- 1School of Science, Engineering and Environment, University of Salford, Salford, United Kingdom
- 2Institute for Biology, Freie Universität Berlin, Berlin, Germany
- 3Division for Small Animal Internal Medicine, University of Veterinary Medicine Vienna, Vienna, Austria
Life emerged in an anoxic world, but the release of molecular oxygen, the by-product of photosynthesis, forced adaptive changes to counteract its toxicity. However, reactive oxygen species can damage all cellular components, including proteins. Therefore, several mechanisms have evolved to balance the intracellular redox state and maintain a reductive environment more compatible with many essential biological functions. In this study, we statistically interrogated the amino acid composition of E. coli proteins to investigate how the proneness or susceptibility to oxidation of amino acids biased their sequences. By sorting the proteins into five compartments (cytoplasm, internal membrane, periplasm, outer membrane, and extracellular), we found that various oxidative lesions constrain protein composition and depend on the cellular compartments, impacting the evenness of distribution or frequency. Our findings suggest that oxidative susceptibility could influence the observed differences in amino acid abundance across cellular compartments. This result reflects how the oxidative atmosphere could restrict protein amino acid composition and impose a codon bias trend.
Introduction
The origin of life and the pristine molecular evolution is still a conundrum regarding several questions about the changes that occurred at the molecular and cellular levels to adapt to higher molecular oxygen concentrations (Lyons et al., 2014). During long evolving processes, protein amino acid compositions were influenced by their functionalities (Tourasse and Li, 2000), stability (Godoy-Ruiz et al., 2004; Mendez et al., 2010), energy efficiency (Akashi and Gojobori, 2002; Smith and Chapman, 2010), and their ability to create secondary structures (Lu and Freeland, 2006). This process involved a dynamic mutation-selection game that produced specific combinations of amino acid sequences (Knight et al., 2001). Proteins represent the ultimate product of the genetic flow of information, and their functions are ultimately determined by the amino acid sequence. However, these functions must be in harmony with biological complexity, which is heavily influenced by environmental conditions (Worth et al., 2009).
Life originally evolved in an anaerobic and reductive atmosphere, but the emergence of oxygen through photosynthesis changed the environment and forced microbes to alter their physiology to cope with oxidative conditions (Cavalier-Smith, 2006; Cavalier-Smith et al., 2006). Oxygen and its reactive species (ROS) can damage all cellular components, including lipids, nucleic acids, and proteins. ROS encompass a group of highly reactive molecules that include the hydroxyl radical (·OH), superoxide radical (·O2), singlet oxygen (1O2), hydrogen peroxide (H2O2), hypochlorous acid (HOCl), and peroxynitrite (ONOO−). These species can be generated during normal cellular metabolism or in response to external factors like radiation or pollutants (Anbar, 2008; Imlay, 2015). Inside the cell, the cytoplasm is kept under reductive conditions due to evolved systems that control ROS. The primary cellular anti-ROS defence systems include enzymatic antioxidants and non-enzymatic antioxidants. Enzymatic antioxidants such as superoxide dismutase (SOD), catalase, and glutathione peroxidase (GPx) work collaboratively to neutralise ROS. SOD converts superoxide radicals into hydrogen peroxide, which is further detoxified by catalase and GPx. GPx also directly utilises reduced glutathione (GSH) to scavenge hydrogen peroxide and lipid peroxides. Non-enzymatic antioxidants such as vitamin C, vitamin E, and glutathione (GSH) act as ROS scavengers by donating electrons or hydrogen atoms, thereby preventing the propagation of oxidative damage (Imlay, 2013).
ROS-produced damage to proteins, primarily oxidative modifications, can compromise their biological activities (Imlay, 2015). Protein oxidation and aggregation have been linked to senescence and ageing in bacteria (Steiner, 2021) and eukaryotic cells (Höhn et al., 2013). The high frequency of protein oxidation, among other causes, forces the cell to perform protein turnover, which accelerates the presence of excessive oxidative agents (Cabiscol et al., 2000; Imlay, 2013). As a proteome quality control mechanism, several proteolytic and chaperone systems work together to eliminate non-functional and structurally altered proteins (Stadtman, 2006). However, secreted proteins that play essential functions for bacteria can evade such quality control mechanisms. Thus, they are likely to encounter more adverse conditions, including more oxidative environments, and should possess certain robustness to carry out their functions.
In oxygen-rich conditions, a decreasing redox potential gradient extends from intracellular compartments to the extracellular environment. This compartmentalised gradient is less complex in Gram-positive bacteria due to the absence of a periplasmic space (which includes cytoplasm, cell envelopes, and extracellular space). In contrast, Gram-negative bacteria have two additional compartments because of the presence of the outer and inner membranes, each generating a periplasmic space. In addition, previous research has shown that bacteria reduce the energetic synthetic cost of extracellular proteins by using less energetically expensive amino acids in their sequences (Smith and Chapman, 2010).
Until recent years, there was a notion that microbes were potentially immortal or resistant to ageing processes due to binary division, which theoretically generates two identical cells (Zimniak, 2008; Gómez, 2010). However, after division, the splitting of bacterial proteins is asymmetric, and this asymmetry is correlated with the accumulation of oxidised proteins, ultimately leading to bacterial ageing and death (Lybarger and Maddock, 2001; Ackermann et al., 2003; Stewart et al., 2005). For example, carbonylation can lead to protein aggregation and intracellular precipitation, harming the cell, promoting senescence, and increasing the probability of viability loss (Gómez, 2010).
In this study, we propose a hypothesis that the redox states within the microenvironment play a pivotal role in shaping the amino acid composition of proteins within various cellular compartments in prokaryotic cells. To substantiate this hypothesis, we comprehensively analysed amino acid frequencies in every protein within the complete proteome of the model bacterium Escherichia coli, clustering them by cellular location and compartments.
Results and discussion
We initially compiled all amino acid sequences from proteins categorised by cellular compartment: cytoplasm, inner membrane, periplasm, outer membrane, and extracellular environment (Table S1). Subsequently, we identified amino acids more susceptible to oxidative damage using the well-annotated Escherichia coli genome K12 MG1655 as a model. However, this type of analysis may be compromised in other microorganisms where information regarding protein location and function is less well-established (Galperin and Koonin, 2010). These oxidative lesions encompass methionine sulfoxidation, disulfide formation, histidine, tyrosine, and tryptophan oxidation, peroxidation, adduct formation, metal-catalysed oxidation, and carbonylation (Shacter, 2000). Carbonylation is likely the most prevalent form of cell oxidative damage (Nyström, 2005). The number of proteins per cellular compartment in E. coli K-12 MG1655 exhibits heterogeneity (Cytoplasm: 2689; Inner Membrane: 941; Periplasm: 349; Outer Membrane: 146; Extracellular: 16; Figure 1). Although this imbalance in protein distribution represents a potential source of bias in the analyses, it is somewhat inevitable.
