017; Yamamoto et al., 2017; Bridle and Hoffmann, 2022).
A species complex that contains several closely related species is well suited to explore the processes and mechanisms that underlie the range shifts and niche evolution of species because its species have a most recent common ancestor, usually occur in diverse habitats, and exhibit different distribution patterns (Pinheiro et al., 2018). In this study, we focused on the Chrysanthemum zawadskii species complex, which has a well-resolved phylogeny and traceable evolutionary history (Lu et al., 2022). Previous studies have shown that the C. zawadskii complex is a monophyletic group that consists of seven narrowly or widely distributed species in China (Shen et al., 2021; Lu et al., 2022). The narrowly distributed species, such as C. hypargyrum and C. oreastrum, typically occur in alpine habitats, while widespread species occur in diverse habitats (Lu et al., 2022). Phylogenetically, four geographic lineages of C. hypargyrum and C. oreastrum resided in basal clades of the phylogenetic tree, which occur in Qinling and its neighboring mountains (Lu et al., 2022). Another late-diverging large lineage, the core C. zawadskii complex, is composed of four widespread species (C. zawadskii, C. maximowiczii, C. naktongense, and C. chanetii) and a narrowly distributed C. mongolicum (Figure 1). Notably, the complex trends to expand toward high latitudes and results in large-range and small-range species with different habitats, which suggests that the distributions of species could covary with niche shifts during the expansion and evolution. However, the eco-evolutionary processes or mechanisms that underlie the range expansion and niche shifts at the lineage or species level of the complex remain unknown.
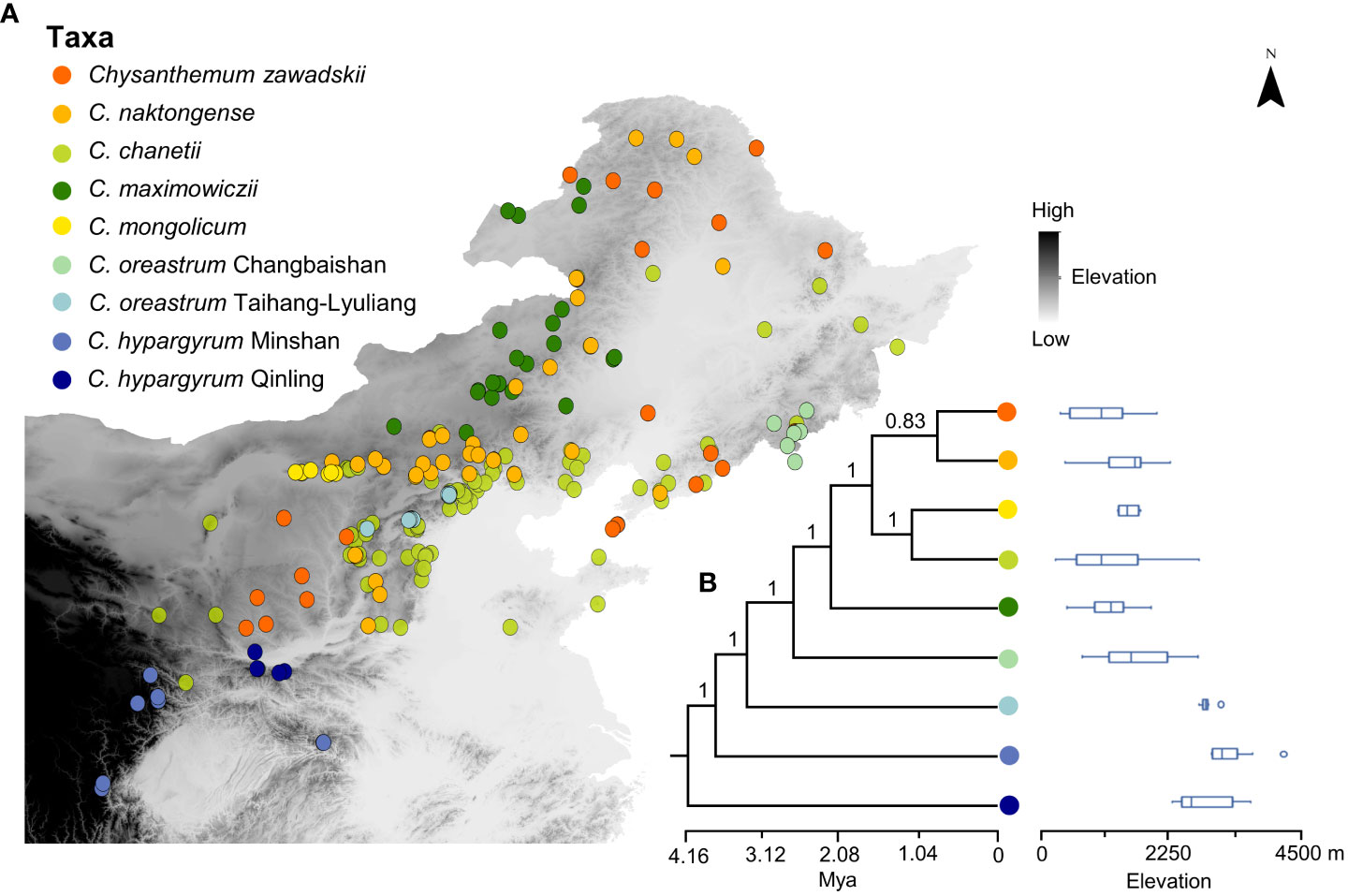
Figure 1 (A) Geographic localities of species within the Chrysanthemum zawadskii species complex in China. Grey shading indicates the elevation gradient, and darker colors represent higher elevation. (B) Phylogenetic relationships of the C. zawadskii complex species and different elevation ranges of the species. Colored circles represent different species and intraspecific lineages of this complex. Numbers at the nodes refer to Bayesian posterior probability. The geographic coordinates of each locality are listed in Table S1.
In this study, we investigated the spatiotemporal variations in niche and geographic range of the C. zawadskii complex in a phylogenetic context to test the hypothesis that geographic range is not merely a proxy for niche breadth of species, while niche evolution, range dynamics or their coupling jointly shape the current distribution of species. Our goals were to address the following questions: 1) whether the niches of species evolve over time in the complex, and what is their evolutionary tendency to specialize or generalize? 2) what are the main climatic factors driving the divergence of niches in the complex? and 3) what are the historical dynamics of range shifts of the complex at multiple evolutionary scales? In particular, we first investigated the niche breadths and overlaps between lineages or species in the complex. Secondly, we tested phylogenetic signals for different climatic variables and estimated the ancestral niches at multiple phylogenetic levels. We then used phyloclimatic modeling to reconstruct the dynamics of range shifts of this complex. Finally, we explored the processes of range shifts and niche evolution and their relative importance in the distribution and diversification of the C. zawadskii complex.
2 Materials and methods
2.1 Data collection
We collected occurrence records of the Chrysanthemum zawadskii complex from our field surveys and relevant databases, such as the Chinese Virtual Herbarium and the Global Biodiversity Information Facility (Figure 2). All the occurrences were manually checked to remove incorrect records and records without herbarium images. To conduct ENM analyses, duplicate records per raster cell (2.5 arc-minute) were filtered out with ENMtools v. 1.4.3 (Warren et al., 2010). In addition, the records of four widespread species (i.e., C. zawadskii, C. maximowiczii, C. naktongense, and C. chanetii) were further thinned using the ‘spThin’ v. 0.2.0 R package (Aiello-Lammens et al., 2015) in R v. 4.1.3 (R Core Team, 2022) to keep pairwise distances between the records greater than 10 km for avoiding spatial sampling biases. Overall, we retained 197 points of occurrence that covered the entire distribution range of this complex in China (Table S1, Figure 1).
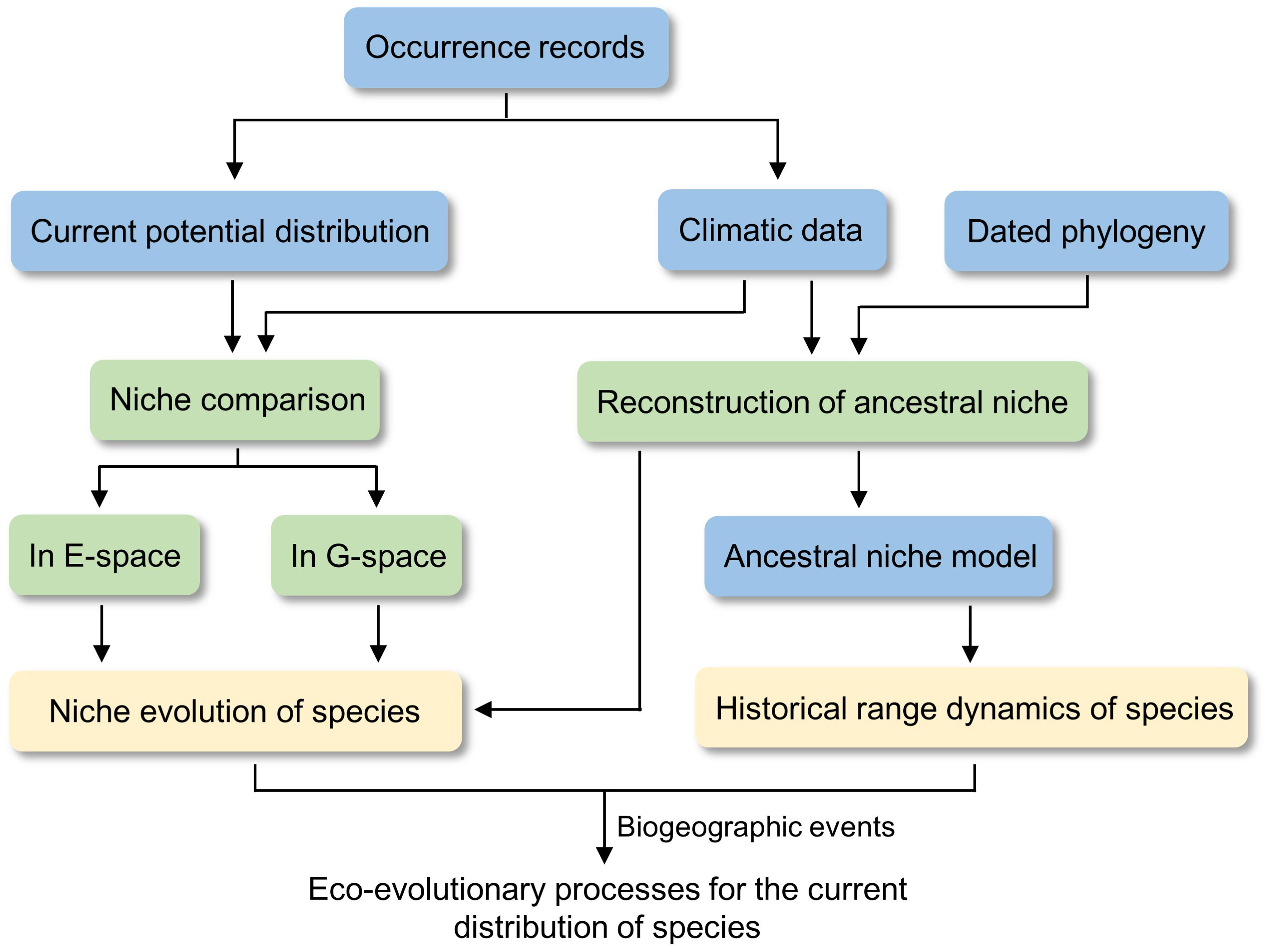
Figure 2 General workflow of the niche comparison and phyloclimatic modeling in this study. E-space and G-space indicate environmental space and geographic space.
