- 1College of Wildlife and Protected Area, Northeast Forestry University, Harbin, China
- 2College of Life Science, Qufu Normal University, Qufu, China
- 3Hulunbuir Academy of Inland Lakes in Northern Cold and Arid Areas, Hulunbuir, China
The harsh environment of Qinghai-Tibet Plateau (QTP) imposes strong selective stresses (e.g., hypoxia, high UV-radiation, and extreme temperature) to the native species, which have driven striking phenotypic and genetic adaptations. Although the mechanisms of high-altitude adaptation have been explored for many plateau species, how the phylogenetic background contributes to genetic adaption to high-altitude of Vulpes is largely unknown. In this study, we sequenced transcriptomic data across multiple tissues of two high-altitude Vulpes (Vulpes vulpes montana and Vulpes ferrilata) and their low-altitude relatives (Vulpes corsac and Vulpes lagopus) to search the genetic and gene expression changes caused by high-altitude environment. The results indicated that the positive selection genes (PSGs) identified by both high-altitude Vulpes are related to angiogenesis, suggesting that angiogenesis may be the result of convergent evolution of Vulpes in the face of hypoxic selection pressure. In addition, more PSGs were detected in V. ferrilata than in V. v. montana, which may be related to the longer adaptation time of V. ferrilata to plateau environment and thus more genetic changes. Besides, more PSGs associated with high-altitude adaptation were identified in V. ferrilata compared with V. v. montana, indicating that the longer the adaptation time to the high-altitude environment, the more genetic alterations of the species. Furthermore, the result of expression profiles revealed a tissue-specific pattern between Vulpes. We also observed that differential expressed genes in the high-altitude group exhibited species-specific expression patterns, revealed a convergent expression pattern of Vulpes in high-altitude environment. In general, our research provides a valuable transcriptomic resource for further studies, and expands our understanding of high-altitude adaptation within a phylogenetic context.
Introduction
As the highest-elevation plateau on Earth, high ultraviolet radiation, thermal extremes, and oxidative stress of the Qinghai-Tibet Plateau (QTP) pose significant challenges to the survival of native species (Ge et al., 2013). The harsh environmental conditions have led to various adaptive responses in a variety of species (Li et al., 2018). Current studies on plateau adaptation of native species have included many species, such as the Tibetan loach (Yang et al., 2019), Himalayan marmot (Bai et al., 2019), the Tibetan locust (Ding et al., 2018), snub-nosed monkey (Yu et al., 2016), yak (Qiu et al., 2012; Lan et al., 2021), freshwater snails (Vinarski et al., 2021), viperine snakes (Souchet et al., 2020), and ectothermic snakes (Li et al., 2018). The mechanisms of adaptation to high-altitude might have undergone convergent evolution in some species. For example, EPAS1 gene has been found to be a positive selection signature in a variety of domestic animals on the QTP, which present a convergent genetic changes (Wu et al., 2020). Many high-altitude animals reduced O2 demand by suppressing total metabolism to compensate for a reduced cellular O2 supply as a response to hypoxia. However, the mechanisms of adaptation to high-altitude among some species might be completely different. For example, deer mice which lived on high-altitude regions have stronger thermogenic capacity to cope with the harsh environment by improving energy metabolism (Cheviron et al., 2012). Therefore, the exploration of the adaptation mechanism of different species in the plateau region is helpful to enrich our understanding of the high-altitude adaptation mechanism. Although the mechanisms of adaptation have been explored for so many plateau species, few studies have been done on plateau adaptation in Canids, limited research has focused on Canis, such as the Tibetan wolf (Zhang et al., 2014) and the Tibetan mastiff (Li et al., 2014). However, how the Vulpes adapts to the harsh local environment on the QTP remains unclear.
Vulpes vulpes montana (also named hill fox) and Vulpes ferrilata (also named Tibetan sand fox) are the two species of Vulpes distributed on the QTP, while their close relatives, Vulpes lagopus (also named arctic fox) and Vulpes corsac (also named sand fox) are lives in low-altitude regions (Imani Harsini et al., 2017; Peng et al., 2021; Lyu et al., 2022). According to previous phylogenetic relationship studies, the divergence time of V. lagopus and V. vulpes was 3.17 Ma, and the divergence time of V. ferrilata and V. corsac was 0.96 Ma (Kumar et al., 2015; Zhao et al., 2016). Despite their time scales of divergence were different, both V. v. montana and V. ferrilata were subjected to the same selection pressures and adapted to the plateau environment. Previous studies have shown that high-altitude species present a similar expression shifts or a tissue-dominated pattern, while it is unknown whether the plateau adaptation strategies of Vulpes are influenced by the phylogenetic background (Yu et al., 2016; Tang et al., 2017; Hao et al., 2019).
With the maturation of transcriptome sequencing technology, it is possible to study more deeply about gene expression patterns in different species and tissues. Previous studies have successfully analyzed the hair color development of giant pandas and the high-altitude adaptation mechanism of birds by using transcriptomics (Xiong et al., 2022; Zheng et al., 2022).
In this study, we combined transcriptome data and sequenced multiple tissues (lung, kidney, and liver) from adult individuals of Vulpes (V. lagopus, V. v. montana, V. ferrilata, and V. corsac) to conduct high- and low-altitude comparison to identify the genes associated with high-altitude adaption. Furthermore, via the comparison of the gene expression profiles in tissues, we explored whether there was a tissue-specific expression pattern in the two high-altitude Vulpes. This study could provide insightful understanding of how the Vulpes respond to high-altitude environment and explore whether there is convergent evolution in the face of the same selection pressure, thus enriching the knowledge of high-altitude adaptation of Vulpes.
Materials and methods
Sample collection
Different Vulpes samples were collected from different regions and years. V. ferrilata was collected from Gande County, Tibetan Autonomous Prefecture of Golog, Qinghai Province in China in 2019, V. v. montana was collected from Golmud city, Haixi Mongolian and Tibetan Autonomous Prefecture, Qinghai Province in China in 2021, and V. corsac was collected from Hailar City, Inner Mongolia Autonomous Region in China in 2020 (Figure 1A). For RNA extraction, three tissues (liver, lung, and kidney) were cut into pieces and mixed with RNAlater. Then, the processed samples were stored in an ultra-low temperature refrigerator at –80°C until further use.
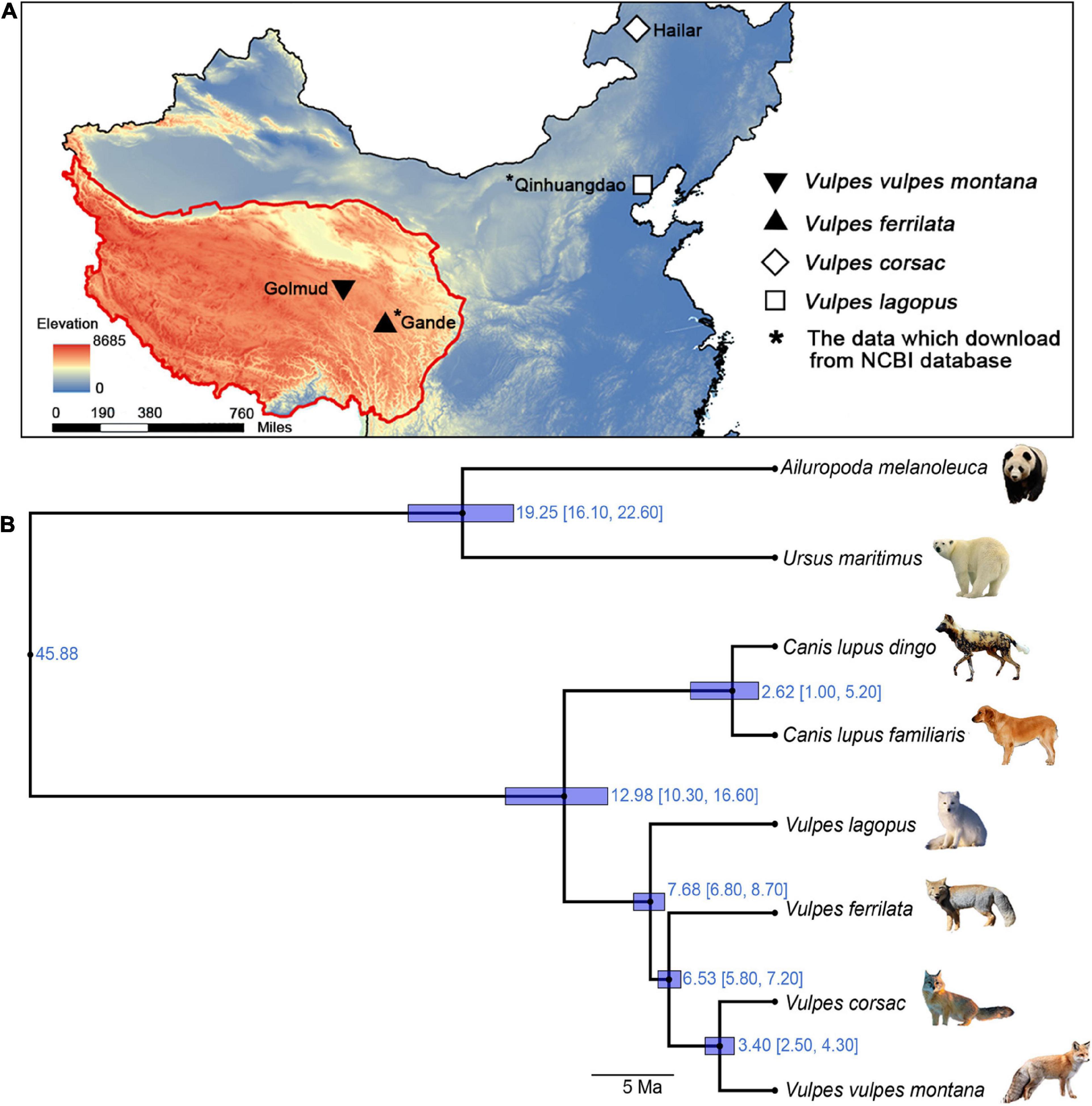
Figure 1. Localities and phylogenetic relationships of the 4 Vulpes. (A) Sampling locations. (B) Phylogenetic relationships and divergence time. Phylogenetic relationships between Vulpes (i.e., Vulpes lagopus, Vulpes vulpes montana, Vulpes ferrilata, and Vulpes corsac) and four Carnivora species (Ailuropoda melanoleuca, Ursus maritimus, Canis lupus familiaris, and Canis lupus dingo) based on one-to-one single-copy genes.
