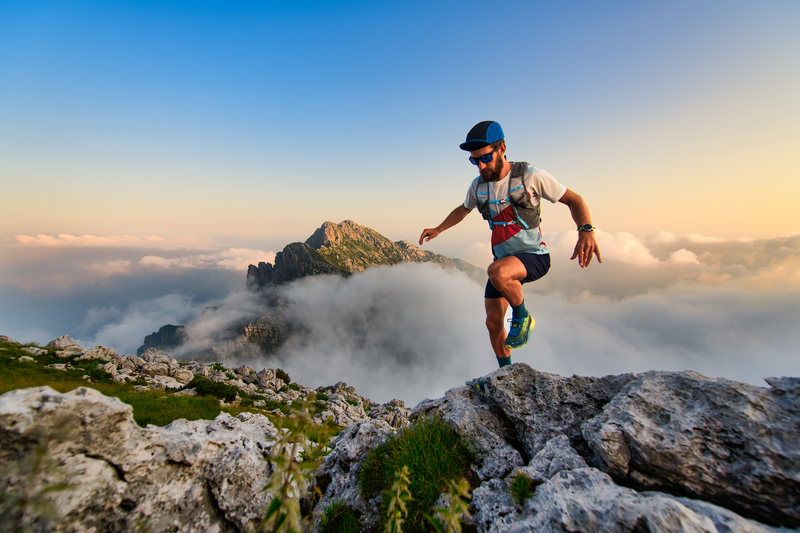
94% of researchers rate our articles as excellent or good
Learn more about the work of our research integrity team to safeguard the quality of each article we publish.
Find out more
ORIGINAL RESEARCH article
Front. Ecol. Evol. , 09 March 2023
Sec. Interdisciplinary Climate Studies
Volume 11 - 2023 | https://doi.org/10.3389/fevo.2023.1131389
Accurate characterization of land use and land cover changes (LULCC) is essential for numerical models to capture LULCC-induced effects on regional meteorology and air quality, while outdated LULC dataset largely limits model capability in reproducing land surface parameters, particularly for complex terrain. In this study, we incorporate land cover data from MODIS in 2019 into the Weather Research and Forecasting (WRF) model to simulate the impacts of LULC on meteorological parameters over the Sichuan Basin (SCB). Further, we conduct Community Multiscale Air Quality (CMAQ) simulations with WRF default LULC and MODIS 2019 to probe the effects on regional air quality. Despite consistency found between meteorological observations and WRF-CMAQ simulations, the default WRF land cover data does not accurately capture rapid urbanization over time compared with MODIS. Modeling results indicate that magnitude changes trigged by LULCC are highly varied across SCB and the impacts of LULCC are more pronounced over extended metropolitan areas due to alteration by urbanization, featured by elevating 2-m temperature up to 2°C and increased planetary boundary layer height (PBLH) up to 400 m. For air quality implications, it is found that LULCC leads to basin-wide O3 enhancements with maximum reaching 21.6 μg/m3 and 57.2 μg/m3 in the daytime and nighttime, respectively, which is mainly attributed to weakening NOx titration effects at night. This work contributes modeling insights into quantitative assessment for impacts of LULCC on regional meteorology and air quality which pinpoints optimization of the meteorology-air quality model.
As urbanization proceeds ever more quickly, the impacts of land use and land cover change (LULCC) on local meteorological conditions, urban air quality, and regional climate change have become increasingly important in the past few decades (IPCC, 2014; Oke et al., 2017). The role of LULCC in land-atmosphere interactions is represented in altering land surface parameters including surface roughness, land surface albedo, and soil properties, subsequently modulating atmospheric circulations, radiative forcing, and energy balances (Brovkin et al., 2013; Song et al., 2018; Patel et al., 2020). Although it has been found the impacts of LULCC varied considerably among different regions, the dramatic LULCC in megacities and major city clusters has become more apparent due to the human-induced urbanization and deforestation processes (Civerolo et al., 2007; Vahmani et al., 2016; Krayenhoff et al., 2018). Therefore, accurate representations of LULCC are crucial for weather predictions and regional air quality modeling.
