- MOE Key Laboratory of Regional Energy and Environmental Systems Optimization, Resources and Environmental Research Academy, North China Electric Power University, Beijing, China
In order to mitigate global warming and help the country achieve its carbon peaking and carbon neutrality targets at the earliest possible time, the emission-control companies should accomplish the carbon compliance in accordance with relevant national policies and regulations. However, these companies frequently face the failure risk of carbon compliance subjected to various factors, including the national carbon quota policy, local carbon market situation, the verification of carbon offset projects, as well as the effectiveness of carbon reduction technologies. To help the enterprises avoid the risk of carbon-compliance failure and design rational carbon asset management strategy, in this research, the innovative combination of interpretive structural modeling (ISM), Bayesian network model, risk calculation and sensitivity analysis method was formulated. Firstly, the ISM method was used to establish a hierarchical relationship of risk factors that contribute to the failure of carbon compliance. Secondly, the probability prediction model of carbon-compliance failure risk based on the Bayesian network model was established by aid of the Netica software. Thirdly, the risk value of enterprise's carbon compliance failure was quantitatively calculated based on its production operation and carbon asset management. Finally, the sensitivity analysis method was used to identify critical risk factors and design risk control measures for six well-known domestic enterprises, laying good foundation for improving the success rate of carbon compliance and facilitating low-carbon green transformation. Compared to traditional qualitative risk assessment method, this combined approach is capable of realizing the quantitative evaluation of failure risk based on comprehensive investigation and analysis of the production and operational situation, which provides effective technical support to enhance enterprise's compliance awareness and improve low carbon competitiveness.
1. Introduction
Global warming is a pressing issue that profoundly affects the development and progress of human society. Developing a low-carbon economy characterized by low energy consumption and low GHG (greenhouse gas) emissions and establishing a low-carbon society have become a basic consensus and critical strategy for countries around the world in order to mitigate climate change and achieve sustainable development (Trotter et al., 2022; Pentz and Klenk, 2023). In the 75th session of the United Nations General Assembly, President Xi Jinping announced the strategic goal that ‘China will improve its independent national contribution, adopt more vigorous policies and measures, strive to reach the peak CO2 emission by 2030, and achieve the carbon neutrality by 2060'. This announcement shows the direction for the next phase of national energy structure adjustment and green development (Zhu et al., 2018). Under the context of energy conservation, carbon emission reduction, and low-carbon development, the emission permits trade market of carbon (abbreviated to the carbon market) of China was established in 2021. The market mechanism and trading rules are capable of regulating and adjusting the carbon asset management strategies of participating parties, significantly improving the flexibility of carbon reduction strategies. This way helps high-emission companies to establish a conception of low-carbon development and realize the goals of carbon peaking and carbon neutrality. Overall, the establishment of the carbon market in China is a crucial step toward the country's green development and transition (Nie, 2022).
Emission control enterprises are a crucial part of the carbon market and are driven by carbon compliance to participate in carbon emission trading, which is the only way for them to complete low-carbon transformation and realize green development. With the rapid development of the carbon market, emission control companies with high GHG emissions will face a bigger challenge. On the one hand, the approaching deadline of the ‘carbon peaking and carbon neutrality goals' has imposed more stringent carbon reduction requirements on these enterprises, leading to tremendous pressure on their production and operation. On the other hand, these enterprises are affected by various factors such as policies, markets, and technologies in fulfilling their compliance and emission reduction obligations, resulting in the failure risk of carbon compliance. Therefore, the accurate evaluation of the carbon compliance failure risk and effective regulation of risk factors are beneficial to enhance enterprises' compliance awareness, increase their enthusiasm to participate in a market transaction, and ultimately realize their green and low-carbon transformation while maintaining sustainable and healthy development. To assist enterprises in completing carbon compliance and avoiding the compliance failure risk, many scholars and experts have conducted extensive and in-depth research on the risk evaluation of enterprises' carbon compliance (Blanco and Rodrigues, 2008; Chevallier et al., 2009; Fan and Wang, 2014; Chen, 2015; Zhao et al., 2017; Liang, 2018; Guan et al., 2019; Wang et al., 2020; Xu, 2020a; Guerin, 2021; Hang and Tan, 2021; Gao and Gao, 2022; Zhu and Hou, 2022; Tian et al., 2023). For example, Chen (2015) summarized the problems associated with the carbon compliance risk management process of enterprises, including the failure to incorporate carbon management into strategic planning, the lack of a unified carbon management information platform, the lack of a carbon compliance risk pre-warning mechanism, and weak carbon asset management capabilities. Xu (2020a) established an integrated evaluation index system of carbon compliance risk coupling the political, market, and operational risk and completed the risk evaluation of carbon compliance based on the multi-attribute evaluation method. Guan et al. (2019) analyzed four types of factors affecting carbon compliance under the carbon trading mechanism, which included policy factors, market factors, industry factors, and enterprise factors, and they also examined the influence of carbon price, carbon quota, and carbon emission levels on enterprises' carbon asset management. Blanco and Rodrigues (2008) considered the impact of incentive effects caused by the carbon trading mechanism on the carbon compliance cost of enterprises and proposed a market operation scheme for driving emission control enterprises to participate in the carbon trading market. Chevallier et al. (2009) investigated the interaction between low-carbon technologies and compliance costs and proved that the successful implementation of energy-saving technologies was capable of reducing the cost and contributing to the successful possibility of enterprises' carbon compliance. Liang (2018) proposed the optimal carbon management strategy based on the production and emission characteristics of iron and steel enterprises for ensuring carbon compliance on schedule. Meanwhile, Hang and Tan (2021) examined the low-carbon transformation path of a petrochemical enterprise and pointed out the challenges encountered by the enterprise during the carbon compliance process.