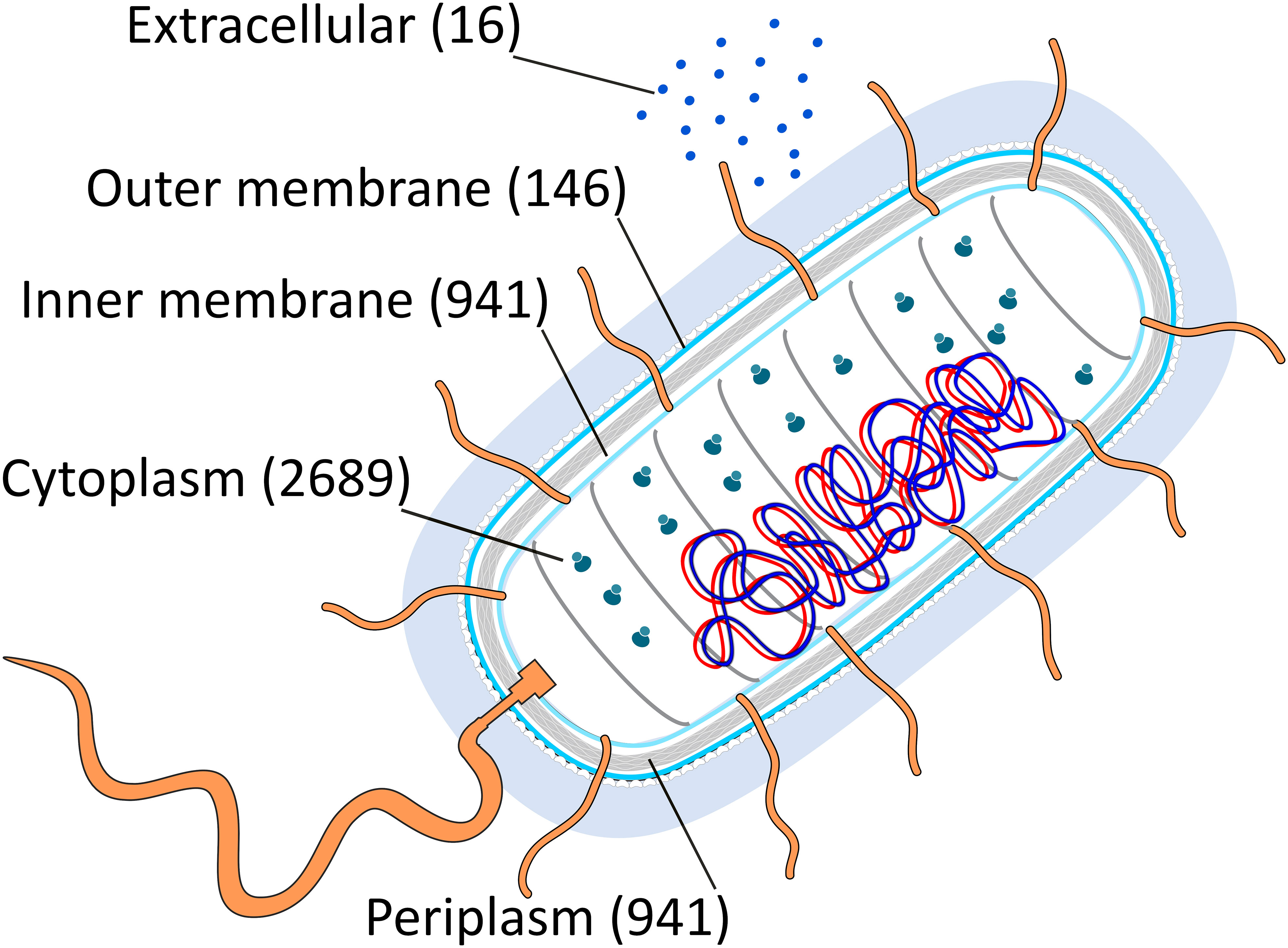
Figure 1 A graphical representation of the E. coli cell compartments and the total number of proteins segregated by each (cytoplasm, inner membrane, periplasm, outer membrane, extracellular media) is presented. The numbers in parentheses indicate the quantity of proteins in each compartment. Flagella proteins were classified based on their location as inner membrane, periplasmic, or outer membrane proteins. Furthermore, only secretable proteins were assigned to the extracellular media. This figure used as a template an image from the Swiss Institute of Bioinformatics (SIB, https://www.sib.swiss/).
In this article, we operate under the assumption that the frequency of a particular amino acid within the proteome, which is susceptible to oxidative damage, logically correlates with the likelihood of such damage occurring. An analysis of protein amino acid composition conducted in E. coli revealed significant differences for all amino acids across cellular compartments (GLM: p=0.0283). Only alanine (A), aspartic acid (D), isoleucine (I), lysine (K), leucine (L), methionine (M), proline (P), and glutamine (Q) did not exhibit a specific preference for a particular cellular compartment (Table S2). Similar findings were obtained when comparing 38 proteomes across the Tree of Life, encompassing Eubacteria, Archaea, and Eukarya. In contrast, low-reactive amino acids, including glycine, alanine, isoleucine, and valine, were predominant in proteins with an extended half-life (Brüne et al., 2018). Therefore, we focused on the amino acid residues susceptible to oxidation. For several of the most oxidation-prone amino acids [cysteine (C), glutamic acid (E), histidine (H), and arginine (R)], there was a significant decrease in their content across subcellular compartments (Figure 2). Based on our hypothesis that amino acid susceptibility to oxidation may limit protein sequences, we analysed the amino acid frequency distribution for all five compartments. While we acknowledge that this correlation does not imply causation, it allows us to explore the differences in amino acid sequences of proteins across compartments. Unfortunately, the majority of evolutionary studies rely on circumstantial evidence. Therefore, correlation-based evolutionary models are required (Nuismer et al., 2010). Due to the nature of the data, no experimental approaches are available to test this hypothesis, as has been the case in previous studies (Akashi and Gojobori, 2002; Smith and Chapman, 2010; Brüne et al., 2018).
