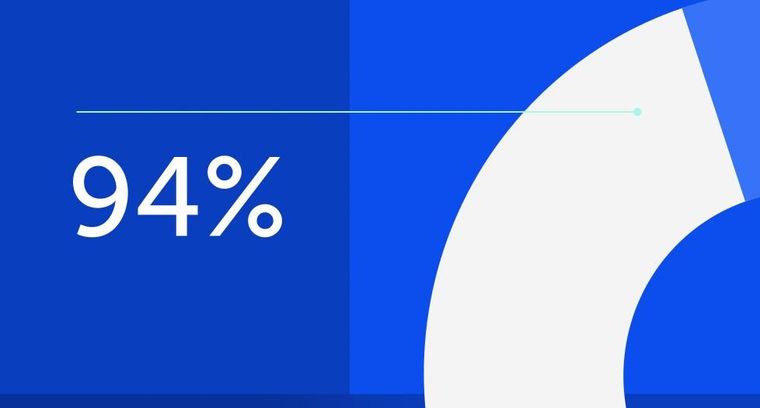
94% of researchers rate our articles as excellent or good
Learn more about the work of our research integrity team to safeguard the quality of each article we publish.
Find out more
ORIGINAL RESEARCH article
Front. Ecol. Evol., 06 September 2022
Sec. Environmental Informatics and Remote Sensing
Volume 10 - 2022 | https://doi.org/10.3389/fevo.2022.990037
This article is part of the Research TopicDigital Resource Vitalization and Urban Intelligent ComputingView all 6 articles
As cities are the main source of carbon emissions for human-social systems, clarifying the characteristics of carbon emission structure and distribution in urban areas is an important foundation for achieving carbon neutrality of cities and also an important challenge for human-social systems to achieve global carbon balance goals. The spatial utilization of cities is often characterized by the agglomeration of construction land, population concentration, and industrial production, with high carbon emission intensity and large total amount. The current research on the quantification of regional carbon emissions is mainly in two categories, namely, bottom-up calculation method system based on emission inventory and top-down method system based on energy balance and input-output model. However, how to clarify both the total regional carbon emissions and their spatial distribution has been a difficult problem in the field of carbon emission quantification. Based on the comprehensive consideration of these two aspects, this study tries to construct an approach that combines the top-down carbon emission measurement method with the bottom-up spatialization process. The total carbon emissions of the human-society system are specified to each land patch and, thereby, the carbon emission pattern of each emission sector in the city could be determined. In this study, we quantified the carbon emissions of Nanjing in 2020 and obtained the spatial pattern of carbon emissions in this city based on land use. The results showed that the carbon emission intensity of the main urban area of Nanjing was much higher than that of other districts, while energy consumption of the industrial production system was the main source of carbon emissions in the human-social system there. The method of this study has a relatively wide applicability and can help researchers and governments to clarify the quantity and location of their carbon emissions clearly, which is meaningful for the implementation of urban carbon reduction strategies.
Achieving carbon balance is one of the most important challenges and tasks facing the development of human society worldwide (Randerson, 2013; Hong et al., 2021). Cities are the main sites of carbon generation and emission in the human-social system; thus, the carbon source and sink characteristics of cities are one of the basic and important research areas for their regulation to achieve urban carbon balance. The shape of future urban development, the development of agglomerative, and clustered urban agglomerations is the main trend of future urban development, which is the core meaning of discussing and considering carbon balance under the scale of urban agglomerations (Yan et al., 2018). The development pattern of urban agglomerations is an intensive development pattern of the human-social system that is partially self-sufficient in a certain land space by interacting with relatively dense urban space and natural ecological space with sufficient supporting capacity, which is the direction of high-performance development of social economy and also puts forward extremely high requirements on the supporting capacity of natural ecological system and proximity resource and energy supporting system (Seneviratne et al., 2016; Nemeth-Durko, 2020). From the perspective of achieving regional carbon balance, although climate change and carbon neutrality are worldwide issues (Acheampong et al., 2022), the underlying logic is that if all perspectives of society can plan their socio-ecological systems with the goal of self-sufficiency, then the carbon neutrality of the whole world means the carbon neutrality of each country and each region (Zhang et al., 2019).
