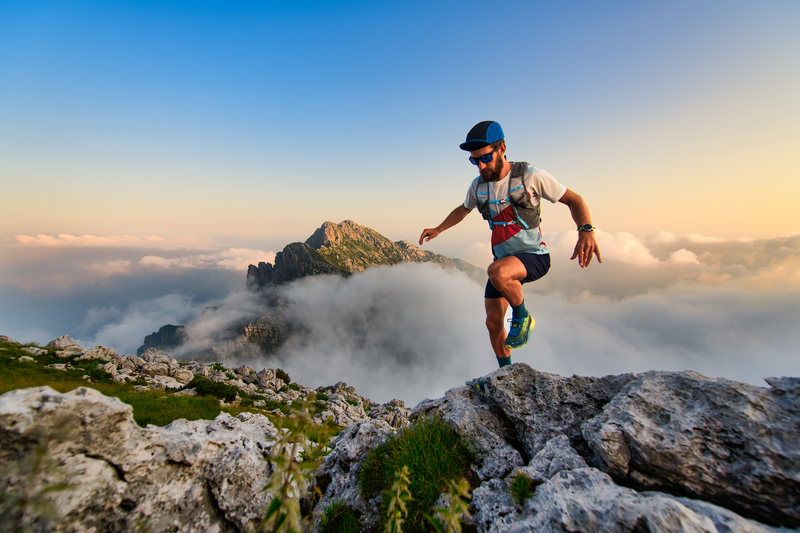
95% of researchers rate our articles as excellent or good
Learn more about the work of our research integrity team to safeguard the quality of each article we publish.
Find out more
ORIGINAL RESEARCH article
Front. Ecol. Evol. , 16 August 2023
Sec. Urban Ecology
Volume 11 - 2023 | https://doi.org/10.3389/fevo.2023.1234374
Introduction: Green technology innovation efficiency (GTIE) growth is an essential route to protect the urban ecological environment in the Chengdu-Chongqing Economic Circle (CCEC). However, the measurement and spatial driving factors of GTIE are still puzzled.
Methods: This study constructs an urban GTIE indicator system including inputs, desired outputs, and undesired outputs, and evaluates urban GTIE in the CCEC using the super-efficiency slacks-based measure (S-SBM). Then, the exploratory spatial data analysis (ESDA) method is applied to analyze the geographical distribution and spatial correlation characteristics of urban GTIE, and a spatial econometric model is used to analyze the influencing factors of urban GTIE from the perspective of spatial spillover.
Results: The results suggest that: (1) From 2006 to 2020, the urban GTIE of CCEC has obviously increased, and its spatial distribution has a prominent unbalanced feature. (2) The urban GTIE of CCEC mainly presents a significant spatial positive correlation, mainly manifested in the "high-efficiency type" and "low-efficiency type" regional agglomeration patterns, and the spatial "core-edge" structure centering on Chengdu and Chongqing tends to be stable. (3) Economic development (ED), government support (GS), and environmental regulation (ER) can promote the urban GTIE. The negative spillover effects of external opening (EO) and ER are significant, and they have negative effects on the GTIE of neighboring cities. (4) Spatial heterogeneity analysis shows that the driving factors of urban GTIE with different efficiency levels are significantly different, and the spatial spillover effect of the driving factors of GTIE is more significant in high-efficiency cities.
Discussion: The urban GTIE in the CCEC is showing a growth trend, but it needs to narrow the gap in GTIE between cities. Firstly, cities can improve the GTIE by improving ED, strengthening GS, and enhancing ER. Secondly, cities need to pay attention to the negative spatial spillover effects of ER and EO in the process of improving the GTIE. Finally, cities with different efficiency types need to develop differentiated green innovation development strategies based on their own conditions.
Green technology innovation (GTI) is an innovation activity that provides new products, processes, services, and management solutions through technological innovation with the fundamental purpose of reducing natural resource consumption, protecting the ecological environment, and improving resource allocation efficiency (Braun and Wield, 1994; Mirata and Emtairah, 2005; Roh et al., 2021). GTI combines technological innovation and environmental protection, becoming an important way to cope with economic recession and climate change. Since entering the 21st century, China’s past economic development model of “high speed and low quality” at the cost of environmental pollution has accumulated a series of environmental pollution problems. The resource dividend and labor dividend in China are gradually disappearing, and environmental pollution problems are frequent. Green development has become an essential way for China’s economy to achieve green and low-carbon transformation. GTI is the driving force of China’s green development and the fulcrum to pivot the development of green industries. In this context, GTI has received unprecedented attention in China (Wang et al., 2019). The 14th Five-Year Plan for National Economic and Social Development and the Outline of Vision 2035 promulgated by the Chinese government has put forward the development goal of “accelerating the green transformation of the development mode and building a market-oriented GTI system”. In this context, China’s State Council, National Development and Reform Commission, and Ministry of Science and Technology have successively issued policy documents to promote the development of GIT, such as “Guiding Opinions on Building a Marketization Green Technology Innovation System” and “Implementation Opinions on Accelerating the Establishment and Improvement of a Green Low Carbon Cycle Development Economic System”.
Existing studies mainly focus on the efficiency measurement of GTI, and the research content mainly focuses on GTIE at industry and enterprise levels. At the industry level, existing studies have measured the GTIE of industrial, manufacturing, and high-tech industries (Wang et al., 2017; Xu et al., 2023; Zhang et al., 2023); at the firm level, existing studies have measured the GTIE of energy-based firms (Jiang et al., 2021). It is not difficult to find that existing studies have paid less attention to urban GTIE. In fact, urbanization is the main driver of regional economic growth. Cities are the unit of action for the implementation of the Paris Agreement in each country and the base unit for policy implementation within countries (Liu K. et al., 2022). China is the world’s largest developing country and is undergoing rapid urbanization, facing serious environmental and sustainable development challenges. Cities are the main vehicle of rapid urbanization in China. Studying the GTIE of Chinese cities can provide policymakers with scientific guidance on the transformation and sustainable development of cities, and promote a win-win situation of ecological protection and economic growth in China. The GTIE of Chinese cities has been studied (Fan et al., 2021; Liao and Li, 2023), but there is a lack of attention to the GTIE of Chinese regional urban agglomerations, which reduces the understanding of the GTIE of Chinese regional urban agglomerations and leads to a lack of reference materials for government departments in formulating regional sustainable development policies. Therefore, the GTIE of regional urban agglomerations in China is an important issue worth studying. As the intersection of China’s Silk Road Economic Belt and Yangtze River Economic Belt, the CCEC has a unique strategic position in China’s “The Silk Road Economic Belt and the 21st-Century Maritime Silk Road” and Yangtze River Economic Belt development strategy, and plays a demonstration and leading role in promoting China’s sustainable development process. In 2021, the State Council of China issued the “Master Plan for Chengdu-Chongqing Economic Circle”, which pointed out that the CCEC faces problems such as weak innovation support capacity and deteriorating ecological environment. Promoting the improvement of urban GTIE is an important way for cities in the CCEC to achieve ecological protection and sustainable development. Therefore, measuring the GTIE of the CCEC is conducive to understanding the spatiotemporal trends and driving mechanisms of urban GTIE, which provides a scientific basis for policymakers and promotes the realization of synergistic economic, social, and environmental development of the CCEC. In addition, analyzing the GTIE of the CCEC can evaluate the implementation effect of China’s regional sustainable development policies, identify the shortcomings of the policies, and propose relevant policy recommendations and optimization solutions, providing examples and inspiration for the overall environmental governance and policy improvement in China.
In this context, this paper uses the S-SBM, ESDA, and spatial econometric models to analyze the spatiotemporal trend and driving factors of urban GTIE in the CCEC from 2006 to 2020. This paper attempts to elaborate on the spatiotemporal divergence pattern and spatial mechanism of urban GTIE, expand and enrich the related research on urban GTIE, and provide a reference for decision-making for cities in the CCEC to achieve the goals of economic green transformation and sustainable development. Compared with the existing literature, the contribution of this paper is mainly in the following aspects: Firstly, existing studies have less consideration for the impact of resource factors and environmental benefits on GTIE when constructing measurement indicators. This paper constructs an urban GTIE index system including inputs, desired outputs, and undesired outputs and innovatively incorporates resource factors and environmental benefits into inputs and desired outputs, respectively, which enriches the research of urban GTIE measurement. Secondly, existing studies have paid less attention to GTIE in Chinese cities, and there are few studies on GTIE in regional cities. This paper uses ESDA to analyze the geographical distribution and spatial correlation of urban GTIE, which is conducive to accurately identifying the spatial characteristics and distribution patterns of urban GTIE in the CCEC and broadening the boundaries of existing research. Thirdly, existing studies have mainly examined the influencing factors of GTIE based on environmental economics, and few studies have examined the spatial drivers of GTIE from the perspective of new economic geography. This paper uses a spatial econometric model to examine the driving factors and heterogeneity of urban GTIE from a spatial perspective, which is conducive to summarizing the driving mechanisms of urban GTIE, provides a scientific basis for exploring the path of sustainable urban development in the CCEC, and expands the perspective of existing research.
