- 1Institute of Forest Growth and Forest Computer Sciences, Department of Forest Sciences, Faculty of Environmental Sciences, Technische Universität Dresden, Tharandt, Germany
- 2Department of Forest Resources Conservation, Faculty of Forestry, Universitas Gadjah Mada, Yogyakarta, Indonesia
- 3Géographie-Cités (UMR 8504), Paris, France
- 4Complex Systems Institute of Paris Ile-de-France (ISC-PIF), Paris, France
- 5Laboratoire en Sciences et Technologies de l'Information Géographique (LASTIG), French National Institute of Geography and Forestry Information, Saint-Mandé, France
- 6Center for Advanced Systems Understanding (CASUS), Helmholtz-Zentrum Dresden-Rossendorf, Görlitz, Germany
- 7Center for International Cooperation in Sustainable Management of Tropical Peatland (CIMTROP), University of Palangka Raya, Palangka Raya, Indonesia
- 8School of Geography, Geology and the Environment, University of Leicester, Leicester, United Kingdom
- 9Centre for Ecology and Conservation, Faculty of Environment, Science and Economy, University of Exeter, Penryn, United Kingdom
Agent-based models have been developed and widely employed to assess the impact of disturbances or conservation management on animal habitat use, population development, and viability. However, the direct impacts of canopy disturbance on the arboreal movement of individual primates have been less studied. Such impacts could shed light on the cascading effects of disturbances on animal health and fitness. Orangutans are an arboreal primate that commonly encounters habitat quality deterioration due to land-use changes and related disturbances such as forest fires. Forest disturbance may, therefore, create a complex stress scenario threatening orangutan populations. Due to forest disturbances, orangutans may adapt to employ more terrestrial, as opposed to arboreal, movements potentially prolonging the search for fruiting and nesting trees. In turn, this may lead to changes in daily activity patterns (i.e., time spent traveling, feeding, and resting) and available energy budget, potentially decreasing the orangutan's fitness. We developed the agent-based simulation model BORNEO (arBOReal aNimal movEment mOdel), which explicitly describes both orangutans' arboreal and terrestrial movement in a forest habitat, depending on distances between trees and canopy structures. Orangutans in the model perform activities with a motivation to balance energy intake and expenditure through locomotion. We tested the model using forest inventory data obtained in Sebangau National Park, Central Kalimantan, Indonesia. This allowed us to construct virtual forests with real characteristics including tree connectivity, thus creating the potential to expand the environmental settings for simulation experiments. In order to parameterize the energy related processes of the orangutans described in the model, we applied a computationally intensive evolutionary algorithm and evaluated the simulation results against observed behavioral patterns of orangutans. Both the simulated variability and proportion of activity budgets including feeding, resting, and traveling time for female and male orangutans confirmed the suitability of the model for its purpose. We used the calibrated model to compare the activity patterns and energy budgets of orangutans in both natural and disturbed forests . The results confirm field observations that orangutans in the disturbed forest are more likely to experience deficit energy balance due to traveling to the detriment of feeding time. Such imbalance is more pronounced in males than in females. The finding of a threshold of forest disturbances that affects a significant change in activity and energy budgets suggests potential threats to the orangutan population. Our study introduces the first agent-based model describing the arboreal movement of primates that can serve as a tool to investigate the direct impact of forest changes and disturbances on the behavior of species such as orangutans. Moreover, it demonstrates the suitability of high-performance computing to optimize the calibration of complex agent-based models describing animal behavior at a fine spatio-temporal scale (1-m and 1-s granularity).
1. Introduction
Orangutans (Pongo pygmaeus) play an essential role in maintaining forest composition and biodiversity by contributing to seed dispersal (Tarszisz et al., 2018a,b). However, they rely on forest architecture and tree connectivity to perform movements in order to find nesting and food trees (Thorpe et al., 2007; Manduell, 2012). This species is considered critically endangered, with their populations in sharp decline due to destruction, degradation, and fragmentation of their habitats, in particular, forest fires (Ancrenaz et al., 2016). Although orangutans can adapt and survive in a fragmented forest, their abundance is significantly lower compared to those in less disturbed and regenerating forests (Seaman et al., 2019). The growing attention directed toward orangutan rehabilitation and reintroduction (Russon, 2009; Sherman et al., 2020; Preuschoft et al., 2021) reflects the high number of displaced orangutan individuals, as well as the importance of this problem for this species' survival.
Understanding and predicting the impacts of forest disturbance on orangutan behavior, health, and population viability is, therefore, of great importance. To this end, extensive field studies have been conducted to understand the interrelationships between orangutans and their habitat. Long-term observation of orangutans' daily behavior in their natural habitat revealed, e.g., that fruit availability changes influence orangutans' activity budgets, diet, and energy balance (Knott, 1998; Morrogh-Bernard et al., 2009; Harrison et al., 2010). Moreover, although orangutans have been observed nesting in burned habitats, their abundance is far lower than in neighboring unburned forests (Rachmawati et al., 2022); peat-fire threatens this species by limiting access to food sources (especially forest fruit), thus presenting challenges for maintaining a healthy energy balance (Ashbury et al., 2022). Orangutans are recognized as arboreal, spending most of their time in the canopy, traveling among the trees using a variety of different techniques (Manduell et al., 2011, 2012). Habitat deterioration could, therefore, impact orangutan populations through increasing terrestrial movement (Ancrenaz et al., 2014), causing emigration to neighboring less disturbed forests (Husson et al., 2015), or—if dispersal is not possible—even to the death of the orangutans.
Among computational modeling approaches, agent-based models (ABMs) are predisposed to assess the interrelations between animal behavior, and the impact of disturbances on habitat quality. The popularity of ABMs owes to their ability to explicitly describe individual organisms, their behavior, interactions, responses to environmental conditions, and many other features influencing the particular fate of organisms in a changing world (McLane et al., 2011; Semeniuk et al., 2011). Furthermore, ABMs are very effective in combining field research with simulation experiments since model descriptions start on the level of individual animals, thus corresponding with field observations (Railsback and Grimm, 2019), and allowing explicit representation of individual features within an animal movement ecology framework (Nathan et al., 2008; Tang and Bennett, 2010).
The use of ABMs to describe animal movement and behavior are manifold. ABMs have been used to explore the impact of habitat fragmentation and disturbances on different species including marine mammal populations (Nabe-Nielsen et al., 2018), tiger populations (Imron et al., 2011), and plant-pollinator networks (Newton et al., 2018). ABMs have also been applied to understand and predict the importance of animal behavior for selected ecosystem functions such as seed dispersal (Bialozyt et al., 2014). In the context of primates, ABMs have been developed to explore the ecology and behavior including emotional dimensions (Evers et al., 2014), as well as orangutans' dispersal and survival chances in a disturbed landscape (Seaman et al., 2021). Seaman et al. (2021) explored orangutan population viability in the function of land use scenarios, including the conversion of natural forests into palm oil plantations with and without forest retention measures. Seaman et al. (2021), however, described animals' movement in a phenomenological way in terms of probabilities to leave, reach or settle in different landscape plots depending on their particular environmental conditions including forest type, disturbance level, or population density.
To the best of our knowledge, an explicit mechanistic description of the orangutan movement decision is still missing. Mechanistic models have the following advantages: (1) they provide us with the ability to understand the behavior of orangutans in environments that are different from the one for which data are available (e.g., forest burnt with different patterns), (2) they allow the incorporation of available expert knowledge about the system and supplementing missing information using data, and (3) they enable the prediction of the impacts of future forest disturbances on orangutans to support population viability and risk analyses, as well as aiding the development of conservation measures. Therefore, the development and deployment of such models could provide important advances in understanding the impact of forest structure, tree connectivity, habitat disturbance, energetics, and mid-term survival in threatened primates such as the orangutan (Thorpe and Crompton, 2006; Thorpe et al., 2007; Manduell et al., 2011; Van Casteren et al., 2012; van Casteren et al., 2013; Morrogh-Bernard et al., 2014).
To address these questions, we propose the BORNEO (arBOReal aNimal movEment mOdel): an agent-based model that simulates orangutan movement behavior through a network of trees within a fine spatio-temporal scale (1-m and 1-s granularity) with a corresponding scale of field observational data (10-cm and 5-min). In this model, the orangutans' choice of locomotion modes (sway, brachiation, climb, descent, and walk) strongly depends on tree connectivity, energy expenditure, food availability, as well as the availability of suitable nesting trees. We exploit field data including (i) habitat surveys to reconstruct an adequate forest structure and (ii) long-term orangutan observational data to parameterize and test our model using pattern-oriented modeling techniques (Grimm and Railsback, 2012). As model parameterization requires extensive computational resources, we took advantage of high-performance computing clusters through the OpenMOLE software platform developed for exploring the behavior of complex computer-based models (Reuillon et al., 2013). Our study, thus, introduces an integrative approach combining field ecology, agent-based modeling, and advanced calibration methods.
2. Study area
Field data were collected in Sebangau National Park, Central Kalimantan, Indonesia (Figure 1). The research site had experienced historic human-induced disturbances involving selective logging by concessions and illegal loggers before the establishment of the national park in 2004 and the control performed by locally-led community patrol teams (Husson et al., 2018). Forest alterations, including the establishment of timber extraction canals by illegal loggers, have led to a legacy of imposed peat drainage and heightened dry-season fire risk (Wösten et al., 2008; Harrison et al., 2009). As such, the area experienced multiple fire episodes in 1992, 1994, 1997, and 2002 (Balai Taman Nasional Sebangau, 2015). During the dry season in 2006, 2015, and 2019 forest fires re-occurred in the area (Hayasaka et al., 2020).
