- 1Department of Earth and Environmental Sciences, Rutgers University–Newark, Newark, NJ, United States
- 2Department of Biological Sciences, Rutgers University–Newark, Newark, NJ, United States
The riverine barrier hypothesis has been extensively explored in Neotropical rainforests, while its importance in drier regions such as the Caatinga, a seasonally dry tropical forest in northeastern Brazil, has only recently received more attention. The Caatinga is bisected by the São Francisco River (SFR), which has long been suggested to be an important biogeographic feature in the region. However, recent studies have found mixed support for the role of the SFR as a hard barrier, most of them relying on the presence or absence of genetic breaks congruent with its course. Here, we used published multilocus and next-generation data from six vertebrate species to test the SFR’s strength as a barrier. Using model-based approaches (approximate Bayesian computation and supervised machine learning), we tested demographic models incorporating full, intermediate, and zero migration across the SFR, estimating divergence times and migration rates for each species. We found support for the SFR’s role as a barrier, allowing gene flow for some species. Estimated divergence times varied among species but are limited to the late Pleistocene, coherent with one of several proposed paleocourse changes in the river’s geological history. Contrary to the mixed results of previous studies, our study supports the SFR as an important phylogeographic barrier across different taxonomic groups, driving diversification in the Caatinga.
Introduction
Rivers have long been proposed to be vicariant barriers driving biological diversification in the Neotropics. Wallace (1854) originally proposed what would come to be called the “riverine barrier hypothesis,” suggesting that the Amazon River structures primate populations. The hypothesis has since proved controversial, with early molecular studies offering both support (Capparella, 1988) and rejection (Patton et al., 1994), and more modern phylogeographical research continuing to show Amazon-driven population structure and speciation in some taxa (Moraes et al., 2016, 2020; Naka and Brumfield, 2018; Pirani et al., 2019; Naka and Pil, 2020), but not in others (Santorelli et al., 2018; Fraga and Carvalho, 2021). Riverine effects on species diversification may indeed be more complex than simply acting as vicariant agents—they may also act as dispersal routes (Lawson, 2013; Fonseca et al., 2021). The role of rivers in Neotropical diversification has nonetheless been explored extensively in rainforests. On the other hand, their importance in drier Neotropical regions such as the Caatinga of Brazil has only recently accrued scientific interest.
The Caatinga, located in northeastern Brazil, is a seasonally dry tropical forest characterized by sporadic rainfall and frequent drought (Salgado et al., 2015). The Caatinga is bisected by the São Francisco River (SFR), the fourth-longest river in Brazil at nearly 3,000 km, and the region’s only perennial watercourse. The SFR runs northward from Minas Gerais and then elbows eastward through the Caatinga before flowing into the South Atlantic ocean, forming the boundary between Alagoas and Sergipe states (Figure 1). Rodrigues (1986) was the first to suggest that the SFR forms a vicariant barrier to Caatinga taxa, later demonstrating allopatric differentiation across the river in several reptile species and populations (Rodrigues, 1996; Rodrigues and Juncá, 2002). Thereafter, the authors of subsequent phylogeographic studies on Caatinga taxa have cited the São Francisco River Hypothesis (SFRH) as an explanation for observed biodiversity patterns in the region. Though literature on the SFR’s geological history is sparse, it indicates that the river shifted from a straight south-to-north paleocourse, emptying into the North Atlantic near present-day Piaui state (Figure 1A; Grabert, 1968), to its eastward-elbowing present course, splitting the formerly continuous Caatinga into northern and southern halves. The SFR may have first reached the South Atlantic through meanders south of its present mouth (Figure 1A) before shifting northward (King, 1957). The principal paleocourse change possibly occurred during the Mindel glaciation of the Middle Pleistocene ∼450 kya (Mabesoone, 1994), but the timing is controversial, with some authors suggesting its occurrence as far back as the Pliocene–early Pleistocene (Ribeiro et al., 2021), mid-Miocene (Potter, 1997), or mid-Eocene (Karner and Driscoll, 1999).
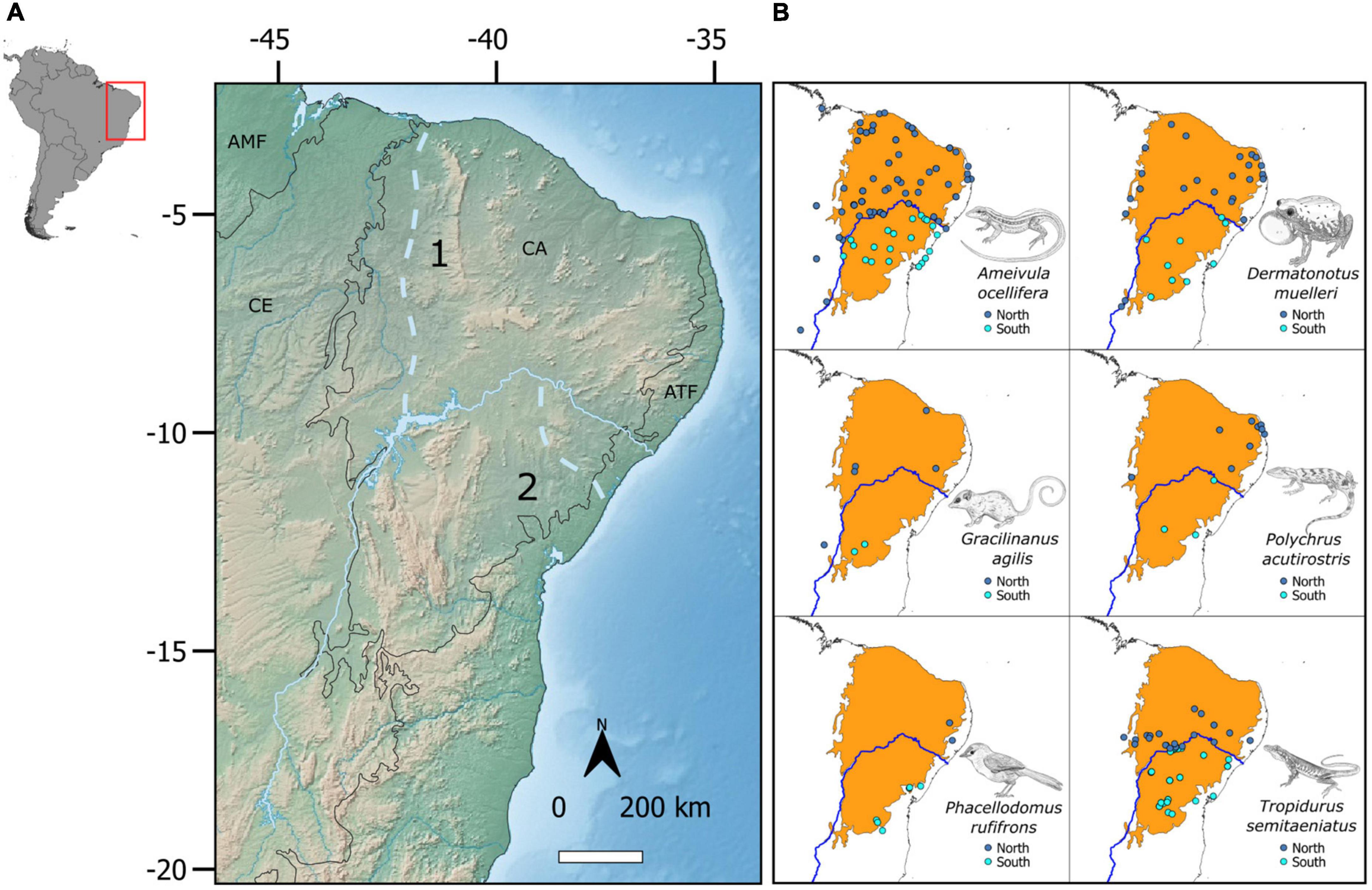
Figure 1. (A) Extent of the Caatinga in Brazil, bisected by the São Francisco River in its current course (light blue continuous line) and paleocourse (light blue dotted line). 1—Older paleocourse before elbowing east; 2—More recent paleocourse south of modern course; CA, Caatinga; AMF, Amazonia; ATF, Atlantic Forest; CE, Cerrado. (B) The distribution of samples of six vertebrate species used to test the São Francisco River (blue line) Hypothesis in the Caatinga.