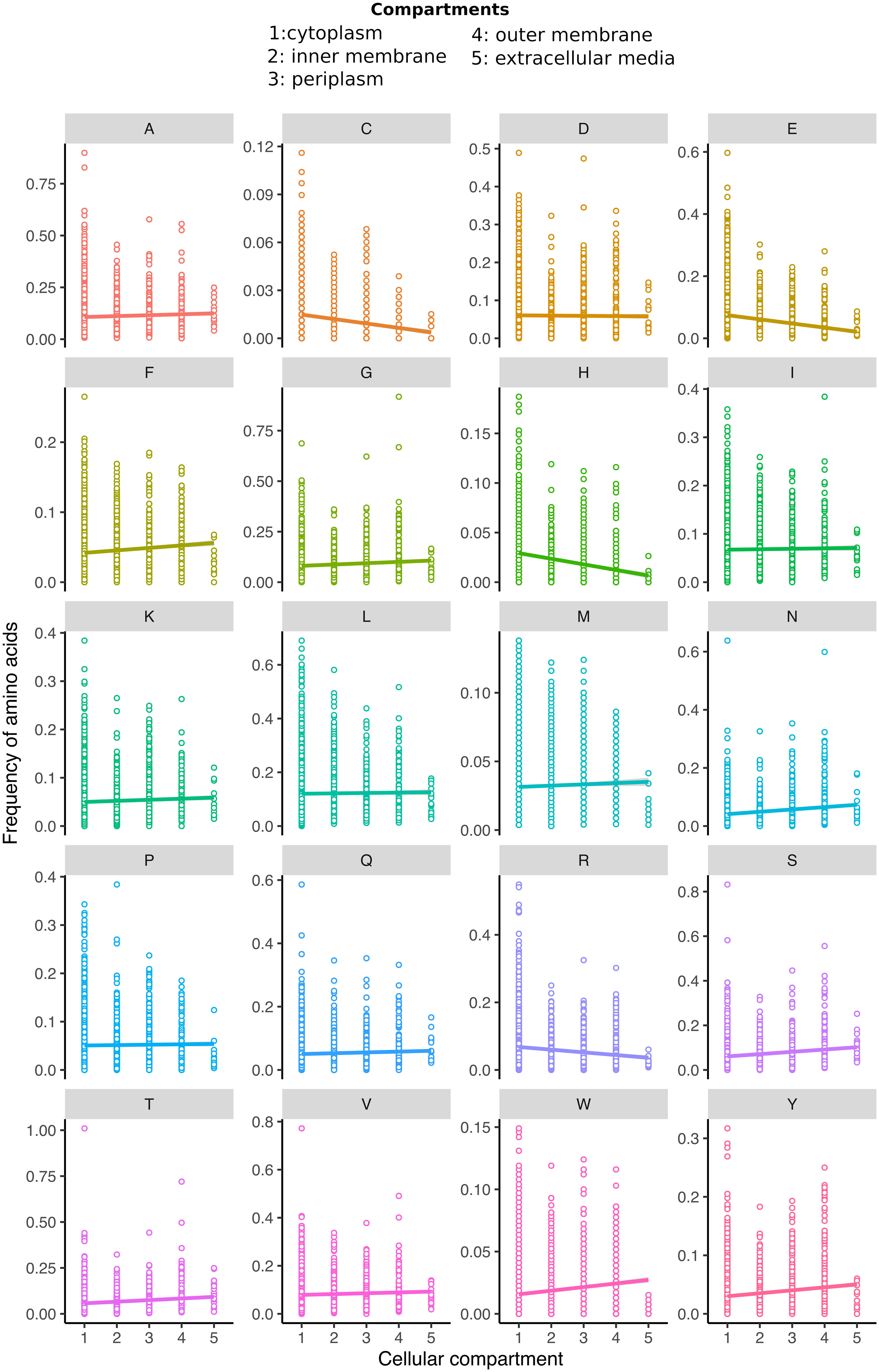
Figure 2 The distribution of each amino acid residue (A–Y) ratio within proteins across all subcellular compartments that entail cytoplasm, inner membrane, periplasm, outer membrane, and extracellular media is displayed. Please note that the amino acid occurrence frequency scale has been individually adjusted to the maximum value, resulting in variations among the cases. The trend lines represent the GLM model, which is statistically significant for all amino acids except alanine, aspartic acid, leucine, isoleucine, lysine, methionine, proline, and glutamine (for detailed statistical values, refer to Table S1).
Aberrant disulfide bond formation and other irreversible oxidative damages of cysteine
Cysteine plays a crucial role in maintaining the redox state of the cytoplasmic compartment (Antelmann and Helmann, 2011). There is a significant difference in cysteine frequency between cytoplasmic proteins and other spaces, including the inner membrane, periplasm, outer membrane, and extracellular medium (Kruskal-Wallis test: p=2.20×10−16). The paired comparison for cysteine (Mann-Whitney U-test) show that all compartments have different histidine frequencies (cytoplasm versus inner membrane, p=9.76×10−35; cytoplasm versus periplasm, p=2.61×10−10; cytoplasm versus outer membrane, p=2.71×10−5; and cytoplasm versus extracellular, p=1.34×10−4). A trend towards decreasing cysteine content from intracellular to extracellular compartments was observed (see Figure 3A) (GLM: slope=−2.71×10−3; p=2.20×10−16; Spearman correlation: rS=−0.20; p=2.20×10−16).
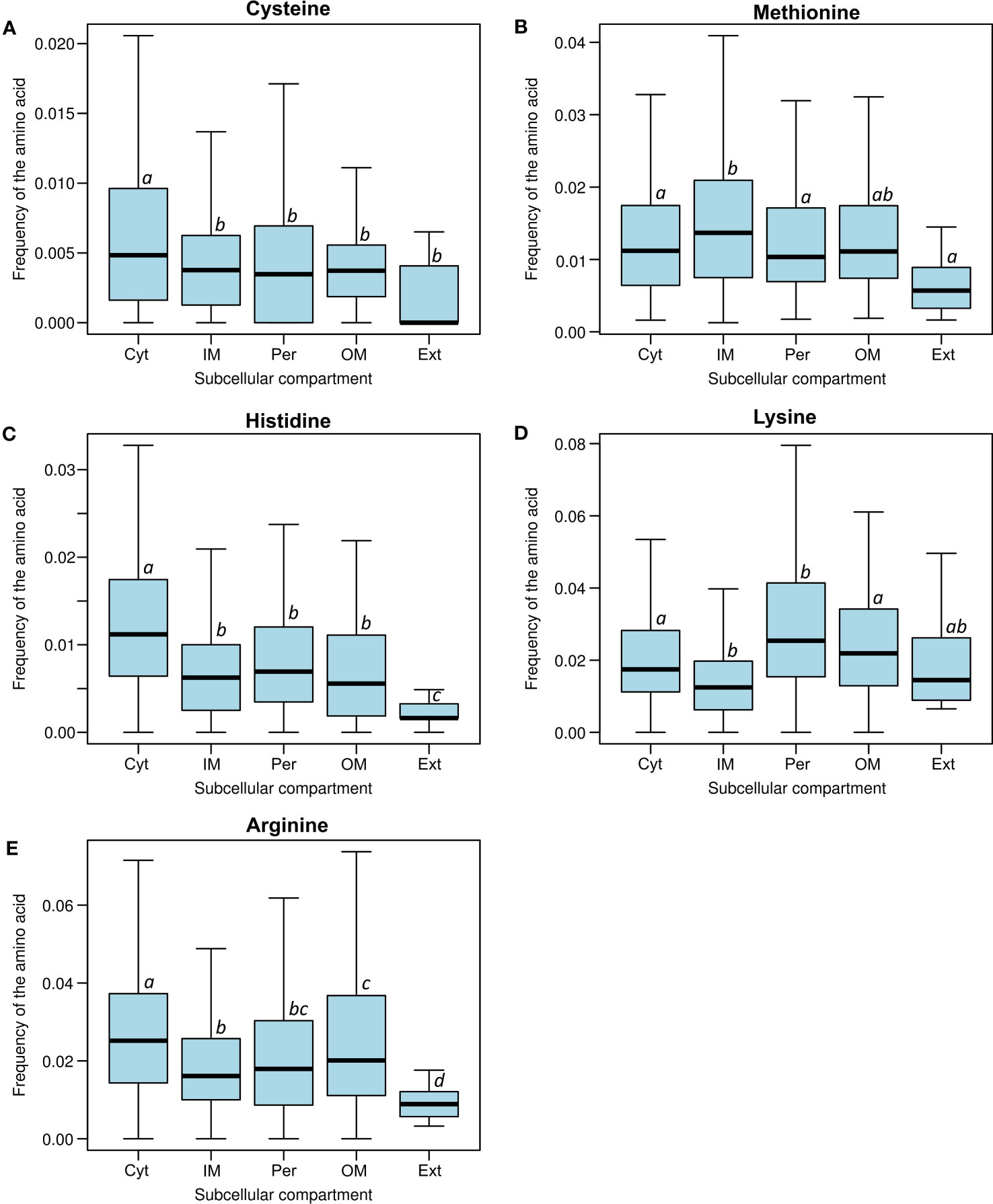
Figure 3 Frequency distribution of the most susceptible amino acids to oxidative damage: (A) cysteine, (B) methionine, (C) histidine, (D) lysine, and (E) arginine in all subcellular compartments (Cyt, cytoplasm; IM, inner membrane; Per, periplasm; OM, outer membrane; Ext, extracellular media). Different letters indicate significant differences, while the same letters indicate no statistical differences (Nemenyi’s test). Please note how the cysteine, histidine, and arginine frequencies significantly decrease from the cytoplasm to the extracellular compartments.
