- 1School of Management Science and Engineering, Chongqing Technology and Business University, Chongqing, China
- 2School of Management Science and Real Estate, Chongqing University, Chongqing, China
Environmental problems, including air pollution, have upset the balance between the environment and economic development. In the face of worsening air pollution, growing attention is being paid to the role of financial institutions. To investigate how finance affects air pollution, this study used data from 30 Chinese provinces from 2014–2020, while considering industrial structure optimization and energy structure as threshold variables to propose a panel threshold model, which elucidates a nonlinear relationship between green finance and air quality in China. The panel data are sorted based on urbanization level, resource dependence, and geospatial distribution to examine the heterogeneous effects of green finance on air pollution. The analysis demonstrated that green finance considerably improves the overall air quality in Chinese provinces, but the effect is gradually weakened with the optimization of industrial structure and the improvement of clean energy efficiency. Moreover, green finance exerts a greater inhibitory impact on air pollution through industrial structure optimization compared to the impact of energy structure optimization. Regional variations in the environmental benefits of green finance were discerned. Resource dependence exacerbates the inhibiting impact of green finance on air pollution in the resource-based regions, while urbanization weakens the environmental benefits of green finance in urbanized regions. In terms of geographical distribution, green finance exhibited the best effect on air quality in western China. These findings shed the light on the positive role of green finance on air quality in China, thereby highlighting its importance for the air quality improvement programs of the provincial scales in China.
1. Introduction
Environmental degradation and climate warming caused by human activities have negative impacts on economic and social development and human production and life (Gan et al., 2021; Liang et al., 2023; Wang et al., 2023). Actively combating climate change and tackling problems with air quality are top priorities for global environmental governance. However, any initiative to reduce air pollution requires massive capital investment. Financial support is urgently needed under such conditions. Recently, an increasing number of people pay attention to the role of economic instruments in environmental governance. In order to better balance environmental and economic development, the identification of environmental pollution liability has forced financial institutions to incorporate environmental factors into their investment and financing decisions (Scholtens, 2017), gradually evolving the concept of “green finance.” In addition, the implementation of clean air actions in China has achieved a cumulative net CO2 emission reduction of 2.43 billion tons (Shi Q. et al., 2022), which indicates that the characteristics of the same root, source and process between air pollutants and CO2 emissions. In order to achieve the goal of ecological environment improvement and carbon peak carbon neutrality as soon as possible, in June 2022, China’s Ministry of Ecology and Environment has clearly proposed to increase the support of green finance to reduce pollution and carbon, and promote the synergistic effect of pollution and carbon reduction. In this context, analyzing the effects of green finance on air quality can help provide guidance and suggestions for air quality improvement and green transition under the new stage of development in China.
Existing studies mainly examined the sustainable impact of green finance on environmental quality. For example, Ma et al. (2023) showed that the introduction of environmental policies focused on green finance may have mixed consequences on environmental quality. On one aspect, green finance policies have pushed enterprises to green transformation by increasing renewable energy investments, limiting funding for inefficient and heavily polluting enterprises, encouraging technological innovation, and increasing financing costs for polluting enterprises (Chen and Chen, 2021; Li M. et al., 2021; Zhang and Vigne, 2021; Zeng et al., 2022), thus reducing emissions of pollutants such as haze. On the other hand, the development of green finance in general has a negative impact on bank loans (He et al., 2019). The contraction of credit volume in general will be unfavorable to the investment of renewable energy enterprises, because the promotion effect of green finance on the investment of renewable energy enterprises is weakened or even offset by the excessive financing constraints (Wang and Fan, 2023). Green finance only improves environmental quality in the short term (Triki et al., 2023), which is not conducive to sustainable development. However, current research on the relationship between green finance and air pollution is inadequate. It is necessary to further examine the impact mechanisms between green finance and air quality and whether it is varied during the development of green finance in China.
Furthermore, air pollution control is a long-term and challenging process, involving energy and industrial restructuring, major emission reduction projects, and environmental economics. Governments are trying to achieve the goal of environmental management by optimizing the industrial structure and adjusting the energy structure. And the adjustment and optimization of industrial structure and energy structure is an important source control measure for air pollution prevention and control in China (Feng et al., 2019). Some scholars have pointed out the phased characteristics of the impact of industrial and energy structures on air pollution (Wang et al., 2019; Zheng et al., 2020). However, it is unclear whether green finance has achieved the desired effect of improving air quality under the path of industrial and energy structure optimization.
On the basis of such a background, this study focuses on two questions:
1. Did the development of green finance improve air quality? And what is the relationship between the two?
2. How can green finance improve the efficiency of improving air quality through the path of industrial structure optimization and energy structure transformation?
To address these issues, this study proposed a panel threshold model based on the provincial level. Industrial structure optimization and energy structure are used as threshold variables to elucidate the dynamic impact of green finance on air quality. And the heterogeneous impact of green finance on air quality is analyzed according to the regional distribution characteristics of industry and energy structure. Our findings will help policy makers to develop and implement differentiated green finance policies based on industrial and energy transition characteristics.
The remainder of this study is organized as follows. Section 2 reviews the literature on green finance and environmental sustainability. Section 3 describes the methodology and data sources. Section 4 shows the empirical results of the econometric model. Section 5 gives a discussion of the empirical results. Finally, the conclusions are summarized in Section 6.
2. Literature review
The greening of finance and environmental sustainability has drawn extensive attention (Xie et al., 2020; Çitil et al., 2023), but studies on green finance and air quality are limited. Such studies mainly examined the impact of green finance on carbon emissions and suggested that the development of green finance was effective in reducing CO2 emissions (Al Mamun et al., 2022; Xiong and Sun, 2022). After that, this finding has been confirmed in different industries such as agriculture, construction, manufacturing, and tourism (Glomsrød and Wei, 2018; Gholipour et al., 2022; Guo et al., 2022; Hailiang et al., 2023). However, existing studies on the impact of green finance development on environmental sustainability can be grouped into two categories.
The first category argued that green finance policies can sustainably reduce environmental pollution (Zhou et al., 2020; Mngumi et al., 2022). Zhang et al. (2022) empirically investigated the relationship between green financial policies and environmental quality using a difference-in-difference model and showed that green financial policies significantly reduced carbon emissions in heavily polluting industries, and the effect increased gradually over time. Liu et al. (2019) used the same approach to examine firm-level pollution emissions to reach the same conclusion. Furthermore, Chen and Chen (2021) used a spatial dynamic panel model to further show that the environmental benefits of green finance policies are spatially spillover, reducing carbon emissions in both local and neighboring areas.
In contrast, some other studies have argued that green finance policies have a limited impact on the sustainability of improving environmental quality, which can be reflected in the role of mechanisms between green finance and the environment. Regarding the level of resource allocation, green finance failed to achieve industrial restructuring due to its limited effect on the allocation of bank credit resources (Liu J.-Y. et al., 2017). Green finance was only effective in the short term to inhibit investment in energy-intensive industries and in the long term to inhibit the efficiency of renewable energy investment (He et al., 2019). From the perspective of corporate governance, the effect of green finance policies in reducing the performance of heavily polluting firms was weakened in a dynamic process due to the passive implementation of environmental responsibilities by enterprises (Zhang and Vigne, 2021). Furthermore, in terms of the effect of policy implementation, Zhang et al. (2022) used a difference-in-difference model to test the effect of implementing green financial reform pilot policies in China in 2017 and found that green financial policies could only partially reduce air pollutant emissions. Li P. et al. (2021) similarly confirmed that the effect of green finance policies on pollution reduction from large coal-fired power plants is limited, especially in areas with strong environmental regulation.