Since Rodrigues’s pioneering SFRH studies, more recent phylogeographic studies on a variety of Caatinga taxa have found mixed support for the SFRH. Certain squamates (Machado et al., 2014; Fonseca et al., 2018), frogs (Oliveira et al., 2018a; Thomé et al., 2021a), birds (Corbett et al., 2020), insects (Andrade-Souza et al., 2017), and plants (Balbino et al., 2018) exhibit no genetic break totally concordant with the SFR. On the other hand, concordant genetic breaks indicate that the river clearly structures other lizards (Passoni et al., 2008; Siedchlag et al., 2010; Werneck et al., 2012; Almeida et al., 2020), frogs (Bruschi et al., 2019), mammals (Nascimento et al., 2011; Faria et al., 2013; Fegies et al., 2021), fishes (Costa et al., 2018), and plants (Menezes et al., 2016). Meanwhile, the SFR acts merely as a soft barrier for certain species, contributing to fine interpopulation structure while allowing gene flow across its margins (Werneck et al., 2015; Miranda et al., 2016; Oliveira et al., 2018b; Lanna et al., 2020; Thomé et al., 2021b).
The apparent lack of a genetic break concordant with the SFR shown in some studies, with mixed individuals on either side of the river, does not necessarily invalidate the SFRH. The size of the population relative to divergence time should temper expectations of perfect concordance. From coalescent theory, the average waiting time for all samples to coalesce is approximately four times population size (in number of generations), with a standard deviation of approximately two times population size (Kingman, 1982). Thus, assuming a population split due to the SFR’s paleocourse change ∼450 kya, the effective sizes of the two daughter populations would have to be fairly small for all gene trees to be concordant with the split. For small populations, where gene tree concordance can be expected, migration can have a strong effect as well. Under the Wright-Fisher model of genetic drift, a single migrant per generation will prevent populations from diverging (Wright, 1931). Thus, even low migration, which is expected in the case of a soft barrier, can prevent allele fixation, producing a “fuzzy” genetic break with coalescent trees discordant with any historical split. It is imperative to model the effects of these factors when testing a geographic barrier, but only a few studies to date have tested the SFRH with a model-based approach (Oliveira et al., 2015; Miranda et al., 2016; Bruschi et al., 2019; Lanna et al., 2020; Thomé et al., 2021b).
Our goals in this study are twofold. We first illustrate with an in silico experiment the effect of population size and migration on expectations of gene tree/population tree concordance. Second, we used coalescent simulations to test whether the SFRH holds for various taxa. We fit published Sanger and next-generation data from six vertebrate species to three demographic models with approximate Bayesian computation (ABC) and supervised machine learning (SML). The (i) Isolation model assumes the SFR is a hard barrier; the (ii) Migration model assumes the SFR is a soft barrier; and the (iii) Panmictic model assumes the SFR is not a barrier at all. Incomplete lineage sorting (ILS) and migration can generate similar patterns of gene tree/species tree discordance (Degnam and Rosenberg, 2006). Therefore, instead of making conclusions based solely on the probability of each model, we also estimated and compared two parameters of interest for each species for models i and ii: divergence time and migration across the river. If the SFR is not a barrier, we expect to find higher support for the Panmictic model. A very recent divergence time posterior, nearing zero, and a very high migration rate posterior, nearing the upper limit of the prior, would reject the SRFH even if the Panmictic model is not of highest probability. If the SFR is a hard barrier, we expect higher support for the Isolation model, with divergence times close to or older than the SFR’s paleocourse change, and low migration rates. Higher support for the Migration model, or high migration rates and recent divergence times, would indicate that the SFR is a soft barrier. We expect a species-specific effect of the river, with the SFR as a hard barrier for terrestrial small-bodied amphibian, reptile, and mammal species, and not a barrier for the bird species.
Materials and methods
Simulation experiment
To demonstrate the effects of population size and migration on gene tree concordance, we performed two simulation experiments with ms (Hudson, 2002) via the phyclust R package (Chen, 2011). In the first experiment, we simulated a two-population isolation model with a divergence time of 400 kya. We sampled 20 haploid individuals in each population and simulated eight different constant effective population sizes with the same 400 kya divergence time. For each population size, we simulated 1,000 replicates and calculated the proportion of coalescent trees that were concordant with the population split, which can be seen as the probability of complete sorting, using the function is.monophyletic from the R package ape (Paradis and Schliep, 2019). In the second experiment, we simulated an isolation-with-migration model with two populations, a fixed population size of 10,000 individuals, and a divergence time of 400 kya. We simulated eight different migration rates (Nm; number of migrants per generation). As in the first experiment, we simulated 1,000 replicates and calculated the proportion of coalescent trees that were concordant with the population split.
Data acquisition and processing
We tested the SFRH with six model species that are distributed both north and south of the river: three lizards (Ameivula ocellifera, Polychrus acutirostris, Tropidurus semitaeniatus), a frog (Dermatonotus muelleri), a mammal (Gracilinanus agilis), and a bird (Phacellodomus rufifrons) (Figure 1B). The only criterion for a species’ inclusion was the availability of more than one locus in the GenBank database, or genomic-scale data available online (Table 1). We did not include available single-locus data because of the intrinsic wide uncertainty of time estimates associated with such datasets. Genetic data consisted of both Sanger data (mtDNA and nDNA) and genome-scale ddRADseq data. For species complexes with resolved phylogenetic relationships, such as Tropidurus semitaeniatus (Werneck et al., 2015), we retained sequences from neighboring clades separated by the SFR, excluding clades that occur in the Caatinga but far from the river. On the other hand, for species without a well-resolved phylogeny (e.g., Ameivula ocellifera), we retained all sequences available throughout its entire distribution in the Caatinga.