As in the research findings mentioned above, it is concluded that most of the existing evaluations of carbon compliance risk are based on a macro-analysis approach, which examines the impact of various factors on an enterprise's carbon compliance, such as market fluctuation, policy development, and carbon reduction technologies. Although these qualitative assessments facilitate the increase in the success probability of carbon compliance, however, the inability to accurately identify the risk-causing effects of the abovementioned factors and quantitatively evaluate the compliance failure probability will lead to weak crisis awareness, inaccurate risk evaluation, and ineffective carbon asset management of the enterprise. This will directly affect healthy development and low-carbon transformation in the future. In other fields, the interpretive structural model (ISM) has been used to display the structural relationships of a complex system by using minimal directed topological diagrams without compromising the system's functionality (Huang et al., 2022; Li et al., 2022; Qian et al., 2022; Zhao et al., 2022). Similarly, the Bayesian network model can be used to calculate and display the occurrence probability of non-specified events by using the pictorial form, where statistical analysis techniques are employed to infer unknown variables based on certain known variable values (Sacchi and Swallow, 2021; Joffard et al., 2022; Ren et al., 2022; Wang and Xu, 2022; Yao et al., 2022). Therefore, the combination of these two methods is suitable for calculating the occurrence probability of carbon compliance failure risk in an enterprise. However, there is little research on their application in the evaluation of carbon compliance failure risk.
Therefore, the aim of this research was to fill this gap and propose a novel combination method for evaluating the carbon compliance failure risk of six prominent domestic enterprises in China, namely, steel, power, cement, petrochemical, aviation, and electrolytic aluminum, which were also the first batch of companies included in the carbon market management (Bin and Zhang, 2022; Wang et al., 2023). This research involved several tasks: (i) the determination of the carbon quota of each enterprise by using the baseline method; (ii) the hierarchical division of risk factors causing carbon compliance failure with the aid of the interpretive structure model; (iii) the establishment of a probability prediction model of carbon compliance failure risk based on the Bayesian network model; (iv) the calculation of the risk value of carbon compliance failure of six enterprises based on the risk definition; and (v) the identification of major risk factors using the sensitivity analysis method, which is beneficial in generating specific risk control measures. On the whole, the aforementioned research achievements provide excellent technical support for high-emission enterprises to avoid carbon compliance failure risk and improve their low-carbon competitiveness.
2. Methodology
2.1. Overall technical route
Figure 1 shows the overall technology roadmap. The interpretive structure model was first used to determine the hierarchical relationship of risk factors causing carbon compliance failure. Then, the occurrence probability of enterprises' carbon compliance failure risk was calculated based on the Bayesian network model. Next, the risk was quantitatively evaluated by incorporating the losses caused by compliance failure into the computational process. Finally, the critical risk-causing factors were identified with the aid of the sensitivity analysis method; correspondingly, the risk control measures were proposed, which provide the technical support for reducing the risk of compliance failure and promoting the green low-carbon transformation of target enterprises.
2.2. Risk calculation of enterprises' carbon compliance failure
As a crucial mechanism in China, the carbon compliance process regulates that the targeted enterprise needs to adjust its carbon emission behavior in order to ensure its annual carbon emission magnitude is less than or equal to the initial carbon quota allocated by the local authority. The enterprise's failure to comply with these regulations will result in a penalty or fine and reputational damage (Tian and Xu, 2020; Xu, 2020b; Yang, 2021a). The carbon compliance failure risk of the enterprise consists of of compliance risk factors, compliance failure risk incidents, and compliance failure loss. Among them, compliance risk factors are the conditions that cause or increase the chance of compliance failure or enlarge the loss magnitude, which are the potential reason for compliance failure. The compliance failure risk incidents are the episodic events that lead to carbon compliance failure, which are the important medium of default loss. The compliance failure loss is the loss due to the failure of carbon compliance tasks, such as a financial penalty, credit damage, and reduction of free carbon quota (Yang, 2021b). The risk evaluation of carbon compliance failure is to quantitatively assess the risk value of carbon compliance failure by measuring the occurrence probability of compliance failure and default loss caused by risk factors, which lays the theoretical foundation for subsequent risk control measures and compliance strategies. The risk value of compliance failure was calculated based on the following equation:
where R is the risk value of compliance failure; P is the probability of compliance failure, %; and C is the economic loss caused by the enterprise's compliance failure, million yuan.