Academic circles are increasingly focusing on GTI, from sustainable development to the growth of the green economy. Existing studies have mainly focused on the efficiency measurement and impact factors of GTI. Some scholars focus on the efficiency measurement of GTI. In terms of research objects, scholars mainly focus on GTIE at the industry level, such as measuring GTIE in manufacturing, high-tech industries, and more broadly in the industrial sector (Wang et al., 2017; Zhu et al., 2018; Yang et al., 2020; Xu et al., 2023; Zhang et al., 2023), while some scholars focus on GTIE at the provincial level in China (Luo and Liang, 2016; Li, 2017; Shen et al., 2022). It is not difficult to find that existing studies lack attention to urban GTIE. Scholars have studied the GTIE of Chinese cities (Fan et al., 2021; Liao and Li, 2023), but there are fewer studies on the GTIE of Chinese regional urban agglomerations. In terms of research methods, existing studies generally use Stochastic Frontier Analysis (SFA) and Data Envelopment Analysis (DEA); for example, Cao and Yu (2015) measured the GTIE of each region in China from 2005 to 2011 based on an improved SFA. Studies using DEA methods are mainly based on improved DEA models; for example, Zhang M. et al. (2022) measured GTIE in 30 provinces in China based on the Slacks-Based Measurement (SBM) model and further discussed the regional differences in GTIE. Fang et al. (2020) used non-radial directional distance function-data envelopment analysis (DDF-DEA) to measure the GTIE of heavy pollution industries in China. With the increasing research on efficiency measurement, Tone, (2001) proposed a non-radial, non-angle SBM model based on slack variables by considering the effect of slack on the basis of traditional DEA, which is able to measure the efficiency of decision-making units with undesired outputs. Zhao et al. (2021) measured the GTIE of Chinese provinces based on the SBM model. Thereafter, Tone (2002) combined the SBM model with super-efficiency and proposed the S-SBM model, which is better able to identify the relative effectiveness of effective decision-making units. However, few studies have applied the S-SBM model to the measurement of urban GTIE. In terms of evaluation indexes, existing studies mainly measure the input elements of GTIE from dimensions such as capital input and human resources, and measure the desired outputs of GTIE from dimensions such as technical and economic benefits (Fan et al., 2011; Cao and Yu, 2015; Wu and Fan, 2023). It is not difficult to find that resource inputs and environmental benefits are not considered in the indicator system of GTIE constructed by existing studies.
Other scholars have turned their research perspectives to the influencing factors of GTIE. GTI combines technological innovation and environmental effects, and it has many characteristics such as spillover and uncertainty, resulting in complex factors that affect GTIE. Enterprises are the main carriers of GTIE, and existing studies have examined the influencing factors of GTIE from both the external and internal levels of enterprises (Demirel and Kesidou, 2011), which mainly include internal factors such as R&D investment and enterprise size, and external factors such as environmental regulation and economic development (Hojnik and Ruzzier, 2016; Demirel and Kesidou, 2019). Ambec and Barla (2002) argue that environmental regulation has the effect of promoting GTI, and the pressure of environmental regulation can effectively incentivize the improvement of GTIE. Hashimoto and Haneda (2008) argue that government financial support and tax incentives can enhance the GTIE of enterprises. Shao et al. (2016) argue that increasing R&D investment increases GTIE, while capital deepening decreases GTIE. Some scholars have also pointed out that management decision-making is an important factor affecting the GTI of enterprises, and management’s environmental awareness is conducive to promoting GTI and enhancing the GTIE of enterprises (Colwell and Joshi, 2013). However, Schaarschmidt (2016) argues that corporate decisions are made by management, but employees are the specific operators of tasks. Only when employees have a strong sense of responsibility and belonging, will they be motivated to improve the GTIE of the enterprise. Zailani et al. (2015) also argue that employees completing work according to green standard processes in production can reduce the environmental costs of the enterprise, and employees are an important factor in enhancing the GTIE of the enterprise. On this basis, Amore and Bennedsen (2016) argue that the improvement of corporate governance levels is beneficial for the GTI of enterprises. It is not difficult to find that there is a wealth of research on the influencing factors of GTIE. However, in discussing the influencing factors of GTIE, existing studies mainly focus on the direct effects of each influencing factor, ignoring the positive externalities of GTI knowledge diffusion. With the deepening of related research on GTIE, academics have gradually focused on the spatial effects of GTIE, and spatial factors have begun to be embedded in the research framework of the influencing factors of GTIE (Sun and Chen, 2021).
In summary, the existing literature has fruitfully explored the measures and influencing factors of GTIE, but there are still elements for further optimization. Firstly, existing studies have not reached a consensus on the connotation of GTI, and have ignored environmental pollution factors in the selection of quantitative indicators for GTIE. The literature on environmental pollution ignores the systematicity and complexity of GTI, only considering human and capital factors in the input indicators of GTIE, and only considering economic and innovative benefits in the desired output indicators of GTIE, ignoring resource and environmental benefits, and failing to accurately explain the connotation of GTIE. Secondly, existing literature on GTIE mainly focuses on the provincial and industry levels in China, with few studies focusing on the influencing factors of GTIE at the urban level in China. Moreover, there have been few studies on GTIE in Chinese urban agglomerations, resulting in insufficient demonstration and guidance from the existing studies. Thirdly, the existing studies mainly focus on the direct effect of the influencing factors of GTIE, ignoring the negative externality of environmental pollution and the positive externality of knowledge diffusion, and fail to reveal the spatial influence mechanism of GTIE. Fourthly, existing studies mainly focus on the mechanisms of influencing factors on GTIE, ignoring the heterogeneity of influencing mechanisms that may result from differences in the level of economic development and resource endowment among cities.
In this context, this paper enriches the existing research in the following aspects: Firstly, based on the connotation of GTI, this paper not only focuses on the human factor and capital factor in the input indicators of GTIE and the economic and innovation benefits in the desired output indicators, but also incorporates the resource factor and the environmental benefits into the input indicators and the desired output indicators of GTIE, respectively, so as to comprehensively consider the double externalities of GTI. Secondly, from the perspective of urban agglomeration, this paper analyzes the spatial characteristics and dynamic transition path of GTIE in CCEC by combining the S-SBM model with undesired output and ESDA. Thirdly, focusing on the double externality characteristics of GTI, this paper examines the spatial influencing factors of GTIE and its spatial heterogeneity in the CCEC based on a spatial econometric model. The conclusions based on the above analysis have great theoretical value and practical significance for promoting the green transformation of cities in the CCEC.
The CCEC covers 16 cities in western China, specifically including Chongqing and 15 cities in Sichuan Province (Chengdu, Luzhou, Mianyang, Zigong, Neijiang, Meishan, Deyang, Suining, Leshan, Guang’an, Nanchong, Dazhou, Yibin, Ziyang and Ya’an), with a total area of 185,000 square kilometers, accounting for 1.9% of China’s total area. In 2021, the resident population of the CCEC is 96 million, accounting for 6.9% of China’s total population, and its regional GDP is 7.39 trillion RMB, accounting for 6.5% of China and 30.8% of western China.
The CCEC is located at the intersection of China’s “Belt and Road” and the Yangtze River Economic Belt, and as the beginning of the new land-sea corridor in western China, which has the distinct advantages of linking southwest and northwest China and facilitating communication with East Asia, Southeast Asia, and South Asia (see Figure 1). The CCEC enjoys excellent ecological endowments, abundant energy and mineral resources, and dense towns, making it the most densely populated, most industrially advanced, most innovative, most open and with the widest market space in western China, with a unique and important strategic position in the national development.
This paper analyzes the spatial influence mechanism of urban GTIE from four perspectives (ED, EO, GS, and ER) based on the existing studies (see Figure 2).
The first influence mechanism is the effect of ED on GTIE. Firstly, compared with traditional innovation, GTI has a higher threshold of entry, and it needs more financial support effect and green human resources agglomeration effect of ED (Fan and Sun, 2020). Higher levels of ED are usually accompanied by greater investment in R&D and technological innovation, which provides GTI with richer resources and conditions (Fan and Teo, 2022). Cities with higher levels of ED can attract more innovative talent and capital, and the resulting economic agglomeration effect promotes technological progress and innovation, which improves urban GTIE. Secondly, with the improvement of ED levels, residents’ awareness of environmental protection and sustainable development is becoming stronger, and they tend to prefer green products in their daily consumption (Zameer and Yasmeen, 2022). The large-scale market demand also provides a broader market space for the promotion of green products and technologies, further contributing to the improvement of urban GTIE. In cities with higher levels of ED, governments are usually more willing to develop and implement policies and plans that support GTI. At the same time, businesses are able to devote more resources to GTI. This positive environment of demand and support will lead to an increase in urban GTIE (Huang and Wu, 2019). Thirdly, the level of ED is closely related to the industrial structure of cities, which also has an impact on GTIE. For example, in economically developed cities, their economic structure is relatively diversified, with richer industrial and supply chains. This provides more opportunities for GTIE enhancement (Su and Fan, 2022).