Cities have probably become the direction of human-social system development all over the world. The agglomeration of cities means the highest possible input-output efficiency and the most intensive land use. Cities are actually a viable strategy for urban and regional development under current resource constraints, climate change constraints, and sustainable development goals (Wang et al., 2022). The resource and energy consumption, ecological load, and additional redundancy from a distance corresponding to concentrating a larger population on a relatively small plot of land are likely to be far less than those of a diffuse urban layout, which may be the fundamental reason for the development of urban agglomerations worldwide. Thus, it is also of great relevance to discuss the carbon balance at the urban scale.
Regional carbon emission accounting, assessment, and land-based spatial patterns of carbon sources are of great significance for realistic applications (Herrero et al., 2016), influencing and driving key factors of urban agglomerations on their carbon balance patterns and the resulting development strategies for urban agglomerations (Chen et al., 2022). Energy carbon emissions are closely related to the level of socioeconomic development, controlling energy carbon emissions or figuring out the intensity and pattern of energy carbon emissions is extremely important for human society to control its own greenhouse gas load (Shan et al., 2017), and the intensity and pattern distribution of energy carbon emissions are often close to or completely consistent with the pattern of urban construction (IPCC, 2019; Yang et al., 2020).
From an efficient point of view, controlling the area of construction land and increasing the carbon emission per unit of construction land area are a feasible land strategy to control human carbon emissions (Stechemesser and Guenther, 2012). The premise of this strategy is that we can know the pattern of construction land and the pattern of energy carbon emission more clearly, and based on the spatial expression of land use, we can know the distribution and change of carbon emission on specific land parcels (Zmami and Ben-Salha, 2020; Ong et al., 2021). This is very consistent with the requirement of carbon neutrality. Although the scale of carbon neutrality is generally global, national, or at least an administrative region, it is still significant to analyze carbon sources and sinks at the scale of land parcels with a carbon neutrality perspective, which helps us to look at the land use types on specific land parcels with a carbon neutrality perspective, and consider the future development direction of each type of land parcel with a carbon neutrality purpose. This also provides a very basic data structure and methodological support for the regional land strategy.
With this overall consideration, this article combines the top-down calculation method with the bottom-up spatial analysis for the human-social system, which covers carbon emission calculation models for different industrial types, energy structures, and living patterns in agriculture, industry, inner-city, and rural areas within the administrative boundary of the city, and also integrates various data sources such as energy balance sheets, socioeconomic statistical yearbooks, and land-use data. We chose the Yangtze River Delta urban agglomeration region (Sun et al., 2010), which has the highest degree of urbanization development and the most drastic changes in the urbanization process in China. For the specific study case, we chose a typical metropolis, Nanjing, the capital city of Jiangsu Province, to conduct specific research on carbon emission calculation and spatialization analysis (Qiao et al., 2016).
The carbon emissions from the human-social system mainly include those from the industrial production system, those from the agricultural production system from human life, and those from the urban or rural support human life system, mainly from direct consumption or combustion of energy, and a smaller part comes from the synthetic link of industrial production, such as cement manufacturing. The total amount and proportion of this part of carbon emissions are small, so they are not included in the calculation boundary. The sources of carbon emissions from human-social systems have different energy consumption characteristics and spatial distribution structures. In this study, we have used the concept of categorical calculation-stratified spatialization-overlay aggregation. The specific calculation and spatial visualization framework are shown in Figure 1.
Figure 1. The framework for regional carbon emission calculation and visualization of human-social system.
Considering the calculation purpose to clarify the overall performance of carbon emissions, a calculation perspective based on the consumption side is therefore adopted (Ong et al., 2021). The calculation of energy carbon emissions is based on the regional energy balance sheet with the actual energy consumption of industries and sectors, including industrial production system energy carbon emissions, agricultural production system energy carbon emissions, intraurban energy carbon emissions, and rural energy carbon emissions, which are independent parts of each other in terms of spatial land-use types and together form the main source of energy carbon emissions. By considering the primary/secondary energy conversion process, we try to identify and deduct the part of thermal power generation in the calculation and turn to calculate the indirect carbon emissions of electricity consumption.
Besides, the socioeconomic system is divided into four parts, namely, industrial carbon emissions, agricultural carbon emissions, urban carbon emissions, and rural living carbon emissions, where carbon emissions from the tertiary sector such as the service sector are also included in the calculation of urban carbon emissions.
where CE is the total carbon emissions from the socioeconomic system in the calculation region, CEfactory is the total carbon emissions from energy consumption of all industrial production systems, CEurban is the total carbon emission of inner cities, CEagriculture is the total carbon emissions from agricultural processes around the city, and CEruralliving is the total carbon emissions of the rural domestic around the city.