Although scholars made richer discussions on the relationship between green finance and environmental quality from numerous aspects, there still exist some limitations in this field. First, numerous scholars have mainly examined the relationships between green finance and carbon emissions, but air quality has not been adequately studied. Furthermore, the impact mechanisms of green finance and environmental quality have been well discussed, but their sustainable effects have not yet reached a consistent conclusion. Meo and Karim (2022) pointed out that the inhibitory effect of green finance on carbon emissions showed significant heterogeneity at different quartiles, however, there is no consensus on the dynamic impact of green finance on air quality. Third, the above studies mainly used econometric models including difference-in-difference model, spatial Durbin model, fixed effects model and Granger causality for empirical analysis. However, the main drawback of these methods is that they only discuss the linear relationship between variables and cannot reveal the dynamic impact of green finance on environmental quality.
Compared with previous studies, we have made the following contributions. (1) The dynamic effect of green finance on air quality was revealed by establishing a panel threshold model. This approach objectively determines the threshold value, avoids bias due to subjective division of samples, and enriches the literature on the dynamic effects of green finance on air quality. (2) We introduced industrial structure optimization and energy structure as threshold variables to investigate in depth the impact mechanism of green finance on air quality, which provides a reference for China to promote the improvement of green finance on air quality through the optimization path of industrial structure and energy structure. (3) In terms of indicator selection, the air quality index (AQI) comprised multiple air pollutants, which better reflects the comprehensive level of air pollution than single pollutants used in the past (Benchrif et al., 2021; Fung et al., 2022; Liu and Guo, 2022). In addition to coal, fossil fuels such as oil and natural gas were identified as major sources of air pollution. The percentage of non-clean energy consumption (coal, oil, and natural gas) was selected as a reverse indicator to measure the energy structure.
3. Data and methods
3.1. Variable selection and data sources
The air quality index (AQI) was a nonlinear composite indicator composed of multiple atmospheric pollutants and was widely used to measure the degree of atmospheric pollution (Liu H. et al., 2017; He et al., 2018). The air quality index (AQI) was used to quantify the air quality according to the Ambient Air Quality Standard (GB3095-2012) issued by the Ministry of Ecology and Environment of the People’s Republic of China (2012a), and was made available at the provincial and municipal levels in 2014. By relying on the validity and availability criteria, we selected panel data from 30 provincial administrative regions in China (excluding Tibet, Hong Kong, Macao, and Taiwan) from 2014 to 2020 for empirical analysis. Among them, the original data of air pollutants that make up the AQI were obtained from the CNRDS Platform. The data of energy consumption were from China Energy Statistics Yearbook. And the index data of industrial structure optimization and control variables were gathered from the National Bureau of Statistics. Given the presence of statistical bias, the interpolation method was used in this study to supplement the individual missing data and outliers in the dataset. The definitions of all variables used in this study and the original data sources were shown in Table 1.
3.1.1. Explained variable
In previous studies, single indicators, such as industrial dust emissions, SO2 emissions, and PM2.5, have been often used as air pollution indicators, whereas air pollution can be defined as a form of composite pollution (Wang et al., 2022). To this end, we relied on the study by Li and Li (2022) to select AQI, which can reflect air pollution effectively. In practice, AQI is a nonlinear dimensionless index that quantitatively describes the conditions of air quality, and the higher its value, the more severe the air pollution. In Figure 1 A–C show the air quality distributions of the 30 Chinese provinces in 2014, 2017 and 2020, respectively. On the whole, the air quality gradually improves from 2014 to 2020, but most northern provinces are still at high risk of pollution. The data processing followed the Ambient Air Quality Standard (GB3095-2012) and the Technical Regulation on Ambient Air Quality Index (HJ633-2012). The annual air quality index was calculated according to Equations (1) and (2) to ensure accuracy and validity (Ministry of Ecology and Environment of the People’s Republic of China, 2012b).
where denotes the air quality sub-index of pollutant item i, while is the mass concentration value of pollutant item i, and represent the high and low values of pollutant concentration limits similar to respectively, while and refer to the air quality sub-index corresponding to and respectively, and denotes the number of pollutant items.
3.1.2. Core explanatory variables
Green finance fundamentally reflects the financial services provided to improve the environment. We relied on the report “Guidelines for Establishing the Green Financial System” (The People’s Bank of China, 2016) released in China in 2016 to select four indicators: green credit, green securities, green insurance, and green investment. These indicators were used to construct a provincial-level green finance indicator system in China (see Table 2 for details). Unlike Huang and Chen (2022), we applied the percentage of A-share market value of provincial high-energy consumption enterprises and the percentage of industrial pollution control investment as the reverse indicators. In this way, we quantified green securities and green investment indicators due to more missing values in the data of total environmental pollution control investment and provincial environmental protection enterprises during the sample study period.
The entropy value method (Lee and Lee, 2022; Yin and Xu, 2022) was used to estimate green finance indicators. First, the indicators were standardized to eliminate incomparability. Positive and negative indicators were obtained according to Equations (3) and (4), respectively.
3.1.3. Threshold variables
As air pollutants mainly originate from industrial pollutant emissions and energy-intensive industries (Li X. et al., 2021), two approaches to green finance that can achieve environmental benefits have been highlighted including industrial structure upgrading (Wang et al., 2017) and energy consumption transformation (Song et al., 2021). On this basis, industrial structure optimization (OIS) and energy structure (ES) were selected as the threshold variables in this study. Of the variables, the ratio of the value of tertiary industry production to the value of secondary industry production was selected to quantify the optimization of industrial structure (Lu et al., 2021). In selecting energy structure indicators, most scholars often adhere to the share of coal consumption for measurement (Wang et al., 2019). However, as the high consumption of fossil energy is the main driver of air pollution in Chinese cities (Wang et al., 2022), we opted to use the share of non-clean energy (including total coal, oil, and natural gas consumption) as an indicator, reflecting the energy structure.
3.1.4. Control variables
The control variables used in this study include the level of economic development ( ), population size ( ), foreign direct investment ( ), and fixed asset investment ( ). As the traditional high-carbon economic development model results in serious air pollution, we selected GDP per capita as the economic growth indicator (Wang et al., 2019). Moreover, as population expansion tends to increase energy and resource consumption, thus exacerbating air pollution, we selected the number of year-end residents as a measure as well (Yi et al., 2020). The impacts of foreign direct investment on local environmental quality have not been previously elucidated. Thus, to elucidate its impact on air pollution in detail, we selected the actual foreign direct investment to quantify regional investment (Guo and Wang, 2023). Shi F. et al. (2022) have previously argued that investments in fixed assets generally exacerbate pollutant emissions. In this study, fixed asset investment was quantified using the share of total social fixed asset investment in the GDP. Table 3 summarizes the descriptive statistics of the main variables.
