- 1College of Civil Engineering and Architecture, Guangxi University, Nanning, China
- 2State Key Laboratory of Water Resources and Hydropower Engineering Science, Wuhan University, Wuhan, China
- 3School of Computing and Engineering, University of West London, London, United Kingdom
- 4Department of Civil, Environmental and Geomatic Engineering, University College London, London, United Kingdom
The Middle Route of the South-to-North Water Diversion Project of China (MRSNWDPC), i.e., the longest inter-basin water diversion project (1,432 km) in the world, has delivered more than 60 billion m3 of water resources to North China and benefiting more than 100 million people since December 2014. However, the abnormal algal proliferation in the main canal under low nutrient background has seriously threatened the water quality safety of this mega project. In this research, 3 years of monitoring data matrix, including water temperature (WT), flow discharge (Q), flow velocity (V), dissolved oxygen (DO), and the algal cell density (ACD), from the main canal of the MRSNWDPC were analyzed. The nonlinear relationships were determined based on multiple regression models, and a composite risk analysis model was constructed by Latin hypercube sampling (LHS) method coupled with Vine Copula function. The impacts of different hydrological and environmental factors on algal proliferation were comprehensively analyzed by Bayesian theory. The results showed that the WT gradually decreased from upstream to downstream, with a narrow range of 16.6–17.4°C, and the annual average concentrations of DO showed a gradual increase from upstream to downstream. The flow velocity of MRSNWDPC had a tendency to increase year by year, and the maximum flow velocity exceeds 0.8 m/s upstream, midstream and downstream by 2018. The ACD accumulated along the main canal, and the annual average ACDs of downstream were the highest, ranging from 366.17 to 462.95 × 104 cells/L. The joint early-warning method considering both water temperature and flow velocity conditions is an effective way for algal proliferation risk warning management. When water temperatures of the upstream, midstream, and downstream were below 26, 26, and 23°C, respectively, the algal proliferation risk can be controlled under 50% by the flow velocity at 0.3 m/s; otherwise, the flow velocity needs to be regulated higher than 0.8 m/s. In order to keep the midstream and downstream avoid abnormal algal proliferation events (ACD ≥ 500 × 104 cells/L), the corresponding ACDs of the upstream and midstream need to be controlled lower than 319 × 104 cells/L and 470 × 104 cells/L, respectively. This study provides a scientific reference for the long-distance water diversion project’s algal control and environmental protection. The proposed coupling Vine Copula models can also be widely applied to multivariate risk analysis fields.
1. Introduction
With rapid population growth and economic development in recent decades, the water resource conflict between regional water supply and consumption has intensified and become an important global challenge (Cai et al., 2021; Chen et al., 2021). Inter-basin water diversion campaign has become one of the essential engineering measures to alleviate the uneven spatiotemporal distribution of water resources (Zhuang, 2016). Over 160 trans-basin water diversion projects have been built worldwide, for instance, the Middle Route of the South-to-North Water Diversion Project of China (MRSNWDPC), i.e., the world’s longest trans-basin water diversion project (1,432 km), which has delivered a total of more than 60 billion m3 of freshwater resources to northern China since December 2014, directly benefiting more than 100 million people.
Although inter-basin water diversion activities can bring significant economic, ecological, and social benefits (Zhao et al., 2017), such mega hydro projects are often controversial due to the complex anthropogenic imposed on the hydrology, water quality, and ecology of relevant areas (Yang et al., 2018; Dai et al., 2020). The above debate focuses on water quality safety management and the risk of water deterioration before and after project operations. Compared with natural riverine water bodies, despite using the long-distance open canal for water supply, the strict policies of waste discharge prohibition can still eliminate the adverse impact of point source pollution on water bodies, resulting in long-term low nutrient concentrations and low pollution loadings for water quality in the MRSNWDPC (Nong et al., 2020). However, non-point source pollution (e.g., atmospheric dry and wet deposition, endogenous release) may be a major input for nutrient accumulation (e.g., nitrogen and phosphorus) and water quality deterioration in water bodies where long-distance open canals as the primary engineering measure (Zeng et al., 2015; Huang et al., 2019), and the nutrient increase often leads to abnormal algal proliferation (Wasson et al., 2017). On the other hand, the complex algal life cycles (death and decay processes) accompanied by the ecotoxin release can easily become a significant risk factor affecting water quality safety in trans-basin diversion projects (Zhu J. et al., 2021), which has aroused widespread concern and research all over the world (Zhu Y. et al., 2021; Long et al., 2022). Some researchers found that the algal proliferation of the MRSNWDPC may be the main driving factor leading to the chemical oxygen demand increase (Wang C. et al., 2022), causing unacceptable odor and water quality degradation (Wang Y. et al., 2022). However, most of the relevant research is limited to the analysis of algae growth trends and environmental driving factors, and there is still a lack of specific research on how to control algal proliferation.
Abnormal algal proliferation, which marks the precursor of eutrophication, has been widely studied in low-flow velocity, high-depth water column bodies such as lakes and reservoirs (Zou et al., 2020; Li et al., 2021). A series of achievements have been reported regarding key limiting nutrient factors and ecological control measures in algal proliferation (Cheng et al., 2019; Brookfield et al., 2021). For instance, there has become a worldwide consensus that nutrient accumulation (primarily nitrogen and phosphorus) promotes algae growth and reproduction (Huo et al., 2019; Yan et al., 2021). However, early-warning and control strategies of eutrophication in riverine bodies often differ from those in lakes and reservoirs. Many studies pointed out that algae growth could strongly respond to the hydrological condition changes, and the flow velocity can also affect the spatiotemporal distribution of algal cell density (Liang et al., 2013). For instance, regulating flow velocity can lead to sediment resuspension, nutrient redistribution, and spectral composition changes in the water column, thus affecting light energy utilization and effectively inhibiting algal growth (Shen et al., 2021). Low water exchange rates can provide a stable environment for algal growth, thus affecting the migration, diffusion, and accumulation processes (Xin et al., 2019). Additionally, the high-velocity condition can somewhat inhibit the algae growth and even cover up the promotion effects of nutrient loading improvement (Tian et al., 2022). Therefore, it is more important for riverine bodies to comprehensively consider hydraulic regulation and nutrition control strategies to reverse the eutrophication problem efficiently. Since the flow velocity of water bodies in inter-basin water diversion projects can be regulated by sluices (Li et al., 2022), it is feasible to control algal proliferation by changing hydraulic conditions.
Worryingly, the abnormal algal proliferation in the inter-basin water diversion project is more complicated than in rivers. The hydraulic sluice regulation of those water supply projects makes the flow discharge and water level change more frequently than in rivers, speeding up the algal life cycle processes (Zhang et al., 2019). Despite some research claiming that the nutrients in the project’s water bodies were kept at a low level than natural rivers and would not cause algal blooms (Chen et al., 2022), abnormal algal proliferation continues to be observed in the projects (Cattaneo et al., 2013; Li et al., 2014). Previous studies have been limited to the effects of individual key or specific indicators on algae growth, which may hide the factual information about the multivariate risk of abnormal algal proliferation (Xin et al., 2020). The quantification and identification of multi-relationships are necessary to fully explore the real-world ecology-environment-hydrological nexuses (Zhang Y. et al., 2015; Zhao Q. et al., 2019). More deepening studying is needed considering the complex hydrodynamic conditions and low nutrition characteristics of the water environment in those projects (Jing et al., 2022).
Based on the above discussion and gap, the main objectives of this research are: (1) to take the Middle Route of the South-to-North Water Diversion Project of China (MRSNWDPC) as a case and explore the critical factors of algal proliferation in the inter-basin water diversion project under different hydraulic and water quality conditions, (2) to propose a multivariate risk early-warning model and management strategies of algal proliferation under different hydrological and water quality scenarios, (3) to quantify the non-linear effects of algal growth in different locations on the spatial distribution of downstream ecological risks. This study can provide a scientific basis and technical approach for understanding the relationship between planktonic algae and key hydrological and water quality indicators in long-distance water diversion projects, contributing to the adaptive early-warning policies and water quality management strategies for algal bloom risk in different water environments worldwide.
2. Study area and data collection
The Middle Route of the South-to-North Water Diversion Project of China (MRSNWDPC, 32°40′–39°58′ N, 111°42′–116°16′ E) originated from the Danjiangkou Reservoir, the largest artificial freshwater lake in Asia, and northward 1,432 km to Tuancheng Lake, Beijing, the capital of China, since December 2014. As the water quality security of MRSNWDPC is related to the human health of nearly 100 million people, the water quality is monitored by the Construction and Administration Bureau (CAB) of the Middle Route of the South-to-North Water Diversion Project of China as part of the national monitoring program. The CAB has a comprehensive quality assurance/quality control (QA/QC) system to ensure that the data obtained from the national monitoring program is reliable and of sufficient accuracy and precision.
Six representative water quality monitoring stations in the main canal were selected for this study (Figure 1 and Table 1). These stations were selected based on the availability of a full set of water quality indicator data and the coverage of algal cell changes throughout the important cities in the MRSNWDPC. Water samples were collected monthly from January 2016 to December 2018 by the CAB’s professional team according to the environmental quality standards for surface water (China, 2002a). All the sampling date are determined by the CAB and in a unified arrangement. According to the actual weather conditions of each station, the water samples were collected on sunny or cloudy days to minimize the interference of rainfall, strong wind, and runoff on the data and ensured the reliability of the data. All water quality data used in this study were obtained from the CAB with official permission. A data matrix, including algal cell density (ACD, cell/L), pH, dissolved oxygen (DO, mg/L), five-day biochemical oxygen demand (BOD5, mg/L), ammonia nitrogen (NH3-N, mg/L), total phosphorus (TP, mg/L), total nitrogen (TN, mg/L), fluoride ( , mg/L), water temperature (WT, °C), sulphate ( , mg/L), faecal coliform bacteria (F. coli, colonies/L), mercury (Hg, μg/L), arsenic (As, μg/L), copper (Cu, μg/L), zinc (Zn, μg/L), selenium (Se, μg/L), flow velocity (V, m/s), and flow discharge (Q, m3/s), was used for the first step of analysis. Since the water quality of the main canal maintained an excellent status (Nong et al., 2020; Tang et al., 2022), and most of the heavy metal elements and physicochemical indicators had narrow vary ranges, the WT, Q, V, DO, NH3-N, TP, and TN were selected as the basic indicators that may affect ACD in the main canal based on the pre-review (Song et al., 2018; Zhang et al., 2018; Gao et al., 2022). The ACD was obtained by collecting 1,000 mL of water samples at 0.5 m below the river center’s surface and adding 10–15 mL of Lugol’s iodine solution. The WT and DO were obtained by multi-parameter probe measurements in-situ. For NH3-N, TP, and TN, water samples were collected at the sampling station and taken in a cool box to the laboratory, where the NH3-N was determined by the spectrophotometric method with nano reagent, the TP was determined by the potassium persulfate digestion method, and the TN was determined by the potassium persulfate oxidation method (China, 2002b). The monitoring standards and sampling methods can be found in the Ministry of Ecology and Environment of China1 (in Chinese) and Wu et al. (2018).