2.3. Hierarchical division of risk factors based on the ISM method
There are many risk factors with a complex interactive relationship that lead to the failure of an enterprise's carbon compliance; it is, thus, extremely difficult to calculate the failure probability of carbon compliance. The first task of failure risk evaluation is to identify the autocorrelation and portray the hierarchical relationship among the affected factors. As a typical system structure analysis method, the ISM (interpretive structural modeling) method is capable of illustrating the intricate relationship among the system factors and formulating a clear hierarchical structure, which is suitable for completing the internal analysis of the system with many elements and unclear relationships. The basic process of the model formulation was as follows:
(i) Through the combined utilization of the data collection, literature review, and expert consultation, the relationship among the influencing factors was identified based on the association rule; the initial determination of the relationship among the affected factors was completed; the adjacency matrix containing 0 and 1 variables was formulated as follows:
where Xi and Xj are the different influencing factors; and aij is the [0,1] element of the adjacency matrix.
(ii) Based on the formulated adjacency matrix, the Boolean logic operation rule was used to generate the reachable matrix, which is written as follows:
where A is the adjacency matrix; I is the unitary matrix; and M is the reachability matrix.
(iii) The hierarchical division method was exploited to decompose the structure of the reachable matrix. As a result, the interpretive structure model was formulated, and the hierarchical relationship diagram of the influencing factors was plotted.
2.4. Probability calculation of the enterprise's carbon compliance failure risk with the aid of the Bayesian network model
The Bayesian network with N nodes is described as follows:
where DAG is the structure of the network; V is the set of node variables in the network; vi is the node variables in the network; E is the set of directed edges with links in the nodes; P is the set of conditional probability distributions, which is described as follows:
where X, Y, and Z are the disjoint subsets in the set of variables; U ={x1, x2,…, xi}; P(Y,Z)>0. It is assumed that X and Y are independent with respect to P(U) under the condition that Z is known, which is denoted as X⊥Y|Z.
The joint probability can be decomposed into equation (8) based on equation (7), indicating that the introduction of the Bayesian network reduces the complexity of joint probability calculation and facilitates the smooth realization of Bayesian inference.
where P(V) is the joint distribution of the network; parent(Xi) is the parent of node Xi; and P(V) is the edge probability of node Xi.
According to the Bayesian theorem, when the occurrence probability of an event is known, the occurrence probability of other relevant nodes is deduced. It is assumed that the event e is given, and the probability of P(V | e) is calculated as follows:
3. Formulation of the probability prediction model for enterprises' carbon compliance failure risk
3.1. Identification of risk factors causing carbon compliance failure
In order to realize carbon compliance on schedule, the emission control enterprises first utilize the historical method to estimate their possible free emission quota based on historical carbon emission amount, then calculate their clearance volume, which is equal to the gap between actual carbon emission and emission quota, and finally, they generate a rational carbon asset management pattern, including appropriate carbon reduction measures, optimal market participation strategies, and suitable China Certified Emission Reduction (CCER) projects. In terms of carbon reduction, enterprises can achieve carbon emission reduction through the advancement of the production technology and implementation of emission reduction technology or the development of CCER projects. Additionally, enterprises with insufficient quotas can purchase the carbon quota in the carbon market for realizing carbon compliance. It is summarized that three types of measures help assist enterprises to realize carbon compliance on schedule, which include appropriate carbon reduction measures, optimal market participation strategies, and suitable CCER projects. Therefore, this study classified the risk factors leading to compliance failure into four categories of factors, namely, carbon market, carbon quota, carbon emission reduction technology, and CCER recognition, respectively. Table 1 provides the specific risk-causing indicators based on the factor identification process, which lays the foundation for subsequent quantitative evaluation of carbon compliance failure risk.
3.2. Hierarchical division of risk factors causing carbon compliance failure based on the ISM method
Based on the identified results of the risk factors in Section 3.1, the adjacency matrix (as shown in Table 2) and reachability matrix (as shown in Table 3) of the influencing factors of carbon compliance failure were generated; correspondingly, Figure 2 shows the hierarchical diagram of a risk factor with the aid of the interpretation structure model.
As shown in Figure 2, the risk factors leading to the failure of enterprises' carbon compliance were divided into four levels, where the division results conformed to the actual situation. It was found that low-level factors will affect high-level factors. For example, the improper operation of personnel at the fourth level may lead to equipment loss at the third level. In addition, the decrease in the free initial quota due to market development exerts an influence on the free quota provided by the government and its allocated way. Moreover, third-level factors, such as asymmetric market information, the change in CCER offset ratio, the deviation in identified CCER's carbon reduction amounts, and the decrease in the number of CCER projects, will force the emission control enterprise to passively enter the carbon market for purchasing the carbon quota in order to complete the quota settlement and payment. This will lead to the over-high carbon quota price. Similarly, the equipment loss and the difficulty in the production technology transformation of the enterprise will impair the implementation of the carbon reduction technology, which is adverse to the realization of the expected carbon reduction.