We extracted 19 current climatic variables from WorldClim v. 1.4 at 2.5 arc-minute resolution (Hijmans et al., 2005) for ENM analyses and reduced the geographical extent of climatic layers to our study area. To avoid potential multicollinearity effects, we calculated Pearson’s correlation coefficients between the climatic variables with ENMTools. We then selected four relatively independent variables (Pearson’s coefficient < 0.8), including annual mean temperature, temperature annual range, annual precipitation, and precipitation seasonality, for further analyses (Table 1).
2.2 Ecological niche modeling
To provide potential distributions of species for subsequent niche comparisons, we generated ENMs for each species and lineage of the complex. According to the phylogeny of the C. zawadskii complex (Lu et al., 2022), each of two narrowly distributed species, C. oreastrum and C. hypargyrum, contains two distinct geographical lineages. Therefore, we conducted ENM analyses for both species and intraspecific lineages in this study (Figure 2). We here chose the well-tuned boosted regression trees (BRT) model, which is regarded as one of the top modeling algorithms for ENM analyses (Valavi et al., 2022). We first generated 10,000 pseudo-absences for this complex based on the “random” strategy in our study area with the ‘biomod2’ v. 4.1.2 R package (Thuiller et al., 2022). Second, we tuned BRT models for each species and lineage of the complex. In detail, three hyperparameters of the BRT model (i.e., “interaction.depth”, “shrinkage”, and “n.trees”) were tuned following the guide of Elith et al. (2008) in the ‘SDMtune’ v. 1.1.6 R package (Vignali et al., 2020). We set five alternative values for “interaction.depth” (1, 3, 5, 7, 9) and three values for “shrinkage” (0.001, 0.005, 0.01), and values of “n.trees” were set at a range from 5,000 to 10,000 in increments of 500. We selected the best combination of those hyperparameters according to the area under the receiver operator characteristic curve (AUC) metric. After model tuning, we finally conducted BRT models for each species and lineage to predict their current potential distributions in ‘biomod2’. More specifically, we set other model parameters as default values (“distribution”: bernoulli; “bag.fraction”: 0.5) in ‘biomod2’ and ran the well-tuned BRT model for each species or lineage with ten replicates of cross-validation. Each replicate of models was trained with 80% of the occurrences and tested based on the remaining data. We assessed the performance of each model according to the true skill statistic (TSS) and AUC metrics. Given that TSS values > 0.7 indicate high model performances, we chose the models whose TSS values are greater than 0.7 to generate the ensemble model for each species or lineage to make subsequent projections. The ensemble models of each species or lineage were generated by using the probability-weighted mean method, and then those ensemble models were projected into the current climatic layers to predict the potential geographic distribution of species in this complex.
2.3 Niche comparison
To compare the niches among different species or lineages of this complex, we quantified their niche overlap and breadth from two different perspectives: in environmental space (E-space) and geographic space (G-space), respectively (Figure 2). Niche comparison in G-space is based on geographic ranges of species which are often inferred by ENMs in many studies, while niche comparison in E-space often uses the environmental information obtained directly from the occurrence locations to quantify the niches of species (Brown and Carnaval, 2019).
For niche comparisons in G-space, we first quantified the niche breadth of each species or lineage using Levins’ B metric, and a higher B value represents a broader ecological niche (Levins, 1968). Then, niche overlap between different species was estimated by Schoener’s D metric (Schoener, 1968) in which high D values indicate that different species may occupy similar niches. It is important to note that the niche overlap and niche breadth in G-space could be overestimated if the areas with very low habitat suitability were included in the calculation (Rödder and Engler, 2011). Thus, we need a threshold to determine the presence or absence of species per raster cell. The “maximizing the sum of sensitivity and specificity” (maxSSS) statistic is regarded as a well-performing threshold selection method for transforming suitability continuous predictions to binary outputs (Liu C. et al., 2013). As a result, we calculated the maxSSS value for each species or lineage with the ‘dismo’ v. 1.3-5 R package (Hijmans et al., 2022), and the habitat suitability value per raster cell below the maxSSS value was set to 0. Finally, we estimated Levins’ B and Schoener’s D based on the binary suitability maps of species and lineages with the ‘ENMtools’ v. 1.0.6 R package (Warren et al., 2021).
We then compared the niches among different species or lineages in E-space. As the Hutchinsonian niche concept describes the niche of a species as an n-dimensional hypervolume, we here used the functional richness (FRic) metric to quantify the niche breadth of species in E-space. FRic is defined as the amount of functional space occupied by the species, and it can be calculated by using probabilistic hypervolumes in trait space (Carmona et al., 2019). Given that some species of the complex, such as C. chanetii, C. naktongense, and C. oreastrum often occur at different elevations on the same mountain, we consider that data from WorldClim may not reflect the niche differences of those species. Therefore, we used ClimateAP v. 2.20 which integrates the local elevation information of species to provide climatic variables with increased spatial accuracy (Wang et al., 2017). We obtained 16 scale-free climatic variables (Table 1) using ClimateAP based on the latitude, longitude, and altitude of species occurrences. We then performed principal component analysis (PCA) for those 16 climatic variables to summarize these variables on two principal component axes with the ‘FactoMineR’ v. 2.6 R package (Lê et al., 2008). We calculated the FRic value for each species and lineage by the ‘TPD’ v. 1.1.0 R package (Carmona et al., 2019) according to the values of PC1 and PC2. To estimate niche overlaps between the species pairs in E-space, we selected the PCA-env approach based on climatic data of presence records and background points (Broennimann et al., 2012). We generated a minimum convex polygon (MCP) for each species or lineage and then defined the geographical backgrounds as MCPs with a one-degree width buffer area. We randomly selected 1,000 points for each species in the background areas and obtained the climatic data of these background points in ClimateAP. We subsequently estimated Schoener’s D and tested the niche similarity for different species pairs in the ‘ecospat’ v. 3.4 R package (Di Cola et al., 2017). In detail, the niche similarity of each species pair was assessed with 1000 randomization tests. Those tests generated a null distribution based on Schoener’s D values between a species and background points of another species in the pair. If the Schoener’s D values of the null distribution are significantly lower than that of the target species pair, these two species may share similar niches than expected by chance.
2.4 Phylogeny reconstruction and divergence time estimation
To reconstruct a dated phylogeny at the species level, we downloaded a set of nuclear loci that included 100 exons with the highest number of parsimony-informative sites (Lu et al., 2022). Because there is no fossil data for Chrysanthemum species, we reconstructed the species tree using the uncorrelated relaxed clock model in *BEAST v. 1.8.4 (Suchard et al., 2018) with the clock rate of 8.37 × 10−4 – 2.61 × 10−3 substitutions/site/million years. This clock rate is based on the standard rate of nucleotide substitution for the Asteraceae (Kay et al., 2006) and three years of the generation time for species of this complex. We selected substitution models based on the Bayesian Information Criterion with ModelFinder (Kalyaanamoorthy et al., 2017), and the optimal model was TN + F + G4. We used the Yule process as the species tree prior and selected the Piecewise linear and constant root as the population size model. We ran two independent analyses with 1 × 107 Markov chain Monte Carlo generations based on a random starting tree, and the trees were sampled every 1,000 generations. The results of these two runs were merged using LogCombiner v. 1.8.4 (Suchard et al., 2018), and then the convergence of these results was evaluated with Tracer v. 1.7 (Rambaut et al., 2018). Finally, a maximum clade credibility tree was summarized by TreeAnnotator v. 1.8.4 (Suchard et al., 2018).
2.5 Reconstructions of ancestral niche and historical distribution
To reconstruct the ancestral niche of the complex, we first tested the phylogenetic signal for the 16 climatic variables obtained from ClimateAP based on the dated species tree in the ‘phylosignal’ v. 1.3 R package (Keck et al., 2016). Three metrics, including Moran’s I, Blomberg’s K, and Pagel’s λ, were calculated, and the significance of these metrics was assessed by 1,000 randomization tests. Moran’s I = 0 indicates that a trait evolved under Brownian motion (BM); I < 0 indicates no phylogenetic signal, and I > 0 suggests that the related species have more similar traits. Similarly, Bloomberg’s K of 1 indicates that trait distribution follows BM; K < 1 indicates no phylogenetic signal, and K > 1 implies that the traits of related species are more similar than those predicted under BM. Pagel’s λ usually ranges between 0 and 1; λ= 1 also indicates that the trait evolved according to the phylogeny, and λ = 0 indicates no phylogenetic signal. However, λ can > 1 when a trait of a related species is more similar than expected under BM (Münkemüller et al., 2012). We then tested the optimal model of niche evolution for those 16 climatic variables using the ‘geiger’ v. 2.0.10 R package (Harmon et al., 2008). Four models including BM, Ornstein–Uhlenbeck (OU), Early Burst (EB), and White Noise (WN) were selected, and we used the Akaike’s Information Corrected Criterion (AICc) value to evaluate model performance. The BM model was the optimal model for all variables (Table S2). Finally, we selected the BM model to estimate the ancestral niches under a maximum likelihood framework by the ‘phytools’ v. 1.2-0 R package (Revell, 2012).