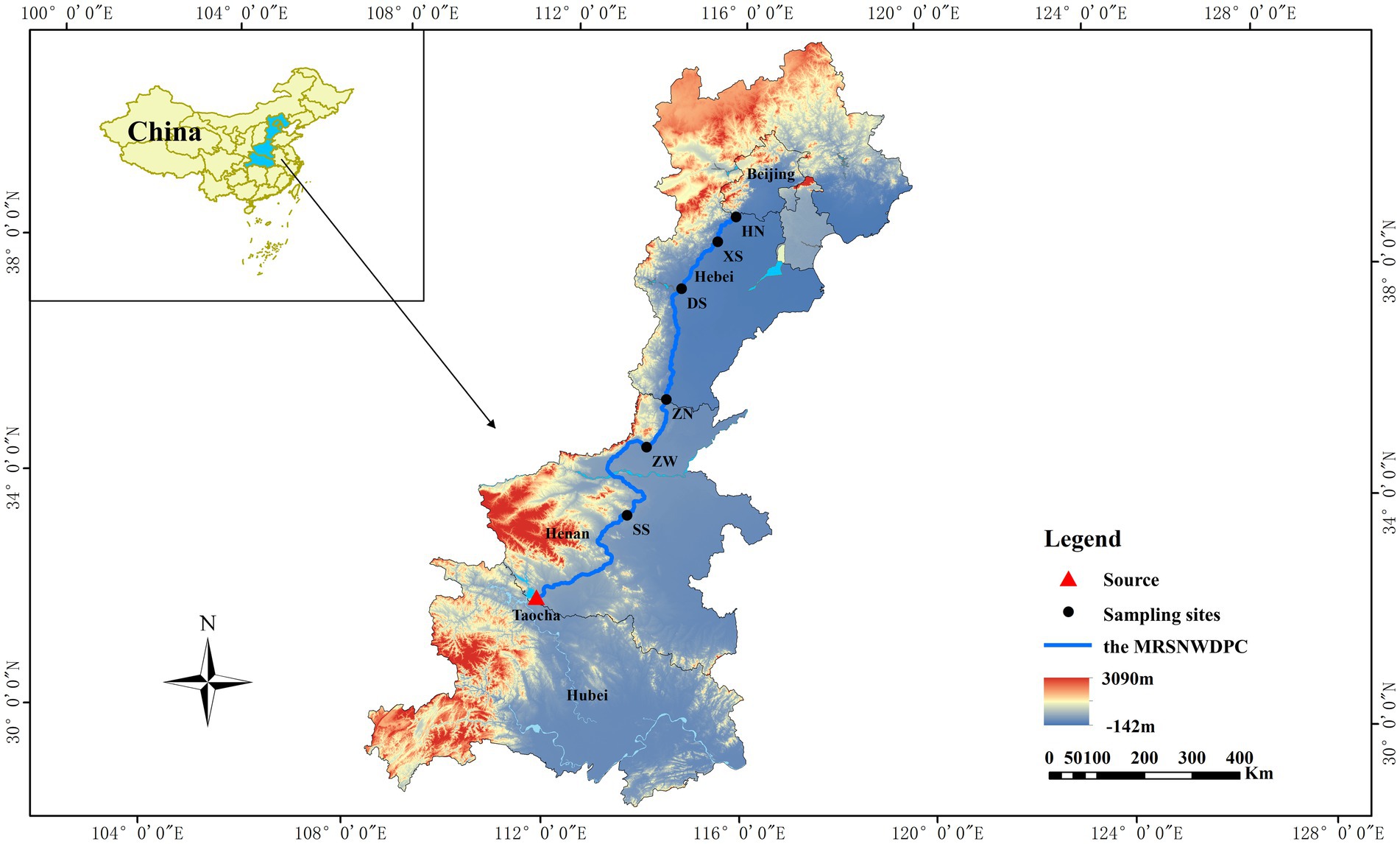
Figure 1. Location of the monitoring station of the MRSNWDPC in this study [Shahenan (SS); Zhengwan (ZW); Zhanghebei (ZN); Da’anshe (DS); Xiheshan (XS); Huinanzhuang (HN)].
3. Materials and methods
3.1. Vine Copula function
The Copula function is a classical tool to connect multivariate distribution with its univariate probability function (marginal distribution). One of the most important advantages is that it can effectively address the construction issues from marginal to joint distributions for multiple independent variables (Liu et al., 2021). Sklar (1959) first gave this famous theorem as follows.
For a d-dimensional random variable with continuous marginal distribution, thus the joint distribution can be expressed by:
Where is the corresponding joint cumulative distribution; is the marginal cumulative distribution of the random variable ; , where . The corresponding density function is (Wan and Li, 2019):
Where is the joint density distribution; is the marginal density distribution of the random variable , where ; and is the density of the Copula function. More details about the Copula can be found in Khozeymehnezhad and Nazeri-Tahroudi (2020) and Nazeri-Tahroudi et al. (2022). Our article mainly introduced and used the six typical Copula functions that were widely applied (Supplementary Table S1). Additionally, there are specific “rotations” structure in some Copula functions, and the corresponding density function transformation can be found in Table 2.
Traditional Copula functions are widely used to solve problems in two dimensionals or, at most, three dimensions, and the simulation results need improvement when solving problems in higher dimensions. To address the above issues, Bedford and Cooke (2001) developed the Vine Copula based on the concept of Regular vine (R-vine) as a graphical model of conditional dependence, where Canonical vine (C-vine) and Drawable vine (D-vine) are two unique forms in R-vine Copula (Liebscher, 2008). The principle of Pair-Copula construction (PCC) is to decompose n-dimensional multivariate density functions into binary Copula density functions, thus making it easier to model high-dimensional problems (Kraus and Czado, 2017). Among them, the Vine Copula structure includes three parts: nested tree , node , and edge . For the d-dimensional R-vine Copula (Dong et al., 2022), there are a set of trees ( ) with the structure as follows:
1. T1 has nodes N1 = 1, 2, ⋯, d and edges E1.
2. For i =2, ⋯, d–1, the tree Ti has nodes Ni = Ei–1.
3. Two edges in tree are associated to tree if they co-use a node in the tree.
A structural diagram of the Vine Copula can be found in Brechmann and Schepsmeier (2013), while the equations of the probability distributions for the Vine Copula are shown in Eq. (3)–(5).
The expression of R-vine Copula can be defined as follows:
The expression of C-vine Copula can be defined by:
The expression of D-vine Copula can be expressed as:
Where is the edge density function, denotes trees, and denotes edges.
The corresponding construction process of the Vine Copula model took the following steps:
1. The Kendell coefficient τ between two variables is calculated, and the structure of Tree 1 in Vine Copula is determined using the maximum spanning tree with τ as the weight.
2. The optimal Pair-Copula among the variables in Tree 1 is determined by the Akaike information criterion (AIC), and the parameter values of the corresponding Copula are calculated using maximum likelihood method.
3. The observed values of Tree 2 are obtained from the parameter values of Tree 1 and Eq. (6), and the optimal Copula of Tree 2 variables is obtained by repeating the previous step.
where is the parameter set of the Copula function and denotes the binary Copula distribution function.
4. Repeat steps 2 and 3 until the final layer of the tree is calculated.
5. The Vine Copula parameters were corrected using the maximum likelihood method.
3.2. Latin hypercube sampling
The Latin Hypercube Sampling (LHS) is a method of approximate random sampling from multivariate parametric distributions, which is a stratified sampling technique widely used to generate random samples of parametric values from multi-dimensional distributions (Zhao Y. et al., 2019). The most important advantages of the LHS are the effect of filtering the variance associated with the additive component of the transformation, and the ability to provide uniform solution space coverage and stable output with a smaller number of simulations (Huang et al., 2018). Since the LHS can make full use of the abundant computational resources to simulate a large number of representative samples with strong correlation and cover the whole search space (Feng et al., 2020), a coupling method was proposed to combine the LHS and the Vine Copula model. The model can better handle the dependencies between multiple factors relative to the common linear correlation coefficients (Huang et al., 2020), and compared with machine learning methods such as decision trees, this model will not have the phenomenon of over-fitting and ignoring attribute correlation (Schepsmeier, 2019). In this study, the dependency structure of indicators in each canal section is constructed by the Vine Copula model. Then the data set is expanded by coupling LHS with the Vine Copula model and generating many pseudo-random numbers that meet the high-dimensional dependence of multiple factors, which are correlated with variable correlations in the risk analysis reliably fitted. Further details on LHS are available at (Shields and Zhang, 2016). In this paper, the Vine Copula model with n-dimensional variables was established and an matrix follow the uniform distribution of [0,1] generated by the LHS were obtained, where was defined:
Where in each column of data, represents the marginal distribution function value of . After the LHS simulation, the algae risk of samples was analyzed according to the Bayesian theory (Fan et al., 2020), and the detailed modelling processes are shown in Figure 2.
4. Results
4.1. Water quality variation and key water quality factors
4.1.1. Water quality variation characteristic
The statistical summary of the ACD, hydrological factors (Q and V), and water quality indicators (DO, TP, TN, NH3-N, and WT) of different sections of the MRSNWDPC during the monitoring period can be found in Table 3.