Extensive efforts have been made for identifying the impacts of LULCC on meteorological fields and urban air pollution through satellite-derived land parameters and numerical models (Lin et al., 2016; Sharma et al., 2017). In previous studies, the Weather Research and Forecasting (WRF)-Community Multiscale Air Quality (CMAQ) modeling system has been widely used for characterizing environmental consequences of LULCC across the globe (Kumar et al., 2014; Glotfelty et al., 2021). For instance, Georgescu et al. (2013) investigated the impacts of urban expansion on Arizona’s Sun Corridor in US and found that the most dramatic urban expansion condition could result in up to 4°C in surface temperature. Gaur et al. (2021) examined the feasibility of land-cover datasets in WRF over the City of Ottawa and reported that urban parameterization with updated land cover map yields better WRF model performance. Furthermore, Glotfelty et al. (2021) indicated that outdated land cover data in WRF may cause considerable bias in reproducing land surface processes. In China, Wang K. et al. (2021) found that incorporating LULCC and four-dimensional data assimilation (FDDA) into WRF-CMAQ could reduce model bias in reproducing temperature and wind speed in Beijing-Tianjin-Hebei area. Similarly, Wang H. et al. (2021) investigated the applicability of urban canopy models in conjunction with Moderate Resolution Imaging Spectroradiometer (MODIS) 2017 land cover data in urban particulate matter pollution over Chengdu city and concluded that updating land cover yields could improve underestimation in PM2.5 concentrations. The abovementioned literature demonstrates the critical need of capturing LULCC in accurate urban modeling.
As the rapidly developing economic zone located in southwestern China, the Sichuan Basin (SCB) has experienced dramatic LULCC and worsened air pollution due to urbanization and industrialization (Qiao et al., 2019; Wu et al., 2021). Using the WRF- Community Multiscale Air Quality (CMAQ) model, Wang H. et al., (2022) concluded that LULCC over Chengdu between 2000 and 2017 weakened ventilation conditions in summer. Although several studies have applied the WRF-CMAQ modeling system to investigate the effects of LULCC on meteorology and air quality, accurate capturing meteorological fields and air pollutants formation remains challenging for WRF-CMAQ especially in complex terrain due to limitations in outdated land surface parameters, thus near real-time land use and land cover datasets from satellites (MODIS, Sentinel-2, etc) are expected to enhance the model capability in representing land-atmosphere interactions and may improve prediction skills of models. In addition, limited prior work mainly focused on megacities (Chengdu and Chongqing), while basin-wide LULCC and subsequent impacts on air quality have received much less attention (Wang H. et al., 2021; Wang Y. et al., 2022).
In this work, we leverage the WRF-CMAQ modeling system and MODIS land cover data in 2019 to investigate the influence of LULCC on regional meteorological fields and air pollutants levels over the SCB by comparing model results of the 2019LULC scenario to the baseline scenario with default WRF LULC. This paper is organized as follows. In Section 2, we introduce the source of surface meteorological observations and air quality data, MODIS land use and land cover products, and the configuration of WRF-CMAQ model. In Section 3 we analyze the differences between MODIS 2019 and the WRF default dataset, evaluate the model performance against the routine monitoring network, and probe the spatial and temporal effects of LULCC. In Section 4, we summarize major findings and implications for regulatory measures.
Hourly observations of 2-m temperature (T2), 2-m relative humidity (RH2), 10-m wind speed (WS10), and 10-m wind direction (WD10) were acquired from long-term observation data sets collected by Sichuan Provincial Meteorological Service. Ground-level air pollutants concentrations were provided by Sichuan Environmental Monitoring Center. These ground-level observations were used for WRF-CMAQ model validation.
To probe the effects of LULCC on basin-wide meteorology and regional air quality, two scenarios are considered. The default land cover and land use dataset in WRF based on Moderate-resolution Imaging Spectroradiometer (MODIS) for the year of 2001. Thus, the simulation conducted using WRF_default MODIS 2001 data is treated as baseline scenario (hereafter denote as WRF_default). In contrast, aiming for capture LULCC over the past few decades, the MODIS MCD12Q1 for 2019 is adopted and further coupled into the WRF model for replacing the default outdated dataset (hereafter denote as 2019LULC).
The WRFv4.1.1 was used to simulate meteorological fields with three nested domains (Skamarock et al., 2019), as shown in Figure 1. The innermost domain covers the metropolitan areas of the SCB (Supplementary Figure S1). The grid resolution for three two-way nested domains was 27, 9, and 3 km, respectively. The simulation period spans from 28 June to 31 August, 2019, with simulation results for June treated as spin-up. National Centers for Environmental Prediction (NCEP) Final (FNL) 1.0° × 1.0° reanalysis data1 which is available every 6 h, were used to provide initial and boundary conditions for WRF. The configurations of physical parameterization scheme are similar to our previous studies due to well-documented behavior, including Lin scheme for microphysics (Chen and Sun, 2002), RRTMG and Goddard for longwave and shortwave radiation (Baek, 2017), YSU scheme for planetary boundary layer (Hong et al., 2006), revised MM5 similarity for surface layer (Jiménez et al., 2012), Grell 3-D scheme for cumulus parameterization (Grell and Dévényi, 2002), Noah land surface model scheme for surface exchange of heat, moisture and momentum (Chen and Dudhia, 2001). The vertical layer is configured for 30 eta levels with 14 layers within the PBL aiming for better characterizing mesoscale processes within the PBL. Two high-resolution WRF model experiments were conducted in this work, including WRF_default and 2019LULC. For the WRF default experiment, we use the original land cover data in WRFv4.1.1. In the 2019LULC experiment, the land cover of WRF was updated by Moderate Resolution Imaging Spectroradiometer (MODIS) MCD12Q1 product for 2019, as discussed in Section 2.2.