The carbon emissions of industrial production systems are calculated through the energy consumption at the ultimate consumption side, considering the direct and indirect carbon emissions of all the industrial production processes, but taking care to deduct the conversion of primary to secondary energy sources.
where CEfactory is the total carbon emissions from energy consumption of all industrial production systems in the calculation region, is the carbon emissions from energy consumption of industrial production enterprise j, ωi is the carbon emission factor of the energy consumption i, which the specific value is derived from the IPCC's greenhouse gas emissions inventory, energyij is the energy consumption i of industrial production enterprise j, and j traverses all industrial production industries.
Urban carbon emissions include the emission from the distribution sectors, urban serving sectors, state organs, political party organs, social organizations, police, and military. Specifically, the sectors include transportation, storage and postal services, information transmission, computer services and software, wholesale and retail, accommodation and catering, finance, real estate, leasing and business services, scientific research, technical services and geological exploration, water, environment and public facilities management, residential services and other services, education, health, social security and social welfare, culture, sports and entertainment, public administration and social organizations, international organizations and other industries, as well as urban residents' energy consumption.
where CEurban is the total carbon emissions of inner city in the calculation region, energyi is the energy consumption, and ωi is the carbon emission factor of the energy consumption i, which the specific value is derived from the IPCC's greenhouse gas emissions inventory.
It is worth noting that urban carbon emissions are mainly indirect carbon emissions from secondary energy consumption such as electricity; however, in this study, in order to focus the study on the actual energy consumption side, carbon emissions from electricity are not accounted for at the electricity production side but at the consumption side through the verification of the grid structure.
Carbon emissions from rural life mainly refer to carbon emissions from energy consumption of industries other than agricultural production and from domestic energy consumption of rural residents that occur in rural areas. Carbon emissions from agricultural production are generated from farmland around Nanjing. Carbon emissions from energy consumption used for agricultural production mainly include energy consumption of agricultural production machinery and devices, and energy consumption of fertilizers and pesticides.
Spatial visualization of carbon emissions needs to be based on a clear distinction and identification of the land use of human-social systems that generate carbon emissions. To clarify the spatial distribution of carbon emissions from industrial production systems, agricultural production systems, intraurban energy, and rural energy, we make a basic classification of each land use based on the 2020 Nanjing land-use data (with an accuracy of 30 m) from China's multi-period land use/land cover remote sensing monitoring database.
The industrial production system land includes three parts, namely, the urban construction land excluding the urban residents, urban infrastructure, organizations; the land for industrial parks; and the point layer of industrial production enterprises. Other construction lands are the data with number 53 in the database, which is the land of factories and mines, large industrial areas, oil fields, salt fields, and quarries; using ArcGIS to spatially correct and supervise the classification of Nanjing Landsat 8 satellite remote sensing images can get industrial park data; based on Baidu map open platform to get Nanjing enterprise point data, a 15-m buffer zone is set as the spatial scope of industrial enterprises The spatial scope of industrial enterprises is set as a 15-m buffer zone. The three parts are combined to obtain industrial production and construction land. Meanwhile, in order to avoid double counting, industrial parks and enterprise ranges are excluded from other land types.
The land for urban construction and residential life is the data of number 51 in the database, which refers to the land for built-up areas of large, medium, and small cities or the county town.
Agricultural land is the data with number 1 in the database, which contains all types of arable land.
In addition, we use ArcGIS to combine the land use and administrative divisions to get the raster data of zoning and land use, assign data of carbon emission to different regions and land use, and get the carbon emission visualization map.
Rural residential land for living is the data with number 52 in the database, which refers to the rural settlements independent of the towns.
Nanjing is in the eastern part of China and the central part of the lower Yangtze River. Nanjing is the capital of Jiangsu Province, a mega-city and a national important gateway city for the development of the central and western regions driven by radiation from the Yangtze River Delta (Dai and Wu, 2011). Nanjing is also an important central city in the eastern region of China, an important national research and education base and a comprehensive transportation hub, as determined by the State Council of China. Nanjing has 12 districts within its administrative boundaries, among which Gulou, Xuanwu, and Qinhuai districts, and Jianye, Qixia, and Yuhuatai districts are the main urban areas, while the rest of the districts are areas surrounding the districts. See in Figure 2.