After reconstructing the ancestral niche of species in the complex, we further used phyloclimatic modeling to estimate the ancestral distribution ranges of those species for exploring their historical shifts in ranges (Figure 2). Firstly, we obtained the paleo-climatic data of the same four climatic variables that were used in previous ENM analyses at seven past time periods (i.e., 3.79 Mya, 3.36 Mya, 2.74 Mya, 2.24 Mya, 1.68 Mya, 1.15 Mya, and 0.80 Mya) from the Oscillayers datasets for phyloclimatic modeling (Gamisch, 2019). We focused on those seven time periods because they correspond to the divergence times of species in the complex. All of those variables were at 2.5 arc-minute, and their geographical extents were also reduced to our research area (Table 1). Next, we constructed Bioclim models for each species and lineage of the complex using their current occurrences and climate data in the ‘machuruku’ v. 1.8.3 R package (Guillory and Brown, 2021). The niches of species were described by three parameters including the mean, standard deviation, and skewness of climatic variables, and those parameters were used as inputs for subsequent analyses. We then estimated ancestral climatic parameters of species based on the dated phylogeny and those climatic parameters. Finally, we converted those ancestral climatic parameters into ancestral niche models of species with the ‘machuruku’. All those niche models were projected into corresponding paleo-climate layers of the above seven time periods to reconstruct the ancestral geographic ranges of species in the complex, and those ancestral ranges were converted to binary suitability maps with the ‘machuruku’.
3 Results
3.1 ENMs and niche comparisons
The AUC and TSS values of all the ENMs were greater than 0.90 (Table S3), indicating that all the models performed well. The ENM results for most of the species and lineages in the current period were consistent with their actual distribution in China, except for C. hypargyrum Qinling lineage and C. oreastrum Taihang-Lyuliang lineage (Figures 1, S1). In addition, the maxSSS values of those species varied from 0.305 (C. zawadskii) to 0.742 (C. mongolicum). Given that BRT models were not effective for those two lineages, we did not estimate their niche breadth in G-space. The Levins’ B values in G-space for other taxa of this complex showed that narrowly distributed species possessed lower Levins’ B values than widespread species (Table S3). The estimations of niche breadth for all species and lineages in E-space also obtained similar results. Species with wider ranges of distribution, such as C. zawadskii, C. naktongense, and C. chanetii, had higher FRic values, and the FRic values of some narrowly distributed species or lineages were even less than one-tenth of the former (Figure 3).
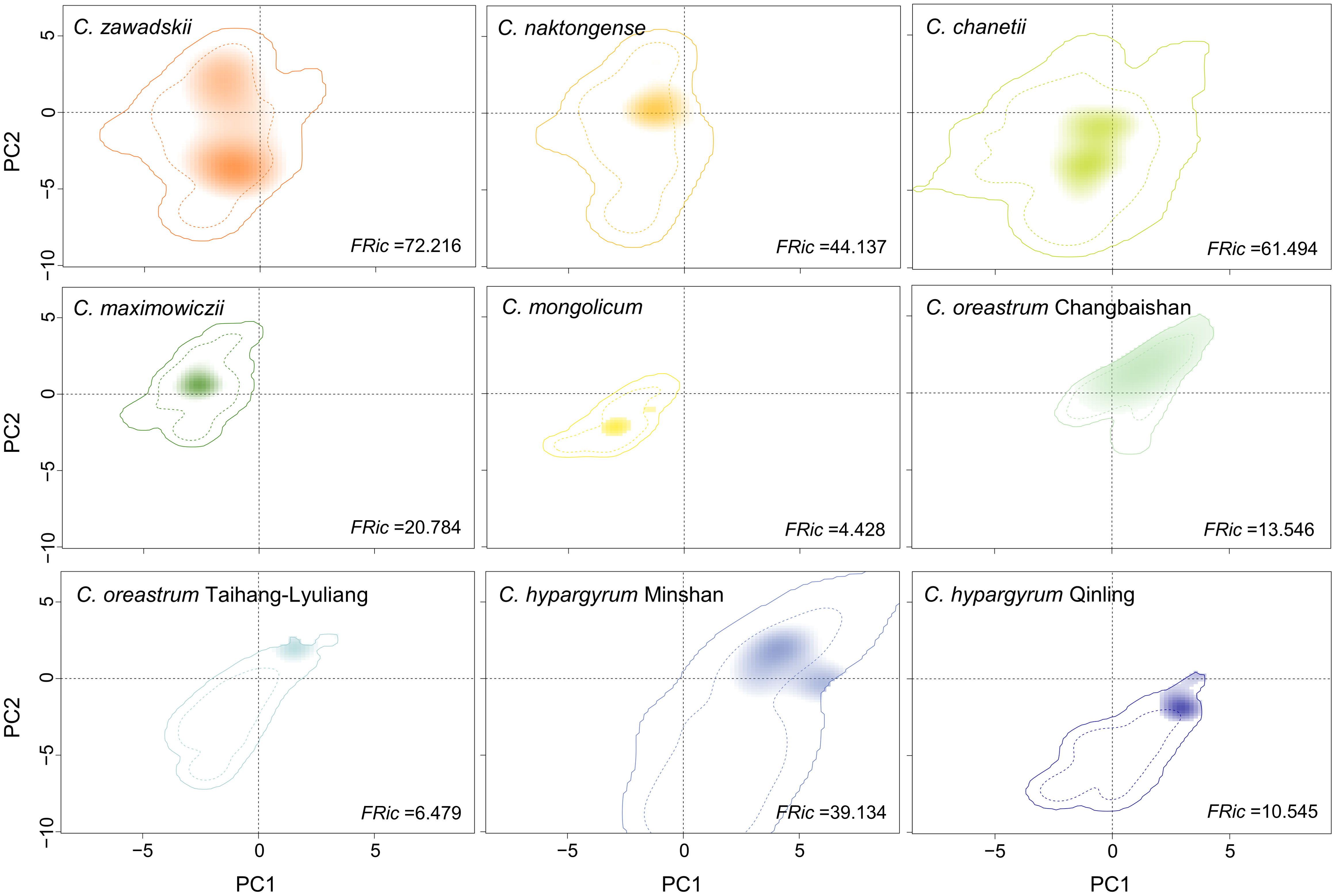
Figure 3 Climatic conditions occupied by different species and intraspecific lineages of the Chrysanthemum zawadskii complex in environmental space. The solid and dashed contour lines represent 100% and 50% of the background environments, respectively. The tint-to-dark shading of each species or intraspecific lineage shows the density of the occurrences by cell. FRic indicates the Functional richness metric.
Analyses of niche overlap in the E-space suggested that the niche differentiation greatly varied among the taxa of this complex in which the Schoener’s D values ranged from 0 to 0.462 (Table 2). The niche overlaps between the three widespread species (C. zawadskii, C. naktongense, and C. chanetii) were relatively high (D = 0.279 to 0.462). Moreover, the niche similarity tests showed that C. chanetii and C. zawadskii possessed significantly more similar environmental conditions than expected by chance (p < 0.05, Table S4). Schoener’s D values between intraspecific lineages of two narrowly distributed species, i.e., C. hypargyrum and C. oreastrum, were relatively low (D = 0 to 0.089). Meanwhile, the results of the niche similarity tests suggested that Schoener’s D values between intraspecific lineages of those two species were not significantly higher than that of the null distribution (Table S4). C. maximowiczii, a species that occurs in grasslands, showed relatively low niche overlap with other species. Similarly, another endemic species, C. mongolicum, also had little or no overlap with other species. Interestingly, the niche overlap in G-space showed a similar pattern to that in the E-space (Table S5).
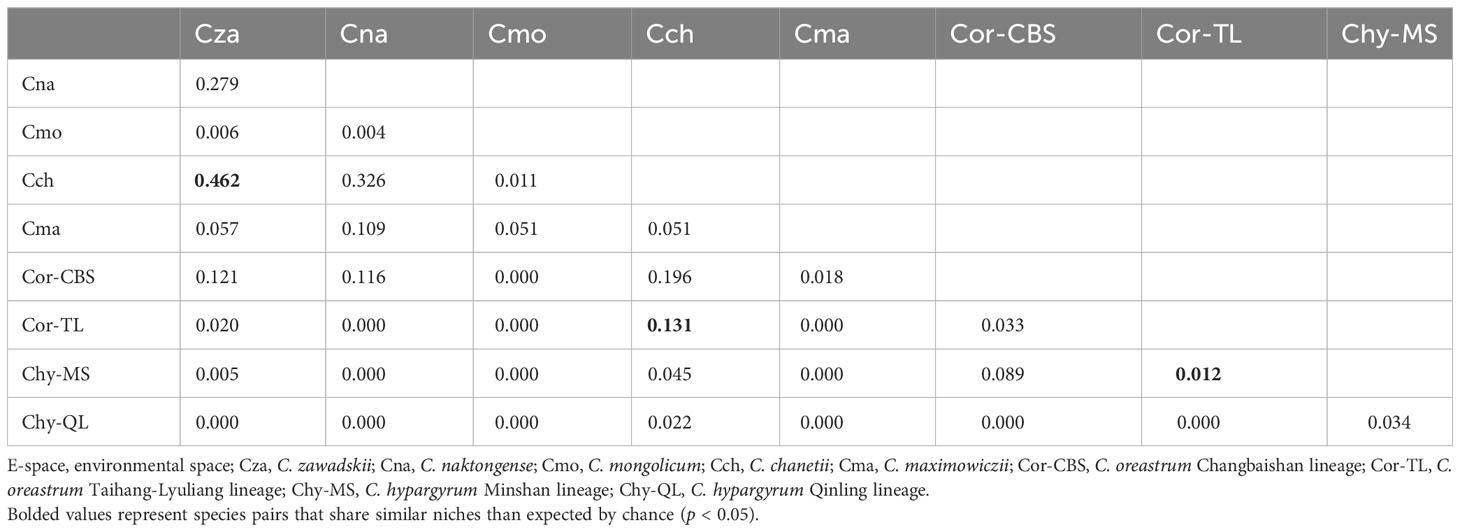
Table 2 The Schoener’s D values between species and lineages of the Chrysanthemum zawadskii species complex in E-space.