3.2. Panel threshold model
As outlined in the introduction, the impact of green finance on air quality may vary depending on the degree of industrial structure optimization and clean energy utilization at different intervals. Notably, a nonlinear relationship between the variables can exist. Traditional methods, such as adding quadratic terms, interaction terms, or group tests, are subjective in the selection of thresholds and do not allow for significance testing of threshold effects and differences in regression results, weakening the validity of parameter estimates. “Threshold regression” is a nonlinear econometric model proposed by Hansen (1999) that searches for threshold variables among the variables that reflect causality. The threshold was estimated from the sample data and tested for significant differences in the parameters of the sample groups according to the threshold (Che, 2013). The selection of threshold value and parameter estimation are more robust. Furthermore, compared with cross-panel regression models and quantile regression models (Çitil et al., 2023; Zaman, 2023), threshold regression allows multiple variables to be placed in the same system. This approach allows examining the effect of the independent variable on the dependent variable when there is a sudden structural change in the independent variable and other economic variables. In this study, the panel threshold regression model was set as follows:
represents the indicative function and takes a value of 0 when the expression in parentheses is false; otherwise, it takes a value of 1. Depending on whether the two threshold variables of industrial structure optimization ( ) and energy structure ( ) are greater than the threshold values and the sample interval can be divided into two regimes. These regimes are distinguished by the slope values and , and , respectively. represents the control variables, including the economic development level ( ), population size ( ), foreign direct investment ( ), and fixed asset investment ( ).
4. Results
4.1. Data stability test
The IPS, Fisher-ADF, and HT tests are often used as unit root tests for variables, while the long-term relationship between panel variables can be estimated by the Kao cointegration method. As shown in Table 4, all the explanatory variables in the three-unit root test passed the stationarity test, at least at the 10% significance level. Moreover, the t-statistics in the cointegration test were all significant, thus indicating the existence of a long-run equilibrium relationship between the variables.
4.2. Threshold regression results
4.2.1. Threshold effect test
In this study, industrial structure optimization and energy structure were selected as the threshold variables for the threshold model estimation. As shown in Table 5, the F-statistics of industrial structure optimization and energy structure were significant at the 1% level in the single-threshold model, while the double-and triple-threshold models failed the significance level test. These statistics suggest that the impact of green finance on air quality was potentially non-linear due to the influence of industrial and energy structures. The single threshold estimates of industrial structure optimization and energy structure were estimated to be 0.0858 and − 0.6237, respectively, before removing the logarithm, as well as 1.090 and 53.60%, respectively, after removing the logarithm.
According to the threshold model principle, the threshold estimate is the value of γ that corresponds to when the likelihood ratio statistic LR tends to zero. Figures 2A,B show the single-threshold model likelihood ratio function plots with industrial structure optimization and energy structure as the threshold variables, respectively. In particular, the single-threshold effect of green finance on air quality was significant at the 95% confidence interval. Notably, the consistency of the threshold effect test and likelihood ratio function plots further confirmed the nonlinear effect of green finance on air pollution.
4.2.2. Threshold model estimation
Tables 6, 7 illustrate the resultant panel regression with industrial structure optimization and energy structure as threshold variables, obtained alongside the threshold values. In these tables, columns (1) to (5) summarize the estimation results of the regressions with the gradual introduction of four control variables: economic development, population size, foreign direct investment, and fixed asset investment, respectively. Column (6) illustrates the relationship between green finance and air quality, affected by the threshold and control variables.
Table 6 summarizes the results of the threshold regression with industrial structure optimization as the threshold variable. The results of the six sets of models indicate that green finance significantly affected air pollution, thus resonating with the results of Zhang et al. (2021) that green finance can improve China’s environmental quality overall through industrial structure upgrading. However, this effect gradually weakened with the enhanced industrial structure optimization. In particular, when industrial structure optimization was below the threshold of 1.090, green finance reduced air pollution with a regression coefficient of −0.400 (significant at the 1% level), as shown in Table 6 (see column 6). When the industrial structure optimization exceeded the threshold, the regression coefficient decreased to −0.317, despite the negative impact on air pollution being significant at the 1% significance level. This indicates that by increasing the level of industrial structure optimization, one can weaken the negative relationship between green finance and air quality index.
Most importantly, in terms of the evolution of the provincial heterogeneity industrial structure optimization (as shown in Figure 3), most provinces and cities were in the first stage of industrial structure optimization from 2014–2017, and green finance effectively improved air quality. All regions except Fujian Province crossed the threshold (OIS = 1.090) by 2020, and the service industry developed rapidly. However, this effect was located at a low level of environmental benefits of green finance. This finding differs from Lan et al. (2023) that green finance showed a positive relationship with industrial pollution emissions in the second stage. Under the path of industrial structure optimization, green finance has a limited role in improving air quality, revealing to some extent the inadequate industry coverage of existing green finance standard system in China. The green finance policies in China mainly serve the high-carbon sector in the secondary industry and promote the green and low-carbon transformation of brown assets, resulting in the ineffectiveness of green finance in pollution control during the development of the service industry. This pattern can be explained by the following drivers. Huang and Chen (2022) argued that green finance is mainly driven by policies. Local officials often adopt different attitudes toward the implementation of green finance policies for different industries to achieve economic growth goals, resulting in a mismatch between green finance and industrial division, reducing the environmental benefits of green finance and even deteriorating the environment. Furthermore, the pollution emissions from the development of the service sector mainly originate from the consumption of fossil energy, and the promotion of clean energy is the most effective means to reduce pollution in the service sector. However, Ren et al. (2020) pointed out that the inhibiting effect of carbon intensity on the expansion of non-fossil energy sources in China and the insufficient green financial support for green resource development and efficient resource utilization have resulted in little investment flowing into green projects. The imbalance in the structure of green finance spending has weakened the environmental efficiency of green finance in the service sector.
As shown in Table 7, green finance exerted a significantly negative impact on air pollution when the energy structure was at the threshold variable, while the negative impact increased as the energy structure crossed the threshold. At ES ≤ 53.6%, the level of air pollution decreased by 0.15% for every 1% increase in the level of green finance, as indicated in Table 7 (see column 5). When the energy structure exceeded the threshold, each 1% increase in green finance improved air quality by 0.4% (significant at the 1% level). This finding implies that green finance had a greater effect on air quality improvement under a highly polluting energy-consumption structure. Considering the spatial and temporal distribution characteristics of the energy consumption structure, we find that the improvement of China’s energy consumption structure from 2010 to 2020 is not obvious. Only Beijing, Tianjin, Shanghai, and Hainan have significantly reduced the proportion of fossil energy consumption at this stage. There are still 86% of provinces and cities located in the second stage of the energy consumption structure (see Figure 4). Green finance is more effective in reducing pollution from a high-carbon fossil energy structure, indicating that China reduces air pollution mainly by increasing the utilization of fossil energy rather than directly using clean energy. The funding gap in R&D investment and the lack of technological innovation capacity have become major bottlenecks in the development of clean energy and new energy industries (Sheng et al., 2021; Pan and Dong, 2022), mainly in renewable hydrogen production and energy storage technologies, wind or tidal energy generation technologies. Notably, as indicated by Model 6, when industrial structure optimization was introduced, the absolute value of the regression coefficients of the core explanatory variables significantly decreased, thereby resonating with Hong et al. (2018) findings. In particular, the authors have previously reported that the transformation of the current energy structure depends on the upgrading of the industrial structure. This is because green finance provides funds for environmental pollution control, thereby bolstering technological innovation and upgrading production processes. In turn, these processes strengthen productivity, reduce resource and energy consumption, and promote energy efficiency, thereby improving air quality through these mechanisms (Ren et al., 2021).