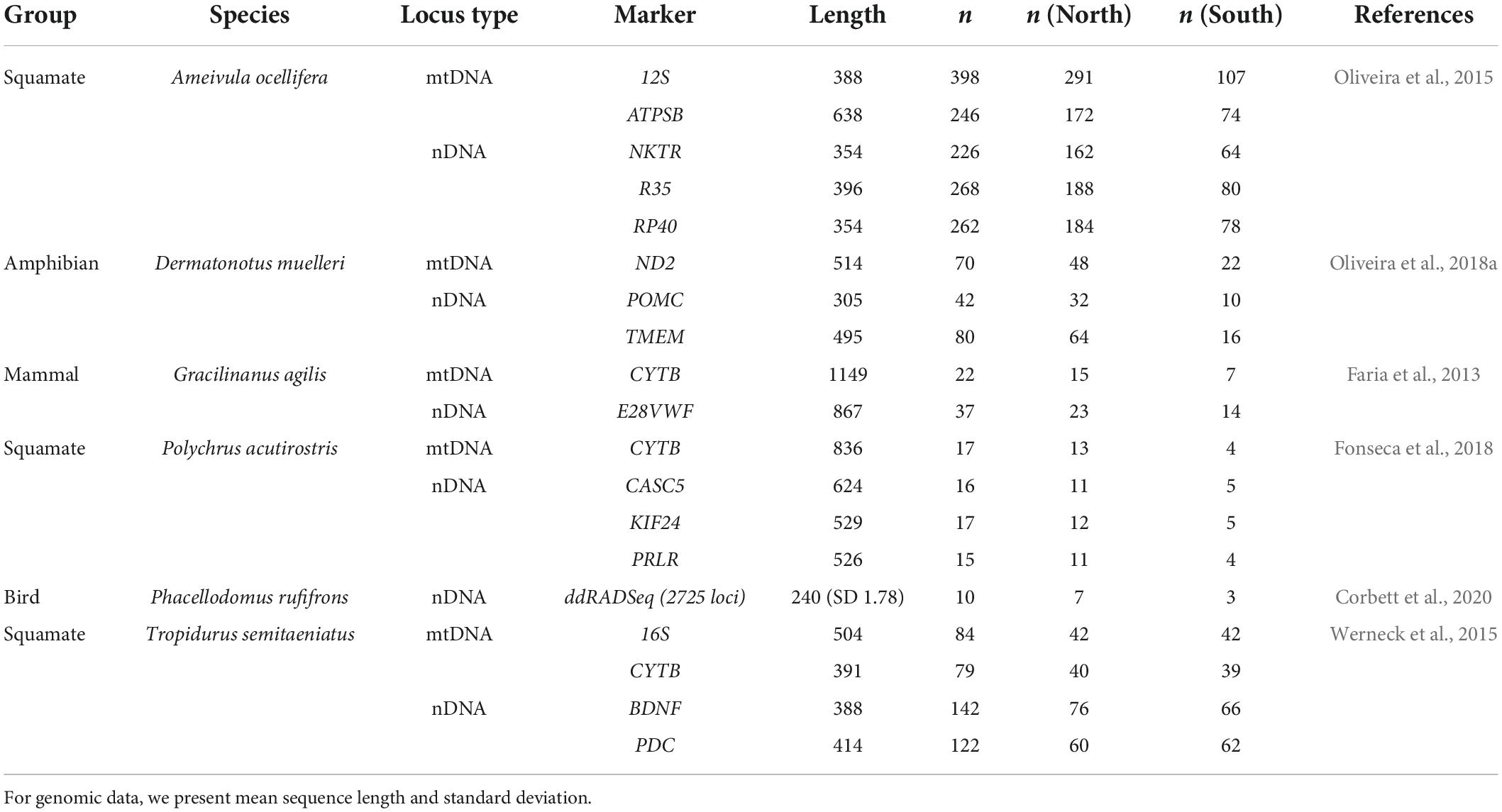
Table 1. Species, genetic markers, and sequence counts used to test the São Francisco River Hypothesis (SFRH).
For Sanger data, we aligned sequences with MUSCLE (Edgar, 2004) as implemented in Geneious 9.0.5 (Kearse et al., 2012). We phased nDNA fragments with PHASE (Stephens et al., 2001; Stephens and Donnelly, 2003) as implemented in DnaSP 5.10.1 (Librado and Rozas, 2009) and removed allele pairs with a probability lower than 0.7.
We downloaded the Ph. rufirons ddRADseq data (Corbett et al., 2020) from the NCBI Sequence Read Archive. We then used ipyrad for de novo assembly (Eaton and Overcast, 2020) and subsequent processing (see Supplementary Table 1 for parameters). Ipyrad outputs a “.alleles” file with phased loci, which we further converted to FASTA format with the function “iPyrad.alleles.loci2fasta” available in the R package PipeMaster (Gehara et al., 2020).1 Our final dataset consisted of 2,725 unlinked loci (Table 1) from 10 individuals. Before analyzing the data, we trimmed and removed all missing data from both Sanger and genomic data alignments to ensure there were no sites with unknown nucleotides.
Model description
We built three models to explore the SFR’s role in the phylogeographic histories of Caatinga species. The Isolation model (Figure 2) assumes that the river acts as a hard barrier, preventing any gene flow across the SFR. Despite the variety of proposed dates for the change in SFR’s paleocourse, for comparison with most of the phylogeographic literature on the SFRH, we assumed a vicariant event beginning ∼450 kya (Mabesoone, 1994). Our aim was to evaluate if the genetic evidence available is consistent with this date. By clearly framing this hypothesis, we allow objective testing, repeatability, and the possibility of refutation. Furthermore, we accounted for uncertainty in the timing of this event by setting a wide divergence time prior (see next section and Supplementary Table 4). The Migration model assumes that the river is a soft barrier, with no divergence time between groups (i.e., no topology) and only migration. By removing a divergence time parameter, we isolated migration as the only process that can explain the gene tree discordance in the data. Differently from an isolation-with-migration model, where both divergence time and migration can account for gene tree discordance, the Migration model allowed us to properly test the maximum migration value supported by the data. Finally, the Panmictic model disregards the river’s vicariant effect entirely so that populations occurring on each side of the river interbreed freely. It is important to emphasize that this model assumes that all samples in the data have equal chances of coalescing with one another (i.e., no population structure). Any population structure present in the data, even the neutral effect of space (i.e., Isolation by distance), violates this assumption. Thus a low probability of this model does not necessarily indicate that the river is a barrier.
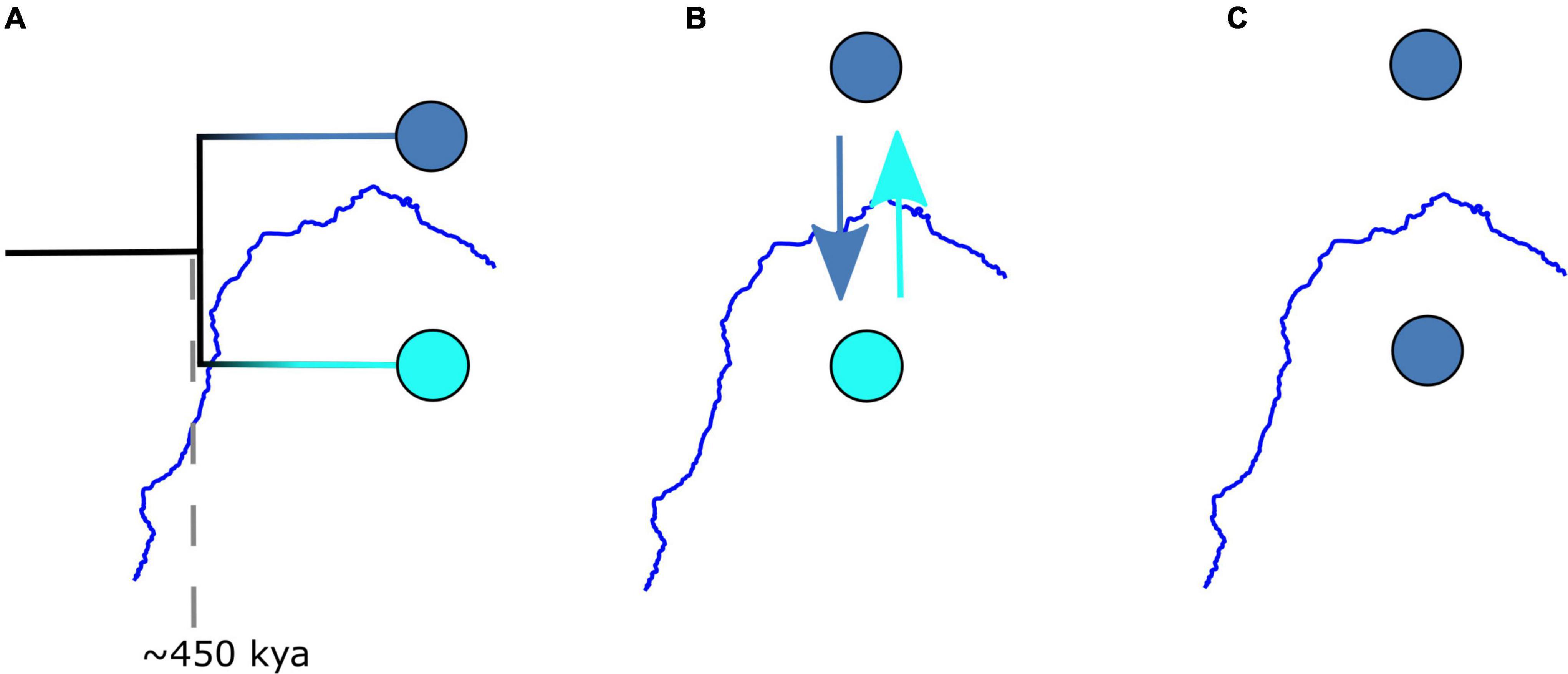
Figure 2. Representation of models tested to explain the phylogeographic history of species distributed on both sides of the São Francisco River (blue line). (A) Isolation model in which the river acts as a strong barrier isolating groups with no gene flow beginning at ∼450 kya. (B) Migration model in which the river acts as a soft barrier, allowing migration across the river with no divergence time. (C) Panmictic model, in which the river is not a barrier and populations interbreed freely.