3.3. Probability prediction model of enterprises' carbon compliance failure risk based on the Bayesian network model
Based on the aforementioned determined hierarchical relationship among risk factors, and considering the advantages of the Bayesian network with its low data requirement and intuitive and great inferential capabilities based on existing information, in this research, the Bayesian network model was adopted to establish the risk probability prediction model of enterprise's carbon compliance failure. The Netica software is a Bayesian network tool with a user-friendly interface, which offers a range of practical features, such as parameter learning, Bayesian inference, and sensitivity analysis. Compared with other software, it is capable of realizing a visual display of the posterior probability and occurrence probability calculation of specific events. Therefore, the Netica software was first chosen to establish the preliminary Bayesian network based on the aforementioned hierarchical diagram of risk factors. Second, combined with an on-site survey and literature review, the risk factors causing the carbon compliance failure of the enterprise were collected and organized, such as the government policy, carbon market fluctuation, carbon reduction situation of the enterprise, and the identification of the CCER offset magnitude. Finally, the Bayesian network-based probability prediction model of the enterprise's carbon compliance failure risk was formulated by using the self-learning process of the Netica software (as shown in Figure 3), which is capable of calculating the occurrence probability of the enterprises' carbon compliance failure risk under various risk factors.
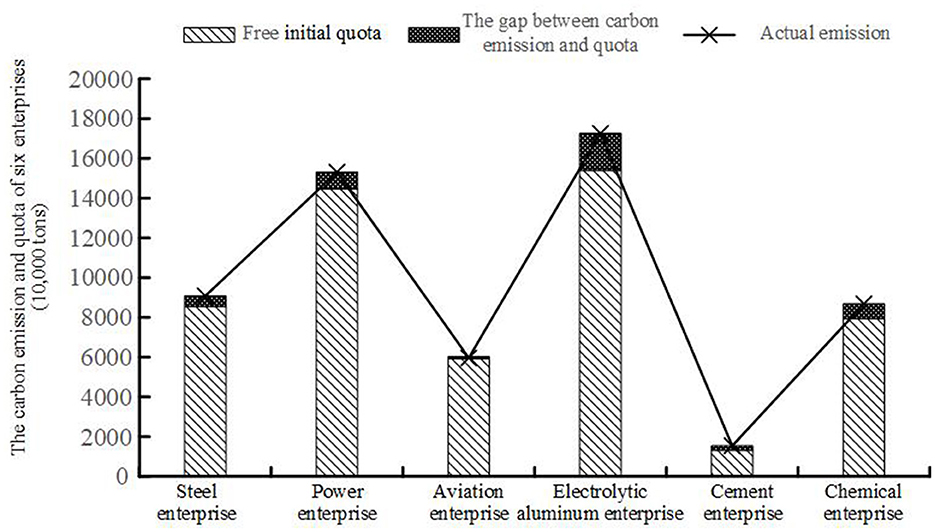
Figure 3. Occurrence probability prediction model of the enterprises' carbon compliance failure risk.
4. Case study
4.1. Determination of targeted enterprise
In order to better reflect the accuracy and rationality of the interpretive structure model and Bayesian network model in the risk evaluation of carbon compliance failure, in this study, the first batch of six wellknown domestic enterprises, including in the carbon market management, was selected as the research objects, i.e., steel, electric power, petrochemical, cement, aviation, and electrolytic aluminum. Referring to the annual reports and social responsibility reports in the last 5 years released by six enterprises, the production and operation situation, carbon emission, and carbon asset management of these six enterprises were analyzed and evaluated. Finally, the risk evaluation of carbon compliance failure for six enterprises was accomplished based on the aforementioned methods.
4.2. Risk probability prediction of enterprises' carbon compliance failure
Based on the production and operation status and actual carbon emission amounts of the six enterprises, combined with the relevant policies of free carbon quota in the region, the free carbon emission quota and the quota gap to be paid were calculated (as shown in Figure 4). As shown in Figure 4, the pressure of quota clearance and the difficulty of carbon compliance were found to be significantly different among the six enterprises. For example, although the chemical enterprise owns the largest free allowance of 153.945 million tons, its quota gap is relatively large due to the high carbon emissions during their production and operation processes, at 18.615 million tons. Conversely, the airline enterprise has the smallest free quota of 13.127 million tons; correspondingly, its quota clearance pressure is also small due to the low annual carbon emission at 15.442 million tons.