All samples were taken from individuals who died of natural causes or accidents and were taken shortly after death to ensure that RNA was not degraded. The sample collection procedures and experiments were conformed to the guidelines established by the Ethics Committee for the Care and Use of Laboratory Animals of Qufu Normal University (Permit Number: QFNU2019-012). In addition, V. lagopus transcriptome data used in this study were downloaded from the National Center for Biotechnology Information (NCBI) Sequence Read Archive (SRA) database1 (Peng et al., 2021). Transcriptome data of V. ferrilata were from our previous research with accession numbers SRR15858292, SRR15858291, and SRR15858290 (Lyu et al., 2022).
RNA extraction, library construction, and transcriptome sequencing
Total RNA was extracted on dry ice by grinding tissue (liver, lung, and kidney) in TRIzol reagent (Tiangen Biotech, China) and processed following the manufacturer’s protocol. Agilent 2100 Bioanalyzer (Agilent Technologies, USA), 0.7% agarose gel pulse (Lonza, USA) electrophoresis, and NanoDrop microspectrophotometer (Thermo Fisher Scientific, USA) were used to detected the RNA integrity, concentration, and purity, respectively. Then the Oligo (dT) 0.5X magnetic beads were used to enrich the mRNA.
The transcriptome sequencing of V. v. montana and V. corsac were performed on Illumina NovaSeq 6000 platform (Illumina, USA). Briefly, a total amount of 3 μg RNA per sample was used as input material for the RNA sample preparations. Then, NEBNext® Ultra RNA™ Library Prep Kit for Illumina® (NEB, USA) was used to generate sequencing libraries according to the guideline which provided by manufacture and index codes were added to attribute sequences to each sample. After that, a cBot Cluster Generation System of TruSeq PE Cluster Kit v3-cBot-HS (Illumina, USA) was used to the clustering of the index-coded samples. Finally, sequencing platform was used to sequence the library and generate the paired-end reads.
Transcriptome assembly and gene function annotation
Quality control of Illumina paired-ended sequenced raw data was handled by Fastp v.0.20.0 (default parameters) (Chen S. F. et al., 2018). After removing low quality reads, reads containing adapters, reads containing Poly-N, and clean data were obtained for and subsequent analysis. Trinity v2.9.0 (min_kmer_cov set to 2) was used to assemble the clean data of transcriptome (Grabherr et al., 2011). Briefly, three independent modules in Trinity v2.9.0 (i.e., Inchworm, Chrysalis and Butterfly) were used to processing the high-quality clean data. At first, reads were decomposed to construct k-mer (k = 25) dictionary, seed k-mer was selected and both sides of contig was extended to form contig. Secondly, the overlapping contigs were clustered to form components, and each component became a set of possible representations of alternative splicing isoform or homologous genes. Each component had a corresponding de Bruijn graph. Finally, the de Bruijn graph of each component was simplified to output the full-length transcript of the alternative splicing subtype, and the transcript corresponding to the paralogous gene was combed to obtain the splicing result file. After de novo assembly, the longest transcript of each gene obtained by Trinity v2.9.0 splicing were used as reference sequences for subsequent analysis.
The unigenes assembled above were used for function annotation based on seven public databases, including NCBI non-redundant protein sequences (Nr), NCBI non-redundant protein sequences (Nt), Protein family (Pfam), Clusters of Orthologous Groups of proteins, and Karyotic Ortholog Groups (KOG/COG), A manually annotated and reviewed protein sequence database (Swiss-Prot), Kyoto Encyclopedia of Genes and Genomes (KEGG), and Gene Ontology (GO). The software and parameters used to annotate the different databases are shown in Supplementary Table 1.
Identification of gene orthologous groups and phylogenetic analyses
OrthoMCL v2.0.9 (default) was used to identify homologous genes between Vulpes (i.e., V. lagopus, V. v. montana, V. ferrilata, and V. corsac) and four Carnivora species (Ailuropoda melanoleuca, Ursus maritimus, Canis lupus familiaris, and Canis lupus dingo) (Chen, 2006). Specifically, BLASTx2 and ESTScan v3.0.3 were used to extract the CDS of each putative genes and determine the direction of sequences that did not have align results, respectively (He et al., 2012). BLASTP (see text footnote 2) was used to conducting for all amino acid sequences which translated from the extracted CDSs with a cut-off e-value of 1e–5. Finally, orthologous groups were constructed from the BLASTP results with OrthoMCL v2.0.9.
The single-copy genes were further used for species phylogenetic analysis. At first, the obtained one-to-one orthologous were aligned by MUSCLE v3.8.31. After alignment, RAxML v8.2.10 (-m PROTGAMMAAUTO -p 12345 -T 8 -f b) was used for phylogenetic tree construction (Stamatakis, 2006). The estimation of divergence time was performed using MCMCTree package in PAML v4.8 (Yang, 2007). The generated tree file was displayed using FigTree v1.4.4 and MEGA v10.1.8 (Sudhir et al., 2016). In this study, we used three secondary calibration points published in previous studies as references (i.e., the most recent common ancestors of A. melanoleuca and U. maritimus, V. ferrilata and C. l. familiaris, and V. lagopus and V. vulpes were calibrated as diverged between 16.1 and 22.6, 10.13 and 16.86, and 4.5 and 10.3 Ma, respectively) (Hu et al., 2016; Peng et al., 2021; Lyu et al., 2022).
Identification of genes under positive selection and quantification of gene expression levels
In the comparisons of homologous genes, a gene with a high Ka/Ks ratio [the ratio of the number of non-synonymous substitutions per non-synonymous site (Ka) to the number of synonymous substitutions per synonymous site (Ks)] was considered to be evolving under positive selection. In this study, in order to explore the similarities and differences of adaptive mechanisms selected by V. ferrilata and V. v. montana in the face of plateau environmental pressures (e.g., high-UV radiation, extreme temperature, and low oxygen content), Codeml in PAML v4.8 was used to test the likely ratio of branching sites to determine positively selected genes (PSGs) in the high-altitude Vulpes groups (Yang, 2007). Genes with P < 0.05 were determined as PSGs. To further explore whether the tissues (liver, lung, and kidney) closely related to energy metabolism and oxygen utilization have a tissue-specific expression pattern between high-altitude Vulpes groups and their low-altitude relatives, gene expression levels were calculated using RSEM v1.3.1 (Li and Dewey, 2011). Input data for gene differential expression was the read-count data obtained in gene expression level analysis. For samples with biological replicates, we employed DESeq2 v3.11 based on a negative binomial distribution for analysis (Maza, 2016). For the research on wild animals, since the samples are extremely precious and difficult to obtain, how to ensure the statistical significance of the limited samples to the maximum extent is our key consideration. For samples without biological replicates, we first employed TMM to normalize read-count data, followed by edgeR v4.2 for differential analysis (Robinson et al., 2009). Briefly, Rlog is selected for standardization according to the sample size. We fit the input DDS objects with negative binomial distribution (fitNbinomGLMs). This step mainly uses negative binomial regression to estimate the value of regression coefficient, and finally returns the coefficient value of negative binomial distribution regression. By introducing negative binomial regression standardization, we can take advantage of the biological duplication of sequencing data to eliminate the influence of outliers to a certain extent.
Results
Sequencing data assembly, function annotation, and CDS prediction
Six transcriptome libraries (Vc1, Vc2, Vc3, Vvm1, Vvm2, and Vvm3) were generated for RNA sequencing from three tissues (i.e., 1: liver, 2: lung, 3: kidney) which play critical roles in metabolism and oxygen utilization across V. v. montana and V. corsac (Haas et al., 2013). After filtering the raw data, a total of 459,782,372 clean reads were remained for further transcriptomic assembly (Table 1).
The length of transcripts and unigene were counted, respectively, and the results are shown in Supplementary Table 2. Briefly, the numbers of unigenes for the four transcriptomic assemblies ranged from 85,094 (V. corsac) to 271,031 (V. lagopus). The mean unigene length was between 676 (V. v. montana) and 898 bp (V. lagopus), while the N50 lengths ranged from 1,108 (V. ferrilata) to 1,847 bp (V. corsac). All these assemblies together generated 638,094 unigenes with an average length of 775 bp.
After assembly, the unigenes assembled above were used for function annotation based on seven public databases (Nr, Nt, GO, PFAM, KOG, Swiss-Prot, and KO). In summary, 83,267, 60,477, 179,003, and 51,653 unigenes of V. ferrilata, V. v. montana, V. lagopus, and V. corsac have been annotated to at least one database, accounting for 45.49, 61.12, 66.04, and 60.7% of the total number of unigenes in each species, respectively. And the unigenes numbers which annotated in all databases were ranged from 4,498 (V. v. montana) to 5,195 (V. ferrilata). More details about the gene function annotation of four Vulpes are shown in Table 2.