The covalent linking of amino acid side chains within a polypeptide adds to the stability and function of several proteins, with disulfide bridges being the most common (Hatahet et al., 2014). However, aberrant disulfide bonds can lead to the mispairing of cysteines, resulting in misfolding, aggregation, and irreversible oxidative damage (Barshishat et al., 2018). Disulfide-bonded proteins are generally restricted to compartments other than the cytoplasmic space (Dutton et al., 2008). Bacteria, in particular, lack internal compartments. Only a few proteins, such as OxyR and some reductases, use disulfide bonds as redox signalling systems. Disulfide bonds are formed solely in extracellular cysteines as part of their structural function, with the fim operon being the most illustrative case (Rodríguez-Rojas et al., 2020).
On the other hand, flagella proteins lack disulfide bonds, and the amount of this amino acid is minimal. In contrast, most of the proteins in the cytoplasm exist in a reduced state due to the high levels of reducing agents like glutathione, which can reach values near 17 mM when E. coli is fed with glucose and grows in the exponential phase (Bennett et al., 2009). This study provides a clear example of how the redox conditions of the environment constrain the susceptibility of amino acid composition, which may be related to the energetic cost of amino acid biosynthesis (Smith and Chapman, 2010).
In addition to aberrant disulfide bond, thiol groups in protein cysteine residues can undergo one- and two-electron oxidation reactions, forming thiyl radicals or sulfenic acids, respectively. Both thiyl radicals and sulfenic acids play integral roles in the catalytic mechanisms of various enzymes and the redox regulation of protein function and signalling pathways. These species are typically short-lived and subsequently engage in further reactions, ultimately forming diverse stable products. These processes lead to various post-translational modifications of the protein, some of which can be reversed through the action of specific cellular reduction systems. However, others irreversibly damage the proteins, rendering them more susceptible to aggregation or degradation (Turell et al., 2020).
Methionine sulfoxidation
In contrast to other amino acids, methionine oxidation is reversible and is catalysed by the methionine sulfoxide reductases family (Msr) (Etienne et al., 2003). This reduction occurs in both free amino acids and protein residues. We did not observe any trend in the distribution of methionine among the subcellular compartments. There is a marginal difference in methionine frequency between the cytoplasm and secreted proteins (Mann-Whitney U-test, p=0.0352). Additionally, we did not find any differences between the cytoplasm and the periplasm (Mann-Whitney U-test, p=0.604), and neither between the cytoplasm and the outer membrane (Mann-Whitney U-test, p=0.281) (Figure 3B). Methionine residue oxidation can cause misfolding or render proteins dysfunctional (Arts et al., 2015). The methionine oxidation repair system is unique among amino acid oxidation repair systems and may contribute to the possibility of more extensive use of these amino acids in all compartments. Thus, methionine function is not easily replaceable, and cells have evolved the Msr system to continue using this amino acid in an oxidative environment. The case of methionine provides strong evidence that amino acid oxidative lesions could bias amino acid frequency in proteins. We did not find differences in methionine abundance (Figure 3B). This could be explained by the methionine oxidation repair, which actively reverses sulfoxidation and is highly conserved across the Tree of Life (Dos Santos et al., 2018).
Histidine oxidation
Upon analysing the frequency of amino acids in protein sequences, it was observed that histidine is one of the rarest amino acids, following cysteine and tryptophan (Table S2) (Smith and Chapman, 2010). Histidine residues in proteins enable the coordination of certain metallic atoms. The cytoplasmic compartment had the highest histidine frequency, while the extracellular compartment had the lowest. A significant decrease in histidine content was observed from intracellular to extracellular compartments (GLM: slope=−5.58×10−3, p=2.20×10−16; Spearman correlation: rS=−0.28, p=2.20×10−16; Table S2; Figure 3C). Even when comparing histidine frequency between compartments, a significant difference was observed between cytoplasmic proteins and the other compartments, from the inner membrane to the extracellular space (Kruskal-Wallis test, p=2.20×10−16). All individual pair comparison were also significant (Mann-Whitney U-test, cytoplasm versus inner membrane, p=3.12×10−68; cytoplasm versus periplasm, p=4.81×10−16; cytoplasm versus outer membrane, p=1.67×10−9; and cytoplasm versus extracellular, p=5.95×10−7).
Among all oxidation products, histidine is the only amino acid that can be oxidised to form two different amino acids: asparagine and aspartic acid (Berlett and Stadtman, 1997). This phenomenon is analogous to phenotypic mutations that can ultimately disrupt protein sequence information (Yanagida et al., 2015). Under oxidative stress, this consequence of oxidative damage is likely to occur proteome-wide.
One of histidine’s roles inside the cell is metal binding and coordination by specific proteins (Capdevila et al., 2016). Oxidative damage could drive the evolution of microbial metal chelation systems toward siderophore biosynthetic pathways rather than histidine-based systems, a strategy necessary for metal assimilation, such as iron, zinc, and manganese. Siderophores like pyoverdine and catecholamine can protect cells against UV- and antibiotic-derived ROS (Kramer et al., 2020). In anoxic conditions, we might expect histidine-rich proteins to evolve as a preferential pathway, replacing the function of siderophores in microbial biology. However, oxygen undermines this possibility due to histidine’s sensitivity to ROS. Histidine-rich proteins are associated with bacterial habitats, mainly found in rhizobia and pathogenic Gram-negative bacteria, but not in obligate intracellular pathogens (Cheng et al., 2013). Some histidine-rich proteins, such as ceruloplasmin and transferrin, are involved in the chelation and transport of copper and iron, respectively (Steere et al., 2010; Koh and Henderson, 2015). Another issue is that histidine oxidation could disrupt those signalling systems where histidine (de)phosphorylation plays a fundamental role (Adam and Hunter, 2018).
Aromatic amino acid oxidation
Aromatic amino acid residues are frequently targeted by ROS (Berlett and Stadtman, 1997). We observed a slightly positive correlation between the levels of tyrosine, tryptophan, and phenylalanine and the pronounced spatial gradient across compartments, extending from the cytoplasm to the extracellular environment. For tyrosine (Tyr), the GLM Slope was 5.003×10−3; p=2.20×10−16; and the Spearman correlation was rS=0.057; p=2.56×10−4. For tryptophan (Trp), the GLM slope was 2.86×10−3; p=2.20×10−16, while Spearman correlation was rS=0.183; p=2.20×10−16. In the case of phenylalanine, the GLM slope was 3.50×10−3; p=4.15×10−9, and the Spearman correlation was rS=0.12; p=2.20×10−16).