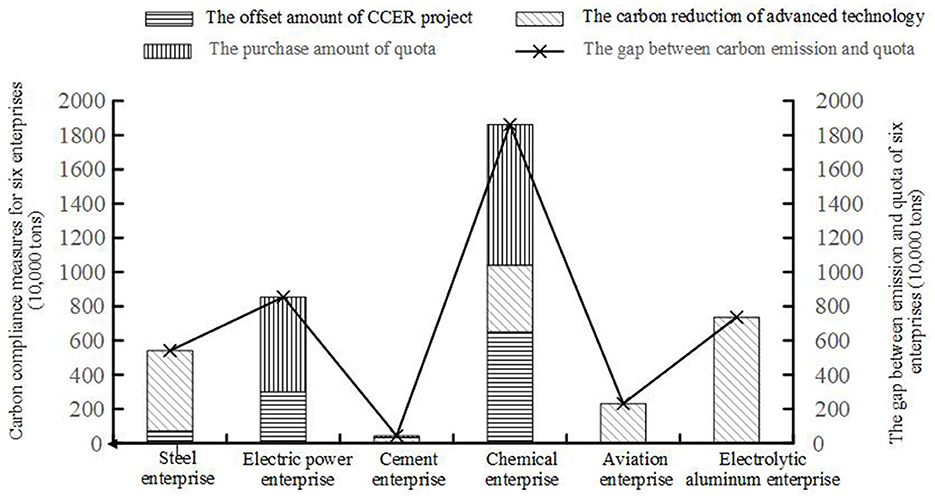
Figure 4. Free carbon quota and the difference between a quota and the actual emissions of the six enterprises.
With the background of the ‘dual carbon goals', the amount of free basic quota for enterprises will be reduced. In order to ensure the smooth realization of carbon compliance, enterprises need to design and execute reasonable and effective quota settlement measures based on their own carbon asset management and external carbon market environment. Table 4 shows the carbon asset management of these six enterprises. Among them, the chemical enterprise pays attention to carbon asset management and widely participates in various fields, since it has the greatest pressure on quota clearance. Conversely, the aviation enterprise attaches importance to its own emission reduction potential due to the small quota gap, leading to the inactive participation of the carbon market and CCER market.
Figure 5 shows the implementation of three types of measures for the six enterprises. In order to effectively reduce the carbon compliance cost, all six enterprises chose to clear the quota gap without the reserve space, resulting in inelasticity in carbon asset management and limited ability to resist the compliance failure risk. Among them, the steel and electrolytic aluminum enterprises adopted production technology innovation for realizing carbon compliance due to a large number of quotas that needed to be cleared and the obvious progress of production technology. Their carbon reduction amounts were 4.70 million tons and 7.36 million tons, respectively. Conversely, electric power and chemical enterprises filled the quota gap by purchasing the quota. This is because the local carbon market was improved with transparent trading, where the purchase amounts reached 5.54 million tons and 8.22 million tons, respectively. In addition, the steel, electric power, and chemical enterprises finished the part of quota settlement by developing CCER projects due to the limited number of CCER projects and the controversy in the identification of carbon reduction, where the offset amounts were 70, 300, and 6.5 million tons, respectively.
Based on the aforementioned carbon asset management measures of the six target enterprises, combined with the carbon quota policy, carbon market price, carbon reduction technology implementation, and CCER project situation of the local region, the occurrence probability of various risk factors corresponding to six enterprises was first predetermined. Next, they were inputted into the probability prediction model of the enterprise's carbon compliance failure risk based on the Bayesian network model. Finally, the probability of enterprises' carbon compliance failure was calculated, which is shown in Figure 6.
As depicted in Figure 6, the compliance failure probability of steel, electric power, cement, chemical, aviation, and electrolytic aluminum is 69.7, 84.1, 84.4, 62, 76, and 65.1%, respectively. As mentioned above, their clearance volume is equal to the gap between the carbon emission and quota, without the reserved space. This leads to the limited ability to resist the compliance failure risk, which is also the main reason why the compliance failure probability of the six enterprises is generally high. In addition, it is concluded that the limited carbon asset management measures may also result in carbon compliance failure. For example, the cement and aviation enterprises have a high probability of failure due to their excessive reliance on their own technological innovation, although their gap is small. Conversely, the chemical enterprise has a large clearance pressure; however, its compliance failure probability is the lowest because it actively participates in the carbon market and develops CCER projects; meanwhile, its carbon reduction technologies are relatively mature.
4.3. Risk evaluation of the enterprise's carbon compliance failure
The compliance failure risk evaluation of the enterprise is not only affected by the probability of compliance failure but also the loss caused by compliance failure. Currently, the penalty institution for an enterprise's carbon compliance failure is slightly different across the country, including two forms: economic punishment and non-economic punishment. Among them, economic punishment means penalties of 3–5 times the average carbon price based on the gap value. The non-economic punishment includes the inclusion of credit history, the cancelation of financial assistance, and the reduction of the free carbon quota in the next year. In order to realize the quantitative failure risk assessment of the six enterprises, it was assumed that the non-economic penalty can be converted into an economic penalty. The economic loss caused by the carbon compliance failure of six enterprises was calculated based on the gap value. Figure 7 demonstrates the economic loss and risk value of the six enterprises.
As shown in Figure 7, the chemical enterprise has the highest risk value of compliance failure, at 2,276.06; correspondingly, the aviation enterprise has the lowest risk value, at only 273.05. Although the chemical enterprise has the most comprehensive carbon asset management measures and the strongest ability to resist the failure risk, leading to the lowest failure probability, its economic cost caused by compliance failure is the highest (36.7107 million yuan) due to the largest gap, resulting in the highest risk value. As for the aviation enterprise, its carbon quota gap is the smallest, leading to a low economic penalty and risk value, although its failure risk probability is higher.
