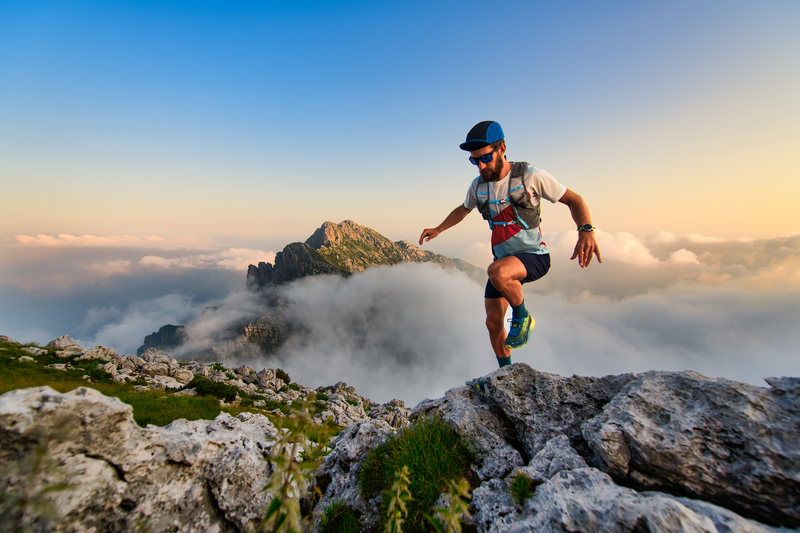
94% of researchers rate our articles as excellent or good
Learn more about the work of our research integrity team to safeguard the quality of each article we publish.
Find out more
ORIGINAL RESEARCH article
Front. Ecol. Evol. , 20 April 2023
Sec. Environmental Informatics and Remote Sensing
Volume 11 - 2023 | https://doi.org/10.3389/fevo.2023.1131175
Xinjiang grasslands play a crucial role in regulating the regional carbon cycle and maintaining ecosystem stability, and grassland net primary productivity (NPP) is highly vulnerable to drought. Drought events are frequent in Xinjiang due to the impact of global warming. However, there is a lack of more systematic research results on how Xinjiang grassland NPP responds to drought and how its heterogeneity is characterized. In this study, the CASA (Carnegie Ames Stanford Application) model was used to simulate the 1982–2020 grassland NPP in Xinjiang, and the standardized Precipitation Evapotranspiration Index (SPEI) was calculated using meteorological station data to characterize drought. The spatial and temporal variability of NPP and drought in Xinjiang grasslands from 1982 to 2020 were analyzed by the Sen trend method and the Mann-Kendall test, and the response characteristics of NPP to drought in Xinjiang grasslands were investigated by the correlation analysis method. The results showed that (1) the overall trend of NPP in Xinjiang grassland was increasing, and its value was growing season > summer > spring > autumn. Mild drought occurred most frequently in the growing season and autumn, and moderate drought occurred most frequently in spring. (2) A total of 64.63% of grassland NPP had a mainly concurrent effect on drought, and these grasslands were primarily located in the northern region of Xinjiang. The concurrent effect of drought on NPP was strongest in plain grassland and weakest in alpine subalpine grassland. (3) The lagged effect is mainly in the southern grasslands, the NPP of alpine subalpine meadows, meadows, and alpine subalpine grasslands showed mainly a 1-month time lag effect to drought, and desert grassland NPP showed mainly a 3-month time lag effect to drought. This research can contribute to a reliable theoretical basis for regional sustainable development.
Since the 20th century, global climate change has become one of humanity’s most serious challenges (Liu Y. et al., 2016). The IPCC Sixth Assessment Report noted that the occurrence of global drought events has been frequent over the past 50 years, and this trend shows signs of further expansion in the future (IPCC, 2021). Drought is a meteorological hazard caused by several factors, including increased temperatures and decreased moisture (Carrão et al., 2017). The occurrence of drought may cause a decline in vegetation productivity and accelerate land desertification, posing a significant threat to natural ecosystems and seriously affecting the quality of ecosystems, mainly arid and semiarid ecosystems where species diversity and productivity are severely limited by precipitation (Easterling et al., 2000; Tong et al., 2019), thus posing a threat to the sustainable development of human society (Sevanto, 2018). Therefore, the study of drought trends and evolution in time and space is essential for regional water and carbon cycles.
Current methods of monitoring drought are mainly based on a composite assessment of regional wet and dry conditions using a drought index. Spinoni et al. (2014) revealed the characteristics of early global dryness changes during 1951–1970, 1971–1990, and 1991–2010 by the standardized precipitation index (SPI); Guo et al. (2018) explored the evolution of drought in Central Asia from 1966 to 2015 by the SPEI. In general, since precipitation is the main source of water for the soil, it is indispensable for the evaluation of drought. However, water balance is also related to evapotranspiration (Graham et al., 2019). Therefore, in analyzing climate effects on grassland vegetation, the drought index containing precipitation and evapotranspiration has an obvious advantage relative to precipitation (Zhou et al., 2019). The commonly used drought indicators are the SPI (Mckee et al., 1993), Palmer drought severity index (PDSI; Palmer, 1965), and SPEI (Vicente-Serrano et al., 2010). The SPEI fully considers the contribution of surface evapotranspiration to drought, making its results more accurate and reliable than the SPI and PDSI (Vicente-Serrano et al., 2013; Liu Z. et al., 2016). Studies have concluded that the SPEI has good applicability in the northwest arid zone (Yao et al., 2018; Zhang H. et al., 2021).
Among terrestrial ecosystems, grasslands account for approximately one-third of the global land area (Xue et al., 2021), and grassland vegetation is indispensable in water conservation, greenhouse gas reduction, and climate stabilization (Piao et al., 2011; Huang et al., 2016). NPP can reflect the health of vegetation and ecosystems, is critical in the terrestrial carbon cycle (Potter et al., 2012; Chen et al., 2015), and is susceptible to climate change (Liu X. et al., 2021). Monitoring the change characteristics in grassland NPP is essential to evaluate ecosystem quality(Ma et al., 2022; Shen et al., 2022). As the most representative and widely used NPP assessment model, the CASA model has a relatively simple algorithm and can comprehensively assess the characteristics of regional NPP spatiotemporal dynamics (Potter et al., 1993; Pei et al., 2018; Wang et al., 2019). As the global climate warms, drought events are frequent (Pei et al., 2013), especially in arid and semiarid regions (Ummenhofer and Meehl, 2017). Frequent droughts can decrease vegetation productivity, with severe implications for vegetation growth, ecosystem function, and stability (Vicca et al., 2016), which in turn has consequences for the global carbon cycle (Huang et al., 2016). Therefore, exploring the effects of drought on grassland vegetation is indispensable to improving the stability and resistance of the ecosystem. In recent years, the relationship between drought and NPP has been studied by many scholars. Zhao and Running (2010) found that widespread periodic droughts in the Southern Hemisphere as well as precipitation deficits and extreme summer temperatures in Eastern and Western Europe reduced vegetation productivity and thus the carbon sequestration capacity of vegetation in terrestrial ecosystems. Sun et al. (2016) revealed that drought was the leading cause of vegetation NPP changes in Northeast China by analyzing the factors influencing vegetation NPP changes. Previous studies have focused on global and large regional scales with short time series, and there are fewer studies on the effects of small regional and long time series drought events on NPP. In addition, grassland is a vegetation type highly susceptible to drought, and its response to drought is complex. However, studies on the response characteristics of grasslands, especially different types of grasslands, to drought events are still insufficient.
