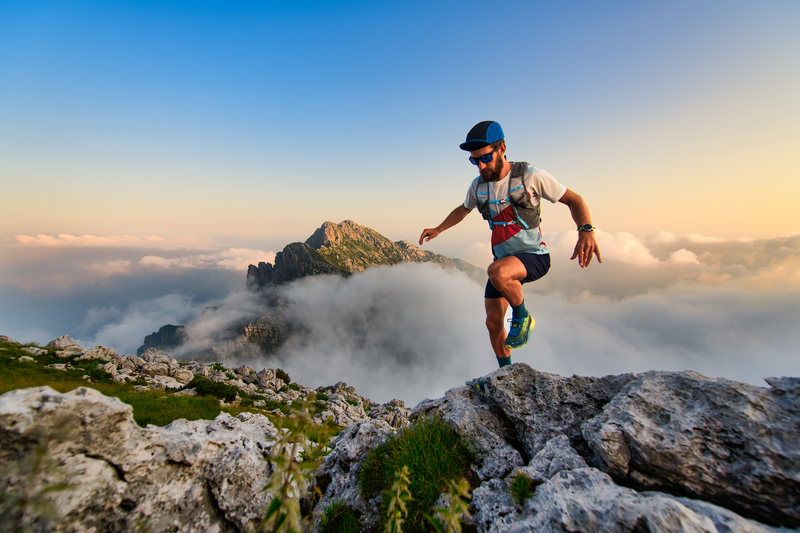
95% of researchers rate our articles as excellent or good
Learn more about the work of our research integrity team to safeguard the quality of each article we publish.
Find out more
ORIGINAL RESEARCH article
Front. Ecol. Evol. , 28 July 2023
Sec. Environmental Informatics and Remote Sensing
Volume 11 - 2023 | https://doi.org/10.3389/fevo.2023.1181332
This article is part of the Research Topic Recent Advances in Scientific Knowledge of Ecological Change and Its Methods of Monitoring and Evaluating the SDGs View all 3 articles
Drought is one of the main factors limiting forest productivity, and thus greatly affects the carbon sink capacity of forests. Here we first chose two drought indices including standardized precipitation evapotranspiration index (SPEI) and self-calibrating Palmer drought severity index (scPDSI) to reflect and analyze the spatiotemporal patterns of drought in the subtropical China. Then, the validated CASA (Carnegie-Amer-Stanford Approach) model was applied to estimate forest net primary productivity (NPP) and further quantify the contributions of drought events and their characteristics on forest NPP. The results showed that drought events during 2000–2015 have resulted in a mean decline of forest NPP of 7.2%. Moderate or severe drought events reduced NPP more significantly than extremely severe drought events. In addition, there was 1–2 years of lagging in the NPP responses to drought, and the lagging time varied with forest types. Our study suggests that forest managers and local governments should pay more attention to the places with moderate and severe drought events, and take measures to avoid NPP decline within the 2 years after drought. Our study also provides data support for further identifying the contribution of drought to ecosystem carbon fluxes in the subtropical China.
In the context of climate change, drought has become a serious and extraordinary natural disaster around the globe. Drought can reduce the growth rate of vegetation by inhibiting photosynthesis and hence reduce the productivity of forest ecosystems (Waring and Law, 2001; Ciais et al., 2005). Drought increases the leaf temperature differences among trees and accelerates plant transpiration, which could aggravate the effect of drought stress on plant growth. Severe drought could cause a lagging of vegetation growth, and the tree growth would slow down by 1–4 years (Anderegg et al., 2015a). The lagging response of forest vegetation productivity to drought is affected by the duration of drought and varies among various land types in different climate zones (Yu and Liu, 2019). The vegetation productivity of forests is less affected by drought in temperate regions than in tropical regions (Nemani et al., 2003; Xu et al., 2012). Besides inhibiting photosynthesis, drought also increases the frequency of forest mortality, wildfires, pests, and diseases, resulting in reduced productivity of forest ecosystems (Nepstad et al., 1999; Nepstad et al., 2004; Piao et al., 2019). Previous studies reported that severe drought in tropical forests increases flammability and tree death (Nepstad et al., 2004). Drought leads to a reduction in the productivity of forests, and more carbon will be emitted from forests to the atmosphere, which further accelerates global warming (Cox et al., 2000). Therefore, drought has been considered as the main meteorological disaster affecting the carbon balance of forest ecosystems, and severe and frequent drought events are the main cause of accelerated forest degradation and death (Lewis et al., 2011). Changes in vegetation net primary productivity (NPP) have a significant impact on the global climate and carbon cycling processes (Hazarika et al., 2005). NPP also provides the physical resources that are needed for human existence and development, such as food, fuel, and wood, and any slight modification to NPP could affect human output and livelihoods (Pritchard et al., 2018). Nearly half of worldwide large-scale ecosystem anomalies are caused by extreme climate events. In Europe, the severe drought and high temperatures in 2003 turned most terrestrial ecosystems into net carbon sources, which released the equivalent amount of carbon fixed in the previous four years back into the atmosphere (Ciais et al., 2005). In Southwest China, the drought occurred in 2009 and 2010 caused a significant decline in vegetation productivity, which would take more than a decade to recover (Xiong, 2013). China’s terrestrial ecosystem plays an important role in the global carbon cycle (Sun et al., 2021), particularly the subtropical southern China (Zhang et al., 2020). The regional carbon storage in the subtropical China’s forest area accounts for about 65% of the national total carbon stock (Li et al., 2003). The impact of regional ecosystem on environment and the response of regional ecosystem to climate change have been hot topics (Li et al., 2015; Yuan et al., 2017; Guo et al., 2020; Ji et al., 2022). However, few studies have specifically addressed the impacts of drought on vegetation productivity in the subtropical China, even though drought occurred frequently and have greatly affected the vegetation productivity (Zhang et al., 2017). It is important to study the drought impact on vegetation NPP in the subtropical China in order to provide a reference for estimating the carbon sink potential of China’s forests, which is important for the national carbon neutrality goal by 2060.
In recent years, with the rapid development of remote sensing technology, the vegetation index that is calculated based on the spectral characteristics of vegetation has been used to monitor vegetation changes, which makes it possible to analyze the vegetation growth response to drought on large spatial scales (Gitelson et al., 2002; Kerr and Ostrovsky, 2003). The number of studies on the effects of drought on vegetation productivity has been gradually increasing (Anderegg et al., 2015b; Anderegg et al., 2016; Camarero et al., 2021; Bauman et al., 2022); and the topics, methods, and depths of these studies have constantly been updated. In this study, our goal is to investigate the effects of drought on the NPP in the subtropical evergreen broad-leaved forests area in the southern China.
In this study, we hypothesized that vegetation NPP in the subtropical China could decline with increasing drought frequency, intensity, and duration. Our specific objectives were to: (1) characterize the spatiotemporal drought patterns using SPEI and scPDSI drought indices; (2) compare the impacts of different drought intensity and durations on vegetation NPP; (3) analyze the overall impacts of drought events on vegetation NPP in the subtropical China during 2000–2015. Our study could help guide the forest managers and local governments to rationally manage forests after droughts, and also provide data support for further identifying contribution of drought to ecosystem carbon fluxes in the subtropical China.
