- 1School of Ecology and Environment, Inner Mongolia University, Hohhot, China
- 2School of Statistics and Mathematics, Inner Mongolia University of Finance and Economics, Hohhot, China
- 3Collaborative Innovation Center for Grassland Ecological Security (Jointly Supported by the Ministry of Education of China and Inner Mongolia Autonomous Region), Hohhot, China
- 4Ministry of Education Key Laboratory of Ecology and Resource Use of the Mongolian Plateau, Hohhot, China
- 5Environmental Monitoring Station, Bayannaoer, China
The quantitative relationship between land use/cover change (LUCC) within basins, lake water environmental factors, and water quality has long been a popular research area. In this study, we investigated three typical basins (Ulansuhai Basin, Daihai Basin, and Dalinor Basin) in arid and semi-arid areas of China. The assessment was based on the China Land Cover Dataset (CLCD), which was used to calculate the land use dynamic degree index and Markov transfer matrix. Moreover, an econometric analysis model and a hybrid evolutionary algorithm (HEA) model were used to explore the quantitative relationship between LUCC and environmental factors on the lake water quality. The results showed that the LUCC of the three lake basins was dominated by cultivated land and grassland over the past 20 years. In all of the basins, grassland and water area were converted into cultivated land, water area decreased to varying degrees, and impervious surface area increased continuously. Moreover, the concentration of TN and TP were generally proportional to chemical oxygen demand concentration (COD), biochemical oxygen demand concentration (BOD5), and dissolved oxygen concentration (DO), but inversely proportional to water depth and transparency; the relationship between Nutrient concentration and pH was usually related to the ecological threshold. On the basin scale, the grassland and forestland played positive roles in improving water quality, while cultivated land and impervious surface were the main factors contributing to water pollution. Overall, this study provides a scientific theoretical basis for the rational utilization of land resources, improvement of lake water quality, and ecological protection of typical lake basins in arid and semi-arid regions.
1 Introduction
Numerous academics believe that land use/cover change (LUCC), which is a direct outcome of human actions and natural forces, is one of the primary causes of global environmental changes (Turner, 1994; Li, 1996). Studies have mainly concentrated on the spatiotemporal changes in LUCC (Dewan and Yamaguchi, 2009), the driving force and mechanism (Lambin et al., 2001), and modeling and prediction (Tan et al., 2015). Studies on the effects of LUCC on the water environment are few (Yan and Chen, 1998). Water is essential for human life and production, and in recent years, water resources in developing countries have become increasingly scarce (Li et al., 2016). Moreover, these areas have undergone significant changes in land use type owing to the need for development, particularly in the arid and semi-arid regions of northern China (Liang et al., 2021). Studies have indicated that changes in land use patterns in basins can affect lake water quality in complex ways. Yang et al. (2019) investigated the relationship between different land uses and the quality of water from the Huaihe River Basin and found that forest land, grassland, and water areas could reduce water pollution, while barren and urban land were the major contributors to it. Ding et al. (2016) sampled basic flow in 56 low-order river basins in summer and classified the basins into two uniform geomorphic groups. Empirical models of multi-scale land use patterns and the water quality of low-order rivers in different geomorphic regions of the Dongjiang River Basin were established. These studies have also shown that land use at the catchment scale is a better explanation for overall water quality changes than land use at the reach-scale riparian, and the spatial scale of land use impact on water quality also varies with specific water parameters and geomorphic basis. Therefore, it is vital to investigate the impact of LUCC on the lake and river water quality at the watershed scale.
Studies of environmental factors on lake water quality have also attracted the attention of many researchers. Many nonlinear methods were used, such as the artificial network method, random forest models, and a generalized additive model (Recknagel et al., 1997; Quan et al., 2020; Shi et al., 2021). Cao et al. (2006) have developed the hybrid evolutionary algorithm (HEA) to determine the evolution of long time-series data through evolutionary simulation and genetic selection, and to make inductive inferences and predictions for multivariate nonlinear data. Using temporal changes in data to reveal ecological relationships. Current studies showed that the HEA model has a high level of prediction and has been successfully applied to drive the modeling of algal bloom data in lakes and rivers around the world (Guallar et al., 2016; Kim et al., 2019).
The Inner Mongolia Autonomous Region is an important ecological security barrier in northern China. Arid and semi-arid lakes in Inner Mongolia are facing problems such as shrinking lake areas and deteriorating water quality caused by human activities and climate change. The three representative lakes are Ulansuhai, Daihai, and Dalinor. Among them, Lake Ulansuhai, a representative lake in the Yellow River Basin, plays an important role in ecological protection, high-quality economic development, water conservancy structure, and the ecological environment of the Yellow River Basin (Li et al., 2020). However, in order to achieve the development of high grain yield, a large amount of fertilizer has been applied, which leads to serious non-point source pollution in the basin. The increase in the concentrations of nitrogen and phosphorus leads to the eutrophication of lake water and the decline of lake water quality (Koebbing et al., 2014). The Daihai Basin is a staggered basin of agriculture and animal husbandry. It is a typical transitional zone of different agricultural and animal husbandry production modes in northern China. Because the ecological environment has a strong transition and volatility, it is vulnerable to the effects of climate change and human activities (Gao et al., 2011). Lake Dalinor, a closed plateau lake, is the second-largest freshwater lake in Inner Mongolia. Over the years, the water quality of Dalinor has declined due to the decreased inflow of water volume, increased evaporation, and high pastoral activities. It is urgent to explore the driving mechanism of water quality change and water environment protection.
This paper has three main aims. The first is to research the characteristics of land use/land cover change and its relationship with water quality change in arid areas of northern China during 2000–2020. The second is the quantitative analysis of the influence of the change of lake water environmental factors on lake water quality in the long-term time scale. The third is to analyze the relative contribution of land use change and water environment change to water quality change. Three typical basins (Ulansuhai, Daihai, and Dalinor Basins) in agricultural regions, farming–pastoral ecotone, and the steppe region of Inner Mongolia were selected as research objects, respectively. This study could serve as a resource for the comprehensive management of water pollution control and provide theoretical support for the management and efficient distribution of watershed land resources to enhance water quality in arid regions.