5. Risk control measures generation for carbon compliance failure
5.1. Identification of major risk factors based on the sensitivity analysis method
Considering that various risk factors exert different influences on the carbon compliance failure of an enterprise, the quantitative evaluation of the impact caused by risk factors on carbon compliance and the accurate identification of critical risk factors are of great importance for enterprises in order to design subsequent risk control measures and execute low-carbon rectification. The occurrence probability of risk factors was designed as three levels: 0, 50, and 100%; moreover, the difference between the maximum and minimum occurrence probability of carbon compliance failure was defined as the sensitivity degree. Based on the risk probability prediction model formulated in section 3.3, the sensitivity degree was calculated for reflecting the influence of risk factors on the occurrence probability of the enterprises' compliance failure. Table 5 provides the occurrence probability of carbon compliance failure and factor sensitivity degree of the six enterprises under the fluctuation of risk factors.
As shown in Table 5, the occurrence probability of an enterprise's compliance failure will change with the variation in the occurrence probability of the risk factors. For example, at three probability levels of CCER offset reduction, the occurrence probability of carbon compliance failure is 25.7, 23.6, and 21.6%, respectively. Similarly, accompanied by the change in the occurrence probability of initial carbon quota reduction caused by varying allocation methods, the occurrence probability of compliance failure reaches 25, 22, and 20.9%, respectively. The comparison results of the sensitivity degree among all the risk factors demonstrated that three risk factors, including the asymmetry of trading information, the free quota reduction caused by the carbon market development, and the deviation in the identification of carbon reduction of CCER projects, exert a significant influence on carbon compliance, with the sensitivity degrees of 9.4, 6.6, and 5.2%, respectively. Therefore, they have been identified as critical risk factors causing carbon compliance failure; correspondingly, effective countermeasures are needed to be generated in order to raise the success rate of the enterprises' carbon compliance.
5.2. Risk control measures for carbon compliance failure of enterprises
To reduce the failure risk of enterprises' carbon compliance, three types of control measures have been proposed corresponding to the three critical risk factors identified in section 5.1, which are as follows: (i) For the asymmetry of carbon market information, on the one hand, the enterprise should comprehensively investigate and analyze the current situation of the local carbon market; moreover, combined with the fluctuation of the carbon price, the enterprise should own enough quota reserve under the low-carbon price. On the other hand, the daily monitoring of the local carbon market should be strengthened, and the daily trading information list of the carbon market should be established in order to generate suitable trading strategies. (ii) In view of the reduction of the free quota caused by the carbon market development, first, enterprises should pay attention to the research and application of CCUS technology and promote the realization of major breakthroughs in green and low-carbon technology. Second, a clean and low-carbon energy supply system should be established, and the energy utilization efficiency of the equipment should be improved. Finally, the enterprise needs to activate the carbon sink projects and participate in the carbon market for generating additional carbon asset value. (iii) With regard to the deviation in the identification of CCER's carbon reduction, based on actively participating in the CCER market and thoroughly understanding the trading rules and the supply and demand situation of the CCER projects, the enterprise should complete the accurate measurement of the CCER emission reduction through the CCER technology management and in-depth research of the CCER methodology. In addition, the combination utilization of the CCER purchase, agreement transfer, listing transaction, and auction should obtain more concerns.
6. Conclusion
In this study, an innovative combination of interpretive structural modeling and Bayesian network algorithm was used to quantitatively evaluate the occurrence probability and risk value of certain enterprises' carbon compliance failure. The obtained results demonstrated that the compliance failure probability of steel, electric power, cement, chemical, aviation, and electrolytic aluminum is generally high, at 69.7, 84.1, 84.4, 62, 76, and 65.1%, respectively. This is because their clearance volume is equal to the gap between the carbon emission and quota, without the reserved space, leading to the limited ability to resist the compliance failure risk. Moreover, the critical risk-causing factors were identified based on the sensitivity analysis method, which is beneficial to help the enterprise design the risk control measures and raise the success rate of carbon compliance. Finally, three risk factors, including the asymmetry of trading information, the free quota reduction caused by the carbon market development, and the deviation in the identification of carbon reduction of CCER projects, were found to exert a large influence on carbon compliance, with sensitivity degrees of 9.4, 6.6, and 5.2%, respectively. Compared to the traditional qualitative risk assessment method, the proposed evaluation model is capable of realizing the quantitative evaluation of failure risk based on the comprehensive investigation and analysis of the production and operational situation and the carbon asset management of the enterprise, which provides effective technical support to enhance an enterprise's compliance awareness and improve low-carbon competitiveness. However, this model still faces some issues that require further improvement. First, as the national carbon quota clearance is still in its infancy, the information on relevant enterprises involved in carbon compliance is limited. There is a serious lack of relevant cases on enterprise compliance failure, which affects the rationality of the ISM method and Netica software. Second, the limited availability of data and information on carbon asset management and the participation situation in the carbon market of the target enterprise is not conducive to quantitative evaluation of the impact of risk factors on the carbon quota clearance. Therefore, it is crucial to accomplish a more thorough investigation of enterprises to improve the accuracy of compliance failure risk assessment. Finally, the model mainly focuses on the risk evaluation of the enterprises' compliance failure and neglects how to improve their compliance performance by designing a rational carbon asset management strategy. In the future, it needs to select the appropriate carbon reduction measures, design optimal market participation strategies based on an accurate prediction of the carbon price, and implement suitable CCER projects.