As for CDS prediction, a total of 85,498 (V. ferrilata: 18,121, V. v. montana: 17,247, V. corsac: 16,639, V. lagopus: 33,491) CDSs and 182,088 (V. ferrilata: 79,768, V. v. montana: 43,337, V. corsac: 36,282, V. lagopus: 22,701) CDSs were obtained from the two steps, respectively (Supplementary Table 3). After that, the CDSs were further filtered to obtain the full-length CDS sequences and performed UTR prediction.
Identification of gene orthologous groups and phylogenetic analyses
OrthoMCL v2.0.9 was used to perform orthologous search analysis of the full-length CDS, and one-to-one orthologous genes were filtered for the phylogenetic analyses (Figure 1B). The phylogenetic tree topology was quite consistent with previous phylogenetic studies except V. ferrilata (Zhao et al., 2016). As shown in Figure 1B, V. v. montana and V. corsac were shown to be sister to each other, with an divergence time of 3.4 Ma (95% CI: 4.30–2.50). In addition, the divergence time between the V. ferrilata and the ancestors of V. v. montana and V. corsac, V. lagopus and the ancestors of V. ferrilata are 6.53 Ma (95% CI: 7.20–5.80) and 7.68 Ma (95% CI: 8.70–6.80), respectively.
Identification of genes under positive selection
In order to explore the similarities and differences of the adaptation mechanism of the two high-altitude Vulpes (i.e., V. ferrilata and V. v. montana), the two Vulpes were analyzed by branch site model as the foreground branch separately. Finally, 111 and 28 PSGs were identified in V. ferrilata and V. v. montana, respectively. Among these genes, 4 genes (TCF20, RASSF5, KRAS, and ZCCHC17) were identified as PSGs in both V. ferrilata and V. v. montana. To further explore the high-altitude adaptive mechanisms of the two Vulpes, we performed GO enrichment analyses for PSGs in V. ferrilata and V. v. montana, respectively (Figures 2A,B). The top 10 enriched GO terms of V. ferrilata were GTPase activity (GO:0003924, 7 genes, p = 0.001853361), GTP binding (GO:0005525, 7 genes, p = 0.006013457), vesicle-mediated transport (GO:0016192, 3 genes, p = 0.04152219), oxidoreductase activity (GO:0016491, 6 genes, p = 0.047099438), membrane (GO:0016020, 7 genes, p = 0.070771409), structural constituent of ribosome (GO:0003735, 2 genes, p = 0.118087218), ribosome (GO:0005840, 2 genes, p = 0.118087218), translation (GO:0006412, 2 genes, p = 0.14136237), integral component of membrane (GO:0016021, 7 genes, p = 0.204249059), and signal transduction (GO:0007165, 3 genes, p = 0.233284218). As for V. v. montana, the top 10 enriched GO terms were nucleus (GO:0005634, 4 genes, p = 0.093514991), translation initiation factor activity (GO:0003743, 1 gene, p = 0.098136352), magnesium ion binding (GO:0000287, 1 gene, p = 0.113562242), protein serine/threonine kinase activity (GO:0004674, 1 gene, p = 0.113562242), intracellular anatomical structure (GO:0005622, 2 genes, p = 0.118067143), GTPase activator activity (GO:0005096, 1 gene, p = 0.128733548), antioxidant activity (GO:0016209, 1 gene, p = 0.128733548), glycosyltransferase activity (GO:0016757, 1 gene, p = 0.128733548), nucleic acid binding (GO:0003676, 3 gene, p = 0.132436046), and endoplasmic reticulum (GO:0005783, 1 gene, p = 0.143654319). More details of GO enrichment are shown in Supplementary Tables 4, 5.
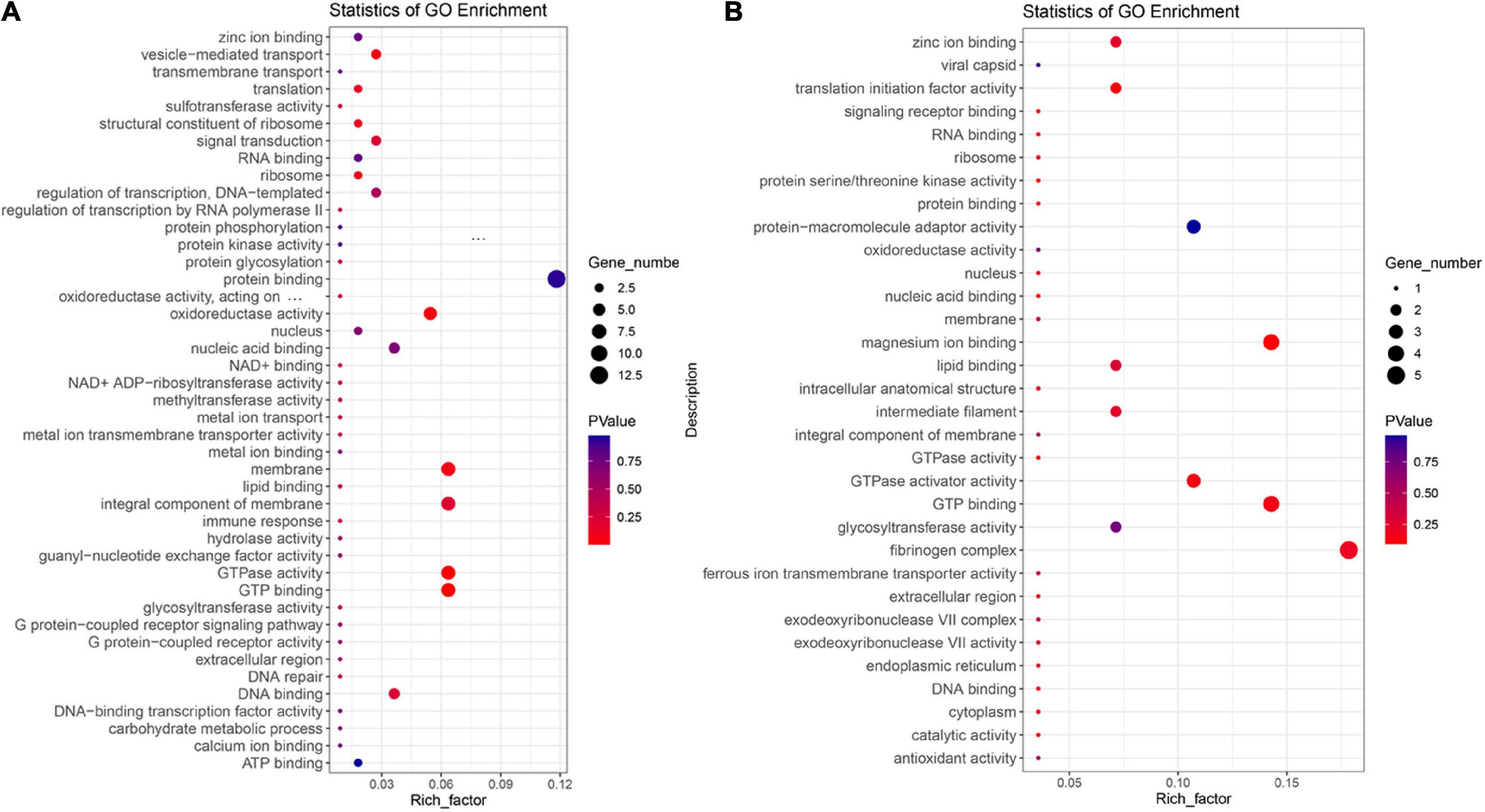
Figure 2. GO enrichment analysis for positive selection genes. (A) GO enrichment analysis for positive selection genes of Vulpes ferrilata. (B) GO enrichment analysis for positive selection genes of Vulpes vulpes montana.
Identification of the differentially expressed genes
In this section, the FPKM (expected number of Fragments Per Kilobase of transcript sequence per Millions base pairs sequenced) of each tissue (1: liver; 2: lung; 3: kidney) of Vulpes were calculated. As is shown in Figure 3, samples of the same tissue from different species clustered together except Vvm3, suggested that the Vulpes present a tissue-specific expression pattern rather than a species-specific pattern. The PCA (principal component analysis) also revealed the tissue-specific expression pattern: samples across all 4 species clustered by tissues (Supplementary Figure 1). To further explore whether there is an effect of different altitudes on gene expression levels, we performed a differential gene expression (DEGs) analysis of different altitude group of Vulpes. The result indicated that a total of 75 genes showed significantly higher expression levels (p < 0.05) in the high-altitude group compared with the low-altitude group (Figure 4A and Supplementary Table 6). In addition, the results of heat-map clustering showed that these 75 genes also showed two different expression trends in the high-altitude group (e.g., Col clustering of heat-map divided 75 genes into two groups, but there was no significant difference) (Figure 4B). We also identified DEGs in different tissues in the two different altitude groups. As shown in Figure 5, We identified 35 DEGs in the lung (27 up-regulated and 8 down-regulated in the high-altitude group), 47 DEGs in the liver (32 up-regulated and 15 down-regulated in the high-altitude group), and 40 DEGs in the kidney (27 up-regulated and 13 down-regulated in the high-altitude group).
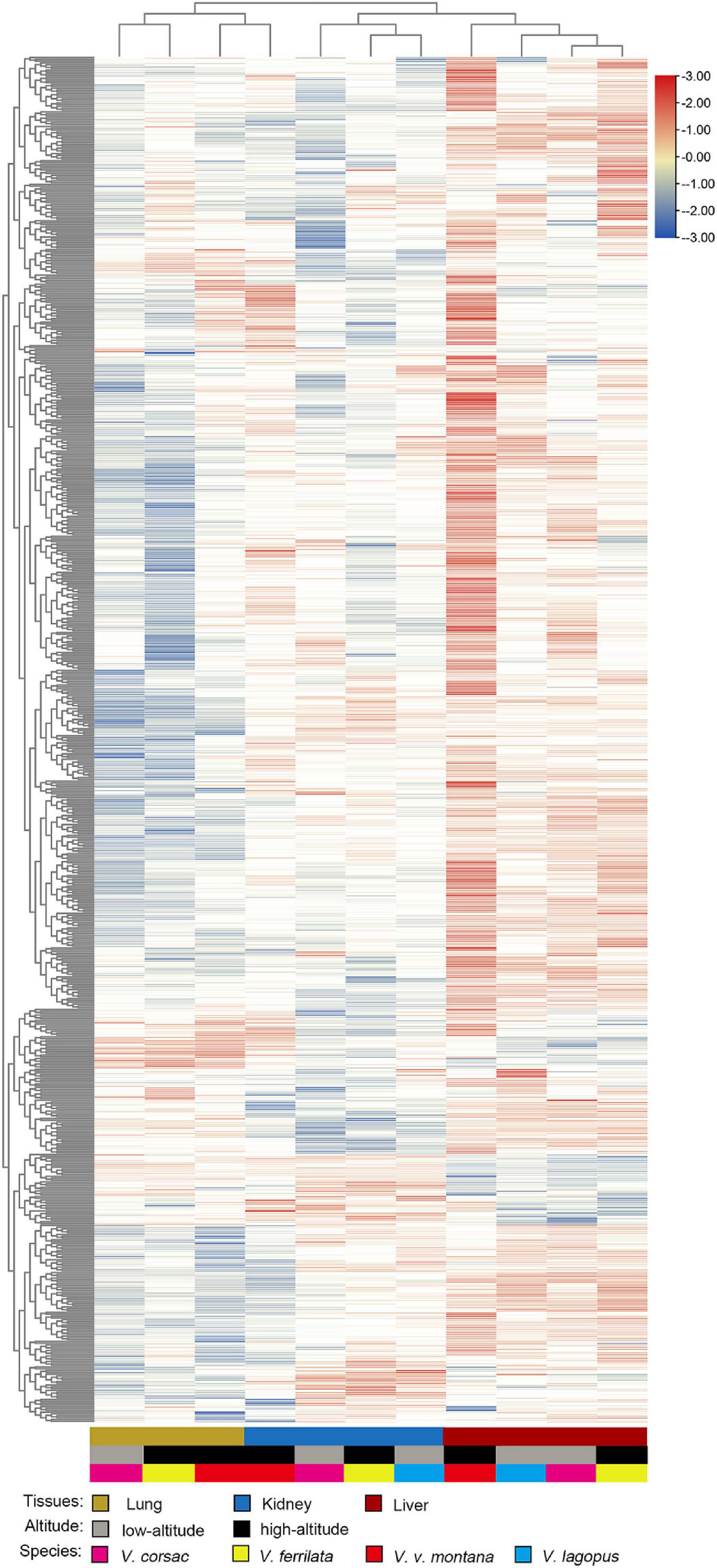
Figure 3. Heat map of genes enriched for expression in each tissue of Vulpes (i.e., Vulpes lagopus, Vulpes vulpes montana, Vulpes ferrilata, and Vulpes corsac). The heat map was generated using hierarchical clustering and complete linkage of the one-to-one orthologous genes. Distances, representing the relative similarity among genes and tissues, were calculated using Pearson’s correlation coefficients. Color represents FPKM value of gene expression after scaling and centering.
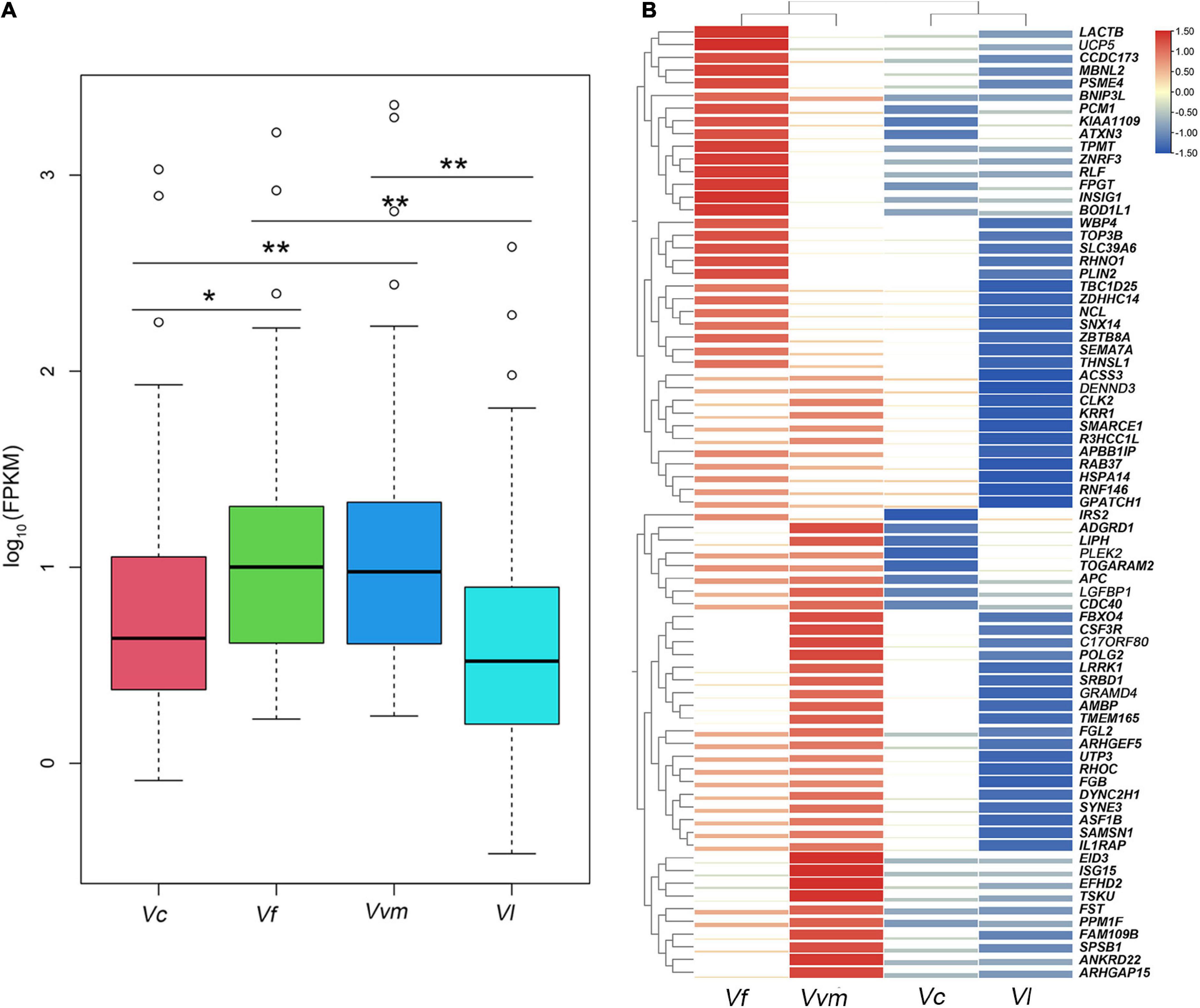
Figure 4. Seventy five differential expressed genes showed significantly higher expression levels in the high-altitude group compared with the low-altitude group. (A) Box-plot analysis presented a significantly higher expression levels in the high-altitude group of these 75 differential expressed genes. *p < 0.05, and **p < 0.01. (B) Heat-map analysis of 75 differential expressed genes. The heat map was generated using hierarchical clustering. Distances, representing the relative similarity among genes and Vulpes, were calculated using Pearson’s correlation coefficients. Color represents FPKM value of gene expression after scaling and centering.
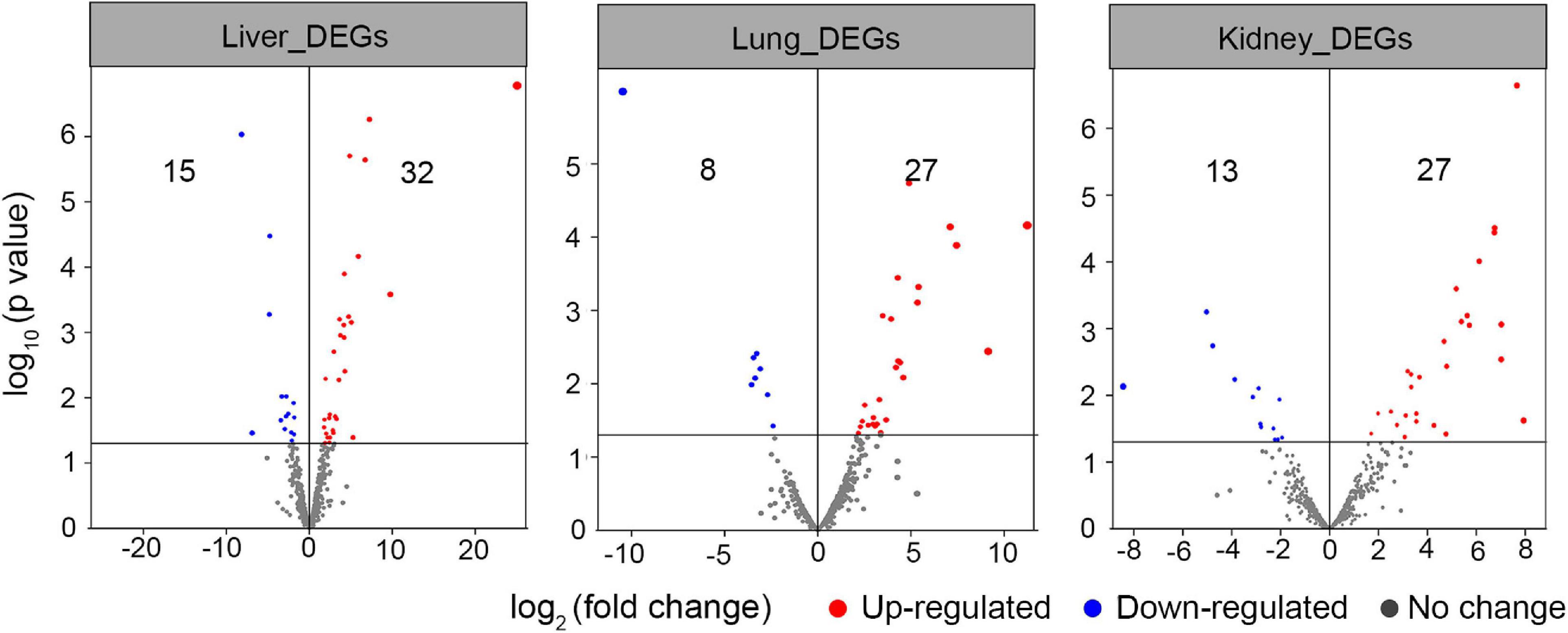
Figure 5. Volcano plot analysis of differential expressed genes in tissues (i.e., liver, lung, and kidney) of different altitude group of Vulpes. The red dots represent the genes up-regulated in Vulpes in the high altitude group (i.e., Vulpes vulpes montana and Vulpes ferrilata), the blue dots represent the genes down regulated in Vulpes in the high altitude group, and the gray dots represent the genes that have not changed significantly.