The subtropical China accounts for about a quarter of the national total land area. Geographically, the subtropical China is located from the south of the Qinling-Huaihe Line, to the north of the Tropic of Cancer, to the east of the boundary between the eastern slope of the Tibet Plateau and Yunnan province, and to the west of the Southeast Coast (Figure 1). This region has complex topography and landforms, including the middle and lower reaches of the Yangtze River plain, the Jiangnan (South of the Yangtze River) hills, Huaiyang Mountains, Qinling (Qin Mountains), Sichuan Basin, Yunnan-Guizhou Plateau, Nanling Mountains, and Taiwan Mountains. The elevation shows a declining trend from the west to the east, ranging from 1,000–2,000 m in the Hengduan Mountains and Yunnan-Guizhou Plateau to 200–500 m in the eastern hills.
Figure 1 The distributions of weather stations (the dotted points) and land cover types in the subtropical China. (A) is tropical forest, (B) is subtropical evergreen forest, (C) is alpine and subalpine meadow, (D) is warm temperate forest, (E) is temperate grassland, (F) is desert, (G) is temperate mixed forest, and (H) is cold temperate conifer forest.
According to Köppen-Geiger climate classification, the study region belongs to the humid subtropical climate zone (Beck et al., 2018). In the east, spring and summer are hot and rainy, winter is cool and slightly dry; while in the west, summer and autumn are rainy while winter and spring are dry. Heat resources declined from the south to the north. The annual mean ≥ 10 °C accumulated temperature ranges between 4,400–7,500 °C·day, and the annual mean temperature ranges between 15–21 °C. Annual precipitation shows a decline from east to the west, and from the south to the north. The annual precipitation ranges between 750–2,000 mm, with less than 1,000 mm in the north. Mean annual Frost-Free Period can reach 330 days in the south. Due to the differences in climatic resources, the vegetation varies substantially among different regions. Wet evergreen broad-leaved forests are dominant the east and subhumid evergreen broad-leaved forests are dominant in the west. The main tree genera in the study region include: Cinnamomum, Phoebe, Machilus, Schima, Cylobalanopsis, Castanopsis, and Lithocarpus.
The daily meteorological data for the 201 study stations (Figure 1) were gathered from China Meteorological Administration Data Center (http://data.cma.cn/) from 2000 to 2015, including maximum, minimum, and average temperatures, sunshine hours, and precipitation, etc. We used the standard sequence interpolation method (Woodruff et al., 1987) to interpolate the missing meteorological data with the standardized anomalies of the same climate element from neighbor stations. The missing value of climate element at time i for the station is calculated using
where Z represents the normalized sequence; j represents the neighboring station(s); Zavg represents the average of normalized sequence for the neighboring stations; and are the multi-year mean and standard deviation of the climate element at stationsss j, respectively; n is the number of neighboring stations; represents the value of the climate element to be interpolated at time i; and and are the multi-year mean and deviation of the climate element at time i for the station that needs interpolation, respectively.
NDVI images are required for NPP calculation in the CASA model. In this study, the NDVI data were downloaded from the MODIS China synthetic products of the Chinese Academy of Sciences Computer Network Information Center Geospatial Data Cloud Platform (http://www.gscloud.cn). We chose the monthly composite product of NDVI (with a resolution of 500 m) that is calculated based on MODND1 by taking the maximum daily value in each month in order to remove the effects of cloudy weather. A total of 192 NDVI images in Tagged Image File Format (TIFF) (with a pixel size of 0.0059 degrees) were collected from January 2000 to December 2015 for the analyses. We simply processed the downloaded data in ArcGIS software to remove or modify the abnormal NDVI images. The normal value range of NDVI is between 0 and 1, and the case of NDVI<1 was processed because it indicates ground cover of cloud, water, and snow. The case of NDVI=0 indicates ground cover of rocks or bare soil; it well represents the vegetation coverage and can be directly used in the calculation of NPP.
Land cover data are important input in the CASA model because they are used to compute the light energy conversion rate ϵ, the fraction of photosynthetically active radiation (FPAR), and other important factors. The raster data of land type maps, with a 1 km spatial resolution, used in this study were extracted from the spatial distribution data of land types in China provided by the Chinese Academy of Sciences Resources and Environmental Sciences Data Center (http://www.resdc.cn, accessed 6 March 2019). We categorized the land type into 22 groups (Figure 1), configured the adjustable parameters for the land types (Table 1), and then generated static-parameter files (Zhu et al., 2007).
Table 1 Configuration of adjustable parameters for the 22 study land types, including the maximum and minimum values of NDVI, the 95th and 5th percentiles of NDVI (SR_max and SR_min), and the maximum value of light energy utilization efficiency (ϵ) (Zhu et al., 2007).
The CASA model is based on remote sensing image data, and fully considers the internal physiological and ecological processes of vegetation. Its parameters are more easily obtained, so it can be generalized more easily than other large-scale NPP mechanistic models (Piao et al., 2001; Sun and Wang, 2012; Bao et al., 2016; Cao et al., 2016; Hadian et al., 2019; Su et al., 2022). In this study, we computed NPP (g C·m−2) in the CASA model as the product of the amount of photosynthetically active radiation that is absorbed by green vegetation (APAR) and the light energy use efficiency () of the radiation that is converted to plant biomass increment (Monteith, 1972; Xiao et al., 2004) as:
Here APAR can be computed by the total solar radiation with the fraction of photosynthetically active radiation (SR, MJ·m−2·a−1) that can be absorbed by green vegetation (FPAR, %) as:
and (g C·MJ−1) can be computed by the maximum of solar energy utilization efficiency (ϵmax) with the stress coefficient of high or low temperature (f(T)) and the stress coefficient of water (f(W)):
The calculation methods of SR, ϵmax, f(T), and f(W) are referred to (Zhu et al., 2007).
According to the regression equation the simulated NPP in the CASA model showed a good fit with the estimated NPP of previous studies in the subtropical evergreen broad-leaved forest area of China (Table S1), with a R2 of 0.6286 (p< 0.01) and a close to 1:1 trend line (Figure S3).
Drought has been one of the most serious disasters affecting human society. Studies on drought mainly focus on the spatial and temporal characteristics of drought indices during a certain period of time in a particular area. According to the statistics of the World Meteorological Organization, international scholars have brought up about 55 drought indices (Wmo and Gwp, 2016). However, no individual drought index can fully depict the drought characteristics among different areas. Therefore, it is essential to choose suitable drought indices in drought assessment and monitoring (Zhang et al., 2011; Li and Li, 2017). The early developed Standardized Precipitation Index (SPI) is solely based on the probability of monthly precipitation. Due to its relative simplicity in calculation and flexibility in temporal and spatial scales, SPI has been widely used in agricultural and ecological drought studies. It was proven that SPI could be used to effectively monitor drought in China (Xu et al., 2012; Pei et al., 2013; Zhang et al., 2020). On the basis of SPI, Vicente-Serrano et al. (2010a; 2010b) brought up the Standardized Precipitation Evapotranspiration Index (SPEI). SPEI retains the advantage of SPI for being suitable for multiple scales and also considers the temperature-sensitive characteristics of evapotranspiration. Due to its advanced features, SPEI has been commonly used in various aspects of drought studies (Ming et al., 2015; Alam et al., 2017).