Xinjiang is an indispensable part of the arid zone of Central Asia, and it has significant temperate continental arid climate characteristics. The region is far from the ocean, precipitation is scarce, evaporation is high, and its ecological environment is exceptionally sensitive to global climate change responses (Yao et al., 2019; Guan et al., 2022). Xinjiang’s grasslands are vast and rich in resources. They are the basis for the development of Xinjiang’s livestock industry, as well as an essential part in maintaining Xinjiang’s natural ecological environment and playing an important ecological function. Due to global warming, drought events frequently occur in Xinjiang, and according to statistics, 17 severe droughts and 9 significant droughts occurred in Xinjiang from 1961 to 2000 (Shi et al., 2007; Li and Sun, 2017). Frequent drought events have had an important impact on the NPP of Xinjiang grasslands. However, there is a shortage of more systematic research on how Xinjiang grassland NPP responds to drought and how its heterogeneity is characterized. We centered on the effects of drought on grassland NPP in Xinjiang from 1982 to 2020, aiming to address the following aspects: (1) study the dynamic trends of the spatiotemporal patterns of the NPP and SPEI in Xinjiang grasslands; (2) explore the concurrent and lagged effect of drought on NPP in Xinjiang grasslands; (3) analyze of the concurrent and lagged effect of drought on NPP in different grassland types. The analysis and study of the characteristics of grassland NPP affected by drought are of great practical significance and can provide decision support for formulating regional sustainable development strategies.
Xinjiang (73°40′–96°18′E, 34°25′–48°10′N) is located in Northwest China. The Altai Mountains are in northern Xinjiang, and the Kunlun Mountains are in the south. The Tianshan Mountains cut across central Xinjiang, dividing Xinjiang into north and south. The Tarim Basin is in the south and the Junger Basin is in the north (Figure 1A). Xinjiang has an annual average temperature between 9 and 12°C (Figure 1C), low annual precipitation, an abnormal shortage of water resources (Jiapaer et al., 2015; Yao et al., 2019), and perennial drought, which is typical of temperate continental arid and semiarid climates. The spatial distribution of precipitation in Xinjiang is not uniform. The average annual precipitation in the north is approximately 100–200 mm, while the average annual precipitation in the south is approximately 16–85 mm (Figure 1D). Xinjiang has abundant light and heat and high evapotranspiration. The average annual evaporation in the north is approximately 1,500–2,300 mm, and the average annual evaporation in the south is approximately 2,100–3,400 mm. The north is wetter than the south. Xinjiang is rich in grassland resources and has a wide distribution of natural grasslands, with an available grassland area of approximately 480,000 km2. Xinjiang’s ecological environment is very fragile, with low land cover, few plant species, and a simple population type structure (Guan et al., 2021; Figure 1B).
Figure 1. Study area. (A) Elevation. (B) Vegetation types. (C) Annual average temperature in Xinjiang from 1982 to 2020. (D) Average annual precipitation in Xinjiang from 1982 to 2020.
The MODIS NDVI for 2000–2020 selected for this study was obtained from NASA’s MOD13A31 with a temporal resolution of 1 month and a spatial resolution of 0.01°, and the 1982–2015 GIMMS NDVI dataset from GIMMS NDVI 3 g2 was released by the NASA Global Monitoring and Simulation Research Group with a temporal resolution of 15 days and a spatial resolution of 1/12°. The spatial resolution of GIMMS NDVI was resampled from 1/12° to 0.01° to match the MODIS NDVI.
The meteorological data for this study were obtained primarily from the China Meteorological Data Network,3 including the daily mean temperature and daily precipitation data for 92 meteorological stations in Xinjiang from 1982 to 2020, and spatially interpolated using ANUSPLIN 4.2 to synthesize the monthly average temperature and monthly precipitation data with a spatial resolution of 0.01°, which were matched with other ecological data. The solar radiation data for 1982–2020 were obtained using the Famine Early Warning Systems Network Land Data Assimilation System (FLDAS) dataset,4 which has a spatial resolution of 0.01° and a temporal resolution of 1 month.
The land cover data were obtained from the Global Land Cover Data 2000 product (GLC2000) of the Spatial Applications Institute (SAI) of the Joint Research Centre (JRC) of the European Union with a spatial resolution of 0.01°.5 This study reclassified land use types in Xinjiang to obtain a map of grassland types in Xinjiang, with five main grassland types: alpine subalpine meadows, plain grasslands, desert grasslands, meadows, and alpine subalpine grasslands.
DEM data obtained from the Resource and Environment Data Cloud platform6 were used in this study to calculate the elevation of the study area with a spatial resolution of 90 m.
The SPEI was selected as the drought monitoring index. We used the Thornthwaite method to calculate the SPEI value, and the required meteorological indicators were the average temperature and site latitude (Thornthwaite, 1948). The detailed procedure for calculating SPEI is available in the literature (Vicente-Serrano et al., 2010). The classification is shown in Table 1 (Ye et al., 2020):
The 1-month time scale SPEI (SPEI-1) was selected to reflect short-term surface moisture anomalies, the 3-month time scale SPEI (SPEI-3) was selected to reflect seasonal variation in drought, the 7-month time scale SPEI (SPEI-7) was selected to represent growing season drought, and the 12-month scale (SPEI-12) was selected to reflect interannual trends in drought.
We used the CASA model to estimate the gridded NPP at a 0.01°0.01° resolution.
where is the photosynthetically active radiation absorbed by image element in month and is the actual light energy utilization of image element in month . and indicate the effect of different temperatures (low and high) on light energy conversion, indicates the effect of different moisture conditions on light energy conversion, and is the maximum light energy utilization under ideal conditions, which refers to the research of (Zhu et al., 2006).
Sen’s trend analysis was used to calculate the trend of the data series (Sen, 1968).
where 1≤≤n.
The Mann-Kendall (M-K) significance test (Mann, 1945) was combined with Sen’s trend analysis for significance testing of serial trends (Gocic and Trajkovic, 2013; Qi et al., 2019).
When the M-K test is used to detect sequence mutations, the statistic needs to be reconstructed:
More details of the calculation process can be found in the literature(Mann, 1945; Kendall, 1990).
Pearson correlation analysis was used to study the relationship between grassland NPP and drought.
where is the correlation coefficient; is the logarithm of the two variables; and denote the values of NPP and the SPEI, respectively; and and denote the mean values of NPP and the SPEI, respectively.
The time-lagged effect was similarly characterized by calculating Pearson correlation coefficients. At each lag-time interval (0 ≤ ≤ 3), the correlation coefficients () between NPP and the 0–3 months SPEI-1 (SPEI0, SPEI1, SPEI2, SPEI3) were first calculated, and the maximum correlation coefficient of was taken as the optimal correlation, with month as the optimal time lag (Zhao et al., 2020).
where is the Pearson correlation index for a lag of months, and ranges from 0 to 3; NPP indicates the month-by-month NPP time series (1982–2020); is the 0- months SPEI-1; is the maximum value of ; and the lag month with the highest correlation is used as an optimal lag month.
We calculated the mean NPP of grasslands in Xinjiang in the range of 141.59–182.03 gC·m−2 during 1982–2022 using the CASA model. The 2018–2020 measured biomass data in this study were provided by the General Grassland Station of Xinjiang Uygur Autonomous Region. 1mx1m sample squares were deployed throughout Xinjiang in June each year from 2018 to 2020, and a total of 643 sample points were obtained, including 221 samples in 2018, 220 samples in 2019, and 192 samples in 2020, the distribution of which is shown in Figure 1. The NPP simulated by the CASA model was compared with the 2018–2020 measured data to verify the accuracy of the results (Figure 2). The NPP simulation results were significantly correlated with the measured data (R2 = 0.701, p < 0.01). Therefore, the NPP simulated in this paper had high accuracy and was applicable to estimate the NPP of Xinjiang grassland.
In terms of interannual variation, the total NPP of the Xinjiang grassland showed an increasing fluctuation during 1982–2020 with an extremely significant change (p < 0.01; Supplementary Figure S1), and the mean NPP of the multiyear grassland was 158.74 gC·m−2.
March–May, June–August, September–November, and April–October have been designated as the spring, summer, autumn, and growing seasons respectively, based on the vegetation growth habits and the phenological characteristics of the region. From 1982 to 2020, the NPP of grassland at the four seasonal scales in Xinjiang increased (Figures 3A–D), and the NPP values of grassland were significantly different. The mean NPP of the whole region in the growing season reached 148.89 gC·m−2 (Figure 3D), and the increasing trend was extremely significant (p < 0.01). The relationship between the NPP values of grassland at three seasonal scales was as follows: summer > spring > autumn, and the mean NPP of grassland was 32.45 gC·m−2 in spring (p < 0.01; Figure 3A). The mean value of NPP in grassland was larger in summer at 95.28 gC·m−2 (Figure 3B), and the increasing trend was significant (p < 0.05). The NPP in grassland was lowest in autumn with an average of 26.95 gC·m−2 (p < 0.05; Figure 3C).