Discussion
To date, a large number of studies have revealed the high-altitude adaptation mechanism of different plateau species in the face of high selection environment (Tang et al., 2017; Li et al., 2018; Yang et al., 2019). The same selection pressure will lead to the same phenotype or molecular convergence between species with different phylogenetic background (Guo et al., 2016; Tian et al., 2021). On the other hand, the similarities and differences of gene expression levels in tissues of many high-altitude species and their close relatives at low altitudes have also been confirmed (Tang et al., 2017; Hao et al., 2019; Xiong et al., 2022). However, how evolutionary history (i.e., phylogenetic background) contributes to similarity and difference in genetic adaptations to high-altitude environments is largely unknown, in particular in Vulpes. By systematically investigating two high-altitude Vulpes and their low-altitude relatives within a phylogenetic context, our comparative transcriptomics expanded our current understanding of the Vulpes respond to a highly selective environment.
With the aim of contributing to and improving the existing transcriptomic resources available for the genus Vulpes, we sequenced the transcriptomes of three tissues (liver, lung, and kidney) of two Vulpes, combined with the transcriptomes data of three identical tissues of V. ferrilata published in our previous research and the data of three tissues (liver, heart, and kidney) of V. lagopus mined from NCBI database (Peng et al., 2021; Lyu et al., 2022). The topological structure of phylogenetic tree constructed based on single-copy genes is quite consistent with previous studies except V. ferrilata (Zhao et al., 2016). Previous research suggested that V. ferrilata and V. corsac have the closest phylogenetic relationship, and the ancestors of them diverged from the ancestors of V. vulpes about 2.43 million years ago (Zhao et al., 2016). It is worth noting that mtDNA phylogeny could be different from nuclear phylogeny due to incomplete lineage sorting or hybridization. However, due to the lack of systematic studies, different studies have given different insights into the time of divergence of the Vulpes (Fritz et al., 2009; Perini et al., 2010; Nyakatura and Bininda-Emonds, 2012; Humphreys and Barraclough, 2014). Our studies also add new insights of the phylogenetic relationship and the divergence time of the four Vulpes, which could provide a reference for further in-depth studies.
Due to the differences in PSGs between V. ferrilata and V. v. montana, the GO enrichment analysis of both showed different functional enrichment results (Figures 2A,B). Furthermore, V. ferrilata have more PSGs that may be related to coping with the selection pressure of plateau environment compared with V. v. montana (Table 3). Among these 111 PSGs in V. ferrilata, 20 PSGs related to high-altitude environmental selection stress mainly include the following five aspects: DNA damage repair (LIG4, ZNF830, CRTAC1, and GRB2) (Jun et al., 2016; Chen G. et al., 2018; Hou et al., 2019; Félix et al., 2021), energy metabolism (ARF6 and IRS1) (Dong et al., 2006; Gamara et al., 2021), myocardial growth (EIF3A, IL6ST, SIRT4, and MZB1) (Luo et al., 2016; Klimushina et al., 2019; Miao et al., 2019; Zhang et al., 2021), angiogenesis (IGFBP3, RND3, APLNR, RBPJ, and ARHGEF15) (Lofqvist et al., 2007; Kusuhara et al., 2012; Díaz-Trelles et al., 2016; Mastrella et al., 2019; Wu et al., 2021), and hypoxia stress response (RHEB, WWOX, COMMD1, LAMA4, and PNN) (He et al., 2017; Murata et al., 2017; Hsu et al., 2020; Baryla et al., 2022; Cai et al., 2022). In contrast, PSGs related to altitude adaptation in V. v. montana only has DNA damage repair (C19ORF57) (Takemoto et al., 2020) and hypoxia response related to HIF1-α regulation (FUT11, USP8, CASP14, VGLL4, and ALS2) (Troilo et al., 2014; Ye et al., 2018; Rivas et al., 2020; Wang et al., 2020; Ruan et al., 2021). In addition to the species-specific PSGs related to altitude adaptation, two of the four PSGs (TCF20 and KRAS) shared by V. ferrilata and V. v. montana are also related to altitude adaptation. Transcription Factor 20 (TCF20) is a key gene that promotes the activity of transcriptional activators C-JUN, which is related to angiogenesis (Folkman, 2004). KRAS gene is also related to angiogenesis. Previous research has demonstrated that KRAS gene can interact with hypoxia conditions to induce vascular endothelial growth factor (VEGF) (Zeng et al., 2010). In general, the PSGs identified by both high-altitude Vulpes are related to angiogenesis, suggesting that angiogenesis may be the result of convergent evolution of Vulpes in the face of hypoxic selection pressure. As for other PSGs identified in two high-altitude Vulpes, V. ferrilata have more genes related to plateau adaption than V. v. montana, and these genes also involve a wider range of functions. This result might be caused by the background of the phylogenetic relationship between the two species. Previous studies have shown that the divergence time of the ancestors of V. ferrilata and V. v. montana coincides with the uplift time of the QTP, while the divergence time of V. v. montana and V. vulpes is much shorter (Zhao et al., 2016; Lyu et al., 2022). We speculated that the longer the time to adapt to high-altitude environment, the more functional changes related to high-altitude selection pressure could be made in species.
Previous study of high-altitude passerine birds and primates suggested that there are two patterns of gene expression in multiple tissues, including species-specific expression and tissue-specific expression (Yu et al., 2016; Hao et al., 2019). In this study, we observed a tissue-specific expression pattern in Vulpes in the context of using all single copy orthologous genes, that is, the expression of the same tissues between different species clustered together, regardless of the influence of altitude. However, due to the difficulty of obtaining samples, the results of expression patterns of multiple repeated samples may be different from that of a single sample. The only tissue (Vvm3) that does not show tissue-specific expression may be related to the difference of a single sample, which needs further research in the future. To investigate the gene expression shifts caused by high-altitude environment between the high- and low-altitude group, a total of 75 highly expressed genes (HEGs) were obtained in high-altitude group and 19 HEGs were identified as high-altitude response genes (Table 4). These 19 high-altitude related HEGs were mainly include the following five aspects: hypoxia response (IRS2, LIPH, PLEK2, IGFBP1, FGL2, EID3, ISG15, BNIP3L, INSIG1, SLC39A6, and PLIN2) (Fei et al., 2004; Mardilovich and Shaw, 2009; Minchenko et al., 2015; Bildirici et al., 2018; Perng and Lenschow, 2018; Fan et al., 2019; Li Y. F. et al., 2019; Hu et al., 2020; Xu et al., 2020; Wang et al., 2021), DNA damage repair (PSME4) (Huang et al., 2020), myocardial growth (TBC1D25 and RNF146) (Gao et al., 2014; Guo et al., 2020), angiogenesis (LACTB, ADGRD1, SEMA7A, and RHOC) (Wang et al., 2008; Bayin et al., 2016; Li H. T. et al., 2019; Krner et al., 2021), and energy metabolism (UCP5) (Sanchez-Blanco et al., 2006). In summary, the HEGs of the two high-altitude Vulpes are mainly reflected in response to hypoxia, indicating that oxygen content is the main factor causing the gene expression shifts of Vulpes. In addition, the expression profiles of HEGs and a combination of all DEGs in different tissues showed a different pattern from those of all genes (e.g., tissue-specific expression) by separating high-altitude Vulpes from low-altitude Vulpes (Figures 4, 5), suggesting that the 2 high-altitude Vulpes have convergent shifted their expression profiles.
In conclusion, our research identified two genes (TCF20 and KRAS) which related to angiogenesis shared positive selection signature in V. ferrilata and V. v. montana, suggesting that angiogenesis may be one of the key functions of Vulpes to adapt to high-altitude environment. In addition, V. ferrilata have more PSGs related to plateau adaption than V. v. montana, which may be related to the earlier adaptation of V. ferrilata to the QTP than V. v. montana, revealing the influence of phylogenetic background on adaptive genetic changes. On the other hand, the HEGs of the two high-altitude Vulpes are mainly reflected in response to hypoxia, indicating that oxygen content is the main factor causing the gene expression shifts of Vulpes. The results of the study on gene expression in three tissues of four Vulpes at high-altitude and low-altitude also showed that there was a convergent change in gene expression between the two groups, revealing a convergent gene expression and regulation mechanism of Vulpes in the face of high-altitude selection pressure. Our research provides a valuable transcriptomic resource for further studies, and expands our understanding of high-altitude adaptation within a phylogenetic background.
