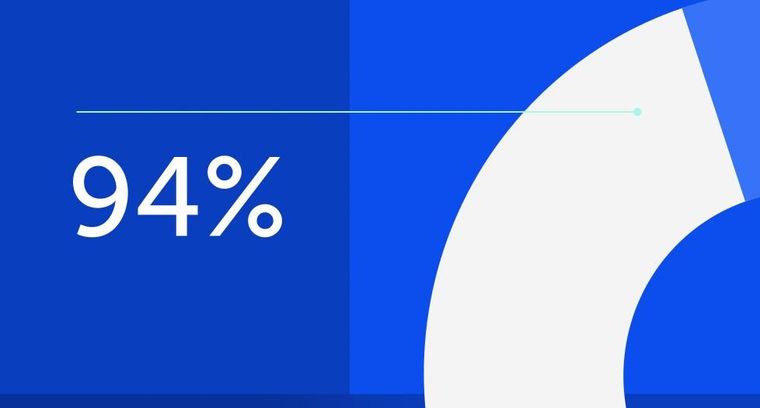
94% of researchers rate our articles as excellent or good
Learn more about the work of our research integrity team to safeguard the quality of each article we publish.
Find out more
ORIGINAL RESEARCH article
Front. Ecol. Evol., 09 February 2023
Sec. Biogeography and Macroecology
Volume 11 - 2023 | https://doi.org/10.3389/fevo.2023.1084949
This article is part of the Research TopicBarren Lives: Biodiversity and Diversification in Arid RegionsView all 5 articles
Background: Multiple components of biodiversity are excellent predictors of precipitation-induced changes in ecosystem function. However, the importance of differing scales (alpha versus beta) is usually overlooked in biodiversity–ecosystem multifunctionality studies. Consequently, little is known about how precipitation regulates the relationship between multifunctionality and multiple components of alpha and beta diversity.
Aims: We investigated geographic patterns of herbaceous plant diversity and ecosystem multifunctionality along a precipitation gradient spanning more than 2010 km in Northwest China.
Methods: We assessed the effects of herbaceous species, phylogenetic, and functional components at different scales on multifunctionality in drylands.
Results: The alpha diversity of species and functional beta diversity were key components explaining the variation in multifunctionality. As the main environmental factor, MAP (mean annual precipitation) affected multifunctionality by changing the mediating variables (i.e., species alpha and functional beta diversity). More importantly, a certain precipitation threshold was detected for the relationship of multifunctionality to species alpha and functional beta diversity. MAPs of approximately 158 mm and 140 mm modulated this relationship (shifting it from uncorrelated to significantly correlated).
Conclusions: Our findings provide insights into previously neglected mechanisms by which diversity in herbaceous layers at different scales affects ecosystem multifunctionality. It is highlighted that MAP regulates the relationship between diversity and multifunctionality in dryland ecosystems at different scales. Further, diversity may have substantial consequences for multifunctionality where MAP is higher. These empirical results provide a comprehensive understanding of the biodiversity–multifunctionality relationship in the context of precipitation, ultimately contributing to conservation and restoration programs for multifunctionality in drylands.
Ecosystem multifunctionality emphasizes the fact that ecosystems provide multiple ecosystem functions simultaneously (Loewen et al., 2020). Ongoing global change is altering biodiversity at an unprecedented rate, adversely impacting natural ecosystems in important ways (Byrnes et al., 2014). Biodiversity can be classified at different component levels as species diversity, phylogenetic diversity reflecting evolutionary history, or functional diversity conveying ecosystem functioning (Valencia et al., 2015; Richter et al., 2021). Many studies have provided extensive evidence for the positive effects of diversity on ecosystem function (Loreau et al., 2001; Hooper et al., 2005; Jing et al., 2015). Yet, it remains unclear which diversity component (i.e., species, phylogenetic, functional) is the better predictor of ecosystem function. Early studies of biodiversity and multifunctionality debated whether the effects of biodiversity upon ecosystem function primarily arose from the effects of species richness. The traditional view in which species diversity considers all species equally, but ignores differences in their evolutionary histories and roles in ecosystem functioning, hence the burgeoning interest in both phylogenetic and functional diversity (Bardgett and Van Der Putten, 2014; Nicholson et al., 2020). Many studies have found that functional diversity is a better predictor of ecosystem function than species diversity (Cadotte, 2017; Laughlin et al., 2020). For example, in the case of plants, compared with the number of species, certain functional traits (e.g., nutrient-use efficiency, leaf area, and height of plants) are more often directly or indirectly related to how species compete and use local resources (Valencia et al., 2015). However, assessing all the functional traits associated with ecosystem function is often impractical; hence, the more easily measurable phylogenetic diversity has moved to the forefront of researchers’ interest (Srivastava et al., 2012). According to some researches on plants, phylogenetic diversity has better predictive power than functional diversity, especially in large-scale or long-term studies (Flynn et al., 2011). This is because ecological niche differences between plant species provide more opportunities for their survival, achieving a higher level of ecosystem function through increased resource diversity that enables the coexistence of more species which are closely related (Cadotte et al., 2012). Although the multiple components of biodiversity are not necessarily correlated, clarifying the relationship between biodiversity and multifunctionality can help further assess the evolutionary history and loss of ecosystem function due to future species extinctions.
Indeed, diversity components can be further partitioned as alpha (diversity variation within-community) and beta diversity (diversity variation among- community) (Thompson et al., 2018). Previous studies have focused on diversity at the alpha scale, finding that alpha diversity can affect ecosystems via selection and complementary effects (Gherardi and Sala, 2015). Recently, beta scale diversity has garnered mounting attention in response to increasing biotic homogenization. High beta diversity can increase the multifunctionality of ecosystems, largely because trait variation allows for species to differentially contribute to ecosystem function (Grman et al., 2018). Not unlike alpha diversity, it is widely acknowledged that species beta diversity is insufficient for understanding the structure of species assemblages and their impact on ecosystem function. This may be due to the fact that the remaining key components of beta diversity, such as function and phylogeny, are not considered (Grman and Brudvig, 2014).
Functional beta diversity is a crucial aspect of biodiversity because it can help to disentangle community assembly processes across environmental gradients or spatial scales (Chao et al., 2019). However, comparing species and functional beta diversity alone cannot reveal the underlying patterns of ecosystem function. In terms of species beta diversity, high levels of functional beta diversity may consist of high levels of functional turnover (i.e., communities having different functional strategies) or low levels of functional turnover (i.e., communities having a subset of diverse functional strategies) (Cardoso et al., 2014; Grman et al., 2018). Similarly, phylogenetic beta diversity measures the phylogenetic distance between communities. As such, it enables us to link local processes, such as biological interactions and environmental filtering, to more regional processes, including trait evolution, dispersal and species formation (Qian et al., 2021). Although species and functional beta diversity can effectively capture the amount of overlap in species composition or trait characteristics between sites (habitats, geographic regions), neither provides information on the depth of separation of these phylogenetic through evolutionary time (Rito et al., 2021). Therefore, it is necessary to consider the impact of phylogenetic beta diversity on ecosystem functioning. Some work has demonstrated that phylogenetic beta diversity is fundamental to understanding how evolutionary and ecological factors interact to influence ecosystem function; for example, phylogenetic beta diversity plays an essential role in regulating the adverse effects of climate change on ecosystems (Mori et al., 2018). While many studies have demonstrated the indelible contribution of alpha and beta diversity to ecosystem function, their relative importance and possible synergistic effects on multiple ecosystem functions are still poorly understood. Therefore, to understand the potential impact of local as well as larger-scale biodiversity upon ecosystem functioning, examining diversity at differing scales can help to maximize the potential for conservation effectiveness for ecosystem functioning (Le Bagousse-Pinguet et al., 2019).