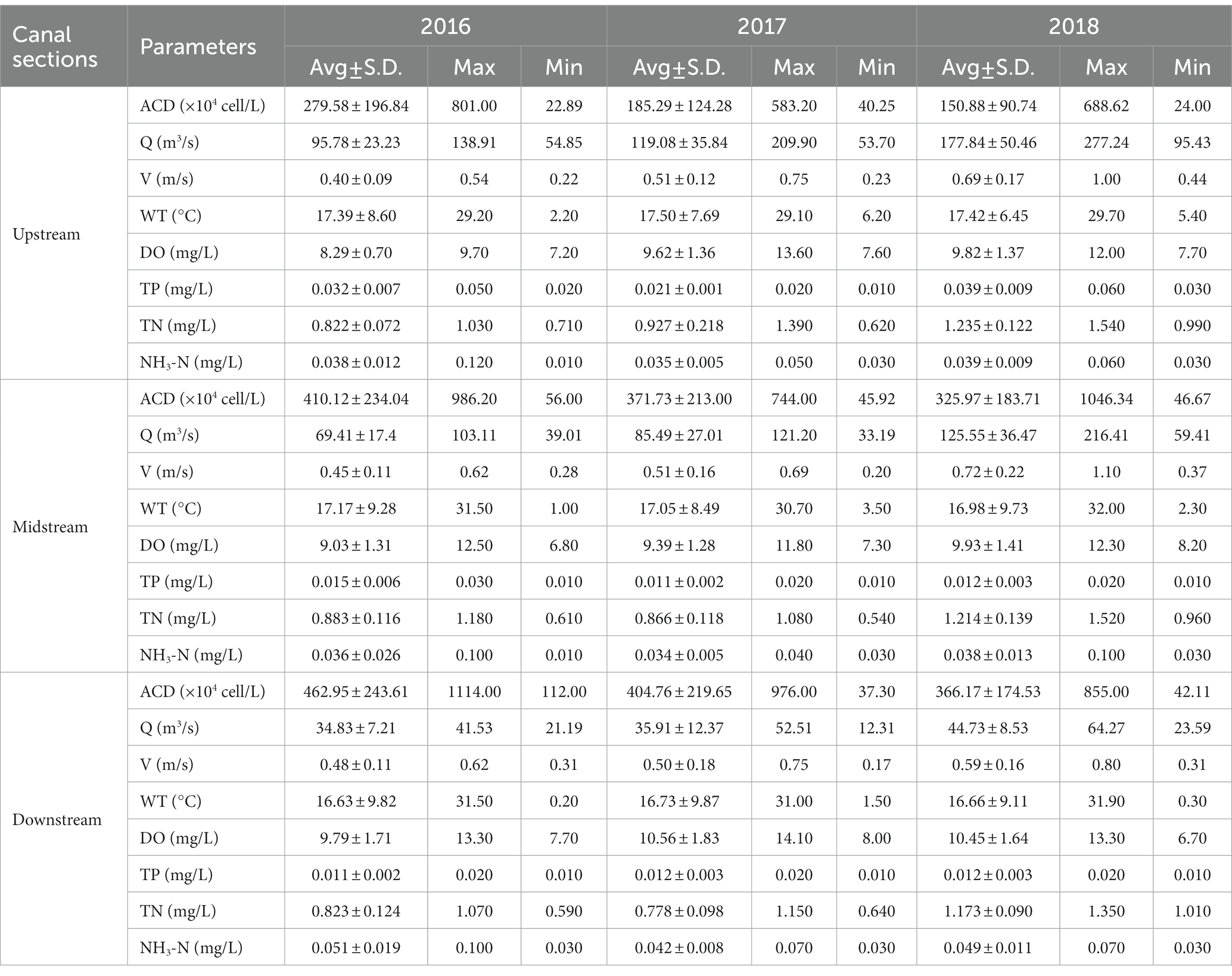
Table 3. Analysis of water quality parameters in each canal section of the MRSNWDPC from 2016 to 2018 (Avg., average; S.D., standard deviation).
Temporally, the annual average values of the ACD in all sections showed a decreasing trend yearly; spatially, the ACD gradually increased from upstream to downstream along the main canal, and the annual average ACDs of downstream were the highest, ranging from 366.17 to 462.95 × 104 cells/L. There are no significant differences in flow velocity among all sections, which means that although the flow discharge decreased along the canal, the adjustability of the flow velocity can be kept consistent. In addition, the velocity of each section tends to increase year by year, and the maximum velocity will exceed 0.8 m/s by 2018. The WT gradually decreased from upstream to downstream, with a narrow range of 16.6–17.4°C. The annual average concentrations of DO showed a gradual increase from upstream to downstream, while the concentrations of TN have opposite trends. The TP concentration in the upstream is higher than that in the midstream and downstream, and there is no significant spatial difference between the TP concentration in the midstream and downstream. In addition, the average annual concentration of NH3-N in each canal section is stable, and there is no obvious spatial difference. The lowest TN/TP ratios were in the upstream section (ranged from 25.69 to 44.14), and the middle and downstream (ranged from 58.86 to 101.16, and 64.83 to 97.75, respectively) were significantly higher than those. The nutrient loadings were kept low, with ammonia nitrogen (NH3-N) ranging from 0.034 to 0.051 mg/L and TP ranging from 0.011 to 0.039 mg/L, respectively. In general, all water quality indicators met the Class I standard, except for TP at the upstream section, which met the Chinese Class II standard, indicating that the MRSNWDPC has excellent water quality conditions and low nutrient loading. In addition, TN (0.778–1.235 mg/L) will not be included in the MRSNWDPC for study according to the Chinese Environmental Quality Standards for Surface Water (China, 2002a).
4.1.2. Relationship between key factors and algal proliferation
The result of the nonlinear relationships between the ACD and the key hydrological and water quality indicators in each canal section based on the multiple regression model can be found in Figure 3, where the R2 (coefficient of determination) is calculated by the ordinary least squares (OLS).
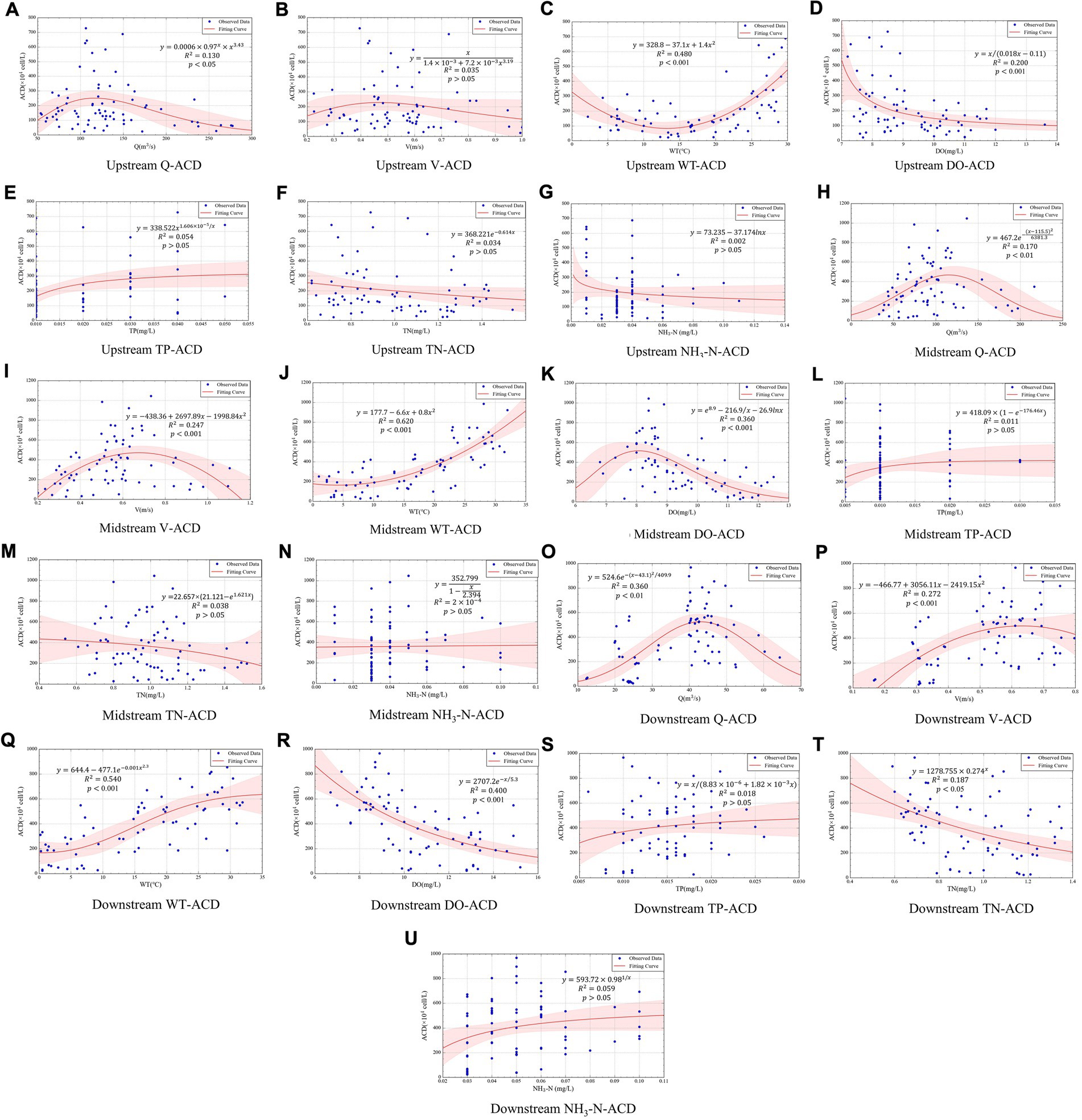
Figure 3. Response of algal cell density to water quality and hydrological factors variations [Upstream Q-ACD (A), Upstream V-ACD (B), Upstream WT-ACD (C), Upstream DO-ACD (D), Upstream TP-ACD (E), Upstream TN-ACD (F), Upstream NH3-N-ACD (G), Midstream Q-ACD (H), Midstream V-ACD (I), Midstream WT-ACD (J), Midstream DO-ACD (K), Midstream TP-ACD (L), Midstream TN-ACD (M), Midstream NH3-N-ACD (N), Downstream Q-ACD (O), Downstream V-ACD (P), Downstream WT-ACD (Q), Downstream DO-ACD (R), Downstream TP-ACD (S), Downstream TN-ACD (T), Downstream NH3-N-ACD (U)].