Tyrosine and tryptophan rank as the second and fifth least abundant amino acids in the E. coli proteome, respectively (Table S2). When comparing tyrosine frequency among compartments, significant differences only exist between cytoplasmic and the periplasm proteins (Mann-Whitney U test, p=3.59×10−5) and between the outer membrane and cytoplasm (Mann-Whitney U-test, p=4.13×10−8). On the other hand, tryptophan frequency is unevenly distributed among compartments, likely due to its hydrophobic nature (Kruskal-Wallis test: p=2.20×10−16). When it comes to pair comparison between compartments, all tests were significant using Mann-Whitney U-test (cytoplasm versus inner membrane, p=1.16×10−43; cytoplasm versus periplasm, p=6.42×10−5; cytoplasm versus outer membrane, p=6.78×10−4, cytoplasm versus extracellular, p=3.63×10−2). This uneven distribution is also observed in phenylalanine (Kruskal-Wallis test: p=2.20×10−16). For phenylalanine, all pair comparisons via Mann-Whitney U-test were also significant (cytoplasm versus inner membrane, p=2.41×10−84; cytoplasm versus periplasm, p=2.19×10−46; cytoplasm versus outer membrane, p=6.48×10−14; and cytoplasm versus extracellular, p=6.94×10−9). Their aliphatic nature and structural properties render them indispensable, explaining their consistent representation among compartments. The over-representation of these amino acids within membranes is attributed to their lack of polarity and their compatibility with hydrophobic environments (De Planque and Killian, 2003). The oxidation of aromatic amino acids is of paramount importance as it takes place proximal to biological membranes, assuming a central role in cellular signalling, managing oxidative stress responses, and governing diverse physiological processes. This dynamic interplay between ROS and membrane constituents is a pivotal aspect of redox biology (Fisher, 2009).
Protein peroxidation
Peroxidation selectively targets valine, leucine, tryptophan, and tyrosine. Although tryptophan and tyrosine are present at low frequencies, leucine and valine are the most common and fourth most abundant amino acids in E. coli (Table S2). This subsection will focus on leucine and valine, as tryptophan and tyrosine were discussed in the preceding section. Notably, we only observed significant differences in the frequency of leucine (Mann-Whitney U-test, p=1.83×10−16) and valine (Mann-Whitney U-test, p=1.46×10−5) between the cytoplasm and the inner membrane. Peroxidation does not appear to significantly influence the bias in the frequency of susceptible amino acids. It is plausible that the ubiquity of this reaction has prompted natural selection to partially mitigate its impact by evolving scavenging systems, such as catalases and peroxidases, aimed at curtailing widespread damage (Imlay, 2008).
Carbonylation
Protein carbonylation, a form of protein oxidation induced by reactive oxygen species (ROS), entails the conversion of alcohol (−OH) groups in side chains into reactive ketones or aldehydes. While all amino acids are susceptible to carbonylation at the protein’s C-terminus, our focus lies on lysine, arginine, proline, and threonine due to their heightened susceptibility to oxidation into carbonyl derivatives (Cabiscol et al., 2000; Shacter, 2000). Notably, carbonylation’s impact extends beyond carbonyl group oxidation, as proteins may undergo this modification through mechanisms unrelated to oxidation (Cabiscol et al., 2000).
All, lysine, arginine, proline, and threonine showed differences in their frequencies between the cytoplasm and periplasm employing the Mann-Whitney U-test (Lys, p=1.02×10−14, (Arg, p=1.28×10−11; p=1.94×10−2; Thr, p=1.45×10−10. Moreover, we detected significant differences in the frequency of lysine, arginine and proline between the cytoplasm and inner membrane also via Mann-Whitney U-test (Lys, p=9.81×10−38, Arg, p=5.14×10−40, Pro p=4.85×10−4). There were also differences in the frequency of lysine, arginine and threonine between the cytoplasm and outer membrane (Mann-Whitney U-test: Lys, p=1.57×10−2; Arg, p=4.53×10−2; Thr, p=4.77×10−3. Finally, differences in the frequency of arginine, proline and threonine were detected between the cytoplasm and extracellular proteins, also using Mann-Whitney U-test (Arg, p= 1.22×10−5; Pro, p=1.275×10−2; Thr, p=1.98×10−3; Figures 2, 3D, E). These frequencies coincide with expected decreased frequencies of amino acids prone to carbonylation from more reducing microenvironment (cytoplasm) to more oxidative ones such as extracellular compartments. Interestingly, we noticed that elevated frequencies of arginine and lysine were significantly higher in outer membrane proteins than in inner membrane ones (Figures 3D-E). Arginine is more frequent in α-helix structural domains, which is also more abundant in outer membrane proteins, while β-barrels are more common in inner membrane proteins (Hristova and Wimley, 2011). A similar use could be expected for lysine due to its chemical similarity, although we did not find any report regarding this amino acid.
Differences in the amino acid composition of inner transmembrane proteins
One unique compartment is the inner membrane, where the same protein has amino acid residues exposed to the cytoplasmic reductive environment and the periplasmic oxidative one. Therefore, we analysed the sequences within the same protein concerning amino acid frequency in different protein segments (cytoplasm, transmembrane, and periplasm). The amino acid composition of 878 transmembrane proteins across regions shows significant differences in amino acid occurrence for all amino acids (Figure 4; Table S3). Additionally, substantial discrepancies exist among unevenly distributed amino acids across the three locations within the same protein. While alanine, cysteine, phenylalanine, isoleucine, leucine, valine, tryptophan, and tyrosine are prevalent in the transmembrane region, lysine and arginine are more common in the cytoplasmic area, and aspartate, glycine, asparagine, proline, serine, and threonine are more abundant in the periplasmic compartment (Figure 4; Table S3). This amino acid bias is primarily constrained by the protein’s secondary structure and function within the membrane (Ulmschneider and Sansom, 2001; Pascal et al., 2006). Hence, no clear pattern regarding amino acid distribution related to oxidation susceptibility exists.
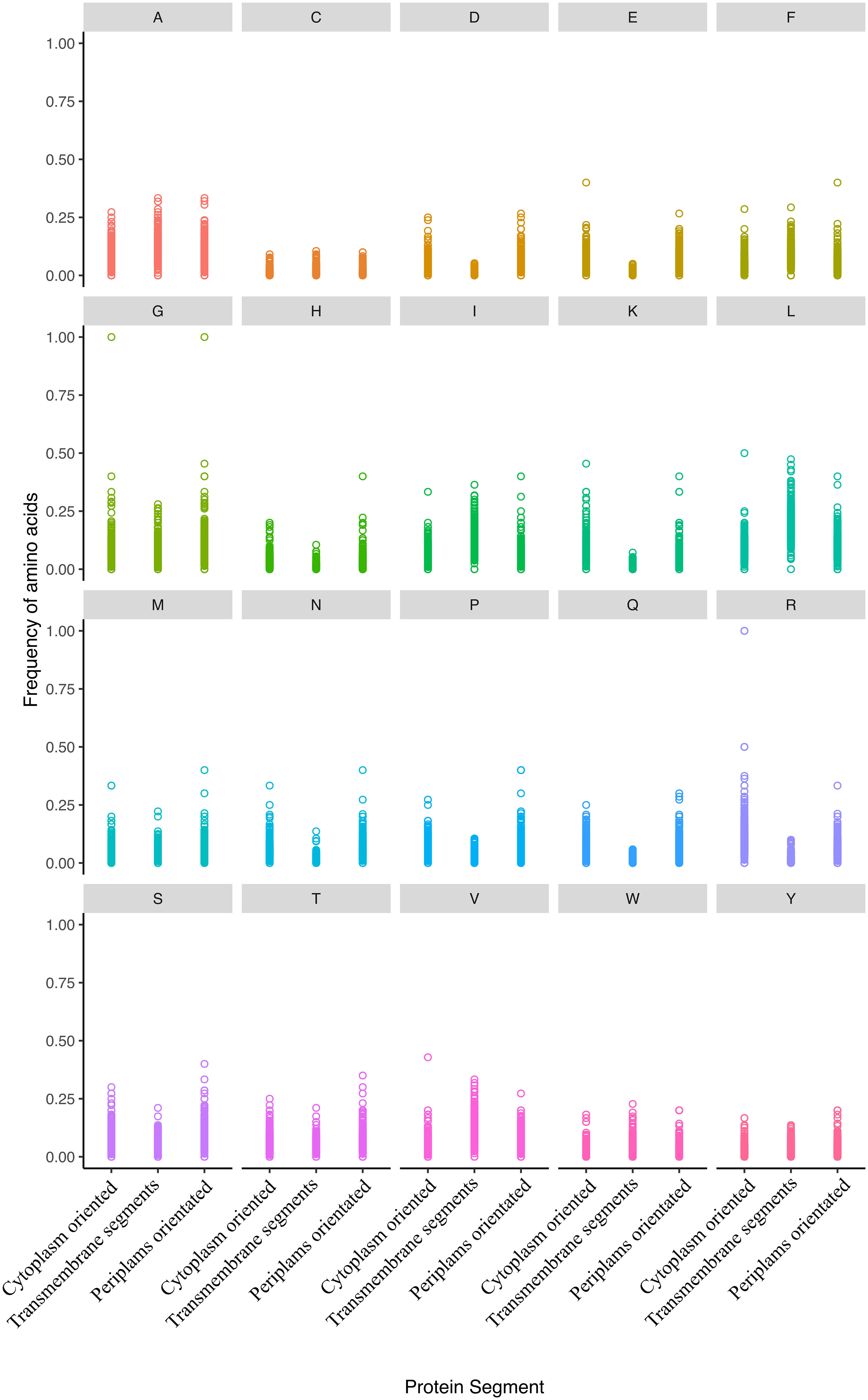
Figure 4 The distribution of amino acid residue ratios in inner membrane proteins is categorized into three groups: cytoplasm-oriented, transmembrane, and periplasm-oriented. The plots illustrate the frequency of all amino acids across these categories. Notably, all amino acid frequencies showed a significant uneven distribution except for cysteine, phenylalanine, and tryptophan. The frequencies of amino acids in the various compartments were published elsewhere (Smith and Chapman, 2010).