Water is one of the main abiotic factors limiting the structure and assembly of dryland ecosystems (Loewen et al., 2020), particularly for arid and semi-arid plant communities (Hu et al., 2022). To date, a large body of research has confirmed the importance of precipitation in dryland ecosystems (Tramblay et al., 2012; Xu et al., 2018). Notably, precipitation induces changes in a variety of ecosystem services and processes (multifunctionality). For example, precipitation increases the productivity of species by improving the availability of plant and soil nutrients (Hu et al., 2022). From a species diversity perspective, changes caused by precipitation can be explained by the fact that it indirectly influences the abundance and distribution (community composition and structure) of plants by creating local microclimates, thereby regulating ecosystem function (Adler and Levine, 2007; Xu et al., 2018). Further, it has been shown that different precipitation regimes have inconsistent effects on functional diversity (Gherardi and Sala, 2015, 2019; Petrie et al., 2018). It could be that the rapid response of functional diversity to environmental change may be specific to a more sensitive ecosystem (e.g., dryland ecosystem). Likewise, phylogenetic diversity’s role in predicting ecosystem function may be underestimated. Yet a recent study also identified a positive impact of precipitation on phylogenetic diversity (Hu et al., 2022). Furthermore, the relative impact of precipitation upon plant diversity may be a scale-dependent phenomenon (Grman et al., 2018). Although precipitation significantly influences alpha diversity, it may be more critical for beta diversity in regulating ecosystem function (Mori et al., 2018). This is because, in dryland ecosystems, precipitation may lead to a significant redistribution of resources driven by topographic factors. This differential resource allocation can, in turn, lead to fragmentation and fragmented distribution of plant communities, further affecting the tight relationship between diversity and ecosystem multifunctionality (Gherardi and Sala, 2015). Previous researchers exploring the effects of precipitation affects multifunctionality through changing diversity have mostly focused on a single component or scale of diversity. It is therefore imperative we try to integrate the alpha and beta dimensions of diversity and simultaneously explore the mediating role of multiple components of diversity (species, phylogenetic, functional).
Dryland is defined as an area with aridity index (AI) of less than 0.65 (Hu et al., 2021). Drylands store about 20% of the global carbon pool, and their net primary production (NPP) accounts for 30–35% of global NPP (Reynolds et al., 2007), which is closely associated with dryland plants. In Northwest China, the arid, semi-arid, and semi-humid arid ecosystems (i.e., drylands) found there form a continuous natural vegetation gradient, from desert to meadow grassland, together accounting for over 35% of China’s land area Su et al., 2021. Unfortunately, global climate change, which cause changing amount of precipitation and this cause changing land use and desertification (Dai, 2013). These changes may have significant impacts on biodiversity and associated ecosystem functions. Moreover, as an essential component of dryland ecosystems, herbaceous plants account for about 67% of the total dryland flora of Northwest China (Dang and Pan, 2002; Meng and Zhang, 2013). Herbaceous plants are not only essential indicator species of regional resource and environmental status (Luo et al., 2021), but they also have a unique role and special status in supporting material cycling, maintaining ecosystem function, and coping with climate change in ecologically fragile and sensitive environments (Li et al., 2021).
Recent studies of ecosystem multifunctionality in Northwest China suggest that herbaceous plant richness, which increases rapidly as drought decreases, is a critical factor limiting multifunctionality, and drives at least 30% of ecosystem function (Hu et al., 2021; Su et al., 2021). Furthermore, in situ simulation experiments revealed that a 40% increase in precipitation significantly increased species alpha diversity by 52% and multifunctionality by 20%, but plant diversity in the context of lower precipitation did not explain multifunctionality well (Hu et al., 2022). Despite this, how precipitation regulates herbaceous plant diversity (both alpha and beta) vis-à-vis multifunctionality is still unclear. To address these outstanding knowledge gaps, here we verified the importance of precipitation. Next, the contribution of herbaceous plant diversity at different scales to multifunctionality was assessed holistically, and its critical components identified. To reveal how precipitation regulates the relationship between diversity and multifunctionality, we quantified the overall relationship between multifunctionality and precipitation or diversity at different scales (critical components). Ultimately, critical thresholds for precipitation to alter the relationship between herbaceous plant diversity (critical components) and multifunctionality were determined. The following hypotheses were proposed and tested in this study:
H1: Herbaceous species alpha and functional beta diversity are critical components influencing ecosystem multifunctionality.
H2: Critical components of diversity do not interact with precipitation, but rather precipitation further regulates ecosystem multifunctionality by changing the mediating variable (i.e., diversity).
H3: Precipitation can regulate the relationship between critical components of diversity and multifunctionality (i.e., a certain threshold of precipitation exists). Critical components of diversity exert positive effects with respect to multifunctionality under conditions of higher precipitation compared with lower precipitation.
This study was conducted along a 2,100-km-long west–east transect spanning arid and semi-arid region in Northwest China, having a stark precipitation gradient ranging from 65 to 443 mm (Supplementary Figure S1). The study area encompassed six deserts—namely, the Gurbantungut, Badangilin, Ulanbuhe, Kubuchi, Mawusu, and Tengri deserts—and also entailed a large diversity of plants, vegetation types, and soil classifications. The dominant shrubs along the transect are Haloxylon ammodendron and Nitraria tangutorum, while the dominant herbs are Agriophyllum squarrosum and Stipagrostis pennata. Our study focused on herbaceous layers, for which there was significant variation in richness between vegetation types (2–14 herbaceous species per site, average = 6.36). The soil types in the study area are predominantly gray and loess, and the climate here is mostly temperate continental.
Vegetation was surveyed during the seasonal peak of plant growth (June–July 2021) according to the local phenology. With 50 sites established at 30–50 km intervals along the precipitation gradient (all sites were set up in hilly lowlands where the vegetation was in good condition and undisturbed). At each site, a 30-m × 30-m plot was set up, with five 2-m × 2-m herbaceous subplots were set up at equal distances within each plot, using a five-point sampling method (i.e., five biological replicates). Afterwards, all live plant species present within the subplots were recorded. Leaf length (LL), leaf width (LW), and plant height (H) were also measured for individuals of all species. In addition, from each plant five of its intact leaves were collected and their leaf area (LA) measured using a leaf area meter (LI-3100 area meter, LI-COR, Lincoln, NE, United States). After obtaining the dry weight (LDM) of the five leaves collected in each plant, the specific leaf area (SLA) was calculated. Finally, the herbaceous plants surveyed in the subplots were harvested and divided. After bringing them to the laboratory, they were oven-dried at 60°C for 12 h and their aboveground biomass and leaf chemical characteristics determined.
A prominent feature of drylands is the ‘fertility island’ effect due to the discontinuous distribution of plants (Su et al., 2021). To avoid a high degree of heterogeneity in soil properties, cores from a 0–10 cm depth were also taken at each subplot, also using the five-point sampling method. Once in the laboratory, the five cores were mixed to create one plot-level replicate. This procedure was replicated five times within the five subplots to generate five biological replicates. Finally, all these collected soil samples were air-dried in preparation for their soil property analysis.
For each plant randomly selected per species, a total carbon analyzer was used to determine the total carbon content of leaves. Leaf total nitrogen and total phosphorus contents were quantified by a continuous-flow ion auto-analyzer (Auto-Analyzer 3, Germany) (Lambers, 2021).
