- Biotechnology, University of Applied Sciences Mittweida, Mittweida, Germany
The possibility to identify plants based on the taxonomic information coming from their pollen grains offers many applications within various biological disciplines. In the past and depending on the application or research in question, pollen origin was analyzed by microscopy, usually preceded by chemical treatment methods. This procedure for identification of pollen grains is both time-consuming and requires expert knowledge of morphological features. Additionally, these microscopically recognizable features usually have a low resolution at species-level. Since a few decades, DNA has been used for the identification of pollen taxa, as sequencing technologies evolved both in their handling and affordability. We discuss advantages and challenges of pollen DNA analyses compared to traditional methods. With readers with little experience in this field in mind, we present a hands-on primer for genetic pollen analysis by nanopore sequencing. As our lab mainly works with pollen collected within agroecological research projects, we focus on pollen collected by pollinating insects. We briefly consider sample collection, storage and processing in the laboratory as well as bioinformatic aspects. Currently, pollen metabarcoding is mostly conducted with next-generation sequencing methods that generate short sequence reads (<1 kb). Increasingly, however, pollen DNA analysis is carried out using the long-read generating (several kb), low-budget and mobile MinION nanopore sequencing platform by Oxford Nanopore Technologies. Therefore, we are focusing on aspects for palynology with the MinION DNA sequencing device.
1. Potential of pollen analysis
Species declines are becoming increasingly serious. Agricultural intensification is considered a major driver of biodiversity decline that also affects functionally relevant species, including pollinators (Díaz et al., 2019; Krehenwinkel et al., 2019; Raven and Wagner, 2021). Land use intensification additionally causes biotic homogenization of plant and animal communities in agricultural landscapes (Parreño et al., 2022). Besides, deforestation, industrialization and urbanization contribute to the elimination of nesting places and habitats for many species leading to a loss of overall biodiversity (Sánchez-Bayo and Wyckhuys, 2019). To counteract this development, mankind needs as much information as possible about the influences of the above-mentioned impacts on existing communities and ecosystems. Biomonitoring methods aim to identify species and conditions to measure changes in ecosystems (Hajibabaei et al., 2011).
Biomonitoring methods are especially in demand for the analysis of plant-pollinator networks, not only in natural and agricultural landscapes, including forests (Carneiro de Melo Moura et al., 2022), but also in urban ecosystems (Udy et al., 2020). In particular, insect pollinators are indispensable due to their pollination services (Porto et al., 2020; Baylis et al., 2021). Detailed knowledge of existing plant-pollinator networks and the foraging behavior of pollinators in different landscapes can help to maintain future pollination services and support management strategies (Leidenfrost et al., 2020; Bell et al., 2022; Namin et al., 2022). Both, plant-pollinator networks and foraging behavior can be reconstructed with the analysis of pollen grains collected by pollinators. This information may be used to guide, for example, urban planting projects or ecological landscaping (Potter et al., 2019). Identification of the plants used for honey production can also provide valuable information to beekeepers and consumers; indeed, marketing and validation of specialty honey, such as Manuka honey, requires information about the floral source (Galimberti et al., 2014). Furthermore, the identification of the pollen source supports the quality control of other bee products such as royal jelly or propolis, whose composition is also influenced by pollen diversity (Danner et al., 2017; Kegode et al., 2022). Finally, since pollen contains carbohydrates, lipids, vitamins, minerals and all the basic amino acids, its correct composition is of great importance for pollinators’ health (Di Pasquale et al., 2013; Frias et al., 2016).
Palynology is very interdisciplinary and has a huge outreach (Figure 1). Besides in agricultural sciences, it also plays a major role in, e.g., aerobiology, a discipline that investigates the passive transport of bioaerosols through air. Here, pollen is mostly studied in the context of allergen monitoring (Fragola et al., 2022; Khan et al., 2022; Polling et al., 2022). In forensic palynology, pollen, which easily attaches to many surfaces such as skin and clothes, which is insensitive to chemical reactions, and that is incredibly durable, provides information about the potential timing and location of a crime scene (Alotaibi et al., 2020). In paleoecological and paleoclimatological research, pollen is applied as well. With fossil pollen from sediment or ice cores, climate reconstructions from the quaternary period (2.6 million years ago) and older were possible (Chevalier et al., 2020).
2. Advantages and challenges of genetic pollen studies
For the microscopic identification of pollen grains, expert knowledge and plenty of time is needed. In contrast, genetic processing of pollen does not require years of experience in palynology but can be carried out by virtually all experienced molecular biologists (Bell et al., 2022). Furthermore, the taxonomic resolution based on morphological traits is limited, as not for all plant families the species can be determined. Pollen of the Rosaceae, e.g., to which many important fruit varieties belong, show a very similar morphology (Lechowicz et al., 2020). This fact also restricts the success of computer-assisted analysis of micrographs (Polling et al., 2022). But with DNA analysis, e.g., DNA metabarcoding, pollen can be identified in more detail (Potter et al., 2019; Ruppert et al., 2019). Additionally, not only single pollen grains but also mixed bulk samples can be processed, which makes DNA metabarcoding an important tool for understanding and monitoring ecosystems (Vamosi et al., 2017). Furthermore, a higher number of taxa than in classical observation trials can be detected (Bell et al., 2016; Pornon et al., 2017).
The fact that DNA could be made readable imposed entirely new perspectives on the term biodiversity since genetic information paved the way for rapid taxa identification, even of previously unknown taxa (Hebert and Gregory, 2005). In addition, high-throughput methods enabled the processing of data volumes greater than ever and thereby allowed the realization of large-scale metagenomic surveys (Fišer Pečnikar and Buzan, 2014; Reuter et al., 2015; Thomsen and Willerslev, 2015). With one pollen sample, e.g., coming from a pollinator insect, multiple interactions can be efficiently analyzed, for which several years of observation would otherwise have been necessary. E.g., from one single intestinal DNA sample one can detect plant-pollinator interactions as well as the microbiome composition. Thus, with molecular palynology high-throughput biodiversity monitoring can be conducted.
Of course, there are a lot of possible error sources during the process of genetic pollen analysis. We will come to these in the “How to” section. And, in contrast to standard laboratory organisms or sample material like bacteria or blood, there are no well-established methods for DNA isolation from pollen originating from different plant taxa (Bell et al., 2016). Furthermore, depending on which sequencing method is used, the read accuracy may differ (van Dijk et al., 2018). Currently, if all steps from pollen sample collection, DNA isolation and all subsequent steps to DNA sequencing and subsequent sequence data analysis are added up, DNA sequencing may initially even require more time-effort than microscopic pollen examination.
3. How to: Pollen identification by DNA sequencing
There are numerous available workflows for molecular palynology, the most common being DNA metabarcoding. In this case, not the entire DNA strand is sequenced, but only a short part of it (Taberlet et al., 2018). Pollen metabarcoding is made up of five steps: pollen collection, DNA isolation, barcode amplification, sequencing and downstream bioinformatic data analysis (Figure 2). Depending on the source of the pollen, the available laboratory equipment or the data that is sought to be generated, different methods may be applied in each step. In order to achieve maximum success and a high significance of the results, a good quality of the intermediate product must be produced in each step, i.e., DNA purity, amplicon purity, read length, quality score or completeness of databases. Therefore, it is important to work in a clean environment and to disinfect all equipment.
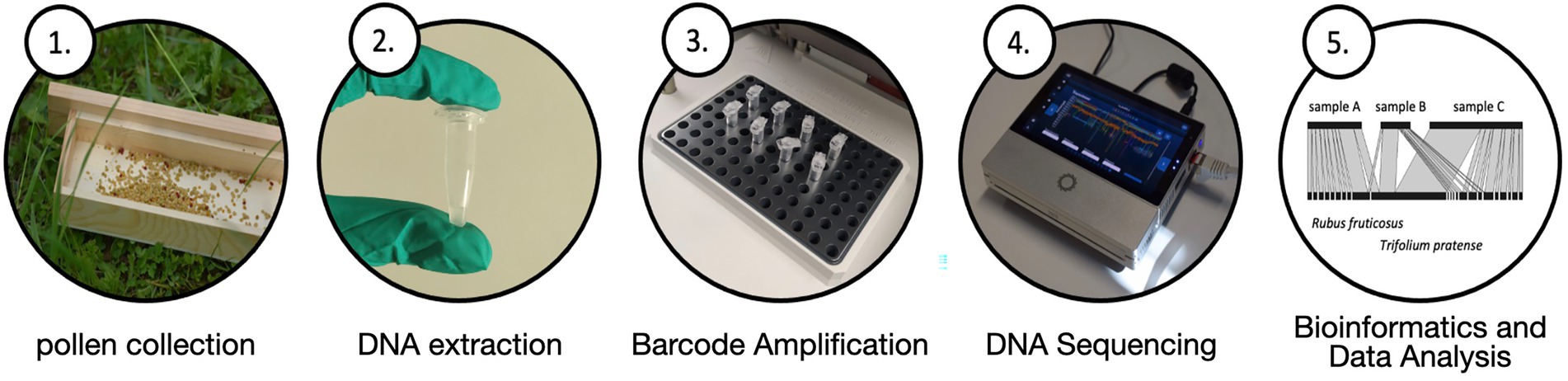
Figure 2. DNA metabarcoding consists of five steps. These steps vary in their execution depending on the sample material and the ecological question. First, the starting material must be prepared in different ways to obtain an appropriate concentration of DNA. Depending on the downstream application, the barcode is amplified and the DNA is read with a selected sequencing method so that the data can later be analyzed accordingly.
3.1. Pollen sampling and storage
Depending on the source, pollen tells different stories. To create a plant-specific pollen image database, it usually has to be collected directly from its origin, the flower (Shivanna and Rangaswamy, 1992). Pollen collection directly from the flower is also necessary when either the success or efficiency of DNA extraction methods, the level of polyploidy, or the presence of plant organelles are of particular interest. However, to establish plant barcode databases, DNA can be collected directly from any DNA containing part of the plant. To infer plant-pollinator networks, though, pollen is collected from pollinators or their nests for molecular palynology.