Conclusions
The present study reveals significant variations in amino acid frequencies that could be partially attributed to the predisposition of amino acids to undergo oxidation across proteins in various cellular compartments in E. coli. These findings suggest an uneven distribution of amino acids intricately linked to a protein’s cellular localization within the organism. This observation aligns with previous research, which proposed a connection between amino acid distribution and the energetic cost (Smith and Chapman, 2010). Furthermore, the susceptibility of residues to different oxidative lesions brought about by oxygen accumulation and the emergence of anaerobic respiration may contribute to this amino acid bias in protein sequences. Notably, primary oxidation-prone amino acids exhibit an overrepresentation in the cytoplasm, diminishing as we move to distinct subcellular compartments, mirroring the redox gradient from reductive to oxidative microenvironments. Several factors, including structural constraints, catalytic amino acids, subcellular compartment polarity, and protein-specific information, could also impact the non-uniform distribution of amino acids across cellular compartments. While we have identified some positive correlations indicating that oxidative stress may influence amino acid sequences, promoting the evolution of a proteome with enhanced resistance to oxidation, establishing a causal relationship requires further research.
Methods
Data mining
The complete proteome of Escherichia coli K-12 MG1655, encompassing 4143 proteins, was retrieved from EcoCyc (Keseler et al., 2021) in FASTA format. Each protein underwent analysis to calculate the occurrences of individual amino acids, facilitated by a custom Python script. This analysis yielded a tabular-separated values (TSV) file, where each row corresponds to a single protein, and each column indicates the absolute frequency of a particular amino acid within that protein. The classification of amino acids’ susceptibility to various oxidative stresses was conducted by a prior study (Shacter, 2000).
Amino acid composition and distribution in subcellular compartment calculation
Given that the protein size distribution in all compartments did not conform to a normal distribution (Sommer and Cohen, 1980), the values were presented as amino acid frequency normalised by the median size of proteins within each cellular compartment. Under the classical protein definition, only polypeptides exceeding 50 amino acids were considered for all analyses (Milo et al., 2010). To assess whether the content of each amino acid among proteins in the various cellular compartments (i.e., cytoplasm, inner membrane, periplasm, outer membrane, and secreted) follows a uniform distribution, the Kruskal-Wallis test was conducted. In cases where significant differences were observed, multiple comparisons were performed using Nemenyi’s test (Nemenyi, 1963), with p-values corrected for false discovery rate (Benjamini and Hochberg, 1995). Additionally, Bonferroni-corrected Mann-Whitney U tests were carried out for specific pair-wise comparisons, as indicated throughout the text. Finally, general linear models (GLMs) were applied per amino acid and subcellular compartment, and Spearman correlations were computed to elucidate the spatial gradient of amino acid oxidative lesions. All these analyses were executed using R 3.2.1 (R Core Team, 2017), with the aid of the HH (Heiberger and Holland, 2015) and PMCMR packages (Pohlert, 2015).
Amino acid composition and distribution in transmembrane proteins
The prediction of transmembrane regions was carried out using Phobius 1.01 (Käll et al., 2004) and TMHMM 2.0 (Krogh et al., 2001) with default parameters. To ensure consistency, all signal peptides were predicted using Signal-P 4.0 (Petersen et al., 2011) and their amino acidic residues excluded prior our analyses. All analyses about the distribution of amino acids within various regions were conducted following previously established protocols.
Data availability statement
The original contributions presented in the study are included in the article/Supplementary Materials, further inquiries can be directed to the corresponding author/s.
Author contributions
AR-R and EG-T conceived and designed the study. EG-T performed all bioinformatic analyses, and AR-R interpreted the data. EG-T and AR-R wrote the manuscript. All authors contributed to the article and approved the submitted version.
Funding
The author(s) declare financial support was received for the research, authorship, and/or publication of this article. AR-R was supported by SFB 973 (Deutsche Forschungsgemeinschaft), CRC973 (http://www.sfb973.de/), and project C5.
Acknowledgments
We thank Arpita Nath, Dr. Dan Roizman and Dr. Flor I. Arias-Sánchez (from Freie Universität Berlin) for their valuable comments on the manuscript. We also acknowledge Prof. Dr. Jens Rolff for his support. We also acknowledge support from the Open Access Publication Fund of Freie Universität Berlin.
Conflict of interest
The authors declare that the research was conducted in the absence of any commercial or financial relationships that could be construed as a potential conflict of interest.
Publisher’s note
All claims expressed in this article are solely those of the authors and do not necessarily represent those of their affiliated organizations, or those of the publisher, the editors and the reviewers. Any product that may be evaluated in this article, or claim that may be made by its manufacturer, is not guaranteed or endorsed by the publisher.
Supplementary material
The Supplementary Material for this article can be found online at: https://www.frontiersin.org/articles/10.3389/fevo.2023.1172785/full#supplementary-material
Supplementary Table 1 | Original data set of Escherichia coli proteins and their amino acid composition modified from EcoCyc database (Keseler et al., 2017). We excluded polypeptides smaller than 50 amino acids and pseudogenes whose original locations are unknown.
Supplementary Table 2 | Amino acid composition and distribution of the E. coli proteome across subcellular compartments. Numbers in columns C to G represent the median of the normalised frequencies of each amino acid relative to the median protein length within each compartment. Different letter(s) indicate significant differences (p<0.05) between compartments, determined by Nemenyi’s test and adjusted for false discovery rate. Spearman’s rS and corresponding p-values demonstrate the correlation between amino acid frequency and subcellular compartments and their significance, respectively. Finally, the slope, coefficient of determination (R2), and the GLM P-value reveal the trend of amino acid distribution across subcellular compartments, the degree to which it aligns with a generalised linear model, and its significance. All p-values shown in bold are significant following Bonferroni correction.