Nanjing is one of the most densely populated cities in China, with a resident population of 9,319,700 in 2020 and a population density of about 1423.97 people per square kilometer, which is 10 times the national average population density, according to data from China's seventh census. Nanjing has a very high level of urbanization, and with the development of social economy (Zhang et al., 2021), Nanjing's urban construction is also accelerating, and now it has basically formed a five-level urban hierarchy, main city—new urban area—new city—key town—general town, with an urbanization rate of over 85%. See in Figures 3, 4.
Besides its super high population density and complete town development system, Nanjing's comprehensive economic strength is perennially ranked among the top ten in China, and has even jumped to fourth in recent years, just behind Beijing, Shanghai, and Shenzhen. Nanjing is an important industrial base in China, an important national comprehensive industrial production base and an international advanced manufacturing base, as well as one of the three major electronic industrial bases in China, with well-developed chemical, manufacturing, energy manufacturing, and equipment industries. While these industries bring economic takeoff and vitality to the city, they are also an important source of carbon emissions for the city.
The data types required for this study are mainly land-use data classified based on remote sensing, energy consumption data based on energy balance sheets and statistics, socioeconomic data based on statistical yearbooks, greenhouse gas conversion coefficient matrix from Intergovernmental Panel on Climate Change (IPCC), and industrial production system location and extent data from government departments. Considering the accuracy requirements of the study area scale, the data of land use are used with 30-m resolution and corrected by the reference data with 10-m resolution in the urban area. See in Table 1.
The general characteristics of land use in Nanjing are shown in Figure 5. Overall, the administrative area of Nanjing is dominated by agricultural land and construction land, respectively, accounting for 50.7 and 26.8% of the total area of Nanjing. This is followed by water areas, including rivers and wetlands, which account for 11.2% of the total area of the administrative district of Nanjing. The six administrative districts of Nanjing's downtown area are obviously dominated by construction land, but Xuanwu, Jianye, Qixia, and Yuhuatai districts also have a certain proportion of forests, farmlands, and waters, which also reflects the relatively high-quality land-use pattern of Nanjing.
Based on the carbon emission from human-social systems' calculation model of this study, the total carbon emission from energy in Nanjing in 2020 is estimated to be 127.1447 million tons of carbon dioxide equivalents. Among them, the total energy carbon emission from the industrial system is 119,166,700 tons, accounting for 93.73% of the total, from inner city and urban residential living about 6.18% of the total, and the total energy carbon emission from the agricultural system and countryside is less than 1%. From the structural characteristics of the carbon emission, the industrial system and the development of the city itself are the main sources of carbon emissions in Nanjing. In the “Results” section of carbon emission characteristics, it should be noted that this study mainly calculates carbon emissions from energy-consuming systems. Parts such as chemical synthesis in industrial production, livestock respiration in agricultural systems, and emissions from plant physiological processes in agricultural cultivation are not covered by the results of this study. See in Table 2.
Based on the spatialization method of this study, a spatial quantification process was carried out for the energy carbon emission results of Nanjing in 2020. Specifically, the point and range information of industrial parks and industrial production enterprises in Nanjing were collated and labeled, the information on urban construction land and residential area locations were determined, and the energy carbon emissions in Nanjing were overlaid with the land-use pattern map of Nanjing according to emission sectors, spatial locations, and emission intensities. The obtained spatial pattern of carbon emissions in Nanjing in 2020 is shown in Figure 6. Overall, the carbon emission intensity of the six districts in the main urban area of Nanjing is significantly higher than that of other regions, and there are a large number of industrial parks at the edge of the urban area, which are the main gathering place of carbon emissions from industrial systems. Such characteristics are consistent with the distribution of industrial production systems in Nanjing. See in Figure 7.
From the results of the subregional carbon emission calculation in Nanjing, it can be seen that Jiangning, Lishui, Pukou, Lihe, and Qixia districts are the five districts with high overall carbon emissions in Nanjing, which are all located around the main urban area and are the more industrialized areas of Nanjing. The results of carbon emissions by the districts also imply the main distributions of industrial carbon emissions in Nanjing. See in Figures 8, 9.