We tested among these models by grouping sequences by locality, either North or South of the river (see Supplementary Table 2 for voucher and locality of individuals in each group). We considered individuals northwest/west of the river as North, and southeast/east as South. Even though some of the species used here do not necessarily present genetic structure delimited by the river’s current course (Werneck et al., 2015; Oliveira et al., 2018a), the lack of congruence with the river should inform model probabilities and parameter estimates. For example, for a species in which genetic structure is not perfectly delimited by the river, we may find a recent divergence time and high migration, supporting the river as a soft barrier. On the other hand, the river may be a hard barrier, and incongruence with the river may result from incomplete lineage sorting, which will result in an old divergence time in the Isolation model and low migration in the Migration model.
Model testing with approximate Bayesian computation
To test among the Isolation, Migration, and Panmictic models (Figure 2) we used the R package PipeMaster (Gehara et al., 2020; see text footnote 1). First, we calculated the following summary statistics for our observed data: segregating sites, nucleotide diversity (π), Watterson’s theta, Kelly’s ZnS, fixation index (FST), % shared polymorphisms for subpopulations, % private polymorphisms for subpopulations (private 1), and % fixed polymorphisms for subpopulations. For each summary statistic, we calculated both the average and variance across all loci for each group, North and South of the SFR.
For the Isolation model, we assumed a two-population topology with a prior divergence time ranging from 0 to 1,000,000 years. Here, we corrected (divided) time by species generation time, assuming a generation time of 2 years for lizards and 1 year for the other taxa (Puida and Paglia, 2015; Gehara et al., 2017; Batalha-Filho et al., 2019). For the Migration model, we assumed two populations with no topology or divergence time, and a migration prior ranging from 0.1–10 migrants per generation, except for Ph. rufifrons, for which we used a prior ranging from 0.1–2.5 to improve model fit. We used the same parameters for the Panmictic model except for a divergence time ranging from 0 to 1 generations. By forcing groups to coalesce in one generation, we imposed panmixia on the populations while allowing for the calculation of the same summary statistics as the other models.
We also specified species- and marker-specific priors for each model, e.g., mutation rate and effective population size. For mutation rate (μ) priors, we used information available in the literature specific to each species and marker (see Supplementary Table 3 for mutation priors and references). Where this information was not available we defined the mutation rate prior according to a general mtDNA mutation rate for vertebrates (1 × 10–8; Allio et al., 2017) and nDNA mutation rates estimated for close relatives of the study species. The mutation rate prior was then corrected by generation time. For effective population size (Ne), we used priors from previous studies (Supplementary Table 4). When this information was not available, we roughly estimated Ne using the formula Ne = π/4 × μ and set up a wide prior around the resulting value.
After calculating summary statistics from the GenBank data we simulated genetic data under the three models. PipeMaster uses a modified version of ms (Hudson, 2002; Pavlidis et al., 2010) to simulate genetic data. For each species, and each of the three models, we performed 1,000,000 and 100,000 simulations for Sanger and genomic data respectively. We assessed model fit by summarizing simulated data in a PCA and plotting against observed data and with the gfit function of abc R package (Csilléry et al., 2012). Then calculating the posterior probability of each model with the function postpr from the same package using a rejection algorithm with a tolerance of 0.0001 and 0.001 for Sanger and genomic data respectively, retaining the 100 closest simulations to the observed data. To examine model identifiability and the reliability of our results, we performed leave-one-out cross-validation with the function “cv4postpr” (Csilléry et al., 2012), using a rejection algorithm with a the same tolerances as above. We also estimated the posterior distributions of each model’s parameters with “abc,” then assessed the reliability of our results with “cv4abc,” using the same algorithm, tolerances, and sample size as before.
Model testing with supervised machine learning
In addition to ABC, we also used supervised machine learning (SML) to test the SFRH. We performed model classification with the Keras interface (Chollet, 2015) in R. We centered and scaled simulated data from PipeMaster, then partitioned 80% of the data for training and 20% for testing an artificial neural network (ANN). We built an ANN with three hidden layers, each with 32 nodes, and three units in the last layer. We used “relu” and “softmax” activation functions for the hidden and last layer respectively. We used the “adam” optimizer with loss function “sparse_categorical_crossentropy.” The ANN was trained for 1,000 epochs using 10,000 batches and a validation split of 0.1. We then used the trained ANN to classify our data into one of the three models.
After classification, we also estimated parameters with another ANN, also via Keras. For each model, we randomly retained 10% of simulations, then centered and scaled simulated data from PipeMaster to train an ANN regression model. To get a posterior density that informs the uncertainty between summary statistics and model parameters, we ran 100 replicates of the ANN regression, randomly sampling a different training dataset for each replicate. The model had the same number of hidden layers and nodes and the same activation function as that used in model classification. For the final layer, we used a single node with a linear activation function. As the optimizer, we used “RMSprop” with a loss function “mse.” We used 88% of the data to train and 12% to test the ANN respectively. We trained the ANN with 1,000 epochs, 10,000 batches, and a 0.1 validation split. Finally, we re-transformed the parameters after estimating them, undoing the steps of centering and scaling the data. We assessed the accuracy of parameter estimates by calculating correlation coefficients between testing data and estimated values. More details about optimizers, activation and loss functions can be found in the Keras manual (keras.io).
Results
Simulation experiment
The simulation experiment shows that for two populations of effective size 400,000 that diverged 400,000 generations ago, less than 0.1% of coalescent trees are concordant with the population trees. That is, 0.1% are completely sorted in both populations (Table 2) and would support reciprocally monophyletic populations on each side of the river (assuming mutation rate variation is negligible and inheritance is autosomal). Only populations with 40,000 individuals or less will have close to 100% of coalescent trees sorted. A migration rate of as few as 0.1 individuals per generation is enough to render more than 70% of coalescent trees showing a paraphyletic topology, that is, discordant with the population split (Table 3). Only very low migration values of 0.01 individuals per generation will result in a large proportion of concordant coalescent trees.
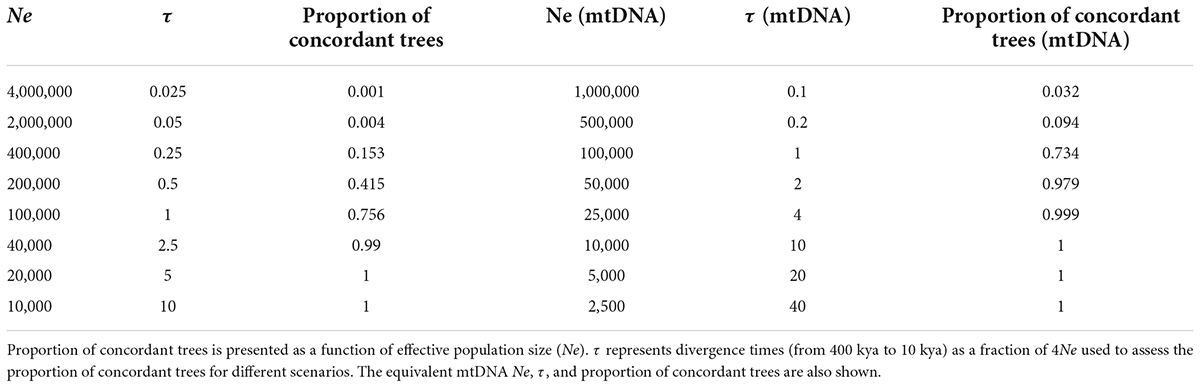
Table 2. Results of in silico experiment exploring the importance of divergence time in generating/preventing the existence of monophyletic groups (concordant gene trees).