3.1.1. Sampling pollen from flowers
For many plants, non-disruptive pollen sampling of the flower can be carried out with sterilized spatula. In some cases, the plant must be shaken or lightly rubbed over a 0.5 mm sieve. However, not every plant is suitable for this, as there is not much free pollen available from all plant species. In such cases, the anthers must be collected from the flowers and dried. After drying, they release pollen from their interior. The sieve method can also be used here. If the flowers are subjected to vibration (e.g., by using electric toothbrush), the pollen released from the flower can be collected directly in a container (Knäbe et al., 2014).
3.1.2. Sampling pollen from pollinators
Pollen collected by pollinators might either be loosely attached to their body or mixed with plant nectar or insect saliva. The latter is usually deposited in the nest. Thus, the pollen might either be sampled directly from the insect or its nest. Pollen sampling from individuals can be used to study the foraging activities of bees.
Honey bees and bumble bees transport the captured pollen grains from the flower to their hive in the form of pollen loads and store it as an energy and protein resource to feed their colony. For honey bee pollen, so called pollen traps can be installed in front of the beehive. The honey bees have to pass through this perforated grid where they lose their pollen loads. These fall into a drawer and can be collected (Bänsch et al., 2020). Pollen traps are also available for bumble bee nests (Judd et al., 2020).
In contrast, wild solitary bees collect pollen at their abdomen and store it in a clump for their offspring in their nest. The pollen they collect must be sampled with a sterilized spatula. In some studies, insect pollinators are caught and the pollen is sampled from them with tweezers, leaving the individual alive (Biella et al., 2019; Leidenfrost et al., 2020; Rivers-Moore et al., 2020).
3.1.3. Extracting pollen from honey
Next, to biomonitoring issues, tracing the origin and composition of honey is also of interest (Wirta et al., 2021; Liu et al., 2022). However, honey usually contains much less than 1% (w/w) pollen. A huge amount of source material, about 3–10 g, is needed to accumulate enough pollen mass for DNA extraction. Mixed with 30 mL of sterile water, the suspension is incubated at 65°C for 30 min. The dissolved honey sample is afterwards centrifuged (30 min, 15,000 rpm) to pelletize the pollen. The resulting pellet can now be used for DNA isolation (de Vere et al., 2017).
3.1.4. Long-term storage of pollen
When the pollen pellet is resuspended 1:4 (pollen:ethanol) in 70% (v/v) undenatured ethanol, an aliquot can be taken as a randomized sample (Leidenfrost et al., 2020). At the same time, the pollen grains are washed from nectar and contaminants.
Immediately after resuspension in ethanol, it is advisable to take aliquots of 100–400 μL in order create identical replicates. It is important to mix the pollen:ethanol suspension really well to prevent the pipette tip from clogging. Subsequently, after a centrifugation step (10 min, 14,000×g) the supernatant is discarded leaving a washed pollen pellet. After drying in a clean bench for 24–72 h, the pellet can be used for DNA isolation. It should have a mass of about 0.015–0.025 g (Bänsch et al., 2020).
3.2. Pollen disruption and DNA isolation
Pollen samples might originate from plants, airborne pollen, bee foragers or bee nests. Thus, depending on its source, the pollen sample is either composed of only a few grains or a bulk sample representing one or more plant species. Pollen collected from pollinators usually constitute mixed samples as pollinators often visit different flowers (Bell et al., 2017a).
As different pollen species have various morphological structures and sizes, it is a challenge to isolate DNA from the pollen grains (Bell et al., 2016; Halbritter et al., 2018). The pollen wall of seed plants, called sporoderm, is composed of two layers: the inner intine and the outer exine. The exine, mainly consists of the polymer sporopollenin, which is very robust as it is acetolysis- and decay-resistant. These morphological traits enable the preservation of the pollen nutrients (Halbritter et al., 2018). Thus, it requires a good cell disruption method to release the DNA (Yang et al., 2019).
3.2.1. Pollen disruption
For pollen disruption, a practical and time efficient way is bead-beating (Leontidou et al., 2021; James et al., 2022; Polling et al., 2022). When available, ball mills can be used. However, a standard vortex device, typically present in every biological laboratory, is usually sufficient (Kamo et al., 2018). Ceramic beads are both hard enough and feature a rough surface helping to break the pollen wall. Due to the different morphological traits of pollen grains, it is recommended to not only use one but two bead sizes simultaneously. Generally, diameters of 2.8 mm and 1.4 mm yield good results (Bänsch et al., 2020; Leidenfrost et al., 2020). With the disrupted pollen suspension, DNA extraction can be performed.
3.2.2. DNA extraction
It is not clear yet, which DNA extraction method suits best. Commercial plant or food DNA extraction kits were tested in several studies (de Vere et al., 2017; Bell et al., 2017a; Potter et al., 2019). The DNeasy Plant Mini Kit from Qiagen is the most commonly used kit for pollen DNA extraction (Galimberti et al., 2014; Hawkins et al., 2015; Baksay et al., 2020; Bänsch et al., 2020; Vaudo et al., 2020; Gous et al., 2021; Jones et al., 2021), closely followed by the NucleoSpin Food Kit from Macherey-Nagel (Bell et al., 2017a; Voulgari-Kokota et al., 2019; Arstingstall et al., 2021; Swenson and Gemeinholzer, 2021). But there are also other column-based DNA extraction kits provided by Qiagen and Macherey-Nagel that are applied (Leontidou et al., 2021; Oliver et al., 2021; Fragola et al., 2022).
DNA extraction results can vary depending on the storage, disruption and isolation method. DNeasy Plant Mini Kit from Qiagen predicts a DNA yield of 38–40 ng/μL. However, when working with pollen, we usually see a much lower DNA yield of 3–20 ng/μL. For accurate DNA quantification a Qubit fluorometer (Thermo Fisher Scientific Inc.) should be used.
3.3. DNA metabarcoding
DNA barcoding describes the identification of taxa based on standardized barcode sequences (Hebert et al., 2003; Kress et al., 2015). A barcode sequence comprises a short, conserved DNA section, e.g., the mitochondrial cytochrome c oxidase I gene, that can be easily PCR amplified and sequenced. In metabarcoding, the same method is applied to a mixed sample that is analyzed by high-throughput sequencing (Taberlet et al., 2012; Lowe et al., 2022). This way, taxonomic identification can be performed without time consuming observation efforts or morphological expert knowledge (Lamb et al., 2019; Ruppert et al., 2019).
3.3.1. Barcode selection
For the identification of plant taxa present in pollen samples, usually not the complete genomic DNA, but a short, standardized barcode section is used. This barcode section has to be (a) short enough to be PCR amplifiable, (b) distinct enough to show inter-species variability, and (c) enclosed by two inter-species conserved regions serving as primer binding sites (Taberlet et al., 2018).
Table 1 lists frequently selected DNA barcodes with their expected amplicon lengths. In the past, plant pollen was predominantly classified with either organelle rDNA, nuclear rDNA, or internal transcribed spacer (ITS) sequences (Danner et al., 2017; Maestri et al., 2019; Suchan et al., 2019). For pollen, several plant barcodes have been established, namely: rbcL, matK, psbA-trnH, trnL. Plastidic barcodes (rbcL and matK) are not recommended anymore as plastid DNA is not present in all pollen grains (Galimberti et al., 2014; Bell et al., 2016; Richardson et al., 2019). A very popular plant barcode in metabarcoding studies is the ITS region (Danner et al., 2017; Nürnberger et al., 2019; Vaudo et al., 2020; Leontidou et al., 2021). It is comprised of ITS1 and ITS2 that are separated by the 5.8S rRNA gene (Figure 3). It was found that ITS1 has a higher discriminatory power and species identification success rate than ITS2 (Wang et al., 2015). Still, ITS2 has a greater popularity (Table 1). Long-read DNA sequencing methods from Oxford Nanopore Technologies and PacBio allow for the analysis of the complete ITS region.
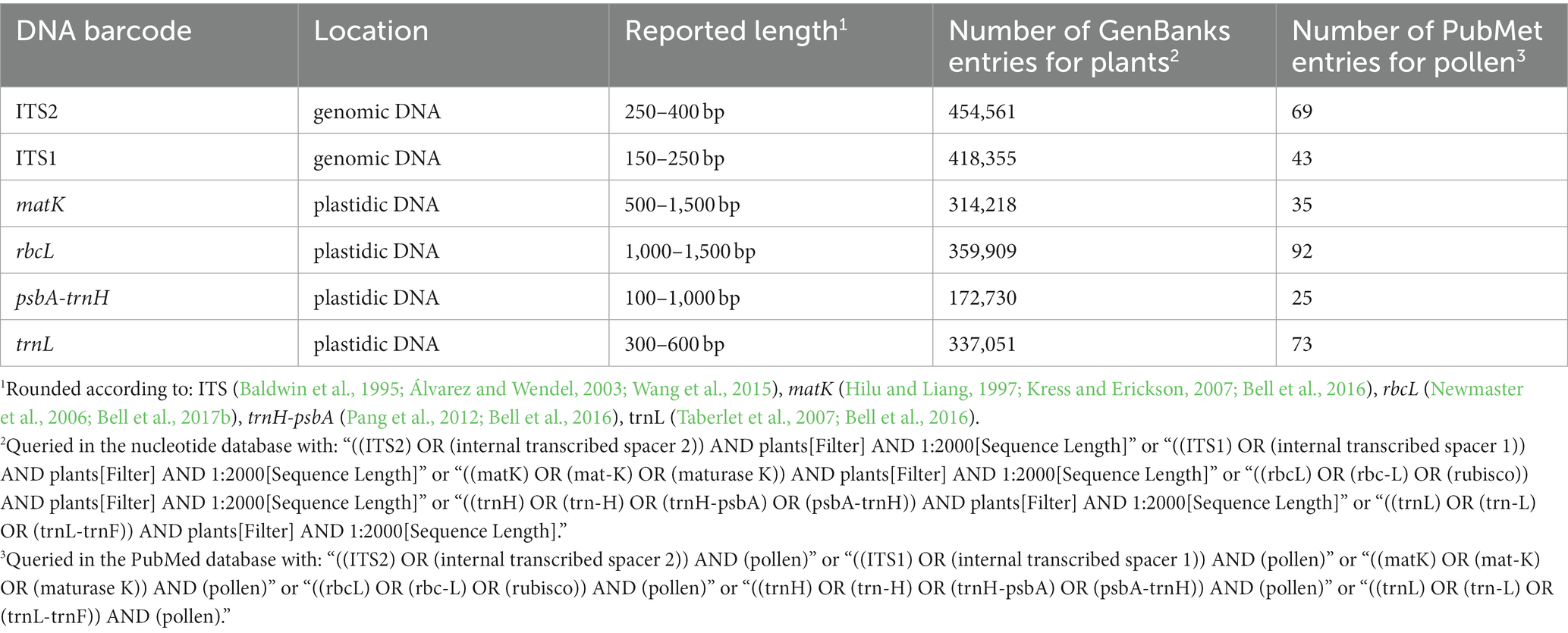
Table 1. Name, location, rounded length and number of GenBank plant and PubMed entries of frequently used plant barcodes (Accessed on 13.01.23).