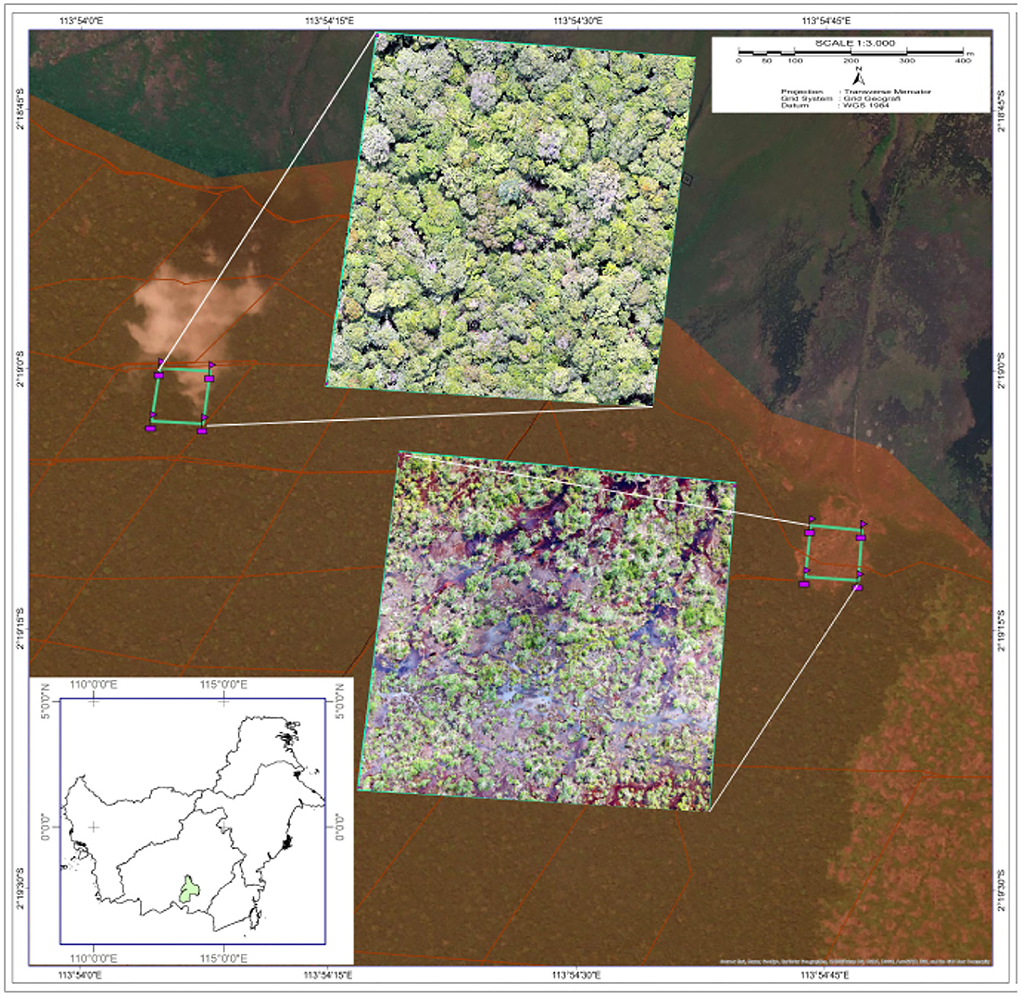
Figure 1. Location of the study area in Sebangau, Central Kalimantan, Indonesia. The red zone in the background represents the area of Sebangau National Park, while the remaining area is the buffer of the park. The inset on the bottom left highlights the location of Sebangau National Park. Two different plots were selected for the tree survey, each sized 1 ha. Aerial drone images were taken at the unburned forest (top) and burned forest (bottom). Source: Borneo Nature Foundation.
3. Materials and methods
3.1. Field data collection
We performed tree surveys by establishing two 1-ha plots in both unburnt and burnt forests (Figure 1) in August 2021, from which we recorded 1,352 and 439 trees, respectively (Widyastuti et al., 2022a). We use the data to realistically represent the orangutan habitat within our simulation model. For this, we acquired data on the trees' species with the assistance of local expert botanists, dbh (diameter at breast height), height, and canopy radius for all trees exceeding 6 cm dbh.
3.2. Model description
We present a complete model description following the ODD (Overview, Design concepts, Details) protocol for describing individual- and agent-based models (Grimm et al., 2006, 2010), as updated by Grimm et al. (2020) in Supplementary material 1. For the sake of simplicity, we only present a summary of the model description here. The model was implemented in NetLogo 6.2.2 (Wilensky, 1999), a free software platform for implementing individual- and agent-based models.
The overall purpose of BORNEO (Widyastuti et al., 2022b) is to understand how patterns in orangutan daily activity budgets are shaped by changes in (1) habitat conditions in terms of forest structure and tree species diversity, and (2) orangutan energy-balancing motivated movement behaviors. The simulation model, thus, focuses on a single orangutan's daytime foraging behavior, monitoring its energy expenditures while feeding, traveling or resting, and the type of locomotion the orangutan selects when it moves from one place to another.
In the model, orangutan and trees are represented as entities. Orangutan is the main entity (agent) in the model, using the network shaped by tree entities to travel. Table 1 presents a list and description of the model's entities and their attributes. The behavior of orangutans in the model is governed by different parameters, including energy-regulation (energy-intake, energy-gain, basal-energy, initial-satiation), movement speeds (walk-speed, brachiate-speed, sway-speed, climb-speed, descent-speed), and a stochastic parameter prob-move-no-feed indicating the proportion of orangutan movement that is not dedicated to foraging. In addition, a variable body-weight is assigned to the orangutan, which affects the magnitude of its energy expenditure throughout the simulation. The above-mentioned orangutan parameters and the body-weight variable are assigned at the model setup and kept constant throughout each simulation.
The orangutan's state variables within a simulated day fluctuate throughout the simulation as a result of the performed activities. The state variables related to activity budgets (feeding-budget, resting-budget, traveling-budget) record the proportion of time spent engaged in up to any given time-step. The orangutan also has a metabolic state variable, satiation, which represents the state of the orangutan's energy reserve. Depending on the satiation level, the orangutan decides the next type of activity to perform: resting, traveling, or feeding. The selected activities, in turn, affect the state of the orangutan's satiation: it increases when feeding and decreases when resting or traveling.
The forest in the model is represented as a tree network, in which a tree is considered as a node connected to other trees via links. Our approach to representing forest structure using concepts in network science is similar to that of Schmid et al. (2020). A link representing a connection between trees is generated if the canopy of both trees overlaps, or if a tree is close to another (< 4 m apart). Connections (links) between trees determine the availability of arboreal travel paths for an orangutan. Depending on the characteristics of trees (heights and crowns diameter) composing the route, the links are classified into two types: brachiation and sway. All arboreal route lengths are understood as the shortest path lengths.
In the model, we illustrate the energy-balancing motivated movement behavior of orangutans, which is triggered by hunger (a condition where orangutans' satiation is below zero; refer to Figure 2). If the orangutan is triggered to search for food, it scans the tree network and selects a fruiting tree with the shortest Euclidean distance from its current position. Since we used a small spatial representation (1 ha), we assume that the orangutan has complete knowledge of the tree network and, thus, is on the most efficient route to fruiting trees. The orangutan is consequently able to perceive the entire simulated tree network within the forest, identify all fruiting trees, and be informed of which tree is the nearest.
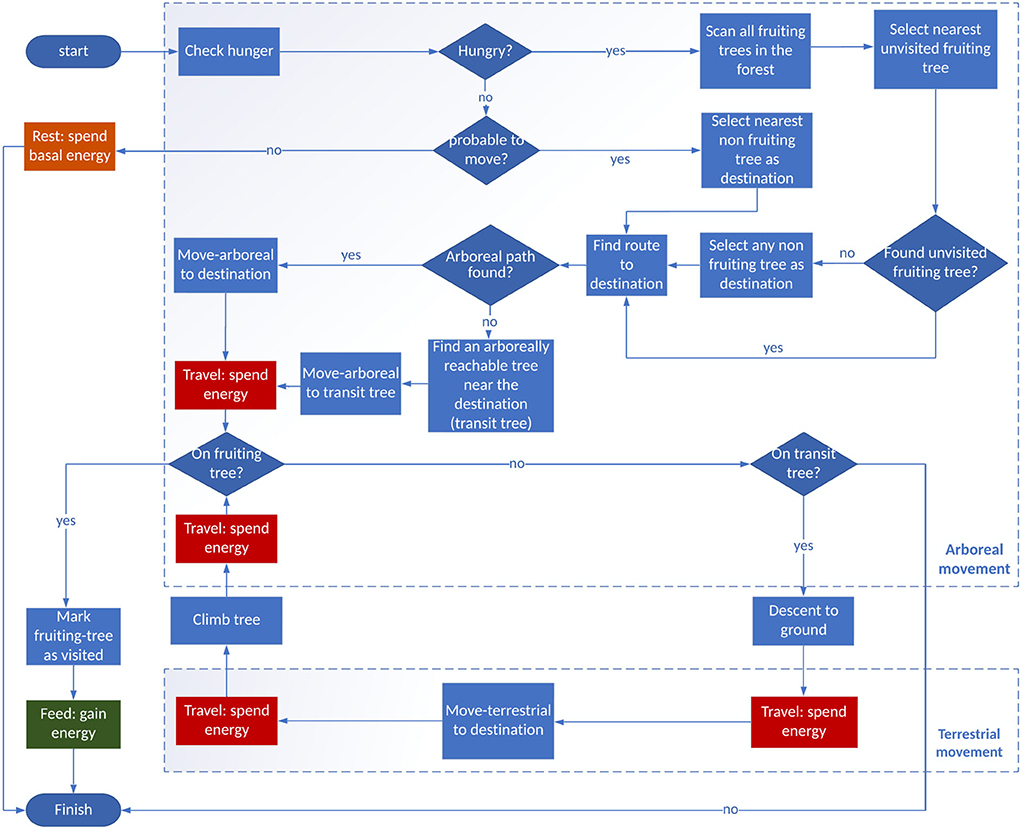
Figure 2. Movement procedure flowchart showing the steps performed by the orangutan in order to fulfill the objective of maintaining its energy balance. Orangutans perform activities involving energy expenditure not only when traveling (spend movement energy), but also while resting (spend basal energy). Energy intake increases when the orangutan is performing feeding activities. Orangutan travels through a tree network to perform activities, which is decided by the state of hunger (satiation level). When hungry, orangutan searches the nearest fruiting tree as a destination. The orangutan then searches for an arboreal route (as a preference) through the tree network to reach the destination from its initial position. If an arboreal route is available (there is a connected path within the network to reach the destination), the orangutan performs a fully arboreal movement. Orangutan switches to terrestrial movement if part or the entire route to the destination lacks arboreal connection.