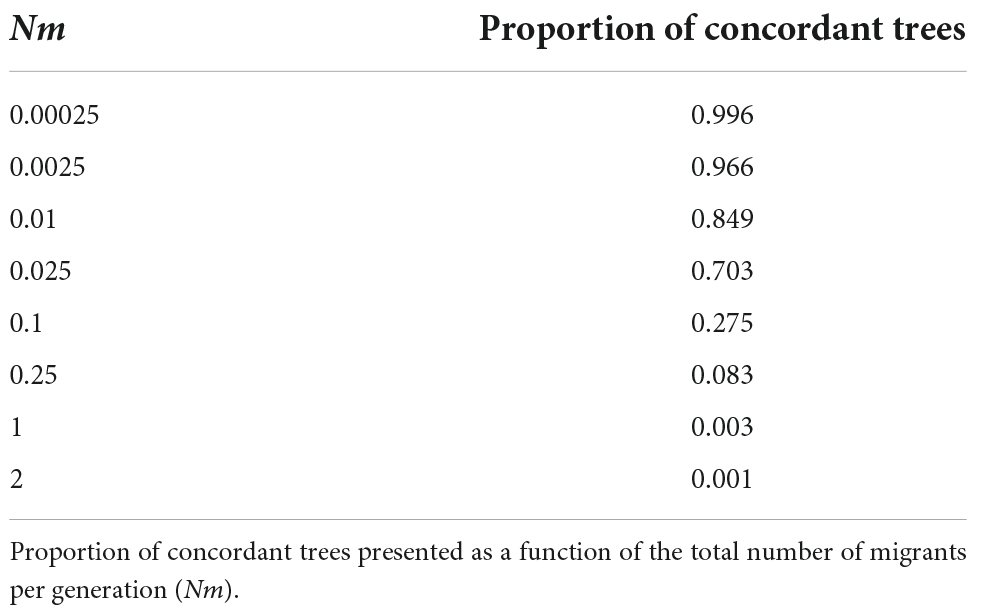
Table 3. Results of in silico experiment exploring the importance of migration in generating/preventing the existence of monophyletic groups (concordant gene trees).
Isolation vs. migration with approximate Bayesian computation
The observed data for all six species fit at least one model well (Supplementary Figure 1). All three models were identifiable as shown by the cross-validation experiment (Supplementary Figure 2). The Isolation model was the most supported model, explaining the data for four of six species (Table 4). On the other hand, the Panmictic model had the lowest probability for all species. We found high support for the Migration model only for the bird Ph. rufifrons, suggesting that the river is a soft barrier for this species. We found mixed results for the lizard Po. acutirostris and the mammal G. agilis, with both Isolation and Migration models having similar posterior probability.
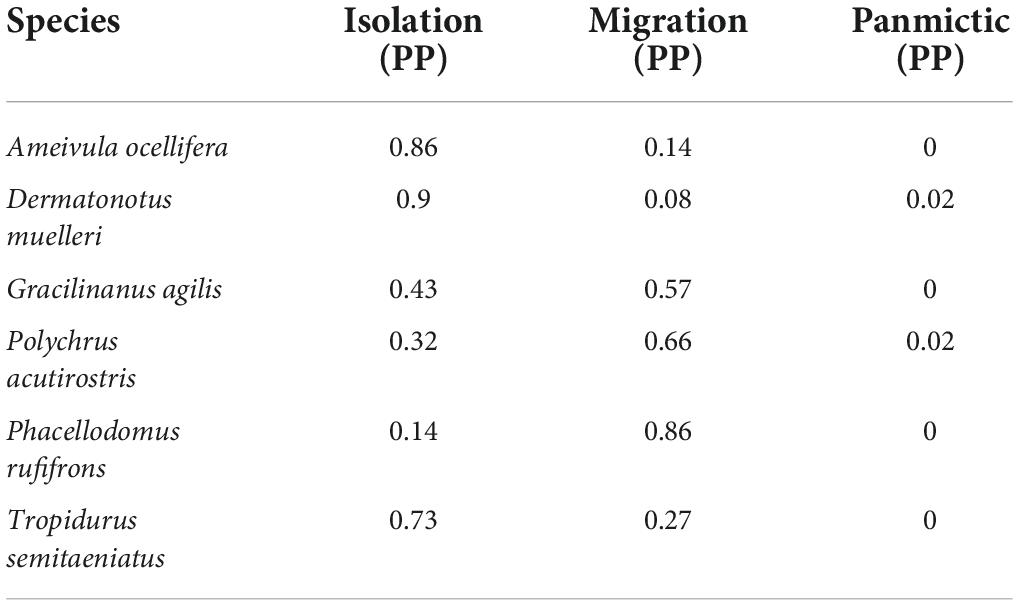
Table 4. Posterior probabilities for each model and study species from approximate Bayesian computation (ABC).
The estimated divergence time for the Isolation model was in general older than 450 kya (Figure 3 and Supplementary Table 5), reinforcing the role of the SFR as a hard barrier. However, despite good performance in cross-validation (Supplementary Figure 3), divergence times should be interpreted with caution, since they were likely affected by their prior distributions. Estimated migration rates for A. ocellifera, Po. acutirostris, and T. semitaeniatus were ∼1 individual per generation, while more than 1 for G. agilis and D. muelleri, and less than one for Ph. rufifrons (Figure 3 and Supplementary Table 5). However, cross-validation for migration showed low accuracy for this parameter for G. agilis (Supplementary Figure 4).