The ACD showed a significantly increased trend first and then decreased concerning the increase of Q and V, except for the flow velocity upstream (Q: R2 = 0.130 to 0.360, p < 0.05; V: R2 = 0.247 to 0.272, p < 0.001). When the WT<13.6°C, the ACD upstream showed a decreased response to the increase of WT, while the ACD of the midstream and downstream showed a simultaneous increase to the WT (R2 ≥ 0.480, p < 0.001). The ACD has a negative response to DO concentrations raised both in upstream and downstream (R2 = 0.200 and 0.400, p < 0.001), while the ACD first increased and then decreased in the midstream (R2 = 0.360, p < 0.001). Except for the TN downstream, the ACD showed no significant response to the variations of TN, TP, and NH3-N (R2 ≤ 0.059, p > 0.05). Based on the above results, the Q, V, WT, and DO were finally determined as the key factors constructing the multivariate risk model with ACD.
4.2. Multivariate risk model construction
The three-dimensional (3D), four-dimensional (4D), and five-dimensional (5D) Vine Copula models of ACD and key water quality and hydrological variables (Q, V, WT, and DO) were constructed, respectively. The data set of the Vine Copula model was simulated by the LHS, and the risk of ACD under different environmental conditions was analysed based on the Bayesian theory. Previous studies have reported that the limited influence of DO on algae proliferation in the MRSNWDPC, and the flow velocity has strong relationship with flow discharge (Zhang et al., 2022). Considering the actual project operation, this study will mainly explore the influence of WT and V on the ACD variations.
4.2.1. Determination of marginal distribution
Nine widely used distribution functions (Normal distribution, Lognormal distribution, Extreme Value distribution, Generalized Extreme Value distribution, Logistic distribution, Pearson III distribution, Weibull distribution, Gamma distribution, and Exponential distribution) were selected to fit the data, and the maximum likelihood method was used to evaluate the parameters. The Kolmogorov–Smirnov (K–S) test was used to test the good fitting of marginal function, and the appropriate marginal distribution was selected by the AIC. Table 4 shows the results of selected marginal functions and the corresponding parameters. It can be seen that both the Generalized Extreme Value distribution and Pearson III distribution were selected 4 times, and the optimal marginal functions of different indicators differed at different locations, indicating the importance of selecting the marginal distribution function by comparison step.
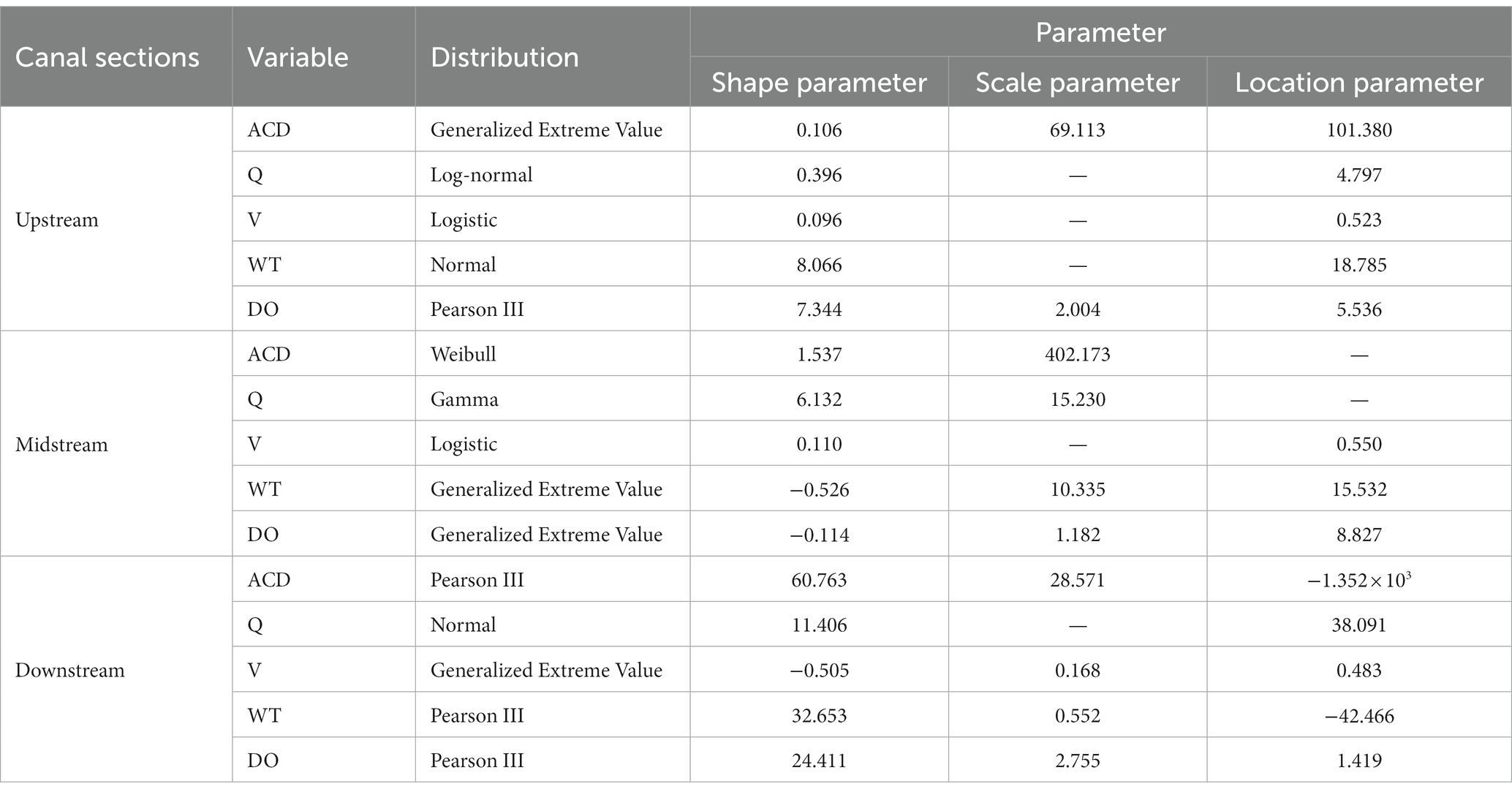
Table 4. Optimal marginal distribution selection of indicators in each canal section of the MRSNWDPC in this study.
4.2.2. Vine Copula model construction
The optimal Vine Copula models for the upstream, midstream, and downstream of MRSNWDPC in different dimensions are shown in Table 5. The C-vine, D-vine, and R-vine were all applied to construct joint distributions based on the AIC, Bayesian information criterions (BIC), and log-likelihood (L-lik). It can be seen in Table 5 that the optimal Vine Copula models of all canal sections selected the R-vine structure. The Vine Copula structure with the lowest AIC and BIC and highest L-lik can be selected as the optimal model (Tahroudi et al., 2022), details can be found in Supplementary Tables S2–S4, and the corresponding Vine Copula structure can be found in Supplementary Figures S1–S3.
4.2.3. Risk analysis of abnormal algal proliferation
Based on the optimal Vine Copula structure and LHS obtained in different dimensions, a set of data sets , , and with the size of 1,000,000 × M (M = 3, 4, and 5) were simulated for each canal section, respectively. The risk probability corresponding to ACD variation for each section under different WT and V conditions was calculated according to Eq. (8).
Where denotes the number of samples of and ; n denotes the number of samples satisfying both and
It can be seen from Supplementary Figures S4–S9 that when the water temperature is within a specific interval, the probability of ACD proliferation of the upstream and midstream will decrease with the increased flow velocity. For instance, when the water temperature of upstream between 26 and 29°C, the ACD had a 61.4% probability of exceeding 300 × 104 cells/L under the V under 0.3 to 0.4 m/s condition, when the V raised to 0.7 to 0.8 m/s, the corresponding rate reduced to 30.9%. However, in the downstream section, the ACD variations showed weak relationships with flow velocity changes. It can be observed that the flow velocity changed from 0.3 to 0.6 m/s had almost no effect on the probability of exceeding a specific ACD value under the same water temperature condition.
4.3. Spatial relationships of ACDs in different canal sections
The histogram, scatter plot, and Kendall coefficient τ after normalizing the ACD data of each channel section were presented in Figure 4. It can be seen that the ACDs did not show normal distributions in each section. There were strong correlations between the ACD of adjacent sections, with the Kendall coefficient τ (p ≤ 0.05) of “up to midstream” and “mid to downstream” were 0.68 and 0.87, respectively.