In this study, the spatialized analysis of carbon emissions from different sources was also conducted by subregion. It can be seen that Jiangning District, as the key new development area of Nanjing, performs very significantly in all sources of carbon emissions. Although the six districts in downtown are significantly higher than other districts in terms of emission intensity, their total carbon emissions are much lower than those of Jiangning and other districts due to the limitation of their area and total population.
This also provides a clear direction for the optimization of Nanjing's urban spatial pattern toward carbon neutrality. The main downtown area is characterized by high emission density, and further improvement of energy efficiency is the key to carbon neutrality in this area. For the areas outside the main city, we need to carry out characteristic planning strategies according to their development direction. In areas where industrial parks and industrial production are the leading industries, circular industrial parks that combine upstream and downstream industries should be set up according to their industrial characteristics, so as to lengthen the energy use chain and improve energy efficiency and industrial production efficiency adequately and efficiently. In regions where agricultural production and natural landscape are the main industries, it is necessary to carry out carbon sink enhancement strategies for natural ecosystems according to local conditions, enhance the carbon sequestration capacity of natural ecosystems in the region, and contribute to carbon neutrality from the carbon sink side.
There are generally two ways to calculate carbon emissions from human-social systems, top-down or bottom-up, and both calculation methods can well portray the greenhouse gas contribution of human-social systems. In this study, we combine the top-down calculation method with the bottom-up spatial analysis model. Carbon emissions from energy statistics and annual statistics of the region are calculated through the top-down method. The carbon emission results are then evenly divided into different land patches using a bottom-up approach based on point of interest (POI) data and regional industrial park point data. This results in higher spatial resolution pattern distribution of carbon emission calculation.
The methodology of this study is ideally consistent with the model, as the raw data, reference systems, and weights used are derived from a uniform standard of caliber yearbook with reference system tables that have been accepted in the field. Thus, it is of considerable significance in methodological generalization. The uncertainty mainly comes from the mismatch of data and inconsistency of scale derivation in the top-down computational model and the bottom-up spatialization process.
In specific regional governance practices, how to integrate the results of carbon emission calculation with spatial distribution organically is often one of the more challenging tasks. The general methods or management tools mainly use carbon emission conversion factors of land use to directly calculate or only characterize those regional scales with statistical caliber. This makes the analysis of the actual pattern of regional carbon emissions vague and unclear. Thus, the calculation method and spatialization process in this study well combine getting an accurate carbon emission and drawing a clear map of carbon emission, making it possible to understand the spatial structure of carbon emission while understanding the total amount and intensity of regional carbon emission. This provides a direction for the management and planning of carbon sources.
However, for carbon emissions and the spatial portrayal, the methodology of this study still suffers from relatively insufficient resolution and relatively low refinement. A more refined instrument should be a bottom-up inventory accounting method combined with the spatialization process. Such a method would allow us to have a clearer picture of the real situation of regional carbon emissions. This method is limited by the great difficulty of data acquisition and the incoherence of trend analysis which makes it difficult to really be used. Therefore, the methodology system in this study is not a bad alternative to the current data basis premises.
This study established the methodology of top-down carbon emission calculation model and bottom-up spatial analysis process, which is expected to quantify the total amount and intensity of regional carbon emissions and, at the same time, to know the spatial distribution pattern of regional carbon emissions. In this study, Nanjing, a metropolitan city in China, was used as a case study for the calculation and analysis. The carbon emission calculation of the human-social system is divided into four major components, namely, industrial production system, inner-city and urban residential life, agricultural production system, and rural residential life. The spatial distribution pattern of carbon emission in Nanjing is obtained through the combination of remote sensing data and point data.
The results of the study showed that the total human-social system energy carbon emissions of Nanjing in 2020 exceeded 120 million tons of carbon dioxide equivalents, of which the important source is the energy consumption from the industrial production system, followed by the energy consumption from the inner city and urban residential life. The spatial distribution of carbon emissions in the six districts of Nanjing's downtown area is significantly higher than that of other districts. However, the total carbon emissions of Jiangning, Lishui, Pukou, Liuhe and Qixia districts surrounding the downtown area were significantly higher than those of the downtown area and other districts, which could be attributed to the major industrial production enterprises and industrial parks of Nanjing.