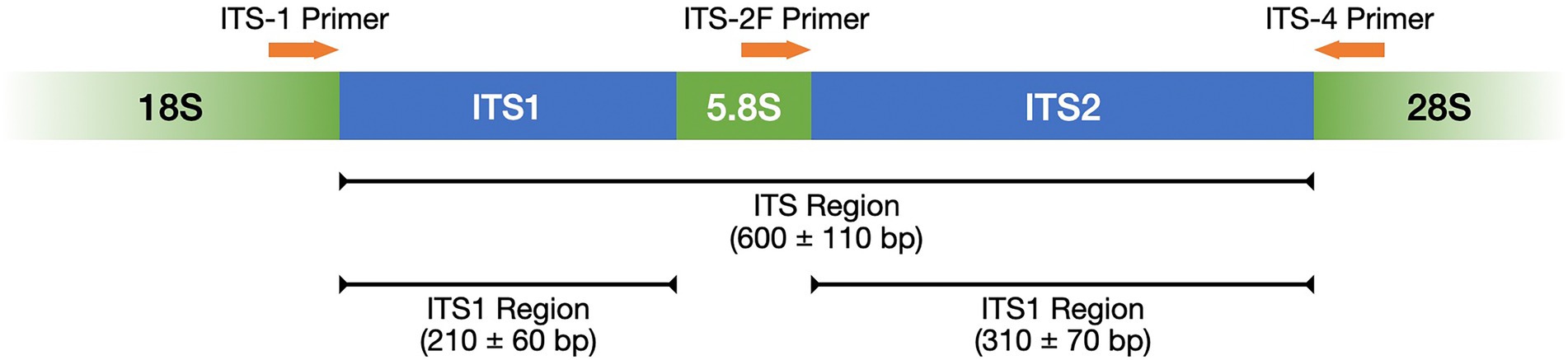
Figure 3. ITS region represented with its subregions ITS1 and ITS2 as well as the complementary primers ITS-1, ITS-2F, and ITS-4. Adopted from Porras-Alfaro et al. (2014).
The discriminatory power of barcodes does not only depend on the sequence length but also on the availability of plant barcodes in sequence databases (Namin et al., 2022). Thus, it is advisable to analyze several barcodes in parallel (see below). However, even if plant barcode reads from pollen cannot be assigned to taxa, their sequence variability can still be used to infer pollen diversity.
3.3.2. PCR amplification of barcode(s)
Before sequencing, all barcodes are amplified by either a standard or multiplex PCR. However, this step may lead to a disproportional, source dependent amplification, a phenomenon called PCR-bias (Liu et al., 2022). For that reason and to ensure a high taxonomic resolution, it is important to use plant barcodes with a high degree of universality across taxonomic groups (Bell et al., 2016; Kamo et al., 2018). Additionally, it has been observed that analysis of one single barcode may lead to ambiguous results. Usually, using a multi-locus approach with more than one barcode increases the discriminatory power (Kamo et al., 2018; Ruppert et al., 2019). Principally, if enough sample is available, plant barcode sequencing can also be performed with raw, unamplified DNA samples. Several samples can still be sequenced in parallel: Multiplexing barcodes can be added to individual samples, e.g., by transposase-assisted tagmentation without PCR (Adey et al., 2010).
3.4. Plant barcode sequencing
Metabarcoding studies are usually performed with high-throughput, next-generation sequencing (NGS), short-read platforms. However, due to high costs and the dependence on external service providers (only few labs have access to their own sequencing device), the cheap, handy and flexible MinION long-read platform from Oxford Nanopore Technologies has become an attractive alternative (Feng et al., 2015; Peel et al., 2019; Srivathsan et al., 2021).
3.4.1. Short-read NGS platforms
Nowadays, mostly next-generation sequencing (NGS) methods are applied for pollen metabarcoding (Figure 4). One popular NGS-method, Illumina sequencing, is largely dominating the market (van Dijk et al., 2018; Lennartz et al., 2021; Leontidou et al., 2021; Tommasi et al., 2022). This sequencing technique relies on the synthesis of a complementary strand via bridging PCR. Drawbacks of Illumina and other NGS methods are that they produce relatively short reads of one hundred to one thousand base pairs, which may cause gaps or incorrect assemblies (Rang et al., 2018; van Dijk et al., 2018). Additionally, there is a need for discussion if the relatively small reads (<250 base pairs) are enough to distinguish between species (Maestri et al., 2019).
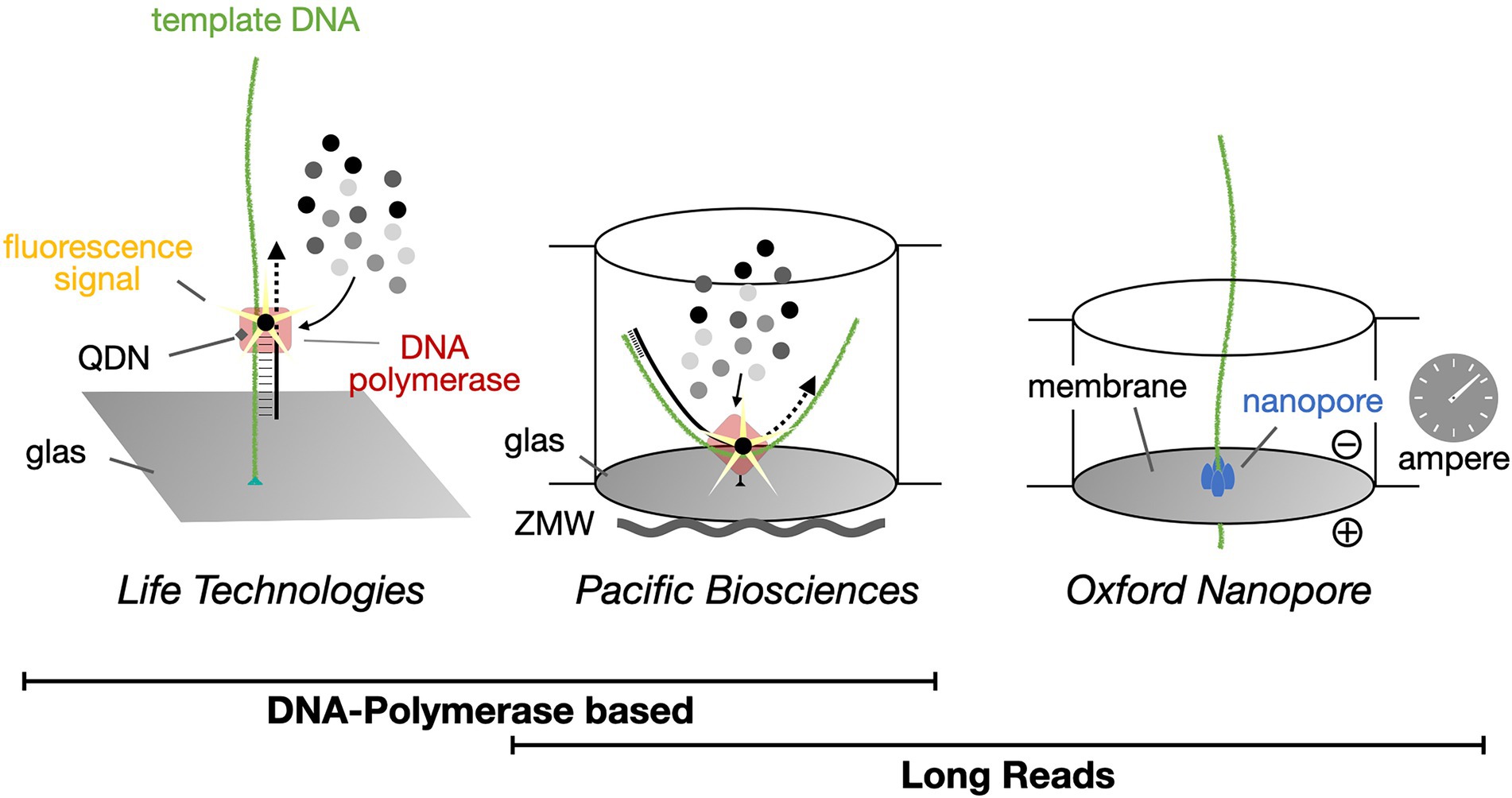
Figure 4. Single-molecule real-time DNA sequencing. Life Technologies Illumina sequencing methodology creates up to 251 bp long high-quality sequence reads and currently dominates the market. In contrast, both Pacific Biosciences (PacBio) and Oxford Nanopore Technologies (ONT) provide platforms for the generation of long (>800 bp) sequence reads, with DNA polymerases or protein nanopores, respectively. QDN, quantum dot nanoparticle; ZMW, zero-mode waveguide.
3.4.2. Long-read MinION platform
Currently, for read lengths over one thousand base pairs, long-read sequencing platforms from either Oxford Nanopore Technologies (ONT) or Pacific Biosciences (PacBio) are available. They can generate read lengths between ten thousand and two million base pairs (Maestri et al., 2019). Here we focus on the application of the portable MinION sequencing device from ONT (Figure 5). With ONT devices, cost-effective, real-time, single-molecule sequencing can be carried out. In principle, even without any intervening amplification step (Krehenwinkel et al., 2019). Depending on the flow cell that is used for sequencing, different read lengths can be achieved. Its nanopore-based sequencing technology allows rapid analyses of DNA samples anywhere and avoids dependency on distant laboratories. For sequencing, extracted, single-stranded DNA fragments are linked to a motor protein that facilitates passage of the DNA molecule through the nanopore. The latter is embedded in a polymer membrane to which a membrane potential is applied (van Dijk et al., 2018). While passing through the membrane, sequence dependent clogging of the pore influences the ion flow through the pore, which in turn can be measured amperometrically. Instead of a fluorogram as obtained from Illumina NGS sequencing methods, the nanopore technique yields a so-called squiggle plot for each DNA molecule, which is then used for base calling (see below). The current MinION technology produces an output of at least five billion bases per run. For the R9.4 flow cell up to twenty billion bases of sequence data can be produced.
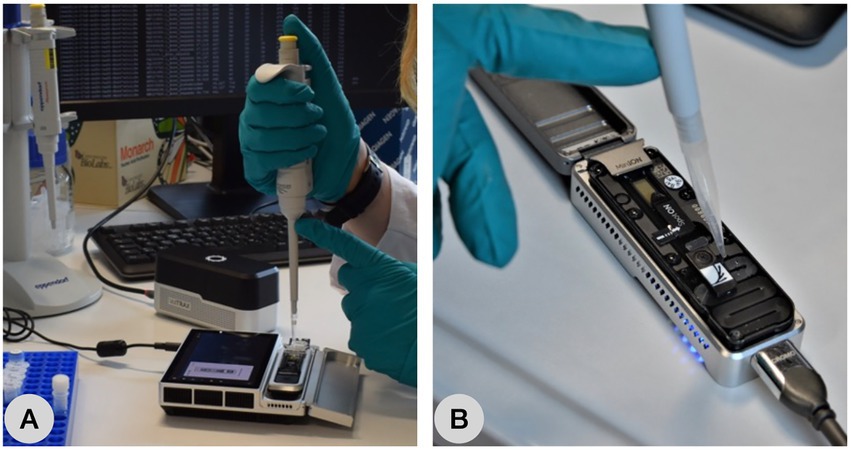
Figure 5. Oxford Nanopore Technologies Sequencing Platforms. (A) Loading the prepared library before nanopore sequencing on a Flongle plugged in the stand-alone device MinION Mk1c and (B) loading the SpotOn Flowcell plugged in the MinION Mk1b connected to a computer via USB3 port.
3.4.3. Portability
Prospectively, the MinION can be used to perform sequencing in the field or areas without laboratory infrastructure (Krehenwinkel et al., 2019). As the MinION sequencer can be powered via USB, it is a useful tool for sequencing projects in field or areas without proper laboratory equipment (van Dijk et al., 2018). With its stand-alone pendant, i.e., the MinION Mk1c, no computer is needed for sequencing as the device performs base calling as well (Figure 5). Since environmental DNA studies become increasingly popular, miniature portable laboratory equipment such as miniaturized thermocyclers or battery powered gel electrophoresis devices are available. ONT offers a customized, portable lab-on-the-chip called VolTRAX for automated library preparation. Thus, with ONT devices, DNA metabarcoding studies under field (Johnson et al., 2017; Krehenwinkel et al., 2019; Maestri et al., 2019; Raymond-Bouchard et al., 2022) and even space (Castro-Wallace et al., 2017) conditions with minimal lab equipment are possible.
3.4.4. Error rate
Despite all advantages such as long-read sequencing and portability, MinION-based nanopore sequencing reads still show a comparatively high error rate. While the quality score of typical NGS techniques and PacBio are usually above 30 (99.9% base call accuracy), ONT reads show currently a quality score around 15–20 (96.8%–99% accuracy, respectively). However, when the MinION was first introduced in 2014, the accuracy of the generated reads was below 60% (Rang et al., 2018). Therefore, the technology still has a bad reputation. Together with a possible PCR bias, it limited the applicability of nanopore sequencing on metabarcoding of mixed samples (Rang et al., 2018; Maestri et al., 2019). However, if a specific reference database is applied and the MinION-specific error model (Krishnakumar et al., 2018) is considered during bioinformatic data processing (see below), MinION is well suited for metabarcoding (Krehenwinkel et al., 2019; Leidenfrost et al., 2020; Baloğlu et al., 2021). Furthermore, the read quality is continuously improving with every release of a new ONT library preparation kit and nanopore design.
3.4.5. Library preparation
The main objective of library preparation is the fragmentation of the sample DNA and attachment of the motor protein. With the ONT Rapid Sequencing Kit (SQK-RAD004) this is done in one step and library preparation requires 10 min and 400 ng of DNA. The price per sample is around 575 US$. By multiplexing, several separate DNA samples can be sequenced simultaneously at one flow cell. The ONT Rapid Barcoding Kit (SQK-RBK004) allows the attachment of multiplexing barcodes to up to twelve individual samples, which reduces the price per sample to 54 US$. The kit requires 400 ng genomic DNA as starting material, too. Hence, the sequence depth is reduced by a factor of twelve. For plant barcode sequencing from pollen samples this suffices (Leidenfrost et al., 2020). Depending on how many samples are to be processed at the same time (and how experienced the laboratory technician is), the laboratory work of sequencing library preparation takes approximately three to six hours. During the library preparation protocol, molarity calculations have to be carried out to proceed with the appropriate amount of DNA. The NEBioCalculator is a convient free online tool (NEBioCalculator, 2021). As mention, for accurate DNA quantification a Qubit fluorometer (Thermo Fisher Scientific Inc.) should be used.
It should be noted that ONT allows for two sequencing strategies: With the 1D approach, only one strand of the template DNA is sequenced. In contrast, with the 1D2 library preparation chemistry, both complementary strands are sequenced and the squiggles of both strands are combined to create a higher-quality consensus read. This slightly increases read accuracy at the cost of sequencing depth (Cornelis et al., 2019).
The resulting library can then be pipetted into a flow cell to start the sequencing process. Typically, after around 10 min, the first one thousand reads are available for downstream data analysis. And after just a few hours, a usable amount of data has been produced. The activity of the pores in the flow cell as well as other parameters such as temperature, sequenced reads or the average quality score can be monitored in real-time during sequencing.
3.5. Bioinformatics and taxonomic assignment
After working both in the field and in the lab, the final steps in molecular palynology are carried out on the computer (Figure 6). Typically, up-to-date tools lack any graphical user interface (GUI). Thus, both data handling and program executions are preferably performed in a UNIX-like command line interface, e.g., macOS Terminal, the PowerShell with a Windows Subsystem for Linux (WSL) for Windows 10 or higher, or a Linux system. It is strongly recommended to acquire the appropriate skills (Wünschiers, 2013).
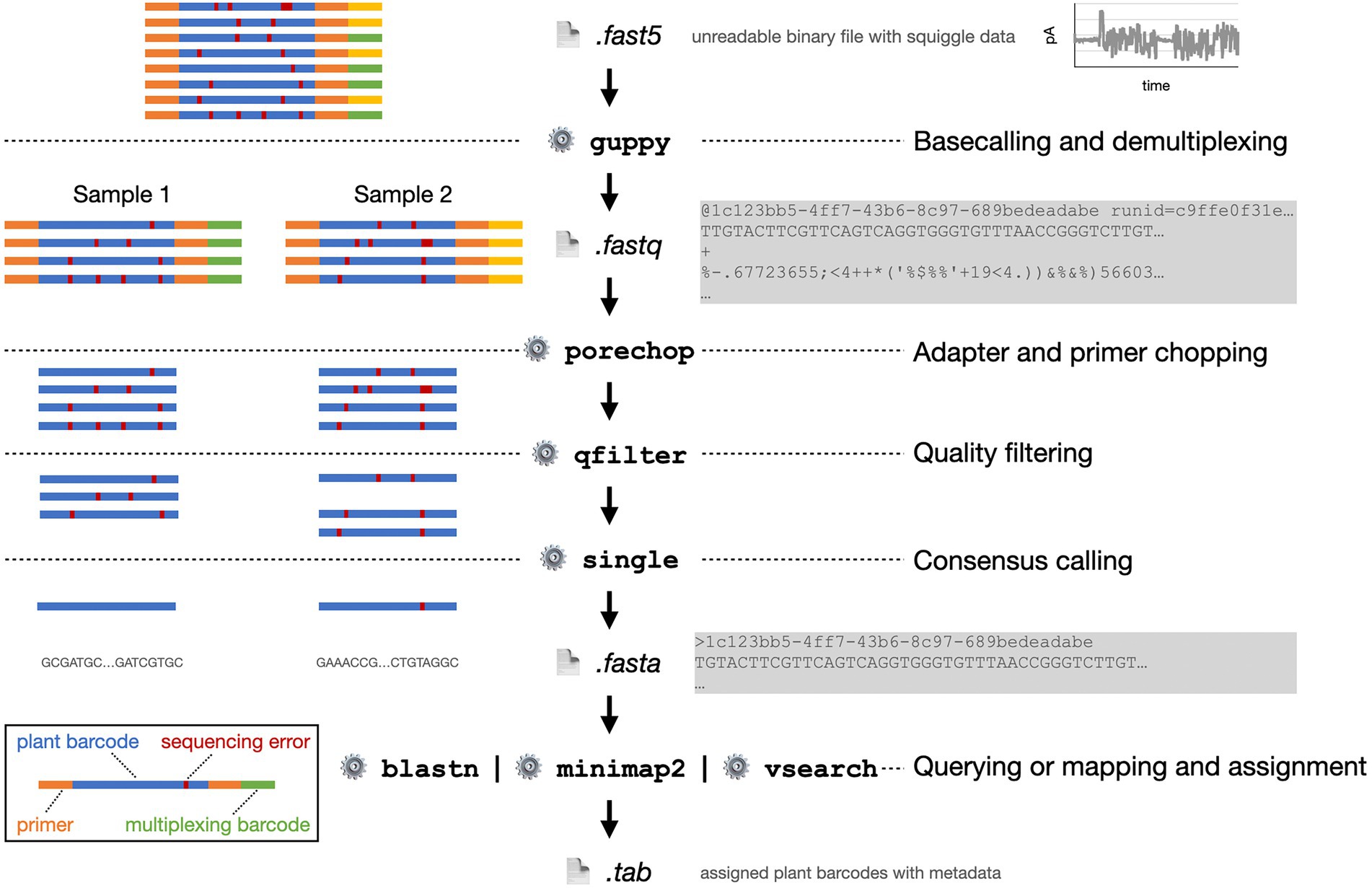
Figure 6. Exemplified bioinformatics pipeline starting with the FAST5 data file as provided by the MinION sequencer. On the left, the processing of eight plant barcode reads from two pooled, multiplexed samples is shown schematically. On the right, abbreviated file contents and software functions are shown.
ONT sequencing platforms provide all sequence run data as a binary encoded FAST5 file. FAST5 is a proprietary format developed by ONT that is derived from the Hierarchical Data Format 5 (HDF5) (The HDF Group, 2010). Most importantly, it encodes the squiggle plot data, i.e., the amperometric changes over the nanopore over time, as the DNA molecule passes through. During base calling, this data is converted into a sequence of nucleotides.
3.5.1. Base calling
The base calling process for nanopore data is rather different from base calling in other sequencing technologies. The main difference lies in the fact that not one single nucleotide but usually a pentamer determines the electric current through the nanopore. Accordingly, not four but 1,024 states have to be distinguished (Wick et al., 2019). Base calling is a very active field of development with contributions from ONT and independent research groups. ONT developed eight base caller software packages, whereof Guppy is the most prominent one (Wick et al., 2019; Kahlke, 2021; Wang et al., 2021).
Guppy does not only transform the squiggles into nucleotide reads but simultaneously removes multiplexing barcodes and adapter sequences from pre-processing, e.g., library preparation. Guppy is integrated into the MinKNOW software. However, only the standalone version is available for Linux operation systems. Base calling with Guppy can be extremely accelerated by the utilization a graphics processing unit (GPU).
3.5.2. Demultiplexing
When several samples were sequenced at the same time, the sequence data has to be demultiplexed. Thereby, the reads are assigned to their actual sample. Again, this can be carried out directly in parallel to sequencing with MinKNOW or afterwards with third-party software like Porechop (Wick, 2018) or DeepBinner (Wick et al., 2018). Unlike Porechop that requires base called FASTQ file, DeepBinner identifies barcodes from the squiggle raw signal in the FAST5 file, which gives it a greater sensitivity. When base calling is performed with Guppy, it can simultaneously be instructed to demultiplex the reads.
3.5.3. Error correction and quality filtering
Assuming that no high-quality short reads from NGS sequencing are available for error correction, one can still improve the nanopore reads based on the known error model: Nanopore reads predominantly suffer from insertions and deletions (indels) in homopolymers (Delahaye and Nicolas, 2021). Thus, several algorithmic approaches have been implemented for standalone, computational error correction (Salmela et al., 2016; Koren et al., 2017; Xiao et al., 2017; Sahlin and Medvedev, 2021).
The error rate can also be mitigated by using multiple reads for one plant barcode to establish a consensus, e.g., with the tool SINGLe (Espada et al., 2022). This consensus calling strategy reduces the read quality at the cost of sequencing depth by a factor of 30–100.
After the optional error correction, reads can be filtered by their quality score. For quality filtering we provide a simple script that may be applied and that allows the setting of different aspects, such as read length and individual nucleotide or average read quality thresholds (Wünschiers, 2022). Primer sequences from the plant barcode amplification step are trimmed afterwards. To that end, again Porechop or Cutadapt are common tools (Martin, 2011).
3.5.4. Assigning reads to taxa
Finally, pollen sequence reads are assigned to plant barcodes (Figure 7). This is usually done either by a local alignment as implemented in BLAST+ (Camacho et al., 2009) or a global aligner, e.g., the freely available VSEARCH software (Rognes et al., 2016). Prerequisite is an appropriate database (Bell et al., 2016). In the case of ITS2, the online database provided by the University of Würzburg, Germany may be used (Ankenbrand et al., 2015). Alternatively, a local customized database is created that contains all relevant barcode sequences, optimally filtered to only contain locally occurring plants to reduce the noise. The required barcode sequences can be downloaded, e.g., from NCBI GenBank. Additionally, the assigned plant species can be filtered and divided by their blooming time. This way, the reliability of the results can be increased. The barcode sequence reads can also be deconvoluted by aligning them to a custom reference using the minimap2 aligner software (Li, 2021). This sequence alignment tool is optimized to map noisy sequence reads to a reference database.
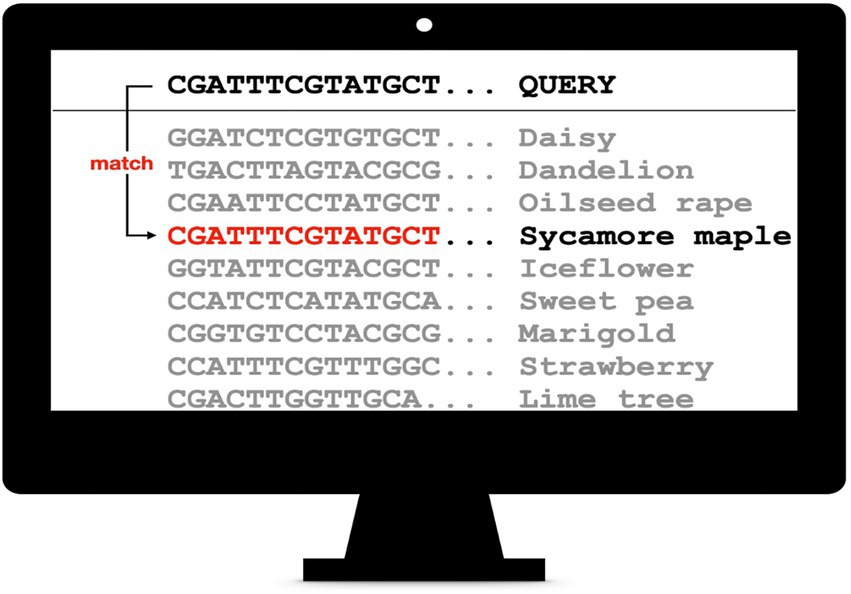
Figure 7. DNA barcode amplicon sequences are queried against a sequence database. Optimally, this database has been filtered to only include locally occurring species.
4. Outlook
What can be exprected in the future? On the one hand we see a trend towards long-read DNA sequencing technologies that will certainly enhance the usability of currently used barcodes. Likewise, it opens possibilities to use longer barcodes. Furthermore, it will help to increase the resolution at the species level. This development will be facilitated by an ever-increasing accuracy of long-reads with affordable and portable devices. Concurrently, we see a trend toward the application of “whole genome barcodes” by an approach that is called genome skimming (Dodsworth, 2015; Bell et al., 2021). In contrast to the targeted-sequencing approach of metabarcoding, shotgun metagenomics involves randomly sequencing short genomic DNA stretches from mixed samples. These can then be used for queries in genome databases. Currently, the number of sequenced plant species, as necessary for pollen identification, is limited. However, Peel et al. showed the feasibility of a reverse metagenomics approach for which they sequenced locally growing plant species with a low coverage (Peel et al., 2019). These species are represented as so-called genome skims. From these genome-wide sequence reads they created a customized sequence database that they queried with shotgun sequenced pollen DNA. They demonstrated that this reverse metagenomics approach could classify plant species present in mixed-species samples at proportions of 1% DNA or higher.
Data availability statement
The original contributions presented in the study are included in the article/supplementary material, further inquiries can be directed to the corresponding author.
Author contributions
LP, BP, and RW: conceptualization and reviewing and editing. LP: writing original draft. RW: supervision. All authors contributed to the article and approved the submitted version.
Funding
This work was funded by the Saxon State Ministry of Science, Culture and Tourism.
Conflict of interest
The authors declare that the research was conducted in the absence of any commercial or financial relationships that could be construed as a potential conflict of interest.
Publisher’s note
All claims expressed in this article are solely those of the authors and do not necessarily represent those of their affiliated organizations, or those of the publisher, the editors and the reviewers. Any product that may be evaluated in this article, or claim that may be made by its manufacturer, is not guaranteed or endorsed by the publisher.
References
Adey, A., Morrison, H. G., Asan,, Xun, X., Kitzman, J. O., Turner, E. H., et al. (2010). Rapid, low-input, low-bias construction of shotgun fragment libraries by high-density in vitro transposition. Genome Biol. 11:R119. doi: 10.1186/gb-2010-11-12-r119
Alotaibi, S. S., Sayed, S. M., Alosaimi, M., Alharthi, R., Banjar, A., Abdulqader, N., et al. (2020). Pollen molecular biology: applications in the forensic palynology and future prospects: a review. Saudi J. Biol. Sci. 27, 1185–1190. doi: 10.1016/j.sjbs.2020.02.019
Álvarez, I., and Wendel, J. F. (2003). Ribosomal ITS sequences and plant phylogenetic inference. Mol. Phylogenet. Evol. 29, 417–434. doi: 10.1016/S1055-7903(03)00208-2
Ankenbrand, M. J., Keller, A., Wolf, M., Schultz, J., and Förster, F. (2015). ITS2 database V: twice as much: Table 1. Mol. Biol. Evol. 32, 3030–3032. doi: 10.1093/molbev/msv174
Arstingstall, K. A., DeBano, S. J., Li, X., Wooster, D. E., Rowland, M. M., Burrows, S., et al. (2021). Capabilities and limitations of using DNA metabarcoding to study plant–pollinator interactions. Mol. Ecol. 30, 5266–5297. doi: 10.1111/mec.16112
Baksay, S., Pornon, A., Burrus, M., Mariette, J., Andalo, C., and Escaravage, N. (2020). Experimental quantification of pollen with DNA metabarcoding using ITS1 and trnL. Sci. Rep. 10:4202. doi: 10.1038/s41598-020-61198-6
Baldwin, B. G., Sanderson, M. J., Porter, J. M., Wojciechowski, M. F., Campbell, C. S., and Donoghue, M. J. (1995). The its region of nuclear ribosomal DNA: a valuable source of evidence on angiosperm phylogeny. Ann. Mo. Bot. Gard. 82:247. doi: 10.2307/2399880
Baloğlu, B., Chen, Z., Elbrecht, V., Braukmann, T., MacDonald, S., and Steinke, D. (2021). A workflow for accurate metabarcoding using nanopore MinION sequencing. Methods Ecol. Evol. 12, 794–804. doi: 10.1111/2041-210X.13561
Bänsch, S., Tscharntke, T., Wünschiers, R., Netter, L., Brenig, B., Gabriel, D., et al. (2020). Using ITS2 metabarcoding and microscopy to analyse shifts in pollen diets of honey bees and bumble bees along a mass-flowering crop gradient. Mol. Ecol. 29, 5003–5018. doi: 10.1111/mec.15675
Baylis, K., Lichtenberg, E. M., and Lichtenberg, E. (2021). Economics of pollination. Annu. Rev. Resour. Econ. 13, 335–354. doi: 10.1146/annurev-resource-101420-110406
Bell, K. L., de Vere, N., Keller, A., Richardson, R. T., Gous, A., Burgess, K. S., et al. (2016). Pollen DNA barcoding: current applications and future prospects. Genome 59, 629–640. doi: 10.1139/gen-2015-0200
Bell, K. L., Fowler, J., Burgess, K. S., Dobbs, E. K., Gruenewald, D., Lawley, B., et al. (2017a). Applying pollen DNA metabarcoding to the study of plant–pollinator interactions1. Appl. Plant Sci. 5:apps.1600124. doi: 10.3732/apps.1600124
Bell, K. L., Loeffler, V. M., and Brosi, B. J. (2017b). An rbcL reference library to aid in the identification of plant species mixtures by DNA Metabarcoding. Appl. Plant Sci. 5:1600110. doi: 10.3732/apps.1600110
Bell, K. L., Petit, R. A., Cutler, A., Dobbs, E. K., Macpherson, J. M., Read, T. D., et al. (2021). Comparing whole-genome shotgun sequencing and DNA metabarcoding approaches for species identification and quantification of pollen species mixtures. Ecol. Evol. 11, 16082–16098. doi: 10.1002/ece3.8281
Bell, K., Turo, K., Lowe, A., Nota, K., Keller, A., Encinas-Viso, F., et al. (2022). Plants, pollinators and their interactions under global ecological change: The role of pollen DNA metabarcoding. Molecular Ecology. doi: 10.1111/mec.16689
Biella, P., Tommasi, N., Akter, A., Guzzetti, L., Klecka, J., Sandionigi, A., et al. (2019). Foraging strategies are maintained despite workforce reduction: a multidisciplinary survey on the pollen collected by a social pollinator. PLoS One 14:e0224037. doi: 10.1371/journal.pone.0224037
Camacho, C., Coulouris, G., Avagyan, V., Ma, N., Papadopoulos, J., Bealer, K., et al. (2009). BLAST+: architecture and applications. BMC Bioinf. 10:421. doi: 10.1186/1471-2105-10-421
Carneiro de Melo Moura, C., Setyaningsih, C. A., Li, K., Merk, M. S., Schulze, S., Raffiudin, R., et al. (2022). Biomonitoring via DNA metabarcoding and light microscopy of bee pollen in rainforest transformation landscapes of Sumatra. BMC Ecol. Evo. 22:51. doi: 10.1186/s12862-022-02004-x
Castro-Wallace, S. L., Chiu, C. Y., John, K. K., Stahl, S. E., Rubins, K. H., McIntyre, A. B. R., et al. (2017). Nanopore DNA sequencing and genome assembly on the international Space Station. Sci. Rep. 7:18022. doi: 10.1038/s41598-017-18364-0
Chevalier, M., Davis, B. A. S., Heiri, O., Seppä, H., Chase, B. M., Gajewski, K., et al. (2020). Pollen-based climate reconstruction techniques for late quaternary studies. Earth Sci. Rev. 210:103384. doi: 10.1016/j.earscirev.2020.103384
Cornelis, S., Gansemans, Y., Vander Plaetsen, A.-S., Weymaere, J., Willems, S., Deforce, D., et al. (2019). Forensic tri-allelic SNP genotyping using nanopore sequencing. Forensic Sci. Int. Genet. 38, 204–210. doi: 10.1016/j.fsigen.2018.11.012
Danner, N., Keller, A., Härtel, S., and Steffan-Dewenter, I. (2017). Honey bee foraging ecology: season but not landscape diversity shapes the amount and diversity of collected pollen. PLoS One 12:e0183716. doi: 10.1371/journal.pone.0183716
de Vere, N., Jones, L. E., Gilmore, T., Moscrop, J., Lowe, A., Smith, D., et al. (2017). Using DNA metabarcoding to investigate honey bee foraging reveals limited flower use despite high floral availability. Sci. Rep. 7:42838. doi: 10.1038/srep42838
Delahaye, C., and Nicolas, J. (2021). Sequencing DNA with nanopores: troubles and biases. PLoS One 16:e0257521. doi: 10.1371/journal.pone.0257521
di Pasquale, G., Salignon, M., le Conte, Y., Belzunces, L. P., Decourtye, A., Kretzschmar, A., et al. (2013). Influence of pollen nutrition on honey bee health: do pollen quality and diversity matter? PLoS One 8:e72016. doi: 10.1371/journal.pone.0072016
Díaz, S., Settele, J., Brondízio, E. S, Ngo, H. T., Guèze, M., Agard, J., et al. (2019). Summary for policymakers of the global assessment report on biodiversity and ecosystem services of the intergovernmental science-policy platform on biodiversity and ecosystem services. IPBES Secretariat, Bonn, Germany.
Dodsworth, S. (2015). Genome skimming for next-generation biodiversity analysis. Trends Plant Sci. 20, 525–527. doi: 10.1016/j.tplants.2015.06.012
Espada, R., Zarevski, N., Dramé-Maigné, A., and Rondelez, Y. (2022). Accurate gene consensus at low nanopore coverage. GigaScience 11:giac102. doi: 10.1093/gigascience/giac102
Feng, Y., Zhang, Y., Ying, C., Wang, D., and Du, C. (2015). Nanopore-based fourth-generation DNA sequencing technology. Genomics Proteomics Bioinf. 13, 4–16. doi: 10.1016/j.gpb.2015.01.009
Fišer Pečnikar, Ž., and Buzan, E. V. (2014). 20 years since the introduction of DNA barcoding: from theory to application. J. Appl. Genet. 55, 43–52. doi: 10.1007/s13353-013-0180-y
Fragola, M., Arsieni, A., Carelli, N., Dattoli, S., Maiellaro, S., Perrone, M. R., et al. (2022). Pollen monitoring by optical microscopy and DNA Metabarcoding: comparative study and new insights. Int. J. Environ. Res. Public Health 19:2624. doi: 10.3390/ijerph19052624
Frias, B. E. D., Barbosa, C. D., and Lourenço, A. P. (2016). Pollen nutrition in honey bees (Apis mellifera): impact on adult health. Apidologie 47, 15–25. doi: 10.1007/s13592-015-0373-y
Galimberti, A., de Mattia, F., Bruni, I., Scaccabarozzi, D., Sandionigi, A., Barbuto, M., et al. (2014). A DNA barcoding approach to characterize pollen collected by honeybees. PLoS One 9:e109363. doi: 10.1371/journal.pone.0109363
Gous, A., Eardley, C. D., Johnson, S. D., Swanevelder, D. Z. H., and Willows-Munro, S. (2021). Floral hosts of leaf-cutter bees (Megachilidae) in a biodiversity hotspot revealed by pollen DNA metabarcoding of historic specimens. PLoS One 16:e0244973. doi: 10.1371/journal.pone.0244973
Hajibabaei, M., Shokralla, S., Zhou, X., Singer, G. A. C., and Baird, D. J. (2011). Environmental barcoding: a next-generation sequencing approach for biomonitoring applications using river benthos. PLoS One 6:e17497. doi: 10.1371/journal.pone.0017497
Halbritter, H., Ulrich, S., Grímsson, F., Weber, M., Zetter, R., Hesse, M., et al. (2018). Illustrated pollen terminology. Cham: Springer International Publishing.
Hawkins, J., de Vere, N., Griffith, A., Ford, C. R., Allainguillaume, J., Hegarty, M. J., et al. (2015). Using DNA Metabarcoding to identify the floral composition of honey: a new tool for investigating honey bee foraging preferences. PLoS One 10:e0134735. doi: 10.1371/journal.pone.0134735
Hebert, P. D. N., Cywinska, A., Ball, S. L., and deWaard, J. R. (2003). Biological identifications through DNA barcodes. Proc. R. Soc. Lond. B 270, 313–321. doi: 10.1098/rspb.2002.2218
Hebert, P. D. N., and Gregory, T. R. (2005). The promise of DNA barcoding for taxonomy. Syst. Biol. 54, 852–859. doi: 10.1080/10635150500354886
Hilu, K., and Liang, H. (1997). The matK gene: sequence variation and application in plant systematics. Am. J. Bot. 84, 830–839. doi: 10.2307/2445819
James, A. R. M., Geber, M. A., and Toews, D. P. L. (2022). Molecular assays of pollen use consistently reflect pollinator visitation patterns in a system of flowering plants. Mol. Ecol. Resour. 22, 361–374. doi: 10.1111/1755-0998.13468
Johnson, S. S., Zaikova, E., Goerlitz, D. S., Bai, Y., and Tighe, S. W. (2017). Real-time DNA sequencing in the Antarctic dry valleys using the Oxford Nanopore sequencer. J. Biomol. Tech. 28, 2–7. doi: 10.7171/jbt.17-2801-009
Jones, L., Brennan, G. L., Lowe, A., Creer, S., Ford, C. R., and de Vere, N. (2021). Shifts in honeybee foraging reveal historical changes in floral resources. Commun. Biol. 4, 37–10. doi: 10.1038/s42003-020-01562-4
Judd, H. J., Huntzinger, C., Ramirez, R., and Strange, J. P. (2020). A 3D printed pollen trap for bumble bee (Bombus) hive entrances. J. Vis. Exp. 161:e61500. doi: 10.3791/61500
Kahlke, T. (2021). Basecalling using guppy. Available at: https://timkahlke.github.io/LongRead_tutorials/BS_G.html (Accessed January 4, 2023).
Kamo, T., Kusumoto, Y., Tokuoka, Y., Okubo, S., Hayakawa, H., Yoshiyama, M., et al. (2018). A DNA barcoding method for identifying and quantifying the composition of pollen species collected by European honeybees, Apis mellifera (hymenoptera: Apidae). Appl. Entomol. Zool. 53, 353–361. doi: 10.1007/s13355-018-0565-9
Kegode, T. M., Bargul, J. L., Mokaya, H. O., and Lattorff, H. M. G. (2022). Phytochemical composition and bio-functional properties of Apis mellifera propolis from Kenya. R. Soc. Open Sci. 9:211214. doi: 10.1098/rsos.211214
Khan, G., Hegge, A., and Gemeinholzer, B. (2022). Development and testing of the A1 volumetric air sampler, an automatic pollen trap suitable for long-term monitoring of eDNA pollen diversity. Sensors (Basel) 22:6512. doi: 10.3390/s22176512
Knäbe, S., Mack, P., Chen, A., and Bocksch, S. (2014). Available methods for the sampling of nectar, pollen, and flowers of different plant species. Julius-Kühn-Archiv. Available at: http://oai.core.ac.uk/oai:jki:article/5330
Knot, I. E., Zouganelis, G. D., Weedall, G. D., Wich, S. A., and Rae, R. (2020). DNA barcoding of nematodes using the MinION. Front. Ecol. Evol. 8:100. doi: 10.3389/fevo.2020.00100
Koren, S., Walenz, B. P., Berlin, K., Miller, J. R., Bergman, N. H., and Phillippy, A. M. (2017). Canu: scalable and accurate long-read assembly via adaptive k -mer weighting and repeat separation. Genome Res. 27, 722–736. doi: 10.1101/gr.215087.116
Krehenwinkel, H., Pomerantz, A., and Prost, S. (2019). Genetic biomonitoring and biodiversity assessment using portable sequencing technologies: current uses and future directions. Genes 10:858. doi: 10.3390/genes10110858
Kress, W. J., and Erickson, D. L. (2007). A two-locus global DNA barcode for land plants: the coding rbcL gene complements the non-coding trnH-psbA spacer region. PLoS One 2:e508. doi: 10.1371/journal.pone.0000508
Kress, W. J., García-Robledo, C., Uriarte, M., and Erickson, D. L. (2015). DNA barcodes for ecology, evolution, and conservation. Trends Ecol. Evol. 30, 25–35. doi: 10.1016/j.tree.2014.10.008
Krishnakumar, R., Sinha, A., Bird, S. W., Jayamohan, H., Edwards, H. S., Schoeniger, J. S., et al. (2018). Systematic and stochastic influences on the performance of the MinION nanopore sequencer across a range of nucleotide bias. Sci. Rep. 8:3159. doi: 10.1038/s41598-018-21484-w
Lamb, P. D., Hunter, E., Pinnegar, J. K., Creer, S., Davies, R. G., and Taylor, M. I. (2019). How quantitative is metabarcoding: a meta-analytical approach. Mol. Ecol. 28, 420–430. doi: 10.1111/mec.14920
Lechowicz, K., Wrońska-Pilarek, D., Bocianowski, J., and Maliński, T. (2020). Pollen morphology of polish species from the genus Rubus L. (Rosaceae) and its systematic importance. PLoS One 15:e0221607. doi: 10.1371/journal.pone.0221607
Leidenfrost, R. M., Bänsch, S., Prudnikow, L., Brenig, B., Westphal, C., and Wünschiers, R. (2020). Analyzing the dietary diary of bumble bee. Front. Plant Sci. 11:287. doi: 10.3389/fpls.2020.00287
Lennartz, C., Kurucar, J., Coppola, S., Crager, J., Bobrow, J., Bortolin, L., et al. (2021). Geographic source estimation using airborne plant environmental DNA in dust. Sci. Rep. 11:16238. doi: 10.1038/s41598-021-95702-3
Leontidou, K., Vokou, D., Sandionigi, A., Bruno, A., Lazarina, M., De Groeve, J., et al. (2021). Plant biodiversity assessment through pollen DNA metabarcoding in Natura 2000 habitats (Italian Alps). Sci. Rep. 11:18226. doi: 10.1038/s41598-021-97619-3
Li, H. (2021). New strategies to improve minimap2 alignment accuracy. Bioinformatics 37, 4572–4574. doi: 10.1093/bioinformatics/btab705
Liu, S., Lang, D., Meng, G., Hu, J., Tang, M., and Zhou, X. (2022). Tracing the origin of honey products based on metagenomics and machine learning. Food Chem. 371:131066. doi: 10.1016/j.foodchem.2021.131066
Lowe, A., Jones, L., Witter, L., Creer, S., and de Vere, N. (2022). Using DNA Metabarcoding to identify floral visitation by pollinators. Diversity 14:236. doi: 10.3390/d14040236
Maestri, S., Cosentino, E., Paterno, M., Freitag, H., Garces, J. M., Marcolungo, L., et al. (2019). A rapid and accurate MinION-based workflow for tracking species biodiversity in the field. Genes (Basel) 10:468. doi: 10.3390/genes10060468
Martin, M. (2011). Cutadapt removes adapter sequences from high-throughput sequencing reads. EMBnet.journal 17, 10–12. doi: 10.14806/ej.17.1.200
Namin, S. M., Son, M., and Jung, C. (2022). Current methodologies in construction of plant-pollinator network with emphasize on the application of DNA metabarcoding approach. J. Ecol. Environ. 46:12. doi: 10.5141/jee.22.003
NEBioCalculator (2021). Available at: https://nebiocalculator.neb.com/ (Accessed January 10, 2023).
Newmaster, S. G., Fazekas, A. J., and Ragupathy, S. (2006). DNA barcoding in land plants: evaluation of rbcL in a multigene tiered approach. Can. J. Bot. 84, 335–341. doi: 10.1139/b06-047
Nürnberger, F., Keller, A., Härtel, S., and Steffan-Dewenter, I. (2019). Honey bee waggle dance communication increases diversity of pollen diets in intensively managed agricultural landscapes. Mol. Ecol. 28, 3602–3611. doi: 10.1111/mec.15156
Oliver, A. E., Newbold, L. K., Gweon, H. S., Read, D. S., Woodcock, B. A., and Pywell, R. F. (2021). Integration of DNA extraction, metabarcoding and an informatics pipeline to underpin a national citizen science honey monitoring scheme. MethodsX 8:101303. doi: 10.1016/j.mex.2021.101303
Pang, X., Liu, C., Shi, L., Liu, R., Liang, D., Li, H., et al. (2012). Utility of the trnH–psbA Intergenic spacer region and its combinations as plant DNA barcodes: a meta-analysis. PLoS One 7:e48833. doi: 10.1371/journal.pone.0048833
Parreño, M. A., Alaux, C., Brunet, J.-L., Buydens, L., Filipiak, M., Henry, M., et al. (2022). Critical links between biodiversity and health in wild bee conservation. Trends Ecol. Evol. 37, 309–321. doi: 10.1016/j.tree.2021.11.013
Peel, N., Dicks, L. V., Clark, M. D., Heavens, D., Percival-Alwyn, L., Cooper, C., et al. (2019). Semi-quantitative characterisation of mixed pollen samples using MinION sequencing and reverse Metagenomics (RevMet). Methods Ecol. Evol. 10, 1690–1701. doi: 10.1111/2041-210X.13265
Polling, M., Sin, M., de Weger, L. A., Speksnijder, A. G. C. L., Koenders, M. J. F., de Boer, H., et al. (2022). DNA metabarcoding using nrITS2 provides highly qualitative and quantitative results for airborne pollen monitoring. Sci. Total Environ. 806:150468. doi: 10.1016/j.scitotenv.2021.150468
Pornon, A., Andalo, C., Burrus, M., and Escaravage, N. (2017). DNA metabarcoding data unveils invisible pollination networks. Sci. Rep. 7:16828. doi: 10.1038/s41598-017-16785-5
Porras-Alfaro, A., Liu, K.-L., Kuske, C. R., and Xie, G. (2014). From genus to phylum: large-subunit and internal transcribed spacer rRNA operon regions show similar classification accuracies influenced by database composition. Appl. Environ. Microbiol. 80, 829–840. doi: 10.1128/AEM.02894-13
Porto, R. G., de Almeida, R. F., Cruz-Neto, O., Tabarelli, M., Viana, B. F., Peres, C. A., et al. (2020). Pollination ecosystem services: a comprehensive review of economic values, research funding and policy actions. Food Sec. 12, 1425–1442. doi: 10.1007/s12571-020-01043-w
Potter, C., de Vere, N., Jones, L. E., Ford, C. R., Hegarty, M. J., Hodder, K. H., et al. (2019). Pollen metabarcoding reveals broad and species-specific resource use by urban bees. PeerJ 7:e5999. doi: 10.7717/peerj.5999
Rang, F. J., Kloosterman, W. P., and de Ridder, J. (2018). From squiggle to basepair: computational approaches for improving nanopore sequencing read accuracy. Genome Biol. 19:90. doi: 10.1186/s13059-018-1462-9
Raven, P. H., and Wagner, D. L. (2021). Agricultural intensification and climate change are rapidly decreasing insect biodiversity. Proc. Natl. Acad. Sci. U. S. A. 118:e2002548117. doi: 10.1073/pnas.2002548117
Raymond-Bouchard, I., Maggiori, C., Brennan, L., Altshuler, I., Manchado, J. M., Parro, V., et al. (2022). Assessment of automated nucleic acid extraction Systems in Combination with MinION sequencing as potential tools for the detection of microbial biosignatures. Astrobiology 22, 87–103. doi: 10.1089/ast.2020.2349
Reuter, J. A., Spacek, D. V., and Snyder, M. P. (2015). High-throughput sequencing technologies. Mol. Cell 58, 586–597. doi: 10.1016/j.molcel.2015.05.004
Richardson, R. T., Curtis, H. R., Matcham, E. G., Lin, C.-H., Suresh, S., Sponsler, D. B., et al. (2019). Quantitative multi-locus metabarcoding and waggle dance interpretation reveal honey bee spring foraging patterns in Midwest agroecosystems. Mol. Ecol. 28, 686–697. doi: 10.1111/mec.14975
Rivers-Moore, J., Andrieu, E., Vialatte, A., and Ouin, A. (2020). Wooded semi-natural habitats complement permanent grasslands in supporting wild bee diversity in agricultural landscapes. Insects 11:812. doi: 10.3390/insects11110812
Rognes, T., Flouri, T., Nichols, B., Quince, C., and Mahé, F. (2016). VSEARCH: a versatile open source tool for metagenomics. PeerJ 4:e2584. doi: 10.7717/peerj.2584
Ruppert, K. M., Kline, R. J., and Rahman, M. S. (2019). Past, present, and future perspectives of environmental DNA (eDNA) metabarcoding: a systematic review in methods, monitoring, and applications of global eDNA. Global Ecol. Conserv. 17:e00547. doi: 10.1016/j.gecco.2019.e00547
Sahlin, K., and Medvedev, P. (2021). Error correction enables use of Oxford Nanopore technology for reference-free transcriptome analysis. Nat. Commun. 12:2. doi: 10.1038/s41467-020-20340-8
Salmela, L., Walve, R., Rivals, E., and Ukkonen, E. (2016). Accurate self-correction of errors in long reads using de Bruijn graphs. Bioinformatics 33, 799–806. doi: 10.1093/bioinformatics/btw321
Sánchez-Bayo, F., and Wyckhuys, K. A. G. (2019). Worldwide decline of the entomofauna: a review of its drivers. Biol. Conserv. 232, 8–27. doi: 10.1016/j.biocon.2019.01.020
Seth, J. K., and Barik, T. K. (2021). DNA barcoding of the family: Leiognathidae in the water of bay of Bengal, Odisha coast, India based on 16s rRNA and COI gene sequences. Thalassas 37, 831–840. doi: 10.1007/s41208-021-00324-1
Shivanna, K. R., and Rangaswamy, N. S. (1992). “Pollen collection” in Pollen biology (Berlin, Heidelberg: Springer Berlin Heidelberg), 5–7.
Srivathsan, A., Lee, L., Katoh, K., Hartop, E., Kutty, S. N., Wong, J., et al. (2021). ONTbarcoder and MinION barcodes aid biodiversity discovery and identification by everyone, for everyone. BMC Biol. 19:217. doi: 10.1186/s12915-021-01141-x
Suchan, T., Talavera, G., Sáez, L., Ronikier, M., and Vila, R. (2019). Pollen metabarcoding as a tool for tracking long-distance insect migrations. Mol. Ecol. Resour. 19, 149–162. doi: 10.1111/1755-0998.12948
Swenson, S. J., and Gemeinholzer, B. (2021). Testing the effect of pollen exine rupture on metabarcoding with Illumina sequencing. PLoS One 16:e0245611. doi: 10.1371/journal.pone.0245611
Taberlet, P., Bonin, A., Zinger, L., and Coissac, E. (2018). Environmental DNA: for biodiversity research and monitoring. 1st ed.. Oxford, United Kingdom: Oxford University Press.
Taberlet, P., Coissac, E., Hajibabaei, M., and Rieseberg, L. H. (2012). Environmental DNA. Mol. Ecol. 21, 1789–1793. doi: 10.1111/j.1365-294X.2012.05542.x
Taberlet, P., Coissac, E., Pompanon, F., Gielly, L., Miquel, C., Valentini, A., et al. (2007). Power and limitations of the chloroplast trnL (UAA) intron for plant DNA barcoding. Nucleic Acids Res. 35:e14. doi: 10.1093/nar/gkl938
The HDF Group (2010). Hierarchical data format version 5. Available at: http://www.hdfgroup.org/HDF5
Thomsen, P. F., and Willerslev, E. (2015). Environmental DNA – an emerging tool in conservation for monitoring past and present biodiversity. Biol. Conserv. 183, 4–18. doi: 10.1016/j.biocon.2014.11.019
Tommasi, N., Biella, P., Maggioni, D., Fallati, L., Agostinetto, G., Labra, M., et al. (2022). DNA metabarcoding unveils the effects of habitat fragmentation on pollinator diversity, plant-pollinator interactions, and pollination efficiency in Maldive islands. Mol. Ecol. doi: 10.1111/mec.16537
Udy, K. L., Reininghaus, H., Scherber, C., and Tscharntke, T. (2020). Plant–pollinator interactions along an urbanization gradient from cities and villages to farmland landscapes. Ecosphere 11:e03020. doi: 10.1002/ecs2.3020
Vamosi, J. C., Gong, Y.-B., Adamowicz, S. J., and Packer, L. (2017). Forecasting pollination declines through DNA barcoding: the potential contributions of macroecological and macroevolutionary scales of inquiry. New Phytol. 214, 11–18. doi: 10.1111/nph.14356
van Dijk, E. L., Jaszczyszyn, Y., Naquin, D., and Thermes, C. (2018). The third revolution in sequencing technology. Trends Genet. 34, 666–681. doi: 10.1016/j.tig.2018.05.008
Vaudo, A. D., Biddinger, D. J., Sickel, W., Keller, A., and López-Uribe, M. M. (2020). Introduced bees (Osmia cornifrons) collect pollen from both coevolved and novel host-plant species within their family-level phylogenetic preferences. R. Soc. Open Sci. 7:200225. doi: 10.1098/rsos.200225
Voulgari-Kokota, A., Ankenbrand, M. J., Grimmer, G., Steffan-Dewenter, I., and Keller, A. (2019). Linking pollen foraging of megachilid bees to their nest bacterial microbiota. Ecol. Evol. 9, 10788–10800. doi: 10.1002/ece3.5599
Wang, X.-C., Liu, C., Huang, L., Bengtsson-Palme, J., Chen, H., Zhang, J.-H., et al. (2015). ITS1: a DNA barcode better than ITS2 in eukaryotes? Mol. Ecol. Resour. 15, 573–586. doi: 10.1111/1755-0998.12325
Wang, Y., Zhao, Y., Bollas, A., Wang, Y., and Au, K. F. (2021). Nanopore sequencing technology, bioinformatics and applications. Nat. Biotechnol. 39, 1348–1365. doi: 10.1038/s41587-021-01108-x
Wick, R. (2018). Porechop. Available at: https://github.com/rrwick/Porechop (Accessed January 4, 2023).
Wick, R. R., Judd, L. M., and Holt, K. E. (2018). Deepbinner: Demultiplexing barcoded Oxford Nanopore reads with deep convolutional neural networks. PLoS Comput. Biol. 14:e1006583. doi: 10.1371/journal.pcbi.1006583
Wick, R. R., Judd, L. M., and Holt, K. E. (2019). Performance of neural network basecalling tools for Oxford Nanopore sequencing. Genome Biol. 20:129. doi: 10.1186/s13059-019-1727-y
Wirta, H., Abrego, N., Miller, K., Roslin, T., and Vesterinen, E. (2021). DNA traces the origin of honey by identifying plants, bacteria and fungi. Sci. Rep. 11:4798. doi: 10.1038/s41598-021-84174-0
Wünschiers, R. (2013). Computational biology: A practical introduction to BioData processing and analysis with Linux, MySQL, and R. Berlin, Heidelberg: Springer Berlin Heidelberg.
Wünschiers, R. (2022). qfilter. Available at: https://github.com/awkologist/qfilter (Accessed January 6, 2023).
Xiao, C.-L., Chen, Y., Xie, S.-Q., Chen, K.-N., Wang, Y., Han, Y., et al. (2017). MECAT: fast mapping, error correction, and de novo assembly for single-molecule sequencing reads. Nat. Methods 14, 1072–1074. doi: 10.1038/nmeth.4432
Yang, Y., Zhang, J.-L., Zhou, Q., Wang, L., Huang, W., and Wang, R.-D. (2019). Effect of ultrasonic and ball-milling treatment on cell wall, nutrients, and antioxidant capacity of rose (Rosa rugosa) bee pollen, and identification of bioactive components. J. Sci. Food Agric. 99, 5350–5357. doi: 10.1002/jsfa.9774
Keywords: pollen, DNA metabarcoding, nanopore sequencing, barcode, palynology
Citation: Prudnikow L, Pannicke B and Wünschiers R (2023) A primer on pollen assignment by nanopore-based DNA sequencing. Front. Ecol. Evol. 11:1112929. doi: 10.3389/fevo.2023.1112929
Edited by:
Chuleui Jung, Andong National University, Republic of KoreaReviewed by:
Christina M. Grozinger, The Pennsylvania State University (PSU), United StatesSaeed Mohamadzade Namin, Andong National University, Republic of Korea
Copyright © 2023 Prudnikow, Pannicke and Wünschiers. This is an open-access article distributed under the terms of the Creative Commons Attribution License (CC BY). The use, distribution or reproduction in other forums is permitted, provided the original author(s) and the copyright owner(s) are credited and that the original publication in this journal is cited, in accordance with accepted academic practice. No use, distribution or reproduction is permitted which does not comply with these terms.
*Correspondence: Röbbe Wünschiers, wuenschi@hs-mittweida.de