Data availability statement
The raw data supporting the conclusions of this article will be made available by the authors, without undue reservation.
Author contributions
XW: software, formal analysis, and writing—original draft. YX: supervision, conceptualization, and methodology. WL: validation. All authors contributed to the article and approved the submitted version.
Funding
This research was supported by the National Natural Science Foundation of China under Grant Number 62073134.
Conflict of interest
The authors declare that the research was conducted in the absence of any commercial or financial relationships that could be construed as a potential conflict of interest.
Publisher's note
All claims expressed in this article are solely those of the authors and do not necessarily represent those of their affiliated organizations, or those of the publisher, the editors and the reviewers. Any product that may be evaluated in this article, or claim that may be made by its manufacturer, is not guaranteed or endorsed by the publisher.
References
Bin, H., and Zhang, Y. D. (2022). Thoughts on constructing and developing China's carbon market. Environ. Protect. 50, 11–15.
Blanco, M. I., and Rodrigues, G. (2008). Can the future EU ETS support wind energy investments. Energy Policy. 36, 1509–1520. doi: 10.1016/j.enpol.2007.12.025
Chen, Y. N. (2015). Research on Compliance Risk and Prevention and Control of Enterprises Participating in China's Carbon Market [D]. Tianjin: Tianjin University of Science and Technology.
Chevallier, J., Ielpo, F., and Mercier, L. (2009). Risk aversion and institutional information disclosure on the European carbon market: a case-study of the 2006 compliance event. Energy Policy. 37, 15–28. doi: 10.1016/j.enpol.2008.07.030
Fan, Y., and Wang, X. (2014). Which sectors should be included in the ets in the context of a unified carbon market in China. Energy Environ. 25, 1–10. doi: 10.1260/0958-305X.25.3-4.613
Gao, Y. F., and Gao, R. S. (2022). Research and analysis of environmental legal compensation mechanisms related to waste incineration in the context of “double carbon” [J]. Front. Ecol. Evol. 10, 979482. doi: 10.3389/fevo.2022.979482
Guan, Z. G., Zhong, R. Y., and Zhao, Y. J. (2019). Research on the action mechanism and optimization path of china's carbon trading Mechanism [J]. Guizhou Soc. Sci. 06, 124–133.
Guerin, T. F. (2021). Tactical problems with strategic consequences: a case study of how petroleum hydrocarbon suppliers support compliance and reduce risks in the minerals sector. Resources Policy. 74, 102310. doi: 10.1016/j.resourpol.2021.102310
Hang, H. P., and Tan, W. C. (2021). Four major measures to ensure timely and full carbon compliance within 7 years [N]. China Petrochem. News. 2021, 31. doi: 10.28130/n.cnki.ncshb.2021.001788
Huang, Y. J., Li, S. Q., Li, Y. X., and Zheng, H. (2022). Comprehensive evaluation of subway operation safety resilience based on DEMATEL-ISM-ANP[J]. Chinese J. Safety Sci. 32, 171–177. doi: 10.16265/j.cnki.issn1003-3033.2022.06.2120
Joffard, N., Buatois, B., Arnal, V., Vela, E., Montgelard, C., and Schatz, B. (2022). Delimiting species in the taxonomically challenging orchid section Pseudophrys: Bayesian analyses of genetic and phenotypic data [J]. Front. Ecol. Evol. 10, 1058550. doi: 10.3389/fevo.2022.1058550
Li, M. Z., Wang, W. D., and Zhang, Z. C. (2022). Research on building construction risk factors based on ISM and MICMAC[J]. J. Safety Environ. 22, 22–28. doi: 10.13637/j.issn.1009-6094.2021.0578
Liang, Z. W. (2018). Construction of Carbon Management Accounting System under the Background of Low Carbon [D]. Wuhan: Capital University of Economics and Business.