Based on the calculation and spatialization results of this study, it is possible to propose a zone-to-stratum carbon reduction strategy for Nanjing. For the areas with high carbon emission intensities and high densities but low total emissions in downtown Nanjing, further strategies should be adopted to improve energy efficiency to enhance the endogenous capacity of regional energy-carbon transformation and sustain the development of downtown areas and urban residential life. For the five districts dominated by industrial production systems and densely distributed industrial parks, the emission reduction approach should be adopted to create circular industrial zones and extend the energy-carbon production chain. For other districts where agricultural production and maintenance of natural ecosystems are dominant, they should enhance natural ecosystem services by multiple approaches and focus on reducing energy consumption in agricultural production.
Quantification of regional carbon emissions is one of the important aspects of carbon neutrality and tackling climate change. Carbon emissions from human-society systems are often closely related to energy consumption, which in most cases is a fixed point source emission. How to spatially express these emissions and effectively integrate them with regional land use is an important prerequisite for the management and planning of the regional carbon sources side. It can be expected that along with more research on data accuracy and emission inventories, it may be possible to fully determine the amount, location, and timing of regional carbon emissions in the future. However, at this stage, we are not yet able to completely count every single chimney, and this study hopes to provide some reference and basis to clarify where and how much carbon is emitted from the human-society system.
The original contributions presented in the study are included in the article/supplementary material, further inquiries can be directed to the corresponding author.
CW and YY conceived and designed the experiments and manuscript. ZW, LZ, and YR performed the experiments. SL, XL, and WS analyzed the data. ZW, XS, and JZ contributed materials tools. CW and ZW wrote the paper. All authors have read and agreed to the published version of the manuscript.
This study was supported by the National Key Research and Development Program of China (Grant No. 2019YFB2102004) and the National Natural Science Foundation of China (Grant No. 42101250).
The authors are grateful to Dr. Feng Wu who provided many helps with methodology and discussion ideas during the research process.
Authors SL and WS are employed by CETC LES Information System Co., Ltd. and authors XL and JZ are employed by Nanjing Rice Information Technology Co., Ltd.
The remaining authors declare that the research was conducted in the absence of any commercial or financial relationships that could be construed as a potential conflict of interest.
All claims expressed in this article are solely those of the authors and do not necessarily represent those of their affiliated organizations, or those of the publisher, the editors and the reviewers. Any product that may be evaluated in this article, or claim that may be made by its manufacturer, is not guaranteed or endorsed by the publisher.
Acheampong, A. O., Dzator, J., and Amponsah, M. (2022). Analyzing the role of economic globalization in achieving carbon neutrality in Australia. Int. J. Sustain. Dev. World Ecol. 29, 559–578. doi: 10.1080/13504509.2022.2056771
Chen, C., Lv, Y. L., and He, G. Z. (2022). Driving mechanisms for decoupling CO2 emissions from economic development in the ten largest emission countries. Ecosyst. Health Sustain. 6, 2059016. doi: 10.1080/20964129.2022.2059016
Dai, L., and Wu, S. (2011). Spatial temporal changes of land-use in Nanjing Region based on Markow model. Resour. Dev. Market. 27, 6, 417–420. doi: 10.1109/ICSDM.2011.5969080
Herrero, M., Henderson, B., Havlik, P., Thornton, P. K., Conant, R. T., Smith, P., et al. (2016). Greenhouse gas mitigation potentials in the livestock sector. Nat. Clim. Chang. 6, 452–461. doi: 10.1038/nclimate2925
Hong, C., Burney, J. A., Pongratz, J., Nabel, J. E. M., Mueller, N. D., Jackson, R. B., et al. (2021). Global and regional drivers of land-use emissions in 1961-2017. Nature 589, 554–561. doi: 10.1038/s41586-020-03138-y
IPCC (2019). Climate Change and Land. Available online at: https://www.ipcc.ch/srccl/
Nemeth-Durko, E. (2020). Determinants of carbon emissions in a European emerging country: evidence from ARDL cointegration and Granger causality analysis. Int. J. Sustain. Dev. World Ecol. 28, 4. doi: 10.1080/13504509.2020.1839808
Ong, T. S., Kasbun, N. F. B., Tac, B. H., Muhammad, H., and Javeed, S. A. (2021). Carbon accounting system: the bridge between carbon governance and carbon performance in Malaysian Companies. Ecosyst. Health Sustain. 7, 927851. doi: 10.1080/20964129.2021.1927851
Qiao, W. F., Mao, G. X., Wang, Y. H., and Chen, Y. J. (2016). Research on urban expansion and land use change in Nanjing over the past 32 years. J. Geoinf. Sci. 18, 200–209. doi: 10.3724/SP.J.1047.2016.00200
Randerson, J. T. (2013). Global warming and tropical carbon. Nature 494, 319. doi: 10.1038/nature11949
Seneviratne, S. I., Donat, M. G., Pitman, A. J., Knutti, R., and Wilby, R. L. (2016). Allowable CO2 emissions based on regional and impact-related climate targets. Nature 529, 477–483. doi: 10.1038/nature16542
Shan, Y., Zheng, H., Guan, D., Li, C., Mi, Z., Meng, J., et al. (2017). Energy consumption and CO2 emissions in Tibet and its cities in 2014. Earths Future 5, 854–864. doi: 10.1002/2017EF000571
Stechemesser, K., and Guenther, E. (2012). Carbon accounting: a systematic literature review. J. Clean. Prod. 36, 17–38. doi: 10.1016/j.jclepro.2012.02.021
Sun, H., Yang, G., Su, W., Zhu, T., and Wan, R. (2010). Ecological risk assessment of land use in the area along Changjiang River: a case study of Nanjing, China. Acta Ecol. Sin. 30, 5616–5625.