3.2 Divergence time and niche evolution
The mean divergence times of the main nodes (Figures 1, S2) estimated under a relaxed clock model were similar to those estimated by Lu et al. (2022). In particular, the C. hypargyrum Qinling lineage diverged first about 4.16 million years ago (Mya), and other lineages or species have then emerged since the mid-Pliocene (ca. 3.78 Mya, Figure S2). Based on Moran’s I, Blomberg’s K, and Pagel’s λ, we detected statistically significant phylogenetic signals for three of the 16 climatic variables, including the annual heat: moisture index, precipitation as snow, and Hargreaves climatic moisture deficit (Table 3). We identified the BM model as the evolution model that best fits these climatic variables according to the AICc statistics (Table S2). The results of ancestral state estimation under the BM model suggested that there is niche divergence between the four early-diverged alpine lineages and the core C. zawadskii complex (Figure 4). In comparison to the alpine lineages, the species of core C. zawadskii complex have evolved toward climatic conditions with less precipitation and higher temperature (Figures 4, S3). The kernel density plots in E-space also suggested that there was differentiation in the climatic conditions occupied by different species (Figure S4).
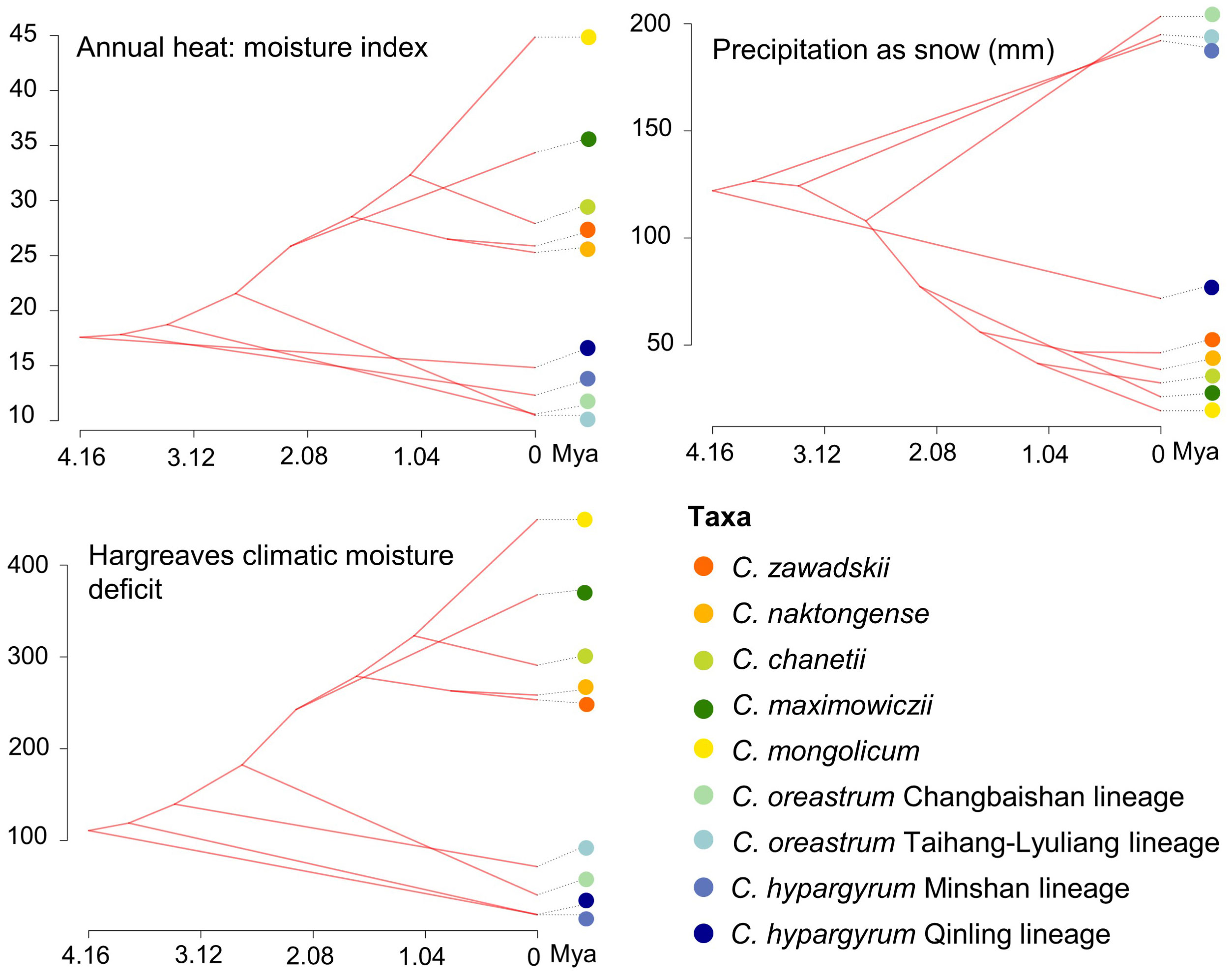
Figure 4 Reconstruction of the ancestral niches for different species and intraspecific lineages of Chrysanthemum zawadskii complex in environmental space. Colored circles represent different species and intraspecific lineages of this complex.
3.3 Changes in the geographic range of the complex across evolutionary times
Our results of phyloclimatic modeling showed that this complex originated in the Qinling mountains and its surrounding areas during the early Pliocene (Figures 5, 6). These results also showed that this complex diverged with its expansion to northern China along mountain ranges (Figures 6, S5). Additionally, the geographical ranges of C. hypargyrum and C. oreastrum showed little change and seem to have contracted over evolutionary time (Figure 5). For example, the suitable habitats of these alpine lineages decreased from 2.24 Mya to the present, except for the C. oreastrum Taihang-Lyuliang lineage. In contrast, there were several range expansions for the core C. zawadskii complex during its diversification (Figure 6). From 2.74 to 2.24 Mya, we detected a large shift in the geographical range of the core C. zawadskii complex. Another two range expansions occurred at 1.68 Mya and 1.15 Mya for the core C. zawadskii complex. We then checked the response curves and statistical values of four climatic variables for the core C. zawadskii complex at four different past periods (Figure 6, Table 4). The 95% confidence interval of those climatic variables indicated that the niche breadth of the core C. zawadskii complex did not increase obviously from 2.74 to 2.24 Mya (Figure 6, Table 4). Notably, the temperature-related climatic variables had high confidence interval overlap, and their mean values showed little difference between the above two past periods. Conversely, the mean values of the precipitation variables changed more clearly. In addition, as the core C. zawadskii complex diversified, the mean values and 95% confidence interval of those climatic variables greatly varied among species of the core complex.
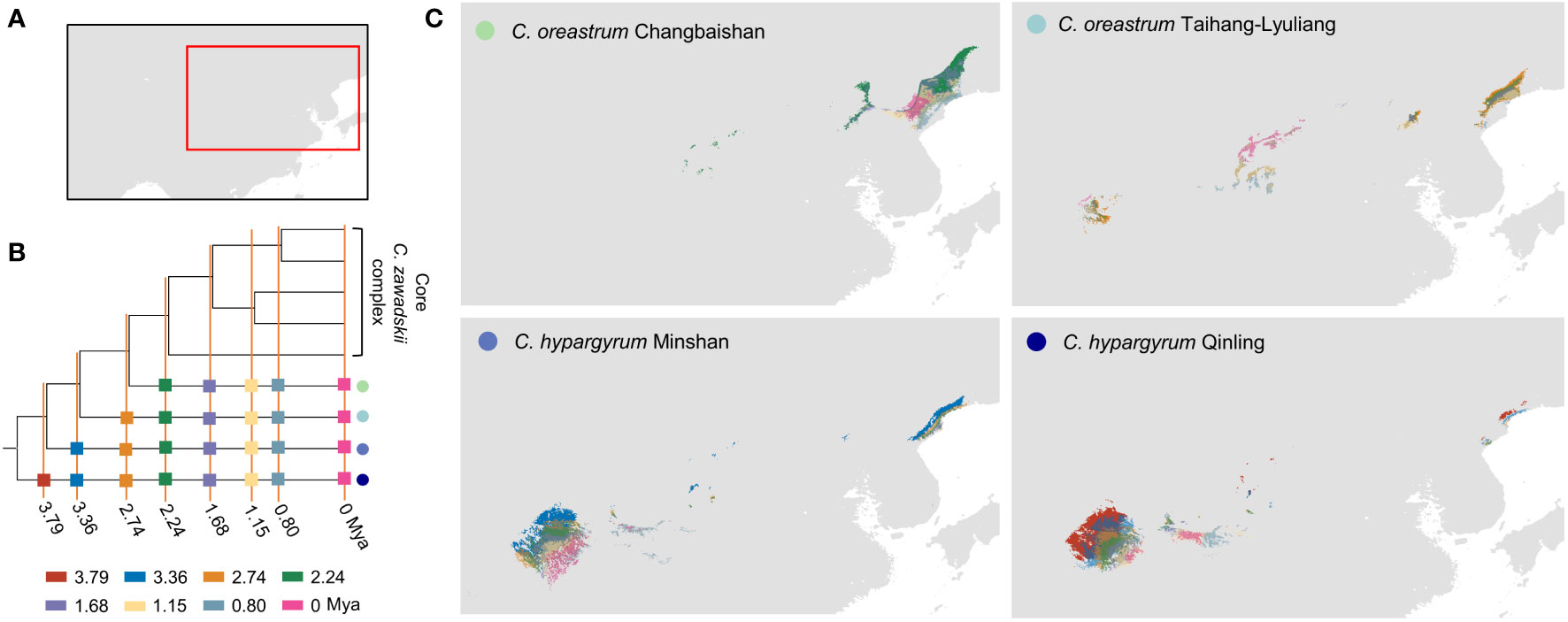
Figure 5 Reconstructions of the ancestral area of narrowly distributed alpine lineages of the Chrysanthemum zawadskii complex. (A) A map shows areas in this study. (B) The phylogenetic tree was used to reconstruct the ancestral niche and distribution, with eight past time periods that were focused on in this study. (C) Dynamics of ancestral distribution for those four alpine lineages of this complex. Colored circles and rectangles represent different intraspecific lineages of this complex and different past time periods, respectively.
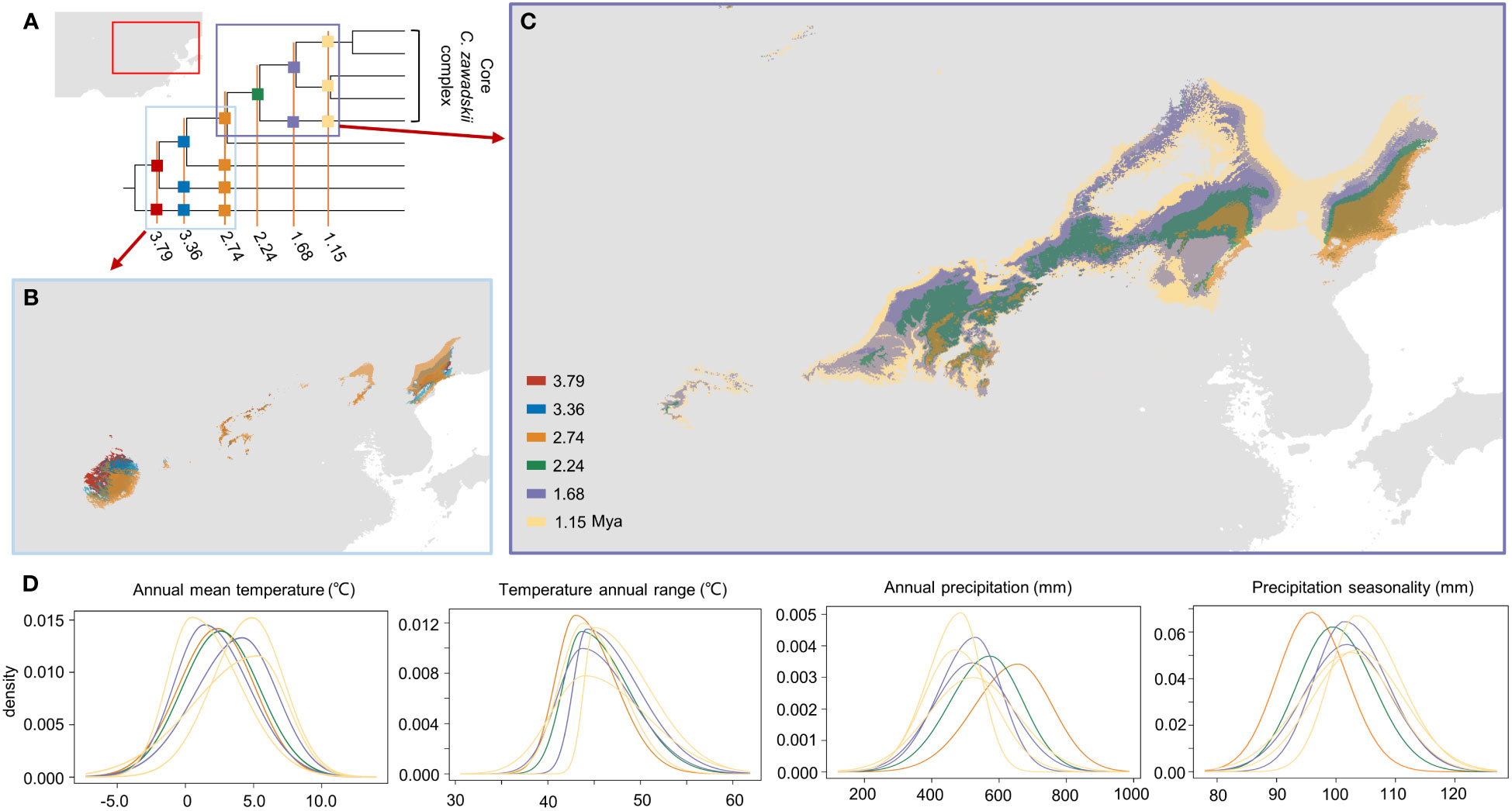
Figure 6 Dynamics of range and niche breadth of species in the Chrysanthemum zawadskii complex. (A) The phylogenetic tree was used to reconstruct the ancestral niches and ranges, and the map inset at the top left shows the study areas in this work. (B) Ancestral area reconstruction of this complex on the first three past time periods (in the blue rectangle: 3.79 Mya, 3.36 Mya, and 2.74 Mya). (C) Range shifts of the core C. zawadskii complex across four past time periods (in the purple rectangle: 2.74 Mya, 2.24 Mya, 1.68 Mya, and 1.15 Mya). (D) Response curves of four climate variables across four past time periods (2.74 Mya, 2.24 Mya, 1.68 Mya, and 1.15 Mya). Colored rectangles and curved lines correspond to different past time periods, separately.
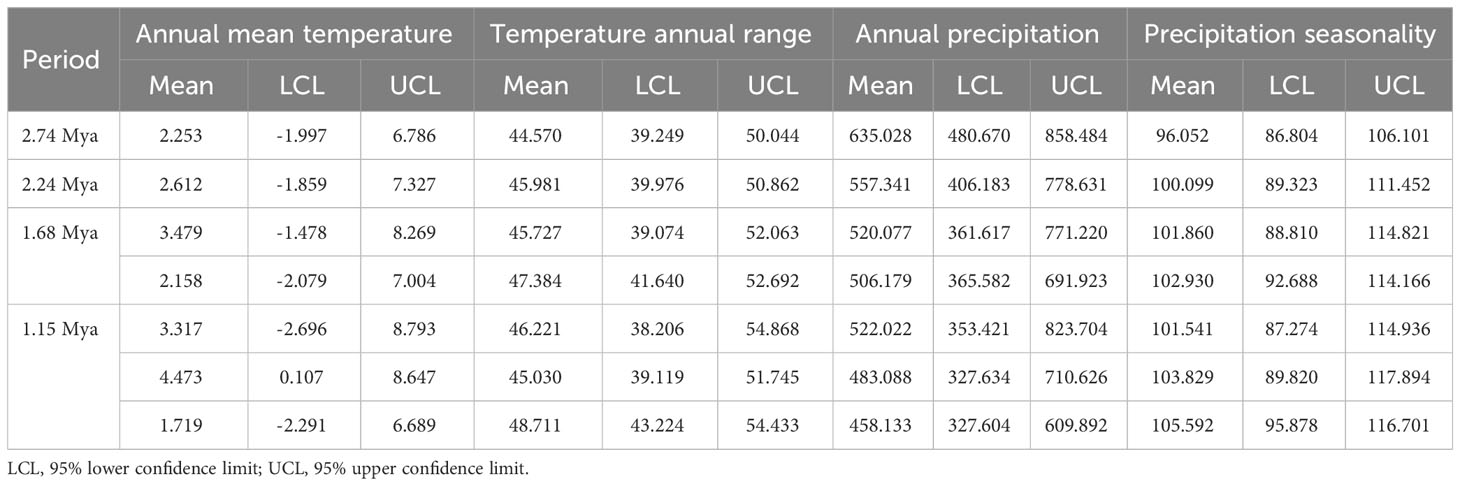
Table 4 Statistical values of the response curves of four climate variables for the core Chrysanthemum zawadskii complex across four past time periods.
4 Discussion
4.1 Closely related yet different: phylogenetic relatedness and niche divergence among species of the C. zawadskii complex
According to our findings, the territories of Qinling Mountains showed favorable environmental conditions to host the ancestor of C. zawadskii complex in the early Pliocene, making this region eligible as probable land where C. hypargyrum, C. oreastrum, and the core C. zawadskii complex might have evolved. The formation of four alpine geographic lineages could result from mountain uplifts, such as those that took place 4.1–3.4 Mya for the Minshan Mountains and 5.3–3.6 Mya and 2.6 Mya for the Taihang Mountains, and the resultant geographical barriers between alpine populations since the early Pliocene (Zhang et al., 2005; Gong, 2010; Shi et al., 2020). In addition, species of the complex have a relatively weak ability to disperse owing to their achenes that lack pappi or wings (Shi et al., 2011). Both geographical barriers and limited dispersal capacity restricted the gene flow between alpine populations or lineages, and then local selection and/or drift facilitated the formation of four alpine lineages.
Notably, although these alpine lineages have diverged, they still prefer habitats with relatively humid climate conditions and low mean warmest month temperature (Figures 4, S3), which suggests their phylogenetic niche conservatism in some niche axes. Unlike alpine lineages, species of the core C. zawadskii complex can tolerate hotter and drier environmental conditions. The ancestral niche estimations indicate that the variables related to precipitation exhibit more pronounced divergence within the complex than those of the temperature factors (Figure 4, Table 3). In fact, the relative importance of temperature and precipitation in driving the distribution and diversification of species is not identical among different plant taxa (Moles et al., 2014; Häkkinen et al., 2022). A recent study indicated that many plants tend to adapt to novel precipitation regimes, whether wetter or drier, rather than adapting to hotter or colder climates, suggesting the importance of precipitation in the niche evolution of species (Häkkinen et al., 2022). In this study, we can deduce that niche differentiation within the complex might be primarily driven by the moisture gradient. According to our results, the common progenitor of the core C. zawadskii complex split from the alpine lineages at approximately 2.73 Mya, and then its distribution range extended to the Chinese Loess Plateau (CLP) and surrounding areas (Figure 6). Previous studies showed that there was a major change in the precipitation gradient on the CLP during the Pliocene-Quaternary boundary, and the climate became dry and cold (Wu et al., 2007; Nie et al., 2013; Peng et al., 2018). The progenitors of the core C. zawadskii complex could have quickly adapted to such a change and then expanded into drier habitats. Additionally, analyses of paleosol pollen assemblages from the CLP suggested that open habitats increased during this period (Hui et al., 2021). Species of this complex generally occur in open habitats, such as grassland, slope, and forest edges (Shi et al., 2011), and such changes could provide more ecological opportunities for range expansion and diversification of the core C. zawadskii complex.
4.2 Evolution of niches: from specialist to generalist or vice versa
Comparative analyses of niches showed that there exists a distinct niche divergence among species of the complex (Table 2, Figure 3). Interspecific variations in the phenotypic traits, habitats, and distribution ranges of the C. zawadskii complex implied that niche evolution occurred (Lu et al., 2022). Multiple niche analyses in this study supported that shifts in niche breadth occurred in the complex. For decades, there was a consensus that specialization is an evolutionary dead end (Moran, 1988; Schluter, 2000; Vamosi et al., 2014). Nonetheless, a growing number of studies have indicated that such a “consensus” might not be general, and that it is possible to transition from specialists to generalists (Armbruster and Baldwin, 1998; Dennis et al., 2011; Donoghue and Edwards, 2014; Vamosi et al., 2014; Day et al., 2016; Sexton et al., 2017; Rombaut et al., 2022). Within this complex, early divergent alpine lineages possess narrow niches, and they are more likely to be specialists than generalists. Another small-range species, C. mongolicum, also has a narrow niche, and it is sympatric with its sister species, C. chanetii with a large range (Lu et al., 2022). Taking together the findings described above and the climatic tolerances of ancestral nodes under the phylogenetic framework, we found that specialists can be converted to generalists and vice versa in this complex. Notably, niche comparison in G-space could be affected by the results of ENMs, so such comparisons may not be applicable to some rare species. By comparison, niche comparisons in E-space based on the environmental information of occurrence locations appear to be more stable and reliable.
Climate variability is often regarded as a critical factor in determining the niche breadth of a species (Quintero and Wiens, 2013). Many studies examined the relationship among species range, niche breadth, and habitat climate variability, and they found that species that originated from regions with variable climates could evolve broader niche breadths than their allied species in stable habitats (Stevens, 1989; Addo-Bediako et al., 2000; Quintero and Wiens, 2013; Pintor et al., 2015; Li et al., 2016; Mumladze et al., 2017). Reconstruction of ancestral niche indicated that species of the core C. zawadskii complex have evolved broader niche breadths since 2.24 Mya (Figure 6). During the early Pleistocene (2.2–1.7 Mya), the East Asian summer monsoon (EASM) gradually intensified in the CLP (Hui et al., 2021), and the dramatic seasonal climatic fluctuations in this region enhanced the niche breadth of the core C. zawadskii complex. Nonetheless, the alpine lineages did not evolve increased niches or undergo range expansion (Figure 5). We hypothesized that alpine lineages could survive in situ mountain refugia with stable climates and adapt to alpine habitats rather than expanding to lower altitudes because of the geographical barriers and their limited ability to disperse (Bai et al., 2010; Zhao et al., 2013; Guo et al., 2014).
4.3 Coupled changes between range size and niche breadth in the evolution of C. zawadskii complex
Speciation is usually a lengthy process with niche evolution and range changes (Stankowski and Ravinet, 2021), and the current pattern of distribution and niche of species represents the consequences of this process. When we integrate snapshots of niches and ranges of species across different historical periods, the complete picture of an eco-evolutionary process that shapes species distribution can be reconstructed. The progenitor of this complex probably adapted to relatively cold climates and diverged from the other Chrysanthemum lineages with the uplift of Qinba mountains since the late Miocene (Liu J. et al., 2013; Meng, 2017; Shi et al., 2020; Lu et al., 2022). Subsequently, the complex spread west- and northwards along the mountain ranges to northern China, and then rode the rising mountains and occupied alpine habitats. Some lineages (C. hypargyrum and C. oreastrum) adapted to survive in situ, and others expanded into drier climates in northern China since the early Pleistocene. Changes in vegetation and the EASM evolution in the CLP during the early Pleistocene enhanced broader niches and larger range sizes of the core C. zawadskii complex.
The spatiotemporal dynamics of the niches and ranges of the C. zawadskii complex indicate that the coupled changes between ranges and niches drive the range expansion of the complex, which is more consistent with the range shift-niche breadth hypothesis (Lancaster, 2022). Climate fluctuation and the decrease of interspecific competition since the Pleistocene provided an opportunity for this herbaceous species complex to expand its range (Wu et al., 2007; Nie et al., 2013; Peng et al., 2018; Hui et al., 2021), and the niche breadth increased in pace with the expansion of its range into areas that have a highly variable climate, which further promoted the range expansion. Notably, even though the species are closely related, the patterns of range shifts are diverse across taxa of the complex, which suggests that historical biogeographic processes also affect the distribution of species.
In sum, by integrating the findings from phyloclimatic modeling, niche comparisons, and biogeographic analyses, we can better understand the processes and mechanisms behind the changes in geographic range and niche evolution. Nonetheless, the reconstruction of ancestral distribution ranges by phyloclimatic modeling is still in the early developmental phase, and some factors, such as the reliability of paleoclimatic data and robustness of phylogeny, could dramatically influence the results (Gamisch, 2019; Rivera et al., 2020; Guillory and Brown, 2021). Although we weighed and chose the appropriate climatic variables for the complex in the current period, we could not say that the paleoclimatic data of the same variables are the best fit for estimating the ancestral distribution of the target species. In addition, we here focused on the niche of species on a larger scale, but microhabitats could also influence the niche differentiation of species and their distribution (Lawson et al., 2014). Therefore, we need to consider more factors that affect niche evolution and range dynamics of species and incorporate more data in our future works.
Data availability statement
The original contributions presented in the study are included in the article/Supplementary Material. Further inquiries can be directed to the corresponding author.
Author contributions
W-XL and G-YR conceived the ideas. W-XL, Z-ZW and X-YH collected the data. W-XL designed methodology and analyzed the data. W-XL and G-YR led the writing of the manuscript. All authors contributed to the article and approved the submitted version.
Funding
The author(s) declare financial support was received for the research, authorship, and/or publication of this article. This work was supported by the National Natural Science Foundation of China (Grant No. 31970226 and 32270235).
Conflict of interest
The authors declare that the research was conducted in the absence of any commercial or financial relationships that could be construed as a potential conflict of interest.
Publisher’s note
All claims expressed in this article are solely those of the authors and do not necessarily represent those of their affiliated organizations, or those of the publisher, the editors and the reviewers. Any product that may be evaluated in this article, or claim that may be made by its manufacturer, is not guaranteed or endorsed by the publisher.
Supplementary material
The Supplementary Material for this article can be found online at: https://www.frontiersin.org/articles/10.3389/fevo.2023.1250491/full#supplementary-material
References
Addo-Bediako A., Chown S. L., Gaston K. J. (2000). Thermal tolerance, climatic variability and latitude. Proc. R. Soc. B: Biol. Sci. 267, 739–745. doi: 10.1098/rspb.2000.1065
Aiello-Lammens M. E., Boria R. A., Radosavljevic A., Vilela B., Anderson R. P. (2015). spThin: an R package for spatial thinning of species occurrence records for use in ecological niche models. Ecography 38, 541–545. doi: 10.1111/ecog.01132
Anacker B. L., Strauss S. Y. (2014). The geography and ecology of plant speciation: range overlap and niche divergence in sister species. Proc. R. Soc. B: Biol. Sci. 281, 20132980. doi: 10.1098/rspb.2013.2980
Armbruster W. S., Baldwin B. G. (1998). Switch from specialized to generalized pollination. Nature 394, 632–632. doi: 10.1038/29210
Bai W.-N., Liao W.-J., Zhang D.-Y. (2010). Nuclear and chloroplast DNA phylogeography reveal two refuge areas with asymmetrical gene flow in a temperate walnut tree from East Asia. New Phytol. 188, 892–901. doi: 10.1111/j.1469-8137.2010.03407.x
Bridle J., Hoffmann A. (2022). Understanding the biology of species’ ranges: when and how does evolution change the rules of ecological engagement? Philos. Trans. R. Soc. B: Biol. Sci. 377, 20210027. doi: 10.1098/rstb.2021.0027
Broennimann O., Fitzpatrick M. C., Pearman P. B., Petitpierre B., Pellissier L., Yoccoz N. G., et al. (2012). Measuring ecological niche overlap from occurrence and spatial environmental data. Global Ecol. Biogeogr. 21, 481–497. doi: 10.1111/j.1466-8238.2011.00698.x
Brown J. H., Maurer B. A. (1989). Macroecology: the division of food and space among species on continents. Science 243, 1145–1150. doi: 10.1126/science.243.4895.1145
Brown J. L., Carnaval A. C. (2019). A tale of two niches: methods, concepts, and evolution. Front. Biogeogr. 11, e44158. doi: 10.21425/F5FBG44158
Carmona C. P., de Bello F., Mason N. W. H., Lepš J. (2019). Trait probability density (TPD): measuring functional diversity across scales based on TPD with R. Ecology 100, e02876. doi: 10.1002/ecy.2876
Carscadden K. A., Emery N. C., Arnillas C. A., Cadotte M. W., Afkhami M. E., Gravel D., et al. (2020). Niche breadth: causes and consequences for ecology, evolution, and conservation. Q. Rev. Biol. 95, 179–214. doi: 10.1086/710388
Culshaw V., Mairal M., Sanmartín I. (2021). Biogeography meets niche modeling: inferring the role of deep time climate change when data is limited. Front. Ecol. Evol. 9, 662092. doi: 10.3389/fevo.2021.662092
Day E., Hua X., Bromham L. (2016). Is specialization an evolutionary dead end? Testing for differences in speciation, extinction and trait transition rates across diverse phylogenies of specialists and generalists. J. Evolution. Biol. 29, 1257–1267. doi: 10.1111/jeb.12867
Dennis R. L., Dapporto L., Fattorini S., Cook L. M. (2011). The generalism–specialism debate: the role of generalists in the life and death of species. Biol. J. Linn. Soc. 104, 725–737. doi: 10.1111/j.1095-8312.2011.01789.x
Di Cola V., Broennimann O., Petitpierre B., Breiner F. T., D’Amen M., Randin C., et al. (2017). ecospat: an R package to support spatial analyses and modeling of species niches and distributions. Ecography 40, 774–787. doi: 10.1111/ecog.02671
Donoghue M. J., Edwards E. J. (2014). Biome shifts and niche evolution in plants. Annu. Rev. Ecol. Evol. System. 45, 547–572. doi: 10.1146/annurev-ecolsys-120213-091905
Elith J., Leathwick J. R., Hastie T. (2008). A working guide to boosted regression trees. J. Anim. Ecol. 77, 802–813. doi: 10.1111/j.1365-2656.2008.01390.x
Evans M. E., Smith S. A., Flynn R. S., Donoghue M. J. (2009). Climate, niche evolution, and diversification of the “bird-cage” evening primroses (Oenothera, sections Anogra and Kleinia). Am. Nat. 173, 225–240. doi: 10.1086/595757
Favre A., Päckert M., Pauls S. U., Jähnig S. C., Uhl D., Michalak I., et al. (2015). The role of the uplift of the Qinghai-Tibetan Plateau for the evolution of Tibetan biotas. Biol. Rev. 90, 236–253. doi: 10.1111/brv.12107
Gamisch A. (2019). Oscillayers: a dataset for the study of climatic oscillations over Plio-Pleistocene time-scales at high spatial-temporal resolution. Global Ecol. Biogeogr. 28, 1552–1560. doi: 10.1111/geb.12979
Gong M. (2010). Uplifting process of Southern Taihang Mountain in Cenozoic. PhD thesis (Beijing, China: Chinese Academy of Geological Science).
Grossenbacher D. L., Veloz S. D., Sexton J. P. (2014). Niche and range size patterns suggest that speciation begins in small, ecologically diverged populations in North American monkeyflowers (Mimulus spp.). Evolution 68, 1270–1280. doi: 10.1111/evo.12355
Guillory W. X., Brown J. L. (2021). A new method for integrating ecological niche modeling with phylogenetics to estimate ancestral distributions. System. Biol. 70, 1033–1045. doi: 10.1093/sysbio/syab016
Guo X.-D., Wang H.-F., Bao L., Wang T.-M., Bai W.-N., Ye J.-W., et al. (2014). Evolutionary history of a widespread tree species Acer mono in East Asia. Ecol. Evol. 4, 4332–4345. doi: 10.1002/ece3.1278
Häkkinen H., Hodgson D., Early R. (2022). Plant naturalizations are constrained by temperature but released by precipitation. Global Ecol. Biogeogr. 31, 501–514. doi: 10.1111/geb.13443
Hardy N. B., Otto S. P. (2014). Specialization and generalization in the diversification of phytophagous insects: tests of the musical chairs and oscillation hypotheses. Proc. R. Soc. B: Biol. Sci. 281, 20132960. doi: 10.1098/rspb.2013.2960
Harmon L. J., Weir J. T., Brock C. D., Glor R. E., Challenger W. (2008). GEIGER: investigating evolutionary radiations. Bioinformatics 24, 129–131. doi: 10.1093/bioinformatics/btm538
Harnik P. G., Simpson C., Payne J. L. (2012). Long-term differences in extinction risk among the seven forms of rarity. Proc. R. Soc. B: Biol. Sci. 279, 4969–4976. doi: 10.1098/rspb.2012.1902
Hijmans R. J., Cameron S. E., Parra J. L., Jones P. G., Jarvis A. (2005). Very high resolution interpolated climate surfaces for global land areas. Int. J. Climatol. 25, 1965–1978. doi: 10.1002/joc.1276
Hijmans R. J., Phillips S., Leathwick J., Elith J. (2022) dismo, version 1.3-9. Available at: https://cran.r-project.org/web/packages/dismo (Accessed 21 May 2022).
Hoorn C., Wesselingh F. P., ter Steege H., Bermudez M. A., Mora A., Sevink J., et al. (2010). Amazonia through time: Andean uplift, climate change, landscape evolution, and biodiversity. Science 330, 927–931. doi: 10.1126/science.1194585
Hui Z., Ran M., Li H., Liu C., Guo B., Zhang J., et al. (2021). Early Pleistocene pollen record from the western Chinese Loess Plateau and its implications for the evolution of the East Asian Summer Monsoon. Sci. Total Environ. 761, 143304. doi: 10.1016/j.scitotenv.2020.143304
Kalkvik H. M., Stout I. J., Doonan T. J., Parkinson C. L. (2012). Investigating niche and lineage diversification in widely distributed taxa: phylogeography and ecological niche modeling of the Peromyscus maniculatus species group. Ecography 35, 54–64. doi: 10.1111/j.1600-0587.2011.06994.x
Kalyaanamoorthy S., Minh B. Q., Wong T. K. F., von Haeseler A., Jermiin L. S. (2017). ModelFinder: fast model selection for accurate phylogenetic estimates. Nat. Methods 14, 587–589. doi: 10.1038/nmeth.4285
Kay K. M., Whittall J. B., Hodges S. A. (2006). A survey of nuclear ribosomal internal transcribed spacer substitution rates across angiosperms: an approximate molecular clock with life history effects. BMC Evolution. Biol. 6, 36. doi: 10.1186/1471-2148-6-36
Keck F., Rimet F., Bouchez A., Franc A. (2016). phylosignal: an R package to measure, test, and explore the phylogenetic signal. Ecol. Evol. 6, 2774–2780. doi: 10.1002/ece3.2051
Lancaster L. T. (2022). On the macroecological significance of eco-evolutionary dynamics: the range shift–niche breadth hypothesis. Philos. Trans. R. Soc. B: Biol. Sci. 377, 20210013. doi: 10.1098/rstb.2021.0013
Lawson C. R., Bennie J., Hodgson J. A., Thomas C. D., Wilson R. J. (2014). Topographic microclimates drive microhabitat associations at the range margin of a butterfly. Ecography 37, 732–740. doi: 10.1111/ecog.00535
Lê S., Josse J., Husson F. (2008). FactoMineR: an R package for multivariate analysis. J. Stat. Softw. 25, 1–18. doi: 10.18637/jss.v025.i01
Li Y., Li X., Sandel B., Blank D., Liu Z., Liu X., et al. (2016). Climate and topography explain range sizes of terrestrial vertebrates. Nat. Climate Change 6, 498–502. doi: 10.1038/nclimate2895
Li P.-S., Thomas D. C., Saunders R. M. (2017). Historical biogeography and ecological niche modelling of the Asimina-Disepalum clade (Annonaceae): role of ecological differentiation in Neotropical-Asian disjunctions and diversification in Asia. BMC Evolution. Biol. 17, 188. doi: 10.1186/s12862-017-1038-4
Liu C., White M., Newell G. (2013). Selecting thresholds for the prediction of species occurrence with presence-only data. J. Biogeogr. 40, 778–789. doi: 10.1111/jbi.12058
Liu J., Zhang P., Lease R. O., Zheng D., Wan J., Wang W., et al. (2013). Eocene onset and late Miocene acceleration of Cenozoic intracontinental extension in the North Qinling range–Weihe graben: insights from apatite fission track thermochronology. Tectonophysics 584, 281–296. doi: 10.1016/j.tecto.2012.01.025
Lu W.-X., Hu X.-Y., Wang Z.-Z., Rao G.-Y. (2022). Hyb-Seq provides new insights into the phylogeny and evolution of the Chrysanthemum zawadskii species complex in China. Cladistics 38, 663–683. doi: 10.1111/cla.12514
Maguire K. C., Nieto-Lugilde D., Fitzpatrick M. C., Williams J. W., Blois J. L. (2015). Modeling species and community responses to past, present, and future episodes of climatic and ecological change. Annu. Rev. Ecol. Evol. System. 46, 343–368. doi: 10.1146/annurev-ecolsys-112414-054441
Meng Q.-R. (2017). Origin of the Qinling mountains (in Chinese). Sci. Sin. Terrae 47, 412–420. doi: 10.1360/N072016-00422
Moles A. T., Perkins S. E., Laffan S. W., Flores-Moreno H., Awasthy M., Tindall M. L., et al. (2014). Which is a better predictor of plant traits: temperature or precipitation? J. Vege. Sci. 25, 1167–1180. doi: 10.1111/jvs.12190
Moran N. A. (1988). The evolution of host-plant alternation in aphids: evidence for specialization as a dead end. Am. Nat. 132, 681–706. doi: 10.1086/284882
Morin X., Lechowicz M. J. (2013). Niche breadth and range area in North American trees. Ecography 36, 300–312. doi: 10.1111/j.1600-0587.2012.07340.x
Mumladze L., Asanidze Z., Walther F., Hausdorf B. (2017). Beyond elevation: testing the climatic variability hypothesis vs. Rapoport’s rule in vascular plant and snail species in the Caucasus. Biol. J. Linn. Soc. 121, 753–763. doi: 10.1093/biolinnean/blx027
Münkemüller T., Lavergne S., Bzeznik B., Dray S., Jombart T., Schiffers K., et al. (2012). How to measure and test phylogenetic signal. Methods Ecol. Evol. 3, 743–756. doi: 10.1111/j.2041-210X.2012.00196.x
Nie J., Song Y., King J. W., Zhang R., Fang X. (2013). Six million years of magnetic grain-size records reveal that temperature and precipitation were decoupled on the Chinese Loess Plateau during ~ 4.5–2.6 Ma. Quater. Res. 79, 465–470. doi: 10.1016/j.yqres.2013.01.002
Peng W., Nie J., Wang Z., Qiang X., Garzanti E., Pfaff K., et al. (2018). A major change in precipitation gradient on the Chinese Loess Plateau at the Pliocene-Quaternary boundary. J. Asian Earth Sci. 155, 134–138. doi: 10.1016/j.jseaes.2017.10.031
Pinheiro F., Dantas-Queiroz M. V., Palma-Silva C. (2018). Plant species complexes as models to understand speciation and evolution: a review of South American studies. Crit. Rev. Plant Sci. 37, 54–80. doi: 10.1080/07352689.2018.1471565
Pintor A. F., Schwarzkopf L., Krockenberger A. K. (2015). Rapoport’s Rule: do climatic variability gradients shape range extent? Ecol. Monogr. 85, 643—659. doi: 10.1890/14-1510.1
Quintero I., Wiens J. J. (2013). What determines the climatic niche width of species? The role of spatial and temporal climatic variation in three vertebrate clades. Global Ecol. Biogeogr. 22, 422–432. doi: 10.1111/geb.12001
Rafajlović M., Alexander J. M., Butlin R. K., Johannesson K. (2022). Introduction to the theme issue ‘Species’ ranges in the face of changing environments. Philos. Trans. R. Soc. B: Biol. Sci. 377, 20210002. doi: 10.1098/rstb.2021.0002
Rambaut A., Drummond A. J., Xie D., Baele G., Suchard M. A. (2018). Posterior summarization in Bayesian phylogenetics using Tracer 1.7. System. Biol. 67, 901–904. doi: 10.1093/sysbio/syy032
R Core Team. (2022). R: a language and environment for statistical computing. (Vienna, Austria: R Foundation for Statistical Computing). Available at: https://www.R-project.org/.
Reed R. N. (2003). Interspecific patterns of species richness, geographic range size, and body size among New World venomous snakes. Ecography 26, 107–117. doi: 10.1034/j.1600-0587.2003.03388.x
Revell L. J. (2012). phytools: an R package for phylogenetic comparative biology (and other things). Methods Ecol. Evol. 3, 217–223. doi: 10.1111/j.2041-210X.2011.00169.x
Rivera J. A., Lawing A. M., Martins E. P. (2020). Reconstructing historical shifts in suitable habitat of Sceloporus lineages using phylogenetic niche modelling. J. Biogeogr. 47, 2117–2128. doi: 10.1111/jbi.13915
Rödder D., Engler J. O. (2011). Quantitative metrics of overlaps in Grinnellian niches: advances and possible drawbacks. Global Ecol. Biogeogr. 20, 915–927. doi: 10.1111/j.1466-8238.2011.00659.x
Rombaut L. M. K., Capp E. J. R., Hughes E. C., Varley Z. K., Beckerman A. P., Cooper N., et al. (2022). The evolution of the traplining pollinator role in hummingbirds: specialization is not an evolutionary dead end. Proc. R. Soc. B: Biol. Sci. 289, 20212484. doi: 10.1098/rspb.2021.2484
Ruggiero A., Lawton J. H., Blackburn T. M. (1998). The geographic ranges of mammalian species in South America: spatial patterns in environmental resistance and anisotropy. J. Biogeogr. 25, 1093–1103. doi: 10.1046/j.1365-2699.1998.00253.x
Saupe E. E., Qiao H., Hendricks J. R., Portell R. W., Hunter S. J., Soberón J., et al. (2015). Niche breadth and geographic range size as determinants of species survival on geological time scales. Global Ecol. Biogeogr. 24, 1159—1169. doi: 10.1111/geb.12333
Schoener T. W. (1968). The Anolis lizards of Bimini: resource partitioning in a complex fauna. Ecology 49, 704–726. doi: 10.2307/1935534
Sexton J. P., Montiel J., Shay J. E., Stephens M. R., Slatyer R. A. (2017). Evolution of ecological niche breadth. Annu. Rev. Ecol. Evol. System. 48, 183–206. doi: 10.1146/annurev-ecolsys-110316-023003
Shen C.-Z., Zhang C.-J., Chen J., Guo Y.-P. (2021). Clarifying recent adaptive diversification of the Chrysanthemum-group on the basis of an updated multilocus phylogeny of subtribe Artemisiinae (Asteraceae: Anthemideae). Front. Plant Sci. 12, 648026. doi: 10.3389/fpls.2021.648026
Sheth S. N., Morueta-Holme N., Angert A. L. (2020). Determinants of geographic range size in plants. New Phytol. 226, 650–665. doi: 10.1111/nph.16406
Shi Z., Humphries C. J., Gilbert M. G. (2011). “Chrysanthemum Linnaeus,” in Flora of China Volume 20–21 (Asteraceae). Eds. Wu Z. Y., Raven P. H., Hong D. Y. (Beijing, China: Science Press & St. Louis, USA: Missouri Botanical Garden Press), 669–676.
Shi X., Yang Z., Dong Y., Qu H., Zhou B., Cheng B. (2020). Geomorphic indices and longitudinal profile of the Daba Shan, northeastern Sichuan Basin: evidence for the late Cenozoic eastward growth of the Tibetan Plateau. Geomorphology 353, 107031. doi: 10.1016/j.geomorph.2020.107031
Sillero N., Arenas-Castro S., Enriquez-Urzelai U., Vale C. G., Sousa-Guedes D., Martínez-Freiría F., et al. (2021). Want to model a species niche? A step-by-step guideline on correlative ecological niche modelling. Ecol. Model. 456, 109671. doi: 10.1016/j.ecolmodel.2021.109671
Slatyer R. A., Hirst M., Sexton J. P. (2013). Niche breadth predicts geographical range size: a general ecological pattern. Ecol. Lett. 16, 1104–1114. doi: 10.1111/ele.12140
Smith S. A., Donoghue M. J. (2010). Combining historical biogeography with niche modeling in the Caprifolium clade of Lonicera (Caprifoliaceae, Dipsacales). System. Biol. 59, 322–341. doi: 10.1093/sysbio/syq011
Stankowski S., Ravinet M. (2021). Defining the speciation continuum. Evolution 75, 1256–1273. doi: 10.1111/evo.14215
Stevens G. C. (1989). The latitudinal gradient in geographical range: how so many species coexist in the tropics. Am. Nat. 133, 240–256. doi: 10.1086/284913
Suchard M. A., Lemey P., Baele G., Ayres D. L., Drummond A. J., Rambaut A. (2018). Bayesian phylogenetic and phylodynamic data integration using BEAST 1.10. Virus Evol. 4, vey016. doi: 10.1093/ve/vey016
Thuiller W., Georges D., Engler R., Breiner F. (2022) biomod2: Ensemble platform for species distribution modeling. R package version 4.1-2. Available at: https://cran.r-project.org/web/packages/biomod2/index.html (Accessed October 2022).
Torres-Martínez L., Porter S. S., Wendlandt C., Purcell J., Ortiz-Barbosa G., Rothschild J., et al. (2021). Evolution of specialization in a plant-microbial mutualism is explained by the oscillation theory of speciation. Evolution 75, 1070–1086. doi: 10.1111/evo.14222
Valavi R., Guillera-Arroita G., Lahoz-Monfort J. J., Elith J. (2022). Predictive performance of presence-only species distribution models: a benchmark study with reproducible code. Ecol. Monogr. 92, e01486. doi: 10.1002/ecm.1486
Vamosi J. C., Armbruster W. S., Renner S. S. (2014). Evolutionary ecology of specialization: insights from phylogenetic analysis. Proc. R. Soc. B: Biol. Sci. 281, 20142004. doi: 10.1098/rspb.2014.2004
Vignali S., Barras A. G., Arlettaz R., Braunisch V. (2020). SDMtune: an R package to tune and evaluate species distribution models. Ecol. Evol. 10, 11488–11506. doi: 10.1002/ece3.6786
Waltari E., Hijmans R. J., Peterson A. T., Nyári Á.S., Perkins S. L., Guralnick R. P. (2007). Locating Pleistocene refugia: comparing phylogeographic and ecological niche model predictions. PloS One 2, e563. doi: 10.1371/journal.pone.0000563
Wang T., Wang G., Innes J. L., Seely B., Chen B. (2017). ClimateAP: An application for dynamic local downscaling of historical and future climate data in Asia Pacific. Front. Agric. Sci. Eng. 4, 448–458. doi: 10.15302/J-FASE-2017172
Warren D. L., Glor R. E., Turelli M. (2010). ENMTools: a toolbox for comparative studies of environmental niche models. Ecography 33, 607–611. doi: 10.1111/j.1600-0587.2009.06142.x
Warren D. L., Matzke N. J., Cardillo M., Baumgartner J. B., Beaumont L. J., Turelli M., et al. (2021). ENMTools 1.0: an R package for comparative ecological biogeography. Ecography 44, 504–511. doi: 10.1111/ecog.05485
Wellborn G. A., Langerhans R. B. (2015). Ecological opportunity and the adaptive diversification of lineages. Ecol. Evol. 5, 176–195. doi: 10.1002/ece3.1347
Wu F., Fang X., Ma Y., Herrmann M., Mosbrugger V., An Z., et al. (2007). Plio–Quaternary stepwise drying of Asia: evidence from a 3-Ma pollen record from the Chinese Loess Plateau. Earth Planet Sci. Lett. 257, 160–169. doi: 10.1016/j.epsl.2007.02.029
Yamamoto M., Ohtani M., Kurata K., Setoguchi H. (2017). Contrasting evolutionary processes during Quaternary climatic changes and historical orogenies: a case study of the Japanese endemic primroses Primula sect. Reinii. Ann. Bot. 120, 943–954. doi: 10.1093/aob/mcx108
Yesson C., Culham A. (2006). Phyloclimatic modeling: combining phylogenetics and bioclimatic modeling. System. Biol. 55, 785–802. doi: 10.1080/1063515060081570
Zhang Y., Yang N., Meng H. (2005). Deep-incised valleys along the Minjiang river upstream and their responses to the uplift of the West Sichuan Plateau, China (in Chinese). J. Chengdu Univ. Technol. (Science Technol. Edition) 32, 331–339. doi: 10.3969/j.issn.1671-9727.2005.04.001
Zhao C., Wang C.-B., Ma X.-G., Liang Q.-L., He X.-J. (2013). Phylogeographic analysis of a temperate-deciduous forest restricted plant (Bupleurum longiradiatum Turcz.) reveals two refuge areas in China with subsequent refugial isolation promoting speciation. Mol. Phylogenet. Evol. 68, 628–643. doi: 10.1016/j.ympev.2013.04.007
Keywords: niche evolution, range dynamics, spatiotemporal coupling, species complex, eco-evolutionary process, phyloclimatic modeling, ecological niche modeling
Citation: Lu W-X, Wang Z-Z, Hu X-Y and Rao G-Y (2023) Echoes of the past: niche evolution, range dynamics, and their coupling shape the distribution of species in the Chrysanthemum zawadskii species complex. Front. Ecol. Evol. 11:1250491. doi: 10.3389/fevo.2023.1250491
Received: 30 June 2023; Accepted: 19 October 2023;
Published: 31 October 2023.
Edited by:
Pedro Tarroso, Centro de Investigacao em Biodiversidade e Recursos Geneticos (CIBIO-InBIO), PortugalReviewed by:
Haibin Wang, Nanjing Agricultural University, ChinaWenpan Dong, Beijing Forestry University, China
Copyright © 2023 Lu, Wang, Hu and Rao. This is an open-access article distributed under the terms of the Creative Commons Attribution License (CC BY). The use, distribution or reproduction in other forums is permitted, provided the original author(s) and the copyright owner(s) are credited and that the original publication in this journal is cited, in accordance with accepted academic practice. No use, distribution or reproduction is permitted which does not comply with these terms.
*Correspondence: Guang-Yuan Rao, cmFvQHBrdS5lZHUuY24=