Supplementary Table 3 | Amino acid composition of E. coli transmembrane proteins on the inner membrane. Numbers in columns D, F, and H represent the median of the normalised frequencies of each amino acid relative to the median protein length within each compartment. Different letter(s) indicate significant differences (p<0.05) between compartments as determined by Nemenyi’s test, adjusted via false discovery rate.
References
Ackermann M., Stearns S. C., Jenal U. (2003). Senescence in a bacterium with asymmetric division. Sci. (80-. ). 300, 1920–1920. doi: 10.1126/science.1083532
Adam K., Hunter T. (2018). Histidine kinases and the missing phosphoproteome from prokaryotes to eukaryotes. Lab. Investig. 98, 233–247. doi: 10.1038/labinvest.2017.118
Akashi H., Gojobori T. (2002). Metabolic efficiency and amino acid composition in the proteomes of Escherichia coli and Bacillus subtilis. Proc. Natl. Acad. Sci. U.S.A. 99, 3695–3700. doi: 10.1073/pnas.062526999
Anbar A. D. (2008). Oceans. Elements and evolution. Science 322, 1481–1483. doi: 10.1126/science.1163100
Antelmann H., Helmann J. D. (2011). Thiol-based redox switches and gene regulation. Antioxid. Redox Signal. 14, 1049–1063. doi: 10.1089/ars.2010.3400
Arts I. S., Gennaris A., Collet J.-F. (2015). Reducing systems protecting the bacterial cell envelope from oxidative damage. FEBS Lett. 589, 1559–1568. doi: 10.1016/j.febslet.2015.04.057
Barshishat S., Elgrably-Weiss M., Edelstein J., Georg J., Govindarajan S., Haviv M., et al. (2018). No title. EMBO J. 37, 413–426. doi: 10.15252/embj.201797651
Benjamini Y., Hochberg Y. (1995). Controlling the false discovery rate: a practical and powerful approach to multiple testing. J. R. Stat. Soc B 57, 289–300. doi: 10.2307/2346101
Bennett B. D., Kimball E. H., Gao M., Osterhout R., Van Dien S. J., Rabinowitz J. D. (2009). Absolute metabolite concentrations and implied enzyme active site occupancy in Escherichia coli. Nat. Chem. Biol. 5, 593–599. doi: 10.1038/nchembio.186
Berlett B. S., Stadtman E. R. (1997). Protein oxidation in aging, disease, and oxidative stress. J. Biol. Chem. 272, 20313–20316. doi: 10.1074/jbc.272.33.20313
Brüne D., Andrade-Navarro M. A., Mier P. (2018). Proteome-wide comparison between the amino acid composition of domains and linkers. BMC Res. Notes 11, 1–6. doi: 10.1186/s13104-018-3221-0
Cabiscol E., Tamarit J., Ros J. (2000). Oxidative stress in bacteria and protein damage by reactive oxygen species. Int. Microbiol. 3, 3–8.
Capdevila D. A., Wang J., Giedroc D. P. (2016). Bacterial strategies to maintain zinc metallostasis at the host-pathogen interface*. J. Biol. Chem. 291, 20858–20868. doi: 10.1074/jbc.R116.742023
Cavalier-Smith T. (2006). Cell evolution and Earth history: stasis and revolution. Philos. Trans. R. Soc Lond. B. Biol. Sci. 361, 969–1006. doi: 10.1098/rstb.2006.1842
Cavalier-Smith T., Brasier M., Embley T. M. (2006). Introduction: How and when did microbes change the world? Philos. Trans. R. Soc Lond. B. Biol. Sci. 361, 845–850. doi: 10.1098/rstb.2006.1847
Cheng T., Xia W., Wang P., Huang F., Wang J., Sun H. (2013). Histidine-rich proteins in prokaryotes: Metal homeostasis and environmental habitat-related occurrence. Metallomics 5, 1423–1429. doi: 10.1039/c3mt00059a
De Planque M. R. R., Killian J. A. (2003). Protein-lipid interactions studied with designed transmembrane peptides: Role of hydrophobic matching and interfacial anchoring (Review). Mol. Membr. Biol. 20, 271–284. doi: 10.1080/09687680310001605352
Dos Santos S. L., Petropoulos I., Friguet B. (2018). The oxidized protein repair enzymes methionine sulfoxide reductases and their roles in protecting against oxidative stress, in ageing and in regulating protein function. Antioxidants 7. doi: 10.3390/antiox7120191
Dutton R. J., Boyd D., Berkmen M., Beckwith J. (2008). Bacterial species exhibit diversity in their mechanisms and capacity for protein disulfide bond formation. Proc. Natl. Acad. Sci. U.S.A. 105, 11933–11938. doi: 10.1073/pnas.0804621105
Etienne F., Spector D., Brot N., Weissbach H. (2003). A methionine sulfoxide reductase in Escherichia coli that reduces the R enantiomer of methionine sulfoxide. Biochem. Biophys. Res. Commun. 300, 378–382.
Fisher A. B. (2009). Redox signaling across cell membranes. Antioxid. Redox Signal. 11, 1349–1356. doi: 10.1089/ARS.2008.2378
Galperin M. Y., Koonin E. V. (2010). From complete genome sequence to “complete” understanding? Trends Biotechnol. 28, 398–406. doi: 10.1016/j.tibtech.2010.05.006
Godoy-Ruiz R., Perez-Jimenez R., Ibarra-Molero B., Sanchez-Ruiz J. M. (2004). Relation between protein stability, evolution and structure, as probed by carboxylic acid mutations. J. Mol. Biol. 336, 313–318. doi: 10.1016/j.jmb.2003.12.048
Gómez J. M. G. (2010). Aging in bacteria, immortality or not-a critical review. Curr. Aging Sci. 3, 198–218. doi: 10.2174/1874609811003030198
Hatahet F., Boyd D., Beckwith J. (2014). Disulfide bond formation in prokaryotes: history, diversity and design. Biochim. Biophys. Acta 1844, 1402–1414. doi: 10.1016/j.bbapap.2014.02.014
Heiberger R. M., Holland B. (2015). Statistical Analysis and Data Display: An Intermediate Course with Examples in R. 2nd ed. (New York, NY: Springer New York).