Wang, C., Quan, Y., Li, J., Yan, Y., Zhang, J., Song, W., et al. (2022). Characterizing and analyzing the sustainability and potential of China's cities over the past three decades. Ecol. Indic. 136, 108635. doi: 10.1016/j.ecolind.2022.108635
Yan, Y., Wang, C., Quan, Y., Wu, G., and Zhao, J. (2018). Urban sustainable development efficiency towards the balance between nature and human well-being: connotation, measurement, and assessment. J. Clean. Prod. 178, 67–75. doi: 10.1016/j.jclepro.2018.01.013
Yang, P., Cui, C., Li, L. X., Chen, W. J., Shi, Y. P., Mi, Z. F., et al. (2020). Carbon emissions in countries that failed to ratify the intended nationally determined contributions: a case study of Kyrgyzstan. J. Environ. Manag. 255, 109892. doi: 10.1016/j.jenvman.2019.109892
Zhang, B., Xie, Z., and Gao, J. (2021). Assessment on the carbon fixation of urban forests and their efficacy on offsetting energy carbon emissions in Shanghai. Acta Ecol. Sin. 41, 8906–8920. doi: 10.5846/stxb202004281026
Zhang, Y., Liu, C., Chen, L., Wang, X., Song, X., and Li, K. (2019). Energy-related CO2 emission peaking target and pathways for China's city: a case study of Baoding City. J. Clean. Prod. 226, 471–481. doi: 10.1016/j.jclepro.2019.04.051
Keywords: carbon emission, calculation framework, energy consumption, land use, human-social systems, spatialization, Nanjing
Citation: Wang C, Wang Z, Zheng L, Lu S, Li X, Rong Y, Shang X, Song W, Zhang J and Yan Y (2022) Strategies for spatial analysis of carbon emissions from human-social systems: A framework based on energy consumption and land use. Front. Ecol. Evol. 10:990037. doi: 10.3389/fevo.2022.990037
Received: 09 July 2022; Accepted: 08 August 2022;
Published: 06 September 2022.
Edited by:
Hao Sheng, Beihang University, ChinaReviewed by:
Yang Zhang, Beijing University of Chemical Technology, ChinaCopyright © 2022 Wang, Wang, Zheng, Lu, Li, Rong, Shang, Song, Zhang and Yan. This is an open-access article distributed under the terms of the Creative Commons Attribution License (CC BY). The use, distribution or reproduction in other forums is permitted, provided the original author(s) and the copyright owner(s) are credited and that the original publication in this journal is cited, in accordance with accepted academic practice. No use, distribution or reproduction is permitted which does not comply with these terms.
*Correspondence: Yan Yan, eXlhbkByY2Vlcy5hYy5jbg==
Disclaimer: All claims expressed in this article are solely those of the authors and do not necessarily represent those of their affiliated organizations, or those of the publisher, the editors and the reviewers. Any product that may be evaluated in this article or claim that may be made by its manufacturer is not guaranteed or endorsed by the publisher.
Research integrity at Frontiers
Learn more about the work of our research integrity team to safeguard the quality of each article we publish.