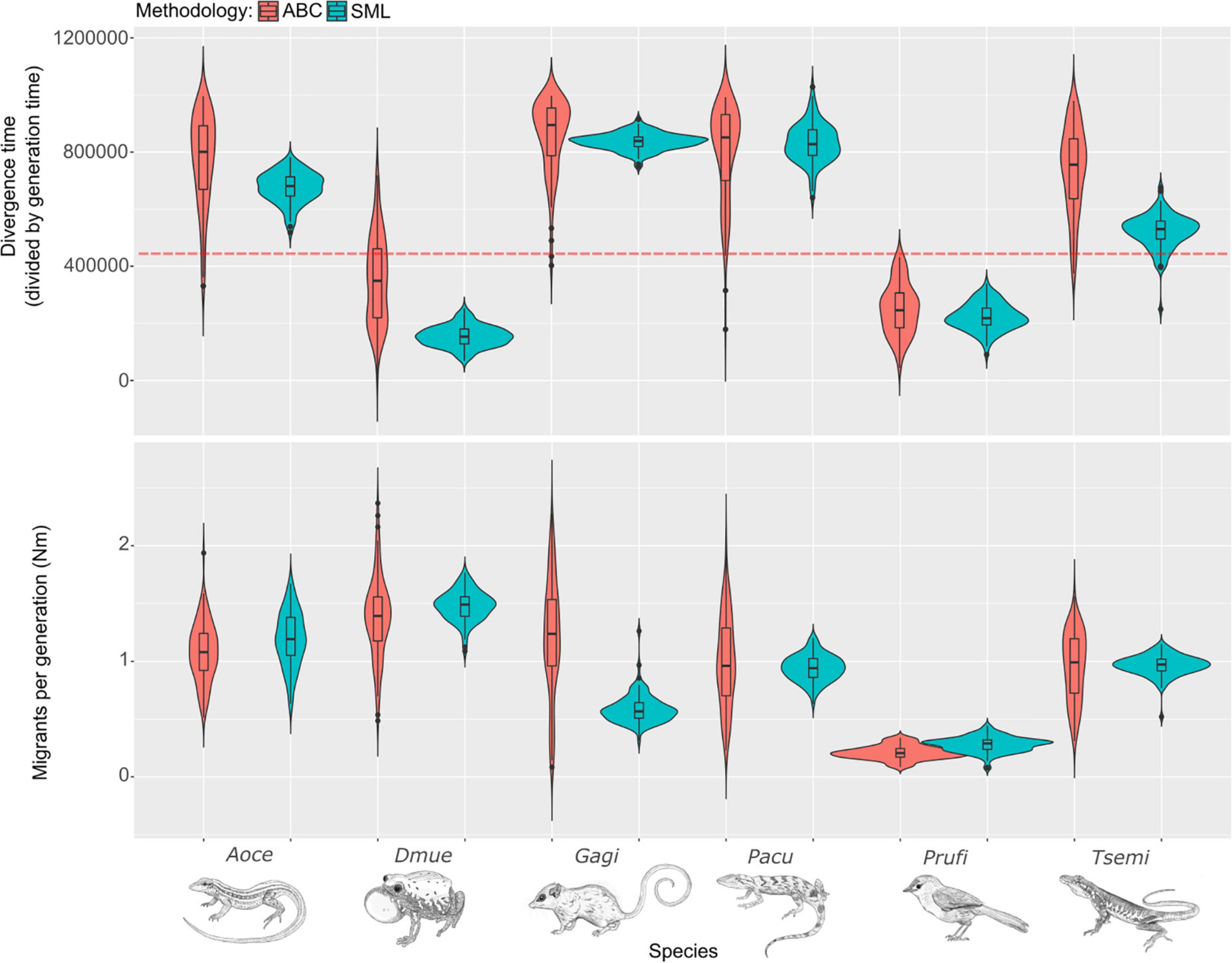
Figure 3. Divergence time and migrants per generation estimated with approximate Bayesian computation (ABC) and supervised machine learning (SML) for each study species. Violins show the density of estimated values for each parameter. The dotted orange line marks ∼450 kya. Boxplots within violins show median, first and third quartile, and minimum and maximum values estimated. Nm, number of migrants; Aoce, Ameivula ocellifera; Dmue, Dermatonotus muelleri; Gagi, Gracilinanus agilis; Pacu, Polychrus acutirostris; Prufi, Phacellodomus rufifrons; Tsemi, Tropidurus semitaeniatus.
Isolation vs. migration with supervised machine learning
Model classification with SML recovered a result similar to that of ABC analysis, with low support for the Panmictic model (Table 5). However, as opposed to ABC, SML recovered high support for the Isolation model for only three of six species. The Migration model was the best not only for the bird Ph. rufifrons but also for the lizard T. semitaeniatus. Similarly to ABC, SML was unable to clearly classify Po. acutirostris, supporting both the Isolation and Migration models with similar probabilities, but strongly supported Isolation model for G. agilis. The overall accuracy of model classification was high (Table 5).

Table 5. Posterior probabilities for each model and study species from supervised machine learning (SML).
For most species, the divergence time estimated with SML was close to or older than 450 kya, except for D. muelleri and P. rufifrons (Figure 3 and Supplementary Table 6), but coherent with the pattern found using ABC. Similarly, estimated migration followed the same trend as ABC results, except for G. agilis, with less than one migrant individual per generation. Accuracy of parameter estimates with SML performed better than ABC, with a high correlation coefficient between estimated and true parameter (Supplementary Table 7 and Supplementary Figure 5).
Discussion
In this study we found strong multi-taxon support for the São Francisco River Hypothesis (SFRH), which posits that the river acts as a vicariant barrier in the Caatinga, a seasonally dry tropical forest in South America. The SFR acts as a barrier driving genetic structure for not only reptiles, for which the SFRH was first proposed (Rodrigues, 1986), but also for other vertebrate groups such as mammals, amphibians, and birds, contradicting our initial hypothesis that it would not be a barrier for a bird species. Some of the previous studies from which our data were obtained suggested mixed or no support for the SFRH (Faria et al., 2013; Oliveira et al., 2015; Corbett et al., 2020), and were contradicted by our results. For instance, Corbett et al. (2020) rejected the SFR as a barrier for the bird Phacellodomus rufifrons due to evidence of gene flow and individuals from the same population occurring on both sides of the river. Different from previous studies, our analytical approach models incomplete lineage sorting and migration, accommodating these two possible sources of discordance in the hypothesis testing (e.g., Lanna et al., 2020; Thomé et al., 2021b). Our results may also contradict previous results due to the scale of the study. Oliveira et al. (2018a) found that populations of the frog D. muelleri are primarily structured by the Central Brazilian Plateau in their broad range across the South American Dry Diagonal, and did not detect any signal from the SFR in the Caatinga, likely because of that study’s large geographic scale. By focusing only on D. muelleri populations in the Caatinga, we support the SFRH as a soft barrier for the species despite high support for the Isolation model; the recent estimated divergence between populations (ABC: ∼348 kya; SML: ∼153 kya) is likely due to migration across the river (Figure 3), increasing genealogical discordance, and pushing divergence time estimates closer to the present when migration is not a parameter of the model.
We found mixed support for divergence times during the late Pleistocene, and different divergence times from different methodologies. Because SML usually performs better than ABC in model-based analysis (Gehara et al., 2020), hereafter we will base our discussion on parameter estimates from that method. The Caatinga potentially passed through several droughts associated with Pleistocene glaciation cycles, possibly decreasing the river’s water level and its corresponding strength as a barrier (Barreto, 1996; Nascimento et al., 2013; Bruschi et al., 2019). This may explain the apparent asynchrony in divergence times; among the six species, we recovered divergence times of < 450 kya (D. muelleri, Ph. rufifrons), ∼450 kya (A. ocellifera, and T. semitaeniatus), and ∼1 Ma (G. agilis and Po. acutirostris). These divergence times are nonetheless notably consistent when compared to the wide range of SFR-related divergence times compiled in the literature, which range from late Miocene (∼11–5 Ma) (Werneck et al., 2012) to early Pleistocene (∼2 Ma) (Nascimento et al., 2011), to late Pleistocene (<300 kya) (Fegies et al., 2021). This incongruence is possibly due to not considering the possibility of both ILS and sporadic migration in their analysis, which can influence gene tree discordance as shown in our in silico experiment, affecting divergence time estimates. However, the most glaring problem with inferring the SFR as a causal mechanism for divergence in the Caatinga is the dearth of information on the river’s geological history, much of it in obscure gray literature (e.g., Ab’Saber, 1969; Tricart, 1974; Mabesoone, 1994; Valadão, 1998). A geological narrative compiled from these sources is contradictory and difficult to reconcile [though for good attempts at synthesis, with some extrapolation, see Werneck et al. (2015) and Thomé et al. (2021b)]. Geological studies have inferred the SFR’s paleocourse change as having occurred in time periods as disparate as the Eocene (Karner and Driscoll, 1999) and the very late Pleistocene [∼12 kya; Tricart (1974)]; as such, authors of phylogeographic studies can indicate the geological study that suits their taxon’s divergence time best to support their narrative of SFR-mediated divergence. This has prevented a coherent phylogeographic narrative from developing in the Caatinga phylogeography literature. On the other hand, the relative consistency of our inferred divergence dates in a variety of vertebrate taxa, with SFR-mediated divergence occurring within the last million years, represents a first step toward this goal, agreeing with our simulation experiment’s demonstration that divergence times ∼400 kya are not enough for populations to be completely monophyletic unless population sizes are small.