Data availability statement
The datasets presented in this study can be found in online repositories. The names of the repository/repositories and accession number(s) can be found below: https://www.ncbi.nlm.nih.gov/, SRR20203493; https://www.ncbi.nlm.nih.gov/, SRR20203494; https://www.ncbi.nlm.nih.gov/, SRR20203495; https://www.ncbi.nlm.nih.gov/, SRR20138901; https://www.ncbi.nlm.nih.gov/, SRR20138902; and https://www.ncbi.nlm.nih.gov/, SRR20138897.
Ethics statement
The animal study was reviewed and approved by the Ethics Committee for the Care and Use of Laboratory Animals of Qufu Normal University.
Author contributions
TL and XY performed the data collection and analysis. TL performed the writing of the initial draft of the manuscript. All authors contributed to revising the manuscript and the acquisition of funding and conceived the study.
Funding
This work was supported by the National Natural Science Foundation of China (31872242 and 32070405).
Acknowledgments
We thank Ting-Bin Lyu for assistance with sample collection. We are particularly grateful to Ga Ta, Director of Qumarlêb County Administration of Eco-Environment and Natural Resources, and Jiangwen Cairen for assistance in this study. We would also like to thank the Qinghai Forestry and Grassland Bureau for support during this project.
Conflict of interest
The authors declare that the research was conducted in the absence of any commercial or financial relationships that could be construed as a potential conflict of interest.
Publisher’s note
All claims expressed in this article are solely those of the authors and do not necessarily represent those of their affiliated organizations, or those of the publisher, the editors and the reviewers. Any product that may be evaluated in this article, or claim that may be made by its manufacturer, is not guaranteed or endorsed by the publisher.
Supplementary material
The Supplementary Material for this article can be found online at: https://www.frontiersin.org/articles/10.3389/fevo.2022.999411/full#supplementary-material
Supplementary Figure 1 | PCA analysis of gene expression pattern of each tissues. Different colors represent different tissues, and different shapes represent different Vulpes.
Footnotes
References
Bai, L., Liu, B. N., Ji, C. M., Zhao, S. H., Liu, S. Y., Wang, R., et al. (2019). Hypoxic and cold adaptation insights from the Himalayan marmot genome. iScience 11, 519–530. doi: 10.1016/j.isci.2018.11.034
Baryla, I., Styczeñ-Binkowska, E., Płuciennik, E., Kośla, K., and Bednarek, A. K. (2022). The wwox/hif1a axis downregulation alters glucose metabolism and predispose to metabolic disorders. Int. J. Mol. Sci. 23:3326. doi: 10.3390/ijms23063326
Bayin, N. S., Frenster, J. D., Kane, J. R., Rubenstein, J., Modrek, A. S., Baitalmal, R., et al. (2016). Gpr133 (adgrd1), an adhesion g-protein-coupled receptor, is necessary for glioblastoma growth. Oncogenesis 5:e263. doi: 10.1038/oncsis.2016.63
Bildirici, I., Schaiff, W. T., Chen, B., Morizane, M., Oh, S. Y., O’Brien, M., et al. (2018). Plin2 is essential for trophoblastic lipid droplet accumulation and cell survival during hypoxia. Endocrinology 159, 3937–3949. doi: 10.1210/en.2018-00752
Cai, H., Kondo, M., Sandhow, L., Xiao, P., Johansson, A., Sasaki, T., et al. (2022). Critical role of lama4 for hematopoiesis regeneration and acute myeloid leukemia progression. Blood 139, 3040–3057. doi: 10.1182/blood.2021011510
Chen, F. (2006). Orthomcl-db: Querying a comprehensive multi-species collection of ortholog groups. Nucleic Acids Res. 34, D363–D368. doi: 10.1093/nar/gkj123
Chen, G., Chen, J. X., Qiao, Y. T., Shi, Y. R., Liu, W., Zeng, Q., et al. (2018). Znf830 mediates cancer chemoresistance through promoting homologous-recombination repair. Nucleic Acids Res. 46, 1266–1279. doi: 10.1093/nar/gkx1258
Chen, S. F., Zhou, Y. Q., Chen, Y. R., and Gu, J. (2018). Fastp: An ultra-fast all-in-one fastq preprocessor. Bioinformatics 34, i884–i890. doi: 10.1093/bioinformatics/bty560
Cheviron, Z. A., Bachman, G. C., Connaty, A. D., McClelland, G. B., and Storz, J. F. (2012). Regulatory changes contribute to the adaptive enhancement of thermogenic capacity in high-altitude deer mice. Proc. Natl. Acad. Sci. U.S.A. 109, 8635–8640. doi: 10.1073/pnas.1120523109
Díaz-Trelles, R., Scimia, M. C., Bushway, P., Tran, D., Monosov, A., Monosov, E., et al. (2016). Notch-independent rbpj controls angiogenesis in the adult heart. Nat. Commun. 7:12088. doi: 10.1038/ncomms12088
Ding, D., Liu, G. J., Hou, L., Gui, W. Y., Chen, B., and Kang, L. (2018). Genetic variation in ptpn1 contributes to metabolic adaptation to high-altitude hypoxia in Tibetan migratory locusts. Nat. Commun. 9:4991.
Dong, X. C., Park, S. M., Lin, X. Y., Copps, K., Yi, X. J., and White, M. F. (2006). Irs1 and irs2 signaling is essential for hepatic glucose homeostasis and systemic growth. J. Clin. Invest. 116, 101–114. doi: 10.1172/JCI25735
Fan, C., Wang, J., Mao, C., Li, W., and Wang, Z. (2019). The fgl2 prothrombinase contributes to the pathological process of experimental pulmonary hypertension. J. Appl. Physiol. 124, 1677–1687. doi: 10.1152/japplphysiol.00396.2019
Fei, P. W., Wang, W. G., Kim, S. H., Wang, S. L., Burns, T. F., Sax, J. K., et al. (2004). Bnip3l is induced by p53 under hypoxia, and its knockdown promotes tumor growth. Cancer Cell 6, 597–609. doi: 10.1016/j.ccr.2004.10.012
Félix, R. C., Anjos, L., Costa, R. A., Letsiou, S., and Power, D. M. (2021). Cartilage acidic protein a novel therapeutic factor to improve skin damage repair? Mar. Drugs. 19:541. doi: 10.3390/md19100541
Fritz, S. A., Bininda-Emonds, O. R. P., and Purvis, A. (2009). Geographical variation in predictors of mammalian extinction risk: Big is bad, but only in the tropics. Ecol. Lett. 12, 538–549. doi: 10.1111/j.1461-0248.2009.01307.x
Gamara, J., Davis, L., Leong, A. Z., Pagé, N., Rollet-Labelle, E., Zhao, C., et al. (2021). Arf6 regulates energy metabolism in neutrophils. Free Radic. Biol. Med. 172, 550–561. doi: 10.1016/j.freeradbiomed.2021.07.001
Gao, Y., Song, C. Y., Hui, L. P., Li, C. Y., Wang, J. Y., Tian, Y., et al. (2014). Overexpression of rnf146 in non-small cell lung cancer enhances proliferation and invasion of tumors through the wnt/b-catenin signaling pathway. PLoS One 1:e85377. doi: 10.1371/journal.pone.0085377
Ge, R. L., Cai, Q. L., Shen, Y. Y., San, A., Ma, L., Zhang, Y., et al. (2013). Draft genome sequence of the Tibetan antelope. Nat. Commun. 4:1858. doi: 10.1038/ncomms2860
Grabherr, M. G., Haas, B. J., Yassour, M., Levin, J. Z., Thompson, D. A., Amit, I., et al. (2011). Full-length transcriptome assembly from RNA-seq data without a reference genome. Nat. Biotechnol. 29, 644–652. doi: 10.1038/nbt.1883
Guo, S., Liu, Y., Gao, L., Xiao, F. K., Shen, J. H., Xing, S. Y., et al. (2020). Tbc1d25 regulates cardiac remodeling through tak1 signaling pathway. Int. J. Biol. Sci. 16, 1335–1348. doi: 10.7150/ijbs.41130
Guo, X. Y., Xie, L., Zhang, X. Z., Ji, Y. F., Chen, J., Pang, B., et al. (2016). Signatures of functional constraint at Fgfr1a Genes in schizothoracine fishes (Pisces: Cypriniformes): The dermal skeleton variation adapted to high-altitude environments. Integr. Zool. 11, 86–97. doi: 10.1111/1749-4877.12178