2 Methods and materials
2.1 Study areas
This study was conducted in Lake Ulansuhai, Daihai, and Dalinor basins, Inner Mongolia, China (Figure 1). Located in northern China, they have a temperate monsoon climate, which is an important barrier for the security of the ecosystem in central and northern China. The total study area was 51863.84 km2. The eutrophication of these three lakes has worsened in recent years owing to the change in human activities and land use, and lake nutrient enrichment.
2.2 Datasets
2.2.1 Land use data
The watershed boundaries were extracted from ASTER GDEM elevation data obtained from remote sensing images of the study area. The land use patterns of the Ulansuhai, Daihai, and Dalinor watersheds were classified and analyzed using the China Land Cover Dataset (CLCD) on an annual basis from 2000 to 2020 (Yang and Huang, 2021).
2.2.2 Water quality data
Monthly water quality monitoring data from environmental monitoring stations in Ulansuhai, Daihai, and Dalinor from 2000 to 2020 were used in this study. Linear interpolation was used to obtain the lacustrine characteristic data, which were used as the daily input data. To build 7-day prediction models, the interpolated daily input data were moved 7 days relative to the daily output data.
2.3 Methods
This study examined the dynamic land use and land use transfer matrix from 2000 to 2020 in order to investigate the relationship between LUCC and lake water quality. It then determined the nonlinear relationship between land use/cover mode and lake TN and TP through an econometric analysis model. At the same time, the quantitative relationship between lake water environment factors and TN and TP was analyzed by HEA. The main research methods used are as follows:
2.3.1 Markov transition matrix
The Markov model was used to describe the transitions between different land types and the direction of different land types. The Markov model was used to quantitatively analyze the changes in land type area in Ulansuhai, Daihai, and Dalinor Basins and the direction of land use transfer. Equation (1) is the Markov transfer matrix.
where Pij refers to the area of a land type changes from the initial stage to the final stage in a certain period of time, and N is the total number of land use types.
2.3.2 Hybrid evolutionary algorithm
The hybrid evolutionary algorithm (HEA) can discover rule sets from ecological time-series data through genetic programming and optimize the parameters of rule sets using a genetic algorithm. The model parameters were optimized through the hill climbing method, and several generations were repeated continuously until the termination criteria of the run were satisfied. “IF-THEN-ELSE” rule sets were constructed, and the threshold data of the model and the sensitivity analysis data of each branch were calculated. Numerous studies have demonstrated that HEA can discover rule sets that predict unseen data and represent the causal relationships between physical and chemical variables and lake eutrophication (Cao et al., 2014; Kim et al., 2019). We define the root mean square error (RMSE) as the fitness function:
where m is the number of data points, and , are the th observed value and the th predicted value of the output variable, respectively. In addition to the RMSE of each rule set, the total R2 value between the predicted and measured values of a whole data set was also calculated to measure the accuracy of the algorithm.
Training and test data were obtained from the entire dataset. The training and test datasets were chosen randomly through the bootstrap method according to a predetermined division percentage (75% in this study) to improve the validity and stability of the model; that is, the computer randomly selected 75% of the total data as training data and the remaining data as test data for each run. Through this approach, more stable models can be produced, and the best model can be chosen according to the validation outcomes for the entire dataset. Figure 2 have showed a conceptual diagram of HEA. HEA for structure optimization were popsize = 100, maximum tree depth = 4, and maximum number of generations = 80 by using the programming language C++.
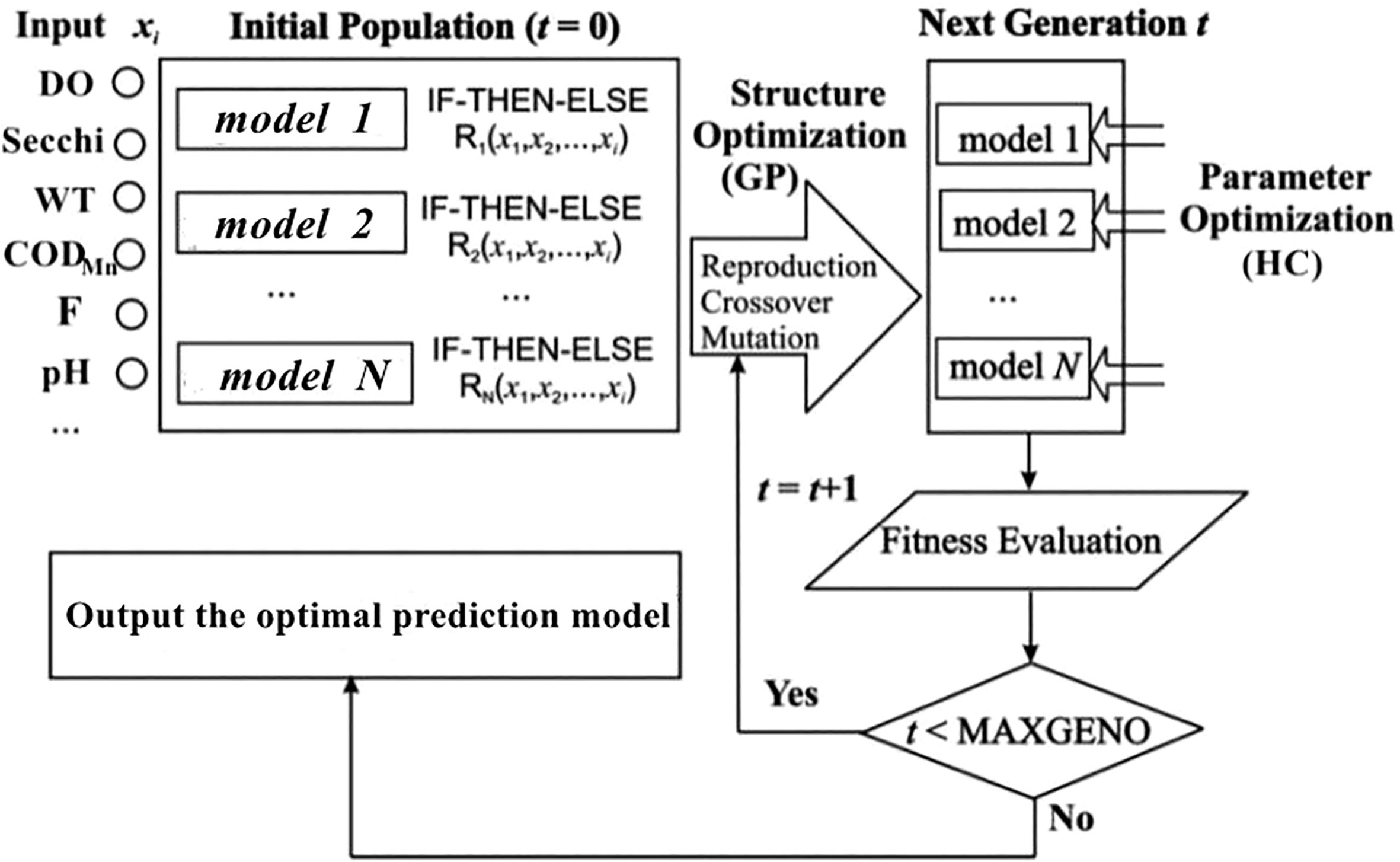
Figure 2 Conceptual diagram of the hybrid evolutionary algorithm (HEA) for evolving the IF-THEN-ELSE rule models.