Höhn A., König J., Grune T. (2013). Protein oxidation in aging and the removal of oxidized proteins. J. Proteomics 92, 132–159. doi: 10.1016/J.JPROT.2013.01.004
Hristova K., Wimley W. C. (2011). A look at arginine in membranes. J. Membr. Biol. 239, 49–56. doi: 10.1007/s00232-010-9323-9
Imlay J. (2008). Cellular defenses against superoxide and hydrogen peroxide. Annu. Rev. Biochem. 77, 755–776. doi: 10.1146/annurev.biochem.77.061606.161055
Imlay J. A. (2013). The molecular mechanisms and physiological consequences of oxidative stress: Lessons from a model bacterium. Nat. Rev. Microbiol. 11, 443–454. doi: 10.1038/nrmicro3032
Imlay J. A. (2015). Diagnosing oxidative stress in bacteria: not as easy as you might think. Curr. Opin. Microbiol. 24C, 124–131. doi: 10.1016/j.mib.2015.01.004
Käll L., Krogh A., Sonnhammer E. L. L. (2004). A combined transmembrane topology and signal peptide prediction method. J. Mol. Biol. 338, 1027–1036. doi: 10.1016/j.jmb.2004.03.016
Keseler I. M., Mackie A., Santos-Zavaleta A., Billington R., Bonavides-Martínez C., Caspi R., et al. (2017). The EcoCyc database: reflecting new knowledge about Escherichia coli K-12. Nucleic Acids Res. 45, D543–D550. doi: 10.1093/nar/gkw1003
Keseler I. M., Gama-Castro S., Mackie A., Billington R., Billington R., Caspi R., et al. (2021). The EcoCyc database in 2021. Front. Microbiol. 12, 711077. doi: 10.3389/fmicb.2021.711077
Knight R. D., Freeland S. J., Landweber L. F. (2001). A simple model based on mutation and selection explains trends in codon and amino-acid usage and GC composition within and across genomes. Genome Biol. 2, 1–13. doi: 10.1186/gb-2001-2-4-research0010
Koh E. I., Henderson J. P. (2015). Microbial copper-binding siderophores at the host-pathogen interface. J. Biol. Chem. 290, 18967–18974. doi: 10.1074/jbc.R115.644328
Kramer J., Özkaya Ö., Kümmerli R. (2020). Bacterial siderophores in community and host interactions. Nat. Rev. Microbiol. 18, 152–163. doi: 10.1038/s41579-019-0284-4
Krogh A., Larsson B., von Heijne G., Sonnhammer E. L. (2001). Predicting transmembrane protein topology with a hidden Markov model: application to complete genomes. J. Mol. Biol. 305, 567–580. doi: 10.1006/jmbi.2000.4315
Lu Y., Freeland S. (2006). On the evolution of the standard amino-acid alphabet. Genome Biol. 7, 102. doi: 10.1186/gb-2006-7-1-102
Lybarger S. R., Maddock J. R. (2001). Polarity in action: asymmetric protein localization in bacteria. J. Bacteriol. 183, 3261–3267. doi: 10.1128/JB.183.11.3261-3267.2001
Lyons T. W., Reinhard C. T., Planavsky N. J. (2014). The rise of oxygen in Earth’s early ocean and atmosphere. Nature 506, 307–315. doi: 10.1038/nature13068
Mendez R., Fritsche M., Porto M., Bastolla U. (2010). Mutation bias favors protein folding stability in the evolution of small populations. PloS Comput. Biol. 6, e1000767. doi: 10.1371/journal.pcbi.1000767
Milo R., Jorgensen P., Moran U., Weber G., Springer M. (2010). BioNumbers–the database of key numbers in molecular and cell biology. Nucleic Acids Res. 38, D750–D753. doi: 10.1093/nar/gkp889
Nemenyi P. (1963). Distribution-free multiple comparisons. Ph.D. thesis. Princeton, NJ: Princeton University.
Nuismer S. L., Gomulkiewicz R., Ridenhour B. J. (2010). When is correlation coevolution? Am. Nat. 175, 525–537. doi: 10.1086/651591
Nyström T. (2005). Role of oxidative carbonylation in protein quality control and senescence. EMBO J. 24, 1311–1317. doi: 10.1038/sj.emboj.7600599
Pascal G., Médigue C., Danchin A. (2006). Persistent biases in the amino acid composition of prokaryotic proteins. BioEssays 28, 726–738. doi: 10.1002/bies.20431
Petersen T. N., Brunak S., von Heijne G., Nielsen H. (2011). SignalP 4.0: discriminating signal peptides from transmembrane regions. Nat. Methods 8, 785–786. doi: 10.1038/nmeth.1701
R Core Team (2017). R: A language and environment for statistical computing (Vienna, Austria: R Foundation for Statistical Computing).
Rodríguez-Rojas A., Kim J. J., Johnston P. R., Makarova O., Eravci M., Weise C., et al. (2020). Non-lethal exposure to H2O2 boosts bacterial survival and evolvability against oxidative stress. PLoS Genet. 16. doi: 10.1371/JOURNAL.PGEN.1008649
Shacter E. (2000). Quantification and significance of protein oxidation in biological samples. Drug Metab. Rev. 32, 307–326. doi: 10.1081/DMR-100102336
Smith D. R., Chapman M. R. (2010). Economical evolution: microbes reduce the synthetic cost of extracellular proteins. MBio 1, e00131–e0010-. doi: 10.1128/mBio.00131-10
Sommer S. S., Cohen J. E. (1980). The size distributions of proteins, mRNA and nuclear RNA. J. Mol. Evol. 15, 37–57.
Stadtman E. R. (2006). Protein oxidation and aging. Free Radic. Res. 40, 1250–1258. doi: 10.1080/10715760600918142
Steere A. N., Byrne S. L., Chasteen N. D., Smith V. C., MacGillivray R. T. A., Mason A. B. (2010). Evidence that His349 acts as a pH-inducible switch to accelerate receptor-mediated iron release from the C-lobe of human transferrin. J. Biol. Inorg. Chem. 15, 1341–1352. doi: 10.1007/s00775-010-0694-2
Steiner U. K. (2021). Senescence in bacteria and its underlying mechanisms. Front. Cell Dev. Biol. 9. doi: 10.3389/FCELL.2021.668915/BIBTEX
Stewart E. J., Madden R., Paul G., Taddei F. (2005). Aging and death in an organism that reproduces by morphologically symmetric division. PLoS Biol. 3, e45. doi: 10.1371/journal.pbio.0030045
Tourasse N. J., Li W. H. (2000). Selective constraints, amino acid composition, and the rate of protein evolution. Mol. Biol. Evol. 17, 656–664.
Turell L., Zeida A., Trujillo M. (2020). Mechanisms and consequences of protein cysteine oxidation: the role of the initial short-lived intermediates. Essays Biochem. 64, 55–66. doi: 10.1042/EBC20190053
Ulmschneider M. B., Sansom M. S. P. (2001). Amino acid distributions in integral membrane protein structures. Biochim. Biophys. Acta – Biomembr. 1512, 1–14. doi: 10.1016/S0005-2736(01)00299-1
Worth C. L., Gong S., Blundell T. L. (2009). Structural and functional constraints in the evolution of protein families. Nat. Rev. Mol. Cell Biol. 10, 709–720. doi: 10.1038/nrm2762
Yanagida H., Gispan A., Kadouri N., Rozen S., Sharon M., Barkai N., et al. (2015). The evolutionary potential of phenotypic mutations. PloS Genet. 11, e1005445. doi: 10.1371/JOURNAL.PGEN.1005445
Keywords: oxidative stress, protein oxidation, amino acid sequence, protein evolution, proteome damage
Citation: González-Tortuero E and Rodríguez-Rojas A (2023) A hypothesis about the influence of oxidative stress on amino acid protein composition during evolution. Front. Ecol. Evol. 11:1172785. doi: 10.3389/fevo.2023.1172785
Received: 23 February 2023; Accepted: 06 November 2023;
Published: 29 November 2023.
Edited by:
Carla Mucignat, University of Padua, ItalyReviewed by:
Nadia Benaroudj, Institut Pasteur, FranceSantosh Kumar C. M., University of Birmingham, United Kingdom
Copyright © 2023 González-Tortuero and Rodríguez-Rojas. This is an open-access article distributed under the terms of the Creative Commons Attribution License (CC BY). The use, distribution or reproduction in other forums is permitted, provided the original author(s) and the copyright owner(s) are credited and that the original publication in this journal is cited, in accordance with accepted academic practice. No use, distribution or reproduction is permitted which does not comply with these terms.
*Correspondence: Enrique González-Tortuero, enriquegleztortuero@gmail.com; Alexandro Rodríguez-Rojas, a.rojas@fu-berlin.de