The Palmer Drought Severity Index (PDSI) defines drought as a persistent abnormal water deficit (Newman, 1987). Though being widely used in international studies for half a century, PDSI has internal limitations: the definition of drought may cause a lag in identifying drought conditions; the parameter settings are determined based on the observation data in the Midwest of the United States, which many not be suitable in other climatic regions. Previous Chinese scholars tried to modify the calculation method of PDSI based on the observation data from Chinese meteorological stations but failed (Liu et al., 2004). Wells et al. (2004) proposed scPDSI to improve the empirical parameter problem in PDSI. Based on the observation data from a given station, scPDSI calculates the corresponding weight coefficient and persistence factor, which makes it superior in comparison among different areas.
In China, other drought indices like the percentage of precipitation anomalies, the Z index of precipitation, the relative humidity index, and the comprehensive drought index etc. were only used in a limited amount of studies due to the oversimplified calculation method or lack of data. Yang et al. (2017) compared the adaptability of seven drought indices in China and concluded that SPEI and scPDSI performed better than others did. Hence, we chose the two drought indices of SPEI and scPDSI in this study, and used the four classifications of D0 (abnormally dry), D1 (moderate dry), D2 (severe dry), and D3 (extreme dry) (Table 2) (Wang et al., 2016; Yao et al., 2021). We analyzed and compared the correlations and the similarities and differences in the drought class classification of two indicators in the subtropical China (Figure S3, S4). SPEI reflects the drought in a certain area by calculating the difference between precipitation and potential evapotranspiration (Zhai et al., 1999; Vicente-Serrano et al., 2010a).
The monthly PET was calculated by:
where Ti is the monthly average air temperature in month i (°C); H is the heat index, which is calculated as the sum of 12 monthly index values
And the monthly index values is derived from monthly average air temperature as the formula
A is the constant, which is calculated as a function of H:
When monthly average air temperature is less than or equal to zero, H is zero, and PET is zero.
So the monthly water surplus or deficit is measured by the difference between the precipitation and potential evapotranspiration as:
where Dj is the monthly water deficit in month j (mm); Pj is the monthly precipitation in month j (mm); and PETj is the monthly PET in monthly j (mm). Then the accumulated difference for one month in particular year I with a 12-month time scale is obtained by
Where is the cumulative difference in year i month j under timescale k; and Di,j is the monthly water deficit in year i and month j. Then, according to the log-logistic distribution, the probability distribution function of the D series is given by
Here α, β, and γ are scale, shape, and location parameters, which are obtained through linear moments fitting. Finally, SPEI is obtained by
where , When p > 0.5, p is replaced by (1−p) and SPEI is negative. = 2.515517, = 0.802853, = 0.010328, = 1.432788, = 0.189269, and = 0.001308. SPEI has different timescales (e.g., 1 month, 3 months, 6 months, and 12 months) and the 12-month timescale is analyzed in this study. scPDSI considers precipitation, air temperature, and soil moisture; it has been proved to perform well in evaluating the wet and dry conditions in the study region (Yang et al., 2017). The scPDSI data were acquired from the global monthly-scale scPDSI dataset product of the University of East Anglia (UEA) Climatic Research Unit (http://www.cru.uea.ac.uk, accessed 20 December 2019), with a 0.5 × 0.5 degrees spatial resolution. We then used ArcGIS software to resample, crop, and rasterize the acquired the 0.0059 × 0.0059 degrees spatial resolution scPDSI data for the analyses.
Based on the unary linear regression analysis, the spatiotemporal change rate of NPP and two drought indices were calculated:
Where slope is the change rate of the variable (NPP, SPEI, and scPDSI), is the number of years. And we use F-test to determine the significance of the change rate. If slope< 0 and p< 0.05, it indicates that NPP, or SPEI, scPDSI is showing a significant decreasing tendency. If slope > 0 and p< 0.05, meaning that it shows a significant increasing tendency, and vice versa. The detailed of the slope and F-test algorithms refer to the work of Gang et al. (2016).
In ArcGIS, we used the raster calculation tool to compute the Person correlation coefficient between NPP and the two drought indices of SPEI and scPDSI from 2000–2015 (Luo et al., 2020) and then exported the spatial distribution map. The correlation coefficient between NPP and the SPEI and scPDSI are calculated by
Where is the correlation coefficient between NPP and the drought index; and are the raster-level drought index and NPP values, respectively; N is the number of total study years.
NPP decrease rate varied under different drought intensities (Huang et al., 2016; Li et al., 2020). To analyze the effects of drought intensities and remove the compounding effects from NPP trends, we applied the change rates (CR) of NPP to represent the drought effects. The CR was calculated using below equation:
Where NPPi and NPPi-1 are the NPP of ith year and the previous year, respectively.
There have time-Lag effect of drought on Vegetation Productivity (Xu et al., 2020; Mairura et al., 2021; Gu et al., 2022). The annual maximum coefficient of correlation between NPP and SPEI or scPDSI can be used to measure how strongly vegetation is responding to drought. According to the work of Zhan et al. (2022), we analyzed the correlations between NPP and drought indices from 2000 to 2015 and defined the lagging in the NPP response to drought as when the maximum correlation ) occurred.
is the correlation coefficient at a scale where the maximum correlation coefficient between SPEI or scPDSI with NPP is found over the study period from 2000 to 2015. In addition, the lag time of drought-affected vegetation is influenced by water availability, which is 1–3 months, 5–7 months, and 10–12 months in arid, semi-arid, and sub-humid regions, respectively (Zhan et al., 2022). Meanwhile, according to Köppen-Geiger climate classification, the study region belongs to the humid subtropical climate zone (Beck et al., 2018). Based on the above-mentioned two considerations, the annual scales SPEI and scPDSI were used in the lagged effect analysis. If the correlation coefficient between SPEI or scPDSI of a certain year and NPP of the same year is the largest, then the delay is 0 years. If the correlation coefficient is the largest with the NPP in the following year, the delay is 1 year. If the correlation coefficient is the largest with the NPP in the following 2 years, it indicates a delay of 2 years, and so on.
According to previous work (Yu and Liu, 2019; Yu et al., 2019), drought events are divided into independent and continued drought events based on the drought duration. In order to exclude the interactive effects of independent drought events that occurred in a short period of time, we defined independent drought events in this study as when the year (SPEI<−0.5, or scPDSI<−1.0) before and the five consecutive years after are all normal years. Continuous drought events are defined as annual SPEI<−0.1 or annual scPDSI<−1.0 for more than two consecutive years.
In the subtropical China, the average SPEI ranged from −0.5 to 0.5 during the study period of 2000–2015, which reflected a normal water balance in the study region (Figure 2A). The change trend of SPEI ranged from −1.3 to 0.8 per decade. The eastern, southern, and northeastern parts showed a positive SPEI change trend, indicating a wetting tendency, while the remaining regions, especially the southwest, showed a negative SPEI trend, indicating a drying tendency (Figure 2C). Due to the unfavorable terrain and climate in the southwest, the drought frequency and intensity have been increasing during 2000–2015. The SD of SPEI ranged from 0.3 to 0.7, indicating that SPEI had a low variability at pixel levels. The SD of SPEI was the largest in the southwest, indicating a greater fluctuation of SPEI than other regions (Figure 2E).
Figure 2 Spatial distribution patterns for the mean (A), slope (C), SD (standard deviation) (E), and change rate significance level (G) of SPEI and the mean (B), slope (D), SD (F), and change rate significance level (H) of scPDSI during 2000–2015.