2.3.3 Econometric analysis model
An index model was used to reveal the impacts of LUCC on different water quality factors of lake basins over long-term scales. An econometric analysis model was established.
where NPS represents the water quality index; α is a constant; β1–β6 are correlation coefficients between the area ratio of each land type and the water quality index. A positive βi value indicates that the land use type has a positive impact on water quality, while a negative βi value indicates that the land use type negatively affects water quality. γ is the land use type, and n is the number of land use types.
3 Results
3.1 LUCC in the three lake basins
CLCD was used to classify the land uses of the Ulansuhai, Daihai, and Dalinor basins from 2000 to 2020 (Figure 3). From 2000 to 2020, the primary land uses of the Ulansuhai basin were grassland and cropland, which accounted for 50% and 30% of the total area of the basin, respectively. Water and forest accounted for quite minimal percentages. During the past 20 years, the majority of the land in the Hetao irrigation region was used for agriculture. The cultivated area has expanded by nearly 1000 km2 since 2000. The Daihai Basin was mainly composed of grassland and cultivated land, which fluctuates, with the area of forest gradually increasing and the area of water gradually decreasing. Darinor basin was mainly grassland, grazing development is relatively developed. Meanwhile, forest area occupies the largest proportion in the three basins, which is larger than the cultivated land area.
According to the dynamic land use attitude (Table 1), the forest area and impervious surface significantly increased, while the area of grassland and barren land decreased. The LUCC of the Ulansuhai basin from 2000 to 2020 was relatively significant based on the Markov transfer matrix (Table 2). Barren land was mostly converted into cropland (with a conversion rate of 56.14%), followed by grassland (20.77%), and then impervious land (23.09%).

Table 1 Dynamic land use attitudes in the Ulansuhai, Daihai, and Dalinor Basins from 2000 to 2020(%).
From 2000 to 2020, the cropland area shifted primarily to grassland and impervious land, and the rate of change decreased over the years in the Daihai basin (Tables 1, 3). The water area in the Daihai Basin showed a dynamic change that resulted in a considerable decline and was primarily transformed into cropland, grassland, and impervious land.
According to the dynamic of Dalinor Basin land use from 2000 to 2020 (Table 1), the Dalinor Basin in cropland and water area decreased to varying degrees. The Markov transition matrix (Table 4) revealed that agricultural land and water area were largely transformed into grassland, which was transformed into forests, indicating that the percentage of forest areas increased. All land use types in the basin were converted into impervious surfaces to different degrees, and the percentage of water area was the highest.
3.2 The effect of LUCC along lake basins on the water quality of three lakes
Based on the econometric analysis model, the significant relationships between the LUCC of lake basins and lake water quality in Ulansuhai, Daihai, and Dalinor indicate that there was a significant overall impact of land use on the water quality of the lakes (Table 5).
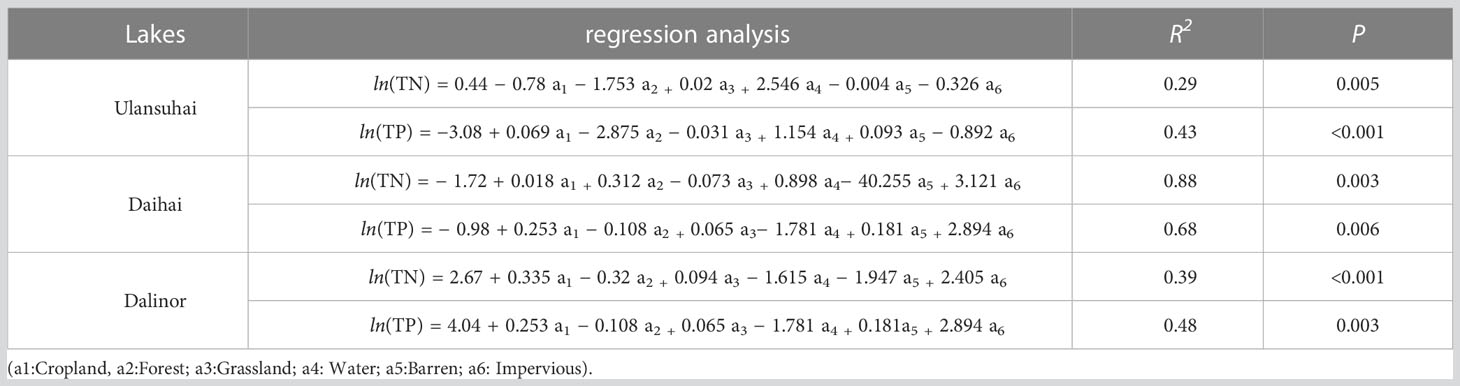
Table 5 Landuse/cover-lake water quality models of Lake Ulansuhia, Daihai, and Dalinor Basin from 2000 to 2020.
The negative correlation between cropland and the other factors, excluding the TN of Ulansuhai, indicates that cropland had an adverse effect on lake water quality. In the Ulansuhai and Daihai Basins, cropland was the most prevalent land type, accounting for 29.06% to 45.88% of the total basin area, respectively, and increasing yearly. The increase in the impervious surface was the most striking feature of urbanization. TN and TP were positively correlated with the impervious surface in the Daihai and Dalinor Basins, indicating that an increase in the impervious surface would facilitate an increase in the TN and TP concentrations of the lake. The TN and TP concentrations in the forestland were negatively correlated, indicating that the increase in forestland area suppressed water pollution and positively affected water quality. The soil layer under the forestland canopy exhibited functions of water conservation, soil erosion, interception, and water quality degradation (Sliva and Williams, 2001; Richardson et al., 2011). TN and TP were negatively correlated with the water area, indicating that the lake had a dilution and purification effect on the pollutants in the water (Zhao et al., 2020).
3.3 Establishment and optimization of simulation and prediction models for TN and TP in Lakes Ulansuhai, Daihai, and Dalinor
3.3.1 HEA of Lake Ulansuhai
The R2 values of the TN and TP of the selected model for Ulansuhai were 0.84 and 0.81, respectively. We established a quantitative relationship model between nutrient concentration and primary water quality parameters of Ulansuhai through HEA. The water quality parameters (i.e., Electrical conductivity [EC], chemical oxygen demand [COD], and depth) were used as input variables, while TN and TP were the output variables.
EC is the ecological threshold that influences the TN calculation method, as shown in the relationship model between TN and environmental components (Equations 3). The THEN branch determines TN when EC ≥ 349.71 ms/m. COD and dissolved oxygen (DO) are positively correlated with TN, while SD is negatively correlated with TN. Otherwise, according to the ELSE branch, TN is negatively correlated with depth and SD and positively correlated with COD.
The HEA of Lake Ulansuahi indicates that pH is the ecological threshold of the TP model (Equations 4). THEN branch analysis shows that TP was inversely correlated with water depth and positively correlated with pH at pH less than 8.92. TP was positively correlated with water temperature (WT) and pH at pH conditions greater than the ecological threshold but negatively correlated with SD and COD at pH conditions less than the ecological threshold.
3.3.2 HEA of Lake Daihai
To simulate and predict the quantitative relationship between TN, TP, and environmental parameters, we established a HEA model (from 2000 to 2020) for TN, TP, and other water quality data on Lake Daihai. The models achieved R2 of 0.68 and 0.67 for the TN and TP of Daihai, respectively.
The HEA revealed that biochemical oxygen demand (BOD5) = 7.8 mg/L was the ecological threshold of the TN model (Equations 5). TN was positively correlated with DO and BOD5 at BOD5 conditions greater than the ecological threshold. The ELSE branch simulated TN, which was positively correlated with DO and negatively correlated with depth.
The HEA revealed that the ecological threshold of the TP model was SD = 56.04 cm (Equations 6). When SD was less than or equal to the ecological threshold, TN was positively correlated with DO and COD and negatively correlated with WT. In contrast, TP was simulated through the ELSE branch, and TP was positively correlated with DO and COD.
3.3.3 HEA of Lake Dalinor
We also established a quantitative relationship model between the TN, TP, and other environmental factors of Lake Dalinor, and the selected models achieved R2 values of 0.57 and 0.60 for TN and TP, respectively.
The relationship model of TN illustrates that pH was the ecological threshold (Equations 7). TN was negatively correlated with SD but positively was correlated with COD at pH ≤ 9.44. The ELSE branch determines TN at pH > 9.44. TN was positively correlated with COD and DO but negatively correlated with SD.
The relationship model of TP illustrates that water depth was the ecological threshold (Equations 8). The THEN branch determines TP at water depth > 9.06 m. TP was positively correlated with the logarithm of COD and negatively correlated with the logarithm of depth. According to the ELSE branch, TP was positively correlated with COD and negatively correlated with pH and depth.
3.4 Sensitivity analysis of TN and TP simulation and prediction models in Lakes Ulansuhai, Daihai, and Dalinor
Sensitivity analysis was performed using recurrent supervised artificial neural networks (ANNs), and ordination and clustering analysis was performed using a non-supervised ANN according to 2000–2020 data on Lake Ulansuahi, Daihai, and Dalinor to study the complex relationships between TN, TP, and water quality conditions.
3.4.1 Sensitivity analysis of TN and TP in Lake Ulansuhai
Figure 4 shows the sensitivity functions for Lake Ulansuhai extracted from TN and TP models. According to the sensitivity analysis of the HEA model of TN in Ulansuhai, water depth exhibited a significant negative relationship with TN content (Figure 4A). As the water depth approached 3.12 m, TN gradually approached zero. With increasing SD, TN eventually decreased. In contrast, TN increased with increasing DO and COD (Figure 4B).
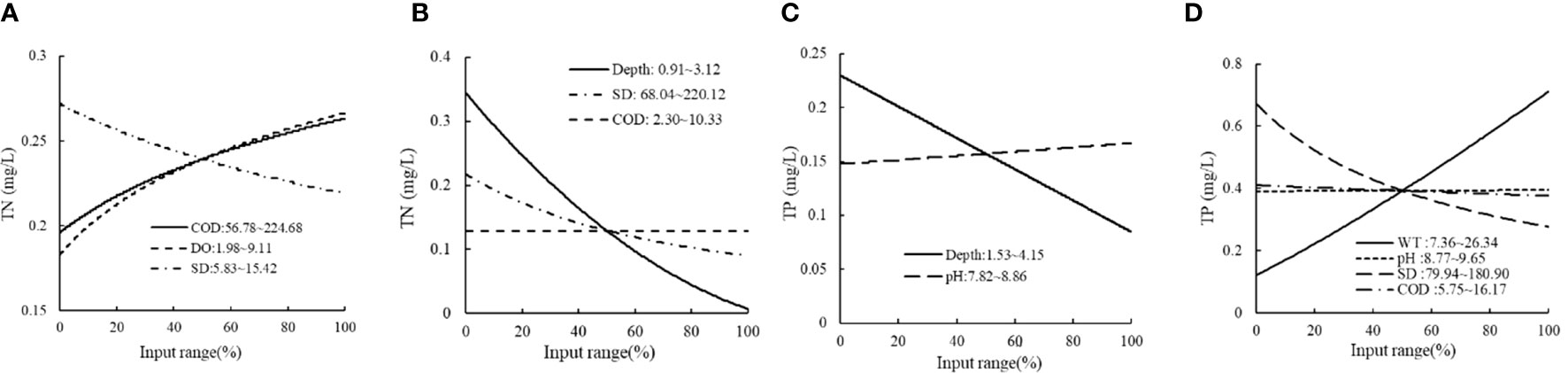
Figure 4 Sensitivity analysis of HEA models of TN and TP in Ulansuhai: (A) THEN branch of TN; (B) ELSE branch of TN; (C) THEN branch of TP; (D) ELSE branch of TP.
The sensitivity analysis of the TP model revealed that the TP concentration at an ecological threshold pH of > 8.92 was significantly higher than that at an ecological threshold pH of< 8.92. This indicates that an alkaline environment was beneficial for TP growth. The THEN branch (Figure 4C) showed that the TP concentration slowly increased as pH increased progressively between 7.82 and 8.86. The ELSE branch (Figure 4D) showed that as WT increased between 7.36°C and 26.34°C, TP concentration rapidly increased, resulting in improved water stability, intensified stratification, and increased nutrient release. TP was inversely related to water depth and clarity.
3.4.2 Sensitivity analysis of TN and TP in Lake Daihai
The sensitivity analysis of TN in Daihai showed that BOD5 and DO significantly increased at a certain concentration range at BOD5 > 7.8 mg/L (Figure 5A). The ELSE branch of TN (Figure 5B) showed that with increasing water depth, TN increasingly decreased. However, BOD5 and DO gradually increased, and the TN concentration remained at 0.5 mg/L.
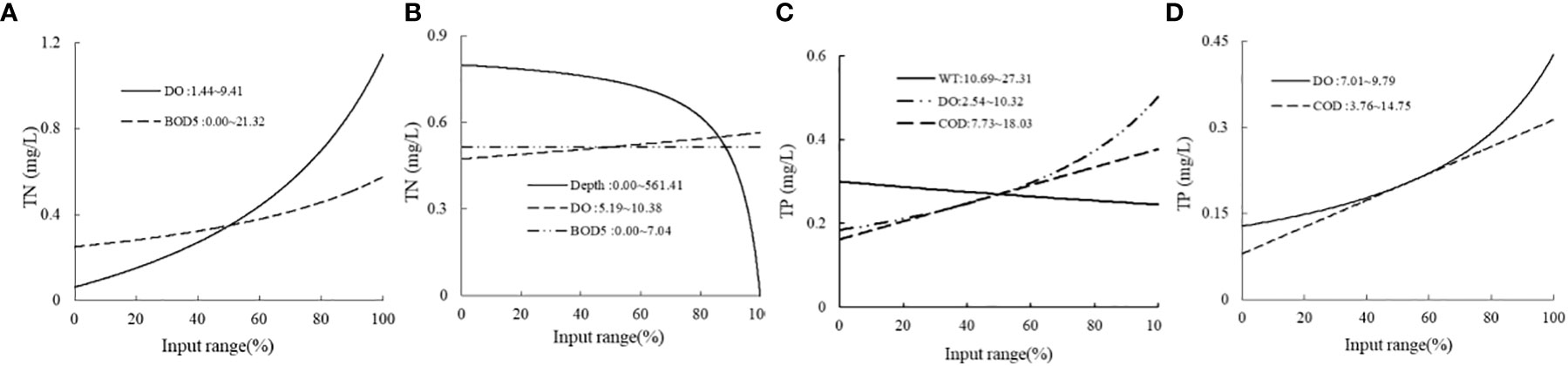
Figure 5 Sensitivity analysis of HEA models of TN and TP in Daihai. (A) THEN branch of TN; (B) ELSE branch of TN; (C) THEN branch of TP; (D) ELSE branch of TP.
At an ecological threshold SD ≤ 56.04 m, TP was estimated using the THEN branch (Figure 5C). With the gradual increase in DO from 2.54 to 10.32 mg/L, the TP concentration exhibited a non-linear increase trend and increasing growth rate, while COD linearly increased between 7.73 and 18.03 mg/L. The TP concentration decreased with the gradual increase in WT from 10.69°C to 27.31°C because low temperatures were more conducive to phosphorus accumulation in areas of lower transparency. In the case in which the IF condition was not satisfied (SD > 56.04 m), TP was estimated using the ELSE branch (Figure 5D) and was positively correlated with DO and COD.
3.4.3 Sensitivity analysis of TN and TP in Lake Dalinor
At pH ≤ 9.44, the TN of Dalinor was calculated using the THEN branch (Figure 6A). With increasing SD, TN concentration sharply decreased from 0.56 to 0.11 mg/L, and TN was positively correlated with COD. At pH conditions greater than the ecological threshold, TN was calculated using the ELSE branch (Figure 6B). With the gradual increase in SD and DO, the TN concentration slightly decreased and remained at 0.2 mg/L.
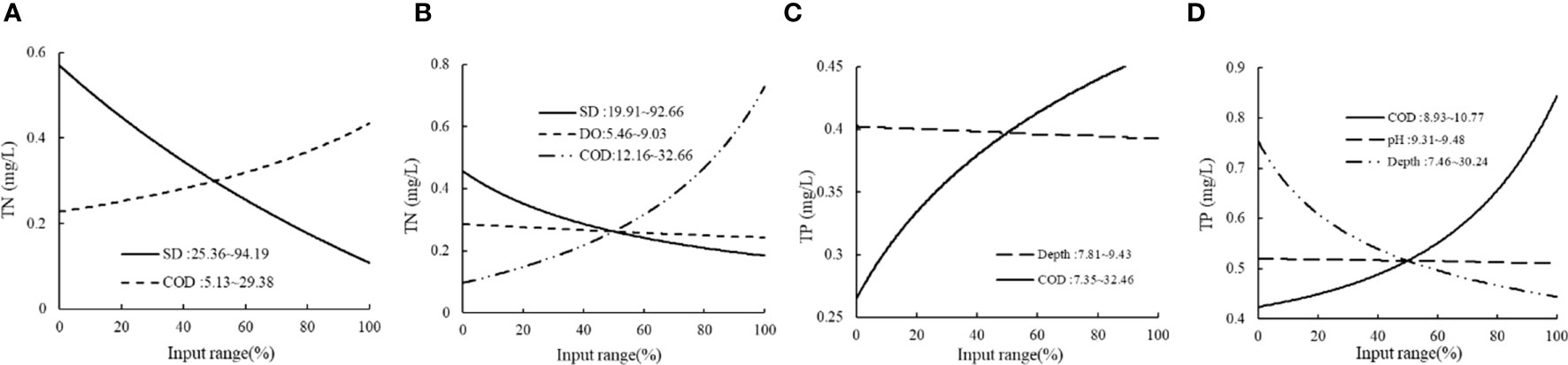
Figure 6 Sensitivity analysis of the HEA model of TN and TP of Dalinor. (A) THEN branch of TN; (B) ELSE branch of TN; (C) THEN branch of TP; (D) ELSE branch of TP.
The sensitivity analysis of TP showed that the TP concentration at an ecological threshold water depth > 9.06 m (THEN branch, Figure 6C) was significantly lower than that at a water depth ≤ 9.06 m. The ELSE branch (Figure 6D) demonstrated that the higher the water depth, the lower the TP concentration.
4 Discussion
4.1 LUCC and its effects on lake water quality
In recent years, owing to the policy of “returning cropland to forests and grazing land to grassland,” cropland has been primarily converted into grassland and forest land in China (Tian et al., 2019). Mountains, rivers, forests, fields, lakes, and grass are interrelated (Lu et al., 2019). In the Daihai Basin, the cultivated land area significantly decreased and was mainly converted into grassland, because Lake Daihai was designated as one of the 365 “Beautiful Lakes” under National Planning Protection in 2014, and the appropriate departments planted trees around the lake and implemented the “returning farmland to wetland” policy (Li et al., 2022). Moreover, the water area of the Daihai Basin was mainly transformed into cropland and impervious land. This change is attributable to the increasing population and demand for cropland and construction land (Cheng et al., 2017). The impervious land area has steadily increased over the past 20 years. Water and grassland were greatly harmed via the transformation process, owing to the increased agriculture, breeding, and tourism in Daihai and the new power plants and breeding farms constructed around the lake (Chen et al., 2018). Moreover, we found that the Dalinor Basin was mainly transformed into grassland and impervious ground because animal husbandry dominated the Dalinor Basin, and grassland was given priority as a land use type in the watershed. In 2019, the Government of Daihai Basin published a breeding balance scheme to protect the valley grassland ecological environment and enhance grassland water conservation. The grassland area tended to decrease from 2000 to 2020, but this trend was not significant. However, a smaller portion of the water area of the river basin was considered, owing to the location of the basin in the farming–pastoral zone of northern China, where animal husbandry has been practiced for a long time. Consequently, the demand for water resources increased, and because the lake is the primary source of animal husbandry, as the grass grows, the lake area decreases (Zhou et al., 2022).
Furthermore, we also explored the multiple regression relationship between land use type and lake nutrient concentration. According to several studies, the higher the negative influence on lake water quality, the higher the cropland area (Li et al., 2017). The runoff of contaminants on the soil surface into the lake during rainfall negatively affects the lake water quality (Jiang et al., 2006; Michael et al., 2018). From 2000 to 2020, impervious surface runoff pollution, caused by urbanization, was the second largest non-point source of pollution after agricultural surface pollution (Wilson and Weng, 2010). The impervious surface features a high population density, and numerous production activities and social activities, resulting in a large amount of domestic and industrial pollution runoff into the lake after limited purification and adsorption, leading to water quality decline, consistent with existing research results (Guo et al., 2006). Over the past 20 years, the temperatures of all three basins have increased, increasing evaporation. Overall, the excessive exploitation of groundwater has reduced the inflow of lake water, subjecting the areas of the three lakes to varying degrees of shrinkage, which is the main reason for the water quality deterioration (Hao et al., 2018; Chen et al., 2021).
4.2 Dynamics and drivers of lake water quality
TN and TP in Lake Ulansuhai showed a significant downward trend over the past 20 years; however, the lake water quality was still V, attributable to the improvement in water quality despite the increasing pressure from population increase and the demand for more farmland. This trend was related to the prior poor water quality of Ulansuhai, and the improvement in the water environment by basin management has exceeded the negative impact of the increased pressure (Chen et al., 2021).
The sensitivity analysis of the HEA model showed that the TN and TP concentrations decreased with increasing water depth and transparency. N and P in the water environment primarily originated from agricultural land fertilization; moreover, agricultural non-point source pollution was primarily concentrated in the lakeshore buffer zone and shallow water area, which led to a lower nutrient concentration and better water quality in the deep water area. These results are consistent with those of previous studies (Liang et al., 2016; Recknagel et al., 2016). In addition, DO and COD in the three lakes were positively correlated with TN and TP (Wang et al., 2019; Shi et al., 2020), but the increased amplitudes in DO and COD were significantly different, which was largely related to the ecological threshold of the model. This demonstrates that the reasons for the change in TN and TP concentrations were complex and influenced by the lake water environment.
5 Conclusion
1) The econometric model and mixed evolution algorithm were used to quantitatively reveal the driving mechanism of water quality change in three typical watersheds in the arid and semi-arid regions of northern China.
2) The econometric analysis model predicted that lake water quality declined with increasing cultivated land and impervious surface area and decreasing water area.
3) The HEA models for the TN and TP of the three lakes quantitatively revealed the relationship between environmental conditions and lake water quality. TN and TP were generally proportional to COD, BOD5, and DO, but inversely proportional to water depth and transparency.
4) The relative contribution analysis showed that the increase in human activities such as cultivated land was the main factor causing water pollution.
To combat emissions from non-point sources of pollution, the rate of urbanization needs to be decreased, and wetland coverage needs to be increased. This study could serve as a guide for the management of the water quality of basins and environmental planning in arid and semi-arid regions.
Data availability statement
The original contributions presented in the study are included in the article/supplementary material. Further inquiries can be directed to the corresponding authors.
Author contributions
LXW, XL, JJ, and YL conceived the ideas and designed this study. XL, HL, YZ, ZX, XC, and LM interpreted data and analysis. XL and HL wrote the manuscript. LXW and HL reviewed the manuscript.
Funding
This work was supported by the National Natural Science Funds, P.R. China (No. 32160279, 32161143025, 31960249), Science and Technology Major Project of Inner Mongolia (No. 2022YFHH0017, 2021ZD0011), the Funds of Yinshanbeilu Grassland Eco-hydrology National Observation and Research Station (No. YSS2021007) and Ordos Science and Technology Plan (No. 2022EEDSKJZDZX010, 2022EEDSKJXM005).
Conflict of interest
The authors declare that the research was conducted in the absence of any commercial or financial relationships that could be construed as a potential conflict of interest.
Publisher’s note
All claims expressed in this article are solely those of the authors and do not necessarily represent those of their affiliated organizations, or those of the publisher, the editors and the reviewers. Any product that may be evaluated in this article, or claim that may be made by its manufacturer, is not guaranteed or endorsed by the publisher.
References
Cao H., Recknagel F., Orr P. T. (2014). Parameter optimization algorithms for evolving rule models applied to freshwater ecosystems. IEEE Trans. Evolution. Comput. 18, 793–806. doi: 10.1109/TEVC.2013.2286404
Cao H., Recknagel F., Kim B., Takamura N. (2006). Hybrid evolutionary algorithm for rule set discovery in time-series data to forecast and explain algal population dynamics in two lakes different in morphometry and eutrophication. (Berlin Heidelberg: Springer) p. 347-367. doi:10.1007/3-540-28426-5_17
Chen H. Y., An L. J., Zhang J. L. (2018). Characteristics of water surface area evolution and correlation analysis with meteorological fac-tors inBohai lake. Environ. Sci. Manage. 43 (12), 62–65. doi: 10.3969/j.issn.1673-1212.2018.12.015
Chen Q., Wang S., Ni Z., Guo Y., Zhang H. (2021). Dynamic and driving evolution of lake basin pressure in cold and arid regions based on a new method: a case study of three lakes in inner Mongolia, China. J. Environ. Manage. 298 (8), 113425. doi: 10.1016/j.jenvman.2021.113425
Cheng Y. Q., Zheng L. J., Fu Z. Q. (2017). Analysis of cause of daihai lake shrinkage and water conservation recommendations. Meteorol. J. Inner Mongolia 5), 24–26. doi: 10.14174/j.cnki.nmqx.2017.05.006
Dewan A. M., Yamaguchi Y. (2009). Land use and land cover change in greater Dhaka, Bangladesh: using remote sensing to promote sustainable urbanization. Appl. Geogr. 29 (3), 390–401. doi: 10.1016/j.apgeog.2008.12.005
Ding J., Jiang Y., Qi L., Hou Z., Liao Z., Fu L., et al. (2016). Influences of the land use pattern on water quality in low-order streams of the dongjiang river basin, China: a multi-scale analysis. Sci. Total Environ. 551/552, 205–216. doi: 10.1016/j.scitotenv.2016.01.162
Gao T., Wang J. G., Li R., Yue Y. J. (2011). Land use change and prediction for ecotone between agriculture and animal raising in northern China. J. Arid Land Resour. Environ. 25 (10), 52–57. doi: 10.13448/j.cnki.jalre.2011.10.013
Guallar C., Delgado M., Diogène J., Fernández-Tejedor M. (2016). Artificial neural network approach to population dynamics of harmful algal blooms in alfacs bay (NW mediterranean): case studies of karlodinium and pseudo-nitzschia. Ecol. Model. 338, 37–50. doi: 10.1016/j.ecolmodel.2016.07.009
Guo Q. H., Ma K. M., Yang L., Yiu Y. (2006). Main sources of urban non-point source pollution and control measures for classified catch-ments. Environ. Sci. 27 (11), 28–33. doi: 10.13227/j.hjkx.2006.11.006
Hao W. G., Liu H., Li Z. G., Jiao R., Liu H. L., Shen J., et al. (2018). The rating mode of eutrophication in dalinuoer lake. IOP Conf. Ser. Earth Environ. Sci. 185, 012033. doi: 10.1088/1755-1315/185/1/012033
Jiang Y. J., Yuan D. X., Xie S. Y., Li L. L., Zhang G., He R. S. (2006). The groundwater quality and land use change in a typical karst agricultural region: a case study of xiaojiang watershed, yunnan. Acta Geographica Sin. 61 (5), 471–481. doi: 10.1007/s11442-006-0403-9
Kim J. H., Lee. D. H., Kang. J. H. (2019). Associating the spatial properties of a watershed with downstream chl-a concentration using spatial analysis and generalized additive models. Water Res. 154, 378–401. doi: 10.1016/j.watres.2019.02.010
Koebbing J. F., Patuzzi F., Baratieri M., Beckmann V., Thevs N., Zerbe S. (2014). Economic evaluation of common reed potential for energy production: a case study in wuliangsuhai lake (Inner Mongolia, China). Biomass Bioenergy 70, 315–329. doi: 10.1016/j.biombioe.2014.08.002
Lambin E. F., Turner B. L., Geist H. J., Agbola S. B., Angelsen A., Bruce J. W., et al. (2001). The causes of land-use and land-cover change: moving beyond the myths. Global Environ. Change 11 (4), 261–269. doi: 10.1016/S0959-3780(01)00007-3
Li X. B. (1996). A review of the international researches on land use /land cover change. Acta Geographica Sin. 51 (6), 553–558. doi: 10.11821/xb199606009
Li J. L., Liu H., Ha S. N., Lu X. Y., Liu X. L., Wang L. X., et al. (2022). Simulation of land use and evaluation of ecosystem service value in daihai basin. Chin. J. Ecol. 41 (2), 343–350. doi: 10.13292/j.1000-4890.202201.023
Li W., Teng F., Yang W. H., Wang F., Yu L. H. (2020). Spatial distribution characteristics and pollution assessment of ice-water pollutants during the ice-sealing period in wuliangsuha. J. Irrigation Drainage 39 (2), 122–128, 144. doi: 10.13522/j.cnki.ggps.20190244
Li B. Y., Zhang Y. J., Peng Q. Z., Song Y. Y., Zhang Y. T., Fang S. B. (2017). Impacts of land use /cover on the water quality of gonghu bay in taihu lake. J. Shanghai Univ. 26 (2), 243–250. doi: 10.12024/jsou.20160501775
Li R. R., Zou Z. H., An Y. (2016). Water quality assessment in qu river based on fuzzy water pollution index method. J. En-vironmental Sci. 50, 87–92. doi: 10.1016/j.jes.2016.03.030
Liang L., Changyou L. I., Shi X., Zhao S., Zhang L. (2016). Analysis on the eutrophication trends and affecting factors in lake hulun 2006-2015. J. Lake Sci. 28, 1265–1273. doi: 10.18307/2016.0612
Liang X., Liu H. M., Ji M. C., Chang M., Wen L., Yu R. H., et al. (2021). Effects of land use /cover change on lake water quality in the semi-arid region of northern China: A case study in Lake Daihai Basin (2000–2018). J. Lake Sci. 33 (3), 727–736. doi: 10.18307/2021.0309
Lu F. F., Zhang Y., Li X., Chen C. (2019). Discussion on wetland protection and restoration along wuliangsuhai basin. For. Resour. Manage. 05, 23–27. doi: 10.13466/j.cnki.lyzygl.2019.05.005
Michael S., Robin H., Swearer S. E., Kirsten M. P. (2018). Frog occupancy of polluted wetlands in urban landscapes. Conserv. Biol. 33 (2), 389–402. doi: 10.1111/cobi.13210
Quan D., Zhang S., Shi X., Sun B., Guo Z. (2020). Impact of water environment factors on eutrophication status of lake ulansuhai based on monitoring data in 2013-2018. J. Lake Sci. 32, 1610–1619. doi: 10.18307/2020.0604
Recknagel F., Adrian R., Köhler J., Cao H. (2016). Threshold quantification and short-term forecasting of anabaena, aphanizomenon and microcystis in the polymictic eutrophic lake müggelsee (Germany) by inferential modelling using the hybrid evolutionary algorithm HEA. Hydrobiologia 778, 61–74. doi: 10.1007/s10750-015-2442-7
Recknagel F., French M., Harkonen P., Yabunaka K. I. (1997). Artificial neural network approach for modelling and prediction of algal blooms. Ecol. Model. 96, 11–28. doi: 10.1016/S0304-3800(96)00049-X
Richardson C. J., Flanagan N. E., Ho M., Pahl J. W. (2011). Integrated stream and wetland restoration: a watershed approach to improved water quality on the landscape. Ecol. Eng. 37 (1), 25–39. doi: 10.1016/j.ecoleng.2010.09.005
Shi R., Mao R. Y., Zhang M., Lü Y., Zhao J. X. (2021). Distribution, sources, and ecological risks of polyfluoroalkyl substances in the surface water of the wuliangsuhai watershed. Environ. Sci. 42, 663–672. doi: 10.13227/j.hjkx.202005216
Shi R., Zhao J., Shi W., Song S., Wang C. (2020). Comprehensive assessment of water quality and pollution source apportionment in wuliangsuhai lake, inner Mongolia, China. Int. J. Environ. Res. Public Health 17, 5054. doi: 10.3390/ijerph17145054
Sliva L., Williams D. D. (2001). Buffer zone versus whole catchment approaches to studying land use impact on river water quality. Water Res. 35 (14), 3462–3472. doi: 10.1016/S0043-1354(01)00062-8
Tan R. H., Liu Y. L., Zhou K. H., Jiao L., Wei T. (2015). A game-theory based agent-cellular model for use in urban growth simulation: a case study of the rapidly urbanizing wuhan area of central China. Computers Environ. Urban Syst. 49, 15–29. doi: 10.1016/j.compenvurbsys.2014.09.001
Tian Y., Feng Q. Y., Tang M. F., Zheng S. N., Wang L. N. (2019). Ecological protection and restoration of forest, wetland, grassland, and cropland based on the perspective of ecosystem assessment: a case study in wuliangsuhai watershed. Acta Ecol. Sin. 39 (23), 8826–8836. doi: 10.5846/stxb201911222531
Turner B. L. (1994). Local faces, global flows: the role of land use and land cover in global environmental change. Land Degrade-on Dev. 5 (2), 71–78. doi: 10.1002/ldr.3400050204
Wang J., Fu Z., Qiao H., Liu F. (2019). Assessment of eutrophication and water quality in the estuarine area of lake wuli, lake taihu, China. Sci. Total Environ. 650, 1392–1402. doi: 10.1016/j.scitotenv.2018.09.137
Wilson C., Weng Q. H. (2010). Assessing surface water quality and its relation with urban land cover changes in the lake calumet area, greater Chicago. Environ. Manage. 45 (5), 1096–1111. doi: 10.1007/s00267-010-9482-6
Yan W. J., Chen F. X. (1998). Pollution characteristics of surface water runoff from various types of land use in the lake chaohu ba-sin. Resour. Environ. Yangtze Basin 7 (3), 274–277.
Yang J., Huang X. (2021). The 30 m annual land cover dataset and its dynamics in China from 1990 to 2019. Earth System Sci. Data 13 (8), 3907–3925. doi: 10.5194/essd-13-3907-2021
Yang Q., Tang Q. H., Zhang Y. Y. (2019). Spatiotemporal changes of water quality inHuai river basin (Henan section) and its correlation with land use patterns. Res. Environ. Sci. 32 (9), 1519–1530. doi: 10.13198/j.issn.1001-6929.2019.01.21
Zhao L., Chen J. Y., Jiang X., Zheng S. F., Wang S. H. (2020). Temporal and spatial distribution characteristics and difference analysis of nitrogen and phosphorus in daihai lake. Environ. Sci. 41 (4), 1676–1683. doi: 10.13227/j.hjkx.201909090
Keywords: econometric analysis model, hybrid evolutionary algorithm, lake basins, land use/cover change, water quality, sensitivity analysis
Citation: Liu X, Liu H, Jing J, Liu Y, Xu Z, Cao X, Ma L, Zhuo Y, Wen L and Wang L (2023) How the land use/cover changes and environmental factors at different scales affect lake water quality in arid and semi-arid regions. Front. Ecol. Evol. 11:1188927. doi: 10.3389/fevo.2023.1188927
Received: 21 March 2023; Accepted: 30 June 2023;
Published: 18 July 2023.
Edited by:
Shovonlal Roy, University of Reading, United KingdomCopyright © 2023 Liu, Liu, Jing, Liu, Xu, Cao, Ma, Zhuo, Wen and Wang. This is an open-access article distributed under the terms of the Creative Commons Attribution License (CC BY). The use, distribution or reproduction in other forums is permitted, provided the original author(s) and the copyright owner(s) are credited and that the original publication in this journal is cited, in accordance with accepted academic practice. No use, distribution or reproduction is permitted which does not comply with these terms.
*Correspondence: Huamin Liu, liuhmimu@aliyun.com; Lixin Wang, Lxwang@imu.edu.cn