The orangutan movement is organized as follows: when not triggered by hunger, the orangutan may rest (according to a prob-move-no-feed probability) or move to a nearby tree. When hungry, the orangutan chooses the nearest fruiting tree as a direct travel destination. If this destination is reachable by the arboreal links of the network, the orangutan reaches the destination tree using the shortest path in the tree network. Otherwise, the orangutan moves to the nearest connected tree, i.e., the tree with the minimum Euclidean distance to the destination tree. If this tree has no arboreal connection to the next tree, this nearest tree is tagged as a transit tree, from which the orangutan will descend to the ground and walk toward the destination tree. A flowchart depicting the process in detail is available in the full ODD description (Supplementary material 1).
Depending on the type of link available between the current position and the target fruiting trees, the selected locomotion type, terrestrial or arboreal (Figure 3), will have a specific energetic cost and speed. The orangutan in the model preferably selects arboreal movement and switches to terrestrial movement only if no arboreal link is available. This agent continues performing activities according to its state of satiation at any given time, until the end of the simulated period. The length of a simulation run represents 12 h of orangutan daytime in seconds, which accounts for 43,200 time-steps in the model.
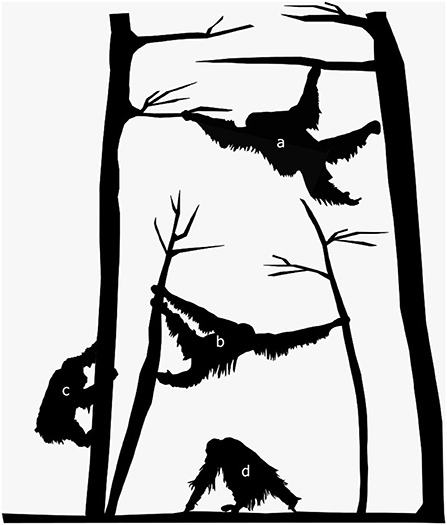
Figure 3. Orangutan performs different locomotion types throughout the simulation, depending on the connectivity of trees. Here, we illustrate orangutan's brachiation (a) through connected canopies, sway (b) by bending a tree to reach another in proximity, tree climbing (c) from the forest floor, and terrestrial walk (d). Illustration by MAI.
Patterns of interest for the BORNEO model are the activity budgets of orangutans, such as the proportion of time spent in feeding, traveling, or resting according to various environmental configurations including fruiting tree density and fragmentation of the habitat induced by forest fires. We evaluate our model by its ability to reproduce observed patterns of orangutans in their natural habitat. To that extent, we use orangutan observational data collected from the site and described in previous publications (Morrogh-Bernard et al., 2009, 2014; Harrison et al., 2010).
3.3. Experimental design
Simulation experiments were carried out in OpenMOLE (Reuillon et al., 2013). OpenMOLE is a platform that performs model exploration on distributed computing environments. It includes built-in methods and algorithms to evaluate simulation models such as sensitivity analysis, calibration, profiling and pattern space exploration (Reuillon et al., 2010, 2013, 2015; Chérel et al., 2015). The model code, data, and R-Scripts for data analysis are available in a public repository (see Section 6).
3.3.1. Sensitivity analysis
Sensitivity analysis evaluates changes in the model output depending on the variations in input parameters. We performed a global sensitivity analysis1 using Morris' screening (Morris, 1991) method available in OpenMOLE. Morris' screening is known as a “one-factor-at-a-time” analysis, which computes the relative influence of each factor on the model output. The output of Morris' screening is interpreted by the values of μ * and σ. The absolute mean μ * demonstrates the magnitude of the parameter's influence on the model outcome, while the SD σ shows the strength of parameter interactions (Imron et al., 2012; Menberg et al., 2016).
In our study, we took into account all orangutans' parameters2 listed in Table 1 for the Morris screening experiment. For the purpose of the sensitivity analysis, we used the reconstructed virtual forest leveraging the data obtained from the relatively undisturbed, unburned plot (Figure 4, left).
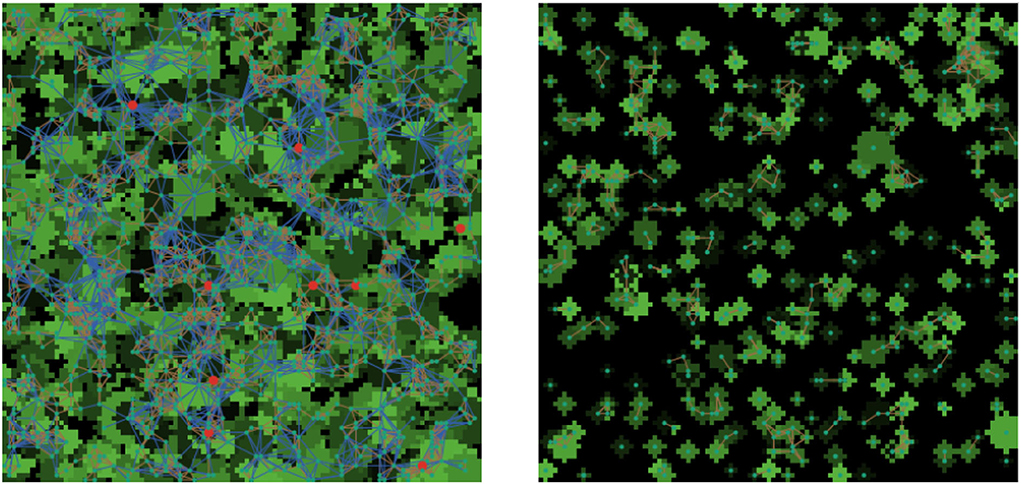
Figure 4. Tree survey data from the study site is visualized in the model simulation setup, including the unburned forest (left) and the burned forest (right). The red dots represent the fruiting trees. We computed a network comprised of links representing the tree connectivity, which is determined by the (i) overlap (blue lines) and (ii) distance (brown lines) between trees. The link color also represents the type of arboreal movement which is available to the orangutan (sway between close trees and brachiate through overlapping canopies).
From the sensitivity analysis result, we ranked the parameters based on the strength of influence on the model output. This information served as a general overview of the model behavior. We used the result of the Morris screening to evaluate the parameters' contribution to the model output and used the results to confirm and justify the model mechanisms.
3.3.2. Multi-criteria calibration
The calibration process searches through different parameter combinations as model inputs and finds the ones that lead to a best-fitting model output in comparison to the real-world observation. The fitness of the model output is evaluated by computing the distance between simulation outputs and real-world observations. In this study, we used observational data presented in Table 2 to calibrate the BORNEO model, by taking three objectives of calibration: the resting budget, the feeding budget, and the traveling budget. Similar to the sensitivity analysis experiment, for the calibration process we used the reconstructed virtual forest leveraging the data obtained from the relatively undisturbed, unburnt forest plot (Figure 4, left).
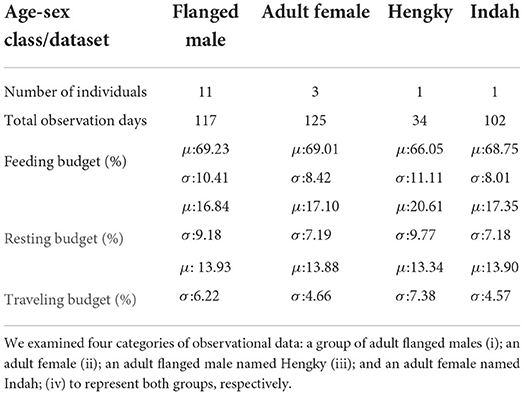
Table 2. Description of observational data which served as a criterion for the calibration process evaluating the model output.
Since the calibration objectives (three different activity budgets) are proportional to each other, they are somehow competing (i.e., within the 12 h days, increasing time on one activity by necessity reduces the time available for the others). Therefore, we performed the multi-criteria calibration. Multi-criteria calibration is the extension of the calibration process to several, sometimes competing, criteria. It aims at producing a set of optimal compromised solutions, or a sample of the Pareto Front. The sample of the Pareto Front is a set of diverse solutions for which each solution is non-dominated: a solution is non-dominated if no solution exists that is better on all criteria at once (Legriel et al., 2010).
We based our calibration experiment3 on the NSGA2 algorithm (Deb et al., 2002), which is a well-known multi-objective genetic algorithm. NSGA2 is available in OpenMOLE and has been used as a calibration method in numerous previous studies (Schmitt et al., 2015; Passerat-Palmbach et al., 2017; Raimbault, 2018; Raimbault et al., 2020).
The NSGA2 algorithm requires a defined search space and a multi-objective fitness function. In our experiment, we defined the search space4 according to the model's parameters shown in Table 1. Since a part of the parameter space is unsound (i.e., it is unlikely that orangutans climb faster than descending), we adjusted the search space using a rejection mechanism available in OpenMOLE. The rejection criteria allow us to omit solutions that do not fulfill our defined requirements. We assumed in the model that, keeping distance constant, an orangutan performs swaying movement at a higher speed than walking and brachiating, and that the orangutan descends faster than climbing up a tree. Based on these assumptions, we omit all solutions which negate the defined condition. Therefore, we defined a rejection criterion5 in OpenMOLE so that the calibration process would reject any solution which suggests that brachiating is faster than swaying, walking is faster than swaying, or climbing is faster than descending:
For this experiment, we selected the fitness function to be the quadratic distance between the deciles of the three budget distributions. We aimed at maximizing the overlap between the distribution functions produced by the model and the empirical distributions produced by the field data. The objective of the calibration process was, thus, to simultaneously minimize the outcome of three fitness functions6 fb:
where :
• o is the observational data vector regarding an activity budget (e.g., resting).
• s is the simulated data vector of the same activity budget (e.g., resting).
• Dobsi is the ith decile of the distribution of observational data.
• Dsimuli is ith decile of the distribution of simulated data.
• b is one of the three budgets, i.e., b∈resting, traveling, feeding.
The distribution produced by the model for a given parameter set was evaluated by running 50 statistically independent runs of the model (replications). The deciles of the distributions produced by the model are then estimated from the 50 samples of the output distributions.
We conducted the model calibration against field observation data of two commonly recognized orangutan age-sex classes (i.e., a group of adult flanged males (dominant male with secondary sexual characteristics) and a group of adult females) obtained from a previous study by Morrogh-Bernard (2009), which also took place in Sebangau National Park. In addition, we selected one individual from each age-sex class (one individual flanged male and one individual adult female), which observation data is also utilized in calibration7. Table 2 shows the categorization of orangutan field observation data. The observed distributions of the particular activity budgets (Figure 6, red curves) present the data for these groups (classified by the row names on the right side). These long-term orangutan observation show variability in daily activity budget allocations due to individual heterogeneity, daily conditions, surrounding forest structure, and other sources of uncertainty. Consequently, we attempted to reproduce the observed distributions rather than an averaged ratio of activity budgets.
Contrary to regular (i.e., mono-objective) calibration, multi-criteria optimization does not exhibit a uni-dimensional list of solutions, but a Pareto Front composed of several solutions. Due to potentially competing objectives, solutions are not comparable, with usually no way to favor one solution above another. In our analysis, several solutions showed satisfying fitness values on all objectives simultaneously. Therefore, we performed further simulations based on calibrations in which the solutions best overlapped the distribution of the observed and simulated budget. To evaluate the calibration results, we used three different quantitative metrics: KL Divergence, Overlapping index (Pastore and Calcagnı̀, 2019), and Pearson correlation. In addition, we examined the parameter values and selected the solution which offered a reasonable value range. We also used the calibrated models to test an illustrative scenario of burned forest (refer to next sub section).
3.3.3. Scenario application
After selecting the calibrated models which best reproduced the pattern of orangutans' activity budgets, we applied scenarios of fire disturbances to the model8. For this, we used the field survey data9 from both unburned and burned forest plots to build the model environment setup. We varied the proportion of unburned and burned forests, resulting in nine tree network configurations as shown in Supplementary Table 7 and illustrated in Supplementary Figure 5.
With this experimental setup, we examined how the model outputs regarding orangutans' activity budgets and movement behavior shifted with increasing forest burning intensity. We selected four metrics to observe from the model output: (1) movement type (arboreal, terrestrial, and climbs); (2) activity budgets (feeding, resting, and traveling); (3) travel distance; and (4) energy balance (energy intake and energy expenditure).
4. Results
4.1. Sensitivity analysis
Figure 5 and Supplementary Table 1 in Supplementary material 2 show the ranks of parameter influences. We observed that the model outputs on feeding-budget and traveling-budget were most sensitive to the energy-related parameters (high values of μ* and σ): energy-intake, basal-energy, and energy-gain. This suggests that fluctuations in activity budgets are mainly driven by the orangutan's speed of feeding (energy-intake), constant energy baseline, energy requirements (basal-energy), and food availability in a fruiting tree (energy-gain).
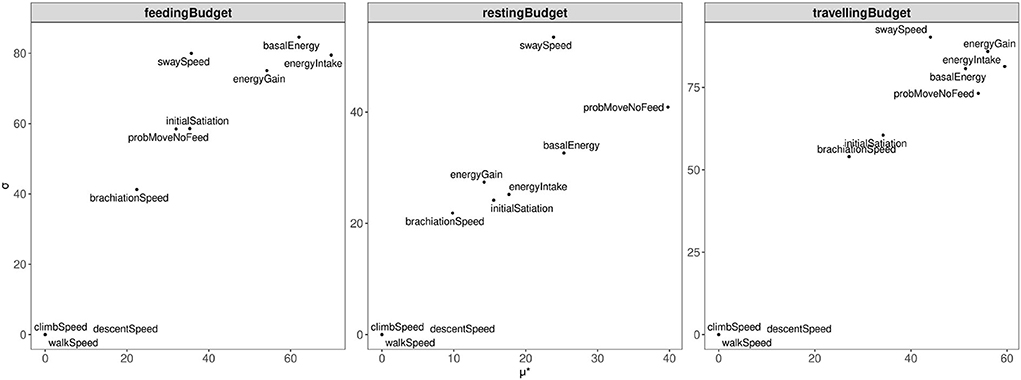
Figure 5. Morris screening results. μ* on the x-axis depicts the overall sensitivity of the parameter. σ on y-axis depicts the overall tendency of the parameter to interact with the others.
The prob-move-no-feed parameter had a relatively high influence on all activity budgets, but in particular, the resting-budget. This suggests that the magnitude of probability for an orangutan to travel without the intent of foraging influences the proportion of the daily activity budget spent resting, traveling, and feeding. In particular, the more likely that the orangutan will travel, the less time the orangutan can allocate for resting and feeding.
The parameters related to terrestrial movement (climb-speed, descent-speed, and walk-speed) did not have any influence on model outputs. In contrast, the parameter sway-speed presented a strong influence on model outputs for all activity types. This showed that the particular forest tree network used in these simulations (refer to Figure 4, left) contained mostly sway links. The brachiation-speed parameter, in comparison, had a weaker influence on model outputs, suggesting lower availability of brachiation than the sway links, due to tree sizes and positions.
Nonetheless, the lack of sensitivity of terrestrial movement parameters could have emerged from the characteristics of the virtual forest used in the simulation experiments (refer to Section 3.3.1). As the undisturbed forest contains more arboreal pathways, orangutans could mostly move via arboreal paths, instead of terrestrial ones.
4.2. Multi-criteria calibration
From the multi-criteria calibration process, we obtained a set of Pareto efficient solutions forming a Pareto Front (refer to Section 3 in Supplementary material 2). For these solutions, no increases in one of the fitness of calibration criteria (picked among traveling, feeding, or resting) could be performed without decreasing at least one other criterium.
We selected one solution from each set of solutions for a group of adult flanged males (AFM), a group of adult females (AF), Hengky (H)—one adult male, and Indah (I)—one adult female, respectively. Figure 6 compares the activity budget distribution obtained field observation and from model simulations. Supplementary Table 2 in Supplementary material 2 provides a quantitative comparison of both distributions.
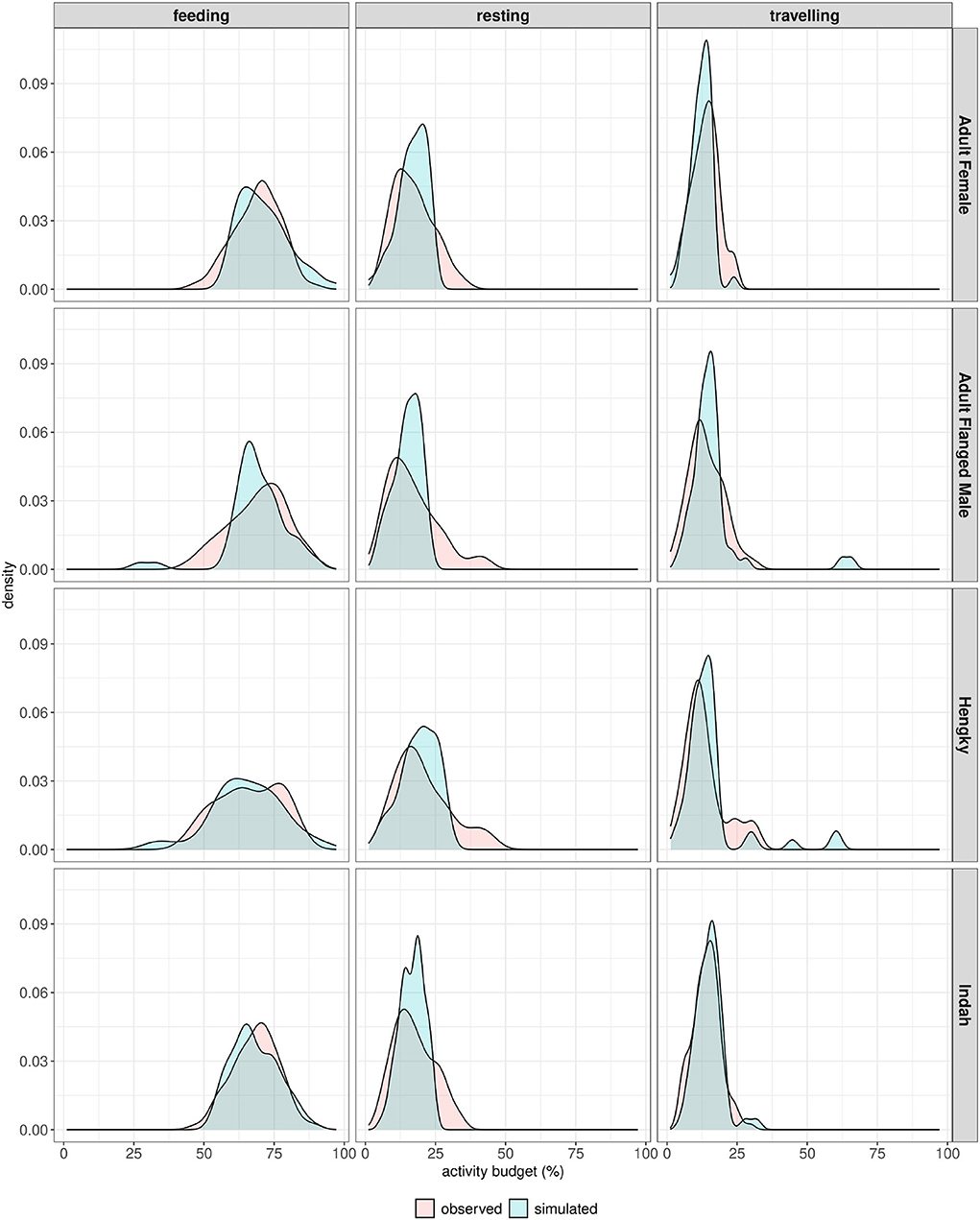
Figure 6. Calibration results to model with selected parameter set. The simulation result (in blue; 50 replicated simulations of one individual) is then compared to the observational data of orangutans follows (in red; refer to Table 2). We compared feeding, resting, and traveling, respectively. Note that we selected one out of several possible solutions shown in Section 3 of Supplementary material 2.
The calibration results demonstrated that the model was able to relatively accurately reproduce the pattern of orangutan activity budget, with the activity budget distribution shapes showing a good overall similarity. The overlapping index for all distribution comparisons was more than 0.5, showing that a majority of the orangutan's activity budget variation was reproduced by the model. The KL-Divergence test on all activity budget distribution comparisons showed values close to zero, confirming the similarity. Pearson correlation test showed values above 0.85 for all distribution comparisons. Table 3 shows in detail two of the selected solutions, i.e., calibrated parameter values, leading to the selected calibrated models for an adult male (H) and an adult female (I), respectively.
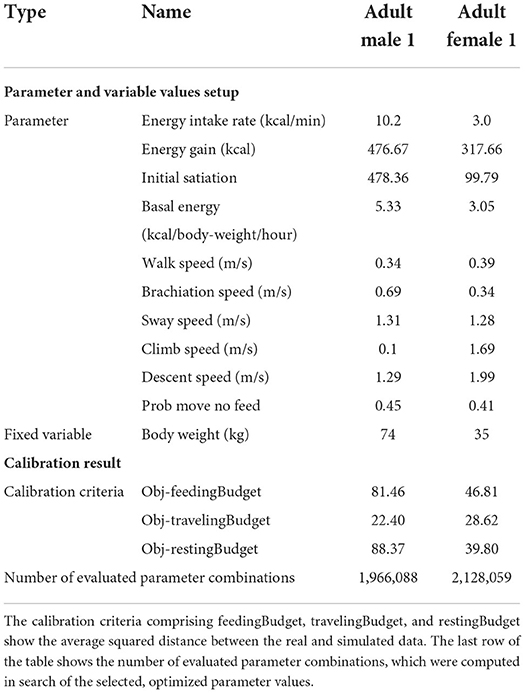
Table 3. The parameter set and fixed variables that have led to the solution selected in Figure 6 for an adult male and adult female.
4.3. Scenario application
To explore the impact of forest fire disturbance on the movement behavior and activity budgets of orangutans, we applied burning scenarios to the selected calibrated model for an adult male (H) and an adult female (I), where we varied the proportion of the burnt and unburnt forests.
Figure 7 shows the impact of increasing burning intensity on the proportion of employed movement types and activity budgets. Within an unburnt forest, the orangutan performed more arboreal moves (>50% of the overall moves), with a moderate amount of climbs and no terrestrial moves. Gradually increasing the intensity of burnt forest caused the proportion of movement types to shift: fewer arboreal movements were performed as compared to the unburnt forest, while the proportion of terrestrial moves emerged and increased with the intensity of burnt forest. For the selected calibrated model on a flanged male (AFM), terrestrial movements emerged as the burning intensity increased to 20%, whereas adult female (AF) terrestrial movements emerged with 30% burning intensity.
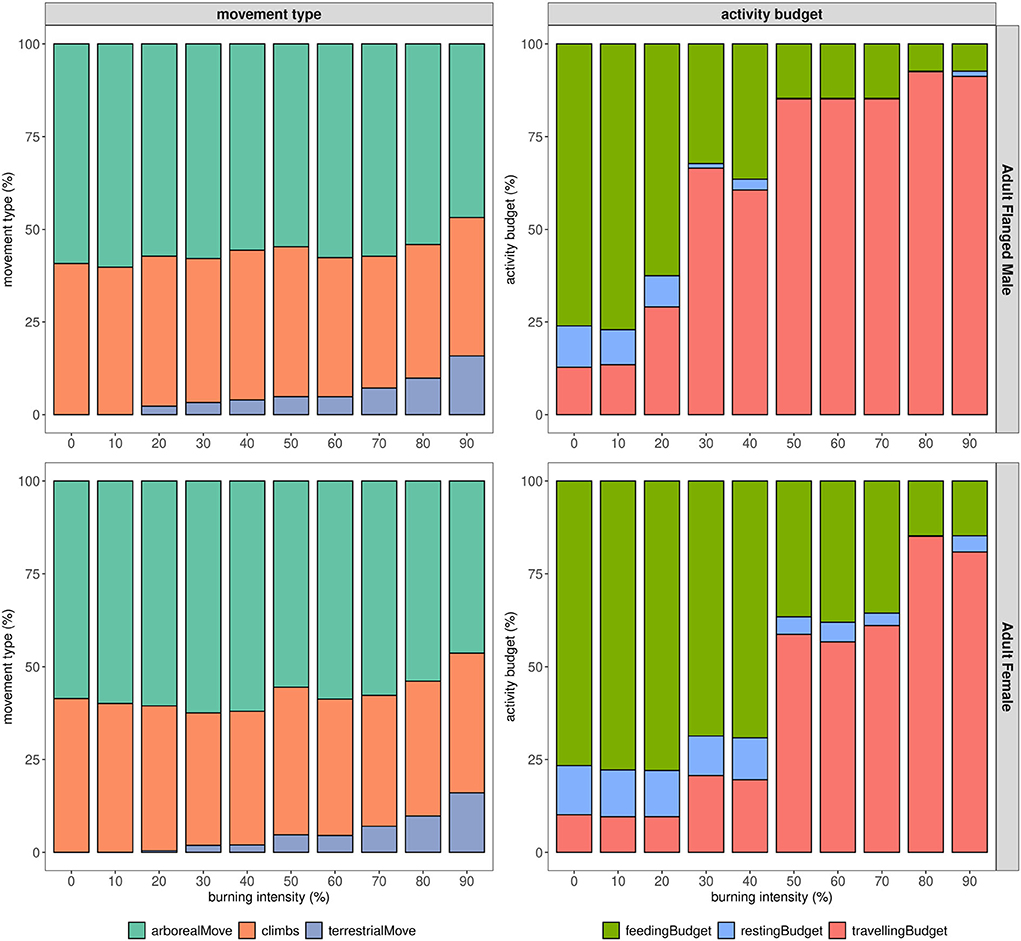
Figure 7. Scenario test with the calibrated model for adult flanged male and adult female visualized in proportional-bar-charts. Each bar is divided into three categories as in the legend, either showing the three different types of move, or the different activity budgets. The model is initialized with the parameter set referring to Table 3. The number of trees and fruiting trees refers to Supplementary Table 7.
The orangutan's activity budget shifted as we increased the intensity of burned forest. On the selected calibrated model for the male orangutan (H), the activity budget was dominated by feeding activity in the unburnt forest scenario. As we increase the burn intensity, the proportion of feeding and resting decreased starting at a burn intensity of 20%, to be replaced by significantly more traveling activity. Similar behavior was observed on the selected calibrated model for the adult females (AF), for which change emerged when burn intensity exceeded 40%.
Figure 8 shows the impact of forest fire disturbance on the travel distance and energy intake/expenditure of simulated orangutans. As we increase the burn intensity in the simulation, the distance traveled by the orangutans also increases. On the selected calibrated model for the flanged male (AFM), the travel distance already increased when the burn intensity reached 20%. For the adult female (AF), this happened when the burn intensity was 50%.
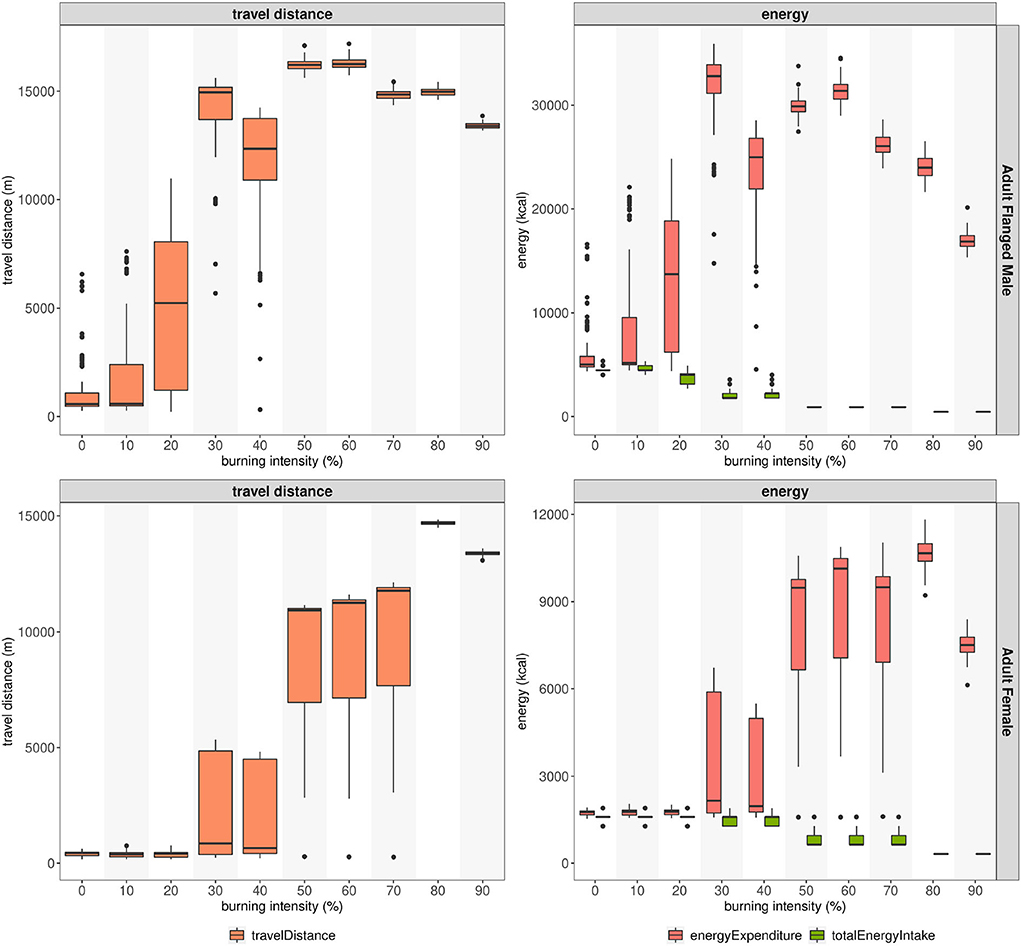
Figure 8. Scenario test with the calibrated model for adult flanged male and adult female visualized in box-plots. The length, center mark, and edges of each box show the interquartile range, median, and quartiles Q1/Q3 of the sampled simulation output, respectively. The model is initialized with the parameter set referring to Table 3. The number of trees and fruiting trees refers to Supplementary Table 7.
The energy intake and expenditure on the simulated orangutans showed opposite threshold behaviors: while energy intake decreased with burn intensity, the energy expenditure increased.
5. Discussion
BORNEO is the first ABM to incorporate complex movement decisions of primates (in particular, orangutans) involving their habitat structure and locomotion behavior. The model (partially) reflects the complexity of the orangutan's habitat by representing forest structure as a tree-network that influences orangutan movement. Such detailed representation of habitat structure has not been considered in similar primate ABMs. Evers et al. (2014) presented a model of primate emotional-social behavior, which described the habitat as simplified grid cells. Meanwhile, Gluckman and Bryson (2011) did not include explicit spatial representation for their primate social structure model. The ABM primates models more similar to ours (e.g., Bonnell et al., 2013; Bialozyt et al., 2014) did not consider the complexity of the forest structure as an influence on movement decisions. While Bialozyt et al. (2014) considered two types of movement toward fruiting trees (i.e., directional and semi-directional types), orangutans in BORNEO actively search for the most efficient way to move to fruit trees using various movement types, depending on the forest structure conditions. Bonnell et al. (2013) included spatial memory, while the current version of BORNEO has not explicitly included spatial memory.
The use of OpenMOLE (Reuillon et al., 2013) as a comprehensive suite to support the simulation experiments has enabled a robust, efficient, and reproducible application of Pattern Oriented Modeling (Railsback and Grimm, 2019). While some agent-based models use systematic, uniform sampling of the parameter space and manual comparison to the desired pattern (e.g., Pietzsch et al., 2021; Widyastuti et al., 2021), the NSGA2 algorithm (Deb et al., 2002) implemented in OpenMOLE allows exhaustive exploration of the model's parameter space. This study, thus, has employed a more recent practice in simulation experiments, which could be beneficial for similar agent-based models.
The findings from our BORNEO model complement findings from Erb et al. (2018), which highlighted the behavioral and energetic response of wild orangutans during fire incidence in a neighboring, ecologically similar habitat to our study site (Ashbury et al., 2022). Changes in forest structure increased the frequency of terrestrial movement in BORNEO. This behavior was also observed in real observations of orangutans in disturbed habitats derived from camera trap observations (Ancrenaz et al., 2014).
Our simulation results clearly show how orangutans are challenged by even a medium-level disturbance: their daily behavior is rapidly disturbed at relatively low fire intensity and is overwhelmed by high fire intensity. Our model showed that males are more sensitive to fires than females, probably due to their greater body mass, and the consequently greater energy intake that especially flanged males have to maintain. At a high burning intensity, orangutans significantly increased the proportion of terrestrial walking for both flanged males (AFM and H) and adult females (AF and I). The simulated orangutans in BORNEO maintained a high proportion of arboreal movement during no and low fire disturbance and increased their proportion of walking when the disturbance was at medium and high. Our findings also suggest that male energy balance is more sensitive to disturbance than that of females: a consequence of the aforementioned increase in travel distance and decreased feeding at lower burn intensity for males.
Since we used a small spatial representation (1 ha), we assumed that the orangutan has complete knowledge of the most efficient route to fruiting trees, though we recognize that in practice this assumption is unlikely to be fully met for wild orangutans in a constantly changing forest-food landscape. Orangutan travel might, therefore, be less efficient than indicated by our models. While the BORNEO model provides a fine scale movement simulation, a future challenge of the model in providing ecological insight for this endangered species would be to incorporate a larger area covering a full orangutan home range size and maintaining important details of habitat features for movement. Such implementations, however, are challenging due to data availability on detailed forest structures at larger scales, as well as simulation computation costs.
Incorporating more orangutans and different species in the model could also provide a platform to investigate competition and resource exploitation among individuals and between different species, in particular individuals living together; i.e., adult females with (semi-)dependent offspring (Goossens et al., 2006; van Noordwijk et al., 2012). Incorporating other individuals in the model could also provide the opportunity to test whether orangutans can perform spatial synchrony (Liebhold et al., 2004) of home-range arrangement (Wang and Grimm, 2007), an important feature in mammals. Furthermore, since fire can occur at large scales beyond a protected area (e.g., Imron et al., 2022), understanding their impacts across landscape levels while maintaining relevant details is a further challenge for BORNEO development. As orangutan movement decisions are also a result of interactions with other individuals, future work could focus on movement decisions (a) in small groups (e.g., females with their infant), (b) due to resource competition, or (c) socio-sexual reasons (i.e., males moving away from each other to avoid fights, or toward each other to fight; males moving toward females to attempt courtship, and females moving away from or toward males depending on mating triggers). Exploring such questions could provide valuable insights into movement ecology.
BORNEO is based on the premise that orangutans are omniscient about the environment. Hence, exploration, learning, and memory of the environment as well as learning processes are important in such environments. Moreover, the body weight is a constant in the present model, which lacks a proper metabolic/energetic submodel. Further model improvements could include incorporating the interaction between learning and metabolism aspects to consider feedback between these (Vincenot et al., 2015). Different search strategies of orangutans, explicit consideration of the Dynamic Energy Budget approach, and forest dynamics would also improve the model.
Although the impact of fire on plant diversity was not specifically modeled, a reduction in the number of trees of particular fruiting trees may have important influences on orangutans' movement target and direction. Incorporating into the BORNEO model other models such as peatlands fire simulations considering incidence (Widyastuti et al., 2021) and burn intensity to simulate movement decisions of orangutans during fires might further improve our understanding of how this species could survive through catastrophic events. In addition, the use of the BORNEO for Population Viability Analysis at the landscape level can provide insights for conservation guidance for this endangered primate as has been demonstrated for other species (Imron et al., 2011). Expansion of BORNEO for mitigating human-orangutan conflicts (Meijaard et al., 2011) would also provide opportunities to further understanding new emerging diseases as part of the one-health issue (Chappell and Thorpe, 2022).
Data availability statement
The datasets presented in this study can be found in online repositories. The names of the repository/repositories and accession number(s) can be found in the article/Supplementary material.
Author contributions
KW designed and developed the model, collected tree survey data, performed simulation experiments, analyzed the simulation result, and wrote the manuscript. RR supervised the simulation experiments execution, analyzed the model simulation result, proposed model improvements, and reviewed the manuscript. PC analyzed the model simulation result and reviewed the manuscript. WA reviewed the model design, proposed model improvements, and reviewed the manuscript. DN, MH, and HM-B supported the field work and data collection and reviewed the manuscript. MI and UB supervised the research project and reviewed the manuscript. All authors contributed to the article and approved the submitted version.
Funding
This research is part of a project funded by UK Research and Innovation (UKRI) through the Global Challenges Research Fund (GCRF), grant number NE/T010401/1. For the purpose of open access, the authors have applied a Creative Commons Attribution (CC BY) license to any Author Accepted Manuscript version arising. KW was supported by DAAD doctoral research grant.
Acknowledgments
We thank Balai Taman Nasional Sebangau and CIMTROP for granting the research permit and supporting the field study. Borneo Nature Foundation provided logistical support during the preparation and execution of field data collection. Agnes Ferisa provided technical advice and supported field work coordination. The local field experts (Hendri, Apri, Arly, Agus, Adul, Iwan, Kades, Sadi, Tomi, Galih, and Santiano) supported the plot establishment, tree measurements, and species identification. Eko Yudi Aditya and Restu Aminullah documented the plots with aerial photographs used in Figure 1. We thank Martin Zwanzig for providing access to virtual machines and Tatiane Micheletti for providing feedback on the later version of our manuscript draft. KW acknowledges the ISC-PIF for hosting her research stay, which focused on analyzing the model with OpenMOLE. We thank the Center for Information Services and High-Performance Computing [Zentrum für Informationsdienste und Hochleistungsrechnen (ZIH)] at TU Dresden for providing its facilities for high throughput calculations. The authors thank the reviewers for their constructive input, which helped improve the manuscript.
Conflict of interest
The authors declare that the research was conducted in the absence of any commercial or financial relationships that could be construed as a potential conflict of interest.
Publisher's note
All claims expressed in this article are solely those of the authors and do not necessarily represent those of their affiliated organizations, or those of the publisher, the editors and the reviewers. Any product that may be evaluated in this article, or claim that may be made by its manufacturer, is not guaranteed or endorsed by the publisher.
Supplementary material
The Supplementary Material for this article can be found online at: https://www.frontiersin.org/articles/10.3389/fevo.2022.983337/full#supplementary-material
Footnotes
1. ^Source code of the method script is available at: https://github.com/kiranaw/Borneo/tree/master/experiments/sensitivity/morris.oms (see lines 5–24).
2. ^See lines 8–18 of the script.
3. ^Source code of the method scripts are available at: https://github.com/kiranaw/Borneo/tree/master/experiments/calibration.
4. ^See lines 40–50 of the https://github.com/kiranaw/Borneo/blob/master/experiments/calibration/calibrationAF.oms script.
5. ^See line 38 of the https://github.com/kiranaw/Borneo/blob/master/experiments/calibration/calibrationAF.oms script.
6. ^See lines 6–13 of the https://github.com/kiranaw/Borneo/blob/master/experiments/calibration/calibrationAF.oms script.
7. ^Therefore, there are four different calibration scripts of the https://github.com/kiranaw/Borneo/blob/master/experiments/calibration/ directory.
8. ^Source code of the scenario scripts are available at: https://github.com/kiranaw/Borneo/tree/master/experiments/scenario/.
9. ^Trees data is open and available, see Section 6 of the paper.
References
Ancrenaz, M., Gumal, M., Marshall, A. J., Meijaard, E., Wich, S. A., and Husson, S. (2016). “Pongo pygmaeus (errata version published in 2018),” in The IUCN Red List of Threatened Species 2016: e.T17975A12380922 (accessed June 24, 2022).
Ancrenaz, M., Sollmann, R., Meijaard, E., Hearn, A. J., Ross, J., Samejima, H., et al. (2014). Coming down from the trees: Is terrestrial activity in Bornean orangutans natural or disturbance driven? Sci. Rep. 4:4024. doi: 10.1038/srep04024
Ashbury, A. M., Meric de Bellefon, J., Kunz, J. A., Abdullah, M., Marzec, A. M., Fryns, C., et al. (2022). After the smoke has cleared: Extended low fruit productivity following forest fires decreased gregariousness and social tolerance among wild female Bornean orangutans (Pongo pygmaeus wurmbii). Int. J. Primatol. 43, 189–215. doi: 10.1007/s10764-021-00263-x
Balai Taman Nasional Sebangau (2015). Buku Statistik Taman Nasional Sebangau Tahun 2014. Balai Taman Nasional Sebangau.
Bialozyt, R., Flinkerbusch, S., Niggemann, M., and Heymann, E. W. (2014). Predicting the seed shadows of a Neotropical tree species dispersed by primates using an agent-based model with internal decision making for movements. Ecol. Model. 278, 74–84. doi: 10.1016/j.ecolmodel.2014.02.004
Bonnell, T. R., Campennì, M., Chapman, C. A., Gogarten, J. F., Reyna-Hurtado, R. A., Teichroeb, J. A., et al. (2013). Emergent group level navigation: an agent-based evaluation of movement patterns in a folivorous primate. PLoS ONE 8:e78264. doi: 10.1371/journal.pone.0078264
Chappell, J., and Thorpe, S. K. S. (2022). The role of great ape behavioral ecology in One Health: Implications for captive welfare and re-habilitation success. Am. J. Primatol. 84:e23328. doi: 10.1002/ajp.23328
Chérel, G., Cottineau, C., and Reuillon, R. (2015). Beyond corroboration: Strengthening model validation by looking for unexpected patterns. PLoS ONE 10, 1–28. doi: 10.1371/journal.pone.0138212
Deb, K., Pratap, A., Agarwal, S., and Meyarivan, T. (2002). A fast and elitist multiobjective genetic algorithm: NSGA-II. IEEE Trans. Evol. Comput. 6, 182–197. doi: 10.1109/4235.996017
Erb, W. M., Barrow, E. J., Hofner, A. N., Utami-Atmoko, S. S., and Vogel, E. R. (2018). Wildfire smoke impacts activity and energetics of wild Bornean orangutans. Sci. Rep. 8:7606. doi: 10.1038/s41598-018-25847-1
Evers, E., De Vries, H., Spruijt, B. M., and Sterck, E. H. M. (2014). The EMO-model: An agent-based model of primate social behavior regulated by two emotional dimensions, anxiety-FEAR and satisfaction-LIKE. PLoS ONE 9:e87955. doi: 10.1371/journal.pone.0087955
Finestone, E. M., Brown, M. H., Ross, S. R., and Pontzer, H. (2018). Great ape walking kinematics: Implications for hominoid evolution. Am. J. Phys. Anthropol. 166, 43–55. doi: 10.1002/ajpa.23397
Gluckman, G. M., and Bryson, J. J. (2011). “An agent-based model of the effects of a primate social structure on the speed of natural selection,” in Proceedings of the 13th Annual Conference Companion on Genetic and Evolutionary Computation, GECCO '11 (New York, NY: Association for Computing Machinery), 535–542. doi: 10.1145/2001858.2002045
Goossens, B., Setchell, J. M., James, S. S., Funk, S. M., Chikhi, L., Abulani, A., et al. (2006). Philopatry and reproductive success in Bornean orang-utans (Pongo pygmaeus). Mol. Ecol. 15, 2577–2588. doi: 10.1111/j.1365-294X.2006.02952.x
Grimm, V., Berger, U., Bastiansen, F., Eliassen, S., Ginot, V., Giske, J., et al. (2006). A standard protocol for describing individual-based and agent-based models. Ecol. Model. 198, 115–126. doi: 10.1016/j.ecolmodel.2006.04.023
Grimm, V., Berger, U., DeAngelis, D. L., Polhill, J. G., Giske, J., and Railsback, S. F. (2010). The ODD protocol: a review and first update. Ecol. Model. 221, 2760–2768. doi: 10.1016/j.ecolmodel.2010.08.019
Grimm, V., and Railsback, S. F. (2012). Pattern-oriented modelling: a 'multi-scope' for predictive systems ecology. Philos. Trans. Biol. Sci. 367, 298–310. doi: 10.1098/rstb.2011.0180
Grimm, V., Railsback, S. F., Vincenot, C. E., Berger, U., Gallagher, C., Deangelis, D. L., et al. (2020). The ODD protocol for describing agent-based and other simulation models: a second update to improve clarity, replication, and structural realism. JASSS 23:7. doi: 10.18564/jasss.4259
Harrison, M., Page, S., and Limin, S. (2009). The global impact of Indonesian forest fires. Biologist 56, 156–163.
Harrison, M. E. (2009). Orang-utan feeding behaviour in Sabangau, Central Kalimantan (Ph.D. dissertation). University of Cambridge, Cambridge, United Kingdom.
Harrison, M. E., Morrogh-Bernard, H. C., and Chivers, D. J. (2010). Orangutan energetics and the influence of fruit Availability in the nonmasting peat-swamp forest of sabangau, Indonesian Borneo. Int. J. Primatol. 31, 585–607. doi: 10.1007/s10764-010-9415-5
Hayasaka, H., Usup, A., and Naito, D. (2020). New approach evaluating Peatland fires in Indonesian factors. Remote Sensing 12:2055. doi: 10.3390/rs12122055
Husson, S. J., Limin, S. H., Adul Boyd, N. S., Brousseau, J. J., Collier, S., et al. (2018). Biodiversity of the Sebangau tropical peat swamp forest, Indonesian Borneo. Mires Peat 22, 1–50.
Husson, S. J., Morrogh-Bernard, H. C., Santiano Purwanto, A., Harsanto, F. A., McLardy, C., and D'Arcy, L. J. (2015). “Bornean orangutans in the Sabangau peat-swamp forest,” in State of the Apes: Industrial Agriculture and Ape Conservation (New York, NY: The Arcus Foundation), 200–207.
Imron, M. A., Gergs, A., and Berger, U. (2012). Structure and sensitivity analysis of individual-based predator-prey models. Reliabil. Eng. Syst. Saf. 107, 71–81. doi: 10.1016/j.ress.2011.07.005
Imron, M. A., Herzog, S., and Berger, U. (2011). The influence of agroforestry and other land-use types on the persistence of a Sumatran tiger (Panthera tigris sumatrae) population: an individual-based model approach. Environ. Manage. 48, 276–288. doi: 10.1007/s00267-010-9577-0
Imron, M. A., Widyastuti, K., Al Bihad, D., Satria, R. A., Prayoga, W., Pradopo, S. T., et al. (2022). Beyond climatic variation: human disturbances alter the effectiveness of a protected area to reduce fires in a tropical Peatland. Front. For. Glob. Change 5:788023. doi: 10.3389/ffgc.2022.788023
Knott, C. D. (1998). Changes in orangutan caloric intake, energy balance, and ketones in response to fluctuating fruit availability. Int. J. Primatol. 19, 1061–1079. doi: 10.1023/A:1020330404983
Legriel, J., Le Guernic, C., Cotton, S., and Maler, O. (2010). “Approximating the pareto front of multi-criteria optimization problems,” in Tools and Algorithms for the Construction and Analysis of Systems, eds J. Esparza and R. Majumdar (Berlin; Heidelberg: Springer Berlin Heidelberg), 69–83. doi: 10.1007/978-3-642-12002-2_6
Liebhold, A., Koenig, W., and Bjornstad, O. (2004). Spatial synchrony in population dynamics. Annu. Rev. Ecol. Evol. Syst. 35, 467–490. doi: 10.1146/annurev.ecolsys.34.011802.132516
Manduell, K. L. (2012). Habitat variation and its influence on the locomotor ecology of wild orangutans (Thesis), University of Birmingham, Birmingham, United Kingdom.
Manduell, K. L., Harrison, M. E., and Thorpe, S. K. S. (2012). Forest structure and support availability influence orangutan locomotion in Sumatra and Borneo. Am. J. Primatol. 74, 1128–1142. doi: 10.1002/ajp.22072
Manduell, K. L., Morrogh-Bernard, H. C., and Thorpe, S. K. S. (2011). Locomotor behavior of wild orangutans (Pongo pygmaeus wurmbii) in disturbed peat swamp forest, Sabangau, Central Kalimantan, Indonesia. Am. J. Phys. Anthropol. 145, 348–359. doi: 10.1002/ajpa.21495
McLane, A. J., Semeniuk, C., McDermid, G. J., and Marceau, D. J. (2011). The role of agent-based models in wildlife ecology and management. Ecol. Model. 222, 1544–1556. doi: 10.1016/j.ecolmodel.2011.01.020
Meijaard, E., Buchori, D., Hadiprakarsa, Y., Utami-Atmoko, S. S., Nurcahyo, A., Tjiu, A., et al. (2011). Quantifying killing of orangutans and human-orangutan conflict in Kalimantan, Indonesia. PLoS ONE 6:e27491. doi: 10.1371/journal.pone.0027491
Menberg, K., Heo, Y., and Choudhary, R. (2016). Sensitivity analysis methods for building energy models: Comparing computational costs and extractable information. Energy Build. 133, 433–445. doi: 10.1016/j.enbuild.2016.10.005
Morris, M. D. (1991). Factorial sampling plans for preliminary computational experiments. Technometrics 33, 161–174. doi: 10.1080/00401706.1991.10484804
Morrogh-Bernard, H. (2009). Orang-utan behavioural ecology in the Sabangau peat-swamp forest, Borneo (Thesis). University of Cambridge, Cambridge, United Kingdom.
Morrogh-Bernard, H. C., Husson, S. J., Harsanto, F. A., and Chivers, D. J. (2014). Fine-scale habitat use by Orang-Utans in a disturbed peat swamp forest, central Kalimantan, and implications for conservation management. Folia Primatol. Int. J. Primatol. 85, 135–153. doi: 10.1159/000358251
Morrogh-Bernard, H. C., Husson, S. J., Knott, C. D., Wich, S. A., Schaik, C. P. V., Noordwijk, M. A. V., et al. (2009). Orangutan Activity Budgets and Diet. New York, NY: Oxford University Press. doi: 10.1093/acprof:oso/9780199213276.003.0008
Nabe-Nielsen, J., van Beest, F. M., Grimm, V., Sibly, R. M., Teilmann, J., and Thompson, P. M. (2018). Predicting the impacts of anthropogenic disturbances on marine populations. Conserv. Lett. 11:e12563. doi: 10.1111/conl.12563
Nathan, R., Getz, W. M., Revilla, E., Holyoak, M., Kadmon, R., Saltz, D., et al. (2008). A movement ecology paradigm for unifying organismal movement research. PNAS. 105, 19052–19059. doi: 10.1073/pnas.0800375105
National Research Council (2003). Nutrient Requirements of Nonhuman Primates: Second Revised Edition. Washington, DC: The National Academies Press.
Newton, A. C., Boscolo, D., Ferreira, P. A., Lopes, L. E., and Evans, P. (2018). Impacts of deforestation on plant-pollinator networks assessed using an agent based model. PLoS ONE 13:e209406. doi: 10.1371/journal.pone.0209406
Passerat-Palmbach, J., Reuillon, R., Leclaire, M., Makropoulos, A., Robinson, E. C., Parisot, S., et al. (2017). Reproducible large-scale neuroimaging studies with the OpenMOLE workflow management system. Front. Neuroinform. 11:21 doi: 10.3389/fninf.2017.00021
Pastore, M., and Calcagnì, A. (2019). Measuring distribution similarities between samples: a distribution-free overlapping index. Front. Psychol. 10:1089. doi: 10.3389/fpsyg.2019.01089
Pietzsch, B. W., Peter, F. J., and Berger, U. (2021). The effect of sanitation felling on the spread of the European spruce bark Beetle-an individual-based modeling approach. Front. For. Glob. Change 4:704930. doi: 10.3389/ffgc.2021.704930
Preuschoft, S., Yassir, I., Putri, A. I., Aoliya, N., Yuliani, E., Badriyah, S. N., et al. (2021). Learning to be an orangutan-implications of life history for orangutan rehabilitation. Animals 11:767. doi: 10.3390/ani11030767
Rachmawati, D., Imron, M. A., and Suyoko, S. (2022). Return after fire: the use of burnt area for nesting of Bornean orangutan (Pongo pygmaeus wurmbii) in Sebangau national Park Indonesia. Am J. Priminol. [Un published].
Railsback, S. F., and Grimm, V. (2019). Agent-Based and Individual-Based Modeling A Practical Introduction, 2nd Edn. New Jersey: Princeton University Press.
Raimbault, J. (2018). Calibration of a density-based model of urban morphogenesis. PLoS ONE 13:e0203516. doi: 10.1371/journal.pone.0203516
Raimbault, J., Broere, J., Somveille, M., Serna, J. M., Strombom, E., Moore, C., et al. (2020). A spatial agent based model for simulating and optimizing networked eco-industrial systems. Resour. Conserv. Recycl. 155:104538. doi: 10.1016/j.resconrec.2019.104538
Rayadin, Y., and Spehar, S. N. (2015). Body mass of wild Bornean orangutans living in human-dominated landscapes: implications for understanding their ecology and conservation. Am. J. Phys. Anthropol. 157, 339–346. doi: 10.1002/ajpa.22709
Reuillon, R., Chuffart, F., Leclaire, M., Faure, T., Dumoulin, N., and Hill, D. (2010). “Declarative task delegation in OpenMOLE,” in 2010 International Conference on High Performance Computing Simulation (Caen), 55–62. doi: 10.1109/HPCS.2010.5547155
Reuillon, R., Leclaire, M., and Passerat-Palmbach, J. (2015). “Model exploration using openmole a workflow engine for large scale distributed design of experiments and parameter tuning,” in 2015 International Conference on High Performance Computing Simulation (HPCS), 1–8. doi: 10.1109/HPCSim.2015.6747237015
Reuillon, R., Leclaire, M., and Rey-Coyrehourcq, S. (2013). OpenMOLE, a workflow engine specifically tailored for the distributed exploration of simulation models. Fut. Gener. Comput. Syst. 29, 1981–1990. doi: 10.1016/j.future.2013.05.003
Russon, A. (2009). “Orangutan rehabilitation and reintroduction,” in Orangutans: Geographic Variation in Behavioral Ecology and Conservation, eds S. A. Wich, S. S. U. Atmoko, T. M. Setia, and C. P. van Schaik (Oxford: Oxford University Press), 327–350. doi: 10.1093/acprof:oso/9780199213276.003.0023
Schmid, J. S., Taubert, F., Wiegand, T., Sun, I. F., and Huth, A. (2020). Network science applied to forest megaplots: tropical tree species coexist in small-world networks. Sci. Rep. 10:13198. doi: 10.1038/s41598-020-70052-8
Schmitt, C., Rey-Coyrehourcq, S., Reuillon, R., and Pumain, D. (2015). Half a billion simulations: evolutionary algorithms and distributed computing for calibrating the simpoplocal geographical model. Environ. Plann. B Plann. Design 42, 300–315. doi: 10.1068/b130064p
Seaman, D. J. I., Bernard, H., Ancrenaz, M., Coomes, D., Swinfield, T., Milodowski, D. T., et al. (2019). Densities of Bornean orang-utans (Pongo pygmaeus morio) in heavily degraded forest and oil palm plantations in Sabah, Borneo. Am. J. Primatol. 81, 1–12. doi: 10.1002/ajp.23030
Seaman, D. J. I., Voigt, M., Bocedi, G., Travis, J. M. J., Palmer, S. C. F., Ancrenaz, M., et al. (2021). Orangutan movement and population dynamics across human-modified landscapes: implications of policy and management. Landsc. Ecol. 36, 2957–2975. doi: 10.1007/s10980-021-01286-8
Semeniuk, C. A., Musiani, M., and Marceau, D. J. (2011). “Integrating spatial behavioral ecology in agent-based models for species conservation,” in Biodiversity, Chapter 1, ed A. Sofo (Rijeka: IntechOpen). doi: 10.5772/23055
Sherman, J., Ancrenaz, M., and Meijaard, E. (2020). Shifting apes: conservation and welfare outcomes of Bornean orangutan rescue and release in Kalimantan, Indonesia. J. Nat. Conserv. 55:125807. doi: 10.1016/j.jnc.2020.125807
Tang, W., and Bennett, D. A. (2010). Agent-based modeling of animal movement: a review. Geogr. Compass 4, 682–700. doi: 10.1111/j.1749-8198.2010.00337.x
Tarszisz, E., Tomlinson, S., Harrison, M. E., Morrogh-Bernard, H. C., and Munn, A. J. (2018a). An ecophysiologically informed model of seed dispersal by orangutans: linking animal movement with gut passage across time and space. Conserv. Physiol. 6:coy013. doi: 10.1093/conphys/coy013
Tarszisz, E., Tomlinson, S., Harrison, M. E., Morrogh-Bernard, H. C., and Munn, A. J. (2018b). Gardeners of the forest: Effects of seed handling and ingestion by orangutans on germination success of peat forest plants. Biol. J. Linnean Soc. 124, 278–278. doi: 10.1093/biolinnean/bly037
Thorpe, S. K. S., and Crompton, R. H. (2006). Orangutan positional behavior and the nature of arboreal locomotion in Hominoidea. Am. J. Phys. Anthropol. 131, 384–401. doi: 10.1002/ajpa.20422
Thorpe, S. K. S., Crompton, R. H., and Alexander, R. M. N. (2007). Orangutans use compliant branches to lower the energetic cost of locomotion. Biol. Lett. 3, 253–256. doi: 10.1098/rsbl.2007.0049
van Casteren, A., Sellers, W. I., Thorpe, S. K. S., Coward, S., Crompton, R. H., and Ennos, A. R. (2013). Factors affecting the compliance and sway properties of tree branches used by the Sumatran orangutan (Pongo abelii). PLoS ONE. 8:e67877. doi: 10.1371/journal.pone.0067877
Van Casteren, A., Sellers, W. I., Thorpe, S. K. S., Coward, S., Crompton, R. H., Myatt, J. P., et al. (2012). Nest-building orangutans demonstrate engineering know-how to produce safe, comfortable beds. Proc. Natl. Acad. Sci. U.S.A. 109, 6873–6877. doi: 10.1073/pnas.1200902109
van Noordwijk, M. A., Arora, N., Willems, E. P., Dunkel, L. P., Amda, R. N., Mardianah, N., et al. (2012). Female philopatry and its social benefits among Bornean orangutans. Behav. Ecol. Sociobiol. 66, 823–834. doi: 10.1007/s00265-012-1330-7
Vincenot, C. E., Mazzoleni, S., Moriya, K., Cartenì, F., and Giannino, F. (2015). How spatial resource distribution and memory impact foraging success: a hybrid model and mechanistic index. Ecol. Complex. 22, 139–151. doi: 10.1016/j.ecocom.2015.03.004
Wang, M., and Grimm, V. (2007). Home range dynamics and population regulation: an individual-based model of the common shrew Sorex araneus. Ecol. Model. 205, 397–409. doi: 10.1016/j.ecolmodel.2007.03.003
Widyastuti, K., Ali, I. M., Tri, P. S., Hatma, S., Maya, S. B., Allan, S., et al. (2021). PeatFire: an agent-based model to simulate fire ignition and spreading in a tropical peatland ecosystem. Int. J. Wildlife. 30, 71–89. doi: 10.1071/WF19213
Widyastuti, K., Harrison, M. E., Morrogh-Bernard, H., Nasir, D., Imron, M. A., and Berger, U. (2022a). Orangutan habitat survey in Sebangau National Park, Central Kalimantan, Indonesia [Data set]. Zenodo. doi: 10.5281/zenodo.6783829
Widyastuti, K., Reuillon, R., Chapron, P., Abdussalam, W., Nasir, D., Harrison, M. E., et al. (2022b). BORNEO: arBOReal aNimal movEment mOdel. Zenodo. doi: 10.5281/zenodo.6784851
Wilensky, U. (1999). NetLogo. Available online at: http://ccl.northwestern.edu/netlogo/
Keywords: primates, peatland, energy budget, calibration, optimization, individual-based model, movement, fires
Citation: Widyastuti K, Reuillon R, Chapron P, Abdussalam W, Nasir D, Harrison ME, Morrogh-Bernard H, Imron MA and Berger U (2022) Assessing the impact of forest structure disturbances on the arboreal movement and energetics of orangutans—An agent-based modeling approach. Front. Ecol. Evol. 10:983337. doi: 10.3389/fevo.2022.983337
Received: 30 June 2022; Accepted: 29 August 2022;
Published: 23 September 2022.
Edited by:
Sandrine Charles, Université de Lyon, FranceReviewed by:
Christian E. Vincenot, Kyoto University, JapanSteven Railsback, Cal Poly Humboldt, United States
Volker Grimm, Helmholtz Association of German Research Centres (HZ), Germany
Copyright © 2022 Widyastuti, Reuillon, Chapron, Abdussalam, Nasir, Harrison, Morrogh-Bernard, Imron and Berger. This is an open-access article distributed under the terms of the Creative Commons Attribution License (CC BY). The use, distribution or reproduction in other forums is permitted, provided the original author(s) and the copyright owner(s) are credited and that the original publication in this journal is cited, in accordance with accepted academic practice. No use, distribution or reproduction is permitted which does not comply with these terms.
*Correspondence: Kirana Widyastuti, a2lyYW5hLndpZHlhc3R1dGlAdHUtZHJlc2Rlbi5kZQ==; Mark E. Harrison, bS5lLmhhcnJpc29uQGV4ZXRlci5hYy51aw==
†These authors share last authorship
‡ORCID: Kirana Widyastuti orcid.org/0000-0003-2507-5570
Mark E. Harrison orcid.org/0000-0002-0729-8407
Muhammad Ali Imron orcid.org/0000-0003-2371-7795