Figure 3. The NPP during the (A) spring, (B) summer, (C) autumn, and (D) growing season for the period from 1982 to 2020.
Grassland NPP exhibited spatial distribution characteristics of south-high-north-low and west-high-east-low types (Supplementary Figure S2). From the whole area, the mean NPP range of Xinjiang from 1982 to 2020 was 0–813.06 gC·m−2, with high values occurring along the Tianshan Mountains, near the Altay Mountains, Kunlun Mountains, and parts of northern Xinjiang, and lower NPP values of grasslands near the Tarim Basin, the Junggar Basin, and southern Xinjiang.
Sen’s trend was overlaid with the M-K test results with a confidence of 0.05, and the results were divided into five categories (Table 2; Guan et al., 2021). Most areas of Xinjiang grassland NPP showed an increasing trend (Figures 4A–D; Supplementary Figures S3A–D). The area with a growing trend of NPP in spring was the largest among the four seasonal scales, accounting for 81.37%. 46.50% has a significant increasing trend, mainly in the Altay Mountains, Kunlun Mountains, and Tianshan Mountains. 18.51% showed decreasing in NPP in spring, mainly around the Turpan Basin. The area of significantly decreasing areas was tiny, with a proportion of 3.41%. NPP increased in summer in 68.54% of the region, mainly distributed around Tianshan, Altun Mountain, and the southern Tianshan Mountains. The areas with a significant increase were mainly in the southern part of the Tianshan Mountains and around the Altun Mountains (31.86%). Areas with a decrease are mainly around the Altay and Turpan basins (31.37%), and those with a significant decrease account for 7.37%. The grassland NPP of 71.75% of the area showed an increase in autumn, and 28.13% showed a decrease. The decreasing areas were mainly near the edge of the Kunlun Mountains and the Turpan Basin. Compared with spring and summer, the proportion of grassland NPP showing a significant increase in autumn was reduced, accounting for 28.28%, and was mainly distributed around the Altay Mountains and Tianshan Mountains. The area showing a significant decrease was also relatively small, accounting for 3.46%. Grassland NPP in 79.40% of the areas showed an increase during the growing season, while 20.58% showed a decreasing trend. 42.20% showed a significant increase, mainly near the Altun Mountains and along the northern Tianshan Mountains. The areas with a decreasing trend were mainly in the Altay Mountains and Turpan Basin, and there were fewer areas with a significant decreasing trend (3.46%), mainly near the Altay Mountains.
Figure 4. Spatial variation pattern of grassland NPP in (A) spring, (B) summer, (C) autumn, and (D) the growing season in Xinjiang from 1982 to 2020.
The trend of the SPEI changed at all time scales and showed a significant decrease (Figure 5A). Nevertheless, the magnitude of the decline was small, indicating a slight increase in drought. The M-K test for the SPEI in Xinjiang at different time scales showed that significant abrupt changes occurred in the SPEI-1 and SPEI-7 in approximately 2002, the UF and UB curves of the SPEI-3 intersected in around 2005, while the curve for SPEI-12 intersected around 2001, indicating that significant abrupt changes occurred in all these periods. Before the abrupt changes, most of the SPEI trends in Xinjiang increased on different time scales, and the wetness in Xinjiang increased during this period. After the abrupt change, the SPEI trends in Xinjiang were opposite at different time scales, and Xinjiang gradually became dry during this period.
Figure 5. (A) Time series and Mann-Kendall tests of SPEI at different scales from 1982 to 2020. (B) Frequency of different drought grades at different scales from 1982 to 2020.
The frequencies of drought events at different time scales in Xinjiang were counted (Figure 5B). The frequency of occurrence of nondrought events was the highest for all scales, with a value greater than 75%. The frequencies of droughts at other scales were mild drought > moderate drought > severe drought > extreme drought. No extreme drought events occurred. The frequency of mild drought was approximately 10% on the SPEI-1 scale and approximately 14% on other time scales. Moderate droughts ranged from 4 to 6% on all time scales, while severe droughts were below 1%. The frequency of severe drought was approximately 0.8 and 0.6% at the SPEI-1 and SPEI-7 scales, and lower at the SPEI-3 and SPEI-12 scales, 0.01 and 0.21%, respectively. The occurrence of drought events under different time scales in Xinjiang was dominated by mild drought.
The M-K test was conducted on the Xinjiang SPEI at the four seasonal scales (Figure 6A). The SPEI at the four seasonal scales showed a significant downward trend. The spring SPEI had a significant abrupt change approximately 2001 and the summer approximately 2005. The autumn and growing seasons had significant abrupt changes in 1992 and 1999, respectively. The mean value of SPEI before and after the change was 0.57 in spring and 0.47 in summer. The mean values of SPEI before and after the change in autumn and growing season were 0.30 and 0.63, respectively.
Figure 6. (A) Time series of the SPEI and Mann-Kendall test. (B) The frequency of different drought grades for the spring, summer, autumn, and growing season from 1982 to 2020.
The frequency of drought events at the four seasonal scales (spring, summer, autumn, and growing season) was statistically analyzed (Figure 6B). The frequency of nondrought events at the four seasonal scales from 1982 to 2020 was the highest, all greater than 70%, followed by the frequency of mild drought. There was no extreme drought or severe drought event during the study period. Mild droughts occurred at the same frequency during the growing season and autumn (17.95%), the frequency in spring was 12.82%, and the frequency in summer was the lowest (7.69%). The frequency of moderate drought was highest in spring (10.26%), followed by autumn (7.69%), the growing season (5.13%), and summer (2.56%).
Figure 7 shows the spatial variation trend of the SPEI at the four seasonal scales in Xinjiang from 1982 to 2020. In spring, the SPEI tended to decrease in most regions, with a significant decrease concentrated in southern Xinjiang (p < 0.05), especially near the Tarim Basin, with an SPEI tendency rate of (−0.15 ~ −0.1)/10a. In summer, significantly rising areas were concentrated around the southern Tianshan Mountains (p < 0.05) with SPEI tendency rates of (0.2 ~ 0.4)/10a, and significantly declining parts of the SPEI in summer were mainly in eastern Xinjiang (p < 0.05), especially near the Turpan Basin with SPEI tendency rates of (−0.8 ~ −0.4)/10a. The SPEI in autumn mainly showed an upward trend along the Tianshan Mountains in northern Xinjiang, and near the southern Tianshan Mountains, with an insignificant upward trend (p > 0.05). Most other areas showed a decreasing trend, with significant decreases occurring mainly in the Tarim Basin, Turpan Basin, and parts of southern Xinjiang (p < 0.05), with an SPEI tendency rate of (−0.6 ~ −0.2)/10a. The growing season SPEI showed a significant decreasing trend mostly in southern Xinjiang (p < 0.05), concentrated near the Tarim Basin and Turpan Basin, with an SPEI tendency rate of (−0.8 to −0.2)/10a. The regions with a decreasing SPEI trend were mainly in southern and eastern Xinjiang. Moreover, the northwestern part mainly showed an increasing trend. The whole region is in drought, but the SPEI tendency rate does not change much, and the degree of drought is weak.
Figure 7. Spatial variation in SPEI series propensity rates in (A) spring, (B) summer, (C) autumn, and (D) the growing season in Xinjiang from 1982 to 2020.