The soil organic carbon (SOC) content was determined by using the dichromate oxidation method (Urbansky, 2001). Soil total nitrogen (TN), ammoniacal nitrogen (NH4+-N) and nitrate nitrogen (NO3−-N) contents were obtained by using a continuous-flow ion auto-analyzer (Shamrikova et al., 2022). It should be noted that fresh soil samples were used for the determination of ammoniacal and nitrate nitrogen. Soil total phosphorus (TP) content was measured by the HClO4-H2SO4 ammonium molybdate-ascorbic acid method (Lambers, 2021). The molybdenum counterstain method was used to determine the soil content of activated phosphorus (AVP) and inorganic phosphorus (IP) (Gilbert et al., 2009). In addition, soil pH was measured using a pH meter (FiveEasy FE20, Switzerland) placed in a 1:2.5 (v/v) soil/water extract. Soil water content (SWC) was calculated from measured soil weight, by expressing soil moisture as a percentage of dry soil weight (Luo et al., 2021). Soil bulk density (ρb) was determined by inserting a 100-cm3 ring knife into the soil to collect it, then drying this (at 105°C–110°C, for 24 h) to obtain the dry soil weight, after which we calculated the dry soil mass per unit volume as follows: mass of the dried soil sample/volume of the ring knife. Soil temperature was measured next to each core at 0 and 10 cm depth (Fluke 51 II Thermometer; Wilmingtion, NC, United States; Oikawa et al., 2015).
Diversity at the alpha scale generally refers to the degree of diversity of species within a habitat (Valencia et al., 2015). We used species richness to indicate the species alpha diversity (species diversity is the most intuitive and commonly used measure of biodiversity and mainly determined by the number of species) (Wang et al., 2021). Species richness was calculated as the total number of species in each subplot. Functional diversity in this study was expressed as FDis (functional dispersion: the mean distance in multidimensional trait space of individual species to the centroid of all species) (Lavorel et al., 2008). To calculate FDis, the LL, LW, SLA, and H of plants were used as functional traits; these all-convent light capture and water tolerance of species (Westoby et al., 2002; Wright et al., 2004; Laliberté and Legendre, 2010). Functional diversity was calculated as species traits for each subplot. Phylogenetic diversity was related to species evolution and calculated in a similar way to species diversity. We have constructed a phylogenetic tree based on the list of plants surveyed and used MNTD (mean nearest taxon distance) to express phylogenetic alpha diversity (Webb et al., 2002; Kembel et al., 2010). Species, functional, and phylogenetic alpha diversity calculations were performed by the vegan, FD, and picante packages (Yan et al., 2020).
Beta diversity is defined as the difference in species composition between communities (Grman et al., 2018). In our study, Diversity at the beta scale was defined as the average pairwise distance of subplots to the centroid within each site, which provides consistent and unbiased estimates of beta diversity (Baselga, 2012). Species, phylogenetic, and functional beta diversity were all calculated based on pairwise-site dissimilarity methods. For species beta diversity, we calculated compositional differences in herbaceous plants based on species abundance at each site, based on the Bray–Curtis similarity index (Blomberg et al., 2003). For phylogenetic beta diversity, we calculated pairwise distances between pairs of loci based on phylogenetic trees for all sites. Finally, functional beta diversity was calculated based on Euclidean distances for the four plant traits (Yan et al., 2020). For species, functional and phylogenetic beta diversity, we used the “beta.pair”, “phylo.beta.pair” and “func.beta.pair” functions in the betapart package to calculate (Baselga, 2012).
Ecosystem multifunctionality is a composite measure of an ecosystem’s ability to simultaneously maintain multiple functions. Eleven functions, grouped into three functional categories C-cycling (SOC: soil organic carbon content; CWM.C: community-weighted mean of leaf carbon content; AGB: aboveground biomass), N-cycling (NH4+-N: soil ammoniacal nitrogen; NO3−-N: soil nitrate nitrogen; TN: soil total nitrogen; CWM.N: community weighted mean of leaf nitrogen content) and P-cycling (TP: soil total phosphorus; IP: soil inorganic phosphorus; CWM.P: community weighted mean of leaf phosphorus content; AVP: soil activated phosphorus), were used for this measure’s calculation (Supplementary Table S1). To obtain the multifunctional index (EMF), we applied the methodology of Maestre et al. (2012). First, all sites were normalized (Z-scores) for the predictor variables and weighted according to each subplot. Next, the Z-scores of all variables were averaged to obtain the EMF. This index indicates the ability of each site to maintain high levels of multiple ecosystem processes simultaneously (Valencia et al., 2015). Because this method is statistically robust, it is being increasingly used in studies assessing multifunctionality (Byrnes et al., 2014; Valencia et al., 2015; Hu et al., 2021). Further, to avoid redundancy between variables, we also examined the correlations between predictor variables: of the 66 bivariate correlations assessed in our dataset, just one had an r-value > 0.6, indicating that redundancy was not very high in our data set (Supplementary Table S1).
In terms of climate, mean precipitation of the coldest quarter (MPC), mean precipitation of the warmest quarter (MPW), mean temperature of the coldest quarter (MTC), and mean temperature of the warmest quarter (MTW), along with mean annual precipitation (MAP), temperature (MAT), and solar radiation (SO-R), were all obtained from the World Climate Database1 at a resolution of 30 arc minutes per sampled site (Hu et al., 2021). The aridity level of each site was calculated as AI (= ratio of precipitation to potential evapotranspiration), using data obtained from the Global Drought Index and Potential Evapotranspiration Climate Database2. For soil variables, we used SWC and ρb in addition to soil temperature (ST) and pH. Furthermore, longitude, latitude, and elevation were recorded for every plot along the transect, and applied as spatial variables in the analysis. These indicators (environmental variables) play an important role in the availability of dryland ecosystem functions.
We standardized all variables by Z-cores and ran a correlation coefficient (Pearson’s r) analysis to examine multiple covariates for diversity, environment, and multifunctionality. When a pair of candidate variables had a correlation coefficient > 0.6, one of the two variables was excluded to avoid high collinearity. At our study sites, the correlation coefficients as diversity indicators were not high; hence, these indicators of herbaceous plant diversity could be directly used in subsequent analyzes. Among the environmental variables, we excluded MPW, MTC, longitude, latitude, pH, soil temperature, and drought index (AI) (Supplementary Table S2). For indicators related to dryland multifunctionality, we excluded inorganic phosphorus (IP) (Supplementary Table S1).
To test the first hypothesis (H1), we fitted multiple regression models to environmental variables using the ‘vegan’ package for R (4.0.4), this was used to determine the importance of precipitation for multifunctionality (Supplementary Table S3). Then, the relationship of multiple components of diversity at different scales (alpha and beta) to multifunctionality was analyzed using a general linear model (Supplementary Figure S2; Wang et al., 2021). To further identify critical components affecting multifunctionality, a linear mixed effects model (LMM) was constructed using the ‘lme4’ package in combination with environmental variables (soil type was used as a random effect and REML was used to obtain variance components (Equations 1 and 2; Supplementary Table S4). The models were also subjected to a selection procedure based on ΔAIC < 2 (Le Bagousse-Pinguet et al., 2019), and the final models (Equations 3 and 4) were distinguished according to their ΔAIC (Akaike Information Criterion; Supplementary Figure S3; Supplementary Table S4). Finally, relative weights were calculated based on the R2-value for each predictor variable. Note that in equations 1 to 4, the model excludes the interaction term to avoid the effect of other confounding effects. The aim is simply to identify the critical biotic and abiotic factors influencing multifunctionality.