It is important to emphasize that migration rates may be lower than estimated here. According to our simulation experiment and the literature (Qu et al., 2012), certain values of migration and τ can generate similar proportions of concordant trees (e.g., Nm = 0.025 and τ = 1 yield 70% and 75% of gene trees concordant, respectively). Our Migration model does not account for the effect of ILS since it lacks a divergence time parameter; migration accounts for all gene tree discordance. Nevertheless, even when assuming an island model as the Migration model does, migration rate estimates are still relatively low and do not indicate free movement of individuals across the river—population identity can be maintained with a “fuzzy” genetic break or a hybrid zone (e.g., Burbrink et al., 2021). Besides the models’ limitations, geological events could also have influenced migration rates. After the SFR’s initial elbowing eastward in the Pleistocene, its mouth, emptying into the South Atlantic, may have shifted northward to its present-day location between Sergipe and Alagoas states (Figure 1A; King, 1957; Tricart, 1974); it is possible that the gene flow recovered here may be an artifactual result of the southern group capturing northern individuals after this paleocourse change (Werneck et al., 2015; Thomé et al., 2021b). The timing and occurrence of this hypothesized event is, of course, uncertain. Despite the particularities described above, our study supports the SFRH and highlights that not only the riverine hypothesis, but any barrier hypothesis, should not be rejected based solely on the evidence of paraphyletic populations.
The strength of the SFR’s vicariant effect varies among species, with dispersal capability or vagility being an important factor. Body size is often used as a proxy of dispersal capability, with small bodied-animals presenting stronger responses to vicariant barriers (Wollenberg et al., 2011; Arteaga et al., 2016). Species-specific traits other than body size, such as habitat use, may also affect dispersal (Zamudio et al., 2016; Reid et al., 2019; Kuhn et al., 2021). For example, phylogenetic studies indicate a strong vicariant effect of the SFR on several species of limbless (or reduced-limb) fossorial squamate (Rodrigues, 1996; Rodrigues and Juncá, 2002; Siedchlag et al., 2010; Almeida et al., 2020), which likely have limited river-crossing abilities due to their underground lifestyles. Three of the species analyzed here, though not limbless, are squamates, and show divergence times matching the hypothesis of a hard barrier. Together with the mammal species, these lizards have the oldest divergence times among the six species. Not surprisingly, the bird and the amphibian species have posteriors that span into more recent times. The complex life history of anurans, with aquatic larvae, and the aerial dispersal abilities of birds, could allow sporadic migration, accounting for the recovered patterns (Figure 3). The bird Ph. rufifrons is able to cross the SFR and sustain some degree of gene flow (Corbett et al., 2020), but its small body size and ground-foraging behavior (Skutch, 1969), traits associated with low dispersal capability, may still play a role in limiting gene flow. The frog D. muelleri is an explosive breeder, reproducing only after heavy rainfall (Fabrezi et al., 2012), which may wash tadpoles from one margin to the other. The variation in the SFR’s vicariant strength could also have arisen from the sequences used herein not being collected for the primary purpose of testing the SFRH, or by the low number of sampled loci. For some species, we do not have sequences covering their entire distributions or from localities near the river (Figure 1). Scattered sampling may contain more noise or miss important genetic variation, generating wide or biased posterior densities. More refined sampling and spatially explicit analyses have the potential to model, together with a physical barrier and landscape features associated to the region (e.g., campos rupestres, sand dunes), other important processes such as the effects of paleoclimate and isolation-by-distance, testing the SFRH as a soft or hard barrier with more precision.
We used a model-based approach to test the São Francisco River Hypothesis, finding support for the river acting as both a hard and soft barrier for six Caatinga vertebrate species. It is likely that the SFR’s paleocourse changes caused at least partial divergences in these taxa within the past million years. Genomic analyses with additional taxa and more complete spatial sampling on both sides of the river along its entire length may clarify this matter. The cause of the limited asynchrony in divergence times can only be properly examined after a more coherent picture of the SFR’s geological history is achieved. Regardless of divergence time, the migration across the river is low, supporting the hypothesized importance of the river as a phylogeographic and biogeographic feature driving diversification in the Caatinga.
Data availability statement
The original contributions presented in this study are included in the article/Supplementary material, further inquiries can be directed to the corresponding author.
Ethics statement
Ethical review and approval was not required for the animal study because the study was carried using DNA sequences available on GenBank, and we did not collect any animal or tissue to obtain the data used here.
Author contributions
MG and FC conceived the study. WG identified studies with data meeting our criteria and produced animal artwork. FC downloaded and prepared the data. FC and WG analyzed the data. MG performed the in silico experiment. All authors aided in interpreting the results, worked on the manuscript, and approved the submitted version.
Acknowledgments
We thank Evandro Marsola Moraes for helping set parameters to run ipyrad. WG was grateful for funding from a Rutgers Presidential Fellowship and additionally thanks his gecko Triceratops for moral support and a winning smile. MG thanks his acoustic guitar for being supportive during the writing of this manuscript.
Conflict of interest
The authors declare that the research was conducted in the absence of any commercial or financial relationships that could be construed as a potential conflict of interest.
The handling editor declared a past co-authorship with one of the authors MG.
Publisher’s note
All claims expressed in this article are solely those of the authors and do not necessarily represent those of their affiliated organizations, or those of the publisher, the editors and the reviewers. Any product that may be evaluated in this article, or claim that may be made by its manufacturer, is not guaranteed or endorsed by the publisher.
Supplementary material
The Supplementary Material for this article can be found online at: https://www.frontiersin.org/articles/10.3389/fevo.2022.983134/full#supplementary-material
Footnotes
- ^ Available at: github.com/gehara/PipeMaster.
References
Ab’Saber, A. N. (1969). Participação das superfícies aplainadas nas paisagens do Nordeste Brasileiro. Geomorfologia 19, 1–38.
Allio, R., Donega, S., Galtier, N., and Nabholz, B. (2017). Large variation in the ratio of mitochondrial to nuclear mutation rate across animals: Implications for genetic diversity and the use of mitochondrial DNA as a molecular marker. Mol. Biol. Evol. 34, 2762–2772. doi: 10.1093/molbev/msx197
Almeida, J. P. F. A., Thomé, M. T. C., Sturaro, M. J., Pereira, R. J., and Mott, T. (2020). The relative role of glacial refugia and longstanding barriers in the diversification of a fossorial squamate. Syst. Biodivers. 18, 447–463. doi: 10.1080/14772000.2020.1783716
Andrade-Souza, V., Silva, J. G., and Hamada, N. (2017). Phylogeography and population diversity of Simulium hirtipupa Lutz (Diptera: Simuliidae) based on mitochondrial COI sequences. PLoS One 12:e0190091. doi: 10.1371/journal.pone.0190091
Arteaga, A., Pyron, R. A., Peñafiel, N., Romero-Barreto, P., Culebras, J., Bustamante, L., et al. (2016). Comparative phylogeography reveals cryptic diversity and repeated patterns of cladogenesis for amphibians and reptiles in northwestern Ecuador. PLoS One 11:e0151746. doi: 10.1371/journal.pone.0151746
Balbino, E., Caetano, B., and Almeida, C. (2018). Phylogeographic structure of Spondias tuberosa Arruda Câmara (Anacardiaceae): Seasonally dry tropical forest as a large and continuous refuge. Tree Genet. Genomes 14:67. doi: 10.1007/s11295-018-1279-4
Barreto, A. M. F. (1996). Interpretacão paleoambiental do sistema de dunas fixadas do médio Rio São Francisco, Bahia. Ph.D. thesis. São Paulo, SP: Universidade de São Paulo.
Batalha-Filho, H., Maldonado-Coelho, M., and Miyaki, C. Y. (2019). Historical climate changes and hybridization shaped the evolution of Atlantic Forest spinetails (Aves: Furnariidae). Heredity 123, 675–693. doi: 10.1038/s41437-019-0234-y
Bruschi, D. P., Peres, E. A., Lourenço, L. B., Bartoleti, L. F. M., Sobral-Souza, T., and Recco-Pimentel, S. M. (2019). Signature of the paleo-course changes in the São Francisco River as source of genetic structure in Neotropical Pithecopus nordestinus (Phyllomedusinae, Anura) treefrog. Front. Genet. 10:728. doi: 10.3389/fgene.2019.00728
Burbrink, F. T., Gehara, M., McKelvy, A. D., and Myers, E. A. (2021). Resolving spatial complexities of hybridization in the context of the gray zone of speciation in North American ratsnakes (Pantherophis obsoletus complex). Evolution 75, 260–277. doi: 10.1111/evo.14141
Capparella, A. P. (1988). Genetic variation in Neotropical birds: Implications for the speciation process. Acta Congressus Int. Ornithol. 19, 1658–1664.