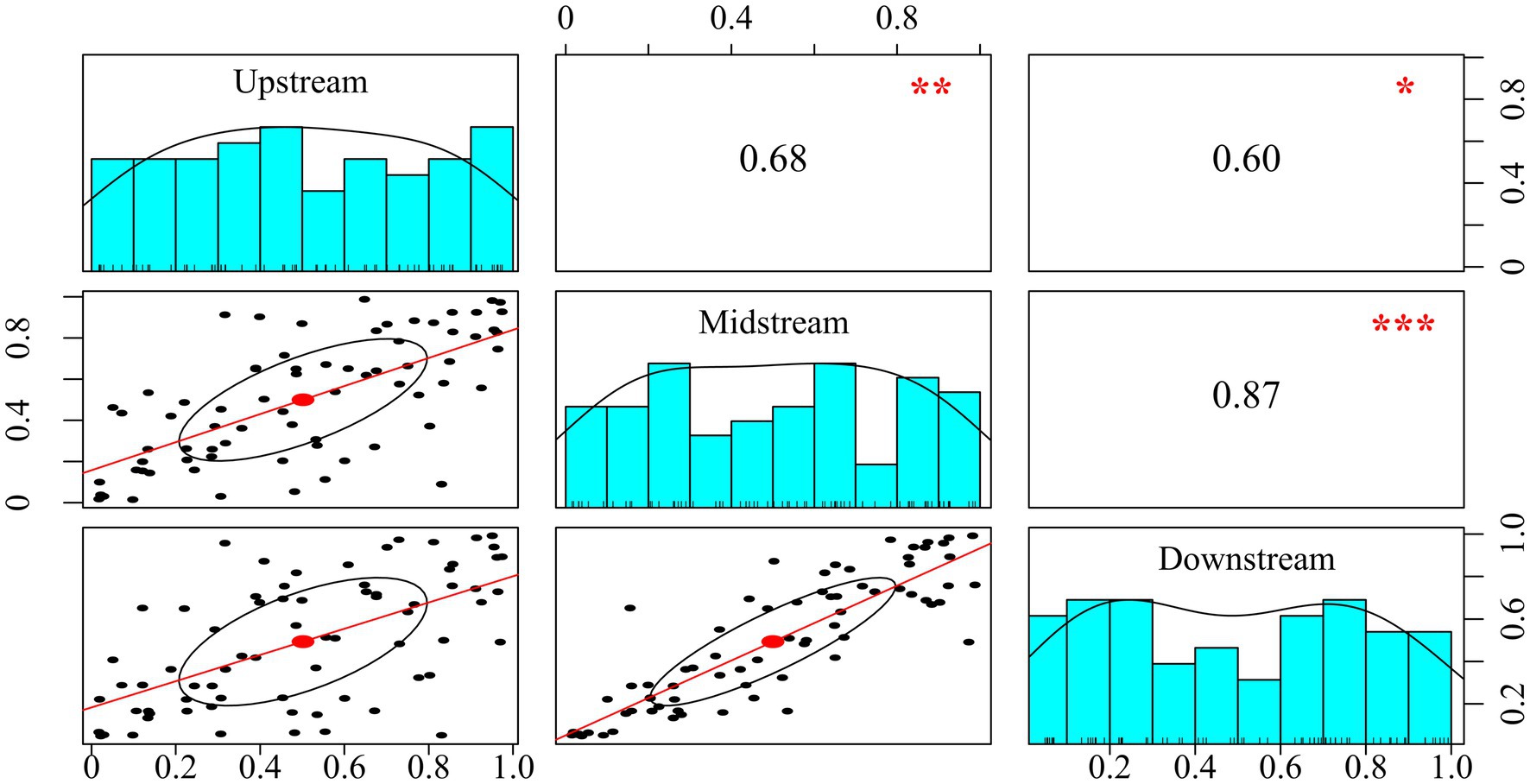
Figure 4. Spatial relationships of ACDs in different canal sections (*p ≤ 0.05; **p ≤ 0.01; ***p ≤ 0.001).
The responses of the ACD between different canal sections were analyzed based on binary Copula models (Supplementary Table S5), and the conditional probabilities of downstream ACD variation under the upstream ACD change were calculated based on the Bayesian theory according to Eq. (9).
Considering the ACD variation range of each section, the conditional probability of ACDMid (ACDDown) changes under the different ACDUp (ACDMid) is shown in Figure 5. With the ACD increased in upstream, the risk probability of ACDMid increased simultaneously, where the ACDMid only had a 2.48% probability exceeding of 500 × 104 cells/L when the ACDUp ≤ 50 × 104 cells/L. However, when the raised to 350–400 × 104 cells/L, that risk probability was higher than 62%. Similar phenomena can also be observed in the mid to downstream section. From the spatial prospect, the ACD control using the flow velocity regulation strategy should be carried out in the upstream and middle sections, which can achieve efficiency.
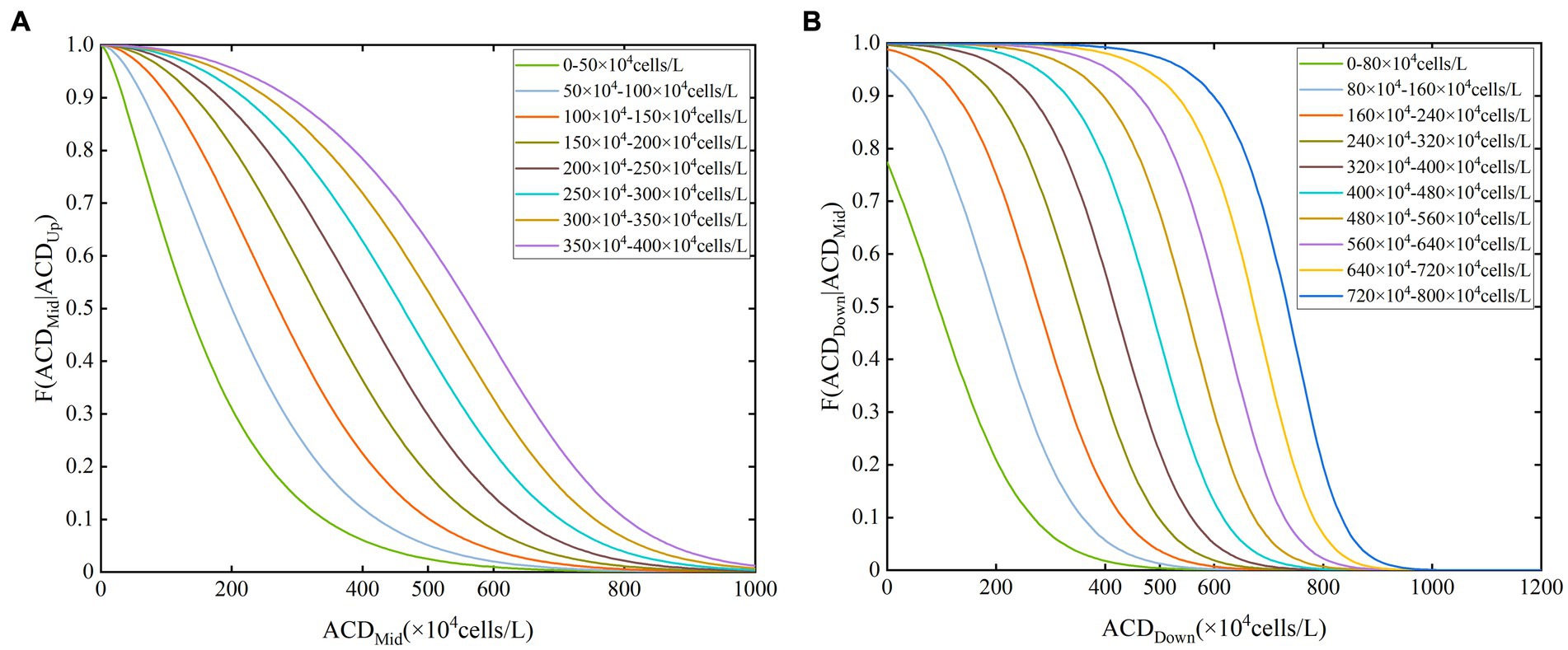
Figure 5. The conditional probability of ACD variations on adjacent canal sections [(A,B) are defined as the effects of ACDUp on ACDMid and ACDMid on ACDDown, respectively].
5. Discussion
5.1. Key driving factors of ACD variations
During the study period, the ACD in MRSNWDPC was maintained at the level of 106 cells/L, in which the dominant algae composition was of Chlorophyta, Cyanophyta, and Bacillariophyta (diatoms). Chlorophyta and Cyanophyta were the dominant species in summer, and the Cyanophyta and Bacillariophyta were the dominant species in the rest of the seasons (Zhang et al., 2021c). Previous studies have shown that water temperature is one of the critical factors that can stimulate algae growth (Zhao et al., 2018; Mei et al., 2022), and the growth rate of Chlorophyta and Cyanophyta can reach the peak when surface water temperature within 25–35°C (Huisman et al., 2018). This study also showed similar results (Figure 6), reporting that the high risk of algal proliferation happened when the upstream, midstream, and downstream had the WT higher than 26, 26, and 23°C, respectively. Those phenomena can be attributed to the dominant species Chlorophyta and Cyanophyta which can have better reproductive ability in higher water temperature environments (Li R. et al., 2015). Considering the seasonal characteristic of the MRSNWDPC (Zhang et al., 2021a), the prevention and control management of ACD in the canal should be strengthened in summer and autumn.
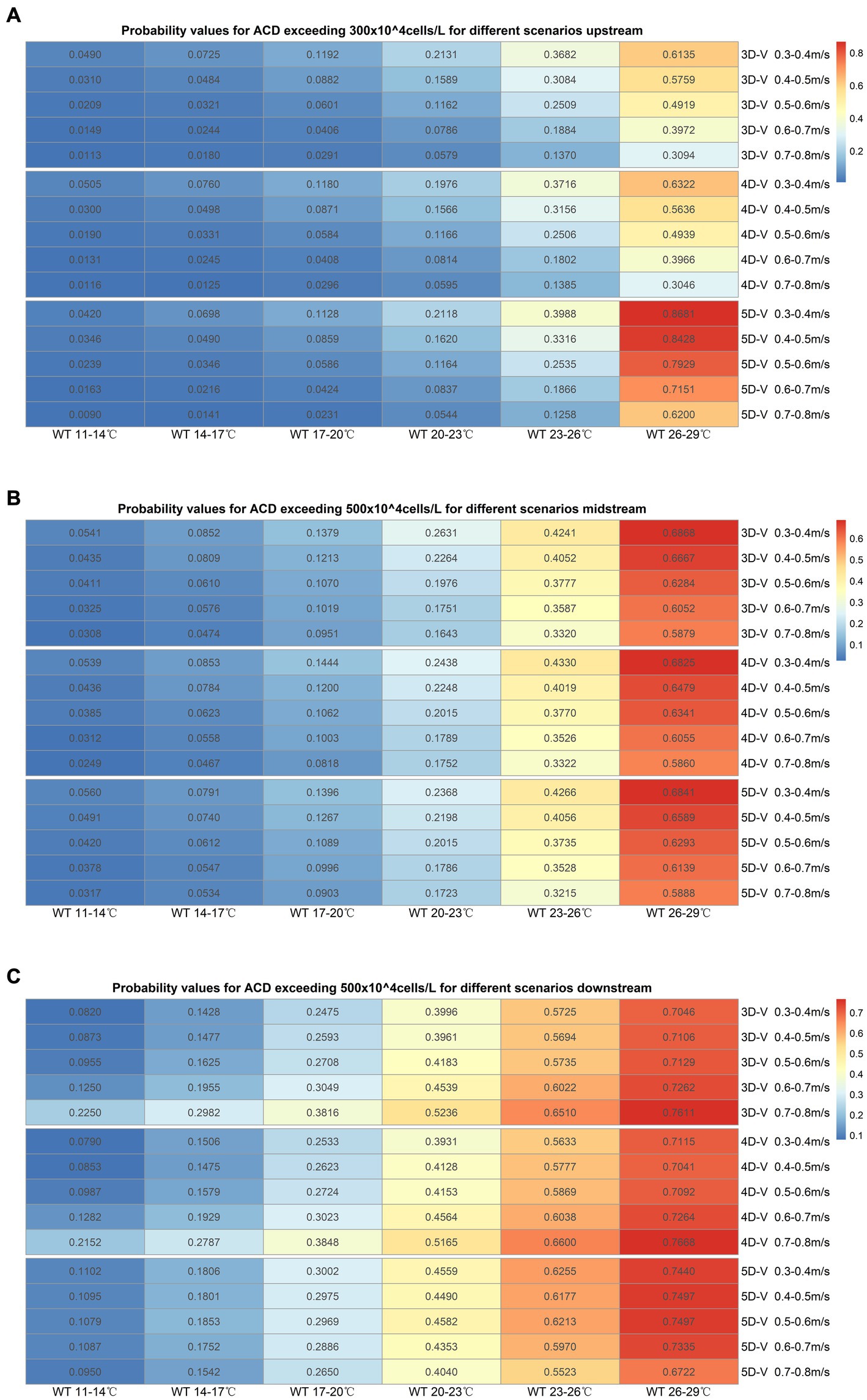
Figure 6. Early-warning thresholds for algal proliferation control under different conditions at upstream (A), midstream (B), and downstream (C).
Flow velocity is an important factor affecting algal habitats (Li Z. et al., 2015). Under suitable velocity conditions, the algae growth rate will be promoted, while high flow velocity can also inhibit algal growth (Zhou et al., 2018). The flow conditions change causes the sediment to resuspend, leading to the nutrient re-distribution and spectrum composition variation. The above processes affect light utilization and the nutrient absorption of algae growth. Additionally, under high-velocity conditions, some algae cells will be damaged by the impact of water flow and accelerate to extinction process (Zhang H. et al., 2015). For the upstream section of the MRSNWDPC, the ACD proliferation risk can be reduced by regulating the flow velocity above 0.6 m/s when the water temperature was higher than 26°C, which may be due to the high flow velocity inhibiting the growth of algae cells and nutrient uptake, and reducing the photosynthetic pigment content and PSII (photosystem II) activity (Li et al., 2022). However, it can be seen that the flow velocity changes between 0.3–0.7 m/s in the midstream and downstream have little effect on the ACD variations (Supplementary Figures S7–S12), which may be due to the increase of algae in the midstream and downstream, which makes a large number of algae adhere to the bend of the canal and the side walls (Hu et al., 2022), resulting in the regulation of algae by relatively low flow velocity showed no significant effects. Therefore, the flow velocity needs to be pre-regulated for the ACD control before the WT exceeding 26 and 23°C, respectively. When the water temperature exceeds the warning threshold, increasing the flow velocity cannot achieve satisfactory results in the middle and downstream sections.
5.2. Algal-controlled threshold for different sections
The early-warning thresholds of algal cell density in the upstream, midstream, and downstream sections of the MRSNWDPC were set to 300 × 104 cells/L, 500 × 104 cells/L, and 500 × 104 cells/L, respectively, according to the actual situation of the project and previous study (Zhang et al., 2022). The event in which the ACD has a 50% probability of exceeding the threshold was defined as “algal proliferation risk.” In this research, it can be seen that the ACD in the relative upstream location can significantly affect the ACD of the downside. When the ACDUp < 182 × 104 cells/L, the ACDMid has a 20% probability exceeding 500 × 104 cells/L. According to the binary Copula model calculation, the ACDUp = 319 × 104 cells/L and ACDMid = 470 × 104 cells/L were selected as the early-warning thresholds for the upstream and midstream section, respectively, which were about ±6% differences from previous study (300 × 104 cells/L and 500 × 104 cells/L, respectively), reflecting that the algal controlled threshold for ACDUp and ACDMid determined by the Copula model was appropriate. For the practical control and management of the project, the early-warning thresholds of ACD in the upstream, midstream, and downstream sections of the MRSNWDPC can be proved reasonably set to 300 × 104 cells/L, 500 × 104 cells/L, and 500 × 104 cells/L, respectively. Additionally, it was found that the average concentration of ACD in summer often exceeded 500 × 104 cells/L in downstream (Nong et al., 2021). Therefore, relevant management can be conducted by adjusting flow velocity higher than 0.8 m/s in the upstream section before summer (Atazadeh et al., 2021).
5.3. Model uncertainty
This research introduced the Vine Copula model to construct multivariate joint distributions for algal proliferation risk analysis. The effects of water temperature and flow velocity on the ACD variations in different dimensions were analyzed. There were significant differences between the results of the binary Copula models (Zhang et al., 2021b) and this study, which may be due to the neglect of the multivariate correlations in the previous research. Therefore, multidimensional joint risk modelling should be conducted by considering the comprehensive effect of multiple factors simultaneously to improve the realism of the simulation results. It was found that the fitting results of R-vine Copula were better than that of C-vine and D-vine Copula, which may be attributed to the fact that the R-vine Copula function can better maintain the statistical characteristics of Kendall and Spearman correlation coefficients of the original sequence (Zeng et al., 2022). The proposed coupling method using the LHS and the Vine Copula model showed robustness and comparable ability. The simulation results were consistent with the Monte Carlo simulation by Zhang et al. (2022). However, when analyzing the probability under special conditions such as the ACD > 0 cells/L (probability = 100%), the results of this study were more in line with the actual situation. Additionally, the risk intervals of the ACDs showed little difference compared with the Monte Carlo simulation (Zhang et al., 2022), which can be attributed to the uniform sampling characteristic of the LHS method (Zhang W. et al., 2015). To sum up, the proposed coupling method in this research can effectively reduce sampling and simulation uncertainty and can be generalized and applied to more multi-dependent structure analysis and risk assessment.
6. Conclusion
This study conducted a spatiotemporal algal proliferation risk analysis in the MRSNWDPC, considering the effects of hydrological and water quality indicators. A comprehensive coupling method based on the Vine Copula model and Latin hypercube sampling (LHS) technique was proposed. The algal proliferation risk under different environmental conditions in the main canal was analyzed. The main conclusions are:
1. All the water quality indicators during the monitoring periods from 2016 to 2018 meet the Class I or II standard, showing the excellent and low nutrient loadings of water quality status of the South-to-North Water Diversion Project of China. However, the algal cell density gradually increased from upstream to downstream along the main canal, and the annual average ACDs in downstream were the highest.
2. The joint early-warning system considering water temperature and flow velocity conditions is an effective way of algal proliferation control. The prevention and control management of ACD in the canal should be strengthened in summer and autumn. From the spatial prospect, the ACD control using the flow velocity regulation strategy should be carried out in the upstream and middle sections, which can achieve efficiency.
3. When water temperatures of the upstream, midstream, and downstream were below 26, 26, and 23°C, respectively, the algal proliferation risk can be controlled under 50% by the flow velocity at 0.3 m/s; otherwise, the flow velocity needs to be regulated higher than 0.8 m/s, which can improve the water quality of the main canal while controlling algae via flow velocity regulation. In order to keep the midstream and downstream avoid abnormal algal proliferation events (ACD ≥ 500 × 104 cells/L), the corresponding ACDs of the upstream and midstream need to be controlled lower than 319 × 104 cells/L and 470 × 104 cells/L, respectively.
4. The coupling method using the Latin hypercube sampling model and the Vine Copula model can effectively reduce sampling and simulation uncertainty in multivariate risk analysis, and can be generalized and applied to more multi-dependent structure analysis and risk assessment.
This study can provide a practical abnormal algal proliferation management strategy in the MRSNWDPC, which can effectively control the algal growth under different water temperatures by regulating flow in specific canal sections, and the early warning threshold for algal prevention can provide a comparable reference for similar water bodies. The proposed coupling method with the Vine Copula model and the LHS method can also be applied for the multivariate risk analysis field. However, due to limitation of data availability, this study may ignore the impact of some meteorological factors (e.g., rainfall and solar radiation) on algal proliferation. Considering that those projects will continue to supply water for large-scale cities for many years, long-term meteorological, hydrological, water quality, and ecology monitoring is still strongly needed, as well as in-depth multivariate risk analysis in future work.
Data availability statement
The original contributions presented in the study are included in the article/Supplementary material, further inquiries can be directed to the corresponding author.
Author contributions
XN: data collection, investigation, conceptualization, methodology, formal analysis, writing – original draft, and funding acquisition. JZ and YM: software and writing – original draft. LC: formal analysis, draft review and editing, and funding acquisition. CZ: data collection, investigation, and draft review and editing. KB: draft review and editing, and supervision. LCC: formal analysis, draft review and editing, and supervision. All authors contributed to the article and approved the submitted version.
Funding
This research was funded by the Specific Research Project of Guangxi for Research Bases and Talents (No. AD22035185), the Youth Science Foundation of Guangxi (No. 2023GXNSFBA026296), The Belt and Road Special Foundation of the National Key Laboratory of Water Disaster Prevention (No. 2022nkms06), and the National Natural Science Foundation of China (No. 51669003).
Acknowledgments
The authors would like to thank the editors and the reviewers for their valuable suggestions and contributions which significantly improved this article, and would also need to acknowledge the Construction and Administration Bureau of the Middle Route of the South-to-North Water Diversion Project of China that supported the data collection.
Conflict of interest
The authors declare that the research was conducted in the absence of any commercial or financial relationships that could be construed as a potential conflict of interest.
Publisher’s note
All claims expressed in this article are solely those of the authors and do not necessarily represent those of their affiliated organizations, or those of the publisher, the editors and the reviewers. Any product that may be evaluated in this article, or claim that may be made by its manufacturer, is not guaranteed or endorsed by the publisher.