Haas, B. J., Papanicolaou, A., Yassour, M., Grabherr, M., Blood, P. D., Bowden, J., et al. (2013). De novo transcript sequence reconstruction from RNA-seq using the Trinity platform for reference generation and analysis. Nat. Protoc. 8, 1494–1512. doi: 10.1038/nprot.2013.084
Hao, Y., Xiong, Y., Cheng, Y. L., Song, G., Jia, C. X., Qu, Y. H., et al. (2019). Comparative transcriptomics of 3 high-altitude passerine birds and their low-altitude relatives. Proc. Natl. Acad. Sci. U.S.A. 116, 11851–11856. doi: 10.1073/pnas.1819657116
He, L. H., Zhang, X., Huang, Y., Yang, H. P., Wang, Y. L., and Zhang, Z. P. (2017). The characterization of rheb gene and its responses to hypoxia and thermal stresses in the small abalone haliotis diversicolor. Comp. Biochem. Phys. B. 210, 48–54. doi: 10.1016/j.cbpb.2017.06.001
He, L., Wang, Q., Jin, X., Wang, Y., Chen, L., Liu, L., et al. (2012). Transcriptome profiling of testis during sexual maturation stages in eriocheir sinensis using illumina sequencing. PLoS One 7:e33735. doi: 10.1371/journal.pone.0033735
Hou, B. L., Xu, S. S., Xu, Y., Gao, Q., Zhang, C. N., Liu, L., et al. (2019). Grb2 binds to pten and regulates its nuclear translocation to maintain the genomic stability in dna damage response. Cell Death Dis. 10:546. doi: 10.1038/s41419-019-1762-3
Hsu, S. Y., Mukda, S., and Leu, S. (2020). Expression and distribution pattern of pnn in ischemic cerebral cortex and cultured neural cells exposed to oxygen-glucose deprivation. Brain Sci. 10:708. doi: 10.3390/brainsci10100708
Hu, B., Yang, X. B., and Sang, X. (2020). Development and verification of the hypoxia-related and immune-associated prognosis signature for hepatocellular carcinoma. J. Hepatocell. Carcinoma 7, 315–330. doi: 10.2147/JHC.S272109
Hu, Y. B., Wu, Q., Ma, S., Song, G., Ma, T. X., Shan, L., et al. (2016). Comparative genomics reveals convergent evolution between the bamboo-eating giant and red pandas. Proc. Natl. Acad. Sci. U.S.A. 116, 11851–11856. doi: 10.1073/pnas.1613870114
Huang, Y. L., Zhang, P. F., Hou, Z., Fu, Q., Li, M. X., Huang, D. L., et al. (2020). Ubiquitome analysis reveals the involvement of lysine ubiquitination in the spermatogenesis process of adult buffalo (Bubalus bubalis) testis. Biosci. Rep. 6:40. doi: 10.1042/BSR20193537
Humphreys, A. M., and Barraclough, T. G. (2014). The evolutionary reality of higher taxa in mammals. Proc. Biol. Sci. 281:20132750. doi: 10.1098/rspb.2013.2750
Imani Harsini, J., Rezaei, H. R., Naderi, S., and Varasteh Moradi, H. (2017). Phylogenetic status and genetic diversity of corsac fox (Vulpes corsac) in golestan province, Iran. Turk. J. Zool. 41, 250–258. doi: 10.3906/zoo-1509-52
Jun, S., Jung, Y., Suh, H. N., Wang, W., Kim, M. J., Oh, Y. S., et al. (2016). Lig4 mediates wnt signalling-induced radioresistance. Nat. Commun. 7:10994. doi: 10.1038/ncomms10994
Klimushina, M. V., Gumanova, N. G., Kutsenko, V. A., Divashuk, M. G., Smetnev, S. A., Kiseleva, A. V., et al. (2019). Association of common polymorphisms in il-6 and il6st genes with levels of inflammatory markers and coronary stenosis. Meta Gene 21:100593. doi: 10.1016/j.mgene.2019.100593
Krner, A., Bernard, A., Fitzgerald, J. C., Alarcon-Barrera, J. C., and Mirakaj, V. (2021). Sema7a is crucial for resolution of severe inflammation. Proc. Natl. Acad. Sci. U.S.A. 118:e2017527118. doi: 10.1073/pnas.2017527118
Kumar, V., Kutschera, E. V., Nilsson, A. M., and Janke, A. (2015). Genetic signatures of adaptation revealed from transcriptome sequencing of arctic and red foxes. BMC Genomics. 16:585. doi: 10.1186/s12864-015-1724-9
Kusuhara, S., Fukushima, Y., Fukuhara, S., Jakt, L. M., Okada, M., Shimizu, Y., et al. (2012). Arhgef15 promotes retinal angiogenesis by mediating vegf-induced cdc42 activation and potentiating rhoj inactivation in endothelial cells. PLoS One 7:e45858. doi: 10.1371/journal.pone.0045858
Lan, D. L., Ji, W. H., Xiong, X. R., Liang, Q. Q., Yao, W. Y., Mipam, T. D., et al. (2021). Population genome of the newly discovered Jinchuan yak to understand its adaptive evolution in extreme environments and generation mechanism of the multirib trait. Integr. Zool. 16, 685–695. doi: 10.1111/1749-4877.12484
Li, B., and Dewey, C. N. (2011). RSEM: Accurate transcript quantification from RNA-seq data with or without a reference genome. BMC Bioinformatics 12:323. doi: 10.1186/1471-2105-12-323
Li, H. T., Dong, D. Y., Liu, Q., Xu, Y. Q., and Chen, L. B. (2019). Overexpression of lactb, a mitochondrial protein that inhibits proliferation and invasion in glioma cells. Oncol. Res. 27, 423–429. doi: 10.3727/096504017X15030178624579
Li, J. T., Gao, Y. D., Xie, L., Deng, C., Shi, P., Guan, M. L., et al. (2018). Comparative genomic investigation of high-elevation adaptation in ectothermic snakes. Proc. Natl. Acad. Sci. U.S.A. 115, 8406–8411. doi: 10.1073/pnas.1805348115
Li, Y. F., Zhou, X. F., Zhang, Q. Y., Chen, E. D., Sun, Y. H., Ye, D. R., et al. (2019). Lipase member h is a downstream molecular target of hypoxia inducible factor-1& α; and promotes papillary thyroid carcinoma cell migration in bcpap and ktc-1 cell lines. Cancer Manag. Res. 11, 931–941. doi: 10.2147/CMAR.S183355
Li, Y., Wu, D., Boyko, A. R., Wang, G., Wu, S., Irwin, D. M., et al. (2014). Population variation revealed high-altitude adaptation of Tibetan mastiffs. Mol. Biol. Evol. 31, 1200–1205. doi: 10.1093/molbev/msu070
Lofqvist, C., Chen, J., Connor, K. M., Smith, A. C., Aderman, C. M., Liu, N., et al. (2007). Igfbp3 suppresses retinopathy through suppression of oxygen-induced vessel loss and promotion of vascular regrowth. Proc. Natl. Acad. Sci. U.S.A. 104, 10589–10594. doi: 10.1073/pnas.0702031104
Luo, Y. X., Tang, X. Q., An, X. Z., Xie, X. M., Chen, X. F., Zhao, X., et al. (2016). Sirt4 accelerates ang ii-induced pathological cardiac hypertrophy by inhibiting manganese superoxide dismutase activity. Eur. Heart J. 38, 1389–1398. doi: 10.1093/eurheartj/ehw138
Lyu, T. S., Wei, Q. G., Wang, L. D., Zhou, S. Y., Shi, L. P., Dong, Y. H., et al. (2022). High-quality chromosome-level genome assembly of Tibetan fox (Vulpes ferrilata). Zool. Res. 43, 362–366. doi: 10.24272/j.issn.2095-8137.2021.399
Mardilovich, K., and Shaw, L. M. (2009). Hypoxia regulates insulin receptor substrate-2 expression to promote breast carcinoma cell survival and invasion. Cancer Res. 69, 8894–8901. doi: 10.1158/0008-5472.CAN-09-1152
Mastrella, G., Hou, M., Li, M., Stoecklein, V. M., Zdouc, N., Volmar, M. N. M., et al. (2019). Targeting apln/aplnr improves antiangiogenic efficiency and blunts proinvasive side effects of vegfa/vegfr2 blockade in glioblastoma. Cancer Res. 79, 2298–2313. doi: 10.1158/0008-5472.CAN-18-0881
Maza, E. (2016). In papyro comparison of tmm (edger), rle (deseq2), and mrn normalization methods for a simple two-conditions-without-replicates rna-seq experimental design. Front. Genet. 7:164. doi: 10.3389/fgene.2016.00164
Miao, B. S., Wei, C. Y., Qiao, Z. J., Han, W. Y., Chai, X. Q., Lu, J. C., et al. (2019). Eif3a mediates hif1-α –dependent glycolytic metabolism in hepatocellular carcinoma cells through translational regulation. Am. J. Cancer Res. 9, 1079–1090.