Chen, W. (2011). Overlapping codon model, phylogenetic clustering, and alternative partial expectation conditional maximization algorithm. Ph.D. thesis. Ames, IA: Iowa State University.
Chollet, F. (2015). Keras. Available online at: https://github.com/fchollet/keras (accessed May 23, 2022).
Corbett, E. C., Bravo, G. A., Schunck, F., Naka, L. N., Silveira, L. F., and Edwards, S. V. (2020). Evidence for the Pleistocene Arc hypothesis from genome-wide SNPs in a Neotropical dry forest specialist, the Rufous-fronted Thornbird (Furnariidae: Phacellodomus rufifrons). Mol. Ecol. 29, 4457–4472. doi: 10.1111/mec.15640
Costa, W. J. E. M., Amorim, P. F., and Mattos, J. L. O. (2018). Synchronic historical patterns of species diversification in seasonal aplocheiloid killifishes of the semi-arid Brazilian Caatinga. PLoS One 13:e0193021. doi: 10.1371/journal.pone.0193021
Csilléry, K., François, O., and Blum, M. G. B. (2012). abc: An R package for approximate Bayesian computation (ABC). Methods Ecol. Evol. 3, 475–479. doi: 10.1111/j.2041-210X.2011.00179.x
Degnam, J. H., and Rosenberg, N. A. (2006). Discordance of species trees with their most likely gene trees. PLoS Genet. 2:e68. doi: 10.1371/journal.pgen.0020068
Eaton, D. A. R., and Overcast, I. (2020). ipyrad: Interactive assembly and analysis of RADseq datasets. Bioinformatics 36, 2592–2594. doi: 10.1093/bioinformatics/btz966
Edgar, R. C. (2004). MUSCLE: A multiple sequence alignment method with reduced time and space complexity. BMC Bioinformatics 5:113. doi: 10.1186/1471-2105-5-113
Fabrezi, M., Quinzio, S., Goldberg, J., and de Sá, R. O. (2012). The development of Dermatonotus muelleri (Anura: Microhylidae: Gastrophryninae). J. Herpetol. 46, 363–380. doi: 10.1670/11-194
Faria, M. B., Nascimento, F. F., De Oliveira, J. A., and Bonvicino, C. R. (2013). Biogeographic determinants of genetic diversification in the mouse opossum Gracilinanus agilis (Didelphimorphia: Didelphidae). J. Hered. 104, 613–626. doi: 10.1093/jhered/est039
Fegies, A. C., Carmignotto, A. P., Perez, M. F., Guilardi, M. D., and Lessinger, A. C. (2021). Molecular phylogeny of Cryptonanus (Didelphidae: Thylamyini): Evidence for a recent and complex diversification in South American open biomes. Mol. Phylogenet. Evol. 162:107213. doi: 10.1016/j.ympev.2021.107213
Fonseca, E. M., Garda, A. G., Oliveira, E. F., Camurufi, F., Magalhães, F. M., Lanna, F. M., et al. (2021). The riverine thruway hypothesis: Rivers as a key mediator of gene flow for the aquatic paradoxical frog Pseudis tocantins (Anura, Hylidae). Landsc. Ecol. 36, 3049–3060. doi: 10.1007/s10980-021-01257-z
Fonseca, E. M., Gehara, M., Werneck, F. P., Lanna, F. M., Colli, G. R., Sites, J. W., et al. (2018). Diversification with gene flow and niche divergence in a lizard species along the South American “diagonal of open formations”. J. Biogeogr. 45, 1688–1700. doi: 10.1111/jbi.13356
Fraga, R., and Carvalho, V. T. (2021). Testing the Wallace’s riverine barrier hypothesis based on frog and Squamata reptile assemblages from a tributary of the lower Amazon River. Stud. Neotrop. Fauna Environ. 1–10. doi: 10.1080/01650521.2020.1870838
Gehara, M., Garda, A. A., Werneck, F. P., Oliveira, E. F., da Fonseca, E. M., Camurugi, F., et al. (2017). Estimating synchronous demographic changes across populations using hABC and its application for a herpetological community from northeastern Brazil. Mol. Ecol. 26, 4756–4771. doi: 10.1111/mec.14239
Gehara, M., Mazzochinni, G. G., and Burbrink, F. (2020). PipeMaster: Inferring population divergence and demographic history with approximate Bayesian computation and supervised machine-learning in R. bioRxiv [Preprint]. doi: 10.1101/2020.12.04.410670
Grabert, H. (1968). Postmesozoische entwässerung und oszillation am ostrande des Brasilianischen schildes. Geol. Rundsch. 58, 166–190. doi: 10.1007/BF01820602
Hudson, R. R. (2002). Generating samples under a Wright-Fisher neutral model of genetic variation. Bioinformatics 18, 337–338. doi: 10.1093/bioinformatics/18.2.337
Karner, G. D., and Driscoll, N. W. (1999). Tectonic and stratigraphic development of the West African and eastern Brazilian margins: Insights from quantitative basin modelling. Geol. Soc. Lond. Spec. Publ. 153, 11–40. doi: 10.1144/GSL.SP.1999.153.01.02
Kearse, M., Moir, R., Wilson, A., Stones-Havas, S., Cheung, M., Sturrock, S., et al. (2012). Geneious basic: An integrated and extendable desktop software platform for the organization and analysis of sequence data. Bioinformatics 28, 1647–1649. doi: 10.1093/bioinformatics/bts199
King, L. C. (1957). A geomorfologia do brasil oriental. Rio de Janeiro: Instituto Brasileiro de Geografia e Estatística, Conselho Nacional de Geografia.
Kingman, J. F. C. (1982). The coalescent. Stoch. Process. Their Appl. 13, 235–248. doi: 10.1016/0304-4149(82)90011-4
Kuhn, A., Gehara, M., Andrianarimalala, M. S. M., Rabibisoa, N., Randriamahatantsoa, B., Overcast, I., et al. (2021). Drivers of unique and asynchronous population dynamics in Malagasy herpetofauna. J. Biogeogr. 49, 600–616. doi: 10.1111/jbi.14315
Lanna, F. M., Gehara, M., Werneck, F. P., Fonseca, E. M., Colli, G. R., Sites, J. W., et al. (2020). Dwarf geckos and giant rivers: The role of the São Francisco River in the evolution of Lygodactylus klugei (Squamata: Gekkonidae) in the semi-arid Caatinga of north-eastern Brazil. Biol. J. Linn. Soc. 129, 88–98. doi: 10.1093/biolinnean/blz170
Lawson, L. P. (2013). Diversification in a biodiversity hot spot: Landscape correlates of phylogeographic patterns in the African spotted reed frog. Mol. Ecol. 22, 1947–1960. doi: 10.1111/mec.12229
Librado, P., and Rozas, J. (2009). DnaSP v5: A software for comprehensive analysis of DNA polymorphism data. Bioinformatics 25, 1451–1452. doi: 10.1093/bioinformatics/btp187
Mabesoone, J. M. (1994). Sedimentary basins of Northeast Brazil. Recife: Federal University of Pernambuco.
Machado, T., Silva, V. X., and Silva, M. J. J. (2014). Phylogenetic relationships within Bothrops neuwiedi group (Serpentes, Squamata): Geographically highly-structured lineages, evidence of introgressive hybridization and Neogene/Quaternary diversification. Mol. Phylogenet. Evol. 71, 1–14. doi: 10.1016/j.ympev.2013.10.003
Menezes, M. O. T., Zappi, D. C., Moraes, E. M., Franco, F. F., Taylor, N. P., