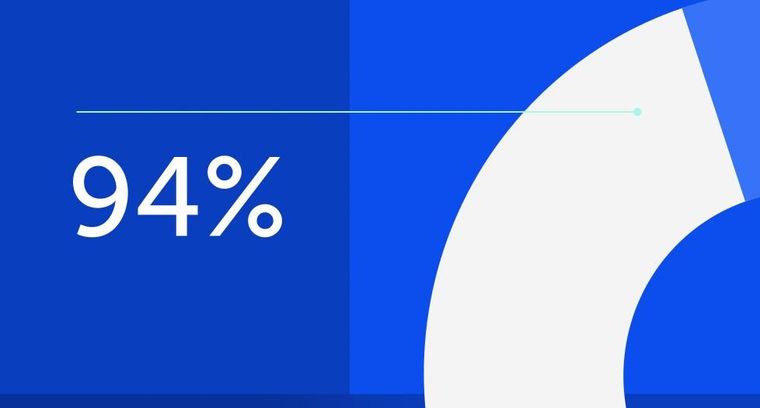
94% of researchers rate our articles as excellent or good
Learn more about the work of our research integrity team to safeguard the quality of each article we publish.
Find out more
ORIGINAL RESEARCH article
Front. Ecol. Evol., 10 January 2024
Sec. Urban Ecology
Volume 11 - 2023 | https://doi.org/10.3389/fevo.2023.1244418
Trees are a prominent feature of urban ecosystems. Urban tree productivity is a key component of urban ecosystem energetics and has been identified as a possible pathway for reducing global greenhouse gas concentrations. Recently, extensive research has been directed to evaluating the carbon dynamics of urban trees in cities throughout the world. Here, we synthesize this research, using results from previous studies from 154 cities to identify the distribution of urban tree productivity globally and the basis for generating urban tree productivity estimates. Reported urban tree productivity shows a strong relationship with estimated tree carbon content and exhibits increases with both temperature and precipitation, with land cover differences influencing the degree of climate sensitivity. Compared with a reference productivity estimate, urban trees showed greatly reduced estimated rates of productivity and the magnitude of reduced productivity was inversely correlated with precipitation but was independent of temperature. Reported rates of productivity across all studies suggest climate restrictions that are more important with less intensively managed land covers. Scaling these results globally suggests a limited opportunity for urban trees to contribute to atmospheric carbon dioxide reductions, especially in the absence of major carbon emission reductions. We found that the majority of results are derived from tree inventories from a single period with rates of productivity estimate through quasi-empirical or allometric models. The majority of studies have been conducted in temperate biomes and North America. These results show that existing urban tree assessments have substantial methodological restrictions and regional biases. Future research of urban tree productivity should look toward improved methods and can use this synthesis as a baseline for comparisons and improvement.
Productivity of vegetation is a key ecosystem rate that is foundational for ecosystem energetics and is closely linked with other biogeochemical cycles and community dynamics. In cities, the rates of vegetation productivity are increasingly of interest from the perspectives of improving theories of urban ecosystem dynamics and contributing to human well-being. Urban trees are an especially important and ubiquitous component of vegetation in cities globally (Ossola et al., 2020). Urban trees are valued for a broad suite of benefits from stormwater management (Berland, 2017), cooling (Ma et al., 2021), and contributions to improved human health (Wolf, 2020). As with growing interest in expanding forests generally as a tool for reducing atmospheric greenhouse gasses (Nave, 2018; Bastin, 2019), increasingly urban trees have been suggested as a potential wedge for reducing global GHG emissions (Nowak et al., 2013; Cook-Patton, 2020, and included in the National Academies of Sciences, Engineering, and Medicine, “Negative Emissions Technologies and Reliable Sequestration: A Research Agenda 2019). Consequently, the number of studies evaluating urban tree carbon storage and productivity has recently increased. How these data have been obtained is unclear, and a synthesis of the individual studies into a global analysis is lacking.
Assessing urban tree productivity is difficult (Nowak et al., 1996; McHale et al., 2017; Sonti et al., 2022; Zhuang et al., 2022). Even under optimal conditions in natural settings, assessing tree productivity is challenging because of the large size and slow growth rates of these organisms coupled with plasticity in many allometric relationships in response to environmental conditions and community interactions (Whittaker et al., 1974). In urban areas, assessing rates of productivity is further challenged by the novel environments (Teixeira and Fernandes, 2020), novel trait responses (Ibsen et al., 2023), diversity of species (Ossola et al., 2020), active management (Vogt et al., 2015), and restraints posed by complexities of land cover (Bigsby et al., 2014). Nevertheless, modeling tools have become available for assessing urban tree productivity, which couples urban tree surveys with algorithms that may include a range of process characterization and empirical relationships (McPherson et al., 2013; Nowak et al., 2013). However, these functions are not standardized and the methods for estimating urban tree productivity varies substantially (Sonti et al., 2022). The geographic distribution of urban tree assessments may also introduce uncertainties in characterizing global patterns of urban tree productivity. In general, urban ecological research has primarily been directed to the continents of North America and Europe and temperate biomes, leaving geographic biases in assessments (Ziter, 2016). Characterizing the basis of knowledge, both from methodological and geographic perspectives, is needed to better identify future needs for characterizing global urban tree productivity while assessing estimated distributions of reported productivity.
The recent proliferation of reported urban tree carbon content and productivity provides an opportunity for an initial assessment of global controls. As with natural forests, urban tree distributions are affected by climate (Jenerette et al., 2016; Hanley et al., 2021) and climatic differences may similarly influence rates of productivity. Temperature and precipitation are frequently key climate drivers of productivity in forests globally (Grosso, 2008). However, their role for urban tree productivity may be moderated by irrigation (Bijoor et al., 2012; Ibsen et al., 2023), which could reduce tree dependence on precipitation inputs, and urban warming (Oke, 1982; Manoli et al., 2019) that could reduce effects of cold temperatures on productivity. Along with climate, land cover within a city may have an important role on productivity (Buyantuyev and Wu, 2009). Urban land covers span a range of environments, including remnant minimally managed forest patches within a city, managed parks, and within the urban fabric itself with close interactions with built structures (Avolio et al., 2021). These latter two land covers may undergo some of the most extreme management in terms of resource inputs and pruning. The effects of climate and land cover may have a direct effect on the absolute rates of productivity and may also influence the deviation of productivity from expected reference rates.
Here, we use a data synthesis approach to generate a global perspective of current estimates of urban tree productivity. We evaluate the sources, both methodological and geographical, to assess the basis of current knowledge. We test a hypothesis of urban tree productivity climate regulation and predict that productivity rates will increase with temperature and precipitation. We test a land cover hypothesis predicting that more intensively managed land covers will have reduced climate sensitivities. We further compare rates of estimated urban tree productivity to a simple reference model of productivity and test a prediction that estimated urban tree productivity will be lower than reference estimates and this difference will be similarly affected by land cover and climate. We use the resulting estimates to evaluate the global potential for urban trees to serve as a climate change mitigation wedge. This synthesis provides a benchmark for estimating global distributions of urban tree productivity and potential contributions to reducing greenhouse gas concentrations.
A literature review was conducted using Web of Science. We obtained an initial list of articles from the search criteria [urban carbon (sequestration or pool*) (plant or vegetation or tree)]. We included all articles from the beginning of the data record to July 2021 (Supplementary Table 1). In the data synthesis, we reported only results for urban tree carbon storage or sequestration per area of land. Our full data collection, though, also included some papers that only reported carbon storage or sequestration per tree for an area of urban landscape. A total of 128 papers reported urban tree carbon data for at least one land use category for at least one measure of tree carbon (storage or sequestration per land area), with 112 for the entire city, 27 for urban forests, 129 for urban land covers, and 46 for parks. In total, the synthesis includes 277 estimates of urban tree productivity as some studies reported values from multiple cities or for multiple components of the urban landscape of a single city.
Our data synthesis only includes those estimates of tree carbon storage or sequestration that are representative of a whole portion of an urban landscape (i.e., all the trees in a defined urban extent). Storage is the standing biomass carbon amount. All data were transformed to Mg C per hectare per year. To convert aboveground only to total biomass C, we divided aboveground by 0.78 to get total C based on a previous estimate that belowground biomass was 22% of total tree biomass (Nowak, 1994). This conversion of aboveground to total was necessary for 78 of the total 128 publications from which we obtained data. Estimated sequestration (also referred to as NPP, net primary productivity) is the annual change in tree carbon storage. Carbon values are for estimated total tree biomass, i.e., aboveground and belowground carbon storage and sequestration.
Storage and sequestration values were reported as a per area basis either for the full extent of a city’s area or for a component of the city, which we organized into three constituent classes—urban, park, and forest—based on their substantive representation in the literature and as meaningful compartmentalization of urban landscapes from a tree quantification perspective. Urban is a mix of land uses that includes residential landscapes, commercial areas, and streetscapes among other common urban land uses. Park is the green or open spaces in the city that are managed for recreation and leisure, typically dominated by vegetative land covers (i.e., lawns and trees). Forest is the more naturalistic, undeveloped, or less managed settings that are similar to traditional forests where climate supports forest growth or as remnant nature patches similar to grassland, savanna, or desert biomes depending on climate context of the city.
A reference net primary productivity (NPP) was estimated for each city where reports of urban carbon were obtained. We used the NCEAS model in Del Grosso et al. (2008), which provides a baseline NPP from a combination of long-term mean temperature and precipitation. Based on the city location, climate data for deriving 30-year (1991–2020) mean annual temperature (MAT) and mean annual precipitation (MAP) for reference NPP modeling was acquired from CRU TS v 4.05 (Harris et al., 2020).
All analyses were performed in R (v. 4.0.1) using base packages plus ‘dplyr’ for data manipulation and ‘ggplot2’ for data visualization. Carbon values for urban trees were log 10 transformed to meet assumptions of analyses and to support visualizations of these log-normal distributed data.
Reports of urban tree carbon distributions have increased dramatically in the past 40 years and now include much of the earth (Figure 1). The earliest study identified was from 1984 (Dorney et al., 1984) reporting tree coverage, density, storage, and sequestration values for a suburban neighborhood of Milwaukee, Wisconsin, USA, and relied on allometric equations from the Hubbard Brook ecosystem study of a forest in New Hampshire, USA. Papers reporting urban tree carbon values remained rare for more than two decades following this initial publication before becoming frequent starting in 2010 and continuing to the present (Figure 1). Geographically, urban tree estimates were primarily from temperate biomes (47%) and the North American continent (57%). Tropical and arid biomes, which include extensive urban centers together, were only 23% of estimates combined. Europe and Asia were included in a second large group of studies (31%), whereas Africa, South America, and Oceania in total were represented in 12% of studies.
Figure 1 Distribution of urban tree carbon inventories. Samples are reported as (A) maps, (B) timeline, (C) continental summary, and (D) biome summary.
The methodology for estimating urban trees varied extensively with more than 30 different approaches reported (Figure 2). The methods overall led to values that did not include uncertainty estimates but instead generated a single estimated value reflecting tree community distribution and life stages at the time when the inventory was collected. In general, the most common approach was based on a single survey of urban tree species composition and biometric measurements of DBH, canopy width, and canopy height. While a large number of different models were used, the suite of iTree software packages or their precursor UFORE (hereafter, iTree Universe) were the most common bases of deriving carbon estimates from tree surveys for the data reported in this meta-analysis. Out of 128 papers included in this meta-analysis, 29 (23%) used products from the iTree Universe either exclusively or partially. Furthermore, papers using iTree Universe contributed more reporting of data for multiple cities or multiple components of a city resulting in an even greater representation in the data. There was not, however, statistical evidence that data from papers using iTree Universe products were distinct from data derived from other bases (SI text). Figures in this manuscript using contrasting data point shapes for iTree Universe versus not iTree Universe were retained as visual evidence of this finding. The second most common basis was Custom which was a class assigned to papers that compiled equations from a custom mix of sources or uniquely applied another method. Overall, many sources of methods for size scaling and growth equations were cited as the main reference once or a few times. We identified only four papers that quantified tree growth through measurements of tree size at multiple time points with each paper contributing one carbon sequestration estimation. Tree rings were used to estimate growth of Park trees in Ningbo, China as 2.1 Mg C ha−1 year−1 (Guo, 2013). Urban trees in Auckland, New Zealand, were estimated at 1.7 Mg C ha−1 year−1 based off their known planting dates (Schwendenmann and Mitchell, 2014). Finally, repeat measures of tree diameters for urban trees in Orlando, FL (Horn et al., 2015), and Boston, MA (Smith et al., 2019), USA, estimate carbon sequestration at 1.5 Mg C ha−1 year−1 and −0.2 Mg C ha−1 year−1, respectively. The Boston finding was the only negative sequestration estimate found; in this case, mortality-driven carbon losses exceeded new growth in street trees. We have excluded this study from subsequent statistical analyses but discuss the importance of this observation.
Figure 2 A word cloud of the models or primary cited source of equations used for generating carbon estimates from tree measures as reported by papers in this meta-analysis. Font size is linearly scaled by number of papers with the smallest size representing 1 and the largest size represented as iTree Universe 29. Text placement is random.
Across studies, a strong relationship was observed between reported magnitudes of productivity and estimated carbon storage (Figure 3). Reported values of carbon sequestration by urban trees spanned much of the range in NPP found globally in natural systems. Reported rates of urban productivity vary dramatically with two orders of magnitude in values for carbon sequestration by urban trees across global climate gradients and all urban land classes (<0.1 Mg C ha−1 year−1 to almost 10 Mg C ha−1 year−1). Despite this range, sequestration across cities and land classes was best modeled by storage alone. Specifically, there was a positive log–log linear relationship of carbon sequestered to carbon stored. For the median value of storage of 25.7 Mg C ha−1, the modeled carbon sequestration rate was 1.1 Mg C ha−1 year−1, a 4.3% growth rate. The log–log linear relationship, though, translates as a decline in sequestration rates as a percentage of carbon storage with increasing carbon storage densities. Here, the lowest values of carbon storage have annual growth rates around 6% declining to 3% at the high end of reported carbon storage levels. Because carbon sequestration values were only provided for a subset of dataset (39%), we derived net primary productivity (NPP), or effective carbon sequestration, rates for urban trees for the full data set based on the modeled relationship between storage and sequestration reported here.
Figure 3 The global relationship between carbon storage by urban trees and their sequestration rates. Points are all paired estimates in the data synthesis. Axes are log 10 transformed.
Estimated urban tree productivity was influenced by both precipitation and temperature (Figure 4). Increasing temperature as associated with higher rates of productivity. Similarly increasing precipitation was associated with higher rates of productivity. Nevertheless, the amount of variation in productivity associated with urban climate was low individually (adj R2 = 0.08 and 0.06 for temperature and precipitation, respectively) and when modeled together in a multiple regression (r2 = 0.12).
Figure 4 Estimated urban tree net primary production in relation to (A) mean annual temperature and (B) mean annual precipitation.
Within cities, land cover was also an important factor in estimated urban tree productivity (Figure 5). Reported rates of productivity different among land covers (p < 0.001 ANOVA) with the highest rates of 3.0 Mg C ha−1 in forest and lowest rates of 1.1 Mg C ha−1 in urban (ANOVA). Across different urban land covers, the role of climate differed substantially. Whole cities and urban forests were closely related to both temperature and precipitation. However, these climate variables were less correlated with the variation in urban and park land covers.
Figure 5 Relationship between estimated net primary production and land cover. Productivity for (A) forests, (B) urban ecosystems, and (C) parks in relation to mean annual precipitation and similarly (D–F) for mean annual temperature.
In comparison with modeled reference productivity, urban productivity was lower and the magnitude of decrease varied with climate (Figure 6). Overall, reported urban tree NPP was 72% lower than modeled reference NPP. In only 4% of cases, urban NPP was higher than the modeled reference NPP. The difference between reference NPP and reported NPP was associated with climate of the city (Figure 6). Increasing precipitation was associated with a greater decrease in urban NPP compared with reference NPP (p < 0.001). Urban temperature was not a significant predictor of the reported urban NPP and reference NPP (p = 1.0). Nevertheless, the magnitude of NPP difference associated with temperature exhibited heteroskedasticity, with the variation in delta NPP increasing with temperature (p < 0.001).
Figure 6 Difference between reference and estimated urban tree productivity in relation to (A) mean annual precipitation and (B) mean annual temperature.
Land cover was also associated with variation in delta NPP (Figure 7). Urban tree NPP and reference NPP were most closely related in urban forests, whereas these NPP estimates were effectively decoupled for urban and park land covers. Urban tree NPP was positively correlated with reference NPP, but increases in urban tree NPP were less than the increases in reference NPP. Furthermore, the relationship between urban trees NPP and reference NPP differed across urban land classes (model: Urban Trees NPP ~ Reference NPP*Land Class, p < 0.001, adj. R2 = 0.24, F-statistic = 13.37, 269). For the whole city, urban tree NPP gained 0.12 Mg C per Mg C in reference NPP (Figure 2). The constituent components of cities, urban, parks, and forest land covers gained at 0.05 Mg C, 0.01 Mg C, and 0.73 Mg C per Mg C increase in the reference, respectively (Figure 2). Urban land uses, the more developed land uses, respond modestly with large differences developing between urban and reference NPP at high reference NPP levels starting from no difference at the lowest levels of reference NPP. Parks are striking in that urban trees NPP for this class were greatest among all classes at low levels of reference NPP (i.e., greatest intercept value); however, Parks’ urban tree NPP was effectively constant in comparison with the reference NPP.
Figure 7 Comparison between estimated net primary productivity and reference productivity for different land uses of (A) forests, (B) parks, and (C) urban ecosystems.
Urban tree productivity rates have been reported extensively beginning in 1984, and the number of reports is increasing rapidly. Urban tree productivity has been estimated in diverse cities throughout the world using a wide array of approaches, although primarily dependent on surveys conducted at a single point in time. As a whole, the reported rates of urban tree carbon productivity were closely linked to estimates of tree carbon storage. Reported urban tree productivity increased with precipitation and temperature, whereas within-city land cover distributions have further effects on productivity magnitudes and sensitivity to climate. Nevertheless, reported urban tree productivity was substantially less than a modeled reference productivity and the degree of reduction increased with precipitation and was sensitive to land cover. These results are consistent with findings of reduced urban primary productivity throughout the United States compared with displaced lands (Imhoff et al., 2004). However, current estimates likely have substantial uncertainties in representing global distributions of urban tree productivity, both through methodological challenges and geographical bias, but these results serve as an extensive baseline for future comparisons. This study—as derived from hundreds of reported estimates of tree carbon storage and productivity—represents a first of its kind estimate of sequestration by urban trees across the climate gradients represented in the global extent of urbanization and critical evaluation of the approaches for this body of research.
Across all reported urban tree productivity rates, a consistent relationship was observed between carbon content and productivity estimates. This relationship in the reported results was best described as a saturating function, implying that as urban trees grew, their reported capacity for productivity decreased. From the resulting estimates of tree productivity, the range of sequestration rates found across cities and their constituent land cover classes was much narrower than what would be expected for natural systems. This finding suggests a degree of convergence in urban tree functioning. While urban tree community composition does not exhibit convergence (Jenerette et al., 2016), other components of urban ecosystems do, including climate (Hall et al., 2016), land management (Polsky et al., 2014), and soils (Trammell et al., 2020). Convergence in urban tree carbon sequestration is consistent with a previous finding of convergent urban vegetation functional distributions across climate gradients through changes in species selection (Ibsen et al., 2020).
Climate and land cover are important contributors to variability in the estimates of urban tree carbon productivity. Long-term temperature and precipitation distributions were only weakly related to reported overall tree productivity. This finding is consistent with urbanization ameliorating environmental constraints through management and species selection (Ibsen et al., 2020). In comparison with a reference productivity rate, though, these climate variables had a more important role. Increases in precipitation were associated with a greater reduction in reported productivity compared with the reference, with more arid cities having little divergence or even in some cases an increase in productivity and the wettest cities having the largest depression of productivity. This finding is consistent with the general finding of urban convergence (Groffman et al., 2014), where reductions in productivity are greatest in locations where reference productivity is highest. In contrast to the precipitation effect, temperature was unrelated to differences between the productivity of reported urban and reference trees. However, the variability of deviation among cities was greater in warmer climates. This heteroskedasticity likely reflects an interaction between temperature and moisture effects. At low temperatures, the deviation in productivity is reduced through temperature limitation; however, as temperatures increase, the role of precipitation similarly increases in determining the relative change in productivity, reflected in hot arid and hot humid locations.
Land cover distributions within cities also led to variation in reported tree productivity. The reported rate of productivity was highest in remnant forest areas than either parks or urban areas within the city. This likely reflects the spatial constraints within more heavily managed city landscapes that reduce tree productivity. While urbanization can lead to increases in productivity for individual trees (Briber et al., 2015), the reduced biomass in urban land covers is generally associated with reduced rates of ecosystem productivity within cities (Buyantuyev and Wu, 2009; McHale et al., 2017). The land cover differences are also evident in tree productivity climate sensitivities. Urban forest productivity was most strongly correlated with precipitation whereas urban and park tree productivity was more strongly correlated with temperature. These findings imply that remnant urban forests may be more reliant on rainfall for meeting water needs whereas irrigation supports productivity more in urban and park environments. The close relationship between remnant forests and reference productivity and divergence between reference productivity with parks and urban land covers likely reflects the role of increased management in these land covers. Parks—the ultimate designed vegetation systems in urban landscapes—were nearly universally alike, consistent with comparative studies of park species distributions (Ibsen et al., 2020) and entirely decoupled from climate drivers with regard to carbon sequestration by urban trees. Together, the effects of climate and land cover influenced reported rates of urban tree productivity and reflect the combination of environmental and management controls.
The large number of reported rates of urban tree productivity supports an evaluation of potential contributions of urban forests for reductions in global greenhouse gas concentrations. Without question, the growth of an urban tree is an immediate sequestration of carbon dioxide into biomass. What remains a question is the potential impact of urban trees to the global atmospheric pool of carbon dioxide (Pataki, 2021). The strong evidence for convergence between reported productivity and carbon storage allows values central in the distribution function as an estimate for the global average. A central estimate of a global carbon sequestration by urban trees, then, is 1.1 Mg C ha−1 year−1 as the modeled-derived carbon sequestration value for the median carbon storage value of 25.7 Mg C ha−1. Similarly, the mean value for studies reporting direct and repeated measures of tree size was 1.3 Mg C ha−1 year−1. Bracketing this range, we can estimate the potential role of urban tree growth to the atmosphere by considering the contributions of urban trees to global carbon cycle for three possible levels of carbon sequestration rates, 0.4, 0.8, and 1.2 Mg C ha−1 year−1. Based on Seto et al.’s (2012) low-to-moderate estimate of urban land extent in 2030 of 1.17 million sq. km, conservative, reliable, and plausible scenarios for total carbon sequestration by urban trees would be 0.05, 0.09, and 0.14 Pg C year−1, respectively, by the end of the decade. Global carbon emissions were an estimated 9.7 Pg C in 2020 driving average atmospheric CO2 concentrations to a new high of 412.5 ppm, up from 409.9 ppm in 2019 representing the fifth largest year-over-year increase since direct measure records began in 1960 (NOAA Climate.gov). These numbers dwarf the potential of urban trees to mitigate carbon emissions. These rates also do not include demographic dynamics associated with tree mortality or planting, which can have important carbon consequences (Smith et al., 2019). These rates are further suppressed by the wide range of life cycle contributions associated with urban trees, from planting, management, and removal (Strohbach et al., 2012; Nicese et al., 2021). These results suggest growing an urban forest as a climate mitigation strategy will have limited contributions to atmospheric CO2 reductions at contemporary emission rates.
While the estimates of urban tree productivity reflect a large number of studies, the basis of knowledge is limited. In a typical sampling protocol, plots are randomly located within a study domain, often using a stratified design based on land use distributions. A field team will visit each plot where all trees are characterized within a prescribed radius of the plot centroid. Data collected include species identification and some biometric variables such as DBH, canopy area, and height. In one common sampling approach (iTree), the individual ground plots are small (0.04 ha) and these plots generally included from zero to three species. The resulting field data are then incorporated into a diverse range of modeling frameworks to estimate carbon content and productivity on an area basis.
A critical limitation is the lack of uncertainty assessments. Uncertainty is introduced in both the characterization of the urban tree community and life stage distribution as well as the algorithms used to transform the tree inventories into estimates of carbon storage and growth. The approaches used in general do not follow recently developed guidelines for such measurements (Sonti et al., 2022). The most common approach used one-time surveys of tree community composition and biometric assessments such as diameter at breast height (DBH), tree height, and canopy width. The scaling of size measures to tree carbon stored in standing tree biomass is often based off of allometric models built from trees measured outside urban contexts, outside the region for which they are being applied, or for comparable but nonetheless different species. Model extrapolations include a combination of empirical and process-based algorithms that in general are derived from reference forests. The resulting carbon content and dynamics are inferred through allometric equations that have not been calibrated or validated within the study region. While the iTree family of sources is the most widely used approach for estimating carbon content and productivity (23% of all papers, SI text) and continues to be used (Prigioniero et al., 2022), many other schemes are also used. Therefore, to the extent iTree or a set of equations core to many models systematically misrepresents reality, our knowledge of urban trees becomes systematically biased. Of the studies included here, only four included repeated surveys of tree distributions but even these studies required applications of allometric models. Highlighting the challenges of most approaches is results from one study that used repeated measurements and because of tree mortality and removal observed net losses in urban carbon content (Smith et al., 2019). Thus, the majority of urban carbon content and productivity estimates should be viewed primarily as a physiological estimate. Before confidence can be attributed to existing estimates of urban tree productivity, characterization and attribution of uncertainty should be conducted.
Similar to methodological challenges, the collection of local surveys represented here bias representation of cities toward a few countries and in particular away from tropical and arid climate cities. This geographical bias in urban ecological research is a widespread phenomenon (Nagendra et al., 2018; Kendal et al., 2020). Since urbanization is most rapidly expanding in these climates in the coming decades, we need to better understand urban trees in these climates to better predict the trajectory of urban forests globally. Furthermore, unknown selection bias in which cities or components within cities were selected for study could further bias the findings here, especially in tropical climates where representation is limited and the potential range in carbon cycling by urban trees the greatest given their climatic potential. Cities from these regions are known to have disproportionately low research but include some of the most rapidly growing urban centers (Ziter, 2016; Nagendra et al., 2018). Extending research to urban trees and ecosystems more generally in these regions is warranted.
To advance theories of urban ecosystems and develop tools to allow cities to manage rates of tree productivity, an improved characterization of urban tree productivity is needed. Given the reliance on single surveys of species and limited biometric data, unclear allometric equations, and unvalidated models, the majority of current urban tree productivity estimates from the literature provide a more of an informed prediction of urban tree productivity. To generate more appropriate data on urban tree productivity, several directions of improvement are warranted to achieve results that are based on emerging protocols for urban ecosystems (Sonti et al., 2022) and are consistent with more general ecosystem approaches. Improved approaches are needed to evaluate urban tree carbon stocks. Improved inventories that include estimates of uncertainty both in species distributions and in current life stages of trees are needed as this information is a key component of urban tree carbon assessments. Regionally specific allometric equations should be developed (McHale et al., 2009) and should include species-specific growth rates and their response to management. LIDAR-based approaches are likely to provide additional data sources for evaluating urban tree carbon stocks and rates of productivity (Raciti et al., 2014; Ucar et al., 2018; Zhuang et al., 2022). Extending aboveground measurements to include belowground estimates is another major challenge. Quantifying urban tree root-to-shoot ratios are a key priority that introduces substantial uncertainty into current estimates of urban tree carbon content. In general, urban tree root distributions are poorly characterized (Cermak et al., 2000; Vico et al., 2014; Giambastiani et al., 2022). In quantifying the rates of productivity, estimates should be grounded on repeated measurements of carbon content. Models may serve as a supplement to repeated measurements, but models should be validated within the study locations. A close examination of the models and their equations is required to identify cases where poor model fit to reality could substantively bias our understanding. Increasingly, research is directed to more rigorous estimates of urban plant productivity and our results support these efforts (Smith et al., 2019; Sonti et al., 2022; Zhuang et al., 2022).
In addition to methodological uncertainties, the collection of local surveys represented here bias representation of cities toward a few countries and in particular away from tropical and arid climate cities. Since urbanization will be most rapidly expanding in these climates in the coming decades, we need to better understand urban trees in these climates to better predict the trajectory of urban forests globally. Furthermore, unknown selection bias in which cities or components within cities were selected could be influencing the findings here, especially in tropical climates where representation is limited and the potential range in carbon cycling by urban trees the greatest given their climatic potential. Growing a research focus on understanding urban trees and ecosystems that achieves a global perspective with more quantifiable assessments of uncertainty and transparency of methodology is a pressing research goal.
To theoretically advance urban ecosystem science and to improve management of cities to provide benefits with limited costs, much interest recently has been directed to assessing urban tree vegetation. At present, the basis of knowledge from both methodological and geographical perspectives is limited and the existing estimates serve as a benchmark for future assessments. Nevertheless, urban nature-based solution policies in the United States and Europe are being implemented that in part depend on expectations of carbon sequestration. Ensuring an unbiased and transparent accounting of urban tree productivity with well-characterized uncertainties is needed to evaluate the effectiveness of urban trees as a nature-based solution to rising greenhouse gas concentrations. Approaches that rely on resampling are essential to reducing uncertainties and new technologies; notably, the proliferation of LIDAR data is poised to rapidly advance urban tree productivity estimates. Geographically, the biases in urban tree productivity estimates are consistent with sampling biases writ-large in urban ecology. Nevertheless, the current literature supports hypotheses of a relationship between tree carbon content and productivity. Furthermore, these results show that reported tree productivity, both in absolute terms and in comparison with modeled reference rates, is affected by both climate and land cover distributions. This synthesis of the current state of the science provides a benchmark for ongoing and future efforts to characterize urban vegetation productivity and assess controls on this key ecosystem process at global scales.
The original contributions presented in the study are included in the article/Supplementary Material. Further inquiries can be directed to the corresponding author.
GJ and DH conceived the project. DH compiled the data and conducted initial analyses. GJ and DH finalized analyses. DH wrote the first draft and GJ and DH finalized the manuscript. All authors contributed to the article and approved the submitted version.
This research was supported by NSF CNH-2: 1924288.
We thank Stuart Schwab for assistance with reviewing articles used in this data synthesis. Diane Pataki and Meghan Avolio provided valuable feedback on an early draft of the manuscript.
The authors declare that the research was conducted in the absence of any commercial or financial relationships that could be construed as a potential conflict of interest.
All claims expressed in this article are solely those of the authors and do not necessarily represent those of their affiliated organizations, or those of the publisher, the editors and the reviewers. Any product that may be evaluated in this article, or claim that may be made by its manufacturer, is not guaranteed or endorsed by the publisher.
The Supplementary Material for this article can be found online at: https://www.frontiersin.org/articles/10.3389/fevo.2023.1244418/full#supplementary-material
Avolio M., Swan C., Pataki D., Jenerette G. (2021). Incorporating human behaviors into theories of urban community assembly and species coexistence. Oikos 130, 1849–1864. doi: 10.1111/oik.08400
Bastin J.-F. (2019). The global tree restoration potential. Science 365, 76–79. doi: 10.1126/science.aax0848
Berland A. (2017). The role of trees in urban stormwater management. Landscape Urban Plann. 162, 167–177. doi: 10.1016/j.landurbplan.2017.02.017
Bigsby K. M., McHale M. R., Hess G. R. (2014). Urban morphology drives the homogenization of tree cover in Baltimore, MD, and Raleigh, NC. Ecosystems 17, 212–227. doi: 10.1007/s10021-013-9718-4
Bijoor N. S., McCarthy H. R., Zhang D., Pataki D. E. (2012). Water sources of urban trees in the Los Angeles metropolitan area. Urban Ecosyst. 15, 195–214. doi: 10.1007/s11252-011-0196-1
Briber B., Hutyra L., Reinmann A., Raciti S., Dearborn V., Holden C., et al. (2015). Tree productivity enhanced with conversion from forest to urban land covers. PloS One 10. doi: 10.1371/journal.pone.0136237
Buyantuyev A., Wu J. (2009). Urbanization alters spatiotemporal patterns of ecosystem primary production: A case study of the Phoenix metropolitan region, USA. J. Arid Environments 73, 512–520. doi: 10.1016/j.jaridenv.2008.12.015
Cermak J., Hruska J., Martinkova M., Prax A. (2000). Urban tree root systems and their survival near houses analyzed using ground penetrating radar and sap flow techniques. Plant Soil 219, 103–116. doi: 10.1023/A:1004736310417
Cook-Patton S. C. (2020). Lower cost and more feasible options to restore forest cover in the contiguous United States for climate mitigation. One Earth 3, 739–752. doi: 10.1016/j.oneear.2020.11.013
Del Grosso S., Parton W., Stohlgren T., Zheng D., Bachelet D., Prince S., et al. (2008). Global potential net primary production predicted from vegetation class, precipitation, and temperature. Ecology 89, 2117–26.
Dorney J. R., Guntenspergen G. R., Keough J. R., Stearns F. (1984). Composition and structure of an urban woody plant community. Urban Ecol. 8, 69–90. doi: 10.1016/0304-4009(84)90007-X
Giambastiani Y., Errico A., Preti F., Guastini E., Censini G. (2022). Indirect root distribution characterization using electrical resistivity tomography in different soil conditions. Urban Forestry Urban Greening 67. doi: 10.1016/j.ufug.2021.127442
Groffman P., Cavender-Bares J., Bettez N., Grove J., Hall S., Heffernan J., et al. (2014). Ecological homogenization of urban USA. Front. Ecol. Environ. 12, 74–81. doi: 10.1890/120374
Grosso S. D. (2008). Global potential net primary production predicted from vegetation class, precipitation, and temperature. Ecology 89, 2117–2126. doi: 10.1890/07-0850.1
Guo P. (2013). Biogenic volatile organic compound emissions in relation to plant carbon fixation in a subtropical urban–rural complex. Landscape Urban Plann 119, 74–84.
Hall S. J., Learned J., Ruddell B., Larson K. L., Cavender-Bares J., Bettez N., et al. (2016). Convergence of microclimate in residential landscapes across diverse cities in the United States. Landscape Ecol. 31, 101–117. doi: 10.1007/s10980-015-0297-y
Hanley P. A., Arndt S. K., Livesley S. J., Szota C. (2021). Relating the climate envelopes of urban tree species to their drought and thermal tolerance. Sci Total Environment 753, 142012.
Horn J., Escobedo F. J., Hinkle R., Hostetler M., Timilsina N. (2015). The role of composition, invasives, and maintenance emissions on urban forest carbon stocks. Environment Manage 55, 431–42.
Harris I., Osborn T. J., Jones P., Lister D. (2020). Version 4 of the CRU TS monthly high-resolution gridded multivariate climate dataset. Scientific Data 7, 109.
Ibsen P. C., Borowy D., Rochford M., Swan C. M., Jenerette G. D. (2020). Influence of climate and management on patterns of taxonomic and functional diversity of recreational park vegetation. Front. Ecol. Evol. 8. doi: 10.3389/fevo.2020.501502
Ibsen P. C. C., Santiago L. S. S., Shiflett S. A. A., Chandler M., Jenerette G. D. (2023). Irrigated urban trees exhibit greater functional trait plasticity compared with natural stands. Biol. Lett. 19. doi: 10.1098/rsbl.2022.0448
Imhoff M., Bounoua L., DeFries R., Lawrence W., Stutzer D., Tucker C., et al. (2004). The consequences of urban land transformation on net primary productivity in the United States. Remote Sens. Environ. 89, 434–443. doi: 10.1016/j.rse.2003.10.015
Jenerette G. D., Clarke L. W., Avolio M. L., Pataki D. E., Gillespie T. W., Pincetl S., et al. (2016). Climate tolerances and trait choices shape continental patterns of urban tree biodiversity. Global Ecol. Biogeogr. 25, 1367–76.
Kendal D., Egerer M., Byrne J., Jones P., Marsh P., Threlfall C., et al. (2020). City-size bias in knowledge on the effects of urban nature on people and biodiversity. Environ. Res. Lett. 15. doi: 10.1088/1748-9326/abc5e4
Ma B., Hauer R. J., Ostberg J., Koeser A. K., Wei H., Xu C. (2021). A global basis of urban tree inventories: What comes first the inventory or the program. Urban Forestry Urban Greening 60. doi: 10.1016/j.ufug.2021.127087
Manoli G., Fatichi S., Schlapfer M., Yu K., Crowther T. W., Meili N., et al. (2019). Magnitude of urban heat islands largely explained by climate and population. Nature 573, 55–60. doi: 10.1038/s41586-019-1512-9
McHale M. R., Burke I. C., Lefsky M. A., Peper P. J., McPherson E. G. (2009). Urban forest biomass estimates: is it important to use allometric relationships developed specifically for urban trees? Urban Ecosyst. 12, 95–113. doi: 10.1007/s11252-009-0081-3
McHale M. R., Hall S. J., Majumdar A., Grimm N. B. (2017). Carbon lost and carbon gained: a study of vegetation and carbon trade-offs among diverse land uses in Phoenix, Arizona. Ecol. Appl. 27, 644–661. doi: 10.1002/eap.1472
McPherson E. G., Xiao Q., Aguaron E. (2013). A new approach to quantify and map carbon stored, sequestered and emissions avoided by urban forests. Landscape Urban Plann. 120, 70–84. doi: 10.1016/j.landurbplan.2013.08.005
Nagendra H., Bai X., Brondizio E., Lwasa S. (2018). The urban south and the predicament of global sustainability. Nat. Sustainability 1, 341–349. doi: 10.1038/s41893-018-0101-5
National Academies of Sciences, Engineering, and Medicine (2019). Negative emissions technologies and reliable sequestration: A research agenda (Washington D. C.: The National Academies Press).
Nave L. E. (2018). Reforestation can sequester two petagrams of carbon in US topsoils in a century. Proc. Natl. Acad. Sci. 115, 2776–2781. doi: 10.1073/pnas.1719685115
Nicese F., Colangelo G., Comolli R., Azzini L., Lucchetti S., Marziliano P., et al. (2021). Estimating CO2 balance through the Life Cycle Assessment prism: A case - Study in an urban park. Urban Forestry Urban Greening 57. doi: 10.1016/j.ufug.2020.126869
Nowak D. (1994). Atmospheric carbon dioxide reduction by Chicago’s urban forest. Pages 83–94 Chicago’s urban forest ecosystem: Results of the Chicago UrbanForest Climate Project. Eds. McPherson E. G., Nowak D. J., Rowntree R. A. (Radnor: USDA Forest Service, Northeastern Forest Experiment Station).
Nowak D. J., Greenfield E. J., Hoehn R. E., Lapoint E. (2013). Carbon storage and sequestration by trees in urban and community areas of the United States. Environ. pollut. 178, 229–236. doi: 10.1016/j.envpol.2013.03.019
Nowak D., Rowntree R., McPherson E., Sisinni S., Kerkmann E., Stevens J. (1996). Measuring and analyzing urban tree cover. Landscape Urban Plann. 36, 49–57. doi: 10.1016/S0169-2046(96)00324-6
Oke T. (1982). The energetic basis f the urban heat-island. Q. J. R. Meteorological Soc. 108, 1–24. doi: 10.1002/qj.49710845502
Ossola A., Hoeppner M. J., Burley H. M., Gallagher R. V., Beaumont L. J., Leishman M. R. (2020). The Global Urban Tree Inventory: A database of the diverse tree flora that inhabits the world’s cities. Global Ecol. Biogeogr. 29, 1907–1914. doi: 10.1111/geb.13169
Pataki D. E. (2021). The benefits and limits of urban tree planting for environmental and human health. Front. Ecol. Evol. 9. doi: 10.3389/fevo.2021.603757
Polsky C., Grove J. M., Knudson C., Groffman P. M., Bettez N., Cavender-Bares J., et al. (2014). Assessing the homogenization of urban land management with an application to US residential lawn care. Proc. Natl. Acad. Sci. United States America 111, 4432–4437. doi: 10.1073/pnas.1323995111
Prigioniero A., Paura B., Zuzolo D., Tartaglia M., Postiglione A., Scarano P., et al. (2022). Holistic tool for ecosystem services and disservices assessment in the urban forests of the Real Bosco di Capodimonte, Naples. Sci. Rep. 12, 16413. doi: 10.1038/s41598-022-20992-0
Raciti S. M., Hutyra L. R., Newell J. D. (2014). Mapping carbon storage in urban trees with multi-source remote sensing data: Relationships between biomass, land use, and demographics in Boston neighborhoods. Sci. Total Environ. 500, 72–83. doi: 10.1016/j.scitotenv.2014.08.070
Schwendenmann L., Mitchell N. (2014). Carbon accumulation by native trees and soils in an urban park, Auckland. New Z. J. Ecol. 38, 213–220.
Seto K. C., Güneralp B., Hutyra L. R. (2012). Global forecasts of urban expansion to 2030 and direct impacts on biodiversity and carbon pools. Proc. Natl. Acad. Sci. U. S. A. 109, 16083–8.
Smith I. A., Dearborn V. K., Hutyra L. R. (2019). Live fast, die young: Accelerated growth, mortality, and turnover in street trees. PloS One 14, e0215846. doi: 10.1371/journal.pone.0215846
Sonti N. F., Groffman P. M., Nowak D. J., Henning J. G., Avolio M. L., Rosi E. J. (2022). Urban net primary production: Concepts, field methods, and Baltimore, Maryland, USA case study. Ecol. Appl. 32. doi: 10.1002/eap.2562
Strohbach M., Arnold E., Haase D. (2012). The carbon footprint of urban green space-A life cycle approach. Landscape Urban Plann. 104, 220–229. doi: 10.1016/j.landurbplan.2011.10.013
Teixeira C. P., Fernandes C. O. (2020). Novel ecosystems: a review of the concept in non-urban and urban contexts. Landscape Ecol. 35, 23–39. doi: 10.1007/s10980-019-00934-4
Trammell T. L. E., Pataki D. E., Pouyat V. R., Groffman P. M., Rosier C., Bettez N., et al. (2020). Urban soil carbon and nitrogen converge at a continental scale. Ecol. Monogr. 90. doi: 10.1002/ecm.1401
Ucar Z., Bettinger P., Merry K., Akbulut R., Siry J. (2018). Estimation of urban woody vegetation cover using multispectral imagery and LiDAR. Urban Forestry Urban Greening 29, 248–260. doi: 10.1016/j.ufug.2017.12.001
Vico G., Revelli R., Porporato A. (2014). Ecohydrology of street trees: design and irrigation requirements for sustainable water use. Ecohydrology 7, 508–523. doi: 10.1002/eco.1369
Vogt J., Watkins S., Mincey S., Patterson M., Fischer B. (2015). Explaining planted-tree survival and growth in urban neighborhoods: A social-ecological approach to studying recently-planted trees in Indianapolis. Landscape Urban Plann. 136, 130–143. doi: 10.1016/j.landurbplan.2014.11.021
Whittaker R. H., Bormann F. H., Likens G. E., Siccama T. G. (1974). The Hubbard brook ecosystem study: Forest biomass and production. Ecol. Monogr. 44, 233–254. doi: 10.2307/1942313
Wolf K. L. (2020). Urban trees and human health: a scoping review. Int. J. Environ. Res. Public Health 17. doi: 10.3390/ijerph17124371
Zhuang Q., Shao Z., Gong J., Li D., Huang X., Zhang Y., et al. (2022). Modeling carbon storage in urban vegetation: Progress, challenges, and opportunities. Int. J. Appl. Earth Observation Geoinformation 114. doi: 10.1016/j.jag.2022.103058
Keywords: urban, carbon, meta-analysis, tree, global
Citation: Jenerette GD and Herrmann DL (2024) A global synthesis of reported urban tree carbon production rates and approaches. Front. Ecol. Evol. 11:1244418. doi: 10.3389/fevo.2023.1244418
Received: 22 June 2023; Accepted: 11 December 2023;
Published: 10 January 2024.
Edited by:
Mark Potosnak, DePaul University, United StatesReviewed by:
Waishan Qiu, Cornell University, United StatesCopyright © 2024 Jenerette and Herrmann. This is an open-access article distributed under the terms of the Creative Commons Attribution License (CC BY). The use, distribution or reproduction in other forums is permitted, provided the original author(s) and the copyright owner(s) are credited and that the original publication in this journal is cited, in accordance with accepted academic practice. No use, distribution or reproduction is permitted which does not comply with these terms.
*Correspondence: G. Darrel Jenerette, ZGFycmVsLmplbmVyZXR0ZUB1Y3IuZWR1
Disclaimer: All claims expressed in this article are solely those of the authors and do not necessarily represent those of their affiliated organizations, or those of the publisher, the editors and the reviewers. Any product that may be evaluated in this article or claim that may be made by its manufacturer is not guaranteed or endorsed by the publisher.
Research integrity at Frontiers
Learn more about the work of our research integrity team to safeguard the quality of each article we publish.