Supplementary material
The Supplementary material for this article can be found online at: https://www.frontiersin.org/articles/10.3389/fevo.2023.1193163/full#supplementary-material
Footnotes
References
Atazadeh, E., Gell, P., Mills, K., Barton, A., and Newall, P. (2021). Community structure and ecological responses to hydrological changes in benthic algal assemblages in a regulated river: application of algal metrics and multivariate techniques in river management. Environ. Sci. Pollut. Res. 28, 39805–39825. doi: 10.1007/s11356-021-13546-w
Bedford, T., and Cooke, R. M. (2001). Probability density decomposition for conditionally dependent random variables modeled by vines. Ann. Math. Artif. Intell. 32, 245–268. doi: 10.1023/A:1016725902970
Brechmann, E. C., and Schepsmeier, U. (2013). Modeling dependence with C- and D-Vine Copulas: the R package CDVine. J. Stat. Softw. 52, 1–27. doi: 10.18637/jss.v052.i03
Brookfield, A. E., Hansen, A. T., Sullivan, P. L., Czuba, J. A., Kirk, M. F., Li, L., et al. (2021). Predicting algal blooms: are we overlooking groundwater? Sci. Total Environ. 769:144442. doi: 10.1016/j.scitotenv.2020.144442
Cai, S., Sun, L., Liu, Q., Ji, Y., and Wang, H. (2021). Research on the dispatching rules of Inter-Basin water transfer projects based on the two-dimensional scheduling diagram. Front. Earth Sci. 9:664201. doi: 10.3389/feart.2021.664201
Cattaneo, A., Hudon, C., Vis, C., and Gagnon, P. (2013). Hydrological control of filamentous green algae in a large fluvial lake (Lake Saint-Pierre, St. Lawrence River, Canada). J. Great Lakes Res. 39, 409–419. doi: 10.1016/j.jglr.2013.06.005
Chen, M., Bai, Z., Wang, Q., and Shi, Z. (2021). Habitat quality effect and driving mechanism of land use transitions: a case study of Henan water source area of the middle route of the south-to-north water transfer project. Land 10:796. doi: 10.3390/land10080796
Chen, H., Jing, Z., Ni, Z., Luo, H., Luo, P., and Li, Q. (2022). Spatiotemporal variation of water quality in the Main Canal of middle route of south-to-north water diversion project based on principal component and cluster analysis. J. Yangtze River Sci. Res. Inst. 39, 36–44. doi: 10.11988/ckyyb.20210272
Cheng, B., Xia, R., Zhang, Y., Yang, Z., Hu, S., Guo, F., et al. (2019). Characterization and causes analysis for algae blooms in large river system. Sustain. Cities Soc. 51:101707. doi: 10.1016/j.scs.2019.101707
China, M. (2002b). Standard methods for the examination of water and wastewater (version 4). MEP, China: China Environmental Science Press.
Dai, J., Wu, S., Wu, X., Lv, X., Sivakumar, B., Wang, F., et al. (2020). Impacts of a large river-to-lake water diversion project on lacustrine phytoplankton communities. J. Hydrol. 587:124938. doi: 10.1016/j.jhydrol.2020.124938
Dong, W., Sun, H., Tan, J., Li, Z., Zhang, J., and Yang, H. (2022). Regional wind power probabilistic forecasting based on an improved kernel density estimation, regular vine copulas, and ensemble learning. Energy 238:122045. doi: 10.1016/j.energy.2021.122045
Fan, Y., Huang, K., Huang, G. H., and Li, Y. P. (2020). A factorial Bayesian copula framework for partitioning uncertainties in multivariate risk inference. Environ. Res. 183:109215. doi: 10.1016/j.envres.2020.109215
Feng, Z.-K., Niu, W.-J., Jiang, Z.-Q., Qin, H., and Song, Z.-G. (2020). Monthly operation optimization of Cascade hydropower reservoirs with dynamic programming and Latin hypercube sampling for dimensionality reduction. Water Resour. Manag. 34, 2029–2041. doi: 10.1007/s11269-020-02545-0
Gao, X.-Y., Xie, W., and Liu, Z.-P. (2022). Algae control in oligotrophic surface water under the joint effect of nutritional competition and microbial algae-lytic substances. Environ. Sci. Water Res. Technol. 8, 375–384. doi: 10.1039/D1EW00563D
Hu, Y., Hu, P., Zhu, J., and Liao, W. (2022). Growth-migration model of attached algae and its application in the middle route of south-to-north water transfer project. J Water Resourc Water Engin 33, 61–68. doi: 10.11705/j.issn.1672-643X.2022.01.09
Huang, Y., Chen, S., Chen, Z., Hu, W., and Huang, Q. (2020). Improved probabilistic load flow method based on D-vine copulas and Latin hypercube sampling in distribution network with multiple wind generators. Iet Gener. Transm. Dis. 14, 893–899. doi: 10.1049/iet-gtd.2019.1126
Huang, S., Nong, X., Liang, J., Shao, D., and Zhong, H. (2019). Environmental problems and risk analysis of the operation of the middle route of the south-to-north water diversion project. Yangtze River 50, 46–51. doi: 10.16232/j.cnki.1001-4179.2019.08.008
Huang, K., Ye, L., Chen, L., Wang, Q., Dai, L., Zhou, J., et al. (2018). Risk analysis of flood control reservoir operation considering multiple uncertainties. J. Hydrol. 565, 672–684. doi: 10.1016/j.jhydrol.2018.08.040
Huisman, J., Codd, G. A., Paerl, H. W., Ibelings, B. W., Verspagen, J. M. H., and Visser, P. M. (2018). Cyanobacterial blooms. Nat. Rev. Microbiol. 16, 471–483. doi: 10.1038/s41579-018-0040-1
Huo, S., He, Z., Ma, C., Zhang, H., Xi, B., Xia, X., et al. (2019). Stricter nutrient criteria are required to mitigate the impact of climate change on harmful cyanobacterial blooms. J. Hydrol. 569, 698–704. doi: 10.1016/j.jhydrol.2018.12.030
Jing, Z., Chen, H., Cao, H., Tang, X., Shang, Y., Liang, Y., et al. (2022). Spatial and temporal characteristics, influencing factors and prediction models of water quality and algae in early stage of middle route of south-north water diversion project. Environ. Sci. Pollut. Res. 29, 23520–23544. doi: 10.1007/s11356-021-16917-5
Khozeymehnezhad, H., and Nazeri-Tahroudi, M. (2020). Analyzing the frequency of non-stationary hydrological series based on a modified reservoir index. Arab. J. Geosci. 13:232. doi: 10.1007/s12517-020-5226-y
Kraus, D., and Czado, C. (2017). D-vine copula based quantile regression. Comput. Stat. Data Anal. 110, 1–18. doi: 10.1016/j.csda.2016.12.009
Li, R., Chen, Q., Zhang, X., and Recknagel, F. (2015). Effects of temperature and macronutrients on phytoplankton communities across three largely different lakes identified by a time-space trade-off approach. Ecol. Inform. 29, 174–181. doi: 10.1016/j.ecoinf.2014.09.004
Li, J., Jin, Z., and Yang, W. (2014). Numerical modeling of the Xiangxi River algal bloom and sediment-related process in China. Ecol. Inform. 22, 23–35. doi: 10.1016/j.ecoinf.2014.03.002
Li, J., Yin, W., Jia, H., and Xin, X. (2021). Hydrological management strategies for the control of algal blooms in regulated lowland rivers. Hydrol. Process. 35:e14171. doi: 10.1002/hyp.14171
Li, B., Zhang, Y., Deng, R., Zhu, Y., Song, G., Mi, W., et al. (2022). Effects of water velocity on the growth and photosynthetic activity of Cyclotella meneghiniana (diatom). Chinese J. Ecol. 41, 1385–1390. doi: 10.13292/j.1000-4890.202207.014
Li, Z., Zhang, Z., Yang, Z., Guo, J., Liu, J., Li, D., et al. (2015). Effects of flow speed on the change of in situ growth rates of algae in Pengxi River backwater zone, Three Gorges Reservoir. J. Lake Sci. 27, 880–886. doi: 10.18307/2015.0514
Liang, P., Wang, X., and Ma, F. (2013). Effect of hydrodynamic conditions on water eutrophication: a review. J. Lake Sci. 25, 455–462. doi: 10.18307/2013.0401
Liebscher, E. (2008). Construction of asymmetric multivariate copulas. J. Multivar. Anal. 99, 2234–2250. doi: 10.1016/j.jmva.2008.02.025
Liu, Z., Guo, S., Xu, X., Xu, S., and Cheng, J. (2021). Application of copula functions in hydrology and water resources: a state-of-the-art review. Adv. Water Resour. 32, 148–159. doi: 10.14042/j.cnki.32.1309.2021.01.015
Long, Y., Feng, M., Li, Y., Qu, J., and Gao, W. (2022). Comprehensive risk assessment of algae and shellfish in the middle route of south-to-north water diversion project. Environ. Sci. Pollut. Res. 29, 79320–79330. doi: 10.1007/s11356-022-21210-0
Mei, X., Gao, S., Liu, Y., Hu, J., Razlustkij, V., Rudstam, L. G., et al. (2022). Effects of elevated temperature on resources competition of nutrient and light between benthic and planktonic algae. Front. Environ. Sci. 10:624. doi: 10.3389/fenvs.2022.908088
Nazeri-Tahroudi, M., Ramezani, Y., De Michele, C., and Mirabbasi, R. (2022). Bivariate simulation of potential evapotranspiration using copula-GARCH model. Water Resour. Manag. 36, 1007–1024. doi: 10.1007/s11269-022-03065-9
Nong, X., Shao, D., Shang, Y., and Liang, J. (2021). Analysis of spatio-temporal variation in phytoplankton and its relationship with water quality parameters in the south-to-north water diversion project of China. Environ. Monit. Assess. 193:593. doi: 10.1007/s10661-021-09391-6
Nong, X., Shao, D., Zhong, H., and Liang, J. (2020). Evaluation of water quality in the south-to-north water diversion project of China using the water quality index (WQI) method. Water Res. 178:115781. doi: 10.1016/j.watres.2020.115781
Schepsmeier, U. (2019). A goodness-of-fit test for regular vine copula models. Econ. Rev. 38, 25–46. doi: 10.1080/07474938.2016.1222231
Shen, L., Dou, M., Xia, R., Li, G., and Yang, B. (2021). Effects of hydrological change on the risk of riverine algal blooms: case study in the mid-downstream of the Han River in China. Environ. Sci. Pollut. Res. 28, 19851–19865. doi: 10.1007/s11356-020-11756-2
Shields, M., and Zhang, J. (2016). The generalization of Latin hypercube sampling. Reliab. Eng. Syst. Safe. 148, 96–108. doi: 10.1016/j.ress.2015.12.002
Sklar, A. (1959). Fonctions de Repartition a n Dimensions et Leurs Marges. Publ.inst.statist.univ.paris 8, 229–231.