The second influence mechanism is the effect of EO on GTIE. EO is crucial for promoting economic growth in Chinese cities, and it also has a significant impact on urban GTIE. On the one hand, EO can promote the dissemination and exchange of knowledge and technology, and have a positive impact on urban GTIE. Through cooperation with foreign enterprises and research institutions, cities can obtain more advanced technologies and management models from around the world. This flow of knowledge and technology can help cities to acquire and assimilate advanced GTI technologies more quickly, thus improving GTIE (Yang et al., 2017; Wang et al., 2022). On the other hand, EO also contributes to the competitiveness and innovation drive of cities. By aligning with the international market, urban enterprises face greater market competition pressure, which will force them to accelerate their innovation pace and improve the technological content of their products. Enterprises and research institutions in cities will also face innovation competition from other countries and regions, and this competitive pressure will drive cities to increase their R&D investment in GTI, which will improve the urban GTIE (Yang et al., 2017). However, the impact of EO on urban GTIE is generally positive, but there is also the phenomenon of “pollution paradise” (Luo et al., 2023). The “pollution paradise” hypothesis suggests that developed regions, in the process of outward investment and industrial transfer, prefer to establish energy-intensive enterprises or production processes in regions with lower environmental standards for their own environmental protection considerations, which has a negative impact on GTIE in areas where foreign capital flows (Qin and Sun, 2019; Ouyang et al., 2020). The higher the EO level of a city, the more susceptible it is to the “pollution paradise” phenomenon, leading to the city becoming a “refuge” for international low-end production capacity and reducing the urban GTIE (Luo et al., 2022).
The third influence mechanism is the effect of GS on GTIE. When the city’s government provides effective support measures, it will directly affect the urban GTIE. Firstly, the research and transformation of GTI have dual externalities, and the high development cost and high commercial uncertainty of GTI are the main obstacles for enterprises to undertake GTI activities. Traditional non-green technologies are still dominant, and enterprises have limited expected returns from GTI (Stucki, 2019). GS has improved urban GTIE by providing financial support in the form of investments, subsidies, and loans to encourage firms to invest and conduct research in GTI (Yang et al., 2021). Secondly, GS has upgraded GTIE by enacting laws, regulations, and policy measures favorable to GTI. For example, the government establishes green industrial parks, provides tax incentives and subsidy policies, and supports the establishment and development of GTI related enterprises (Wu et al., 2021). At the same time, the government has promoted GTIE by strengthening intellectual property protection and encouraging knowledge creation and sharing. Thirdly, GS promotes the cultivation and exchange of GTI talents by establishing a talent cultivation system for GTI and providing opportunities for educational training and research institution cooperation. The government’s support and investment have improved the quality and quantity of talent, provided talent support for enterprises to conduct GTI, and promoted the improvement of urban GTIE (Liu F. et al., 2022).
The fourth influence mechanism is the effect of ER on GTIE. GTI combines technological innovation and environmental protection, which is closely related to environmental regulation; ER has a significant impact on GTIE. Firstly, strict ER can drive enterprises to invest and develop in technological innovation to meet prescribed environmental standards and limitations. It has strengthened the research and development of green technology and innovation, and improved GTIE (Ahmed, 2020; Peng et al., 2021). Secondly, ER can promote the development and promotion of more environmentally friendly technologies and products by enterprises through market-oriented mechanisms, such as carbon emissions trading and carbon pricing. This change in market demand can prompt enterprises to shift towards GTI and improve GTIE (Fu et al., 2023). Thirdly, ER can drive enterprises to optimize resource utilization and improve the efficiency of production and operations. Regulations to limit emissions and reduce waste generation are forcing enterprises to look for more environmentally friendly production processes and resource utilization methods to enhance GTIE (Popp, 2006). However, the impact of ER on urban GTIE is usually positive, but there is also a “cost of compliance” hypothesis. The “cost of compliance” hypothesis suggests that strict ER may require enterprises to invest significant additional resources and funds to meet environmental standards and requirements. This could increase the cost burden on enterprises, reduce their incentives and capacity for GTI, and inhibit urban GTIE (Conrad and Wastl, 1995).
DEA, as a measure of the efficiency of complex systems, is highly applicable to the measurement of GTIE with multiple input and output indicators. However, the traditional DEA assumes that all outputs are desired outputs, so it is impossible to measure the efficiency value when there are undesired outputs. To overcome the above shortcomings, Tone (2001) proposed a non-radial, non-angular SBM model based on slack variables, which is able to quantify the effectiveness of decision units with undesired outputs, by considering the effect of slack on the basis of traditional DEA. Thereafter, Tone (2002) combined the SBM model with super-efficiency and proposed the S-SBM model. In this context, this paper uses the S-SBM model to measure GTIE in the CCEC.
The S-SBM model assumes that there are n decision-making units (DMUs) in the production system, each containing an input vector X, a desired output vector Y, and a undesired output vector Z. The vector elements can be represented as , , and . Define the matrices , , , where , , . The formula for S-SBM model that includes undesired outputs is shown in Equation (1).
where is the value of GTIE, , , represent the input, desired outputs, and undesired outputs of DMU, respectively, m, l, h represent the number of inputs, desired outputs and undesired outputs, respectively, , , represent the slack vectors for inputs, desired outputs and undesired outputs, respectively, and is the weight of DMU.
The ESDA includes global and local spatial correlation analysis. To analyze the spatial correlation and distribution pattern of GTIE in CCEC, this paper adopts the global Moran’s I to test the global spatial agglomeration characteristics of GTIE, and its formula is shown in Equation (2).
where I is the global Moran’s I, n is the number of cities, xi, xj represent the GTIE of city i and city j, respectively, S2 represents the sample variance, and wij represents the elements of the spatial weight matrix. The level of economic development of cities is closely related to the GTIE (Li, 2017), and the economic distance matrix based on the level of economic development is a more realistic reflection of the spatial correlation between cities than the traditional adjacency matrix. In this context, this paper refers to the method of Lin et al. (2005) to measure the economic distance between cities using the difference in per capita regional GDP of cities. The elements of the economic distance matrix between cities are , where is the city’s gross regional product per capita, and the economic distance between the city and itself is specified as zero. The global Moran’s I takes a value between −1 and 1. If I > 0, it means that there is a positive spatial correlation in the GTIE of cities. If I< 0, it means that there is a negative spatial correlation in the GTIE of cities. If I is close to zero, it means that the spatial distribution of GTIE is random and there is no spatial correlation.
The global spatial correlation analysis is difficult to reveal the location and local correlation degree of GTIE. Therefore, this paper uses the local Moran’s I to test the local spatial agglomeration characteristics of GTIE, and its formula is shown in Equation (3).
where Ii is the local Moran’s I and the rest of the symbols are consistent with Equation (2). If Ii > 0, it means that cities with similar GTIE characteristics are clustered together, which means that cities with high (low) GTIE are surrounded by cities with high (low) GTIE, defined as “high-high (low-low)” clustering. If Ii< 0, it means that cities with different GTIE characteristics are clustered together, which means that cities with high (low) GTIE are surrounded by cities with low (high) GTIE, defined as “high-low (low-high)” clustering.
The GTI has the property of double externality, and the traditional econometric model, which assumes inter-regional independence, is no longer applicable. The GTI has dual externalities, and the traditional econometric model based on the premise of mutual independence between regions is no longer applicable. Therefore, spatial econometric modeling becomes an effective method to analyze the spatial influence mechanism of GTIE. The Spatial Error Model (SEM), Spatial Lag Model (SLM), and Spatial Durbin Model (SDM) are three of the most widely used spatial econometric models, but the SEM and SLM ignore the spatial dependence of the explained and explanatory variables, which will lead to biased estimation results of the models (Lesage and Pace, 2009), and the two models have defects in the analysis of direct and indirect effects (Elhorst, 2014). However, the SDM takes into account both endogenous and external spatial interaction effects and is better able to examine the spatial influence mechanism of GTIE. Meanwhile, to solve the problem of omitted variables due to regional differences and time differences, urban effects and time effects are added to the SDM. The model is set as in Equation (4).
where TEC represents the GTIE, α represents the constant term, ρ represents the spatial autoregressive coefficient of GTIE, W represents the spatial weight matrix, which is consistent with the spatial weight matrix setting in ESDA, β and χ represent parameters to be estimated, δ represents the coefficient of spatial effects, X represents the influencing factors of GTIE, Z represents control variables, μ represents unobservable urban effects, γ represents time effects, and ϵ represents the spatial error term.
In the spatial Durbin model, the explanatory variables of the city affect not only the explained variables of the city (direct effects), but also the explained variables of the spatially adjacent cities (indirect effects). The SDM has direct effects (β) and indirect effects (δ), but when the coefficient of the spatial lag term of the explained variables (ρ) is significantly not equal to zero, the direct and indirect effects cannot be identified by the parameters in the model (Lesage and Pace, 2009). Therefore, this paper decomposes the spatial effects of the SDM based on the partial differential approach. In this paper, Equation (4) is expressed as a matrix form as shown in Equation (5).
where the partial derivatives of the explained variables with respect to the explanatory and control variables are shown in Equation (6) and (7).
where n represents the number of cities and k represents the number of explanatory or control variables. The spatial effect in Equation (6) is decomposed into direct effect and indirect effect. According to the average indicator method of Lesage and Pace (2009), the mean of the main diagonal elements of the rightmost partial derivative matrix in Equation (6) represents the direct effect of the model, which represents the degree of influence of the city’s explanatory variables on the GTIE of the city. The mean value of the non-diagonal elements in each column or row of the partial derivative matrix indicates the indirect effect of the model, representing the degree of influence of the city’s explanatory variables on the GTIE of spatially adjacent cities, and the sum of the direct and indirect effects indicates the total effect of the model. If δ of Equation (4) is significantly 0, the ratio of direct and indirect effects of all explanatory variables is equal, and the results are shown in Equation (7).