We further simplified the equations to test the second hypothesis (H2) of this study. Focusing only on the relationship between MAP or critical components of herbaceous plant diversity (species alpha and functional beta diversity) and multifunctionality (Equations 5 and 6; Supplementary Table S4). We did this because excluding additional biotic and abiotic factors does not change the main results (i.e., the difference between the two models’ ΔAIC is <2; Supplementary Figure S4; Hu et al., 2022). Therefore, we used the simplest model to test our hypothesis more clearly. Herbaceous plant diversity (species alpha and functional beta diversity), MAP and multifunctionality were first regressed using generalized additive models (GAMs) (Khoda Bakhshi and Ahmed, 2022) to test for an interaction effect between diversity and MAP on multifunctionality. To assess whether species alpha and functional beta diversity mediated the indirect relationship between precipitation and multifunctionality (Equation 5, Equation 6; Supplementary Table S4), we used the ‘mediation’ package to conduct a mediator model analysis (Rijnhart et al., 2021). If the independent variable MAP affects the dependent variable multifunctionality via herbaceous plant diversity (species alpha and functional beta diversity), then diversity is deemed a mediator variable between MAP and multifunctionality. We implemented Equations 7 and 8 (Supplementary Table S4) to establish regression relationships for the ending variable, the mediating variable, and the independent variable. Further validation was performed using a sensitivity analysis applied to the results of the mediating effect analysis. If the Rho (sensitivity) value is greater than zero in absolute value, the mediating effect is considered reliable.
Finally, to test the third hypothesis (H3), we used a rolling window model (Hu et al., 2021) to detect thresholds for changes in the relationship between species alpha or functional beta diversity and multifunctionality as the MAP changes. The presence of such a MAP threshold would mean that once a certain level of MAP is reached, a given variable either abruptly changes its value (i.e., a discontinuous threshold) or abruptly changes its relationship with MAP (i.e., a continuous threshold) (Berdugo et al., 2020). Given that in this study we only consider thresholds for shifts in the relationship between diversity and multifunctionality, only regressions with continuous thresholds (i.e., slope change at a given MAP level) were analyzed here (Hu et al., 2021). In addition, we should note the following: (1) the data were sorted according to the target gradient variable (MAP), generally in order from smallest to largest values; (2) there should not be too many MAP gradients spanned by each rolling window, otherwise it may not be possible to find an exact threshold location. Here we continued with Equations 7 and 8 (Supplementary Table S4), for which the number of windows was defined as 20 (i.e., 20 sites along the transect), this being reasonable because it does not fully cover more than half of the threshold locations. The same number of calculations was repeated at the remaining locations (i.e., 30 sites along the transect) to determine the MAP threshold for the change in a diversity factor. Finally, based on this determined MAP level, a segmented function fit was used to assess the accuracy of the shift in either the species alpha or functional beta diversity with respect to multifunctionality. To fit and run the rolling window model, we used the “tidyverse”, “piecewiseSEM”, “parallel”, and “doSNOW” packages in R.
Across the transect, except for phylogenetic diversity, both species and functional components of different diversity scales (alpha and beta) had a significant positive effect on multifunctionality (Supplementary Figure S2). Furthermore, the relationship between diversity at different scales and multifunctionality progressively increased with an increasing MAP (Supplementary Figure S2).
When climate, soil, and geographic variables were considered, the results from multiple regression models confirmed MAP as the main abiotic factor influencing changes in multifunctionality. At the same time, based on the relative weights of the predictor variables, alpha and beta diversity explained 56.29 and 86.46% of the variation in multifunctionality. The contributions of species alpha and functional beta diversity were 34.76 and 46.24%. This indicates that species alpha and functional beta diversity were the best indicators for explaining changes in multifunctionality (Figure 1; Supplementary Table S5).
Figure 1. Standardized regression coefficients and associated 95% confidence intervals for the multifunctional model predictor variables. The left insets are relative importance of variables explained by the model. The right insets represent the standardized regression coefficients. See Supplementary Table S5 for the standardized regression coefficients for each predictor variable. (A) alpha diversity; (B) beta diversity; MAP, mean annual precipitation; ρb, soil bulk density; SD, species diversity; FD, functional diversity.
Whether MAP and diversity have an interaction or mediating effect can provide a deeper explanation of their relationship to ecosystem function. Models of interaction effects showed that critical diversity components at different scales (species alpha and functional beta diversity) did not interact with the effect of MAP on multifunctionality (Supplementary Figure S5). This implied MAP may unilaterally regulate herbaceous plant diversity, independently affecting multifunctionality. It was then necessary to develop a mediating model to analyze the mechanism further. According to the results of this mediated effects analysis, the direct effect of MAP on multifunctionality (ADE) was not significant, explaining only 30.10 and 23.81% of the variation. However, the indirect effects of species alpha and functional beta diversity (ACME) were significant and positive, explaining 69.90 and 76.19% of the variation in multifunctionality. In further sensitivity analyzes, the Rho (sensitivity) values for species alpha and functional beta diversity were 0.1 and 0.2 (i.e., > 0; Figure 2; Supplementary Table S6). This validates the reliability of diversity acting as a mediating variable and justified our further speculation that MAP may regulate the relationship between diversity and multifunctionality.
Figure 2. Direct and indirect effects of mean annual precipitation (MAP) and diversity at different scales on multifunctionality. The inset on the left indicates the standardized regression coefficients of the mediation model. The middle inset shows the path relationship of the mediating effects. The inset on the right shows the validation of the sensitivity analysis. See Supplementary Table S6 for the standardized regression coefficients for each predictor variable. ACME, average causal mediation effect; ADE, average direct effects; Total Effect, the sum of the average causal mediation and direct effects. (A) alpha diversity; (B) beta diversity.
Next, we performed a rolling window analysis to clarify how MAP regulates the relationship between diversity and multifunctionality. These results revealed a positive relationship between species alpha diversity and multifunctionality at around 158 mm, which became more pronounced as values for MAP increased. In addition, the correlation between functional beta diversity and multifunctionality became less negative as the MAP increased (Figure 3). This suggested that with a greater MAP, species alpha and functional beta diversity show a stronger positive correlation with multifunctionality. We went on to validate this threshold, finding that the relationship of multifunctionality to species alpha and functional beta diversity did not hold below 158 mm and 140 mm, respectively; however, above these two thresholds, a significant positive correlation was evident for each (Figure 4). These results suggested that an increase in MAP in dryland ecosystems would lead to a shift in the relationship between diversity and multifunctionality; i.e., this positive effect becomes more pronounced where MAP is higher.
Figure 3. Thresholds for shifts in diversity-functionality relationships driven by mean annual precipitation (MAP). (A) alpha diversity; (B) beta diversity.
Figure 4. Validation of the relationship between diversity and multifunctionality at different scales under mean annual precipitation (MAP) thresholds. (A) alpha diversity; (B) beta diversity.
The simultaneous effects of multiple biodiversity components at different scales on multifunctionality highlights the need to move from a single taxonomic approach to a more multidimensional view of biodiversity, which is essential for the restoration and management of dryland ecosystems (Le Bagousse-Pinguet et al., 2019). When climate, geographic and soil variables are considered simultaneously, we find that alpha and beta diversity have a strong positive impact on multifunctionality. This finding was also reported in another study (Grman et al., 2018). In dryland ecosystems, herbaceous alpha diversity may enhance multifunctionality via complementary resource use and facilitation between species. In other words, plants engage in the rational use of resources within a more limited range, and how their resources are used is also influenced by the complementarity of ecological niches (Grime, 1998; Soliveres et al., 2014). However, it is unrealistic to maintain all functions at a high level at a single site all the time, as ecosystems are inherently heterogeneous (featuring spatial differences in species richness, identity, and composition), and some functions may be mutually exclusive (Grman et al., 2018). Therefore, maintaining multiple functions requires the establishment of different local species assemblages (i.e., beta diversity) in heterogeneous environments. That is, sites with larger species pools should have higher beta diversity and closer matching of species to environmental conditions (Thompson et al., 2018).