Tables 6, 7 illustrate the comparison of the regression results of Model 6. As seen, the regression coefficients of green finance and air quality in the model with energy structure as the threshold are larger than those in the model with industrial structure as the threshold. Overall, the effect of green finance in improving air quality through optimizing the industrial structure was clearly better than that of the energy structure. Fan et al. (2023) pointed out that industrial structure upgrading has a significant positive effect on energy structure optimization, and there is a transmission path of “industrial structure upgrading-energy structure optimization-carbon emission reduction.” When the industrial structure optimization increases by 1%, the consumption proportion of the new energy increases by 0.0434% (Hong et al., 2018). Hence, China should use green financial policies to increase clean energy consumption by promoting industrial structure optimization, which can transform the dual structural effects represented by industrial and energy structures to achieve pollution reduction. At the same time, the influence direction of the control variables was found to be the same in all the models. Of these variables, population size had a significant positive effect on air pollution. The coefficient of foreign real direct investment was insignificantly negative, thus indicating that increasing foreign investment can somewhat mitigate air pollution. In contrast to the findings of Zhang et al. (2022), economic development and fixed asset investments significantly reduced the air quality index in this study. This finding suggests that steady high levels of economic growth and increased fixed investment are both important for environmental sustainability during the transition to a low-carbon economy (Song, 2021).
5. Discussion
The previous section examined a nonlinear relationship between green finance and air quality using two influencing mechanisms: industrial structure and energy structure. Furthermore, this study elucidated regional heterogeneity of the environmental benefits of green finance using the regional variables of the influencing mechanisms, such as urbanization level and resource dependency level. In general, urbanization and resource dependence both tend to affect the industrial and energy consumption structures of a region. On one hand, the urbanization process is often accompanied by industrial restructuring and energy transition, thereby promoting the economic growth and affecting environmental quality (Bai et al., 2018), with significant regional differences (Huo et al., 2021). On the other hand, the energy structure is mainly related to regional resource endowment and resource dependence, but resource dependence is the key to the “resource curse” phenomenon and environmental degradation (Brunnschweiler and Bulte, 2008). Therefore, the ratio of fixed asset investment in the mining industry (Zheng et al., 2020) was selected to reflect the level of resource dependence. To this end, the sample regions were classified, whereas the provinces with ratios exceeding the mean are defined as “resource-based regions” and those with ratios lower than the mean are defined as “non-resource-based regions.” At the same time, the sample was classified according to the mean urbanization ratio according to Zhang et al. (2021). In particular, the provinces above the mean were considered as urbanized regions, and the provinces below the mean were considered as under-urbanized regions. Table 8 summarizes the regression results of the panel fixed effects and instrumental variables methods for the sample regions.
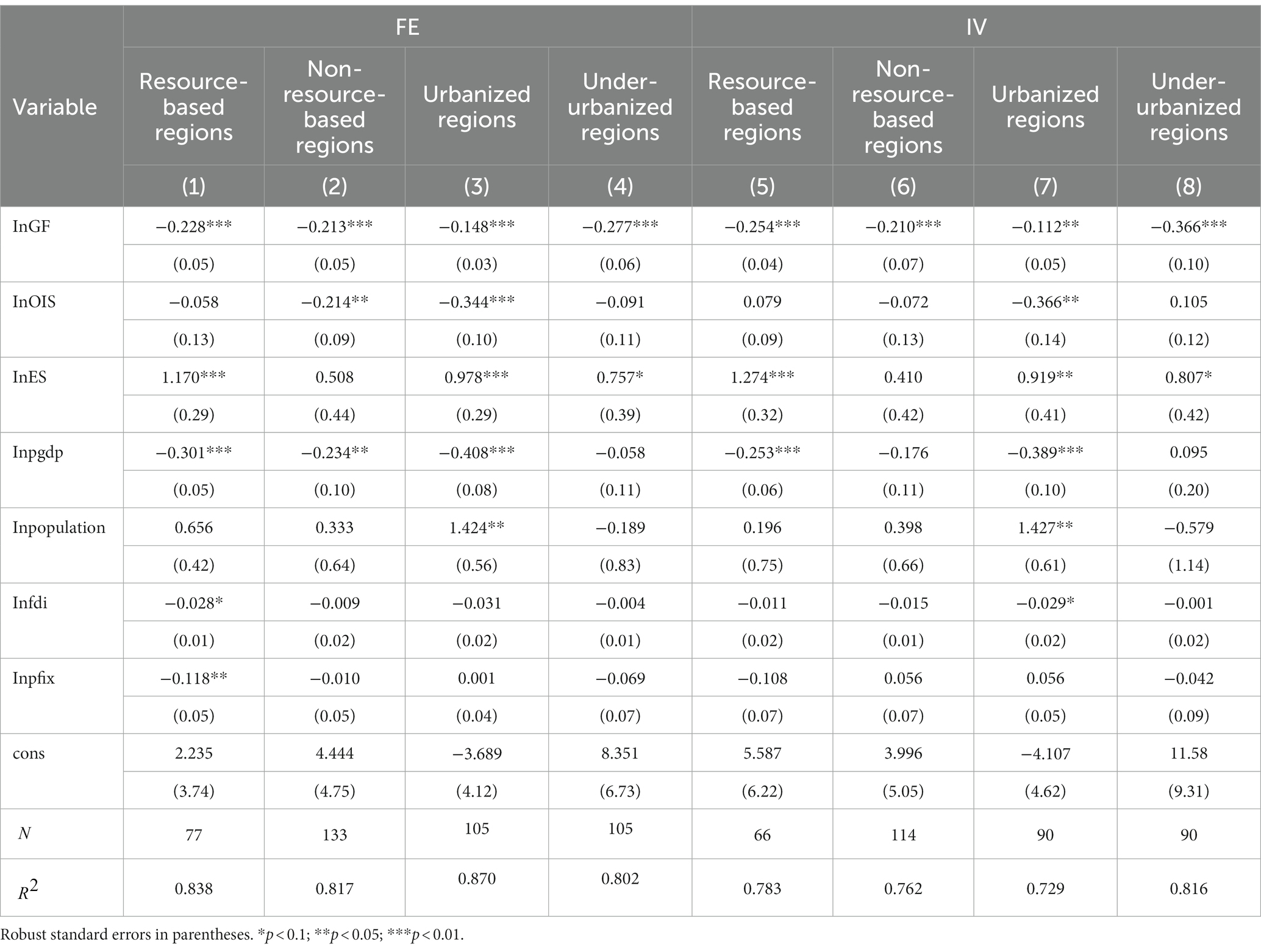
Table 8. Analysis of the spatial heterogeneity impact based on regional differences in threshold variables.
As shown in Table 8, green finance considerably suppressed air pollution in terms of both resource dependence and urbanization level, thus confirming the threshold model results. Specifically, the effect of green finance on air quality was better in the resource-based regions compared to the non-resource-based ones. However, the effect of industrial structure optimization on improving the environment was not significant. This finding suggests that resource dependence hampered the improvements of the industrial structure but exacerbated the negative effect of green finance on air pollution. Interestingly, these findings differ from the Li et al. (2019) results, potentially because the policy-related incentives of green finance in resource-based regions had a more pronounced effect on energy efficiency (Peng and Zheng, 2021). Notably, the energy structure exhibited a strong positive correlation with air pollution, while the estimated coefficients of foreign direct investment and fixed asset investment were significantly negative. Therefore, it is necessary for Chinese government to consider a combination of green financial policies and market instruments to further promote environmentally sustainable development. For instance, by increasing foreign direct investment and fixed asset investment, one can promote the introduction of high technology and industrial structure adjustment in resource-based regions of China, such as Shanxi, Inner Mongolia, and Xinjiang. Moreover, in this way, green finance would play greater role in promoting the improvement of industrial structure and the transformation of energy consumption structure (Chen, 2018).
In terms of the urbanization process, the inhibitory effect of green finance on air pollution was stronger in under-urbanized regions than in urbanized regions. This indicates that urbanization processes weaken the environmental benefits of green finance due to the current incompatibility between green finance and urbanization development in China (Dong et al., 2021). On one hand, the levels of both environmental pollution and green finance development were relatively low in under-urbanized regions, but the coordination between the two was high. On the other hand, the tertiary industry in urbanized regions was relatively developed, while green finance had a minor effect on reducing the pollution, induced by energy consumption in the process of tertiary industry development. Overall, the size of resident population and the proportion of fixed asset investment such as infrastructure were larger in urbanized areas than in urbanized regions. The regression results showed that the level of air pollution increased by 1.424% for every 1% increase in population size (significant at the 5% level), while the regression coefficient for fixed asset investment size was 0.001. This indicates that expanding the population size and increasing the proportion of fixed asset investment such as infrastructure in urbanized areas may exacerbate air pollution. Hence, urbanized regions should control the total population and fixed asset investment appropriately. In under-urbanized regions, the regression coefficients of both population size and fixed asset investment were negative, suggesting that appropriate expansion of population size and increase in-vestment in fixed assets can facilitate the improvement of air quality of under-urbanized regions.
As a significant spatial spillover effect of air pollution had been previously reported (Hao et al., 2021), this study further explored the heterogeneous impact of green finance on air quality based on geographical distribution. To this end, the country was divided into the eastern region (Beijing, Tianjin, Hebei, Shanghai, Jiangsu, Zhejiang, Fujian, Shandong, Guangdong, and Hainan), central region (Shanxi, Anhui, Jiangxi, Henan, Hubei, and Hunan), western region (Inner Mongolia, Guangxi, Chongqing, Sichuan, Guizhou, Yunnan, Shaanxi, Gansu, Qinghai, Ningxia, and Xinjiang), and north-eastern region (Liaoning, Jilin, and Heilongjiang) according to the latest classification criteria of the National Bureau of Statistics in China. As shown in Figure 5, the negative impacts of green finance on air quality exhibited significant spatial variability. Furthermore, the effect of improving air quality was significantly higher in the eastern and western regions than in the central and northeastern regions, while the inhibitory effect was the strongest in the western region and weakest in the northeastern region. PM2.5 and NO2 concentrations were the main air pollutants in the eastern region, due to the dense urban population and manufacturing industries in the region (Wang et al., 2022). The increase of heavy industries and automobiles with high pollution and energy consumption led to the emission of many air pollutants, especially, industrial coal combustion and centralized heating in winter can aggravate air pollution (Miao et al., 2022).
The model regression results in Table 9 suggest that the eastern region should control energy consumption emissions in the process of population size and industrial structure transformation, such as emissions from coal-fired power plants and heating. Moreover, the efficiency of green finance policies should be maximized and foreign investment attracted to encourage the development of clean energy and technological innovation, thus ultimately promoting sustainable environmental development. The effect of green finance on improving air quality in the western region was significantly higher than in other regions, mainly because of the green finance pilot policy, which substantially bolstered the coordinated development of production capacity in the western region. As a crucial energy and raw material base in China, the central region was rich in coal and oil resources. Numerous coal-fired power plants and industrial boilers emitted large amounts of air pollutants, such as sulfur dioxide and carbon monoxide. The regression results showed that the effect of green finance on air quality in the central region was less significant, potentially because green finance promoted industrial low-carbon development with a time lag and mainly relied on green credit and green bonds to support green low-carbon industries, resulting in limited support from a single financing channel to local green low-carbon enterprises for green production, which was not conducive to the adjustment of the energy consumption structure of the region (Sun and Chen, 2022). The significant negative effect of fixed asset investment on air quality index (see Table 9) suggested that the adjustment of the fixed asset investment structure, such as increasing investment in manufacturing and transportation, helped curbing regional air pollution. The impact of green finance on air quality in the northeast region was not significant because the region is still facing such problems as resource depletion, unbalanced industrial structure, and population loss nowadays. Thus, by promoting technological advancements of industrial structure and rationalizing their use at this stage, one can further improve air quality in the northeast China.
6. Conclusions and recommendations
This study elucidated the impact of green finance on air quality in China using panel data from 30 Chinese provinces from 2014 to 2020. The threshold effect of green finance on air pollution was confirmed, thereby indicating that green finance had a nonlinear effect on air quality.
The analysis showed that green finance significantly improved air quality, but its role in such improvement gradually weakened with the optimization of the industrial structure and with the improvement of clean energy efficiency. At the same time, green finance had a greater inhibitory effect on air pollution through optimizing the industrial structure than that of the energy structure. Moreover, significant regional heterogeneity in the impact of green finance on air quality was identified. Compared to non-resource-based regions, the effect of green finance on improving air quality was stronger in resource-based regions, indicating that resource dependence strengthens the impact of green finance on air quality. The inhibitory effect of green finance on air pollution was stronger in under-urbanized regions than in urbanized regions, indicating that urbanization weakens the environmental benefits of green finance. In terms of geographical distribution, green finance suppressed air pollution in the East, Central, West, and Northeast regions, but the significance was higher in the East and West than in the Central and Northeast regions. Notably, the strongest effect was identified in the West, followed by the East and Central regions, and the worst effect was registered in the Northeast.
Overall, our findings provide a new theoretical foundation for promoting further improvements in green finance policies and emission reduction measures in China. First, it is further important to elucidate the characteristics of green finance to enhance air quality and limit of the improvement effect. In this light, the emission reduction path of green finance was formulated under different levels of industrial structure optimization and energy consumption. For the regions with a high level of industrial transformation and upgradation with large proportion of clean energy consumption, the targets of green finance implementation should rather focus on pollution emissions in the development of service industries. Second, the regional heterogeneity analysis indicated that resource-based regions should increase investment in fixed assets, actively introduce foreign capital, expand the scale of high-tech industries in the region, and make full use of green finance to guide the transformation and upgrading of highly polluting and energy-consuming industries. In this way, the achievement of economic and environmental goals can be facilitated. Finally, the shrinking effect of urbanization on the environmental effect of green finance suggests that by strictly adhering to the coordinated development of urbanization and green finance when formulating economic development strategies, provincial governments and relevant departments can potentially maximize the environmental benefits of green finance in China.