CMAQ v5.3.2 was used to simulate ozone and its precursors (Appel et al., 2021). The Carbon-Bond chemical reaction mechanism (CB06) and the Aerosol 06 (AERO6) aerosol mechanism were applied in the CMAQ model (Pye et al., 2017; Luecken et al., 2019). The boundary condition used for the largest domain of CMAQ is clean air, while the BCs for the nested domains are extracted from the CMAQ Chemical Transport Model (CCTM) concentration files of the larger domain. Anthropogenic emissions were based on Multi-resolution Emission Inventory for China (MEIC) for 2019, with a grid resolution of 0.25° × 0.25° (Zheng et al., 2018, 2021). The Model of Emissions of Gases and Aerosols from Nature (MEGAN, version 2.1) was adopted to estimate the biogenic emissions based on emission factors from global database, leaf area index, and plant function types from MODIS products (Guenther et al., 2012; Wu et al., 2020). It should be noted that physical parameterizations, anthropogenic emission inventory, and chemical mechanisms are the same, thus the comparison between modeling results of WRF_default and 2019LULC reflects effects on meteorology and air quality due to LULCC alone.
To identify the contributions of atmospheric dynamics and chemical reactions to O3 formation, the integrated process rate (IPR) module embedded in CMAQ model is implemented for the diagnosis of hourly O3 variations and underlying factors (Czader et al., 2013; Hogrefe et al., 2018). These processes include dry deposition (DDEP), gas phase chemical production (CHEM), cloud process (CLDS), horizontal advection (HADV), horizontal diffusion (HDIF), vertical advection (ZADV), and turbulent mixing (VDIF).
Figure 2 presents the map of LULC based on land use datasets in WRFv4.1.1 model and MODIS MCD12Q1 products for 2019 over the SCB. As expected, croplands occupy most areas of SCB and some forest types are visible in northern and southwestern SCB. In addition, deciduous broadleaf trees are depicted in Leshan and northern Mianyang based on MODIS 2019, suggesting the potential influence of biogenic VOC emissions on air quality due to relatively high BVOC emission potential (Zhang et al., 2022). It is worth noting that the expansion of urban areas due to rapid urbanization are well captured in MODIS 2019 as compared with outdated WRF default profile, particularly for the enlargement of urban core areas (Chengdu and Chongqing cities) and build-up of extended metropolitan regions.
Figure 2. Land use classification in WRF model over Sichuan Basin (A) WRF default data (B) MODIS MCD12Q1 for 2019.
The simulated meteorological parameters and air pollutants levels were paired with observations in time and space for model evaluation. The statistical metrics, including mean bias (MB), mean fractional bias (MFB), mean fractional error (MFE), index of agreement (IOA), and root-mean-square error (RMSE) (definitions shown in Supplementary Text S1), are calculated.
The evaluation for WRF model performance is detailed in our previous work (Wang Y. et al., 2022). Briefly, WRF well reproduced the day-to-day variations of T2 with IOA higher than 0.8 and NMB less than 0.2 for all cities within the SCB. For RH2, the MB and NMB are ranged 1.4–1.5 and −0.21-0.63 among cities, respectively, indicating strong capability of WRF in reproducing RH2 variability across the SCB. However, the wind speed was slightly overestimated by the WRF model in the SCB, which might be associated with uncertainties in atmospheric dynamics and physical PBL parameterizations. In general, the meteorological fields simulated by WRF well reflected the features and variability of atmospheric dynamics and the model bias was comparable to previous studies (Zhang et al., 2019; Yang et al., 2020).
Figure 3 compares the observed and simulated hourly O3 concentrations for cities within the SCB in August 2019 in the WRF_default scenario. Evidently, the diurnal variations and day-to-day variability of O3 levels are both well captured by the CMAQ model, with all IOA values higher than 0.7. Specifically, the CMAQ model yields excellent performance in simulating Chengdu, Mianyang, Deyang, and Meishan cities with MFB values less than 0.3 (Emery et al., 2017). Despite the good agreement between observations and CMAQ simulations, it can be seen that CMAQ underestimates O3 peaks in Chengdu, Deyang, and Meishan, which might be related to bias in meteorological parameters and uncertainty in MEIC emission inventory. Overall, the WRF-CMAQ modeling system successfully captured and reproduced the meteorological variability and variations air pollutants concentrations over the study period.