Song, Y., Zhang, L.-L., Li, J., Chen, M., and Zhang, Y.-W. (2018). Mechanism of the influence of hydrodynamics on Microcystis aeruginosa, a dominant bloom species in reservoirs. Sci. Total Environ. 636, 230–239. doi: 10.1016/j.scitotenv.2018.04.257
Tahroudi, M. N., Ramezani, Y., De Michele, C., and Mirabbasi, R. (2022). Trivariate joint frequency analysis of water resources deficiency signatures using vine copulas. Appl Water Sci 12:67. doi: 10.1007/s13201-022-01589-4
Tang, M., Xu, W., Zhang, C., Shao, D., Zhou, H., and Li, Y. (2022). Risk assessment of sectional water quality based on deterioration rate of water quality indicators: a case study of the main canal of the middle route of south-to-north water diversion project. Ecol. Indic. 135:108592. doi: 10.1016/j.ecolind.2022.108592
Tian, J., Guo, S., Wang, J., Zhang, D., and Luo, C. (2022). Identification and threshold analysis of key factors of algal bloom in middle and lower reaches of the Hanjiang River. Water Resour. Prot. 38, 196–203. doi: 10.3880/j.issn.1004-6933.2022.05.026
Wan, J., and Li, S. (2019). Modeling and application of industrial process fault detection based on pruning vine copula. Chemometr. Intell. Lab. Syst. 184, 1–13. doi: 10.1016/j.chemolab.2018.11.005
Wang, Y., Zhang, C., Bi, Y., Zhu, Y., Song, G., Wang, S., et al. (2022). Spatial-temporal dynamics of odor substances in the middle line of the south-to-north water diversion project and their influencing factors. Acta Hydrobiol. Sin. 46, 149–159. doi: 10.7541/2022.2020.206
Wang, C., Zhang, H., Lei, P., Xin, X., Zhang, A., and Yin, W. (2022). Evidence on the causes of the rising levels of CODMn along the middle route of the south-to-north diversion project in China: the role of algal dissolved organic matter. J. Environ. Sci. 113, 281–290. doi: 10.1016/j.jes.2021.06.003
Wasson, K., Jeppesen, R., Endris, C., Perry, D. C., Woolfolk, A., Beheshti, K., et al. (2017). Eutrophication decreases salt marsh resilience through proliferation of algal mats. Biol. Conserv. 212, 1–11. doi: 10.1016/j.biocon.2017.05.019
Wu, Z., Wang, X., Chen, Y., Cai, Y., and Deng, J. (2018). Assessing river water quality using water quality index in Lake Taihu Basin. China. Sci. Total Environ. 612, 914–922. doi: 10.1016/j.scitotenv.2017.08.293
Xin, X., Wang, Y., Hu, S., and Li, J. (2019). Analysis of the genesis of diatom blooms in the lower reaches of the Han River in 2018. Water Resour. Pow. 37, 25–28.
Xin, X., Zhang, H., Lei, P., Tang, W., Yin, W., Li, J., et al. (2020). Algal blooms in the middle and lower Han River: characteristics, early warning and prevention. Sci. Total Environ. 706:135293. doi: 10.1016/j.scitotenv.2019.135293
Yan, X., Zhang, Y., Li, Y., Jiang, Y., Cui, Z., Gao, X., et al. (2021). Hydrologic and physicochemical factors co-drive seasonal changes of phytoplankton during dynamic water diversion processes in the Danjiangkou reservoir. J. Lake Sci. 33, 1350–1363. doi: 10.18307/2021.0505
Yang, S., Bai, J., Zhao, C., Lou, H., Zhang, C., Guan, Y., et al. (2018). The assessment of the changes of biomass and riparian buffer width in the terminal reservoir under the impact of the south-to-north water diversion project in China. Ecol. Indic. 85, 932–943. doi: 10.1016/j.ecolind.2017.11.011
Zeng, Q., Qin, L., and Li, X. (2015). The potential impact of an inter-basin water transfer project on nutrients (nitrogen and phosphorous) and chlorophyll a of the receiving water system. Sci. Total Environ. 536, 675–686. doi: 10.1016/j.scitotenv.2015.07.042
Zeng, W., Xu, M., Song, S., and Wu, H. (2022). Joint probability distribution and risk identification of extreme precipitation based on R-vine copula function. Water Resour. Prot. 38, 96–103. doi: 10.3880/j.issn.1004-6933.2022.06.013
Zhang, W., Che, Y., and Liu, Y. (2015). Improved Latin hypercube sampling method for reliability evaluation of power systems. Autom. Electr. Power Syst. 39, 52–57. doi: 10.7500/AEPS20140220002
Zhang, H., Chen, R., Li, F., and Chen, L. (2015). Effect of flow rate on environmental variables and phytoplankton dynamics: results from field enclosures. Chin. J. Oceanol. Limnol. 33, 430–438. doi: 10.1007/s00343-015-4063-4
Zhang, Z., Du, Z., Lei, X., Quan, J., Cao, H., Zheng, T., et al. (2019). Mathematical analysis methods and suggestions on algae proliferation potential in main canal of middle route of south-to-north water transfer project. South North Water Trans Water Sci Technol 17, 123–128. doi: 10.13476/j.cnki.nsbdqk.2019.0092
Zhang, H., Jia, J., Chen, S., Huang, T., Wang, Y., Zhao, Z., et al. (2018). Dynamics of bacterial and fungal communities during the outbreak and decline of an algal bloom in a drinking water reservoir. Int. J. Environ. Res. Public Health 15:361. doi: 10.3390/ijerph15020361
Zhang, Y., Li, W., and Chen, Q. (2015). Algae bloom risk zoning of Lake Taihu. J. Lake Sci. 27, 1133–1139. doi: 10.18307/2015.0619
Zhang, C., Mi, W., Xu, Y., Song, G., Zhu, Y., and Bi, Y. (2021a). Characteristics of phytoplankton communities and water environment assessment of the main trunk canal of the south-to-north water diversion project. J. Hydroecol. 42, 47–54. doi: 10.15928/j.1674-3075.201906280161
Zhang, C., Nong, X., Shao, D., Zhong, H., Shang, Y., and Liang, J. (2021b). Multivariate water environmental risk analysis in long-distance water supply project: a case study in China. Ecol. Indic. 125, 107577–107582. doi: 10.1016/j.ecolind.2021.107577
Zhang, C., Nong, X., Zhong, H., Shao, D., Chen, L., and Liang, J. (2022). A framework for exploring environmental risk of the longest inter-basin water diversion project under the influence of multiple factors: a case study in China. J. Environ. Manag. 322:116036. doi: 10.1016/j.jenvman.2022.116036
Zhang, C., Zhu, Y., Song, G., Mi, W., Bi, Y., Wang, S., et al. (2021c). Spatiotemporal pattern of phytoplankton community structure and its determining factors in the channel of the middle route of south-to-north water diversion project. J. Lake Sci. 33, 675–686. doi: 10.18307/2021.0305
Zhao, Y., Guo, Z., Niu, F., Yu, Y., and Wang, S. (2019). Global sensitivity analysis of passive safety systems of FHR by using meta-modeling and sampling methods. Prog. Nucl. Energy 115, 30–41. doi: 10.1016/j.pnucene.2019.03.002
Zhao, Q., Sun, G., Wang, J., Yu, Z., and Jiang, B. (2018). Coupling effect of water temperature and light energy on the algal growth in Lake Taihu. J. Lake Sci. 30, 385–393. doi: 10.18307/2018.0210
Zhao, Q., Wang, J., Wang, J., and Wang, J. X. L. (2019). Seasonal dependency of controlling factors on the phytoplankton production in Taihu Lake. China. J. Environ. Sci. 76, 278–288. doi: 10.1016/j.jes.2018.05.010
Zhao, Y., Zhu, Y., Lin, Z., Wang, J., He, G., Li, H., et al. (2017). Energy reduction effect of the south-to-north water diversion project in China. Sci. Rep. 7:15956. doi: 10.1038/s41598-017-16157-z
Zhou, J., Gou, T., Zhang, L., Lan, Y., Ma, Q., Liang, R., et al. (2018). The effect of flow velocity on the growth of different phytoplankton. Ecol. Sci. 37, 75–82. doi: 10.14108/j.cnki.1008-8873.2018.06.010
Zhu, Y., Mi, W., Li, B., Liang, J., Song, G., and Bi, Y. (2021). The influences of two hydraulic structures on periphytic algal communities in the middle channel of the south-to-north water diversion project. Acta Hydrobiol. Sin. 45, 817–825. doi: 10.7541/2021.2020.107
Zhu, J., Zhang, Z., Lei, X., Jing, X., Wang, H., and Yan, P. (2021). Ecological scheduling of the middle route of south-to-north water diversion project based on a reinforcement learning model. J. Hydrol. 596:126107. doi: 10.1016/j.jhydrol.2021.126107
Zhuang, W. (2016). Eco-environmental impact of inter-basin water transfer projects: a review. Environ. Sci. Pollut. Res. 23, 12867–12879. doi: 10.1007/s11356-016-6854-3
Keywords: algal proliferation, risk analysis, Vine Copula, Latin hypercube sampling, the South-to-North Water Diversion Project of China
Citation: Nong X, Zeng J, Ma Y, Chen L, Zhang C, Behzadian K and Campos LC (2023) Algal proliferation risk assessment using Vine Copula-based coupling methods in the South-to-North Water Diversion Project of China. Front. Ecol. Evol. 11:1193163. doi: 10.3389/fevo.2023.1193163
Edited by:
Chengcheng Xia, Sichuan University, ChinaReviewed by:
Jian Sha, Tianjin Normal University, ChinaYuliang Zhou, Hefei University of Technology, China
Copyright © 2023 Nong, Zeng, Ma, Chen, Zhang, Behzadian and Campos. This is an open-access article distributed under the terms of the Creative Commons Attribution License (CC BY). The use, distribution or reproduction in other forums is permitted, provided the original author(s) and the copyright owner(s) are credited and that the original publication in this journal is cited, in accordance with accepted academic practice. No use, distribution or reproduction is permitted which does not comply with these terms.
*Correspondence: Luiza C. Campos, bC5jYW1wb3NAdWNsLmFjLnVr