While our findings have made new findings on the relationship between green finance and air quality from the perspective of industrial optimization and energy transition, it still has some limitations. First, we used macro data for the study due to limitations in the availability of green finance data at the city, district, and county levels. The relationships among variables at the city, district, and county levels can be used for future studies. Also, the indicators of green fund and environmental liability insurance have not been included in the green finance index with sample size limitation. Furthermore, methodologically, panel threshold models provide quite an accurate way to show the overall effect of our study. Air pollution and financial development tend to be typically spatially correlated and can also be further investigated empirically using spatial econometric models. Moreover, we focus on analyzing the effects and dynamic changes of green finance on air quality at different stages of industrial and energy structure development. It is possible to make further comparisons of the impacts of various green finance instruments on different air pollutants in the future, as well as to explore the actual efficiency of green finance in promoting synergistic effects on pollution reduction and carbon reduction.
Data availability statement
The original contributions presented in the study are included in the article/supplementary material, further inquiries can be directed to the corresponding author.
Author contributions
WX: conceptualization, methodology, and validation. QQ: writing—original draft preparation and supervision. LG: writing—review and editing and investigation. All authors contributed to the article and approved the submitted version.
Funding
This work was supported by the Chongqing Social Science Planning Project (2019ZDGL06); Chongqing municipal departments commissioned project (2171090).
Acknowledgments
We thank the editor and referees, whose remarks have been very constructive and inspiring in preparing the final version of the paper. We are solely responsible for the opinions expressed in this paper.
Conflict of interest
The authors declare that the research was conducted in the absence of any commercial or financial relationships that could be construed as a potential conflict of interest.
Publisher’s note
All claims expressed in this article are solely those of the authors and do not necessarily represent those of their affiliated organizations, or those of the publisher, the editors and the reviewers. Any product that may be evaluated in this article, or claim that may be made by its manufacturer, is not guaranteed or endorsed by the publisher.
References
Al Mamun, M., Boubaker, S., and Nguyen, D. K. (2022). Green finance and decarbonization: evidence from around the world. Financ. Res. Lett. 46:102807. doi: 10.1016/j.frl.2022.102807
Bai, Y., Deng, X., Jiang, S., Zhang, Q., and Wang, Z. (2018). Exploring the relationship between urbanization and urban eco-efficiency: evidence from prefecture-level cities in China. J. Clean. Prod. 195, 1487–1496. doi: 10.1016/j.jclepro.2017.11.115
Benchrif, A., Wheida, A., Tahri, M., Shubbar, R. M., and Biswas, B. (2021). Air quality during three covid-19 lockdown phases: AQI, PM2.5 and NO2 assessment in cities with more than 1 million inhabitants. Sustain. Cities Soc. 74:103170. doi: 10.1016/j.scs.2021.103170
Brunnschweiler, C. N., and Bulte, E. H. (2008). The resource curse revisited and revised: a tale of paradoxes and red herrings. J. Environ. Econ. Manag. 55, 248–264. doi: 10.1016/j.jeem.2007.08.004
Che, C. M. (2013). Panel threshold analysis of Taiwan's outbound visitors. Econ. Model. 33, 787–793. doi: 10.1016/j.econmod.2013.06.006
Chen, C. (2018). Impact of China's outward foreign direct investment on its regional economic growth. China World Econ. 26, 1–21. doi: 10.1111/cwe.12240
Chen, X., and Chen, Z. (2021). Can green finance development reduce carbon emissions? Empirical evidence from 30 Chinese provinces. Sustain-Basel 13, 12137–12155. doi: 10.3390/su132112137
Çitil, M., İlbasmış, M., Olanrewaju, V. O., Barut, A., Karaoğlan, S., and Ali, M. (2023). Does green finance and institutional quality play an important role in air quality. Environ. Sci. Pollut. R. 1–15. doi: 10.1007/s11356-023-26016-2
Dong, G., Ge, Y., Zhu, W., Qu, Y., and Zhang, W. (2021). Coupling coordination and spatiotemporal dynamic evolution between green urbanization and green finance: a case study in China. Front. Env. Sci-Switz. 8:621846. doi: 10.3389/fenvs.2020.621846
Fan, G., Zhu, A., and Xu, H. (2023). Analysis of the impact of industrial structure upgrading and energy structure optimization on carbon emission reduction. Sustain-Basel 15, 3489–3512. doi: 10.3390/su15043489
Feng, Y., Ning, M., Lei, Y., Sun, Y., Liu, W., and Wang, J. (2019). Defending blue sky in China: effectiveness of the “air pollution prevention and control action plan” on air quality improvements from 2013 to 2017. J. Environ. Manag. 252:109603. doi: 10.1016/j.jenvman.2019.109603
Fung, P. L., Sillanpää, S., Niemi, J. V., Kousa, A., Timonen, H., Zaidan, M. A., et al. (2022). Improving the current air quality index with new particulate indicators using a robust statistical approach. Sci. Total Environ. 844:157099. doi: 10.1016/j.scitotenv.2022.157099
Gan, T., Yang, H., and Liang, W. (2021). How do urban haze pollution and economic development affect each other? Empirical evidence from 287 Chinese cities during 2000–2016. Sustain. Cities Soc. 65:102642. doi: 10.1016/j.scs.2020.102642
Gholipour, H. F., Arjomandi, A., and Yam, S. (2022). Green property finance and CO2 emissions in the building industry. Glob. Financ. J. 51:100696. doi: 10.1016/j.gfj.2021.100696
Glomsrød, S., and Wei, T. (2018). Business as unusual: the implications of fossil divestment and green bonds for financial flows, economic growth and energy market. Energy Sustain. Dev. 44, 1–10. doi: 10.1016/j.esd.2018.02.005
Guo, X., and Wang, J. (2023). Outward foreign direct investment, green financial development, and green total factor productivity: evidence from China. Environ. Sci. Pollut. R. 1–16. doi: 10.1007/s11356-023-25651-z
Guo, L., Zhao, S., Song, Y., Tang, M., and Li, H. (2022). Green finance, chemical fertilizer use and carbon emissions from agricultural production. Agriculture 12:313. doi: 10.3390/agriculture12030313
Hailiang, Z., Chau, K. Y., and Waqas, M. (2023). Does green finance and renewable energy promote tourism for sustainable development: empirical evidence from China. Renew. Energy 207, 660–671. doi: 10.1016/j.renene.2023.03.032
Hansen, B. E. (1999). Threshold effects in non-dynamic panels: estimation, testing, and inference. J. Econ. 93, 345–368. doi: 10.1016/s0304-4076(99)00025-1
Hao, Y., Gai, Z., Yan, G., Wu, H., and Irfan, M. (2021). The spatial spillover effect and nonlinear relationship analysis between environmental decentralization, government corruption and air pollution: evidence from China. Sci. Total Environ. 763:144183. doi: 10.1016/j.scitotenv.2020.144183
He, L., Liu, R., Zhong, Z., Wang, D., and Xia, Y. (2019). Can green financial development promote renewable energy investment efficiency? A consideration of bank credit. Renew. Energy 143, 974–984. doi: 10.1016/j.renene.2019.05.059
He, L., Wu, M., Wang, D., and Zhong, Z. (2018). A study of the influence of regional environmental expenditure on air quality in China: the effectiveness of environmental policy. Environ. Sci. Pollut. R. 25, 7454–7468. doi: 10.1007/s11356-017-1033-8
Hong, Y., Can, P., Xiaona, Y., and Ruixue, L. (2018). Does change of industrial structure affect energy consumption structure: a study based on the perspective of energy grade calculation. Energ. Explor. Exploit. 37, 579–592. doi: 10.1177/0144598718784032
Huang, Y., and Chen, C. (2022). The spatial spillover and threshold effect of green finance on environmental quality: evidence from China. Environ. Sci. Pollut. R. 29, 17487–17498. doi: 10.1007/s11356-021-16892-x
Huo, T., Cao, R., Du, H., Zhang, J., Cai, W., and Liu, B. (2021). Nonlinear influence of urbanization on China's urban residential building carbon emissions: new evidence from panel threshold model. Sci. Total Environ. 772:145058. doi: 10.1016/j.scitotenv.2021.145058
Lan, J., Wei, Y., Guo, J., Li, Q., and Liu, Z. (2023). The effect of green finance on industrial pollution emissions: evidence from China. Resour. Policy 80:103156. doi: 10.1016/j.resourpol.2022.103156
Lee, C.-C., and Lee, C.-C. (2022). How does green finance affect green total factor productivity? Evi. China Energ. Econ. 107:105863. doi: 10.1016/j.eneco.2022.105863
Li, M., Hamawandy, N. M., Wahid, F., Rjoub, H., and Bao, Z. (2021). Renewable energy resources investment and green finance: evidence from China. Resour Policy 74:102402. doi: 10.1016/j.resourpol.2021.102402
Li, Z., and Li, C. (2022). How industrial upgrading can improve China’s air quality: empirical analysis based on multilevel growth model. Environ. Sci. Pollut. R. 29, 54456–54466. doi: 10.1007/s11356-022-19719-5
Li, P., Lin, Z., Du, H., Feng, T., and Zuo, J. (2021). Do environmental taxes reduce air pollution? Evidence from fossil-fuel power plants in China. J. Environ. Manag. 295:113112. doi: 10.1016/j.jenvman.2021.113112
Li, Z., Shao, S., Shi, X., Sun, Y., and Zhang, X. (2019). Structural transformation of manufacturing, natural resource dependence, and carbon emissions reduction: evidence of a threshold effect from China. J. Clean. Prod. 206, 920–927. doi: 10.1016/j.jclepro.2018.09.241
Li, X., Xu, Y., and Yao, X. (2021). Effects of industrial agglomeration on haze pollution: a Chinese city-level study. Energ Policy 148:111928. doi: 10.1016/j.enpol.2020.111928
Liang, H., Zhou, X., Zhu, Y., Li, D., Jing, D., Su, X., et al. (2023). Association of outdoor air pollution, lifestyle, genetic factors with the risk of lung cancer: a prospective cohort study. Environ. Res. 218:114996. doi: 10.1016/j.envres.2022.114996
Liu, H., Fang, C., Zhang, X., Wang, Z., Bao, C., and Li, F. (2017). The effect of natural and anthropogenic factors on haze pollution in Chinese cities: a spatial econometrics approach. J. Clean. Prod. 165, 323–333. doi: 10.1016/j.jclepro.2017.07.127
Liu, X., and Guo, H. (2022). Air quality indicators and AQI prediction coupling long-short term memory (LSTM) and sparrow search algorithm (SSA): a case study of Shanghai. Atmos. Pollut. Res. 13:101551. doi: 10.1016/j.apr.2022.101551
Liu, X., Wang, E., and Cai, D. (2019). Green credit policy, property rights and debt financing: quasi-natural experimental evidence from China. Financ. Res. Lett. 29, 129–135. doi: 10.1016/j.frl.2019.03.014
Liu, J.-Y., Xia, Y., Fan, Y., Lin, S.-M., and Wu, J. (2017). Assessment of a green credit policy aimed at energy-intensive industries in China based on a financial CGE model. J. Clean. Prod. 163, 293–302. doi: 10.1016/j.jclepro.2015.10.111
Lu, J., Li, B., Li, H., and Al-Barakani, A. (2021). Expansion of city scale, traffic modes, traffic congestion, and air pollution. Cities 108:102974. doi: 10.1016/j.cities.2020.102974
Ma, Q., Liu, X., Wang, W.-G., and Xue, J. (2023). Natural resources extraction and COP26 target: evaluating the role of green finance. Resour. Policy 82:103432. doi: 10.1016/j.resourpol.2023.103432
Meo, M. S., and Karim, M. Z. A. (2022). The role of green finance in reducing CO2 emissions: an empirical analysis. Borsa Istanbul Rev. 22, 169–178. doi: 10.1016/j.bir.2021.03.002
Miao, L., Liu, C., Yang, X., Kwan, M.-P., and Zhang, K. (2022). Spatiotemporal heterogeneity analysis of air quality in the Yangtze River Delta. China. Sustain. Cities Soc. 78:103603. doi: 10.1016/j.scs.2021.103603
Ministry of Ecology and Environment of the People’s Republic of China (2012a). Ambient air quality standard (GB3095-2012) [online]. Available at: https://www.mee.gov.cn/ywgz/fgbz/bz/bzwb/dqhjbh/dqhjzlbz/201203/W020120410330232398521.pdf [Accessed 24 May 2022].
Ministry of Ecology and Environment of the People’s Republic of China (2012b). Technical regulation on ambient air quality index on trial (HJ633-2012) [online]. Available at: https://www.mee.gov.cn/ywgz/fgbz/bz/bzwb/jcffbz/201203/W020120410332725219541.pdf [Accessed 24 May 2022].