The relationship between NPP and SPEI for the four seasons of Xinjiang grassland from 1982 to 2020 is shown in Figure 8. They are negatively correlated in the spring (r = −0.16). 73.51% of the regions were negatively correlated in spring, of which 16.53% were highly significant negative regions (p < 0.01), mainly around the Altay Mountains, Tianshan Mountains, Kunlun Mountains and Altun Mountains. Both summer and autumn NPP and SPEI were generally positively correlated, with a correlation coefficient of 0.22 in summer and a smaller correlation of 0.01 in autumn, indicating that spring and summer grassland NPP are more susceptible to drought than autumn grassland NPP. In summer, the proportion of regions with a positive correlation was the largest (74.47%), among which 31.81% had an extremely significant positive correlation (p < 0.01). Extremely significant positive correlations were mainly in northern Xinjiang, near the Altay Mountains, along the Tianshan Mountains, and in the southern Tianshan Mountains. The percentage of areas with positive and negative correlations in autumn was similar, at 54.83 and 49.59%, respectively, and both positive and negative correlations were insignificant (p > 0.05). Positive correlations exist along the Tianshan Mountains, while negative correlations exist near the Altay Mountains, Altun Mountains, and Kunlun Mountains. The growing season NPP was positively correlated with the SPEI overall (r = 0.12), accounting for 63.52%. 20.95% were extremely positively correlated (p < 0.01). Its spatial correlation was similar to that in summer. An extremely significant negative correlation (p < 0.01) was observed around Altun Mountain at 3.79%. The correlation was insignificant in most areas of southern Xinjiang. The correlation between the monthly NPP and SPEI of the grassland growing seasons in Xinjiang from 1982 to 2020 was analyzed to further explore the concurrent effect of drought on NPP in Xinjiang grasslands, and the concurrent effect dominated 64.63% of grasslands with NPP on drought. These grasslands were mainly concentrated along the Tianshan Mountains, the edges of the Altay Mountains and most of northern Xinjiang (Figure 8).
Figure 8. Spatial pattern distribution of NPP and SPEI correlations in (A) spring, (B) summer, (C) autumn, and (D) the growing season in Xinjiang from 1982 to 2020.
NPP responds differently to drought in different types of grasslands (Figure 9). NPP and SPEI for all five grassland types showed a negative correlation in spring, the opposite in summer, and a weak correlation in autumn. In spring, both alpine subalpine meadow and desert grasslands showed an extremely significant negative correlation (p < 0.01). In summer, the NPP in plain meadows had the strongest correlation with drought (p < 0.01), while alpine subalpine meadows and meadows were significantly positively correlated (p < 0.05). Desert meadows correlation and alpine subalpine meadows were negatively correlated with the SPEI, while the four other grassland types were positively correlated in autumn. The strongest positive correlation between plain meadow NPP and drought (p < 0.01), significant positive correlation between meadow NPP and SPEI (p < 0.05), negative correlation in desert meadows, and weak correlation in other meadow types (p > 0.05) during the growing season, similar to summer.
Figure 9. Correlation between SPEI and NPP for different grassland types in Xinjiang from 1982 to 2020. (* indicates a significance level of 0.05, whereas ** indicates a significance level of 0.01).
From the correlation between monthly NPP and SPEI of different grassland types in Xinjiang during the growing season from 1982 to 2020, the concurrent effect of drought on NPP was strongest in plain grasslands, followed by desert grasslands and alpine subalpine meadows, and the concurrent effect of drought was weakest in alpine subalpine grasslands (Figure 10).
Figure 10. Correlation of the time lag effect of NPP on SPEI for different grassland types in Xinjiang from 1982 to 2020. (* indicates a significance level of 0.05, whereas ** indicates a significance level of 0.01).
The sensitivity of grassland NPP to drought was revealed by analyzing the correlation between the monthly lags of NPP and SPEI in the growing season (Figure 11). The time-lag effect of each image element was counted, in which 64.63% of the grasslands had no time-lag effect, mainly in the northern border, and 35.37% of the grasslands had a time-lag effect, mainly in the southern part of Xinjiang. The time-lag effect showed more apparent differences between the north and south. Xinjiang grasslands mainly showed 1-month and 3-month time-lag effects of drought, accounting for 11.80 and 13.74% of the region, respectively, and 9.82% of the regional grassland NPP showed 2-month time-lag effects. The time lag areas were mainly in the Altay Mountains, Kunlun Mountains, Altun Mountains, Junggar Basin, Tarim Basin, and parts of southern Xinjiang.
Figure 11. (A) Spatial distributions of the rmax-lag between the SPEI and NPP during 1982–2020. (B) Spatial distribution of the corresponding lagged months where the rmax-lag occurred.
The correlation analysis between growing season NPP and 1-month scale lag SPEI of different grassland types in Xinjiang was used to obtain the lag months of drought on the NPP of varying grassland types in Xinjiang (Figure 10). The lag effects of different grassland types on drought were as follows: plain grassland NPP showed mainly no lag to drought, and alpine subalpine meadow, meadow, and alpine subalpine grassland NPP all showed mainly 1-month time-lag effects to drought. Desert grassland NPP showed mainly 3-month time-lag effects.
In this study, the CASA model simulated an average NPP of 158.74 gC·m−2 for 1982–2020 in the grasslands of Xinjiang. Zhang R. et al. (2021) and Yang et al. (2014) used the CASA model to simulate the grassland NPP of Xinjiang for 2001–2014 and 2009, respectively, and obtained an average NPP of 113.5 gC·m−2 and 124.61 gC·m−2, respectively. In contrast to previous studies, this study has a long time series and can better reflect the long-term changes in NPP in Xinjiang grasslands. Due to the study area, period, and model parameters, the estimation of NPP is uncertain to some extent, but the difference between these estimates is reasonable (Zhang et al., 2018). The total NPP of Xinjiang grasslands has increased in the past 40 years, indicating a growing role for regional carbon sinks. This result may be attributed to the increase in CO2 concentration; warming can stimulate enzyme activity and accelerate photosynthesis in vegetation (Saxe et al., 2001; Weng and Luo, 2008), and the fertilization effect of CO2 may promote vegetation growth (Sun and Mu, 2018). In addition, the increase in atmospheric CO2 concentration can reduce leaf stomatal conductance, improve water use efficiency, and alleviate the water deficit of plants (Knapp and Hamerlynck, 1993; Morgan et al., 2004). It has been shown that extreme precipitation events have increased significantly in Xinjiang (Guan et al., 2022), which may also lead to a trend of growing grassland NPP in Xinjiang in all seasons. In addition, the growth of grassland NPP is also related to the positive influence of human activities, such as the implementation of ecological restoration projects.
This study found that the trend of drought is increasing, but the increasing degree was weak, which was a kind of mild drought. The relationship between grassland vegetation and single-scale drought has been the focus of previous studies, with less research focusing on multiple scales (Liberato et al., 2017). In this study, we considered the drought conditions reflected by different time scales of the SPEI. The results show that there are differences in the degree of drought reflected by different time scales of the SPEI, but in general the direction of their changes is consistent, and the selection of different time scales can weaken the differences between single-month water balances, thus highlighting the characteristics of seasonal scales and annual scales. Precipitation and evapotranspiration are typical factors influencing drought occurrence (Zhang et al., 2019). Most of Xinjiang’s water vapor transport is from the midlatitude western belt and Indian monsoon, with external water vapor transportation accounting for over 90% of Xinjiang’s total precipitation (Yao et al., 2020). Evapotranspiration in arid and semiarid regions is generally much more than precipitation (Ran et al., 2015), making the difference between regional precipitation and potential evaporation increasingly significant (Miao et al., 2020). Additionally, increased evapotranspiration due to global warming may exacerbate regional droughts (Dai, 2013). The frequent occurrence of extreme heat events exacerbates evapotranspiration, offsetting the increase in precipitation (Guan et al., 2022). Therefore, against the background of extreme heat and frequent precipitation events and given the limited precipitation and high regional evaporation in Xinjiang, further deterioration of drought conditions is expected in the future (Miao et al., 2020; Guan et al., 2022). Human activities can also significantly impact drought. Greenhouse gas emissions, expansion of urban agglomerations, and changes in land use patterns can all exacerbate drought events as well (Gu et al., 2019; Lin et al., 2020).
Global terrestrial ecosystems are mainly influenced by solar radiation, temperature, and water resource conditions (Nemani et al., 2003; Chen et al., 2013). However, in arid and semiarid regions, the availability of water resources due to sufficient heat and light is recognized as a major factor affecting vegetation productivity. Previous research studies have shown that precipitation affects the growth of vegetation in arid areas (Zhang et al., 2016). This study provides a more comprehensive study of vegetation change in arid areas by using the SPEI index, which includes precipitation, and shows that changes in grassland NPP are to some extent related to drought. Drought in these areas is usually due to insufficient precipitation or high evapotranspiration (Wang et al., 2018). Grassland vegetation growing in arid and semiarid regions is more drought tolerant (Zhang et al., 2014). When drought occurs, plant stomata are closed to limit transpiration (Lefi et al., 2004), resulting in increased vegetation NPP. As the northern border of Xinjiang is wet and the southern border is dry, grassland NPP also showed a high north and low south pattern. In this study, the grassland NPP and SPEI showed positive correlations in northern Xinjiang but negative correlation patterns relations in most of southern Xinjiang, indicating that grassland NPP was more influenced by precipitation.
Seasonal scale analysis indicated that grassland NPP and SPEI showed the most areas of significant positive correlation in summer, followed by the growing season, and the least was in spring. Most of the precipitation is concentrated in summer, which provides good conditions for vegetation growth and an advantage for the increase in grassland NPP. The grassland NPP and SPEI showed a negative correlation in spring because the grass was in the pregrowth stage, and the temperature gradually increased in spring, which promoted NPP accumulation. In addition, the melting of snow in spring in most areas of the northern border provided a specific water demand. Furthermore, the rising temperature increased the supply of melting snow, and ensured the return of grassland despite the lack of precipitation and the increase in drought. Recent warming has also contributed to the rise in NPP, and the effect of drought on vegetation productivity can be offset by the fertilization effect of rising CO2 concentrations (Morgan et al., 2011).
Previous studies have noted that different vegetation types have different response characteristics to drought (Liu et al., 2023) and the characteristics regarding the response of different grassland types to drought remain to be explored. This study shows that the response of NPP to drought was also correlated with grassland type. The main grassland types in Xinjiang are desert grasslands and plain grasslands. The results showed that the NPP of plain grasslands had a strong correlation with the SPEI, which may be due to most plain grasslands having large vegetation leaf areas and strong transpiration, thus requiring a greater water supply, so the productivity of plain grasslands generally increases with increasing rainfall (Shi et al., 2014) and responds rapidly to drought. The weaker sensitivity of desert grassland NPP to drought may be due to the extensive drought-resistant vegetation in desert grasslands enhancing water storage capacity through physiological activities, which gives it some ability to resist and adapt to drought (Zhang et al., 2014). This reduces the effect of drought on NPP in desert grasslands to some extent. It is noticeable that the drought effect on vegetation will show significant spatial and temporal heterogeneity (Liu Y. et al., 2021) and should be considered more comprehensively in future research combined with geographical characteristics.
This study showed a time lag of 1–3 months in the response of grassland NPP to drought, but the response was mainly dominated by the current month. Liu et al. (2023) studied the response of vegetation to drought and its time lag in arid areas, showing that the time lag between woodland and shrubs and drought was mainly 1 month. This may be because grassland is shallow-rooted vegetation, and its growth condition is mainly governed by soil surface moisture. In contrast, woodlands and shrubs have deeper root systems and usually rely on deep soil moisture supply, so changes in soil surface moisture do not have a significant impact on this type of vegetation. Different grassland types have different physiological characteristics and different response characteristics to drought. Han et al. (2023) explored the differences in the probability of drought stress in Xinjiang from different grassland types, but the hysteresis characteristics of different grassland types to drought need to be further explored. Soil moisture is a primary limiting factor for vegetation growth in arid and semiarid regions. The physiological mechanisms inherent in vegetation allow the vegetation in these areas to tolerate and adapt to water deficits for longer than vegetation in humid regions (Anderegg et al., 2015; Wen et al., 2019). Therefore, the NPP in arid and semiarid grasslands has a lagged effect on the drought response. For different grassland types, most alpine subalpine meadows and alpine subalpine grasslands in Xinjiang are distributed around mountain ranges, and glacial meltwater can be the main source of soil moisture for grasslands. Their productivity response to drought generally has a time-lag effect. Desert grasslands are the largest grassland type in Xinjiang, with a wide distribution, and their area distribution is larger in the south than in the north. Desert grassland vegetation generally shows a certain degree of drought adaptability, prolonging the drought response time of desert grassland. In arid and semiarid regions, meadows primarily grow in low-lying areas where surface runoff collects, and the water table is high. Water is provided mainly by rainfall, so meadows generally have a time-lag effect on drought response. Time scales should be fully taken into account when discussing drought and its effects, and lagged effects may be more important in the impact of drought on vegetation over time (Li et al., 2018).
Some uncertainties remain in the estimation of CASA models. Most studies have used the results of Potter et al. (1993) and Zhu et al. (2007) for the maximum light energy utilization () of vegetation. Nevertheless, the above parameters may have spatial differences in different regions, which lead to the estimated grassland vegetation. However, spatial parameter differences may occur in the different areas, leading to errors in the NPP calculations of estimated grassland vegetation. Therefore, it is necessary to optimize the values of the simulation parameters with geographical characteristics to reduce the errors in the model simulation results in future research. Some of the meteorological stations are unevenly distributed, resulting in some differences in the spatial interpolation results. More meteorological data should be integrated for analysis in the future. Meanwhile, to explore the response characteristics of different grassland types NPP to drought, this study used the GLC2000 dataset to classify grassland types, which has a high spatial resolution and detailed classification and is widely used in grassland studies (Wang et al., 2016; Liu et al., 2019; Han et al., 2023). However, as land use/cover is dynamic and its changes may have some impact on grassland NPP and drought (Shen et al., 2020; Ma et al., 2022), future studies can integrate multiple land cover datasets to reflect grassland distribution more accurately. In addition, vegetation phenological characteristics were less considered in this study, which may lead to the neglect of the differences among the vegetation of each grassland type in the selection and analysis of growing seasons (Wu et al., 2021; Shen et al., 2022). Therefore, the next step can be a more comprehensive and specific study based on the present study.
We analyzed the spatiotemporal characteristics of grassland NPP and drought in Xinjiang from 1982 to 2020 based on the CASA model and SPEI, respectively. In addition, we further analyzed the effects of drought on NPP in Xinjiang grasslands and explored in detail the characteristics of NPP response to drought in different grassland types in Xinjiang. This study provides a comprehensive explanation of the effects of drought on grasslands in Xinjiang and highlights the importance of the effects of drought on different grassland types, which can provide scientific references for vegetation conservation, grassland restoration, and sustainable development of terrestrial ecosystems in the region. The results reveal the following conclusions.
(1) The results of estimating the NPP of grassland in Xinjiang using the CASA model were better. The NPP of grassland in Xinjiang showed an overall increasing trend from 1982 to 2020, and the relationship between the size of grassland NPP values was growing season > summer > spring > autumn. The NPP of Xinjiang grasslands showed a spatial distribution of south-high-north-low and west-high-east-low types, with the largest proportion of areas showing rising NPP in spring.
(2) The severity of drought in Xinjiang from 1982 to 2020 showed an increasing trend, but all were dominated by mild drought. Mild drought occurred most frequently in the growing season and autumn, and moderate drought occurred most frequently in spring. Drought severity increased in most areas of Xinjiang in spring, summer, autumn, and the growing season, but the increase in drought severity was weaker. The regions with a significant increase in drought were primarily southern and eastern Xinjiang, with weakening drought in the northwest.
(3) A total of 64.63% of grassland NPP had a mainly concurrent effect on drought, and these grasslands were primarily located in the northern region of Xinjiang. The NPP and SPEI were positively correlated in summer, negatively correlated in spring, negatively correlated in autumn for desert and alpine subalpine grasslands, and negatively correlated in the growing season only for desert grasslands. The concurrent effect of drought on NPP was strongest in plain grasslands and weakest in alpine subalpine grasslands.
(4) The lag effect of drought on the growing season of grasslands in Xinjiang differed significantly from north to south, and the southern grasslands dominated the grasslands with a time-lag effect. The time-lag effect of different grassland types on drought was different, with plain grassland NPP showing mainly no time-lag effect on drought; alpine subalpine meadow, meadow, and alpine subalpine grassland NPP showing mainly a 1-month time-lag effect on drought; and desert grassland NPP showing mainly a 3-month time-lag effect on drought.
The raw data supporting the conclusions of this article will be made available by the authors, without undue reservation.
YL: conceptualization, methodology, writing – original draft, and visualization. YL and JG: methodology and writing – review and editing. YL and WH: formal analysis and investigation. YL and LL: data curation and validation. JZ: writing – review and editing and project administration. All authors contributed to the article and approved the submitted version.
This study was supported by the Response Mechanism of Net Primary Productivity of Xinjiang Grassland to Extreme Drought Project of Xinjiang Uygur Autonomous Region (No. 2022D04009) and the Tianshan Cedar Project of Xinjiang Uygur Autonomous Region (No. 2020XS04).
The authors declare that the research was conducted in the absence of any commercial or financial relationships that could be construed as a potential conflict of interest.
All claims expressed in this article are solely those of the authors and do not necessarily represent those of their affiliated organizations, or those of the publisher, the editors and the reviewers. Any product that may be evaluated in this article, or claim that may be made by its manufacturer, is not guaranteed or endorsed by the publisher.
The Supplementary material for this article can be found online at: https://www.frontiersin.org/articles/10.3389/fevo.2023.1131175/full#supplementary-material
1. ^https://ladsweb.modaps.eosdis.nasa.gov/search/
2. ^https://ecocast.arc.nasa.gov/data/pub/gimms/
4. ^https://ldas.gsfc.nasa.gov/index.php/fldas/
Anderegg, W. R. L., Schwalm, C., Biondi, F., Camarero, J. J., Koch, G., Litvak, M., et al. (2015). Pervasive drought legacies in forest ecosystems and their implications for carbon cycle models. Science 349, 528–532. doi: 10.1126/science.aab1833
Carrão, H., Naumann, G., and Barbosa, P. (2017). Global projections of drought hazard in a warming climate: a prime for disaster risk management. Clim. Dyn. 50, 2137–2155. doi: 10.1007/s00382-017-3740-8
Chen, L., Li, H., Zhang, P., Zhao, X., Zhou, L., Liu, T., et al. (2015). Climate and native grassland vegetation as drivers of the community structures of shrub-encroached grasslands in Inner Mongolia, China. Landsc. Ecol. 30, 1627–1641. doi: 10.1007/s10980-014-0044-9
Chen, T., Werf, G. R., Jeu, R. A. M., Wang, G., and Dolman, A. J. (2013). A global analysis of the impact of drought on net primary productivity. Hydrol. Earth Syst. Sci. 17, 3885–3894. doi: 10.5194/hess-17-3885-2013
Dai, A. (2013). Increasing drought under global warming in observations and models. Nat. Clim. Chang. 3, 52–58. doi: 10.1038/nclimate1633
Easterling, D. R., Meehl, G. A., Parmesan, C., Changnon, S. A., Karl, T. R., and Mearns, L. O. (2000). Climate extremes: observations, modeling, and impacts. Science 289, 2068–2074. doi: 10.1126/science.289.5487.2068
Gocic, M., and Trajkovic, S. (2013). Analysis of changes in meteorological variables using mann-kendall and sen’s slope estimator statistical tests in Serbia. Glob. Planet. Chang. 100, 172–182. doi: 10.1016/j.gloplacha.2012.10.014
Graham, S. L., Laubach, J., Hunt, J. E., Eger, A., Carrick, S., and Whitehead, D. (2019). Predicting soil water balance for irrigated and non-irrigated lucerne on stony, alluvial soils. Agric. Water Manag. 226:105790. doi: 10.1016/j.agwat.2019.105790
Gu, X., Zhang, Q., Li, J., Singh, V. P., and Sun, P. (2019). Impact of urbanization on nonstationarity of annual and seasonal precipitation extremes in China. J. Hydrol. 575, 638–655. doi: 10.1016/j.jhydrol.2019.05.070
Guan, J., Yao, J., Li, M., Li, D., and Zheng, J. (2022). Historical changes and projected trends of extreme climate events in Xinjiang, China. Clim. Dyn. 59, 1753–1774. doi: 10.1007/s00382-021-06067-2
Guan, J., Yao, J., Li, M., and Zheng, J. (2021). Assessing the spatiotemporal evolution of anthropogenic impacts on remotely sensed vegetation dynamics in Xinjiang, China. Remote Sens. 13:4651. doi: 10.3390/rs13224651
Guo, H., Bao, A., Liu, T., Jiapaer, G., Ndayisaba, F., Jiang, L., et al. (2018). Spatial and temporal characteristics of droughts in central Asia during 1966–2015. Sci. Total Environ. 624, 1523–1538. doi: 10.1016/j.scitotenv.2017.12.120
Han, W., Guan, J., Zheng, J., Liu, Y., Ju, X., Liu, L., et al. (2023). Probabilistic assessment of drought stress vulnerability in grasslands of Xinjiang, China. Front. Plant Sci. 14:1143863. doi: 10.3389/fpls.2023.1143863
Huang, L., He, B., Chen, A., Wang, H., Liu, J., Lű, A., et al. (2016). Drought dominates the interannual variability in global terrestrial net primary production by controlling semi-arid ecosystems. Sci. Rep. 6:24639. doi: 10.1038/srep24639
IPCC. (2021). “Summary for policymakers.” in Climate change 2021: The physicalscience basis. Contribution of working group i to the sixth assessment report of the intergovernmental panel on climate change: Cambridge University Press.
Jiapaer, G., Liang, S., Yi, Q., and Liu, J. (2015). Vegetation dynamics and responses to recent climate change in Xinjiang using leaf area index as an indicator. Ecol. Indic. 58, 64–76. doi: 10.1016/j.ecolind.2015.05.036
Knapp, A. K., and Hamerlynck, E. P. (1993). Photosynthetic and water relations responses to elevated co2 in the c4 grass Andropogon gerardii. Int. J. Plant Sci. 154, 459–466. doi: 10.1086/297129
Lefi, E., Medrano, H., and Cifre, J. (2004). Water uptake dynamics, photosynthesis and water use efficiency in field-grown Medicago arborea and Medicago citrina under prolonged mediterranean drought conditions. Ann. Appl. Biol. 144, 299–307. doi: 10.1111/j.1744-7348.2004.tb00345.x
Li, C., Leal Filho, W., Yin, J., Hu, R., Wang, J., Yang, C., et al. (2018). Assessing vegetation response to multi-time-scale drought across Inner Mongolia plateau. J. Clean. Prod. 179, 210–216. doi: 10.1016/j.jclepro.2018.01.113
Li, Y., and Sun, C. (2017). Impacts of the superimposed climate trends on droughts over 1961–2013 in Xinjiang, China. Theor. Appl. Climatol. 129, 977–994. doi: 10.1007/s00704-016-1822-x
Liberato, M. L. R., Ramos, A. M., Gouveia, C. M., Sousa, P., Russo, A., Trigo, R. M., et al. (2017). Exceptionally extreme drought in madeira archipelago in 2012: vegetation impacts and driving conditions. Agric. For. Meteorol. 232, 195–209. doi: 10.1016/j.agrformet.2016.08.010
Lin, L., Gao, T., Luo, M., Ge, E., Yang, Y., Liu, Z., et al. (2020). Contribution of urbanization to the changes in extreme climate events in urban agglomerations across China. Sci. Total Environ. 744:140264. doi: 10.1016/j.scitotenv.2020.140264
Liu, X., Ma, Q., Yu, H., Li, Y., Li, L., Qi, M., et al. (2021). Climate warming-induced drought constrains vegetation productivity by weakening the temporal stability of the plant community in an arid grassland ecosystem. Agric. For. Meteorol. 307:108526. doi: 10.1016/j.agrformet.2021.108526
Liu, L., Peng, J., Li, G., Guan, J., Han, W., Ju, X., et al. (2023). Effects of drought and climate factors on vegetation dynamics in central asia from 1982 to 2020. J. Environ. Manag. 328:116997. doi: 10.1016/j.jenvman.2022.116997
Liu, Z., Wang, Y., Shao, M., Jia, X., and Li, X. (2016). Spatiotemporal analysis of multiscalar drought characteristics across the loess plateau of China. J. Hydrol. 534, 281–299. doi: 10.1016/j.jhydrol.2016.01.003
Liu, Y., Wang, Q., Zhang, Z., Tong, L., Wang, Z., and Li, J. (2019). Grassland dynamics in responses to climate variation and human activities in China from 2000 to 2013. Sci. Total Environ. 690, 27–39. doi: 10.1016/j.scitotenv.2019.06.503
Liu, Y., Zhou, R., Wen, Z., Khalifa, M., Zheng, C., Ren, H., et al. (2021). Assessing the impacts of drought on net primary productivity of global land biomes in different climate zones. Ecol. Indic. 130:108146. doi: 10.1016/j.ecolind.2021.108146
Liu, X., Zhu, X., Pan, Y., Li, S., Liu, Y., and Ma, Y. (2016). Agricultural drought monitoring: progress, challenges, and prospects. J. Geogr. Sci. 26, 750–767. doi: 10.1007/s11442-016-1297-9
Ma, R., Xia, C., Liu, Y., Wang, Y., Zhang, J., Shen, X., et al. (2022). Spatiotemporal change of net primary productivity and its response to climate change in temperate grasslands of China. Front. Plant Sci. 13:899800. doi: 10.3389/fpls.2022.899800
Mann, H. B. (1945). Non-parametric tests against trend. Econometrica 13, 245–259. doi: 10.2307/1907187
Mckee, T. B., Doesken, N. J., and Kleist, J. (1993). The relationship of drought frequency and duration to time scales. In proceedings of the 8th conference on applied climatology. 17(22), 179–183.