In our study, Functional alpha and species beta diversity have a relatively lower impact on multifunctionality. This may under environmental constraints, different species can develop similar drought-tolerant traits over long periods of evolution, resulting in a degree of functional redundancy (Mori et al., 2018; Hu et al., 2021). Similarly, herbaceous plants growing in different locations can have difficulty tolerating local environmental stresses, leading to more ecotypes of individuals (conspecifics) and thus species redundancy in the studied region. For species beta diversity, although the number of species increases with increasing precipitation, the intensity of the effect of trait variation on functionality is higher than the number of species at different sites. As we hypothesized, the species alpha diversity and functional beta diversity of herbaceous plants are critical components that influence dryland ecosystem multifunctionality. Regarding species alpha diversity, species will use small amounts of precipitation to reproduce rapidly and in large numbers, thus maximizing multiple ecosystem functions (Schwinning and Sala, 2004; Levine and HilleRisLambers, 2009). Similar to our findings, Isbell et al. (2018) found that increasing the number of species enhanced ecosystem function in a grassland plant diversity experiment. Species trait variation is the main reason why beta diversity can increase ecosystem multifunctionality. This may be because functional beta diversity strongly depends upon the dominant species’ identity and traits (Gherardi and Sala, 2015). For example, with increasing precipitation gradients, dominant species recur in specific vegetation types, being relatively large individuals that exhibit a wide range of resource use and which make a significant contribution to biomass (selection effect) (Grime, 1998). In addition, recent work found that the renewal of specific traits (floral traits) in species (not necessarily dominant species) could reinforce the relationship of multifunctionality to species alpha and functional beta diversity (Grman et al., 2018; Yan et al., 2020). This evidence suggests that factors influencing multifunctionality depend not only on the combination of functions associated with the ecosystem but also on the characteristics of the biodiversity scales under assessment.
Water is an important limiting factor for soil nutrients and vegetation development in dryland ecosystems. The fact that MAP and diversity have no interactive effect on multifunctionality is consistent with our second hypothesis. Instead, MAP influences multifunctionality by regulating herbaceous plant diversity and thus multifunctionality. This could have important implications for developing management strategies for augmenting multifunctionality under mitigation of or adaptation to changes in precipitation (Pasari et al., 2013). For species alpha diversity, herbaceous plant community can potentially harbor ectopically broad competitors due to the variation in MAP. These competitors are more adept in using minor resources in resource-poor drylands (Bond and Chase, 2002; Wang and Loreau, 2016). Positive relationships occur when competitors contribute more to the ecosystem function of interest (e.g., N cycling) (Wang et al., 2021). In contrast to our results, species alpha diversity did not mediating multifunctionality in a study of Inner Mongolian grasslands in China, perhaps because resource enrichment induces a shift from one limitation to another, thus accelerating competitive exclusion and resulting in this diversity’s weaker role (Yan et al., 2020). Some research suggested that if herbaceous species in dryland plant communities have greater nutrient utilization efficiency and are more competitive in resource-rich contexts, this may drive an irreversible shift from herbaceous to shrubby communities (Miao et al., 2022). This would reduce the number of herbaceous plants, thus weakening the positive relationship between species alpha diversity and multifunctionality. However, studies in desert grasslands provide evidence that species alpha diversity is critical for predicting multifunctionality in those ecosystems (Hu et al., 2022). This stronger relationship may be due to the greater relative impact on plants per unit change in precipitation where water is generally scarce (e.g., different life types of plants in drylands), which fosters a stronger relationship between species alpha diversity and ecosystem multifunctionality. In addition, our study’s relationship between species alpha diversity and ecosystem multifunctionality may not reflect true natural levels. Due to data limitations for shrubs, focusing on herbaceous plants alone underestimates the relationship. This is because shrubs, as important group species of dryland plant communities, have a non-negligible contribution to ecosystem function, despite being far less numerous than herbaceous plants (Valencia et al., 2015; Wang et al., 2021). Many studies in recent years have concluded that more precipitation leads to an increase in functional beta diversity and thus ecosystem function (Jiang and Ma, 2015; Milici et al., 2020). Likewise, this view was confirmed in our study and could be explained by MAP-induced trait variation permitting different species to contribute to different ecosystem functions. While trait variation across species can lead to trade-offs, functional beta diversity avoids trade-offs between ecosystem functions when it spatially separates the performance of these functions (Grman and Brudvig, 2014). As suggested by Valencia et al. (2015), multiple ecosystem functioning is closely related to the functional traits of the main contributors Diaz and Cabido, 2001. In summary, an in-depth understanding of the effects of species alpha and functional beta diversity on ecosystem multifunctionality in the context of precipitation variability can help identify effective dryland management strategies.
As predicted by our third hypothesis, species alpha and functional beta diversity and multifunctionality show positive effects under higher than lower precipitation. This may be due to the fact that as MAP increases, the available resource space (or niche space) for herbaceous species increases, enhancing the potential for ecological niche complementarity between species and thus bolstering resource inputs to ecosystem function (Jiang et al., 2008; Levine and HilleRisLambers, 2009). In other words, MAP selects for plant species whose ecological niches are similar. Accordingly, environmental pressures in these areas may reduce the ability of species alpha diversity to maintain multifunctionality (Hu et al., 2022). Similar to our results, Hu et al. (2021) found that the positive relationship between species alpha diversity and multifunctionality became weaker when going from less to more arid areas in northern China. Thus, species alpha diversity can contribute to natural capital in the form of ecosystem functioning by increasing resources utilization efficiency in environments with more precipitation.
Conversely, slower resource utilization efficiency of plants may weaken the positive correlation with multifunctionality where less precipitation occurs (Grman and Brudvig, 2014; Omidipour et al., 2021). Similar to species alpha diversity, the relationship between functional beta diversity and multifunctionality was more sensitive to the response of changes in MAP. In those areas with low MAP, there was no association between functional beta diversity and multifunctionality. On the one hand, it may be the lack of shrub data, as studies restricted to herbaceous diversity may underestimate the relationship between diversity and multifunctionality. On the other hand, it may be due to negative plant–soil feedbacks. A negative correlation between plant traits and soil nutrient content was found in Mediterranean grasslands, where fast-growing species were replaced by slow-growing species (Garnier et al., 2004). In dryland ecosystems, plants use small amounts of water to rapidly complete their life cycle, which could accelerate nutrient uptake by the soil (i.e., the plant inputs nutrients to the soil through apoplastic material and dead roots) (Lavorel and Garnier, 2002; Wang et al., 2021). In tandem, the high variability of MAP at different locations can promote increased runoff in the short term (Hu et al., 2022). As a result, these effects may accelerate the loss of soil nutrients throughout the ecosystem (Garnier et al., 2004; Grman et al., 2018), while the relationship between functional beta diversity and multifunctionality is significantly reduced. Furthermore, MAP acts as an environmental filter as it increased along the gradient (transect sampled). The scope for convergence of dominant species’ functional traits (e.g., plant height) at the beta scale was diminished, enabling greater multifunctionality (Grman and Brudvig, 2014). Our study proves that the biodiversity–ecosystem multifunctionality relationship varies along the precipitation gradient. At the same time, MAP areas below 158 mm (species alpha diversity) and 140 mm (functional beta diversity) require enhanced protection and further research.