Figure 3. Time series of observed and simulated ambient O3 for cities within the SCB in August 2019.
The LULCC could affect land surface parameters including albedo, stomatal resistance, and roughness length, thus subsequently further influencing ground-level energy budget and mass exchange. To investigate the impacts of land use and land cover changes on regional meteorology, the modeled 2-m temperature and planetary boundary layer height (PBLH) in 2019LULC are compared with results in WRF_default, as shown in Figures 4–6. The most significant effects induced by LULCC were mainly found over extended metropolitan regions across the SCB which correspond to substantial alteration in land use types induced by rapid urbanization, and the largest changes are found in Chengdu and Chongqing cities due to strong anthropogenic activities occurred in these areas. In rural areas, the shift of land use resulted in slight changes in T2. Evidently, T2 in the afternoon and nighttime T2 increased by 1.0–2.0°C across extended metropolitan areas (areas experience land use transition from croplands to urban and built up), and increases in T2 were much more prominent in the afternoon. This phenomenon could be associated with enhanced absorption capacity of radiation from urban surface, which results in much heat storage in the daytime. At night, the relatively high thermal inertia of urban canopy could weaken the cooling process, thus leading to higher nighttime T2 compared with the WRF_default scenario. In contrast, slight decreases in T2 were found in the morning. It is also worth noting that T2 considerably decreased over the intersection of Chongqing, Suining, and Guangan cities.
Figure 4. Differences in modeled monthly-averaged T2 (°C) between 2019LULC and WRF_default scenarios at 02:00, 08:00, 14:00, 20:00 over the SCB in August 2019.
Figure 5. Differences in modeled monthly-averaged PBLH (m) between 2019LULC and WRF_default scenarios at 02:00, 08:00, 14:00, 20:00 over the SCB in August 2019.
Figure 6. Differences in simulated monthly-averaged O3 between 2019LULC and WRF_default scenarios at 02:00, 08:00, 14:00, 20:00 over the SCB in August 2019.
Figure 5 displays the spatial changes in PBLH across the SCB between 2019LULC and WRF_default. While the influential magnitudes vary strongly across the study domain, it can be found that LULCC caused increases of PBLH up to 400 m in the afternoon over extended metropolitan regions, particularly for Chengdu and Chongqing cities. However, WRF simulations depicted distinctly different patterns at night, with substantial PBLH increases over Mianyang (Figures 5A,C). It is worth noting that noticeable effects depicted by model simulations exhibit excellent agreement with grids featured by urbanization, indicating that changes in meteorological parameters are largely determined by urbanization processes. These spatial patterns and magnitude changes in T2 and PBLH are comparable to previous modeling work by Wang H. et al., (2022), who reported that intense thermal inertia and high heat capacity in urban canopy led to nocturnal T2 increased by 3–4°C in Chengdu, the megacity situated at western SCB.
The formation of ambient O3 is determined by the abundance of precursor emissions (NOx and VOCs) and meteorological conditions which influence the photochemical reactions in the atmosphere. Prior studies have indicated that O3 variations are largely attributed to changes in T2 and PBLH (Hu et al., 2021). Figures 6, 7 display the spatial distribution of hourly O3 and average changes in MDA8 O3 between 2019LULC and WRF_default scenarios over the study domain. Further, hourly contributions from each physical and chemical process to ambient O3 in Chengdu are shown in Figure 8. It can be clearly seen that LULCC led to moderate O3 enhancements both in the daytime and nighttime in August 2019. Spatially, the increases of MDA8 O3 ranged from 4.6–24.7 μg/m3 and largest changes mainly correspond to grid cells with substantial LULCC (extended metropolitan). Further analysis based on IPR (Figure 8) suggests that such increases are primarily attributed to weak dry deposition which consume less ambient O3 due to land use alteration, while daytime gas-phase chemistry changed only slightly in response to the variations of T2 and PBLH. Specifically, lead area index (LAI) and vegetation fraction are key factors which governs the quantification of deposition velocities in the CMAQ model. As the vegetation fraction was changed by updating land use and land cover, depositions velocities of O3 in CMAQ simulations might be overestimated in the WRF_default scenario. In contrast, the increment magnitude of O3 at night was much higher than daytime and peak O3 increases (up to 57.2 μg/m3) were found in metropolitan Chengdu and Chongqing at night. In southern SCB, moderate nighttime O3 enhancements around 12.0 μg/m3 were mainly depicted over Luzhou, Neijiang, and Zigong cities. In addition to obvious changes in densely populated city clusters, northern SCB also exhibited considerable changes in MDA8 O3, despite the relatively low anthropogenic emissions and slow urbanization process over this region. Interestingly, nighttime O3 changes do not correspond to PBLH because of insignificant variations in PBLH (Figure 5), which suggests that reduced NOx act as the dominant factor in explaining this phenomenon. As seen in Figure 9, nighttime NOx levels in 2019LULC were much less than that in WRF_default scenario with maximum reductions reaching 26.8 μg/m3 across densely populated areas. This phenomenon is primarily linked to increase of PBLH over these areas, which results in intense NOx dilution within the PBL then weaken photochemical reactions because of insufficient precursors. At night, NOx titration is the governing process in determining O3 chemistry and lowered NOx concentrations generally lead to less O3 consumption, subsequently causing high O3 levels to endure. A similar pattern in nighttime O3 changes has also been observed in Beijing-Tianjin-Hebei city cluster (Tao et al., 2018).
Figure 7. Map of simulated monthly-averaged MDA8 O3 in (A) 2019LULC and (B) WRF_default scenarios and (C) differences of MDA8 O3 between 2019LULC and WRF_default over the SCB in August 2019.
Figure 8. Diurnal pattern of hourly physical and chemical processes contributions to ambient O3 concentrations in Chengdu city in August 2019.
Figure 9. Differences in simulated NO2 between 2019LULC and WRF_default scenarios at night over the SCB in August 2019.
In this study, we adopt land use and land cover data derived from the MODIS satellite into the WRF-CMAQ modeling system to probe the impacts of LULCC on meteorological fields and regional air quality over the SCB in 2019. The LULCC is well represented in MODIS 2019, particularly for the rapid urbanization and development of extended metropolitan areas.
The land alteration results in changes in meteorological parameters with magnitudes that vary strongly across regions. Modeling results show that LULCC leads to obvious increases in T2 (up to 2°C) in the afternoon and the warming endured during the nighttime with the most prominent changes found over urban areas and extended metropolitan, which could be attributed to higher radiation absorption capacity and high thermal inertia of urban surface. For PBLH, LULCC generally elevates the PBLH up to 400 m in the afternoon, while insignificant variations are depicted at night, with moderate ascending PBLH over Mianyang city. These changes in meteorological parameters subsequently affect O3 air quality. We found basin-wide substantial O3 enhancements in both daytime and nighttime, with the increment of O3 up to 23.8 μg/m3 in daytime and 54.8 μg/m3 at night. The O3 enhancement during daytime is mainly affected by weakened dry deposition and the nighttime increase of O3 could be explained by reduced NOx titration effects.
Previous studies have noted that chemical transport models (CTMs) generally underpredict summertime O3 levels, particularly for O3 peaks (Yang et al., 2020; Wu et al., 2022; Zhang et al., 2022). Here, we show that accounting for LULCC in CTMs could dramatically improve O3 underestimation by the CMAQ model over the SCB, demonstrating the urgent need for characterizing LULCC in land surface parameters for better model capability. Overall, the findings of this work reveal the importance of LULCC in altering regional meteorology and air quality, shedding insights on urban planning and climate adaptation strategy.
The original contributions presented in the study are included in the article/Supplementary material, further inquiries can be directed to the corresponding author.
YL and XY designed this work and wrote the original draft. YL, HW, and XW conducted the formal analysis and edited the manuscript. LM contributed to the data collection and analysis. XY and XZ supervised this work and provide project administration. All authors contributed to the manuscript and approved the submission.
This study was funded by the National Natural Science Foundation of China (No. 42175174 and No. 41905025), Open Research Fund Program of Plateau Atmosphere and Environment Key Laboratory of Sichuan Province (No. PAEKL-2020-C6), Chengdu University of Information Technology Scientific and Technological Innovation Capacity Improvement Plan (No. KYQN202239 and No. KYQN202203), and the project “Assessment of the impact of optimized urban underlying surface and land surface parameters on air quality simulations in Chengdu-Chongqing city cluster” funded by Chengdu University of Information Technology Training Program of Innovation and Entrepreneurship for Undergraduates (No. 202310621001).
The authors acknowledge the MEIC team from Tsinghua University for providing the Multi-resolution Emission Inventory for China (MEIC) and Chengdu Plain Urban Meteorology and Environment Observation and Research Station of Sichuan Province for support in collecting air quality data.
The authors declare that the research was conducted in the absence of any commercial or financial relationships that could be construed as a potential conflict of interest.
All claims expressed in this article are solely those of the authors and do not necessarily represent those of their affiliated organizations, or those of the publisher, the editors and the reviewers. Any product that may be evaluated in this article, or claim that may be made by its manufacturer, is not guaranteed or endorsed by the publisher.
The Supplementary material for this article can be found online at: https://www.frontiersin.org/articles/10.3389/fevo.2023.1131389/full#supplementary-material
Appel, K. W., Bash, J. O., Fahey, K. M., Foley, K. M., Gilliam, R. C., Hogrefe, C., et al. (2021). The community multiscale air quality (CMAQ) model versions 5.3 and 5.3.1: system updates and evaluation. Geosci. Model Dev. 14, 2867–2897. doi: 10.5194/gmd-14-2867-2021
Baek, S. (2017). A revised radiation package of G-packed McICA and two-stream approximation: performance evaluation in a global weather forecasting model. J. Adv. Model. Earth Syst. 9, 1628–1640. doi: 10.1002/2017MS000994
Brovkin, V., Boysen, L., Arora, V. K., Boisier, J. P., Cadule, P., Chini, L., et al. (2013). Effect of anthropogenic land-use and land-cover changes on climate and land carbon storage in CMIP5 projections for the twenty-first century. J. Clim. 26, 6859–6881. doi: 10.1175/JCLI-D-12-00623.1
Chen, F., and Dudhia, J. (2001). Coupling an advanced land surface–hydrology model with the Penn State–NCAR MM5 modeling system. Part I: model implementation and sensitivity. Mon. Weather Rev. 129, 569–585. doi: 10.1175/1520-0493(2001)129<0569:CAALSH>2.0.CO;2
Chen, S.-H., and Sun, W.-Y. (2002). A One-dimensional Time Dependent Cloud Model. J. Meteorol. Soc. Japan. Ser. 80, 99–118. doi: 10.2151/jmsj.80.99
Civerolo, K., Hogrefe, C., Lynn, B., Rosenthal, J., Ku, J.-Y., Solecki, W., et al. (2007). Estimating the effects of increased urbanization on surface meteorology and ozone concentrations in the new York City metropolitan region. Atmos. Environ. 41, 1803–1818. doi: 10.1016/j.atmosenv.2006.10.076
Czader, B. H., Li, X., and Rappenglueck, B. (2013). CMAQ modeling and analysis of radicals, radical precursors, and chemical transformations: CMAQ MODELING. J. Geophys. Res. Atmos. 118, 11,376–11,387. doi: 10.1002/jgrd.50807
Emery, C., Liu, Z., Russell, A. G., Odman, M. T., Yarwood, G., and Kumar, N. (2017). Recommendations on statistics and benchmarks to assess photochemical model performance. J. Air Waste Manage. Assoc. 67, 582–598. doi: 10.1080/10962247.2016.1265027
Gaur, A., Lacasse, M., Armstrong, M., Lu, H., Shu, C., Fields, A., et al. (2021). Effects of using different urban parametrization schemes and land-cover datasets on the accuracy of WRF model over the City of Ottawa. Urban Clim. 35:100737. doi: 10.1016/j.uclim.2020.100737
Georgescu, M., Moustaoui, M., Mahalov, A., and Dudhia, J. (2013). Summer-time climate impacts of projected megapolitan expansion in Arizona. Nat. Clim. Change 3, 37–41. doi: 10.1038/nclimate1656
Glotfelty, T., Ramírez-Mejía, D., Bowden, J., Ghilardi, A., and West, J. J. (2021). Limitations of WRF land surface models for simulating land use and land cover change in sub-Saharan Africa and development of an improved model (CLM-AF v. 1.0). Geosci. Model Dev. 14, 3215–3249. doi: 10.5194/gmd-14-3215-2021
Grell, G. A., and Dévényi, D. (2002). A generalized approach to parameterizing convection combining ensemble and data assimilation techniques. Geophys. Res. Lett. 29, 38-1–38-4. doi: 10.1029/2002GL015311
Guenther, A. B., Jiang, X., Heald, C. L., Sakulyanontvittaya, T., Duhl, T., Emmons, L. K., et al. (2012). The model of emissions of gases and aerosols from nature version 2.1 (MEGAN2.1): an extended and updated framework for modeling biogenic emissions. Geosci. Model Dev. 5, 1471–1492. doi: 10.5194/gmd-5-1471-2012
Hogrefe, C., Liu, P., Pouliot, G., Mathur, R., Roselle, S., Flemming, J., et al. (2018). Impacts of different characterizations of large-scale background on simulated regional-scale ozone over the continental United States. Atmos. Chem. Phys. 18, 3839–3864. doi: 10.5194/acp-18-3839-2018
Hong, S.-Y., Noh, Y., and Dudhia, J. (2006). A new vertical diffusion package with an explicit treatment of entrainment processes. Mon. Weather Rev. 134, 2318–2341. doi: 10.1175/MWR3199.1
Hu, C., Kang, P., Jaffe, D. A., Li, C., Zhang, X., Wu, K., et al. (2021). Understanding the impact of meteorology on ozone in 334 cities of China. Atmos. Environ. 248:118221. doi: 10.1016/j.atmosenv.2021.118221
IPCC. (2014). Observations: Cryosphere, climate change 2013–the physical science basis: Working group I contribution to the fifth assessment report of the intergovernmental panel on climate change. Cambridge: Cambridge University Press, 317–382.
Jiménez, P. A., Dudhia, J., González-Rouco, J. F., Navarro, J., Montávez, J. P., and García-Bustamante, E. (2012). A revised scheme for the WRF surface layer formulation. Mon. Weather Rev. 140, 898–918. doi: 10.1175/MWR-D-11-00056.1
Krayenhoff, E. S., Moustaoui, M., Broadbent, A. M., Gupta, V., and Georgescu, M. (2018). Diurnal interaction between urban expansion, climate change and adaptation in US cities. Nat. Clim. Chang. 8, 1097–1103. doi: 10.1038/s41558-018-0320-9
Kumar, A., Chen, F., Barlage, M., Ek, M. B., and Niyogi, D. (2014). Assessing impacts of integrating MODIS vegetation data in the weather research and forecasting (WRF) model coupled to two different canopy-resistance approaches. J. Appl. Meteorol. Climatol. 53, 1362–1380. doi: 10.1175/JAMC-D-13-0247.1
Lin, C.-Y., Su, C.-J., Kusaka, H., Akimoto, Y., Sheng, Y.-F., Huang, J.-C., et al. (2016). Impact of an improved WRF urban canopy model on diurnal air temperature simulation over northern Taiwan. Atmos. Chem. Phys. 16, 1809–1822. doi: 10.5194/acp-16-1809-2016
Luecken, D. J., Yarwood, G., and Hutzell, W. T. (2019). Multipollutant modeling of ozone, reactive nitrogen and HAPs across the continental US with CMAQ-CB6. Atmos. Environ. 201, 62–72. doi: 10.1016/j.atmosenv.2018.11.060
Oke, T. R., Mills, G., Christen, A., and Voogt, J. A. (2017). Urban Climates. Cambridge: Cambridge University Press
Patel, P., Karmakar, S., Ghosh, S., and Niyogi, D. (2020). Improved simulation of very heavy rainfall events by incorporating WUDAPT urban land use/land cover in WRF. Urban Clim. 32:100616. doi: 10.1016/j.uclim.2020.100616
Pye, H. O. T., Murphy, B. N., Xu, L., Ng, N. L., Carlton, A. G., Guo, H., et al. (2017). On the implications of aerosol liquid water and phase separation for organic aerosol mass. Atmos. Chem. Phys. 17, 343–369. doi: 10.5194/acp-17-343-2017
Qiao, X., Guo, H., Wang, P., Tang, Y., Ying, Q., Zhao, X., et al. (2019). Fine particulate matter and ozone pollution in the 18 cities of the Sichuan Basin in southwestern China: model performance and characteristics. Aerosol Air Qual. Res. 19, 2308–2319. doi: 10.4209/aaqr.2019.05.0235
Sharma, A., Fernando, H. J. S., Hamlet, A. F., Hellmann, J. J., Barlage, M., and Chen, F. (2017). Urban meteorological modeling using WRF: a sensitivity study. Int. J. Climatol. 37, 1885–1900. doi: 10.1002/joc.4819
Skamarock, W. C., Klemp, J. B., Dudhia, J., Gill, D. O., Liu, Z., Berner, J., et al. (2019). A description of the advanced research WRF version 4. NCAR Tech., Note NCAR/TN-556+STR, 145
Song, X.-P., Hansen, M. C., Stehman, S. V., Potapov, P. V., Tyukavina, A., Vermote, E. F., et al. (2018). Global land change from 1982 to 2016. Nature 560, 639–643. doi: 10.1038/s41586-018-0411-9
Tao, H., Xing, J., Zhou, H., Chang, X., Li, G., Chen, L., et al. (2018). Impacts of land use and land cover change on regional meteorology and air quality over the Beijing-Tianjin-Hebei region, China. Atmos. Environ. 189, 9–21. doi: 10.1016/j.atmosenv.2018.06.033
Vahmani, P., Sun, F., Hall, A., and Ban-Weiss, G. (2016). Investigating the climate impacts of urbanization and the potential for cool roofs to counter future climate change in Southern California. Environ. Res. Lett. 11:124027. doi: 10.1088/1748-9326/11/12/124027
Wang, H., Liu, Z., Wu, K., Qiu, J., Zhang, Y., Ye, B., et al. (2022). Impact of urbanization on meteorology and air quality in Chengdu, a Basin City of southwestern China. Front. Ecol. Evol. 10:845801. doi: 10.3389/fevo.2022.845801
Wang, H., Liu, Z., Zhang, Y., Yu, Z., and Chen, C. (2021). Impact of different urban canopy models on air quality simulation in Chengdu, southwestern China. Atmos. Environ. 267:118775. doi: 10.1016/j.atmosenv.2021.118775
Wang, K., Tong, Y., Gao, J., Gao, C., Wu, K., Yue, T., et al. (2021). Impacts of LULC, FDDA, topo-wind and UCM schemes on WRF-CMAQ over the Beijing-Tianjin-Hebei region, China. Atmos. Pollut. Res. 12, 292–304. doi: 10.1016/j.apr.2020.11.011
Wang, Y., Yang, X., Wu, K., Mei, H., Lu, Y., Smedt, I. D., et al. (2022). Long-term trends of ozone and precursors from 2013 to 2020 in a megacity (Chengdu), China: evidence of changing emissions and chemistry. Atmos. Res. 278:106309. doi: 10.1016/j.atmosres.2022.106309
Wu, K., Wang, Y., Qiao, Y., Liu, Y., Wang, S., Yang, X., et al. (2022). Drivers of 2013–2020 ozone trends in the Sichuan Basin, China: impacts of meteorology and precursor emission changes. Environ. Pollut. 300:118914. doi: 10.1016/j.envpol.2022.118914
Wu, K., Yang, X., Chen, D., Gu, S., Lu, Y., Jiang, Q., et al. (2020). Estimation of biogenic VOC emissions and their corresponding impact on ozone and secondary organic aerosol formation in China. Atmos. Res. 231:104656. doi: 10.1016/j.atmosres.2019.104656
Wu, K., Zhu, S., Liu, Y., Wang, H., Yang, X., Liu, L., et al. (2021). Modeling ammonia and its uptake by secondary organic aerosol over China. J. Geophys. Res. Atmos. 126. doi: 10.1029/2020JD034109
Yang, X., Wu, K., Wang, H., Liu, Y., Gu, S., Lu, Y., et al. (2020). Summertime ozone pollution in Sichuan Basin, China: meteorological conditions, sources and process analysis. Atmos. Environ. 226:117392. doi: 10.1016/j.atmosenv.2020.117392
Zhang, L., Guo, X., Zhao, T., Gong, S., Xu, X., Li, Y., et al. (2019). A modelling study of the terrain effects on haze pollution in the Sichuan Basin. Atmos. Environ. 196, 77–85. doi: 10.1016/j.atmosenv.2018.10.007
Zhang, S., Lyu, Y., and Yang, X. (2022). Modeling biogenic volatile organic compounds emissions and subsequent impacts on ozone air quality in the Sichuan Basin, southwestern China. Front. Ecol. Evol. 10:11. doi: 10.3389/fevo.2022.924944
Zheng, B., Tong, D., Li, M., Liu, F., Hong, C., Geng, G., et al. (2018). Trends in China’s anthropogenic emissions since 2010 as the consequence of clean air actions. Atmos. Chem. Phys. 18, 14095–14111. doi: 10.5194/acp-18-14095-2018
Keywords: land use, land cover, air quality, urban meteorology, Sichuan Basin
Citation: Lu Y, Yang X, Wang H, Jiang M, Wen X, Zhang X and Meng L (2023) Exploring the effects of land use and land cover changes on meteorology and air quality over Sichuan Basin, southwestern China. Front. Ecol. Evol. 11:1131389. doi: 10.3389/fevo.2023.1131389
Received: 25 December 2022; Accepted: 10 February 2023;
Published: 09 March 2023.
Edited by:
Yuqiang Zhang, Shandong University, ChinaReviewed by:
Ying Wang, Nanjing University of Information Science and Technology, ChinaCopyright © 2023 Lu, Yang, Wang, Jiang, Wen, Zhang and Meng. This is an open-access article distributed under the terms of the Creative Commons Attribution License (CC BY). The use, distribution or reproduction in other forums is permitted, provided the original author(s) and the copyright owner(s) are credited and that the original publication in this journal is cited, in accordance with accepted academic practice. No use, distribution or reproduction is permitted which does not comply with these terms.
*Correspondence: Xianyu Yang, eHlhbmdAY3VpdC5lZHUuY24=
Disclaimer: All claims expressed in this article are solely those of the authors and do not necessarily represent those of their affiliated organizations, or those of the publisher, the editors and the reviewers. Any product that may be evaluated in this article or claim that may be made by its manufacturer is not guaranteed or endorsed by the publisher.
Research integrity at Frontiers
Learn more about the work of our research integrity team to safeguard the quality of each article we publish.