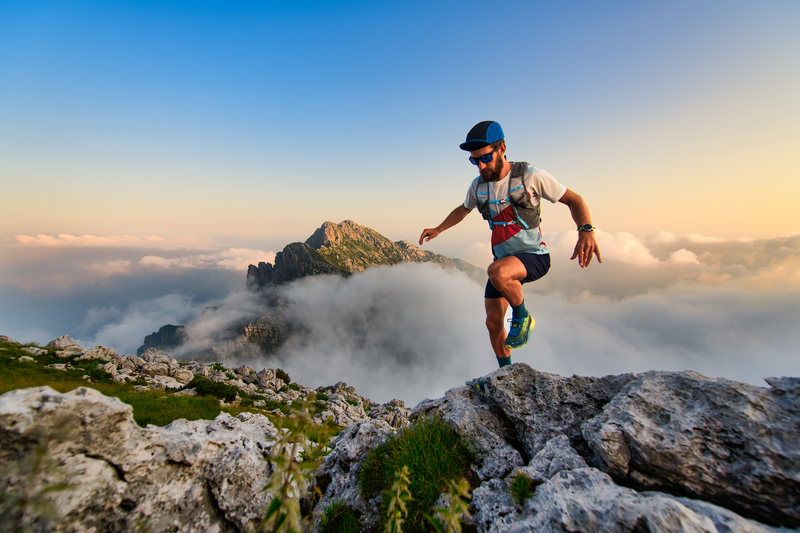
95% of researchers rate our articles as excellent or good
Learn more about the work of our research integrity team to safeguard the quality of each article we publish.
Find out more
ORIGINAL RESEARCH article
Front. Ecol. Evol. , 21 September 2022
Sec. Interdisciplinary Climate Studies
Volume 10 - 2022 | https://doi.org/10.3389/fevo.2022.998945
This article is part of the Research Topic Climate Change and Adaptive Capacity Building View all 15 articles
It is of great significance for global environmental governance to guide farmers to effectively perceive climate change. Based on the survey data of 540 farmers in Sichuan Province, China, this study used binary Logit model and Multinomial Logistic Regression model to explore the effects of farmers’ space-time perception of climate change and their interaction effects on farmers’ adaptation behavior to climate change. The results showed that: (1) 88.51% of farmers took adaptation measures to climate change, and 61.11% of them took both passive and active adaptation measures. Among the 7 measures, the highest rate of “Increase irrigation” is 23%, and the lowest rate of “Migrant work” is only 5%. (2) The scale difference of farmers’ time perception of climate change has a significant positive impact on their adaptive behavior of climate change. In terms of time: climate change perception in the next 5 years > in the next 10 years > in the next 15 years. (3) The scale difference of farmers’ space perception of climate change has a significant positive impact on their adaptation behavior to climate change. In other words, spatially, farmers’ perception of climate change is global > national > local village (the perception of local province is not significant). (4) Farmers’ space-time perception of climate change significantly affects farmers’ adaptive behavior. Among them, “farmers’ perception of climate change in the next 5 years” and their own “village’s perception of climate change” play an important role. This study will help deepen the understanding of farmers’ perception of climate change and their adaptive behavior, and provide reference for national policy making.
Global climate change has become a human consensus (Waldman et al., 2017). Since 1850–1900, the global average surface temperature has risen by about 1°C, while the average surface temperature in China has risen by about 1.46°C in the past 60 years (Wang et al., 2016). It is predicted by IPCC that the global temperature will increase by at least 1.5°C in the next 20 years (Pielke et al., 2022), and the annual mean temperature change rate in China will further increase by 2030 (Chao et al., 2020), rising from 0.32°C/ (10 a) to 0.48°C/ (10 a). The intensification of climate change has a severe impact on the global ecological, economic and social systems (Feng and Xu, 2014; Clayton, 2020; Malhi et al., 2020). For example, in 2016, Canada was affected by high temperatures and strong winds, leading to the largest forest fire in history, causing economic losses of 3 billion US dollars (Sun et al., 2017). From 2001 to 2020, floods in China caused over 100 million people to be affected annually, with direct economic losses up to 167.86 billion Yuan (Li and Zhao, 2022). Increasingly prominent meteorological problems have attracted extensive attention of the international community (Dietz et al., 2020). In this context, the 2016 Paris Agreement sets forth goals such as “achieving carbon neutrality by the second half of the century between anthropoid emissions and removal of greenhouse gases,” marking a new stage in global climate governance (Li and Chai, 2017).
China has been adhering to the concept of green development and has attached great importance to climate change response (Chai et al., 2020). As early as 1990, China formulated policies to address climate change, calling for better understanding and management of climate change. In 2020, China proposed its first “dual carbon target,” aiming to peak carbon dioxide emissions by 2030 and achieve carbon neutrality by 2060, demonstrating the country’s determination to tackle climate change (Bo and Zhuang, 2018). However, China’s total carbon dioxide emissions currently rank first in the world and its carbon intensity is 130% of the global average (Zhang et al., 2021). Climate change mitigation is a great challenge and cannot be separated from the broad support of subjects at all levels of society (Liu et al., 2021). As the main unit of agricultural production, farmers’ response to climate change will have an important impact on national climate governance. On the one hand, the agricultural production activities of farmers are the main emission sources of carbon dioxide (Zhang et al., 2021). On the other hand, due to the limitations of income, education level and other conditions, farmers have insufficient understanding of climate change and limited emission reduction ability (Lv and Chen, 2010). Therefore, how to effectively improve farmers’ ability to perceive and cope with climate change will become the only way to achieve the “dual carbon goal” and mitigate climate change.
Adaptation to climate change refers to the process in which people attempt to mitigate the hazards of climate change while taking advantage of the positive benefits of climate change (Ogunbode et al., 2019; Gao et al., 2021). At present, the academic circle has conducted extensive studies on farmers’ adaptive behavior to climate change, mainly involving farmers’ perception of climate change (Rayamajhee et al., 2021), adaptive behavior of climate change (Phuong et al., 2018a,b), willingness to respond to climate change (Hossain et al., 2022). Among them, adaptive behavior of climate change has always been the focus of academic attention. According to the existing research, the factors affecting farmers’ adaptive behavior decisions on climate change mainly focus on the following aspects: First, basic personal characteristics, such as farmer’s gender (Jin et al., 2015), age (Islam et al., 2013) and education level (Fosu-Mensah et al., 2012), have been proved to significantly affect farmer’s adaptation to climate change. Second, basic family characteristics, for example, Abid et al. (2016); Thinda et al. (2020) found that household income, arable land area and agricultural product disaster experience were important factors affecting farmers’ response to climate change.
To sum up, although many existing literatures focus on the core factors that affect farmers’ adaptive behavior to climate change, most of these factors focus on the basic characteristics of individual farmers and families. From the perspective of temporal and spatial perception of climate change, few studies have focused on the impact of temporal and spatial perception and interaction effects of climate change on farmers’ adaptive behaviors. In the only studies, they either focused only on the climate change trend at the macro level, adopted more qualitative analysis methods, or only analyzed farmers’ perception of climate change in time or space (Pahl et al., 2014; Shi, 2016), lack of multi-dimensional analysis of micro-subject climate change perception. In the vast rural areas, limited information channels result in farmers’ perception of climate change can only be judged by their own experience. The deviation between climate change perception experience and reality will have a negative effect on farmers’ response to climate change. For example, when farmers’ perception of small regional climate change threat is not obvious, it may cause farmers’ enthusiasm to deal with it is insufficient. Poor understanding of long-term climate change risks may lead farmers to choose short-term measures and ignore long-term effects and environmental hazards. At present, it has been confirmed by quantitative methods that differences in individual climate change risk perception in a certain period will significantly affect adaptive behaviors (Wheeler et al., 2021). However, in the context of increasingly obvious climate change, the impacts of temporal and spatial perception and interaction effects of climate change on farmers’ adaptive behaviors are still relatively neglected. So in the process of farmers coping with climate change, can they perceive climate change in different time and space? If so, will the temporal and spatial scales of climate change perception and their interaction affect farmers’ adaptation to climate change differently? This is the problem to be solved in this paper.
Climate change adaptation refers to people’s attempt to avoid the damage of climate change to personal life and property, while taking advantage of the positive effects of climate change. At present, some scholars believe that farmers’ adaptation measures to climate change can be divided into engineering and non-engineering, the former including Wells and channels, etc., and the latter including agricultural adjustment and agricultural insurance purchase, etc. (Chen et al., 2014). According to the sequence of drought and coping behaviors, some scholars divided climate change adaptation into pre-event and post-event remedial behaviors. Among them, ex ante remedial actions include mulching film, while ex post remedial actions include increasing irrigation and fertilizer application, etc. (Feng et al., 2016). Based on the research of Lv and Chen (2010) and Chen et al. (2014), this paper divided farmers’ climate change adaptation measures into active adaptation and passive adaptation. In the process of farmers’ response to climate change, climate change perception is the key factor affecting their decision-making. When the perceived risk of climate change is high, the possibility of adapting to climate change will increase (Zhao, 2014).
Scale features refer to the temporal and spatial representation of geographical phenomena and processes. Since scale features are internal features of things, they often need observation to identify them, thus forming the observation scale. That is, the changes revealed by observations at different time and spatial scales. The change of observation scale will change the spatial and temporal observation resolution, which will lead to the change of geographical phenomena and process information characteristics, and finally show a certain scale effect (Fu, 2014).
According to the “multi-scale conceptual framework” in spatial scale theory, spatial scale can be divided into global scale, regional scale and local scale (Li, 2014). Among them, global scale refers to the global scope; Regional scale refers to countries, provinces, towns, economic zones, etc. Local scale refers to municipalities, villages, etc. (Zhang et al., 2020). Different spatial scales have significant differences in agricultural production, regional environment, economic level and other aspects (Kobayashi et al., 2014; Zorrilla-Miras et al., 2014). In the local, the impact of population, land and other factors changes will be transmitted locally to the region and the world. At present, spatial scale theory is mostly applied in the field of ecosystem and administrative management, mainly focusing on landscape layout (Fan et al., 2018), urban planning (Liu and Zhang, 2015), and regional economy (Guan et al., 2015).
With the deepening of research, scale effect has also been developed in the field of time. Construal Level Theory holds that object events and self-time, space, social distance are closely related to individual psychological distance cognition, which has an important influence on the psychological construction of object events. People will adopt high-level construction for things with a psychological distance, that is, their perception is more abstract, generalized and context-removed. For things with close psychological distance, they will adopt low level construction, that is, the perception is more specific, clear and contextual. Different levels of construction will affect individuals’ cognitive and emotional experience and influence their behavioral choices (Bar-Anan et al., 2006; Trope and Liberman, 2010). Based on the constructional level theory, some scholars found that the expansion of psychological distance in time would reduce people’s concern about climate change and thus reduce their willingness to take measures against climate change (Spence et al., 2012). Vivid and accurate cognition of the future will enhance people’s perception of climate change risk and thus enhance their willingness to cope with climate change (D’Argembeau et al., 2011; Lee et al., 2020). Nowadays, constructively-related theories have been preliminarily applied in the fields of pro-environmental behavior and climate change (Lin et al., 2020).
However, space-time scale theory also seems to have more general applicability. For example, Zhang et al. (2019, 2020) discussed the impact of land use change on regional ecosystem services based on spatial and temporal differences. Therefore, there may also be time and space scale effects in the process of climate change perception on farmers’ adaptive behaviors. However, the existing literature often takes climate change perception as a whole, which covers the difference of the impact of climate change perception on farmers’ coping behavior in different time and space. Therefore, farmers’ perception of climate change can be divided into two categories in this paper: first, space perception of climate change, that is, perception of climate change in the global, national, provincial and village. Time perception of climate change refers to the degree of perception of climate change in the next 5, 10 and 15 years. Compared with the long time distance, the short time distance people’s prediction is more specific and accurate, and has a greater direct impact on farmers’ adaptation behavior to climate change. Compared with far-space distance, near-space distance is more difficult to detect climate change and has less impact on farmers’ response to climate change. Based on this, the following hypotheses are made in this study (Figure 1).
H1: Farmers’ space-time perception and interaction effects of climate change will positively affect their adaptive behaviors.
H2: Compared with the perception of climate change over a long time distance, the perception of climate change over a short time distance has a greater impact on farmers’ adaptation behavior decisions to climate change.
H3: Compared with far-space distance climate change perception, near-space distance climate change perception has less impact on farmers’ adaptive behavior decisions of climate change.
Sichuan is one of China’s major grain-producing areas, forming subtropical humid monsoon climate, subtropical monsoon climate and other climates. According to statistics, the farmland with perennial drought and water shortage in Sichuan Province accounts for more than 60% of the province’s cultivated land area (Xu et al., 2019). As a typical climate vulnerable area, Sichuan Province is also common with meteorological disasters such as rainstorm, flood and high temperature. The data used in this study are from the research group’s survey and statistics of 27 villages in 3 districts and counties, 9 towns and 27 villages in Sichuan Province, China in July 2021. The survey method is one-to-one interview, and each questionnaire lasts about 1–1.5 h. The respondents are the family members who know more about family agricultural production, and 61.30% of them are household heads. The research content mainly includes the basic characteristics of individual farmers and families, the perception of time and space of climate change, and the response measures to climate change. In order to ensure the representativeness of samples, stratified equal probability sampling is adopted in this study, with specific procedures as follows.
First of all, according to the topography (plain, mountain and hill) and other indicators, the 183 districts and counties in Sichuan province were divided into three categories: good, medium and poor, and one district were randomly selected. Secondly, according to the three districts and counties in the distance from the county government, the level of economic development and other indicators, each district and county randomly selected three sample towns, good, medium and poor, a total of 9 sample towns. Thirdly, according to the distance of villages to the town government and the level of economic development, each sample village was randomly selected as good, medium and poor villages, totaling 27 sample villages. Then, 20 farmers were selected from the village roster according to the preset random number table as the investigation object. After the village cadres determined the time with the interviewed farmers in advance, 16 researchers with strict training went to the farmers’ homes for one-to-one investigation. Finally, a total of 540 effective peasant household questionnaires were obtained from 9 townships and 27 villages in 3 districts and counties, and the questionnaire recovery rate was 100% (Figures 2, 3).
The purpose of this paper is to investigate the impact of space-time perception of climate change on farmers’ adaptive behavior to climate change. Based on the research of Deressa et al. (2009); Lv and Chen (2010) and Chen et al. (2014) and combined with field research experience, this paper divides the adaptation measures of farmers to climate change into active adaptation and passive adaptation. The former includes adjusting crop type/variety, building infrastructure, learning about climate change technology, and going out to work because of climate change. The latter includes increasing pesticide/fertilizer, increasing irrigation, and adjusting crop time. If the farmer does not take active and passive adaptation measures, the value of “are you taking action on climate change” is 0, otherwise the value is 1. Among the 540 data of farmers, 478 households have adopted measures to cope with climate change, accounting for 88.51% of the total. According to Wheeler et al. (2021), farmers’ perception of climate change may differ at space-time scales. The core variable of this paper is time perception: “Do you think climate change will intensify in the next 5 years,” “Do you think climate change will intensify in the next 10 years,” and “Do you think climate change will intensify in the next 15 years.” Second, space perception: “How serious do you think the current global climate change impact is,” “How serious do you think the current global climate change impact on China,” “How serious do you think the current global climate change impact on Sichuan Province,” “How serious do you think the current global climate change impact on your village.”
In addition, considering that farmers’ decision-making on coping with climate change may be affected by a variety of other factors, this paper included the individual characteristics of respondents, family characteristics and meteorological disaster experience into the model as control variables. Among them, the personal characteristics of interviewees include gender, age, education level and other indicators of interviewees, which are generally considered to be related to farmers’ response to climate change (Wang et al., 2010; Habtemariam et al., 2016; Harvey et al., 2018). Household characteristics include household gross income per capita (Obayelu et al., 2014), arable land per capita (Abid et al., 2015) and other indicators. In addition, crop disaster experience may directly affect farmers’ adaptation behavior decisions to climate change (Rozaki et al., 2021). Therefore, “Have crops been damaged by the weather” is included in the model assessment as family experience. Finally, considering that regional differences may affect farmers’ responses to climate change, dummy variables of districts and counties are set to control the regression errors caused by regional differences. Variable definitions and basic statistics are shown in Table 1.
The above descriptive statistical analysis of farmers’ spatio-temporal perception of climate change and its adaptive behavior measures is difficult to distinguish the impact of climate change perception on farmers’ adaptive behavior at different time and spatial scales. In addition, farmers’ personal characteristics, family characteristics and regional factors may also influence their adaptive behaviors (Niles et al., 2013; Wood et al., 2014). Therefore, in order to better quantify the impact of various factors on farmers’ adaptation measures, this paper intends to adopt the following measurement model:
Equation 1 is a binary Logit model, where Pij is the probability of household i in village j taking measures to cope with climate change. If the farmers adopts adaptation measures (active adaptation or passive adaptation), the value is assigned to 1; otherwise, it is 0. β0 is a constant term; X1⋯Xi is the core independent variable, control variable and regional dummy variable; β1⋯βi is the regression coefficient; εis the residual term. Among them, there are 7 core independent variables, namely, farmers’ perception of climate change in the next 5, 10, and 15 years from time perception of climate change; Farmers’ perception of global, national, Sichuan province and their own village climate change in their perception of climate spatial change.
On the basis of Equations 1, 2 adds the product item Hi of the interaction term between time perception and space perception of climate change. Thus, the impacts of farmers’ perception of climate change at different time and spatial scales on their adaptive behaviors can be measured. In the formula, Qij is the probability of farmers i in village j taking measures against climate change. γ0 is a constant term; X1⋯Xi is the core independent variable, control variable and regional dummy variable; γ1γi, σ1σi is the regression coefficient; k is the residual term. In order to avoid possible multicollinearity problems, Hayes (2013) is referred to in this paper for centralized processing of Equation 2 to improve the accuracy of the model.
Equation 3 is similar to Equation 1. Mij is the probability of farmers i in village j taking measures to cope with climate change, and its values are 1, 2, 3 and 4, respectively, indicating that household does not take measures to climate change, only adopts active adaptive measures, only adopts passive measures, and both measures are adopted. ∂0 is a constant term; X1⋯Xi is the core independent variable, control variable and regional dummy variable; ∂1∂i is regression coefficient; τis the residual term.
Considering the difference of dependent variables in the equation, different estimation methods are adopted in this paper. Since the dependent variable in Equations 1, 2, namely whether farmers take countermeasures against climate change is a dichotomous variable, binary Logit model was used for regression in this study. In Equation 2, V1Vi represents farmers’ perception of global, national, provincial and village climate change. Time perception of climate change H1Hi is set to three dummy variables dum1 (farmers think climate change will intensify in the next 5 years is 1; otherwise, it is 0), dum2 (farmers think climate change will intensify in the next 10 years is 1; otherwise, it is 0), and dum3 (farmers think climate change will intensify in the next 15 years is 1; otherwise, it is 0). The three dummy variables were, respectively, multiplied with the four variables of spatial perception of climate change, and the interaction termsHiVi were included in Equation 1. In Equation 3, the types of coping measures of farmers are composed of four discrete values (1, 2, 3 and 4), which are independent of each other. Therefore, the MNL model (disordered multi-classification Logit model) is used for analysis in this paper. Firstly, the utility function of the decision maker is constructed, and it is assumed that the farmers choose to realize the principle of utility maximization. Then the maximum likelihood was used to estimate the model parameters, and the influence of different factors on different choices of farmers’ adaptive behavior was calculated. In order to reflect the influence of independent variables on dependent variables, Tables 2–4, report the marginal coefficients of the model and the standard errors of cluster at the county level.
Table 3. Logit regression results of space-time perception and moderating effect of farmers’ climate change.
Table 4. Impacts of time-space perception of climate change on farmers’ adaptive behavior selection.
What adaptation measures will farmers adopt in the context of climate change? Table 5 shows farmers’ choice of adaptation measures to climate change. The results showed that about 88.51% of the 540 peasant households had adopted measures to cope with climate change. Among them, 61.11% farmers adopted both passive adaptation and active adaptation measures. 7.59% of farmers only used active measures and 19.81% only used passive measures. This may be because the active measures require higher economic and cultural levels of farmers and are difficult to implement.
Among the farmers taking measures to cope with climate change (Figure 4), the highest rate of increase irrigation adoption is 23% in the passive adaptive measures, and the similar rate of increase fertilizer/pesticide application and adjusted crop time adoption is 17%. Among the active adaptation measures, the adoption rate of adjusting crop type/variety was the highest at 17%, while the number of people who chose to cope with climate change by out-migrating for work accounted for only 5%. In general, whether in active adaptation or passive adaptation, farmers tend to choose the measures with low economic and time costs. Increasing irrigation measures are the most frequently used response measures by farmers. This reflects that in the context of climate change, how to reduce the behavioral cost of farmers coping with climate change and improve the stability of irrigation water are important directions to improve farmers’ ability to cope with climate change.
In this paper, farmers’ perception of climate change can be divided into three categories: obvious perception, uncertain perception and not obvious perception. Specifically, when farmers’ perception score is 4/5, they are classified as “obvious perception” (type I), 3 as “uncertain” (type II), and 1/2 as “not obvious perception” (type III). Table 6 shows the descriptive statistical table of farmers’ perception of climate change in time and space. The results show that farmers’ perception of climate change in space is more obvious than their perception of climate change in time.
(1) Time perception of climate change: in type I, farmers’ time perception of climate change in the next 5 years is the most obvious, accounting for 50.19%, which is higher than 47.96 and 46.11% in the next 10 and 15 years. In type II, 42.78% of farmers are most difficult to determine their perception of climate change in the next 15 years, which is larger than 40.37 and 38.89% in the next 10 and 5 years. In type III, farmers’ perception of climate change in the next 5, 10, and 15 years has little difference. Comparatively speaking, farmers’ perception of climate change in the next 10 years is the least obvious, accounting for 11.67%, which is higher than that of 11.11 and 10.93% in the next 15 and 5 years. In conclusion, farmers’ perception of the time of short-range climate change is more obvious, and its perception degree decreases with time extension. The possible explanation for this is that farmers are more concerned about the recent situation and have higher cognitive accuracy than identifying long-term risks.
(2) Spatial perception of climate change: In type I, farmers’ spatial perception of global climate change is most obvious, accounting for 66.85%, which is higher than 61.85, 56.11, and 50.93% in China, Sichuan province and this village. In type II, the uncertainty of farmers’ spatial perception of climate change in Sichuan province accounts for the highest 31.30%, which is higher than 28.52, 25.56, and 24.07% in China, the world and the village. In type III, farmers’ perception of climate change varies greatly, especially their perception of climate change in their own village accounts for 25.00%, which is larger than 12.59% in Sichuan Province, 9.63% in China and 7.59% in the world. In conclusion, farmers’ spatial perception of long-distance climate change is more obvious, and their perception degree increases with the expansion of space. The possible explanation is that the phenomenon of climate change is more obvious in a larger region, and it is easier for farmers to obtain information related to this region through the Internet, TV and other channels.
Table 2 shows the regression results of time and space perception of climate change on farmers’ climate change response behaviors. Model 1–7 takes into account the influence of control variables and regional dummy variables on the premise that the core variables time perception and space perception of climate change are included. To better explain the results, we tested the marginal effect of each variable in Model 1–7.
In terms of time perception of climate change, the regression results of Model 1, 2 and 3 show that farmers’ time perception of climate change has a positive significance on their adaptive behavior decisions at 1% level, and there is a scale difference, that is, the next 5 years > the next 10 years > the next 15 years. When farmers’ perception of climate change increases by 1% in the next 5, 10, and 15 years, their probability of coping with climate change increases by 2.8, 2.4, and 2.3%, respectively. This may be because, influenced by factors such as knowledge level, personal experience and preference, farmers care more about immediate interests and neglect long-term situations (Ou, 2003; Peng, 2012). In terms of space perception of climate change, the regression results of Model 4, 5, 6 and 7 showed that farmers’ spatial perception of climate change had a positive impact on their adaptive behavior of climate change, and there was a scale difference, that is, global > China > local village. Among them, the climate change perception of global, Chinese and local villages has a positive impact on farmers’ adaptive behaviors at the levels of 5, 5, and 1%, respectively. Only the climate change perception of Sichuan province has no significant impact on farmers’ adaptive behaviors. When the farmers’ perception of global, Chinese and local climate change increased by 1%, the probability of taking response measures increased by 3.6, 3.5, and 2.4%, respectively. The explanation is that farmers are less sensitive to climate change in smaller regions because they have lived longer in their own villages and provinces than in large regions such as China and the world. In addition, farmers’ perception of regional climate change may also be affected by the difficulty in observing small-scale regional climate change and obtaining relevant information (Xu et al., 2015; Mei et al., 2019).
In terms of control variables, gender, household per capita income, and household per capita arable land area have positive effects on the adaptation behavior of farmers to climate change. The possible reason is that the higher the household income of farmers, the less restrictive effect the economic cost of coping measures has on farmers (Hisali et al., 2011; Akter et al., 2016). Age has negative and significant effect on farmers’ response to climate change at 5% level. This may be because the older the farmers are, the less able they are to acquire information and receive information, and the lower their sensitivity to climate change. Education level and disaster experience had no significant effect on farmers’ adaptation to climate change. This may be due to the limitation of sample characteristics.
The impact of each core independent variable on farmers’ adaptation to climate change has been verified above. Next, this paper discusses the impact difference of climate change perception on farmers’ adaptive behavior of climate change from three dimensions of climate change temporal perception, climate change spatial perception, and climate change temporal and spatial perception (Table 3).
Model 1 shows the results of only including climate change time perception and control variables and regional variables. Compared with Table 2, when the three core variables of climate change time perception are included in the regression, the estimation effect of the model is weakened. Only the climate change perception in the next 5 years has a positive impact on farmers’ adaptive behavior at 1% level. This may be because the perception of the time of climate change will significantly affect farmers’ adaptation to climate change, but farmers’ perception of future climate change is only a general cognition, and it is difficult to clearly distinguish the changes in different time periods. At the same time, because farmers pay more attention to the recent situation and are easier to identify, their perception of climate change in the next 5 years is more obvious, and even their perception may be confused with the perception of longer-term climate change. Model 2 shows the results of only including spatial perception and control variables and regional variables of climate change. Compared with Table 2, when the four core variables of spatial perception of climate change are included in the regression, the estimation effect of the model is not obvious. This may be because the spatial awareness of climate change will be obvious when farmers adapt to climate change behavior. However, when the global, China, Sichuan province and local village spatial perception variables of climate change are included in the model, they may influence each other and thus reduce the role of farmers in coping with climate change. For example, when farmers’ perception of climate change in their village or province is not obvious, and their information channels and cognition level are limited, they may think that changes in China and the world are not obvious either.
Model 3 shows the regression results of integrating temporal perception of climate change, spatial perception of climate change, control variables and regional variables. Compared with Model 1 and 2, when the seven core variables of climate change perception were included in the regression, the estimated effect of the Model changed little. Only the temporal perception of climate change in the next 5 years has a positive significant effect on farmers’ adaptive behavior decisions at 1% level. This indicates that farmers are more sensitive to the short-term risk perception under the temporal and spatial dimensions of climate change.
Based on Model 3, Model 4 shows the result after the interaction product and centralization of three time-sensing variables of climate change and four spatial variables. The results show that only the time perception of climate change in the next 5 years and the perception of climate change in the village have positive significance on the behavior decision of farmers’ adaptation to climate change at 5 and 10% levels. Among the interaction items, “Earth * F5,” “China * F5,” and “Village * F10” have significant negative impacts on farmers’ climate change response behaviors at 1, 5, and 1% levels, respectively. The impacts of “Village * F5” and “Village * F15” on farmers’ climate change response behaviors are significantly positive at 1% level, respectively. This indicates that farmers have a low perception of climate change in short time distance and short time distance, and may even have a negative response to climate change due to their underestimation of risks. However, in the case of farmers’ perception of near-spatial climate change, the fuzziness of their perception of far-temporal climate change has little impact on farmers, and farmers are still actively responding to climate change.
In terms of control variables, farmers’ age has a significant negative impact on their adaptation behavior to climate change, and per capita income and arable land area have a significant positive impact on farmers’ adaptation to climate change. Gender, disaster experience and education level have no significant impact on farmers’ adaptation to climate change. Therefore, more training should be given to farmers on climate change to improve their awareness and coping ability. At the same time, reduce the economic and time costs of farmers to cope with climate change.
It has been discussed above that farmers’ perception of time and space of climate change at different scales will affect their adaptive behaviors. In this context, what is the difference between farmers’ choice of measures to cope with climate change? To answer this question, this section uses the MNL model to take the initiative to adaptive, passive adaptive to the control group is not used, to discuss climate change awareness of time and space to I use only passive adaptive measures, II only with active adaptive measures and type III both measures adopted three to adapt to the effects of policy decision, Such as Table 4. The results show that the time perception of remote climate change has a more significant impact on farmers’ specific adaptation measures, while the national and provincial climate change perception has no significant impact on farmers. Specifically:
(1) In terms of climate change time perception, the impact of climate change perception on farmers’ adaptation measures in the next 5 years is not obvious. In the next 10 years, the perception of climate change for farmers to adopt passive measures and active measures is positively significant at 5 and 1% level, respectively, but the perception of climate change for farmers to adopt both measures is negatively significant at 5% level. When farmers’ perception of climate change in the next 10 years increases by 1%, the probability of adopting passive adaptation measures and active adaptation measures increases by 13.2 and 9.6%, respectively, and the probability of adopting both measures decreases by 16.6%. What’s interesting is that the perception of climate change over the next 10 and 15 years is the opposite. In the next 15 years, farmers’ perception of climate change is negatively significant at the level of 1% for passive measures and 5% for active measures, but positively significant at the level of 1% for both measures. For each 1% increase in farmers’ perception of climate change in the next 15 years, the probability of adopting passive adaptation measures and active adaptation measures will decrease by 13.5 and 6.9%, respectively, and the probability of adopting both measures will increase by 17.4%. This may indicate that when specific measures are taken to cope with climate change, farmers have no obvious preference to cope with short-term climate change. However, when dealing with the risk of medium-term climate change in the future (the next 10 years), farmers choose to adopt certain targeted measures because they have a certain level of awareness of the risk. When dealing with the long-term threat of climate change (the next 15 years), the difficulty of predicting the risk leads them to prefer both measures, thus reducing the potential damage.
(2) In terms of spatial perception of climate change, the impact of climate change perception in China and Sichuan province on farmers’ adaptation measures is not obvious. The perception of global climate change for farmers only adopting active adaptation measures and adopting both measures is significantly negative and positive at 1% level, respectively. When the farmers’ perception of global climate change increased by 1%, the probability of adopting active adaptation measures decreased by 5.5%, and the probability of adopting both measures increased by 12.0%. The climate change perception of the village is positively significant to the passive adaptation measures adopted by farmers at 1% level. When farmers’ perception of climate change increased by 1%, the probability of adopting passive adaptation measures increased by 5.9%. This may indicate that in response to the large-scale climate change crisis (global), farmers’ willingness to adopt both active and passive adaptation measures for personal life and property, production and operation has been greatly enhanced. However, when farmers perceive only small scale climate change (their village), they tend to choose only passive adaptation measures. This may be because farmers underestimate the threat of small-scale climate change or the effectiveness of individual coping behaviors, and tend to choose only passive adaptation measures with low time and economic cost.
In terms of control variables, age of individual characteristics was negatively significant to both measures at the level of 10%. Education level is only passive adaptive measure, and both measures are negative significant at 5% level and positive significant at 1% level, respectively. The per capita arable land area and crop disaster experience of households were negatively significant at the level of 5% and positively significant at the level of 1%. In addition, the per capita arable land has a significant positive impact on the passive adaptation measures adopted by farmers. This may indicate that households with larger per capita land area and disaster experience have higher awareness of climate change risk prevention and higher willingness to adopt active and passive adaptation measures.
Based on the survey data of 540 farmers in Sichuan Province, China, this study used binary Logit model and Multinomial Logistic Regression model (MNL) to explore the effects of farmers’ space-time perception of climate change and their interaction effects on their adaptation behavior to climate change. Compared with previous studies, the marginal contribution of this paper is as follows: First, from the perspective of farmers’ space-time perception of climate change, the impact of climate change on farmers’ response to climate change is analyzed based on scale effect. Secondly, Logit model were used to quantify the space-time scale differences of farmers’ perception of climate change. In addition, MNL model was used to discuss the impact of different spatial and temporal climate change perceptions on farmers’ adaptive measures. Thirdly, representative micro-individual data were used to focus on the behavior of farmers in rural areas of China in response to climate change, so as to provide reference for the formulation of climate change policies in China and other developing countries.
Global climate change is an indisputable fact (Hase et al., 2021), which will have a huge impact on human production and life. Horowitz (2009) showed that there was a strong negative correlation between national per capita GDP and temperature, and global GDP would decrease by 3.8% for every 1°C increase in national temperature. Climate change also threatens global environmental governance, national food security, and children’s nutritional health (McMahon and Gray, 2021; Baste and Watson, 2022). In response to the threat of climate change, 153 countries and the European Community signed the United Nations Framework Convention on Climate Change (UNFCCC) in 1992, hoping to control the rate of global warming by reducing greenhouse gas emissions. Since then, the international community has held many meetings calling on countries to actively change climate and discharge carbon dioxide within the agreed limits. However, the climate change crisis appears to be more severe than predicted. According to the latest assessment report from the Intergovernmental Panel on Climate Change, global surface temperatures in the 21st century will exceed 1.5 and 2°C without deep controls on emissions of greenhouse gases such as carbon dioxide. Climate change is a matter of human welfare and equity, and the efforts of governments at all levels, the private sector and the public are indispensable.
China has always played an important role in promoting global climate governance. From 1998, when China officially promulgated the Energy Conservation Law of the People’s Republic of China, to 2007, when China explicitly proposed to “strengthen capacity building to cope with climate change and make contribution to global climate protection,” to 2020, when China proposed the “dual carbon target,” China’s participation in the global cause of climate change mitigation has been on the rise (Hu, 2012). Although all sectors of society attach great importance to the response to climate change, residents, especially small farmers, as the main body of adaptation to climate change, are not optimistic about the perception and response to climate change. Low awareness of climate change, high cost of adaptation measures and poor response effect restrict the implementation of relevant policies at the micro individual level. Therefore, how to help farmers out of the plight of climate change will have far-reaching significance for global climate governance.
In the context of intensified climate change, the academic community has conducted extensive research on the adaptation behavior of farmers to climate change. However, in general, these factors are mostly concentrated in the gender, age, economic income and other aspects of farmers, and few studies have explored from the perspective of space-time perception of climate change. In the only studies, either only focus on the trend of climate change at the macro level, or mostly adopt qualitative analysis methods, or only analyze the perception of farmers’ climate change in single dimension of time or space. For example, Yu et al. (2011) used descriptive statistics to study the perception intensity of local precipitation and temperature changes in the past 50 years by residents of different age groups in Shaanxi. Wang and Yu (2015) used the perception intensity formula to show that there were differences in the accuracy of residents’ climate perception in different small areas in southern Shaanxi. In Hanzhong, Ankang, and Shangluo, the consistency rates between the perceived precipitation change and the measured precipitation change were 100, 44.4 and 66.7%, respectively. In general, the existing research still lacks the quantitative analysis of micro-subject climate change perception in multiple dimensions. Therefore, this paper used binary Logit model and MNL model to explore the impact of farmers’ spatio-temporal climate change perception and their interaction effects on farmers’ climate change adaptive behavior.
There are still some shortcomings in this study, which can be further expanded in future research. For example, differences in the impact of spatio-temporal perception of farmers’ climate change on farmers’ response to climate change under different climate types and meteorological natural disasters and the reasons can be discussed. Secondly, the spatial and temporal perception intensity of climate change and its impact on the adoption of specific measures can be further studied in different dimensions. Thirdly, from the perspective of farmers’ perception of climate change in time and space in the past, it can be discussed whether it will affect their current climate change adaptive behavior and the difference in impact. Finally, there may be a “perception-behavior” cycle affecting the temporal and spatial perception of climate change among farmers. For example, farmers have a strong perception of climate change risks in the past 5 years and may take active measures to deal with them. The guarantee of corresponding measures may reduce the current perception of climate change risk and the enthusiasm of farmers to cope with it. The mechanism and characteristics of “perception-behavior” in the space-time perception of climate change of farmers can be further studied.
Based on the survey data of 540 farmers in Sichuan Province, China, this study used binary Logit model and MNL model to explore the effects of farmers’ space-time perception of climate change and their interaction effects on farmers’ adaptation behavior to climate change. The results showed that: (1) 88.51% of farmers took adaptation measures to climate change, and 61.11% of them took both passive and active adaptation measures. (2) The scale difference of farmers’ time and space perception of climate change has a significant positive impact on their adaptive behavior of climate change. In terms of time: climate change perception in the next 5 years > in the next 10 years > in the next 15 years. In terms of space: farmers’ perception of climate change is global > national > local village (the perception of local province is not obvious). (3) Farmers’ space-time perception of climate change significantly affects farmers’ adaptive behavior. Among them, “farmers’ perception of climate change in the next 5 years” and their own “village’s perception of climate change” play an important role.
This paper has important implications for guiding farmers to effectively adapt to climate change, and its conclusions are also applicable to other developing countries. (1) The state should improve policies related to climate change and issue regulations to support farmers in coping with climate change. The study shows that farmers may make blind choices when making decisions on climate change response measures due to the influence of time and economic cost. The state should attach importance to the construction and maintenance of rural infrastructure and strengthen the funding support for infrastructure construction of grassroots governments. We will formulate overall reference standards for rural infrastructure construction, urge local governments to implement them in light of local conditions, and supervise them by setting up responsibility mechanisms and supervision groups. At the same time, the state also should increase investment in rural infrastructure and provide certain economic subsidies to farmers responding to climate change, so as to enhance their willingness to cope with climate change. (2) Local governments should combine local characteristics to develop green and low-carbon agricultural products and reduce greenhouse gas emissions from production, logistics and packaging of agricultural products while implementing orders from higher authorities. The study found that the excessive use of chemical fertilizers in the adaptation process of farmers to climate change will increase carbon dioxide emissions and deteriorate soil properties. In addition, at present, farmers mostly adopt passive adaptive measures such as increasing irrigation and adjusting farming time. By buying agricultural insurance and planting subsidies, the local government can guide them to take measures to deal with climate change. In addition, the government should give play to the demonstration and guidance role of community-level agricultural technology departments and major farmers in villages, strengthen training and services in agricultural technology for farmers, reduce their blind application of chemical fertilizers and pesticides, and improve their ability to adapt to climate change in a scientific way. (3) Village collectives should actively implement the instructions of their superiors and expand climate change-related knowledge training for farmers. In our survey, farmers paid more attention to the immediate situation and had no obvious perception of long-term threat of climate change and surrounding environment. In this regard, the village’s collectives should use radio, mobile phones, brochures and other media to strengthen publicity on the long-term potential risks of climate change and surrounding environmental changes. Keep a close eye on climate change information. For example, when disasters such as flood and drought are likely to occur in the local area, inform households in time and assist farmers to take preventive measures. (4) Farmers should actively respond to the call of the government, actively participate in village collective climate change related training sessions. They are supposed to close and village cadres, neighbors and other groups of communication and other ways, effectively expand the family information channels. Thus, they can strengthen their own green production awareness and take appropriate measures to deal with climate change.
The original contributions presented in this study are included in the article/supplementary material, further inquiries can be directed to the corresponding author.
The studies involving human participants were reviewed and approved by School of Management, Sichuan Agricultural University. The patients/participants provided their written informed consent to participate in this study. Written informed consent was obtained from the individual(s) for the publication of any potentially identifiable images or data included in this article.
JM and DX: conceptualization, writing—original draft preparation, and writing—review and editing. JM, WZ, and DX: methodology. JM, SG, and JS: formal analysis. XD, JS, and DX: investigation. DX: supervision and funding acquisition. All authors read and agreed to the published version of the manuscript.
The authors declare that the research was conducted in the absence of any commercial or financial relationships that could be construed as a potential conflict of interest.
All claims expressed in this article are solely those of the authors and do not necessarily represent those of their affiliated organizations, or those of the publisher, the editors and the reviewers. Any product that may be evaluated in this article, or claim that may be made by its manufacturer, is not guaranteed or endorsed by the publisher.
Abid, M., Scheffran, J., Schneider, U. A., and Ashfaq, M. J. E. S. D. (2015). Farmers’ perceptions of and adaptation strategies to climate change and their determinants: the case of Punjab province, Pakistan. Earth Syst. Dyn. 6, 225–243. doi: 10.5194/esd-6-225-2015
Abid, M., Schneider, U. A., and Scheffran, J. (2016). Adaptation to climate change and its impacts on food productivity and crop income: perspectives of farmers in rural Pakistan. J. Rural Stud. 47, 254–266. doi: 10.1016/j.jrurstud.2016.08.005
Akter, S., Krupnik, T. J., Rossi, F., and Khanam, F. (2016). The influence of gender and product design on farmers’ preferences for weather-indexed crop insurance. Glob. Environ. Change 38, 217–229. doi: 10.1016/j.gloenvcha.2016.03.010
Bar-Anan, Y., Liberman, N., and Trope, Y. (2006). The association between psychological distance and construal level: evidence from an implicit association test. J. Exp. Psychol. Gen. 135:609. doi: 10.1037/0096-3445.135.4.609
Baste, I. A., and Watson, R. T. (2022). Tackling the climate, biodiversity and pollution emergencies by making peace with nature 50 years after the Stockholm Conference. Glob. Environ. Change 73:102466. doi: 10.1016/j.gloenvcha.2022.102466
Bo, F., and Zhuang, G. Y. (2018). Evolution and phased characteristics of China’s climate change policy. Collect 6:24.
Chai, Q. M., Guo, H. Y., Liu, C. Y., Dong, L., Ju, L. X., Liu, C. S., et al. (2020). Global Climate Change and China’s Action Plan – China’s Climate Governance during the 14th Five-Year Plan period (In writing). J. Yuejiang Univ. 12, 36–58.
Chao, Q. C., Yan, Z. W., Sun, Y., Jiang, Z. H., Liao, H., Jia, G. S., et al. (2020). New scientific understanding of climate change in China. China Popul. Resour. Environ. 30, 1–9.
Chen, H., Wang, J., and Huang, J. (2014). Policy support, social capital, and farmers’ adaptation to drought in China. Glob. Environ. Change 4, 193–202. doi: 10.1016/j.gloenvcha.2013.11.010
Clayton, S. (2020). Climate anxiety: psychological responses to climate change. J. Anxiety Disord. 74:102263. doi: 10.1016/j.janxdis.2020.102263
D’Argembeau, A., Renaud, O., and Van der Linden, M. (2011). Frequency, characteristics and functions of future-oriented thoughts in daily life. Appl. Cogn. Psychol. 25, 96–103. doi: 10.1002/acp.1647
Deressa, T. T., Hassan, R. M., Ringler, C., Alemu, T., and Yesuf, M. (2009). Determinants of farmers’ choice of adaptation methods to climate change in the Nile Basin of Ethiopia. Glob. Environ. Change 19, 248–255. doi: 10.1016/j.gloenvcha.2009.01.002
Dietz, T., Shwom, R. L., and Whitley, C. T. (2020). Climate change and society. Annu. Rev. Sociol. 46, 135–158. doi: 10.1146/annurev-soc-121919-054614
Fan, M., Peng, Y., Wang, Q. H., Mi, K., and Qing, F. T. (2018). Relationship between landscape pattern and plant diversity and its spatial scale effect: a case study of otindag Sandy Land. Acta Ecol. Sin. 8, 2450–2461.
Feng, S., and Xu, C. L. (2014). Review of global climate change and its Impact on human society and economy. Popul. Resour. Environ. China 24, 6–10.
Feng, X. L., Chen, Z. X., and Huo, X. X. (2016). An empirical study on farmers’ adaptive behavior under drought conditions: data from 1079 apple farmers. J. Arid Land Resour. Environ. 3, 43–49.
Fosu-Mensah, B. Y., Vlek, P. L., and MacCarthy, D. S. (2012). Farmers’ perception and adaptation to climate change: a case study of Sekyedumase district in Ghana. Environ. Dev. Sustain. 14, 495–505. doi: 10.1007/s10668-012-9339-7
Fu, B. J. (2014). Approaches and methods of integrated geographical research: coupling of pattern and process. Acta Geogr. Sin. 69:578.
Gao, X., Li, G. C., and Yin, C. J. (2021). Household adaptive behavior and its impact on grain yield per unit area under climate change. J. China Agric. Univ. 26, 240–248.
Guan, W. H., Peng, X., Zhang, H., and Wei, Y. H. (2015). Regional economic disparities in Jiangsu Province at different spatial scales. Resour. Environ. Yangtze Basin 24:2003R2011. doi: 10.1016/j.jenvman.2020.110901
Habtemariam, L. T., Gandorfer, M., Kassa, G. A., and Heissenhuber, A. (2016). Factors influencing smallholder farmers’ climate change perceptions: a study from farmers in Ethiopia. Environ. Manag. 58, 343–358. doi: 10.1007/s00267-016-0708-0
Harvey, C. A., Saborio-Rodríguez, M., Martinez-Rodríguez, M. R., Viguera, B., Chain-Guadarrama, A., Vignola, R., et al. (2018). Climate change impacts and adaptation among smallholder farmers in Central America. Agric. Food Security 7, 1–20. doi: 10.1186/s40066-018-0209-x
Hase, V., Mahl, D., Schäfer, M. S., and Keller, T. R. (2021). Climate change in news media across the globe: an automated analysis of issue attention and themes in climate change coverage in 10 countries (2006–2018). Glob. Environ. Change 70:102353. doi: 10.1016/j.gloenvcha.2021.102353
Hayes, A. F. (2013). Introduction to mediation, moderation, and conditional process analysis: a regression-based approach. New York, ny: the guilford press. J. Educ. Meas. 51, 335–337. doi: 10.1111/jedm.12050
Hisali, E., Birungi, P., and Buyinza, F. (2011). Adaptation to climate change in Uganda: evidence from micro level data. Glob. Environ. Change 21, 1245–1261. doi: 10.1016/j.gloenvcha.2011.07.005
Horowitz, J. K. (2009). The income-temperature relationship in a cross-section of countries and its implications for global warming. Environ. Resour. Econ. 44, 475–493. doi: 10.1007/s10640-009-9296-2
Hossain, M. S., Alam, G. M., Fahad, S., Sarker, T., Moniruzzaman, M., and Rabbany, M. G. (2022). Farmers’ willingness to pay for flood insurance as climate change adaptation strategy in northern Bangladesh. J. Clean. Product. 2022:130584. doi: 10.1016/j.jclepro.2022.130584
Hu, A. G. (2012). China’s climate change policies: background, goals and debates. Country Rep. 12:2009.
Islam, M. M., Barnes, A., and Toma, L. (2013). An investigation into climate change scepticism among farmers. J. Environ. Psychol. 34, 137–150. doi: 10.1016/j.jenvp.2013.02.002
Jin, J., Wang, X., and Gao, Y. (2015). Gender differences in farmers’ responses to climate change adaptation in Yongqiao District, China. Sci. Total Environ. 538, 942–948. doi: 10.1016/j.scitotenv.2015.07.027
Kobayashi, H., Watando, H., and Kakimoto, M. (2014). A global extent site-level analysis of land cover and protected area overlap with mining activities as an indicator of biodiversity pressure. J. Clean. Product. 84, 459–468. doi: 10.1016/j.jclepro.2014.04.049
Lee, P. S., Sung, Y. H., Wu, C. C., Ho, L. C., and Chiou, W. B. (2020). Using episodic future thinking to pre-experience climate change increases pro-environmental behavior. Environ. Behav. 52, 60–81. doi: 10.1177/0013916518790590
Li, J. F., and Chai, Q. M. (2017). The significance of the entry into force of the paris agreement. World Environ. 1, 16–18.
Li, Y., and Zhao, S. S. (2022). Flood disaster loss and disaster risk in China from 2001 to 2020. Adv. Clim. Change Res. 18:12
Lin, F., Chen, X. W., Yao, W. Y., Fang, Y. H., Deng, H. J., Wu, J. F., et al. (2020). Multi-time scale analysis of water conservation in forest discontinuous watershed based on swat model. Acta Geogr. Sin. 75:14.
Liu, Q., and Zhang, Z. Y. (2015). The significance of Spatial scale – Reflections on the planning of residential public facilities in Zhuhai under the neighborhood Center model. Urban Plann. Rev. 9, 45–52.
Liu, Y. H., Li, Y. H., and Wang, W. T. (2021). Challenges, opportunities and actions for China to achieve “dual carbon” goals. China Popul. Resour. Environ. 9:57.
Lv, Y. R., and Chen, S. F. (2010). Farmers’ perception of climate change and analysis of adaptive behaviors. China Rural Econ. 07, 75–86. doi: 10.1007/s00267-016-0742-y
Malhi, Y., Franklin, J., Seddon, N., Solan, M., Turner, M. G., Field, C. B., et al. (2020). Climate change and ecosystems: Threats, opportunities and solutions. Philos. Trans. R. Soc. B 375:20190104. doi: 10.1098/rstb.2019.0104
McMahon, K., and Gray, C. (2021). Climate change, social vulnerability and child nutrition in South Asia. Glob. Environ. Change 71:102414. doi: 10.1016/j.gloenvcha.2021.102414
Mei, J. M., Huang, X. H., Zhou, R. Z., Ren, Y. H., Hou, L., and Hao, W. Y. (2019). Perception and adaptation of farmers and herdsmen to climate change at different altitudes. Acta Ecol. Sin. 39:7805R7814.
Niles, M. T., Lubell, M., and Haden, V. R. (2013). Perceptions and responses to climate policy risks among California farmers. Glob. Environ. Change 23, 1752–1760. doi: 10.1016/j.gloenvcha.2013.08.005
Obayelu, O. A., Adepoju, A. O., and Idowu, T. (2014). Factors influencing farmers’ choices of adaptation to climate change in Ekiti State, Nigeria. J. Agric. Environ. Int. Dev. 108, 3–16. doi: 10.12895/jaeid.20141.140
Ogunbode, C. A., Demski, C., Capstick, S. B., and Sposato, R. G. (2019). Attribution matters: revisiting the link between extreme weather experience and climate change mitigation responses. Glob. Environ. Change 54, 31–39. doi: 10.1016/j.gloenvcha.2018.11.005
Ou, X. O. (2003). Research on Agricultural Land Use and Land Conversion in Huaihua City. Dissertation. Changsha: Hunan Normal University.
Pahl, S., Sheppard, S., Boomsma, C., and Groves, C. (2014). Perceptions of time in relation to climate change. Wiley Interdiscip. Rev. Clim. Change 5, 375–388. doi: 10.1002/wcc.272
Peng, D. Y. (2012). The cooperative nature of Chinese farmers – with comments on Professor Cao Jinqing’s opinion. Rural Discov. China 3:5.
Phuong, L. T. H., Biesbroek, G. R., Sen, L. T. H., and Wals, A. E. (2018a). Understanding smallholder farmers’ capacity to respond to climate change in a coastal community in Central Vietnam. Clim. Dev. 10, 701–716. doi: 10.1080/17565529.2017.1411240
Phuong, L. T. H., Wals, A., Sen, L. T. H., Hoa, N. Q., Van Lu, P., and Biesbroek, R. (2018b). Using a social learning configuration to increase Vietnamese smallholder farmers’ adaptive capacity to respond to climate change. Local Environ. 23, 879–897. doi: 10.1080/13549839.2018.1482859
Pielke, R. Jr., Burgess, M. G., and Ritchie, J. (2022). Plausible 2005–2050 emissions scenarios project between 2° C and 3° C of warming by 2100. Environ. Res. Lett. 17:024027. doi: 10.1088/1748-9326/ac4ebf
Rayamajhee, V., Guo, W., and Bohara, A. K. (2021). The perception of climate change and the demand for weather-index microinsurance: evidence from a contingent valuation survey in Nepal. Clim. Dev. 14, 557–570. doi: 10.1080/17565529.2021.1949574
Rozaki, Z., Wijaya, O., Rahmawati, N., and Rahayu, L. (2021). Farmers’ disaster mitigation strategies in Indonesia. Rev. Agric. Sci. 9, 178–194. doi: 10.7831/ras.9.0_178
Shi, X. M. (2016). Research progress on public perception of climate change and adaptive behavior. Bull. Soil Water Conserv. 36:8.
Spence, A., Poortinga, W., and Pidgeon, N. (2012). The psychological distance of climate change. Risk Anal. Int. J. 32, 957–972. doi: 10.1111/j.1539-6924.2011.01695.x
Sun, S., Li, D., Liu, L. L., Wang, Z. Y., and Yin, Y. Z. (2017). Global major synoptic and climatic events in 2016 and their causes. Meteorol. Mthly 43, 477–485.
Thinda, K. T., Ogundeji, A. A., Belle, J. A., and Ojo, T. O. (2020). Understanding the adoption of climate change adaptation strategies among smallholder farmers: evidence from land reform beneficiaries in South Africa. Land Use Policy 99:104858. doi: 10.1016/j.landusepol.2020.104858
Trope, Y., and Liberman, N. (2010). Construal-level theory of psychological distance. Psychol. Rev. 117, 440–463. doi: 10.1037/a0020319
Waldman, K. B., Blekking, J. P., Attari, S. Z., and Evans, T. P. (2017). Maize seed choice and perceptions of climate variability among smallholder farmers. Glob. Environ. Change 47, 51–63. doi: 10.1016/j.gloenvcha.2017.09.007
Wang, C. Y., and Yu, Y. C. (2015). Spatial-temporal variation of people’s perception of climate change in southern Shaanxi. Chinese Agric. Sci. Bull. 31, 244–251.
Wang, J., Mendelsohn, R., Dinar, A., and Huang, J. (2010). How Chinese farmers change crop choice to adapt to climate change. Clim. Change Econ. 1, 167–185. doi: 10.1142/S2010007810000133
Wang, Y. J., Zhou, B. T., Ren, Y. Y., and Sun, C. H. (2016). Impacts of global climate change on China’s climate security. J. Appl. Meteorol. 27, 750–758.
Wheeler, S. A., Nauges, C., and Zuo, A. (2021). How stable are Australian farmers’ climate change risk perceptions? New evidence of the feedback loop between risk perceptions and behaviour. Glob. Environ. Change 68:102274. doi: 10.1016/j.gloenvcha.2021.102274
Wood, S. A., Jina, A. S., Jain, M., Kristjanson, P., and DeFries, R. S. (2014). Smallholder farmer cropping decisions related to climate variability across multiple regions. Glob. Environ. Change 25, 163–172. doi: 10.1016/j.gloenvcha.2013.12.011
Xu, B., Li, X. H., and Xue, Y. T. (2019). Drought disaster risk analysis and zoning in Sichuan Province. Climate Change Res. Lett. 8:571.
Xu, X. X., Yu, J., and Lei, X. (2015). Cognitive processing components of imagining the future and their brain networks. Adv. Psychol. Sci. 23:394.
Yu, Y. C., Zhou, Q., and Wang, C. Y. (2011). Spatial-temporal variation of public perception of climate change in Northern Shaanxi Province. J. Northwest Univ. 41, 134–138.
Zhang, F., Yushanjiang, A., and Jing, Y. (2019). Assessing and predicting changes of the ecosystem service values based on land use/cover change in Ebinur Lake Wetland National Nature Reserve, Xinjiang, China. Sci. Total Environ. 656, 1133–1144. doi: 10.1016/j.scitotenv.2018.11.444
Zhang, W. J., Yan, S. J., Zhang, J., Jiang, Y., and Deng, A. X. (2021). Win-win strategy of national food security and agricultural dual carbon goals. Sci. Agric. Sin. 54, 3892–3902.
Zhang, Y. S., Wu, D. T., and Lv, X. (2020). Effects of land use/cover change on ecosystem services: a review from a spatial perspective. J. Nat. Resour. 35, 1172–1189.
Zhao, X. Y. (2014). Review of research on farmers’ perception and adaptation to climate change. Chinese J. Appl. Ecol. 25, 2440–2448.
Keywords: space-time perception of climate change, adaptive behavior, scale effect, farmers, China
Citation: Ma J, Zhou W, Guo S, Deng X, Song J and Xu D (2022) Space-time perception and behavioral response of farmers to climate change: Evidence from Sichuan Province, China. Front. Ecol. Evol. 10:998945. doi: 10.3389/fevo.2022.998945
Received: 20 July 2022; Accepted: 08 August 2022;
Published: 21 September 2022.
Edited by:
Wei Shui, Fuzhou University, ChinaReviewed by:
Hua Lu, Jiangxi University of Finance and Economics, ChinaCopyright © 2022 Ma, Zhou, Guo, Deng, Song and Xu. This is an open-access article distributed under the terms of the Creative Commons Attribution License (CC BY). The use, distribution or reproduction in other forums is permitted, provided the original author(s) and the copyright owner(s) are credited and that the original publication in this journal is cited, in accordance with accepted academic practice. No use, distribution or reproduction is permitted which does not comply with these terms.
*Correspondence: Dingde Xu, ZGluZ2RleHVAc2ljYXUuZWR1LmNu
Disclaimer: All claims expressed in this article are solely those of the authors and do not necessarily represent those of their affiliated organizations, or those of the publisher, the editors and the reviewers. Any product that may be evaluated in this article or claim that may be made by its manufacturer is not guaranteed or endorsed by the publisher.
Research integrity at Frontiers
Learn more about the work of our research integrity team to safeguard the quality of each article we publish.