Minchenko, D. O., Kharkova, A. P., Karbovskyi, L. L., and Minchenko, O. H. (2015). Expression of insulin-like growth factor binding protein genes and its hypoxic regulation in u87 glioma cells depends on ern1 mediated signaling pathway of endoplasmic reticulum stress. Endocr. Regul. 49, 73–83. doi: 10.4149/endo_2015_02_73
Murata, K., Fang, C., Terao, C., Giannopoulou, E. G., Lee, Y. J., Lee, M. J., et al. (2017). Hypoxia-sensitive commd1 integrates signaling and cellular metabolism in human macrophages and suppresses osteoclastogenesis. Immunity 47, 66–79. doi: 10.1016/j.immuni.2017.06.018
Nyakatura, K., and Bininda-Emonds, O. R. (2012). Updating the evolutionary history of carnivora (mammalia): A new species-level supertree complete with divergence time estimates. BMC Biol. 10:12. doi: 10.1186/1741-7007-10-12
Peng, Y. D., Li, H., Liu, Z. Z., Zhang, C. S., Li, K. Q., Gong, Y. F., et al. (2021). Chromosome-level genome assembly of the arctic fox (Vulpes lagopus) using pacbio sequencing and hi-c technology. Mol. Ecol. Resour. 21, 2093–2108. doi: 10.1111/1755-0998.13397
Perini, F. A., Russo, C. A. M., and Schrago, C. G. (2010). The evolution of south american endemic canids: A history of rapid diversification and morphological parallelism. J. Evol. Biol. 23, 311–322. doi: 10.1111/j.1420-9101.2009.01901.x
Perng, Y. C., and Lenschow, D. J. (2018). Isg15 in antiviral immunity and beyond. Nat. Rev. Microbiol. 16, 423–439. doi: 10.1038/s41579-018-0020-5
Qiu, Q., Zhang, G., Ma, T., Qian, W., Wang, J., Ye, Z., et al. (2012). The yak genome and adaptation to life at high altitude. Nat. Genet. 44, 946–949. doi: 10.1038/ng.2343
Rivas, S., Silva, P., Reyes, M., Sepúlveda, H., Solano, L., Acuña, J., et al. (2020). The rabgef als2 is a hypoxia inducible target associated with the acquisition of aggressive traits in tumor cells. Sci. Rep. 10:22302. doi: 10.1038/s41598-020-79270-6
Robinson, M. D., McCarthy, D. J., and Smyth, G. K. (2009). Edger: A bioconductor package for differential expression analysis of digital gene expression data. Bioinformatics 26, 139–140. doi: 10.1093/bioinformatics/btp616
Ruan, W. Y., Yang, Y. S., Yu, Q. H., Huang, T. J., Wang, Y. F., Hua, L., et al. (2021). Fut11 is a target gene of hif1-α that promotes the progression of hepatocellular carcinoma. Cell Biol. Int. 45, 2275–2286. doi: 10.1002/cbin.11675
Sanchez-Blanco, A., Fridell, Y. C., and Helfand, S. L. (2006). Involvement of drosophila uncoupling protein 5 in metabolism and aging. Genetics 172, 1699–1710. doi: 10.1534/genetics.105.053389
Souchet, J., Gangloff, E. J., Micheli, G., Bossu, C., Trochet, A., Bertrand, R., et al. (2020). High-elevation hypoxia impacts perinatal physiology and performance in a potential montane colonizer. Integr. Zool. 15, 544–557. doi: 10.1111/1749-4877.12468
Stamatakis, A. (2006). Raxml-vi-hpc: Maximum likelihood-based phylogenetic analyses with thousands of taxa and mixed models. Bioinformatics 22, 2688–2690. doi: 10.1093/bioinformatics/btl446
Sudhir, K., Glen, S., and Koichiro, T. (2016). Mega7: Molecular evolutionary genetics analysis version 7.0 for bigger datasets. Mol. Biol. Evol. 7:1870. doi: 10.1093/molbev/msw054
Takemoto, K., Tani, N., Takada-Horisawa, Y., Fujimura, S., Tanno, N., Yamane, M., et al. (2020). Meiosis-specific c19orf57/4930432k21rik/brme1 modulates localization of rad51 and dmc1 to dsbs in mouse meiotic recombination. Cell Rep. 31:107686. doi: 10.1016/j.celrep.2020.107686
Tang, Q. Z., Gu, Y. R., Zhou, X. M., Jin, L., Guan, J. Q., Liu, R., et al. (2017). Comparative transcriptomics of 5 high-altitude vertebrates and their low-altitude relatives. Gigascience. 6, 1–9. doi: 10.1093/gigascience/gix105
Tian, R., Geng, Y. P., Yang, Y., Seim, I., and Yang, G. (2021). Oxidative stress drives divergent evolution of the glutathioneperoxidase (GPX) gene family in mammals. Integr. Zool. 16, 696–711. doi: 10.1111/1749-4877.12521
Troilo, A., Alexander, I., Muehl, S., Jaramillo, D., Knobeloch, K. P., and Krek, W. (2014). Hif1alpha deubiquitination by usp8 is essential for ciliogenesis in normoxia. EMBO. Rep. 15, 77–85. doi: 10.1002/embr.201337688
Vinarski, M. V., Von Oheimb, P. V., Aksenova, O. V., Gofarov, M. Y., Kondakov, A. V., Nekhaev, I. O., et al. (2021). Trapped on the roof of the world: Taxonomic diversity andevolutionary patterns of Tibetan Plateau endemic freshwatersnails (Gastropoda: Lymnaeidae: Tibetoradix). Integr. Zool. 1–24. doi: 10.1111/1749-4877.12600
Wang, J. Y., Sun, Z., Wang, J., Tian, Q. H., Huang, R. D., Wang, H. Y., et al. (2021). Expression and prognostic potential of plek2 in head and neck squamous cell carcinoma based on bioinformatics analysis. Cancer Med. 10, 6515–6533. doi: 10.1002/cam4.4163
Wang, W., Wu, F., Fang, F., Tao, Y. M., and Yang, L. Y. (2008). Rhoc is essential for angiogenesis induced by hepatocellular carcinoma cells via regulation of endothelial cell organization. Cancer Sci. 99, 2012–2018. doi: 10.1111/j.1349-7006.2008.00902.x
Wang, Y. Q., Liu, X. H., Xie, B. S., Yuan, H., Zhang, Y. Y., and Zhu, J. (2020). The notch1-dependent hif1-α/vgll4/irf2bp2 oxygen sensing pathway triggers erythropoiesis terminal differentiation. Redox Biol. 28:101313. doi: 10.1016/j.redox.2019.101313
Wu, D. D., Yang, C. P., Wang, M. S., Dong, K. Z., Yan, D. W., Hao, Z. Q., et al. (2020). Convergent genomic signatures of high-altitude adaptation among domestic mammals. Natl. Sci. Rev. 7, 952–963. doi: 10.1093/nsr/nwz213
Wu, N., Zheng, F., Li, N., Han, Y., Xiong, X. Q., Wang, J. J., et al. (2021). Rnd3 attenuates oxidative stress and vascular remodeling in spontaneously hypertensive rat via inhibiting rock1 signaling. Redox Biol. 48:102204. doi: 10.1016/j.redox.2021.102204
Xiong, Y., Hao, Y., Cheng, Y., Fan, L. Q., Song, G., Li, D. M., et al. (2022). Comparative transcriptomic and metabolomic analysis reveals pectoralis highland adaptation across altitudinal songbirds. Integr. Zool. 1–17. doi: 10.1111/1749-4877.12620
Xu, D. Q., Wang, Z., Xia, Y., Shao, F., Xia, W. Y., Wei, Y. K., et al. (2020). The gluconeogenic enzyme pck1 phosphorylates insig1/2 for lipogenesis. Nature 580, 530–535. doi: 10.1038/s41586-020-2183-2
Yang, X. F., Liu, H. P., Ma, Z. H., Zou, Y., Zou, M., Mao, Y. Z., et al. (2019). Chromosome-level genome assembly of Triplophysa tibetana, a fish adapted to the harsh high-altitude environment of the tibetan plateau. Mol. Ecol. Resour. 19, 1027–1036. doi: 10.1111/1755-0998.13021
Yang, Z. (2007). Paml 4: Phylogenetic analysis by maximum likelihood. Mol. Biol. Evol. 24, 1586–1591. doi: 10.1093/molbev/msm088
Ye, I. C., Fertig, E. J., DiGiacomo, J. W., Considine, M., Godet, I., and Gilkes, D. M. (2018). Molecular portrait of hypoxia in breast cancer: A prognostic signature and novel hif-regulated genes. Mol. Cancer Res. 16, 1889–1901. doi: 10.1158/1541-7786.MCR-18-0345
Yu, L., Wang, G. D., Ruan, J., Chen, Y. B., Yang, C. P., Cao, X., et al. (2016). Genomic analysis of snub-nosed monkeys (Rhinopithecus) identifies genes and processes related to high-altitude adaptation. Nat. Genet. 48, 947–952. doi: 10.1038/ng.3615
Zeng, M., Kikuchi, H., Pino, M. S., and Chung, D. C. (2010). Hypoxia activates the k-ras proto-oncogene to stimulate angiogenesis and inhibit apoptosis in colon cancer cells. PLoS One 5:e10966. doi: 10.1371/journal.pone.0010966
Zhang, L., Wang, Y. N., Ju, J. M., Shabanova, A., Li, Y., Fang, R. N., et al. (2021). Mzb1 protects against myocardial infarction injury in mice via modulating mitochondrial function and alleviating inflammation. Acta Pharmacol. Sin. 42, 691–700. doi: 10.1038/s41401-020-0489-0
Zhang, W., Fan, Z., Han, E., Hou, R., Zhang, L., Galaverni, M., et al. (2014). Hypoxia adaptations in the grey wolf (Canis lupus chanco) from Qinghai-Tibet plateau. PLoS Genet. 10:e1004466. doi: 10.1371/journal.pgen.1004466
Zhao, C., Zhang, H. H., Liu, G. S., Yang, X. F., and Zhang, J. (2016). The complete mitochondrial genome of the tibetan fox (Vulpes ferrilata) and implications for the phylogeny of canidae. C R. Biol. 339, 68–77. doi: 10.1016/j.crvi.2015.11.005
Keywords: comparative transcriptomic, high-altitude, Vulpes, adaption, convergent evolution
Citation: Lyu T, Yang X, Zhao C, Wang L, Zhou S, Shi L, Dong Y, Dou H and Zhang H (2022) Comparative transcriptomics of high-altitude Vulpes and their low-altitude relatives. Front. Ecol. Evol. 10:999411. doi: 10.3389/fevo.2022.999411
Received: 21 July 2022; Accepted: 05 September 2022;
Published: 23 September 2022.
Edited by:
Wen Bo Liao, China West Normal University, ChinaCopyright © 2022 Lyu, Yang, Zhao, Wang, Zhou, Shi, Dong, Dou and Zhang. This is an open-access article distributed under the terms of the Creative Commons Attribution License (CC BY). The use, distribution or reproduction in other forums is permitted, provided the original author(s) and the copyright owner(s) are credited and that the original publication in this journal is cited, in accordance with accepted academic practice. No use, distribution or reproduction is permitted which does not comply with these terms.
*Correspondence: Honghai Zhang, zhanghonghai67@126.com
†These authors have contributed equally to this work and share first authorship