Mngumi, F., Shaorong, S., Shair, F., and Waqas, M. (2022). Does green finance mitigate the effects of climate variability: role of renewable energy investment and infrastructure. Environ. Sci. Pollut. R. 29, 59287–59299. doi: 10.1007/s11356-022-19839-y
Pan, Y., and Dong, F. (2022). Dynamic evolution and driving factors of new energy development: fresh evidence from China. Technol. Forecast. Soc. Chang. 176:121475. doi: 10.1016/j.techfore.2022.121475
Peng, J., and Zheng, Y. (2021). Does environmental policy promote energy efficiency? Evidence from China in the context of developing green finance. Front. Env. Sci-Switz. 9:299. doi: 10.3389/fenvs.2021.733349
Ren, S., Hao, Y., and Wu, H. (2021). How does green investment affect environmental pollution? Evidence from China. Environ. Resour. Econ. 81, 25–51. doi: 10.1007/s10640-021-00615-4
Ren, X., Shao, Q., and Zhong, R. (2020). Nexus between green finance, non-fossil energy use, and carbon intensity: empirical evidence from China based on a vector error correction model. J. Clean. Prod. 277:122844. doi: 10.1016/j.jclepro.2020.122844
Scholtens, B. (2017). Why finance should care about ecology. Trends Ecol. Evol. 32, 500–505. doi: 10.1016/j.tree.2017.03.013
Sheng, Z., Yuan, G., Xiru, D., Minglong, Z., Yi, W., and Yu, H. (2021). Research on high quality evaluation and influencing factors of China energy finance: evidence from A-share new energy companies. Front. Env. Sci-Switz. 9:662424. doi: 10.3389/fenvs.2021.662424
Shi, F., Ding, R., Li, H., and Hao, S. (2022). Environmental regulation, digital financial inclusion, and environmental pollution: an empirical study based on the spatial spillover effect and panel threshold effect. Sustain-Basel 14:6869. doi: 10.3390/su14116869
Shi, Q., Zheng, B., Zheng, Y., Tong, D., Liu, Y., Ma, H., et al. (2022). Co-benefits of CO2 emission reduction from China’s clean air actions between 2013-2020. Nat. Commun. 13:5061. doi: 10.1038/s41467-022-32656-8
Song, Z. (2021). Economic growth and carbon emissions: estimation of a panel threshold model for the transition process in China. J. Clean. Prod. 278:123773. doi: 10.1016/j.jclepro.2020.123773
Song, M., Xie, Q., and Shen, Z. (2021). Impact of green credit on high-efficiency utilization of energy in China considering environmental constraints. Energ. Policy 153:112267. doi: 10.1016/j.enpol.2021.112267
Sun, H., and Chen, F. (2022). The impact of green finance on China's regional energy consumption structure based on system GMM. Resour. Policy 76:102588. doi: 10.1016/j.resourpol.2022.102588
The People’s Bank of China (2016). Guidelines for establishing the green financial system (Yinfa (2016) no.228) [online]. Available at: http://enrccef.cufe.edu.cn/info/1002/1171.htm (Accessed 24 May 2022).
Triki, R., Kahouli, B., Tissaoui, K., and Tlili, H. (2023). Assessing the link between environmental quality, green finance, health expenditure, renewable energy, and technology innovation. Sustainability-Basel [Online] 15:4286. doi: 10.3390/su15054286
Wang, Y., Duan, X., Liang, T., Wang, L., and Wang, L. (2022). Analysis of spatio-temporal distribution characteristics and socioeconomic drivers of urban air quality in China. Chemosphere 291:132799. doi: 10.1016/j.chemosphere.2021.132799
Wang, Q., and Fan, Z. (2023). Green finance and investment behavior of renewable energy enterprises: a case study of China. Int. Rev. Financ. Anal. 87:102564. doi: 10.1016/j.irfa.2023.102564
Wang, S., Li, C., and Zhou, H. (2019). Impact of China's economic growth and energy consumption structure on atmospheric pollutants: based on a panel threshold model. J. Clean. Prod. 236:117694. doi: 10.1016/j.jclepro.2019.117694
Wang, C., Nie, P.-Y., Peng, D.-H., and Li, Z.-H. (2017). Green insurance subsidy for promoting clean production innovation. J. Clean. Prod. 148, 111–117. doi: 10.1016/j.jclepro.2017.01.145
Wang, L., Shi, T., and Chen, H. (2023). Air pollution and infant mortality: evidence from China. Econ. Human Biol. 49:101229. doi: 10.1016/j.ehb.2023.101229
Xie, H., Ouyang, Z., and Choi, Y. (2020). Characteristics and influencing factors of green finance development in the Yangtze River Delta of China: analysis based on the spatial Durbin model. Sustain-Basel [Online] 12:9753. doi: 10.3390/su12229753
Xiong, Q., and Sun, D. (2022). Influence analysis of green finance development impact on carbon emissions: an exploratory study based on fsQCA. Environ. Sci. Pollut. R., 1–12. doi: 10.1007/s11356-021-18351-z
Yi, M., Wang, Y., Sheng, M., Sharp, B., and Zhang, Y. (2020). Effects of heterogeneous technological progress on haze pollution: evidence from China. Ecol. Econ. 169:106533. doi: 10.1016/j.ecolecon.2019.106533
Yin, X., and Xu, Z. (2022). An empirical analysis of the coupling and coordinative development of China's green finance and economic growth. Resour. Policy 75:102476. doi: 10.1016/j.resourpol.2021.102476
Zaman, K. (2023). A Note on Cross-Panel Data Techniques. Latest Dev. Econom. 1, 1–7. doi: 10.5281/zenodo.7565625
Zeng, Y., Wang, F., and Wu, J. (2022). The impact of green finance on urban haze pollution in China: a technological innovation perspective. Energies [Online] 15:801. doi: 10.3390/en15030801
Zhang, A., Deng, R., and Wu, Y. (2022). Does the green credit policy reduce the carbon emission intensity of heavily polluting industries?-evidence from China's industrial sectors. J. Environ. Manag. 311:114815. doi: 10.1016/j.jenvman.2022.114815
Zhang, S., Li, Z., Ning, X., and Li, L. (2021). Gauging the impacts of urbanization on CO2 emissions from the construction industry: evidence from China. J. Environ. Manag. 288:112440. doi: 10.1016/j.jenvman.2021.112440
Zhang, K., Li, Y., Qi, Y., and Shao, S. (2021). Can green credit policy improve environmental quality? J. Environ. Manage. 298:113445. doi: 10.1016/j.jenvman.2021.113445
Zhang, D., and Vigne, S. A. (2021). The causal effect on firm performance of China's financing-pollution emission reduction policy: firm-level evidence. J. Environ. Manag. 279:111609. doi: 10.1016/j.jenvman.2020.111609
Zhang, A., Wang, S., and Liu, B. (2022). How to control air pollution with economic means? Exploration of China's green finance policy. J. Clean. Prod. 353:131664. doi: 10.1016/j.jclepro.2022.131664
Zheng, Y., Peng, J., Xiao, J., Su, P., and Li, S. (2020). Industrial structure transformation and provincial heterogeneity characteristics evolution of air pollution: evidence of a threshold effect from China. Atmos. Pollut. Res. 11, 598–609. doi: 10.1016/j.apr.2019.12.011
Keywords: green finance, air quality, non-linear effects, threshold model, China
Citation: Xiang W, Qi Q and Gan L (2023) Non-linear effects of green finance on air quality in China: New evidence from a panel threshold model. Front. Ecol. Evol. 11:1162137. doi: 10.3389/fevo.2023.1162137
Edited by:
Yuqiang Zhang, Shandong University, ChinaReviewed by:
Ying Zhu, Xi'an University of Architecture and Technology, ChinaKhalid Zaman, The University of Haripur, Pakistan
Copyright © 2023 Xiang, Qi and Gan. This is an open-access article distributed under the terms of the Creative Commons Attribution License (CC BY). The use, distribution or reproduction in other forums is permitted, provided the original author(s) and the copyright owner(s) are credited and that the original publication in this journal is cited, in accordance with accepted academic practice. No use, distribution or reproduction is permitted which does not comply with these terms.
*Correspondence: Lei Gan, 20200301007@cqu.edu.cn