The explained variable is GTIE. Based on the existing research (Shu et al., 2016; Zhu et al., 2018; Lv et al., 2019), this paper defines the connotation of GTI as a new innovation activity that takes into account technological innovation and ecological protection, which has the positive externality of knowledge spillover and the negative externality of environmental pollution, constructs the evaluation index system of GTIE from three aspects: factor input, desired output and undesired output (see Table 1), and uses the S-SBM model to measure the GTIE of each city in CCEC.
In the selection of input indicators, compared with the existing literature which only considers capital input and human input (Huang and Wu, 2019; Lv et al., 2019), this paper innovatively adds resource input, and measures the factor input from capital, human and resource, respectively. In the selection of desired output indicators, this paper not only covers general innovation outputs, but also adds environment-related outputs, and innovatively measures desired outputs in terms of economic benefits, technological benefits and environmental benefits. In the selection of undesired output indicators, this paper measures the undesired outputs in terms of air pollution, water pollution and dust pollution.
The explanatory variables are the main influencing factors of GTIE. According to the findings in the previous section of influence mechanism analysis, the main factors affecting urban GTIE of the CCEC are ED, EO, GS, and ER. Therefore, the above four variables are selected as explanatory variables in this paper. ED is measured by the city’s per capita gross regional product, EO is measured by the proportion of actual foreign investment used in the city to the total social fixed asset investment, GS is measured by the proportion of the city’s science and technology expenditure to the local fiscal expenditure, and ER is measured by the proportion of the city’s total investment in environmental pollution control to the regional gross product.
The control variables are other variables that affect urban GTIE. Referring to the findings of existing literature (Huang and Wu, 2019; Sun and Chen, 2021), this paper adds control variables such as industrial structure (IS), human capital (HC), and urbanization rate (UR) to the model. IS is measured by the share of value added of secondary industry in regional GDP in cities, HC is measured by the share of employment in secondary industry in total employment in cities, and UR is measured by the share of urban resident population in total population in cities at the end of the year.
On the basis of data availability and comparability, this paper selects the panel data of 16 cities in the CCEC from 2006 to 2020. The data in this paper are obtained from the 2007 to 2021 Chongqing Statistical Yearbook, Sichuan Statistical Yearbook, Sichuan Science and Technology Statistical Yearbook, Sichuan Science and Technology Yearbook, Chongqing Science and Technology Statistical Yearbook, China Urban Statistical Yearbook, China Environmental Statistical Yearbook and the official website of the Sichuan Intellectual Property Service Promotion Center. For the treatment of missing values and outliers in the data, this paper adopts the Lagrangian interpolation method and the linear interpolation method to fill in the missing values and outliers according to the change trend of the data samples.
Figure 3 shows that the GTIE of CCEC shows a growth trend with obvious characteristics of stage evolution, which can be divided into three stages during the sample period. The first stage is the “growth period” from 2006 to 2010, in which GTIE showed a trend of decreasing and then increasing, from 0.78 in 2006 to 0.85 in 2010. This stage is the early stage of the construction of the CCEC, technological innovation collaboration and joint construction of ecological barrier are the key tasks of Chengdu and Chongqing to promote the construction of the economic circle, and a series of policies are implemented to promote GTIE. The second phase is the “oscillation period” from 2010 to 2014, in which the GTIE shows a fluctuating trend and reaches the maximum value of 0.92 in the sample period in 2013. This stage is the alternate transition period of China’s “11th Five-Year Plan” and “12th Five-Year Plan”, and the pace of industrial restructuring is fast. Meanwhile, in 2011, China’s National Development and Reform Commission released the Regional Plan for Chengdu-Chongqing Economic Zone. The dual influence of industrial restructuring and government planning is an important reason for the fluctuation of GTIE. The third phase is the “revitalization period” from 2014 to 2020, in which GTIE shows an increase from 0.81 in 2014 to 0.83 in 2020. This stage is China’s “13th Five-Year Plan” period. Green development has become an important goal of China’s economic and social development, and the quality of the ecological environment has been widely valued by governments at all levels. The CCEC has actively promoted energy efficiency and emission reduction, and the implementation of environmental protection policies and the development of green industries have further promoted GTI.
In this paper, we classify and spatially visualize the time-series data of GTIE based on the natural fracture method for hierarchical classification and spatial visual representation. Figure 4 shows that the GTIE is spatially unbalanced, and that the “double core” position of Chengdu and Chongqing in the economic circle is gradually prominent. From 2006 to 2010, the higher-efficiency cities of GTIE are transformed from Chengdu to Chongqing, the GTIE in medium-efficiency cities is significantly improved, and the high-efficiency cities are transformed from Meishan, Ziyang and Chongqing to Mianyang, Chengdu, Meishan, Ziyang, Neijiang, Guang’an and Dazhou. From 2010 to 2015, Chongqing continued to maintain its status as a higher-efficiency city, and high-efficiency cities shifted from the north to the south of the economic circle, forming a cluster of low-efficiency and medium-efficiency cities in the north and a cluster of high-efficiency and medium-efficiency cities in the south, with an obvious north–south distribution difference in the spatial distribution of GTIE. From 2015 to 2020, the spatial distribution of GTIE changes from a single-core pattern in Chongqing to a double-core pattern centered on Chengdu and Chongqing, with a significant reduction in high-efficiency cities and a contiguous development of medium-efficiency and low-efficiency cities. It is worth noting that the GTI in the CCEC may fall into a “medium efficiency trap” in its future development. For low-efficiency and medium-efficiency cities, there is an invisible “ceiling” of GTIE. The “siphon effect” of higher-efficiency cities makes green innovation resources over-concentrated in a few cities, and the loss of green innovation resources in medium-efficiency and low-efficiency cities is serious, and their industrial structure has been dominated by secondary industries for a long time, which leads to insufficient endogenous power of green innovation. The combination of these factors hinders the progress of low-efficiency and medium-efficiency cities to higher gradient efficiency cities.
In Table 2, it is shown that the global Moran’s I of GTIE is significant at different test levels, indicating that there is a significant spatial correlation of GTIE. Specifically, from 2006 to 2007, the global Moran’s I of GTIE is positive, showing a significant positive spatial correlation, which is characterized by the spatial clustering of “high (low)-efficiency cities surrounded by high (low)-efficiency cities”. However, the absolute value of the Moran’s I gradually decreases, and the positive spatial correlation gradually decreases. From 2008 to 2015, the global Moran’s I of GTIE changed from positive to negative, showing a significant negative spatial correlation, which is characterized by the spatial agglomeration of “high-efficiency cities surrounded by low-efficiency cities”, and the spatial “polarization effect” is prominent. The possible reason is that the 2008 global financial crisis had a negative effect on the economic growth of cities in the CCEC, especially hitting the financial systems of each city, which led to a significant shortage of innovation capital investment in each city. However, cities with high GTIE are rich in green innovation resources, and green innovation factor inputs are less affected by the financial crisis, thus further increasing the green innovation development gap among cities in the economic circle, and GTIE shows a negative spatial correlation. From 2016 to 2020, the global Moran’s I of GTIE turns from negative to positive, showing a significant positive spatial correlation, which is manifested by cities with similar GTIE clustering with each other. The possible reason is the flow of capital, talent, technology and other innovation factors among cities in the economic circle accelerates with the gradual implementation of the government’s economic stimulus policies, driving the upgrading of urban industrial structure and the transformation of green low-carbon development, and the GTIE of each city improves significantly, and the gap of green innovation development among cities in the economic circle gradually narrows, making GTIE turn to be spatially positively correlated.
To analyze the local clustering dynamics of GTIE, this paper organized the distribution of each city in each quadrant of the local Moran’s I scatter plot. Table 3 shows that there are 10 cities located in the first and third quadrants in 2006, increasing to 11 in 2020, accounting for 62.5% and 68.8% of all cities, respectively. It shows that the GTIE in 2006–2020 is mainly spatially positively correlated, and its regional agglomeration pattern is mainly “high-efficiency type” and “low-efficiency type”.
In 2006, there are four cities located in the first quadrant, including Chengdu, Mianyang, Guang’an, and Chongqing. There are six cities in the third quadrant, including Zigong, Deyang, Leshan, Nanchong, Yibin, and Ya’an, which have relatively fewer green innovation resources and lower GTIE. There are relatively few cities in the second and fourth quadrants, accounting for only 37.5% of all cities. There are four cities in the second quadrant, including Luzhou, Suining, Neijiang and Dazhou, which have relatively low efficiency in GTI. Although they are adjacent to high-efficiency cities, they do not effectively undertake the technology spillover of green innovation from high-efficiency cities. There are two cities in the fourth quadrant, including Meishan and Ziyang, which have high GTIE but less green technology spillover to neighboring cities and less spatial radiation. In summary, the GTIE of CCEC is low in 2006, and the spatial agglomeration is mainly “high-efficiency type” and “low-efficiency type”.
In 2020, there are seven cities in the first quadrant, with Luzhou, Neijiang, Meishan and Ziyang added, and Chongqing reduced compared to 2006. Luzhou and Neijiang have changed from “hollow type” to “high-efficiency type”, and Meishan and Ziyang have changed from “polarization type” to “high-efficiency type”. There are four cities in the third quadrant, with Leshan and Yibin reduced compared to 2006. There are relatively few cities in the second and fourth quadrants, accounting for only 31.3% of all cities. There are two cities in the second quadrant, with Luzhou and Neijiang reduced compared to 2006. There are three cities in the fourth quadrant, including Leshan, Yibin, and Chongqing, with Chongqing changing from “high-efficiency type” to “polarization type”, while Leshan and Yibin change from “low-efficiency type” to “polarization type”.
To analyze the spatial leap path of GTIE of cities in the economic circle, this paper extends the scatter plot of the local Moran’s I by referring to the spatiotemporal analysis of Rey and Janikas (2006). Table 4 shows that the leap path of Type I is between “high-efficiency type” and “polarization type”, and the cities include Chongqing, Meishan and Ziyang. No cities leapfrogged in the path of Type II. The leap path of Type III is between “hollow type” and “high-efficiency type”, “low-efficiency type” and “polarization type”, and the cities include Luzhou, Neijiang, Leshan and Yibin. The rest of the cities in the economic circle belong to Type IV which no leap occurs. In summary, GTIE has obvious spatial agglomeration and low mobility characteristics, and its spatial leap shows obvious stability. More than half of the cities do not break away from the original spatial agglomeration pattern, and the double core spatial distribution pattern of the GTIE with Chengdu and Chongqing as the core has stability, and the “core-edge” structure of green innovation development in the CCEC tends to be stable.
The estimation results of SDM are obtained based on the previous model settings (see Table 5). To compare the robustness of the model estimation results, this paper reports the estimation results of SLM and SEM. In Table 4, it is shown that the significance of the parameters of models (2), (4), and (6) is significantly improved after controlling for city effect and time effect, which indicates that GTIE is influenced by individual city attributes and time trends. It is necessary for the model to control for individual and time effects so that the net effect of each influencing factor on the GTIE can be more accurately identified. In models (3) to (6), the spatial effect coefficients of GTIE are all significantly positive, which indicates that there is a significant positive spatial spillover effect of GTIE. The enhancement of GTIE in this city can lead to GTI in spatially adjacent cities. In models (1) and (2), the spatial effect coefficients of the spatial error terms are significantly positive, indicating that there is a spatial spillover effect of the spatially perturbed factors of the model, and the unobserved factors affecting GTIE in this city also have a positive effect on GTIE in spatially adjacent cities.
In addition, the results of the Likelihood Ratio (LR) test show that the test statistics of models (5) and (6) reject the original hypothesis that SDM can degenerate into SLM or SEM at the 1% significance level, which indicates that SDM is optimal. From the estimation results of the models, the spatial autoregressive coefficients of GTIE in models (5) and (6) are significantly positive at the 5% or 1% test level, which indicates that the direct and indirect effects cannot be identified through the estimated parameters in the models, and further partial derivative matrices need to be constructed to decompose the spatial effects. Therefore, this paper decomposes the spatial effects on the basis of model (6) (see Table 6).
The results in Table 6 show that the coefficient of the direct effect of ED is positive at the 5% level of significance, and the coefficient of its indirect effect is not significant. It indicates that ED has a positive effect on the GTIE of cities, but its spatial spillover effect on spatially adjacent cities is not significant. The improvement of the economic development level can stimulate the demand of urban GTI and provide more financial support for GTI activities, which in turn can promote the improvement of urban GTIE. However, Shi and Pan (2021) showed that the CCEC is at the stage of agglomeration development, and an orderly synergistic mechanism of industrial chain division has not yet been formed among cities in the economic circle, and there is even an obvious competition relationship. Therefore, the GTI dividends brought by urban economic development are more locally absorbed, while the promotion of GTI in spatially neighboring cities is limited.
The direct effect coefficient of EO is not significant, and the coefficient of its indirect effect is negative at the 1% level of significance. This indicates that the effect of EO on urban GTIE is not significant, but it shows a negative spillover effect on neighboring cities, which deviates from the outcome that was anticipated. The possible reason is that there is a substantial disparity between China’s degree of innovation in green technology and that of other countries, especially in the key core areas. The expansion of China’s opening to the outside world can increase foreign investment and bring advanced green innovation technologies and management models to cities, accelerating their green development process. However, the breadth and depth of the opening up of the CCEC is still low, and its resource-dependent economic growth model has not yet been completely changed, which leads to the insignificant incentive role of foreign investment in urban GTI. In addition, EO has a negative inhibitory effect on the GTIE of the neighboring cities. In the process of building the strategic highland of inland opening, cities in the CCEC are competing to introduce foreign investment, which to a certain extent leads to the tendency of bottom-up competition in the environment, and neighboring cities take over the low-end production capacity eliminated internationally, resulting in the phenomenon of “pollution paradise” (Huang and Wu, 2019), which inhibits the improvement of GTIE in neighboring cities.
The direct effect coefficient of GS is positive at the 1% significance level, and the coefficient of its indirect effect is not significant. This indicates that GS has a positive effect on the GTIE of the city, but it does not support the neighboring cities enough. The government has weakened the negative externalities and cost uncertainties of green innovation R&D activities mainly through R&D subsidies and low-carbon compensation, thus incentivizing companies to invest resources in GTI projects and enhancing GTIE of cities (Dong et al., 2021). In the process of actively creating China’s GTI center in the CCEC, the government’s financial support is an important guarantee to enhance GTIE. The economic growth model of the CCEC is changing from “factor driven” to “innovation driven”, and the guiding role of green innovation policy is increasingly prominent. The government has given policy and financial support in GTI activities of enterprises, which has improved the GTIE of cities. However, the policy and financial support of the local government are more inclined to local enterprises, which makes it difficult for the neighboring cities to obtain the policy dividend of the local government.
The direct effect coefficient of ER is positive at the 10% level of significance, and its indirect effect coefficient is negative at the 10% level of significance. It shows that ER can improve GTIE of cities, but it shows a negative spillover effect on neighboring cities. Dong et al. (2021) argue that environmental regulation enhances urban GTIE through the “innovation compensation” effect. In the process of jointly building the ecological barrier in the upper reaches of the Yangtze River, the CCEC has continuously strengthened environmental protection standards, which forces enterprises to innovate production technology, creates the “innovation compensation” effect to compensate for the production burden of enterprises under high environmental protection standards, and promotes GTI of enterprises. However, the strict environmental protection standards of cities will not only restrict the environmental pollution of local enterprises, but also force local pollution-oriented enterprises to transfer to neighboring cities with relatively loose environmental protection supervision (Dong and Wang, 2019), and hinder the GTI of neighboring cities.
In order to test the robustness of the spatial effect decomposition results, this paper re-estimates the model parameters in terms of replacing the spatial weight matrix and the measurement indicators of the explained variable, respectively. Firstly, this paper adopts the binary adjacency matrix of cities in the CCEC as the new spatial weight matrix. Secondly, this paper collects green patent application data of cities in the CCEC from 2006 to 2020 based on the International Green Patent Classification List released by the World Intellectual Property Organization in 2010 and the patent search and analysis system of the State Intellectual Property Office of China with reference to the processing methods of existing studies (Zhang C. et al., 2022), and re-measures the urban GTIE by using green patent application data as a measure of desired output. Based on the above two approaches, this paper re-estimates the model (6) in Table 5 and decomposes its spatial effects. The results in Table 7 show that after the introduction of the new spatial weight matrix and the replaced urban GTIE, the absolute values of the direct effect coefficients and indirect effect coefficients of each variable increase or decrease to some extent, and the significance of some variables increases or decreases, but the influence direction and significance of each variable are basically consistent with the results in Table 5. To sum up, the estimation results of the spatial econometric model in this paper are robust.
The cities in the CCEC are at different stages of industrial transformation and upgrading, and their capacities for GTI and economic development are different. Therefore, this paper takes the quantile of the mean value of GTIE of each city from 2006 to 2020 as the basis for classification, and classifies those with the mean value after the 75% quantile as high-efficiency cities, those with the mean value before the 25% quantile as low-efficiency cities, and the rest as medium-efficiency cities. Then, this paper uses the SDM and partial differential method to analyze the spatial heterogeneity of the influencing factors of GTIE in different types of cities.
The results in Table 8 show that, from the perspective of direct effect, EC is significantly positive in high-efficiency and low-efficiency cities, and its absolute value of coefficient in low-efficiency cities is large. EO is significantly positive only in medium-efficiency cities. GS is significantly positive only in low-efficiency cities. ER is significantly positive only in high-efficiency cities. It shows that ED significantly promotes GTIE in both high-efficiency and low-efficiency cities, and the positive effect is stronger in low-efficiency cities. EO promotes GTIE in medium-efficiency cities. GS promotes GTIE in low-efficiency cities. ER improves GTIE in high-efficiency cities. The economic growth and green development of high-efficiency cities are coordinated with each other, and the stronger economic strength enhances their “siphon effect” on green innovation resources, thus stimulating the vitality of urban GTI, and the “innovation compensation” effect of urban environmental regulation is more obvious. The breadth and depth of opening to the outside world in the CCEC is still low, and increasing foreign investment can improve GTIE in medium-efficiency cities to a certain extent. Low-efficiency cities are limited by the backward development of green industries, the cost burden of enterprises to carry out GTI relies on government financial support, and the incentive effect of economic development level on GTIE is more obvious.
From the perspective of indirect effect, EO is significantly negative in high-efficiency and medium-efficiency cities. GS is significantly positive only in high-efficiency cities. ER is significantly negative only in high-efficiency cities. It shows that EO of high-efficiency and medium-efficiency cities has significant spatial negative spillover effects on neighboring cities, GS of high-efficiency cities have significant spatial positive spillover effects on neighboring cities, and ER of high-efficiency cities have significant spatial negative spillover effects on neighboring cities. High-efficiency and medium-efficiency cities have obvious advantages in green innovation capacity and green industry clustering, and are more attractive to green and innovative foreign enterprises. The cities’ environmental standards are also more stringent, prompting high-polluting enterprises to move to neighboring cities with lower environmental standards. Neighboring cities are hindering their own GTI by taking on relatively low-end local spillover capacity and low-end international capacity. At the same time, in the process of industrial transformation and upgrading in high-efficiency cities, the government has played an important supporting role in building a basic green innovation platform and green innovation R&D investment, which has strengthened the green innovation links within the region, and the neighboring cities have improved GTIE under the radiation of high-efficiency cities.
The summary of results is presented in Table 9. The results in the first, second, and third parts show that the green innovation development in the CCEC has achieved remarkable results, and the implementation of a series of environmental protection policies and the development of green industries have promoted the urban GTIE (Hu et al., 2023). However, as the core cities in the CCEC, Chengdu and Chongqing have much higher GTIE than other cities, leading to the unbalanced spatial characteristics of urban GTIE. Due to the “siphoning effect” of high-efficiency cities, green innovation resources are excessively concentrated in Chongqing and Chengdu, while green innovation resources in low-efficiency cities are seriously lost and become “marginal cities” in the CCEC. Therefore, the “core-edge” structure of urban GTIE in the CCEC tends to be stable and shows positive spatial correlation.
The results in the fourth part show that there is a significant difference in the effect of each influencing factor on urban GTIE in the CCEC. ED can improve urban GTIE (Behera and Sethi, 2022), but as the CCEC is still in the stage of agglomeration development, it has not formed a synergistic mechanism of industrial chain division. The “green innovation dividend” of urban economic development mainly acts on its own GTIE improvement (Liu et al., 2023), and there is no spatial spillover effect on the GTIE of adjacent cities. The low breadth and depth of the opening up of the CCEC to the outside world leads to the insignificant effect of EO on urban GTIE. However, in the process of reform and opening up, in order to introduce more foreign capital, cities actively lower environmental standards and undertake low-end production capacity eliminated internationally, resulting in the problem of “environmental bottoming-out competition”, which led to the negative spatial spillover effect of EO on the GTIE of adjacent cities (Behera and Sethi, 2022). Government financial support is an important basis for enhancing the urban GTIE (Ronaldo and Suryanto, 2022), which can provide policy and financial support for green innovation projects of urban enterprises. However, the GS is focused on local enterprises, and there is no spatial spillover effect of GS on the GTIE of adjacent cities. ER promotes urban enterprises to innovate production technology and improve urban GTIE (Zhang et al., 2020); However, ER in cities forces polluting enterprises to move to adjacent cities with relatively lax environmental regulations, which leads to the negative spatial spillover effect of ER on the GTIE of adjacent cities.
The results in the fifth part show that there are significant differences in the effects of the influencing factors in cities with different efficiency types. The higher level of ED enhances the “siphon effect” of high-efficiency cities on green innovation resources and improves urban GTIE, and the “innovation compensation” effect of ER on GTIE in high-efficiency cities is also more significant. Although the breadth and depth of external opening in the CCEC are low, medium-efficiency cities are in the growth period of green innovation development, and EO still has a stimulating role in urban GTIE. The green innovation resources in low-efficiency cities are scarce, and the green technology research and development activities of urban enterprises mainly rely on GS. The government’s fiscal revenue is affected by the level of urban ED, and ED can improve the GTIE in low-efficiency cities. In addition, high-efficiency cities are rich in green innovation resources and industries, and they have a radiating effect on adjacent cities through inter-regional green innovation linkages. Therefore, the influencing factors have a more significant spatial spillover effect in high-efficiency cities.
In summary, the urban GTIE in the CCEC is showing a growth trend, but it needs to narrow the gap in GTIE between cities. Firstly, cities can improve the GTIE by improving ED, strengthening GS, and enhancing ER. Secondly, cities need to pay attention to the negative spatial spillover effects of ER and EO in the process of improving the GTIE, in order to reduce the negative impact on the GTIE of adjacent cities. Finally, cities with different efficiency types need to develop differentiated green innovation development strategies based on their own conditions.
Based on the panel data of 16 cities in the CCEC from 2006 to 2020, this paper reveals the spatiotemporal evolution characteristics and spatial leap paths of urban GTIE based on S-SBM and ESDA, and analyzes the spatial influence mechanism of urban GTIE and its spatial heterogeneity through a spatial econometric model. The core conclusions are as follows:
(1) The urban GTIE of CCEC has been improved in general, and it has gone through three stages of “growth period”, “shock period” and “revitalization period” in the time dimension. In the city dimension, the GTIE of each city has obvious spatial non-equilibrium characteristics, and the GTI development pattern with Chengdu and Chongqing as the core is gradually stabilized.
(2) The urban GTIE shows significant positive spatial correlation, and its clustering pattern is mainly of “high-efficiency type” and “low-efficiency type”. The results of spatial leapfrog analysis show that the leapfrog of urban GTIE has obvious low mobility characteristics, the spatial distribution pattern with Chengdu and Chongqing as the core has high stability, and the “core-edge” structure of green innovation development in the CCEC tends to be stable.
(3) ED and GS promote the urban GTIE, but neither of their spatial spillover effects is significant. ER has an incentive effect on the urban GTIE, and it has a negative spatial spillover effect, which has a significant hindering effect on the GTIE of neighboring cities. The effect of EO on the urban GTIE is not significant, but it has a hindering effect on the GTIE of neighboring cities.
(4) The spatial heterogeneity analysis shows that ED significantly improves the GTIE of high-efficiency and low-efficiency cities. EO has a positive effect on the GTIE of medium-efficiency cities. GS has a positive effect on the GTIE of low-efficiency cities. ER improves the GTIE of high-efficiency cities. EO has a negative spatial spillover effect in high-efficiency and medium-efficiency cities. GS and ER have spatial spillover effects in high-efficiency cities, with the former showing positive spatial spillover and the latter showing negative spatial spillover.
Green technological innovation, which takes into account both environmental protection and economic benefits, is essential route to achieve sustainable urban development. Therefore, governments should promote the development of green innovation in urban planning and construction.
Firstly, the spatial non-equilibrium of GTIE in CCEC is obvious, and the difference of GTIE among cities is large, so it is urgent to bring into play the comparative advantage of GTI. Chengdu and Chongqing, as the core cities in the economic circle, can build on their own advantages in technology and capital, enhance the radiation intensity to neighboring cities when gathering innovative talents, and release GTI dividends by jointly building innovative industrial parks and jointly creating green innovation centers to narrow the gradient difference in GTIE between cities. The low-efficiency cities of GTI represented by Nanchong, Suining, Deyang and Ya’an focus on promoting the green transformation of traditional industries and enhancing their own GTI capabilities based on their existing industrial base.
Secondly, the government’s financial support is an important guarantee to enhance GTIE of cities, but it is not enough to support GTI in neighboring cities. Therefore, local governments in cities need to continuously enhance their support for GTI. The government can encourage local enterprises to increase their R&D investment in GTI projects through fiscal and financial policy instruments, and set standards for environmental protection and energy consumption to promote production technology innovation. In addition, local governments should actively promote the construction of regional industrial innovation centers, engineering research centers and other innovation platforms, build green innovation collaboration mechanisms between cities, and continuously enhance the positive spillover effect of government financial support to neighboring cities.
Finally, the tendency of environmental “bottom-up competition” in the CCEC is beginning to emerge, and the environmental regulations of cities have a hindering effect on the GTI of neighboring cities. The environmental pollution problem has obvious externalities, and it must rely on the government to intervene forcibly, and the government’s environmental policy plays an essential role in the environmental pollution management of the region. On the one hand, the local government should formulate a more guiding and effective environmental policy for the current industrial situation of the city, take the highly polluting enterprises in the city as the target of treatment, promote the enterprises to complete the improvement and upgrading of green technology in an orderly manner, and realize the win-win situation of environmental protection and GTI. On the other hand, cities in the economic circle should coordinate to develop unified environmental protection standards and environmental warning and monitoring systems to prevent the transfer of low-end production capacity to cities with low environmental regulation intensity, and build a green industrial system covering the whole area of the economic circle.
There are some limitations in this paper. Firstly, due to the unavailability of county data in Chongqing, this paper includes Chongqing as a city in the scope of research and does not exclude the county areas belonging to Chongqing that are not included in the CCEC, which to a certain extent affects the relevance of the findings of this paper to promote the sustainable development of the CCEC. Secondly, due to the untimely update of indicator data at the urban level of the CCEC, this paper only studies the spatiotemporal characteristics of urban GTIE in the CCEC from 2006 to 2020, which leads to a lack of realistic description of the development status of urban GTIE in the CCEC. Finally, this paper discusses the factors affecting the urban GTIE as much as possible. However, as a demonstration area for China’s green transformation and development, the CCEC is in a constantly changing state of GTIE. Therefore, the factors affecting the urban GTIE in the CCEC are also subject to new changes, and this paper needs to focus on other factors that may have an impact on the urban GTIE.
Future research on the urban GTIE needs to be continuously improved. On the one hand, we can combine data mining and text analysis in our future research to collect data on relevant indicators of urban GTIE in the CCEC, so as to expand the research area and time range of the article and improve the representativeness and realistic guidance of the research conclusions. On the other hand, we will continue to pay attention to the new characteristics and changes of urban GTIE in the CCEC, and explore other factors that may affect urban GTIE in order to enrich the research content of the article.
The raw data supporting the conclusions of this article will be made available by the authors, without undue reservation.
SD and YW contributed to conception and design of the study. SD organized the database and performed the statistical analysis. SD wrote the first draft of the manuscript. YW wrote sections of the manuscript. Both authors contributed to manuscript revision, read, and approved the submitted version.
This research was funded by National Natural Science Foundation of China, grant number 72073045.
The authors declare that the research was conducted in the absence of any commercial or financial relationships that could be construed as a potential conflict of interest.
All claims expressed in this article are solely those of the authors and do not necessarily represent those of their affiliated organizations, or those of the publisher, the editors and the reviewers. Any product that may be evaluated in this article, or claim that may be made by its manufacturer, is not guaranteed or endorsed by the publisher.
Ahmed K. (2020). Environmental policy stringency, related technological change and emissions inventory in 20 OECD countries. J. Environ. Manage. 274, 111209. doi: 10.1016/j.jenvman.2020.111209
Ambec S., Barla P. (2002). A theoretical foundation of the Porter hypothesis. Economics Lett. 75 (3), 355–360. doi: 10.1016/S0165-1765(02)00005-8
Amore M. D., Bennedsen M. (2016). Corporate governance and green innovation. J. Environ. Economics Manage. 75, 54–72. doi: 10.1016/j.jeem.2015.11.003
Behera P., Sethi N. (2022). Nexus between environment regulation, FDI, and green technology innovation in OECD countries. Environ. Sci. pollut. Res. 29 (35), 52940–52953. doi: 10.1007/s11356-022-19458-7
Braun E., Wield D. (1994). Regulation as a means for the social control of technology. Technol. Anal. Strategic Manage. 6 (3), 259–272. doi: 10.1080/09537329408524171
Cao X., Yu J. (2015). Regional innovation efficiency in China from the green low-carbon perspective. China Population Resour. Environ. 25 (5), 10–19. doi: 10.3969/j.issn.1002-2104.2015.05.002
Colwell S. R., Joshi A. W. (2013). Corporate ecological responsiveness: antecedent effects of institutional pressure and top management commitment and their impact on organizational performance. Business Strategy Environ. 22 (2), 73–91. doi: 10.1002/bse.732
Conrad K., Wastl D. (1995). The impact of environmental regulation on productivity in German industries. Empirical Economics 20 (4), 615–633. doi: 10.1007/BF01206060
Demirel P., Kesidou E. (2011). Stimulating different types of eco-innovation in the UK: Government policies and firm motivations. Ecol. Economics 70 (8), 1546–1557. doi: 10.1016/j.ecolecon.2011.03.019
Demirel P., Kesidou E. (2019). Sustainability-oriented capabilities for eco-innovation: Meeting the regulatory, technology, and market demands. Business Strategy Environ. 28 (5), 847–857. doi: 10.1002/bse.2286
Dong Z. Q., Wang H. (2019). Local-neighborhood effect of green technology of environmental regulation. China Ind. Economics 1, 100–118. doi: 10.19581/j.cnki.ciejournal.2019.01.006
Dong J. R., Zhang W. Q., Chen Y. K. (2021). The impact of environmental regulation tools and government support on green technological innovation. Ind. Economics Res. 3, 1–16. doi: 10.13269/j.cnki.ier.2021.03.001
Elhorst J. P. (2014). Spatial econometrics: from cross-sectional data to spatial panels. Physica-Verlag HD. 1, 37–86. doi: 10.1007/978-3-642-40340-8
Fan F., Lian H., Liu X., Wang X. (2021). Can environmental regulation promote urban green innovation Efficiency? An empirical study based on Chinese cities. J. Cleaner Production 287, 125060. doi: 10.1016/j.jclepro.2020.125060
Fan Q. L., Shao Y. F., Tang X. W. (2011). Research on the classification characteristics of regional environmental technology innovation capability: a case of China. China Population Resour. Environ. 21 (6), 31–36. doi: 10.3969/j.issn.1002-2104.2011.06.006
Fan D., Sun X. T. (2020). Environmental regulation, green technological innovation and green economic growth. China Population Resour. Environ. 30 (6), 105–115. doi: 10.12062/cpre.20200123
Fan J., Teo T. (2022). Will China’s R&D investment improve green innovation performance? An empirical study. Environ. Sci. pollut. Res. 29 (26), 39331–39344. doi: 10.1007/s11356-021-18464-5
Fang Z., Bai H., Bilan Y. (2020). Evaluation research of green innovation efficiency in China’s heavy polluting industries. Sustainability 12 (1), 146. doi: 10.3390/su12010146
Fu L., Yi Y., Wu T., Cheng R., Zhang Z. (2023). Do carbon emission trading scheme policies induce green technology innovation? New evidence from provincial green patents in China. Environ. Sci. Pollut. Res. 30 (5), 13342–13358. doi: 10.1007/s11356-022-22877-1
Hashimoto A., Haneda S. (2008). Measuring the change in R&D efficiency of the Japanese pharmaceutical industry. Res. Policy 37 (10), 1829–1836. doi: 10.1016/j.respol.2008.08.004
Hojnik J., Ruzzier M. (2016). The driving forces of process eco-innovation and its impact on performance: Insights from Slovenia. J. Cleaner Production 133, 812–825. doi: 10.1016/j.jclepro.2016.06.002
Hu J., Hu M. J., Zhang H. (2023). Has the construction of ecological civilization promoted green technology innovation? Environ. Technol. Innovation 29, 102960. doi: 10.1016/j.eti.2022.102960
Huang L., Wu C. Q. (2019). Industrial green development efficiency and spatial driven mechanism in cities of the Yangtze River Economic Belt. China Population Resour. Environ. 29 (8), 40–49. doi: 10.12062/cpre.20190320
Jiang T., Ji P., Shi Y., Ye Z., Jin Q. (2021). Efficiency assessment of green technology innovation of renewable energy enterprises in China: a dynamic data envelopment analysis considering undesirable output. Clean Technol. Environ. Policy 23, 1509–1519. doi: 10.1007/s10098-021-02044-9
Lesage J., Pace R. K. (2009). Introduction to spatial econometrics. US: CRC Press Taylor Francis Group 1, 25–207. doi: 10.1201/9781420064254
Li W. H. (2017). Spatial-temporal evolution and factors of industrial green technological innovation output in China's Provinces: an empirical study of 30 provinces’ data. J. Ind. Eng. Eng. Manage. 31 (2), 9–19. doi: 10.13587/j.cnki.jieem.2017.02.002
Liao B., Li L. (2023). Urban green innovation efficiency and its influential factors: The Chinese evidence. Environment Dev. Sustainability 25 (7), 6551–6573. doi: 10.1007/s10668-022-02316-4
Lin G. P., Long Z. H., Wu M. (2005). A spatial analysis of regional economic convergence in China:1978-2002. China Economic Q. 4 (S1), 71–86. Available at: https://kns.cnki.net/kcms2/article/abstract?v=3uoqIhG8C44YLTlOAiTRKgchrJ08w1e7F1IFNsBV5UvbxL8UKasSQ1lDdHfs6hFCExBb4Dizi155StVl8KWzBY2_HaWopM2S&uniplatform=NZKPT.
Liu K., Liu W., Wu J., Chen Z., Zhang W., Liu F. (2022). Spatial differences and influencing factors of urban water utilization efficiency in China. Front. Environ. Sci. 10. doi: 10.3389/fenvs.2022.890187
Liu F., Nan T., Wang X. (2022). Causes and evolution characteristics of green innovation efficiency loss: the perspective of factor mismatch under local government competition. Sustainability 14 (14), 8338. doi: 10.3390/su14148338
Liu K., Xue Y., Chen Z., Miao Y. (2023). The spatiotemporal evolution and influencing factors of urban green innovation in China. Sci. Total Environ. 857, 159426. doi: 10.1016/j.scitotenv.2022.159426
Luo L. W., Liang S. R. (2016). Green technology innovation efficiency and factor decomposition of China’s industrial enterprises. China Population Resour. Environ. 26 (9), 149–157. doi: 10.3969/j.issn.1002-2104.2016.09.018
Luo Y., Lu Z., Salman M., Song S. (2022). Impacts of heterogenous technological innovations on green productivity: An empirical study from 261 cities in China. J. Cleaner Production 334, 130241. doi: 10.1016/j.jclepro.2021.130241
Luo Y., Wang Q., Long X., Yan Z., Salman M., Wu C. (2023). Green innovation and SO2 emissions: Dynamic threshold effect of human capital. Business Strategy Environ. 32 (1), 499–515. doi: 10.1002/bse.3157
Lv Y. W., Xie Y. X., Lou X. J. (2019). Study on the convergence of China’s regional green innovation efficiency. Sci. Technol. Prog. Policy 36 (15), 37–42. doi: 10.6049/kjjbydc.2019020287
Mirata M., Emtairah T. (2005). Industrial symbiosis networks and the contribution to environmental innovation: The case of the Landskrona industrial symbiosis programme. J. cleaner production 13 (10-11), 993–1002. doi: 10.1016/j.jclepro.2004.12.010
Ouyang X., Li Q., Du K. (2020). How does environmental regulation promote technological innovations in the industrial sector? Evidence from Chinese provincial panel data. Energy Policy 139, 111310. doi: 10.1016/j.enpol.2020.111310
Peng H., Shen N., Ying H., Wang Q. (2021). Can environmental regulation directly promote green innovation behavior? based on situation of industrial agglomeration. J. Cleaner Production 314, 128044. doi: 10.1016/j.jclepro.2021.128044
Popp D. (2006). International innovation and diffusion of air pollution control technologies: the effects of NOx and SO2 regulation in the US, Japan and Germany. J. Environ. Economics Manage. 51 (1), 46–71. doi: 10.1016/j.jeem.2005.04.006
Qin X., Sun Y. (2019). Cross-regional comparative study on environmental–economic efficiency and driving forces behind efficiency improvement in China: a multistage perspective. Int. J. Environ. Res. Public Health 16 (7), 1160. doi: 10.3390/ijerph16071160
Rey S. J., Janikas M. V. (2006). Stars: space-time analysis of regional systems. Geographical Anal. 38 (1), 67–86. doi: 10.1111/j.0016-7363.2005.00675.x
Roh T., Lee K., Yang J. Y. (2021). How do intellectual property rights and government support drive a firm's green innovation? The mediating role of open innovation. J. Cleaner Production 317, 128422. doi: 10.1016/j.jclepro.2021.128422
Ronaldo R., Suryanto T. (2022). Green finance and sustainability development goals in Indonesian Fund Village. Resour. Policy 78, 102839. doi: 10.1016/j.resourpol.2022.102839
Schaarschmidt M. (2016). Frontline employees' participation in service innovation implementation: the role of perceived external reputation. Eur. Manage. J. 34 (5), 540–549. doi: 10.1016/j.emj.2016.02.005
Shao S., Luan R., Yang Z., Li C. (2016). Does directed technological change get greener: empirical evidence from Shanghai's industrial green development transformation. Ecol. Indic. 69, 758–770. doi: 10.1016/j.ecolind.2016.04.050
Shen T., Li D., Jin Y., Li J. (2022). Impact of environmental regulation on efficiency of green innovation in China. Atmosphere 13 (5), 767. doi: 10.3390/atmos13050767
Shi Y. L., Pan Z. Y. (2021). Spatial structure characteristics and spatial pattern optimization of Chengdu-Chongqing Economic Circle. Macroeconomic Manage. 7, 21–27. doi: 10.19709/j.cnki.11-3199/f.2021.07.007
Shu C., Zhou K. Z., Xiao Y., Gao S. X. (2016). How green management influences product innovation in China: the role of institutional benefits. J. Business Ethics 133 (3), 471–485. doi: 10.1007/s10551-014-2401-7
Stucki T. (2019). What hampers green product innovation: The effect of experience. Industry Innovation 26 (10), 1242–1270. doi: 10.1080/13662716.2019.1611417
Su Y., Fan Q. M. (2022). Renewable energy technology innovation, industrial structure upgrading and green development from the perspective of China's provinces. Technological Forecasting Soc. Change 180, 121727. doi: 10.1016/j.techfore.2022.121727
Sun Y. M., Chen S. M. (2021). The spatiotemporal evolutionary pattern and driving forces mechanism of green technology innovation efficiency in the Yangtze River Delta region. Geographical Res. 40 (10), 2743–2759. doi: 10.11821/dlyj020210102
Tone K. (2001). A slacks-based measure of efficiency in data envelopment analysis. Eur. J. Operational Res. 130 (3), 498–509. doi: 10.1016/S0377-2217(99)00407-5
Tone K. (2002). A slacks-based measure of super-efficiency in data envelopment analysis. Eur. J. Operational Res. 143 (1), 32–41. doi: 10.1016/S0377-2217(01)00324-1
Wang T., Ding Y., Gao K., Sun R., Wen C., Yan B. (2022). Toward sustainable development: Unleashing the mechanism among international technology spillover, institutional quality, and green innovation capability. Front. Psychol. 13. doi: 10.3389/fpsyg.2022.912355
Wang Q., Qu J., Wang B., Wang P., Yang T. (2019). Green technology innovation development in China in 1990–2015. Sci. Total Environ. 696, 134008. doi: 10.1016/j.scitotenv.2019.134008
Wang W., Yu B., Yan X., Yao X., Liu Y. (2017). Estimation of innovation’s green performance: A range-adjusted measure approach to assess the unified efficiency of China’s manufacturing industry. J. Cleaner Production 149, 919–924. doi: 10.1016/j.jclepro.2017.02.174
Wu X., Fan D. (2023). Spatial and temporal evolution characteristics of urban green innovation efficiency in Northeast China. Front. Energy Res. 11. doi: 10.3389/fenrg.2023.1167330
Wu W., Sheng L., Tang F., Zhang A., Liu J. (2021). A system dynamics model of green innovation and policy simulation with an application in Chinese manufacturing industry. Sustain. Production Consumption 28, 987–1005. doi: 10.1016/j.spc.2021.07.007
Xu X., Cui X., Zhang Y., Chen X., Li W. (2023). Carbon neutrality and green technology innovation efficiency in Chinese textile industry. J. Cleaner Production 395, 136453. doi: 10.1016/j.jclepro.2023.136453
Yang Z., Chen H., Du L., Lin C., Lu W. (2021). How does alliance-based government-university-industry foster cleantech innovation in a green innovation ecosystem? J. Cleaner Production 283, 124559. doi: 10.1016/j.jclepro.2020.124559
Yang H. C., Li L. S., Zhang F. M. (2020). High-tech industrial agglomeration and green technological innovation performance. Sci. Res. Manage. 41 (9), 99–112. doi: 10.19571/j.cnki.1000-2995.2020.09.010
Yang Z., Shao S., Yang L., Liu J. (2017). Differentiated effects of diversified technological sources on energy-saving technological progress: Empirical evidence from China's industrial sectors. Renewable Sustain. Energy Rev. 72, 1379–1388. doi: 10.1016/j.rser.2016.11.072
Zailani S., Govindan K., Iranmanesh M., Shaharudin M. R., Chong Y. S. (2015). Green innovation adoption in automotive supply chain: the Malaysian case. J. Cleaner Production 108, 1115–1122. doi: 10.1016/j.jclepro.2015.06.039
Zameer H., Yasmeen H. (2022). Green innovation and environmental awareness driven green purchase intentions. Marketing Intell. Plann. 40 (5), 624–638. doi: 10.1108/MIP-12-2021-0457
Zhang Y., An Y., Wang Y. (2023). Evaluation of green industry innovation efficiency based on three-stage DEA model: a case study of Chinese information technology industry. Sustainability 15 (8), 6368. doi: 10.3390/su15086368
Zhang C., Cheng X. W., Ma Y. Y. (2022). Research on the impact of green finance policy on regional green innovation-based on evidence from the pilot zones for green finance reform and innovation. Front. Environ. Sci. 10. doi: 10.3389/fenvs.2022.896661
Zhang M., Hong Y., Wang P., Zhu B. (2022). Impacts of environmental constraint target on green innovation efficiency: evidence from China. Sustain. Cities Soc. 83, 103973. doi: 10.1016/j.scs.2022.103973
Zhang J., Kang L., Li H., Ballesteros-Pérez P., Skitmore M., Zuo J. (2020). The impact of environmental regulations on urban green innovation efficiency: the case of Xi'an. Sustain. Cities Soc. 57, 102123. doi: 10.1016/j.scs.2020.102123
Zhao N., Liu X., Pan C., Wang C. (2021). The performance of green innovation: From an efficiency perspective. Socio-Economic Plann. Sci. 78, 101062. doi: 10.1016/j.seps.2021.101062
Keywords: urban ecological protection, green technology innovation efficiency, spatiotemporal evolution, driving factors, spatial spillover effect
Citation: Deng S and Wu Y (2023) Spatiotemporal evolution and driving factors of urban green technology innovation efficiency in the Chengdu-Chongqing Economic Circle of China. Front. Ecol. Evol. 11:1234374. doi: 10.3389/fevo.2023.1234374
Received: 04 June 2023; Accepted: 31 July 2023;
Published: 16 August 2023.
Edited by:
Xianyou Pan, Shanghai University of Electric Power, ChinaReviewed by:
Xiaobao Yu, Shanghai University of Electric Power, ChinaCopyright © 2023 Deng and Wu. This is an open-access article distributed under the terms of the Creative Commons Attribution License (CC BY). The use, distribution or reproduction in other forums is permitted, provided the original author(s) and the copyright owner(s) are credited and that the original publication in this journal is cited, in accordance with accepted academic practice. No use, distribution or reproduction is permitted which does not comply with these terms.
*Correspondence: Shicheng Deng, c2hpY2hlbmdkY25AMTI2LmNvbQ==
Disclaimer: All claims expressed in this article are solely those of the authors and do not necessarily represent those of their affiliated organizations, or those of the publisher, the editors and the reviewers. Any product that may be evaluated in this article or claim that may be made by its manufacturer is not guaranteed or endorsed by the publisher.
Research integrity at Frontiers
Learn more about the work of our research integrity team to safeguard the quality of each article we publish.