The average scPDSI ranged from −2.5 to 1.3, and the region was in an overall drought condition. The central and northern regions had a positive scPDSI, indicating a wet condition; while the remaining regions had a negative scPDSI, indicating a dry condition) (Figure 2B). The trends of scPDSI ranged from −4.8 to 2.6 per decade. The eastern, southern, and northwestern parts had a positive trend of scPDSI, indicating a wetting tendency, while the remaining parts, especially had a negative trend of scPDSI, indicating a drying tendency (Figure 2D). The SD of scPDSI ranged from 0.5 to 2.7, with relatively high values in the southwest, partial east, and partial south, indicating greater interannual variations in scPDSI in these regions (Figure 2F). The F-test results for both SPEI and scPDSI showed a significant increasing trend (significant wetting trend) in the northwestern parts, and a significant decreasing trend (significant drying trend) in the southwestern parts (Figures 2G, H).
The average annual NPP was 2,214 g C·m−2 in the subtropical China during 2000–2015. The spatial distribution of the mean NPP showed an obvious subregional pattern, with relatively high values in the Hengduan Mountains, Daba Mountains, Wuyi Mountains, and Nanling Mountains (Figure 3A). The change trend of NPP ranged from −24 to 760 g C·m−2 per decade. The northern part and the border between Yunnan and Guizhou showed a higher increasing trend. The southern part of the eastern humid region and the northern part of the western subhumid region generally showed a negative change trend, though the magnitude was small (Figure 3B). The SD of NPP was overall small, ranging from 33 to 179 g C·m−2.yr−1. High SD of NPP coincided with high average of NPP, indicating that NPP was relatively unstable in these areas (Figure 3C). The F-test results showed a significant increasing trend in southwestern parts (Guizhou) and the Middle and Lower reaches of the Yangtze River, and a significant decreasing trend in southern parts (especially in Guangxi) (Figure 3D).
Figure 3 Spatial distribution patterns of the mean (A), slope (B), SD (C), and change rate significance level (D) of NPP during 2000–2015.
Based on scPDSI, the four categories of drought intensity (D0, D1, D2, and D3) have caused a declined NPP by 3.2%, 4.8%, 11.0%, and 9.9%, respectively. The D2 drought intensity caused the greatest decrease in NPP, followed by D3, D1, and D0. Based on SPEI, the four categories of drought intensity (D0, D1, D2, and D3) have caused a declined NPP by 0.35%, 12.7%, 8.77%, and 0.0%, respectively. The greatest decrease in NPP was caused by D2, and D0 showed the smallest decrease. Combining the two drought indices, the mean decreasing rate in NPP was 7.2% for all four drought intensities (Table 3).
Based on SPEI, NPP did not significantly decrease in the independent drought year, a slight increase in the 1st year after drought, a larger decrease in the 2nd year after drought, and a small increase in the 3–5 years after drought. Based on scPDSI, NPP started to decrease in the 2nd year after independent drought events, and such decrease continued in the 3rd year after drought. NPP showed an increase in the 4th and 5th years after drought (Table 4). Overall, the NPP response to SPEI- and scPDSI-based drought durations showed a 2-year lagging time from the drought year. However, the numbers of independent drought events were few during 2000–2015, which might affect our drawn conclusions.
We further analyzed both 2-year and multi-year continuous drought events. NPP showed a decrease in the first normal year after the SPEI-based and scPDSI-based 2-year continuous drought events, with a declining rate of 64 and 65 g C·m−2.yr−1, respectively. NPP did not continue to decrease in the second normal year after the 2-year continuous drought, but increased instead (Table 5). NPP started to decrease two years after the beginning of the 2-year continuous drought, which was similar to the 2-year lagging for the independent drought. However, the 2-year continuous drought decreased NPP by more than the independent drought. The SPEI-based multi-year continuous drought rarely occurred during the study period. Based on scPDSI, multi-year continuous drought events caused 3–4 years of continuous decrease in NPP starting from the second year after drought, although the amplitude of decrease was declining over time. The decrease in NPP tended to level off in the fifth-to-sixth year after the multi-year continuous drought (Table 6). NPP is also affected by other non-drought factors that might offset the negative effects of drought, resulting in a long-term ecological balance in the study region.
The correlation coefficient between SPEI and NPP ranged from −0.173 to 0.999. The negative correlations mainly occurred in Guizhou (Figure 4A). The coefficient of variation (CV) of NPP is usually high in Guizhou, indicating a minimal response of NPP to drought. The correlation coefficient between scPDSI and NPP ranged from −0.276 to 0.999 (Figure 4C). At pixel level, the correlation coefficients between SPEI and NPP showed a similar spatial distribution pattern with the correlation coefficients between scPDSI and NPP, with 1–3 years of lagging in the southeast and a 3–4 years of lagging in the southwest. The areas with the strongest correlations between SPEI and NPP in the drought years only accounted for 7% of the total area of the study region. In 27%, 20%, and 16% of the study region, the strongest correlations between SPEI and NPP showed a lagging of 1 year, 2 years, and 3 years, respectively (Figure 4B). The areas with the strongest correlation between scPDSI and NPP in the drought year only accounted for 8% of the total area of the study region. 29% of the study region showed the strongest correlations between scPDSI and NPP in a 2-year lagging, followed by a 1-year lagging (23%), and then the 3-year lagging (11%) (Figure 4D). Overall, the strongest correlations between the drought indices and NPP in the drought year only occurred in about 7–8% of the study region. By contrast, about 64% of the study region showed the strongest correlations between drought indices and NPP in the first-to-third years after drought (Figure 5D). This indicated that the response of NPP to SPEI and scPDSI had a 1–3-years of lagging in the study region. Based on both drought indices, the greatest positive (negative) correlation coefficient between NPP and drought indices was 0.999 (0.112). The negative correlations between NPP and drought indices were mostly located in the Guizhou. NPP showed a higher correlation coefficient with SPEI (scPDSI) in 49% (51%) of the study region. The strongest correlation between drought indices and NPP in the drought year occurred in 7% of the study region. 25%, 24%, and 14% of the study region showed a 1-year, 2-years, and 3-years of lagging in the strongest correlation between drought indices and NPP, respectively (Figures 4E, F).
Figure 4 The maximum correlation coefficients between NPP and SPEI (A), the lagging time of the strongest correlations between NPP and SPEI (B), the maximum correlation coefficients between NPP and scPDSI (C), the lagging time of the strongest correlations between NPP and scPDSI (D), the relatively greater maximum correlation coefficient between NPP vs. SPEI and NPP vs. scPDSI (E), and the lagging time of the relatively stronger correlation between NPP vs. SPDI and NPP vs. scPDSI (F) during the period of 2000–2015 in the study region. Note: 0, 1, 2, 3, 4 and 5 denote the lagging years after a detected drought event.
Figure 5 Percentages of area with lagging time (unit: year) for the maximum correlation coefficient between NPP and drought indices to the area of the total study region for selected vegetation types. (A) Evergreen coniferous forests. (B) Evergreen broad-leaved forests. (C) Deciduous broad-leaved forests. (D) Shrubs. (E) Sparse forests. (F) Grassland (including alpine and subalpine meadows, sloping grassland, plain grassland, desert grassland, meadows, and alpine and subal-pine grassland).
In addition, we compared the maximum correlation coefficient between NPP and drought indices among the 22 land types in the study region. In the evergreen coniferous forests (evergreen broad-leaved forests), the strongest correlation between NPP and drought indices showed a 1-year and 2-years of lagging in 32% and 28% (36% and 24%) of the study vegetation area, respectively (Figures 5A, B). In the deciduous broad-leaved forests, 55% of the vegetation area showed a 2-years of lagging for the maximum correlation coefficient between NPP and drought indices, followed by the 1-year of lagging in 30% of the vegetation area (Figure 5C). In the shrubs, the area with 1-year and 2-years of lagging in the maximum correlation coefficient between NPP and drought indices accounted for 27% and 23% of the vegetation area, respectively (Figure 5D). In the sparse forests, NPP was more sensitive to drought indices than any other land type was, with 23% of the vegetation area showing the maximum correlation coefficient between NPP and drought indices in the drought year. 35% of the sparse forests showed a 1-year of lagging for the maximum correlation coefficient between NPP and drought indices (Figure 5E). In a variety of grassland, 39% of the subtotal vegetation area showed a 2-years of lagging for the maximum correlation coefficient between NPP and drought indices, followed by the 1-year of lagging in 22% of the subtotal vegetation area (Figure 5F).
Many people have analyzed the impact of drought on NPP, NDVI or GPP, but most of them only use SPEI (Liu et al., 2021), or PDSI (Zhang et al., 2019), and do not use the two together to study the impact of drought on NPP. scPDSI is the modified PDSI, which considers the issues of precipitation, air temperature, and soil water content, and describes the problem of soil drought. SPEI considers rainfall and potential evapotranspiration and focuses more on the problem of describing atmospheric drought (Xu et al., 2021). Some studies have pointed out that vegetation growth is not only affected by rainfall and air temperature, but also increasingly dependent on soil moisture (Girardin et al., 2016). To this end, this study used these two indicators to analyze and compare the impact of drought on NPP in southern China. Our results showed that the subtropical China were overall in a dry condition (Figures S1, S2). The SPEI- and scPDSI-based drought condition was consistent with the historical drought condition in 2004. Starting from July 2003, the southern China was in a severe drought condition that continued throughout the rest of the year; most areas had severe water loss, some areas had extreme water shortages, and the water deficit kept worsening. The drought-affected area included many provinces/autonomous regions (e.g., Zhejiang, Fujian, Jiangxi, Hubei, Hu’nan, Guangdong, Guangxi, Guizhou, and Yunnan etc.), both the drought coverage and drought intensity were historically the worst since 1963. This find is consistent with the study of Bai et al. (2010). In addition, we found that scPDSI showed similar spatial distribution patterns to SPEI. However, scPDSI-based drought could be more severe (with extreme drought) than SPEI-based drought (Figures S1, S2, S4). This result is consistent with (Zhao et al., 2017).
However, whether it is the drought reported by SPEI or scPDSI, like others work (Li et al., 2015; Zhou et al., 2018; Zhang et al., 2019; Lei et al., 2020; Zhang et al., 2022), drought has resulted in a decline in NPP. But small NPP did not necessarily mean there was a drought event, and large NPP did not necessarily mean that the area was wet. It was not reasonable to fit NPP with the station-level drought indices alone. NPP was concentrated in 400–700 g C·m−2, with the corresponding SPEI showing moderate wetness to moderate drought. On the other hand, SPEI was concentrated in −0.5–0.5, with the corresponding NPP ranging from low to high (Figures 2, 3). These results indicated that the spatial distribution of NPP was not directly corresponding to the drought indices (Lei et al., 2020; Li et al., 2020). Therefore, it is necessary to explore the relationship between temporal changes in NPP and temporal changes in drought indices (Huang et al., 2016). It is also important to factor in the lagging response of NPP to drought types (independent or continued) among various land types. In general, NPP showed a lagging response to drought; the NPP of vegetation in the study region started to decrease about 1–2 years after drought occurrence. This result is similar to the work of Yu and Liu (2019).
As for the drought intensity, NPP was reduced the most by moderate drought. Temperature is a critical factor affecting vegetation growth and development, and drought only shows the greatest impact on vegetation growth when the temperature is within a certain range (Shi, 2019). Drought duration also affected the changes in NPP; the longer the drought lasted, the longer it affected NPP. The changes in NPP tended to ease up around the 5th–6th year after drought occurrence. This could be explained by: 1) the actual continued drought events might differ from the drought indices-based drought events; 2) NPP is also affected by other non-drought factors, the negative effects of drought on NPP might be gradually offset by the positive effects of other ecological factors on NPP, resulting in a non-significant change trend in NPP in the study region. NPP was negatively correlated with the two study drought indices in Guizhou. Guizhou has severe surface weathering due to the effect of Karst, coupled with man-made damage and disturbance, the ecological risk in Guizhou is fairly high, resulting in, unstable NPP (with a high coefficient of variation), and poor response of NPP to drought.
In this study, the 16-year study period was too short to reveal any significant trends in NPP and the drought indices (which usually recalls at least 30-years of data). Besides drought events, there are many other factors that could affect NPP, including temperature- and precipitation-related meteorological disasters (Fu et al., 2018; Zhang et al., 2021; Fu and Sun, 2022; Han et al., 2023), plant phenology (Fu and Shen, 2022), human activities, topography, terrain, slope, and aspect etc. Therefore, we suggest future studies to extend the study period and consider more factors to comprehensively analyze the response of NPP to drought.
Based on the meteorological data from 2000–2015, the CASA model was applied to compute vegetation NPP in the subtropical China. According to the correlation analyses between SPEI/scPDSI and NPP, we evaluated the NPP response to drought intensity and drought frequency. On average, the SPEI-based D0, D1, and D2 reduced the regional NPP by 0.35%, 12.72%, and 8.77%, respectively; the scPDSI-based D0, D1, D2, and D3 reduced the regional NPP by 3.18%, 4.78%, 11.04%, and 9.87%, respectively. The SPEI- and scPDSI-based drought reduced NPP by a composite amplitude of 7.20%. The greatest reduction in NPP was caused by the moderate or severe drought instead of extreme drought. Abnormally dry condition showed the least negative effect on NPP in the study region.
The duration of drought events, whether independent or continued, also affected the change in NPP. Both SPEI- and scPDSI-based independent drought started to affect NPP two years after the occurrence, indicating a 2-years of lagging NPP response to independent drought. There was a 1-year of lagging in the NPP response to SPEI- and scPDSI-based two-year continued drought, with an amplitude of 64 and 65 g C·m−2, respectively. The 2-year continued drought reduced NPP by more than the independent drought did. The multi-year continued drought showed an even greater effect on NPP: NPP started to decrease in the second year after drought occurrence; the decrease of NPP lasted for about 3–4 years; and the decreasing amplitude of NPP was relatively large at the beginning but then gradually declined.
The original contributions presented in the study are included in the article/Supplementary Material. Further inquiries can be directed to the corresponding author.
Conceptualization, QY and WF; methodology, QY and WF; software, QY; validation, WF and GJ; formal analyse, QY and WF; investigation, QY, WF, and G.J.; resources, QY and WD; data curation, QY and WF; writing—original draft preparation, QY and GJ; writing—review and editing, WF, GJ, and WD; project administration, QY and WD; funding acquisition, QY and WD. All authors contributed to the article and approved the submitted version.
This research was funded by the National Natural Science Foundation of China, grant number 31860236.
The authors declare that the research was conducted in the absence of any commercial or financial relationships that could be construed as a potential conflict of interest.
All claims expressed in this article are solely those of the authors and do not necessarily represent those of their affiliated organizations, or those of the publisher, the editors and the reviewers. Any product that may be evaluated in this article, or claim that may be made by its manufacturer, is not guaranteed or endorsed by the publisher.
The Supplementary Material for this article can be found online at: https://www.frontiersin.org/articles/10.3389/fevo.2023.1181332/full#supplementary-material
Alam N. M., Sharma G. C., Moreira E., Jana C., Mishra P. K., Sharma N. K., et al. (2017). Evaluation of drought using SPEI drought class transitions and log-linear models for different agro-ecological regions of India. Phys. Chem. Earth Parts a/B/C 100, 31–43. doi: 10.1016/j.pce.2017.02.008
Anderegg W. R. L., Hicke J. A., Fisher R. A., Allen C. D., Aukema J., Bentz B., et al. (2015a). Tree mortality from drought, insects, and their interactions in a changing climate. New Phytol. 208, 674–683. doi: 10.1111/nph.13477
Anderegg W. R. L., Klein T., Bartlett M., Sack L., Pellegrini A. F. A., Choat B., et al. (2016). Meta-analysis reveals that hydraulic traits explain cross-species patterns of drought-induced tree mortality across the globe. Proc. Natl. Acad. Sci. 113, 5024–5029. doi: 10.1073/pnas.1525678113
Anderegg W. R. L., Schwalm C., Biondi F., Camarero J. J., Koch G., Litvak M., et al. (2015b). Pervasive drought legacies in forest ecosystems and their implications for carbon cycle models. Science 349, 528. doi: 10.1126/science.aab1833
Bai Y., Zhi X., Qi H., Zhang L. (2010). Severe drought monitoring in south China based on the standardized precipitation index at different scales. Scientia Meteorologica Sinca 30, 292–300. doi: 10.3969/j.issn.1009-0827.2010.03.002
Bao G., Bao Y., Qin Z., Xin X., Bao Y., Bayarsaikan S., et al. (2016). Modeling net primary productivity of terrestrial ecosystems in the semi-arid climate of the Mongolian Plateau using LSWI-based CASA ecosystem model. Int. J. Appl. Earth Obs Geoinf 46, 84–93. doi: 10.1016/j.jag.2015.12.001
Bauman D., Fortunel C., Delhaye G., Malhi Y., Cernusak L. A., Bentley L. P., et al. (2022). Tropical tree mortality has increased with rising atmospheric water stress. Nature. 608, 528–533. doi: 10.1038/s41586-022-04737-7
Beck H. E., Zimmermann N. E., Mcvicar T. R., Vergopolan N., Berg A., Wood E. F., et al. (2018). Present and future Köppen-Geiger climate classification maps at 1-km resolution. Sci. Data 5, 180214.
Camarero J. J., Gazol A., Sangüesa-Barreda G., Vergarechea M., Alfaro-Sánchez R., Cattaneo N., et al. (2021). Tree growth is more limited by drought in rear-edge forests most of the times. For Ecosyst. 8, 25. doi: 10.1186/s40663-021-00303-1
Cao S., Sanchez-Azofeifa G. A., Duran S. M., Calvo-Rodriguez S. (2016). Estimation of aboveground net primary productivity in secondary tropical dry forests using the Carnegie–Ames–Stanford approach (CASA) model. Environ. Res. Lett. 11, 75004. doi: 10.1088/1748-9326/11/7/075004
Ciais P., Reichstein M., Viovy N., Granier A., Ogée J., Allard V., et al. (2005). Europe-wide reduction in primary productivity caused by the heat and drought in 2003. Nature 437, 529–533. doi: 10.1038/nature03972
Cox P. M., Betts R. A., Jones C. D., Spall S. A., Totterdell I. J. (2000). Acceleration of global warming due to carbon-cycle feedbacks in a coupled climate model. Nature 408, 184–187. doi: 10.1038/35041539
Fu G., Shen Z. (2022). Asymmetrical warming of growing/non-growing season increases soil respiration during growing season in an alpine meadow. Sci. Total Environ. 812, 152591. doi: 10.1016/j.scitotenv.2021.152591
Fu G., Shen Z., Zhang X. (2018). Increased precipitation has stronger effects on plant production of an alpine meadow than does experimental warming in the Northern Tibetan Plateau. Agric. Meteorol 249, 11–21. doi: 10.1016/j.agrformet.2017.11.017
Fu G., Sun W. (2022). Temperature sensitivities of vegetation indices and aboveground biomass are primarily linked with warming magnitude in high-cold grasslands. Sci. Total Environ. 843, 157002. doi: 10.1016/j.scitotenv.2022.157002
Gang C., Wang Z., Zhou W., Chen Y., Li J., Chen J., et al. (2016). Assessing the spatiotemporal dynamic of global grassland water use efficiency in response to climate change from 2000 to 2013. J. Agron. Crop Sci. 202, 343–354. doi: 10.1111/jac.12137
Girardin M. P., Bouriaud O., Hogg E. H., Kurz W., Zimmermann N. E., Metsaranta J. M., et al. (2016). No growth stimulation of Canada’s boreal forest under half-century of combined warming and CO2 fertilization. Proc. Natl. Acad. Sci. 113, E8406–E8414. doi: 10.1073/pnas.1610156113
Gitelson A. A., Kaufman Y. J., Stark R., Rundquist D. (2002). Novel algorithms for remote estimation of vegetation fraction. Remote Sens Environ. 80, 76–87. doi: 10.1016/S0034-4257(01)00289-9
Gu X., Guo E., Yin S., Wang Y., Mandula N., Wan Z., et al. (2022). Differentiating cumulative and lagged effects of drought on vegetation growth over the Mongolian Plateau. Ecosphere 13. doi: 10.1002/ecs2.4289
Guo B., Zang W., Yang F., Han B., Chen S., Liu Y., et al. (2020). Spatial and temporal change patterns of net primary productivity and its response to climate change in the Qinghai-Tibet Plateau of China from 2000 to 2015. J. Arid Land 12, 1–17. doi: 10.1007/s40333-019-0070-1
Hadian F., Jafari R., Bashari H., Tartesh M., Clarke K. D. (2019). Estimation of spatial and temporal changes in net primary production based on Carnegie Ames Stanford Approach (CASA) model in semi-arid rangelands of Semirom County, Iran. J. Arid Land 11, 477–494. doi: 10.1007/s40333-019-0060-3
Han F., Yu C., Fu G. (2023). Non-growing/growing season non-uniform-warming increases precipitation use efficiency but reduces its temporal stability in an alpine meadow. Front. Plant Sci. 14, 1090204. doi: 10.3389/fpls.2023.1090204
Hazarika M. K., Yasuoka Y., Ito A., Dye D. (2005). Estimation of net primary productivity by integrating remote sensing data with an ecosystem model. Remote Sens Environ. 94, 298–310. doi: 10.1016/j.rse.2004.10.004
Huang L., He B., Chen A., Wang H., Liu J., Lű A., et al. (2016). Drought dominates the interannual variability in global terrestrial net primary production by controlling semi-arid ecosystems. Sci. Rep. 6, 24639. doi: 10.1038/srep24639
Ji S., Ren S., Li Y., Fang J., Zhao D., Liu J. (2022). The response of net primary productivity to climate change and its impact on hydrology in a water-limited agricultural basin. Environ. Sci. pollut. Res. Int. 29, 10277–10290. doi: 10.1007/s11356-021-16458-x
Kerr J. T., Ostrovsky M. (2003). From space to species: ecological applications for remote sensing. Trends Ecol. Evol. 18, 299–305. doi: 10.1016/S0169-5347(03)00071-5
Lei T., Feng J., Lv J., Wang J., Song H., Song W., et al. (2020). Net Primary Productivity Loss under different drought levels in different grassland ecosystems. J. Environ. Manage 274, 111144. doi: 10.1016/j.jenvman.2020.111144
Lewis S. L., Brando P. M., Phillips O. L., van der Heijden G. M. F., Nepstad D. (2011). The 2010 amazon drought. Science 331, 554. doi: 10.1126/science.1200807
Li Y., Li Y. (2017). Advances in adaptability of meteorological drought indices in China. J. Arid Meteorology 35, 709–723. doi: 10.11755/j.issn.1006-7639(2017)-05-0709
Li S., Lü S., Zhang Y., Gao Y., Ao Y. (2015). The change of global terrestrial ecosystem net primary productivity (NPP) and its response to climate change in CMIP5. Theor. Appl. Climatol 121, 319–335. doi: 10.1007/s00704-014-1242-8
Li K., Wang S., Cao M. (2003). Carbon storage of vegetation and soil in terrestrial ecosystems of China. Sci. China (Series D) 33, 72–80. doi: 10.3969/j.issn.1674-7240.2003.01.008
Li J., Wang Z., Lai C. (2020). Severe drought events inducing large decrease of net primary productivity in mainland China during 1982–2015. Sci. Total Environ. 703, 135541. doi: 10.1016/j.scitotenv.2019.135541
Liu W., An S., Liu G., Guo A. (2004). The farther modification of Palmer drought severity model. J. Appl. Meteorological Sci. 15, 207–216. doi: 10.3969/j.issn.1001-7313.2004.02.009
Liu Y., Zhou R., Wen Z., Khalifa M., Zheng C., Ren H., et al. (2021). Assessing the impacts of drought on net primary productivity of global land biomes in different climate zones. Ecol. Indic 130, 108146. doi: 10.1016/j.ecolind.2021.108146
Luo X., Li Y., Yin H., Sui M. (2020). Response of NDVI to SPEI at different temporal scales in Northeast China. Chinaese J. Ecol. 39, 412–421. doi: 10.13292/j.1000-4890.202002.025
Mairura F. S., Musafiri C. M., Kiboi M. N., Macharia J. M., Ng'Etich O. K., Shisanya C. A., et al. (2021). Determinants of farmers' perceptions of climate variability, mitigation, and adaptation strategies in the central highlands of Kenya. Weather Clim Extrem 34, 100374. doi: 10.1016/j.wace.2021.100374
Ming B., Guo Y. Q., Tao H. B., Liu G. Z., Shao-Kun L. I., Wang P. (2015). SPEIPM-based research on drought impact on maize yield in North China Plain. J. Integr. Agric. 14, 660–669. doi: 10.1016/S2095-3119(14)60778-4
Monteith J. L. (1972). Solar radiation and productivity in tropical ecosystems. J. Appl. Ecol. 9, 747. doi: 10.2307/2401901
Nemani R. R., Keeling C. D., Hashimoto H., Jolly W. M., Piper S. C., Tucker C. J., et al. (2003). Climate-driven increases in global terrestrial net primary production from 1982 to 1999. Science 300, 1560. doi: 10.1126/science.1082750
Nepstad D., Lefebvre P., Lopes Da Silva U., Tomasella J., Schlesinger P., Solórzano L., et al. (2004). Amazon drought and its implications for forest flammability and tree growth: a basin-wide analysis. Glob Chang Biol. 10, 704–717. doi: 10.1111/j.1529-8817.2003.00772.x
Nepstad D. C., Verssimo A., Alencar A., Nobre C., Lima E., Lefebvre P., et al. (1999). Large-scale impoverishment of Amazonian forests by logging and fire. Nature 398, 505–508. doi: 10.1038/19066
Pei F., Li X., Liu X., Lao C. (2013). Assessing the impacts of droughts on net primary productivity in China. J. Environ. Manage 114, 362–371. doi: 10.1016/j.jenvman.2012.10.031
Piao S., Fang J., Guo Q. (2001). Application of CASA model to the estimation of Chinese terrestrial net primary productivity. Acta Phytoecologica Sin. 25, 603. doi: 10.1088/0256-307X/18/11/313
Piao S., Zhang X., Chen A., et al. (2019). The impacts of climate extremes on the terrestrial carbon cycle: A review. Sci. China Earth Sci. 62, 1551–1563. doi: 10.1007/s11430-018-9363-5
Pritchard R., Ryan C. M., Grundy I., van der Horst D. (2018). Human appropriation of net primary productivity and rural livelihoods: Findings from six villages in Zimbabwe. Ecol. Econ 146, 115–124. doi: 10.1016/j.ecolecon.2017.10.003
Shi S. (2019). Temporal and spatial variation of meteorological drought and vegetation vulnerability to drought on Loess Plateau (Beijing: Chinese Academy of Sciences and Ministry of Education).
Su S., Zeng Y., Zhao D., Zheng Z., Wu X. (2022). Optimization of net primary estimation model for terrestrial vegetation in China based on CERN data. Acta Ecologica Sin. 42, 1276–1289. doi: 10.5846/stxb202011263031
Sun R., Chen S., Su H. (2021). Climate dynamics of the spatiotemporal changes of vegetation NDVI in Northern China from 1982 to 2015. Remote Sens (Basel) 13, 187. doi: 10.3390/rs13020187
Sun S., Wang R. (2012). Estimation and characteristics analysis of artificial vegetation biomass based on CASA models. For. Resour. Manage. 0, 61–66. doi: 10.3969/j.issn.1002-6622.2012.06.014
Vicente-Serrano S. M., Beguería S., López-Moreno J. I. (2010b). A multiscalar drought index sensitive to global warming: the standardized precipitation evapotranspiration index. J. Clim 23, 1696–1718. doi: 10.1175/2009JCLI2909.1
Vicente-Serrano S. M., Beguería S., López-Moreno J. I., Angulo M., El Kenawy A. (2010a). A new global 0.5° Gridded dataset, (1901–2006) of a multiscalar drought index: comparison with current drought index datasets based on the palmer drought severity index. J. Hydrometeorol 11, 1033–1043. doi: 10.1175/2010JHM1224.1
Wang Z., Li J., Huang Z., Zhong R., Chen J., Qiu Z. (2016). Spatiotemporal variations analysis of meteorological drought in China based on scPDSI. Trans. Chin. Soc. Agric. Eng. (Transactions Csae) 32, 161–168. doi: 10.11975/j.issn.1002-6819.2016.02.024
Waring R. H., Law B. E. (2001). The ponderosa pine ecosystem and environmental stress: past, present and future. Tree Physiol. 21, 273–274. doi: 10.1093/treephys/21.5.273
Wells N., Goddard S., Hayes M. J. (2004). A self-calibrating palmer drought severity index. J. Clim 17, 2335–2351. doi: 10.1175/1520-0442(2004)017<2335:ASPDSI>2.0.CO;2
Wmo and Gwp (2016). “Handbook of Drought Indicators and Indices,” in Integrated Drought Management Programme (IDMP), Integrated Drought Management Tools and Guidelines Series 2. Eds. Svoboda M., Fuchs B. A.(Geneva: WMO).
Woodruff S. D., Slutz R. J., Jenne R. L., Steurer P. M. (1987). A comprehensive ocean-atmosphere data set. Bull. Am. Meteorol Soc. 68, 1239–1250. doi: 10.1175/1520-0477(1987)068<1239:ACOADS>2.0.CO;2
Xiao X., Hollinger D., Aber J., Goltz M., Davidson E. A., Zhang Q., et al. (2004). Satellite-based modeling of gross primary production in an evergreen needleleaf forest. Remote Sens Environ. 89, 519–534. doi: 10.1016/j.rse.2003.11.008
Xiong G. (2013). Studies on characteristics and causes of drought climate change with different time scales in Southwest China in the past 50 Years (Lanzhou: Lanzhou University).
Xu F., Chen S., Wang L., Ye Q., Xing J., Peng L., et al. (2020). Effects of drought on radial growth of Cryptomoria japonica. Acta Agriculturae Universitatis Jiangxiensis 42, 811–820. doi: 10.13836/j.jjau.2020092
Xu X., Piao S., Wang X., Chen A., Ciais P., Myneni R. B. (2012). Spatio-temporal patterns of the area experiencing negative vegetation growth anomalies in China over the last three decades. Environ. Res. Lett. 7, 35701. doi: 10.1088/1748-9326/7/3/035701
Xu H., Wang X., Zhao C., Shan S., Guo J. (2021). Seasonal and aridity influences on the relationships between drought indices and hydrological variables over China. Weather Clim Extrem 34, 100393. doi: 10.1016/j.wace.2021.100393
Yang Q., Li M., Zheng Z., Ma Z. (2017). Regional applicability of seven meteorological drought indices in China. Sci. China Earth Sci. 47, 337–353. doi: 10.1007/s11430-016-5133-5
Yao J., Mao W., Chen J., Dilinuer T. (2021). Recent signal and impact of wet-to-dry climatic shift in Xinjiang, China. J. Geogr. Sci. 31, 1283–1298. doi: 10.1007/s11442-021-1898-9
Yu C., Liu D. (2019). Response in productivity of natural vegetation to drought in northeast China based on MODIS. Acta Ecologica Sin. 39, 3978–3990. doi: 10.5846/stxb201803300655
Yu C., Liu D., He F., Han J. (2019). Dynamic characteristics of natural vegetation fires and their response to drought in Northeast China. Bull. Soil Water Conserv. 39, 9–16. doi: 10.13961/j.cnki.stbctb.2019.04.002
Yuan Q., Wu S., Dai E., Zhao D., Ren P., Zhang X. (2017). NPP vulnerability of the potential vegetation of China to climate change in the past and future. J. Geogr. Sci. 27, 131–142. doi: 10.1007/s11442-017-1368-6
Zhai P., Sun A., Ren F., Liu X., Gao B., Zhang Q. (1999). Changes of climate extremes in China. Clim Change 42, 203–218. doi: 10.1023/A:1005428602279
Zhan C., Liang C., Zhao L., Jiang S., Niu K., Zhang Y. (2022). Drought-related cumulative and time-lag effects on vegetation dynamics across the Yellow River Basin, China. Ecol. Indic 143, 109409. doi: 10.1016/j.ecolind.2022.109409
Zhang S., Cui Y., Fu S., Fu Y., Liu X., Tang X., et al. (2020). Modeling greenhous gas sequestration potential of forest change in China. Acta Ecologica Sin. 40, 1140–1149. doi: 10.5846/stxb201812312853
Zhang L., Du H., Xia J., Xu X. (2011). Progress in the study of extreme hydrologic events under climate change. Prog. Geogr. 30, 1370–1379. doi: 10.11820/dlkxjz.2011.11.006
Zhang Z., Ju W., Zhou Y., Li X. (2022). Revisiting the cumulative effects of drought on global gross primary productivity based on new long-term series data, (1982–2018). Glob Chang Biol. 28, 3620–3635. doi: 10.1111/gcb.16178
Zhang G., Shen Z., Fu G. (2021). Function diversity of soil fungal community has little exclusive effects on the response of aboveground plant production to experimental warming in alpine grasslands. Appl. Soil Ecol. 168, 104153. doi: 10.1016/j.apsoil.2021.104153
Zhang Q., Yao Y., Wang Y., Wang S., Wang J., Yang J., et al. (2017). Risk characteristics and control technology measures of drought disaster in Southern China. Acta Ecologica Sin. 37, 7206–7218. doi: 10.5846/stxb201608111644
Zhang Z., Zhong R., Li X., Sun H. (2019). Change of NPP and its response to drought in Northwest China. Res. Environ. Sci. 32, 431–439. doi: 10.13198/j.issn.1001-6929.2018.07.17
Zhao H., Gao G., An W., Zou X., Li H., Hou M. (2017). Timescale differences between SC-PDSI and SPEI for drought monitoring in China. Phys. Chem. Earth Parts a/B/C 102, 48–58. doi: 10.1016/j.pce.2015.10.022
Zhou L., Wang S., Chi Y., Wang J. (2018). Drought impacts on vegetation indices and productivity of terrestrial ecosystems in Southwestern China during 2001–2012. Chin. Geogr. 28, 784–796. doi: 10.1007/s11769-018-0967-1
Keywords: net primary productivity (NPP), drought, SPEI, scPDSI, CASA model, the subtropical China
Citation: Ye Q, Feng W, Jiao G and Deng W (2023) Drought characteristics and their impacts on vegetation net primary productivity in the subtropical China. Front. Ecol. Evol. 11:1181332. doi: 10.3389/fevo.2023.1181332
Received: 07 March 2023; Accepted: 10 July 2023;
Published: 28 July 2023.
Edited by:
Changchun Huang, Nanjing Normal University, ChinaReviewed by:
Gang Fu, Chinese Academy of Sciences (CAS), ChinaCopyright © 2023 Ye, Feng, Jiao and Deng. This is an open-access article distributed under the terms of the Creative Commons Attribution License (CC BY). The use, distribution or reproduction in other forums is permitted, provided the original author(s) and the copyright owner(s) are credited and that the original publication in this journal is cited, in accordance with accepted academic practice. No use, distribution or reproduction is permitted which does not comply with these terms.
*Correspondence: Qing Ye, eWVxaW5nQGp4YXUuZWR1LmNu
Disclaimer: All claims expressed in this article are solely those of the authors and do not necessarily represent those of their affiliated organizations, or those of the publisher, the editors and the reviewers. Any product that may be evaluated in this article or claim that may be made by its manufacturer is not guaranteed or endorsed by the publisher.
Research integrity at Frontiers
Learn more about the work of our research integrity team to safeguard the quality of each article we publish.