Understanding how precipitation affecting herbaceous plant diversity is related to multifunctionality is essential for maintaining the sustainable functioning of dryland ecosystem. This study provides empirical evidence that species alpha and functional beta diversity are both key factors driving multifunctionality in natural dryland ecosystems. At the same time, MAP further modulates multifunctionality by changing the mediating variables involved (i.e., species alpha and functional beta diversity). Finally, the relationship multifunctionality to species alpha and functional beta diversity and shifted from uncorrelated to positively correlated when MAP was approximately 158 mm and 140 mm. Taken together, the results highlight the need to consider multiple components of biodiversity (especially beta diversity, which needs further study) to understand that relationship better. Also, when analyzing the mechanisms underpinning ecosystem multifunctionality, it is essential to evaluate how ecosystem functioning is improved by changes in precipitation across different scales. In an era marked by a global biodiversity crisis, our findings provide a timely ecological perspective for maintaining biodiversity and optimizing ecological restoration processes that can also contribute to enhancing management and policy actions in drylands.
The original contributions presented in the study are included in the article/Supplementary material, further inquiries can be directed to the corresponding author.
XZ and YZ conceived the idea of this study and designed the experiments. HG, YT, JY, LZ, and XG conducted the field sampling. CL and YL analyzed the laboratory. HG wrote the manuscript and bioinformatics analyzes. All authors contributed critically to the drafts and gave final approval for publication.
This research was supported by the Chinese Academy of Sciences Pioneer a Project (XDA2005020402), the National Natural Science Foundation of China (U2003214) and a special project of the Chinese Ministry of Science and Technology (2019QZKK0502).
The authors declare that the research was conducted in the absence of any commercial or financial relationships that could be construed as a potential conflict of interest.
All claims expressed in this article are solely those of the authors and do not necessarily represent those of their affiliated organizations, or those of the publisher, the editors and the reviewers. Any product that may be evaluated in this article, or claim that may be made by its manufacturer, is not guaranteed or endorsed by the publisher.
The Supplementary material for this article can be found online at: https://www.frontiersin.org/articles/10.3389/fevo.2023.1084949/full#supplementary-material
Adler, P. B., and Levine, J. M. (2007). Contrasting relationships between precipitation and species richness in space and time. Oikos 116, 221–232. doi: 10.1111/j.0030-1299.2007.15327.x
Bardgett, R. D., and Van Der Putten, W. H. (2014). Belowground biodiversity and ecosystem functioning. Nature 515, 505–511. doi: 10.1038/nature13855
Baselga, A. (2012). The relationship between species replacement, dissimilarity derived from nestedness, and nestedness. Glob. Ecol. Biogeogr. 21, 1223–1232. doi: 10.1111/j.1466-8238.2011.00756.x
Berdugo, M., Delgado-Baquerizo, M., Soliveres, S., Hernández-Clemente, R., Zhao, Y., Gaitán, J. J., et al. (2020). Global ecosystem thresholds driven by aridity. Science 367, 787–790. doi: 10.1126/science.aay5958
Blomberg, S. P., Garland, T. Jr., and Ives, A. R. (2003). Testing for phylogenetic signal in comparative data: behavioral traits are more labile. Evolution 57, 717–745. doi: 10.1111/j.0014-3820.2003.tb00285.x
Bond, E. M., and Chase, J. M. (2002). Biodiversity and ecosystem functioning at local and regional spatial scales. Ecol. Lett. 5, 467–470. doi: 10.1046/j.1461-0248.2002.00350.x
Byrnes, J. E., Gamfeldt, L., Isbell, F., Lefcheck, J. S., Griffin, J. N., Hector, A., et al. (2014). Investigating the relationship between biodiversity and ecosystem multifunctionality: challenges and solutions. Methods Ecol. Evol. 5, 111–124. doi: 10.1111/2041-210X.12143
Cadotte, M. W. (2017). Functional traits explain ecosystem function through opposing mechanisms. Ecol. Lett. 20, 989–996. doi: 10.1111/ele.12796
Cadotte, M. W., Dinnage, R., and Tilman, D. (2012). Phylogenetic diversity promotes ecosystem stability. Ecology 93, S223–S233. doi: 10.1890/11-0426.1
Cardoso, P., Rigal, F., Carvalho, J. C., Fortelius, M., Borges, P. A., Podani, J., et al. (2014). Partitioning taxon, phylogenetic and functional beta diversity into replacement and richness difference components. J. Biogeogr. 41, 749–761. doi: 10.1111/jbi.12239
Chao, A., Chiu, C. H., Villéger, S., Sun, I. F., Thorn, S., Lin, Y. C., et al. (2019). An attribute-diversity approach to functional diversity, functional beta diversity, and related (dis) similarity measures. Ecol. Monogr. 89:e01343. doi: 10.1002/ecm.1343
Dai, A. (2013). Increasing drought under global warming in observations and models. Nat. Clim. Chang. 3, 52–58. doi: 10.1038/nclimate1633
Dang, R. L., and Pan, X. L. (2002). Floristic analysis of seed plant families in west-North Desert of China. Acta Botan. Boreal. Occid. Sin. 22, 24–32.
Diaz, S., and Cabido, M. (2001). Vive la différence: plant functional diversity matters to ecosystem processes. Trends Ecol. Evol. 16, 646–655. doi: 10.1016/S0169-5347(01)02283-2
Flynn, D. F., Mirotchnick, N., Jain, M., Palmer, M. I., and Naeem, S. (2011). Functional and phylogenetic diversity as predictors of biodiversity–ecosystem-function relationships. Ecology 92, 1573–1581. doi: 10.1890/10-1245.1
Garnier, E., Cortez, J., Billès, G., Navas, M. L., Roumet, C., Debussche, M., et al. (2004). Plant functional markers capture ecosystem properties during secondary succession. Ecology 85, 2630–2637. doi: 10.1890/03-0799
Gherardi, L. A., and Sala, O. E. (2015). Enhanced interannual precipitation variability increases plant functional diversity that in turn ameliorates negative impact on productivity. Ecol. Lett. 18, 1293–1300. doi: 10.1111/ele.12523
Gherardi, L. A., and Sala, O. E. (2019). Effect of interannual precipitation variability on dryland productivity: a global synthesis. Glob. Chang. Biol. 25, 269–276. doi: 10.1111/gcb.14480
Gilbert, J., Gowing, D., and Wallace, H. (2009). Available soil phosphorus in semi-natural grasslands: assessment methods and community tolerances. Biol. Conserv. 142, 1074–1083. doi: 10.1016/j.biocon.2009.01.018
Grime, J. P. (1998). Benefits of plant diversity to ecosystems: immediate, filter and founder effects. J. Ecol. 86, 902–910. doi: 10.1046/j.1365-2745.1998.00306.x
Grman, E., and Brudvig, L. A. (2014). Beta diversity among prairie restorations increases with species pool size, but not through enhanced species sorting. J. Ecol. 102, 1017–1024. doi: 10.1111/1365-2745.12267
Grman, E., Zirbel, C. R., Bassett, T., and Brudvig, L. A. (2018). Ecosystem multifunctionality increases with beta diversity in restored prairies. Oecologia 188, 837–848. doi: 10.1007/s00442-018-4248-6
Hooper, D. U., Chapin, F. S. III, Ewel, J. J., Hector, A., Inchausti, P., Lavorel, S., et al. (2005). Effects of biodiversity on ecosystem functioning: a consensus of current knowledge. Ecol. Monogr. 75, 3–35. doi: 10.1890/04-0922
Hu, Y., Li, X., Guo, A., Yue, P., Guo, X., Lv, P., et al. (2022). Species diversity is a strong predictor of ecosystem multifunctionality under altered precipitation in desert steppes. Ecol. Indic. 137:108762. doi: 10.1016/j.ecolind.2022.108762
Hu, W., Ran, J., Dong, L., Du, Q., Ji, M., Yao, S., et al. (2021). Aridity-driven shift in biodiversity–soil multifunctionality relationships. Nat. Commun. 12:5350. doi: 10.1038/s41467-021-25641-0
Isbell, F., Cowles, J., Dee, L. E., Loreau, M., Reich, P. B., Gonzalez, A., et al. (2018). Quantifying effects of biodiversity on ecosystem functioning across times and places. Ecol. Lett. 21, 763–778. doi: 10.1111/ele.12928
Jiang, Z., and Ma, K. (2015). Environmental filtering drives herb community composition and functional trait changes across an elevational gradient. Plant Ecol. Evol. 148, 301–310. doi: 10.5091/plecevo.2015.1124
Jiang, L., Pu, Z., and Nemergut, D. R. (2008). On the importance of the negative selection effect for the relationship between biodiversity and ecosystem functioning. Oikos 117, 488–493. doi: 10.1111/j.0030-1299.2008.16401.x
Jing, X., Sanders, N. J., Shi, Y., Chu, H., Classen, A. T., Zhao, K., et al. (2015). The links between ecosystem multifunctionality and above-and belowground biodiversity are mediated by climate. Nat. Commun. 6, 1–8. doi: 10.1038/ncomms9159
Kembel, S. W., Cowan, P. D., Helmus, M. R., Cornwell, W. K., Morlon, H., Ackerly, D. D., et al. (2010). Picante: R tools for integrating phylogenies and ecology. Bioinformatics 26, 1463–1464. doi: 10.1093/bioinformatics/btq166
Khoda Bakhshi, A., and Ahmed, M. M. (2022). Real-time crash prediction for a long low-traffic volume corridor using corrected-impurity importance and semi-parametric generalized additive model. J. Transp. Saf. Secur. 14, 1165–1200. doi: 10.1080/19439962.2021.1898069
Laliberté, E., and Legendre, P. (2010). A distance‐based framework for measuring functional diversity from multiple traits. Ecology 91, 299–305. doi: 10.1890/08-2244.1
Lambers, H. (2021). Phosphorus acquisition and utilization in plants. Annu. Rev. Plant Biol. 73, 17–42. doi: 10.1146/annurev-arplant-102720-125738
Laughlin, D. C., Gremer, J. R., Adler, P. B., Mitchell, R. M., and Moore, M. M. (2020). The net effect of functional traits on fitness. Trends Ecol. Evol. 35, 1037–1047. doi: 10.1016/j.tree.2020.07.010
Lavorel, S., and Garnier, É. (2002). Predicting changes in community composition and ecosystem functioning from plant traits: revisiting the holy grail. Funct. Ecol. 16, 545–556. doi: 10.1046/j.1365-2435.2002.00664.x
Lavorel, S., Grigulis, K., McIntyre, S., Williams, N. S., Garden, D., Dorrough, J., et al. (2008). Assessing functional diversity in the field–methodology matters! Funct. Ecol. 22, 134–147. doi: 10.1111/j.1365-2435.2007.01339.x
Le Bagousse-Pinguet, Y., Soliveres, S., Gross, N., Torices, R., Berdugo, M., and Maestre, F. T. (2019). Phylogenetic, functional, and taxonomic richness have both positive and negative effects on ecosystem multifunctionality. Proc. Natl. Acad. Sci. 116, 8419–8424. doi: 10.1073/pnas.1815727116
Levine, J. M., and HilleRisLambers, J. (2009). The importance of niches for the maintenance of species diversity. Nature 461, 254–257. doi: 10.1038/nature08251
Li, J., Li, Z., Dong, S., Wei, M., and Zhou, J. (2021). Spatial and temporal changes in vegetation and desertification (1982–2018) and their responses to climate change in the Ulan Buh Desert, Northwest China. Theor. Appl. Climatol. 143, 1643–1654. doi: 10.1007/s00704-021-03522-2
Loewen, C. J., Strecker, A. L., Gilbert, B., and Jackson, D. A. (2020). Climate warming moderates the impacts of introduced sportfish on multiple dimensions of prey biodiversity. Glob. Chang. Biol. 26, 4937–4951. doi: 10.1111/gcb.15225
Loreau, M., Naeem, S., Inchausti, P., Bengtsson, J., Grime, J. P., Hector, A., et al. (2001). Biodiversity and ecosystem functioning: current knowledge and future challenges. Science 294, 804–808. doi: 10.1126/science.1064088
Luo, Y., Peng, Q., Li, K., Gong, Y., Liu, Y., and Han, W. (2021). Patterns of nitrogen and phosphorus stoichiometry among leaf, stem and root of desert plants and responses to climate and soil factors in Xinjiang, China. Catena 199:105100. doi: 10.1016/j.catena.2020.105100
Maestre, F. T., Quero, J. L., Gotelli, N. J., Escudero, A., Ochoa, V., Delgado-Baquerizo, M., et al. (2012). Plant species richness and ecosystem multifunctionality in global drylands. Science 335, 214–218. doi: 10.1126/science.1215442
Meng, H. H., and Zhang, M. L. (2013). Diversification of plant species in arid Northwest China: species-level phylogeographical history of Lagochilus Bunge ex Bentham (Lamiaceae). Mol. Phylogenet. Evol. 68, 398–409. doi: 10.1016/j.ympev.2013.04.012
Miao, C., Bai, Y., Zhang, Y., She, W., Liu, L., Qiao, Y., et al. (2022). Interspecific interactions alter plant functional strategies in a revegetated shrub-dominated community in the mu Us Desert, China. Ann. Bot. 130, 149–158. doi: 10.1093/aob/mcac039
Milici, V. R., Dalui, D., Mickley, J. G., and Bagchi, R. (2020). Responses of plant–pathogen interactions to precipitation: implications for tropical tree richness in a changing world. J. Ecol. 108, 1800–1809. doi: 10.1111/1365-2745.13373
Mori, A. S., Isbell, F., and Seidl, R. (2018). β-Diversity, community assembly, and ecosystem functioning. Trends Ecol. Evol. 33, 549–564. doi: 10.1016/j.tree.2018.04.012
Nicholson, C. C., Ward, K. L., Williams, N. M., Isaacs, R., Mason, K. S., Wilson, J. K., et al. (2020). Mismatched outcomes for biodiversity and ecosystem services: testing the responses of crop pollinators and wild bee biodiversity to habitat enhancement. Ecol. Lett. 23, 326–335. doi: 10.1111/ele.13435
Oikawa, P. Y., Ge, C., Wang, J., Eberwein, J. R., Liang, L. L., Allsman, L. A., et al. (2015). Unusually high soil nitrogen oxide emissions influence air quality in a high-temperature agricultural region. Nat. Commun. 6, 8753–8710. doi: 10.1038/ncomms9753
Omidipour, R., Tahmasebi, P., Faizabadi, M. F., Faramarzi, M., and Ebrahimi, A. (2021). Does β diversity predict ecosystem productivity better than species diversity? Ecol. Indic. 122:107212. doi: 10.1016/j.ecolind.2020.107212
Pasari, J. R., Levi, T., Zavaleta, E. S., and Tilman, D. (2013). Several scales of biodiversity affect ecosystem multifunctionality. Proc. Natl. Acad. Sci. 110, 10219–10222. doi: 10.1073/pnas.1220333110
Petrie, M. D., Peters, D. P., Yao, J., Blair, J. M., Burruss, N. D., Collins, S. L., et al. (2018). Regional grassland productivity responses to precipitation during multiyear above-and below-average rainfall periods. Glob. Chang. Biol. 24, 1935–1951. doi: 10.1111/gcb.14024
Qian, H., Jin, Y., Leprieur, F., Wang, X., and Deng, T. (2021). Patterns of phylogenetic beta diversity measured at deep evolutionary histories across geographical and ecological spaces for angiosperms in China. J. Biogeogr. 48, 773–784. doi: 10.1111/jbi.14036
Reynolds, J. F., Smith, D. M. S., Lambin, E. F., Turner, B., Mortimore, M., Batterbury, S. P., et al. (2007). Global desertification: building a science for dryland development. Science 316, 847–851. doi: 10.1126/science.1131634
Richter, A., Nakamura, G., Agra Iserhard, C., and da Silva Duarte, L. (2021). The hidden side of diversity: effects of imperfect detection on multiple dimensions of biodiversity. Ecol. Evol. 11, 12508–12519. doi: 10.1002/ece3.7995
Rijnhart, J. J., Valente, M. J., MacKinnon, D. P., Twisk, J. W., and Heymans, M. W. (2021). The use of traditional and causal estimators for mediation models with a binary outcome and exposure-mediator interaction. Struct. Equ. Model. Multidiscip. J. 28, 345–355. doi: 10.1080/10705511.2020.1811709
Rito, K. F., Arroyo-Rodriguez, V., Cavender-Bares, J., Santo-Silva, E. E., Souza, G., Leal, I. R., et al. (2021). Unraveling the drivers of plant taxonomic and phylogenetic β-diversity in a human-modified tropical dry forest. Biodivers. Conserv. 30, 1049–1065. doi: 10.1007/s10531-021-02131-9
Schwinning, S., and Sala, O. E. (2004). Hierarchy of responses to resource pulses in arid and semi-arid ecosystems. Oecologia 141, 211–220. doi: 10.1007/s00442-004-1520-8
Shamrikova, E. V., Kondratenok, B. M., Tumanova, E. A., Vanchikova, E. V., Lapteva, E. M., Zonova, T. V., et al. (2022). Transferability between soil organic matter measurement methods for database harmonization. Geoderma 412:115547. doi: 10.1016/j.geoderma.2021.115547
Soliveres, S., Maestre, F. T., Eldridge, D. J., Delgado-Baquerizo, M., Quero, J. L., Bowker, M. A., et al. (2014). Plant diversity and ecosystem multifunctionality peak at intermediate levels of woody cover in global drylands. Glob. Ecol. Biogeogr. 23, 1408–1416. doi: 10.1111/geb.12215
Srivastava, D. S., Cadotte, M. W., MacDonald, A. A. M., Marushia, R. G., and Mirotchnick, N. (2012). Phylogenetic diversity and the functioning of ecosystems. Ecol. Lett. 15, 637–648. doi: 10.1111/j.1461-0248.2012.01795.x
Su, Y. G., Liu, J., Zhang, Y. M., and Huang, G. (2021). More drought leads to a greater significance of biocrusts to soil multifunctionality. Funct. Ecol. 35, 989–1000. doi: 10.1111/1365-2435.13761
Thompson, P. L., Isbell, F., Loreau, M., O'Connor, M. I., and Gonzalez, A. (2018). The strength of the biodiversity–ecosystem function relationship depends on spatial scale. Proc. R. Soc. B 285:38. doi: 10.1098/rspb.2018.0038
Tramblay, Y., Badi, W., Driouech, F., El Adlouni, S., Neppel, L., and Servat, E. (2012). Climate change impacts on extreme precipitation in Morocco. Glob. Planet. Chang. 82-83, 104–114. doi: 10.1016/j.gloplacha.2011.12.002
Urbansky, E. T. (2001). Total organic carbon analyzers as tools for measuring carbonaceous matter in natural waters. J. Environ. Monit. 3, 102–112. doi: 10.1039/b006564l
Valencia, E., Maestre, F. T., Le Bagousse-Pinguet, Y., Quero, J. L., Tamme, R., Börger, L., et al. (2015). Functional diversity enhances the resistance of ecosystem multifunctionality to aridity in Mediterranean drylands. New Phytol. 206, 660–671. doi: 10.1111/nph.13268
Wang, S., and Loreau, M. (2016). Biodiversity and ecosystem stability across scales in metacommunities. Ecol. Lett. 19, 510–518. doi: 10.1111/ele.12582
Wang, H., Lv, G., Cai, Y., Zhang, X., Jiang, L., and Yang, X. (2021). Determining the effects of biotic and abiotic factors on the ecosystem multifunctionality in a desert-oasis ecotone. Ecol. Indic. 128:107830. doi: 10.1016/j.ecolind.2021.107830
Webb, C. O., Ackerly, D. D., McPeek, M. A., and Donoghue, M. J. (2002). Phylogenies and community ecology. Annu. Rev. Ecol. Syst. 33, 475–505. doi: 10.1146/annurev.ecolsys.33.010802.150448
Westoby, M., Falster, D. S., Moles, A. T., Vesk, P. A., and Wright, I. J. (2002). Plant ecological strategies: some leading dimensions of variation between species. Annu. Rev. Ecol. Syst. 33, 125–159. doi: 10.1146/annurev.ecolsys.33.010802.150452
Wright, I. J., Reich, P. B., Westoby, M., Ackerly, D. D., Baruch, Z., Bongers, F., et al. (2004). The worldwide leaf economics spectrum. Nature 428, 821–827. doi: 10.1038/nature02403
Xu, Z., Li, M. H., Zimmermann, N. E., Li, S. P., Li, H., Ren, H., et al. (2018). Plant functional diversity modulates global environmental change effects on grassland productivity. J. Ecol. 106, 1941–1951. doi: 10.1111/1365-2745.12951
Keywords: biodiversity, ecosystem multifunctionality, herbaceous plants, alpha diversity, beta diversity, life history strategies
Citation: Guo H, Zhou X, Tao Y, Yin J, Zhang L, Guo X, Liu C, Lin Y and Zhang Y (2023) Precipitation preferences alter the relative importance of herbaceous plant diversity for multifunctionality in the drylands of China. Front. Ecol. Evol. 11:1084949. doi: 10.3389/fevo.2023.1084949
Received: 31 October 2022; Accepted: 26 January 2023;
Published: 09 February 2023.
Edited by:
Fernando Faria Franco, Federal University of São Carlos, BrazilReviewed by:
Weiwei She, Beijing Forestry University, ChinaCopyright © 2023 Guo, Zhou, Tao, Yin, Zhang, Guo, Liu, Lin and Zhang. This is an open-access article distributed under the terms of the Creative Commons Attribution License (CC BY). The use, distribution or reproduction in other forums is permitted, provided the original author(s) and the copyright owner(s) are credited and that the original publication in this journal is cited, in accordance with accepted academic practice. No use, distribution or reproduction is permitted which does not comply with these terms.
*Correspondence: Yuanming Zhang, ✉ eW16aGFuZ0Btcy54amIuYWMuY24=
Disclaimer: All claims expressed in this article are solely those of the authors and do not necessarily represent those of their affiliated organizations, or those of the publisher, the editors and the reviewers. Any product that may be evaluated in this article or claim that may be made by its manufacturer is not guaranteed or endorsed by the publisher.
Research integrity at Frontiers
Learn more about the work of our research integrity team to safeguard the quality of each article we publish.