Miao, L., Li, S., Zhang, F., Chen, T., Shan, Y., and Zhang, Y. (2020). Future drought in the dry lands of asia under the 1.5 and 2.0 °c warming scenarios. Earth’s Future 8:e2019EF001337. doi: 10.1029/2019EF001337
Morgan, J. A., LeCain, D. R., Pendall, E., Blumenthal, D. M., Kimball, B. A., Carrillo, Y., et al. (2011). C4 grasses prosper as carbon dioxide eliminates desiccation in warmed semi-arid grassland. Nature (London) 476, 202–205. doi: 10.1038/nature10274
Morgan, J. A., Pataki, D. E., Körner, C., Clark, H., del Grosso, S. J., Grünzweig, J. M., et al. (2004). Water relations in grassland and desert ecosystems exposed to elevated atmospheric CO2. Oecologia 140, 11–25. doi: 10.1007/s00442-004-1550-2
Nemani, R. R., Keeling, C. D., Hashimoto, H., Jolly, W. M., Piper, S. C., Tucker, C. J., et al. (2003). Climate-driven increases in global terrestrial net primary production from 1982 to 1999. Science 300, 1560–1563. doi: 10.1126/science.1082750
Pei, Y., Huang, J., Wang, L., Chi, H., and Zhao, Y. (2018). An improved phenology-based casa model for estimating net primary production of forest in Central China based on landsat images. Int. J. Remote Sens. 39, 7664–7692. doi: 10.1080/01431161.2018.1478464
Pei, F., Li, X., Liu, X., and Lao, C. (2013). Assessing the impacts of droughts on net primary productivity in China. J. Environ. Manag. 114, 362–371. doi: 10.1016/j.jenvman.2012.10.031
Piao, S., Wang, X., Ciais, P., Zhu, B., Wang, T., and Liu, J. (2011). Changes in satellite-derived vegetation growth trend in temperate and boreal Eurasia from 1982 to 2006. Glob. Chang. Biol. 17, 3228–3239. doi: 10.1111/j.1365-2486.2011.02419.x
Potter, C., Klooster, S., and Genovese, V. (2012). Net primary production of terrestrial ecosystems from 2000 to 2009. Clim. Chang. 115, 365–378. doi: 10.1007/s10584-012-0460-2
Potter, C. S., Randerson, J. T., Field, C. B., Matson, P. A., and Klooster, S. A. (1993). Terrestrial ecosystem production: a process model based on global satellite and surface data. Glob. Biogeochem. Cycles 7, 811–841. doi: 10.1029/93GB02725
Qi, X., Jia, J., Liu, H., and Lin, Z. (2019). Relative importance of climate change and human activities for vegetation changes on china’s silk road economic belt over multiple timescales. Catena 180, 224–237. doi: 10.1016/j.catena.2019.04.027
Ran, M., Zhang, C., and Feng, Z. (2015). Climatic and hydrological variations during the past 8000 years in northern Xinjiang of China and the associated mechanisms. Quat. Int. 358, 21–34. doi: 10.1016/j.quaint.2014.07.056
Saxe, H., Cannell, M. G. R., Johnsen, Y., Ryan, M. G., and Vourlitis, G. (2001). Tree and forest functioning in response to global warming. New Phytol. 149, 369–399. doi: 10.1046/j.1469-8137.2001.00057.x
Sen, P. K. (1968). Estimates of the regression coefficient based on kendall’s tau. J. Am. Stat. Assoc. 63, 1379–1389. doi: 10.1080/01621459.1968.10480934
Sevanto, S. (2018). Drought impacts on phloem transport. Curr. Opin. Plant Biol. 43, 76–81. doi: 10.1016/j.pbi.2018.01.002
Shen, X., Liu, B., Henderson, M., Wang, L., Jiang, M., and Lu, X. (2022). Vegetation greening, extended growing seasons, and temperature feedbacks in warming temperate grasslands of China. J. Clim. 35, 5103–5117. doi: 10.1175/JCLI-D-21-0325.1
Shen, X., Liu, B., Jiang, M., and Lu, X. (2020). Marshland loss warms local land surface temperature in China. Geophys. Res. Lett. 47:e2020GL087648. doi: 10.1029/2020GL087648
Shi, Y., Shen, Y., Kang, E., Li, D., Ding, Y., Zhang, G., et al. (2007). Recent and future climate change in Northwest China. Clim. Chang. 80, 379–393. doi: 10.1007/s10584-006-9121-7
Shi, Z., Thomey, M. L., Mowll, W., Litvak, M., Brunsell, N. A., Collins, S. L., et al. (2014). Differential effects of extreme drought on production and respiration: synthesis and modeling analysis. Biogeosciences 11, 621–633. doi: 10.5194/bg-11-621-2014
Spinoni, J., Naumann, G., Carrao, H., Barbosa, P., and Vogt, J. (2014). World drought frequency, duration, and severity for 1951-2010. Int. J. Climatol. 34, 2792–2804. doi: 10.1002/joc.3875
Sun, G., and Mu, M. (2018). Assessing the characteristics of net primary production due to future climate change and co 2 under rcp4.5 in China. Ecol. Complex. 34, 58–68. doi: 10.1016/j.ecocom.2018.04.001
Sun, B., Zhao, H., and Wang, X. (2016). Effects of drought on net primary productivity: roles of temperature, drought intensity, and duration. Chin. Geogr. Sci. 26, 270–282. doi: 10.1007/s11769-016-0804-3
Thornthwaite, C. W. (1948). An approach toward a rational classification of climate. Geogr. Rev. 38, 55–94. doi: 10.2307/210739
Tong, S., Li, X., Zhang, J., Bao, Y., Bao, Y., Na, L., et al. (2019). Spatial and temporal variability in extreme temperature and precipitation events in Inner Mongolia (China) during 1960–2017. Sci. Total Environ. 649, 75–89. doi: 10.1016/j.scitotenv.2018.08.262
Ummenhofer, C. C., and Meehl, G. A. (2017). Extreme weather and climate events with ecological relevance: a review. Philos. Trans. R. Soc. B Biol. Sci. 372:20160135. doi: 10.1098/rstb.2016.0135
Vicca, S., Balzarolo, M., Filella, I., Granier, A., Herbst, M., Knohl, A., et al. (2016). Remotely-sensed detection of effects of extreme droughts on gross primary production. Sci. Rep. 6:28269. doi: 10.1038/srep28269
Vicente-Serrano, S. M., Beguería, S., and López-Moreno, J. I. (2010). A multiscalar drought index sensitive to global warming: the standardized precipitation evapotranspiration index. J. Clim. 23, 1696–1718. doi: 10.1175/2009JCLI2909.1
Vicente-Serrano, S. M., Gouveia, C., Camarero, J. J., Beguería, S., Trigo, R., López-Moreno, J. I., et al. (2013). Response of vegetation to drought time-scales across global land biomes. Proc. Natl. Acad. Sci. 110, 52–57. doi: 10.1073/pnas.1207068110
Wang, Q., Yang, Y., Liu, Y., Tong, L., and Li, J. (2019). Assessing the impacts of drought on grassland net primary production at the global scale. Sci. Rep. 9, 1–8. doi: 10.1038/s41598-019-50584-4
Wang, Z., Zhang, Y., Yang, Y., Zhou, W., Gang, C., Zhang, Y., et al. (2016). Quantitative assess the driving forces on the grassland degradation in the Qinghai–Tibet plateau, in China. Ecol. Inform. 33, 32–44. doi: 10.1016/j.ecoinf.2016.03.006
Wang, Z., Zhong, R., Lai, C., Zeng, Z., Lian, Y., and Bai, X. (2018). Climate change enhances the severity and variability of drought in the pearl river basin in South China in the 21st century. Agric. For. Meteorol. 249, 149–162. doi: 10.1016/j.agrformet.2017.12.077
Wen, Y., Liu, X., Xin, Q., Wu, J., Xu, X., Pei, F., et al. (2019). Cumulative effects of climatic factors on terrestrial vegetation growth. J. Geophys. Res. Biogeosci. 124, 789–806. doi: 10.1029/2018JG004751
Weng, E., and Luo, Y. (2008). Soil hydrological properties regulate grassland ecosystem responses to multifactor global change: a modeling analysis. J. Geophys. Res. 113:G03003. doi: 10.1029/2007JG000539
Wu, L., Ma, X., Dou, X., Zhu, J., and Zhao, C. (2021). Impacts of climate change on vegetation phenology and net primary productivity in arid central Asia. Sci. Total Environ. 796:149055. doi: 10.1016/j.scitotenv.2021.149055
Xue, Z., Kappas, M., and Wyss, D. (2021). Spatio-temporal grassland development in Inner Mongolia after implementation of the first comprehensive nation-wide grassland conservation program. Land 10:38. doi: 10.3390/land10010038
Yang, H., Mu, S., and Li, J. (2014). Effects of ecological restoration projects on land use and land cover change and its influences on territorial npp in Xinjiang, China. Catena 115, 85–95. doi: 10.1016/j.catena.2013.11.020
Yao, J., Chen, Y., Zhao, Y., Guan, X., Mao, W., and Yang, L. (2020). Climatic and associated atmospheric water cycle changes over the Xinjiang, China. J. Hydrol. 585:124823. doi: 10.1016/j.jhydrol.2020.124823
Yao, J., Hu, W., Chen, Y., Huo, W., Zhao, Y., Mao, W., et al. (2019). Hydro-climatic changes and their impacts on vegetation in Xinjiang, central asia. Sci. Total Environ. 660, 724–732. doi: 10.1016/j.scitotenv.2019.01.084
Yao, J., Zhao, Y., Chen, Y., Yu, X., and Zhang, R. (2018). Multi-scale assessments of droughts: a case study in Xinjiang, China. Sci. Total Environ. 630, 444–452. doi: 10.1016/j.scitotenv.2018.02.200
Ye, C., Sun, J., Liu, M., Xiong, J., Zong, N., Hu, J., et al. (2020). Concurrent and lagged effects of extreme drought induce net reduction in vegetation carbon uptake on Tibetan plateau. Remote Sens. 12:2347. doi: 10.3390/rs12152347
Zhang, R., Guo, J., and Yin, G. (2021). Response of net primary productivity to grassland phenological changes in Xinjiang, China. PeerJ 9:e10650. doi: 10.7717/peerj.10650
Zhang, Y., He, B., Guo, L., Liu, J., and Xie, X. (2019). The relative contributions of precipitation, evapotranspiration, and runoff to terrestrial water storage changes across 168 river basins. J. Hydrol. 579:124194. doi: 10.1016/j.jhydrol.2019.124194
Zhang, C., Lu, D., Chen, X., Zhang, Y., Maisupova, B., and Tao, Y. (2016). The spatiotemporal patterns of vegetation coverage and biomass of the temperate deserts in central Asia and their relationships with climate controls. Remote Sens. Environ. 175, 271–281. doi: 10.1016/j.rse.2016.01.002
Zhang, H., Song, J., Wang, G., Wu, X., and Li, J. (2021). Spatiotemporal characteristic and forecast of drought in northern Xinjiang, China. Ecol. Indic. 127:107712. doi: 10.1016/j.ecolind.2021.107712
Zhang, X., Susan Moran, M., Zhao, X., Liu, S., Zhou, T., Ponce-Campos, G. E., et al. (2014). Impact of prolonged drought on rainfall use efficiency using Modis data across China in the early 21st century. Remote Sens. Environ. 150, 188–197. doi: 10.1016/j.rse.2014.05.003
Zhang, R., Wang, Z., Han, G., Schellenberg, M. P., Wu, Q., and Gu, C. (2018). Grazing induced changes in plant diversity is a critical factor controlling grassland productivity in the desert steppe, northern China. Agric. Ecosyst. Environ. 265, 73–83. doi: 10.1016/j.agee.2018.05.014
Zhao, M., and Running, S. W. (2010). Drought-induced reduction in global terrestrial net primary production from 2000 through 2009. Science 329, 940–943. doi: 10.1126/science.1192666
Zhao, A., Yu, Q., Feng, L., Zhang, A., and Pei, T. (2020). Evaluating the cumulative and time-lag effects of drought on grassland vegetation: a case study in the Chinese loess plateau. J. Environ. Manag. 261:110214. doi: 10.1016/j.jenvman.2020.110214
Zhou, Y., Zhang, R., Wang, S. X., Wang, F. T., and Qi, Y. (2019). Comparative an anlysis on responses of vegetation productivity relative to different drought monitor patterns in karst regions of southwestern China. Appl. Ecol. Environ. Res. 17, 85–105. doi: 10.15666/aeer/1701_085105
Zhu, W., Pan, Y., and Hao, H. E. (2006). Simulation of maximum light use efficiency for some typical vegetation types in China. Chin. Sci. Bull. 51, 457–463. doi: 10.1007/s11434-006-0457-1
Keywords: grassland, net primary productivity, drought, concurrent and lagged effect, remote sensing - GIS
Citation: Liu Y, Zheng J, Guan J, Han W and Liu L (2023) Concurrent and lagged effects of drought on grassland net primary productivity: a case study in Xinjiang, China. Front. Ecol. Evol. 11:1131175. doi: 10.3389/fevo.2023.1131175
Received: 24 December 2022; Accepted: 04 April 2023;
Published: 20 April 2023.
Edited by:
Changchun Huang, Nanjing Normal University, ChinaReviewed by:
Xiangjin Shen, Chinese Academy of Sciences, ChinaCopyright © 2023 Liu, Zheng, Guan, Han and Liu. This is an open-access article distributed under the terms of the Creative Commons Attribution License (CC BY). The use, distribution or reproduction in other forums is permitted, provided the original author(s) and the copyright owner(s) are credited and that the original publication in this journal is cited, in accordance with accepted academic practice. No use, distribution or reproduction is permitted which does not comply with these terms.
*Correspondence: Jianghua Zheng, emhlbmcuamlhbmdodWFAeGp1LmVkdS5jbg==
Disclaimer: All claims expressed in this article are solely those of the authors and do not necessarily represent those of their affiliated organizations, or those of the publisher, the editors and the reviewers. Any product that may be evaluated in this article or claim that may be made by its manufacturer is not guaranteed or endorsed by the publisher.
Research integrity at Frontiers
Learn more about the work of our research integrity team to safeguard the quality of each article we publish.