Nie, Z. B. (2022). Promote high-quality development of carbon emission trading market under the “double carbon” goal [J]. Macroecon. Manage. 11, 37–44. doi: 10.19709/j.cnki.11-3199/f.2022.11.012
Pentz, B., and Klenk, N. (2023). Will climate change degrade the efficacy of marine resource management policies? Marine Policy. 148, 105462. doi: 10.1016/j.marpol.2022.105462
Qian, Z., He, Y., Shang, D., Zhao, H., and Zhai, J. (2022). Efficient computational approach for predicting the 3d acoustic radiation of the elastic structure in pekeris waveguides. J. Ocean Univ. China. 21, 903–916. doi: 10.1007/s11802-022-4908-3
Ren, J. Q., Wang, L. Y., Wu, J. H., Ni, S. J., and Weng, W. G. (2022). Operational risk assessment of new energy power equipment based on scenario construction and deduction[J]. J. Tsinghua Univ. 62, 1571–1578. doi: 10.16511/j.cnki.qhdxxb.2022.21.008
Sacchi, G., and Swallow, B. (2021). Toward efficient bayesian approaches to inference in hierarchical hidden markov models for inferring animal behavior [J]. Front. Ecol. Evol. 9, 623731. doi: 10.3389/fevo.2021.623731
Tian, C. X., and Xu, C. (2020). Analysis of enterprise performance cost and its influencing factors under the carbon trading mechanism—based on the case of aviation service enterprises [J]. Finance Account. Monthly. 03, 80–85. doi: 10.19641/j.cnki.42-1290/f.2020.03.012
Tian, J., Feng, C. T., Fu, G., Fan, L. Q., and Wang, W. (2023). Contribution of different types of terrestrial protected areas to carbon sequestration services in China: 1980–2020 [J]. Front. Ecol. Evol. 11, 1074410. doi: 10.3389/fevo.2023.1074410
Trotter, P. A., Mannan, I., Brophy, A., Sedzro, D., Yussuff, A., Kemausuor, F., et al. (2022). How climate policies can translate to tangible change: evidence from eleven low- and lower-middle income countries. J. Clean. Product. 346, 131014. doi: 10.1016/j.jclepro.2022.131014
Wang, K., Li, S. L., Li, S. Y., and Wang, Z. X. (2023). Reviews of China's carbon market and prospects of its optimal rolling out Plan (2023) [J]. J. Beijing Inst. Technol. 01, 1–10. doi: 10.15918/j.jbitss1009-3370.2023.9070
Wang, M., Wu, J., Kafa, N., and Klibi, W. (2020). Carbon emission-compliance green location-inventory problem with demand and carbon price uncertainties. Transport. Res. Part E: Logistics Transport. Rev. 142, 102038. doi: 10.1016/j.tre.2020.102038
Wang, P., and Xu, J. L. (2022). Research on risk assessment of information system based on bayesian networks[J]. J. Ocean Univ. China(Natural Science Edition). 52, 131–138.
Xu, C. (2020a). Research on compliance cost and its influencing factors under carbon trading mechanism[D]. Beijing: Northern University of Technology.
Xu, C. (2020b). Research on The Corporate Compliance Cost and Its Influencing Factors under Carbon Trading Mechanism [D]. Beijing: North China University of Technology.
Yang, J. J. (2021a). Study on Performance Mechanism of Carbon emission Trading in China [D]. Jiangxi: Jiangxi University of Science and Technology.
Yang, J. J. (2021b). Study on the compliance mechanism of carbon emission trading in China [D]. Jiangxi: Jiangxi University of Technology.
Yao, C. Y., Han, D. D., Chen, D. N., and Liu, Y. M. (2022). A novel continuous-time dynamic Bayesian network reliability analysis method considering common cause failure[J]. J. Instr. Instr. 43, 174–184. doi: 10.19650/j.cnki.cjsi.J2209135
Zhao, J. W., Xie, L., Yang, Y., Hu, X. Y., Ou, C. K., and Zeng, R. (2022). Research on Risk Factors of Inland Vessel Navigation Based on ISM-BN [J]. Chinese J. Safety Sci. 32, 37–44. doi: 10.16265/j.cnki.issn1003-3033.2022.08.2009
Zhao, R., Zhou, X., Jin, Q., Wang, Y., and Liu, C. (2017). Enterprises' compliance with government carbon reduction labelling policy using a system dynamics approach. J. Clean. Product. 163, 303–319. doi: 10.1016/j.jclepro.2016.04.096
Zhu, B., and Hou, R. (2022). Carbon risk and dividend policy: evidence from China. Int. Rev. Financ. Anal. 84, 102360. doi: 10.1016/j.irfa.2022.102360
Keywords: carbon compliance, risk evaluation, ISM method, Bayesian network, sensitivity analysis method
Citation: Wang X, Xu Y and Li W (2023) Research on the risk evaluation of enterprises' carbon compliance failure. Front. Ecol. Evol. 11:1152804. doi: 10.3389/fevo.2023.1152804
Received: 28 January 2023; Accepted: 27 March 2023;
Published: 25 April 2023.
Edited by:
Chuanbao Wu, Shandong University of Science and Technology, ChinaReviewed by:
Chao Dai, Sun Yat-sen University, ChinaMeirong Su, Dongguan University of Technology, China
Copyright © 2023 Wang, Xu and Li. This is an open-access article distributed under the terms of the Creative Commons Attribution License (CC BY). The use, distribution or reproduction in other forums is permitted, provided the original author(s) and the copyright owner(s) are credited and that the original publication in this journal is cited, in accordance with accepted academic practice. No use, distribution or reproduction is permitted which does not comply with these terms.
*Correspondence: Ye Xu, xuye@ncepu.edu.cn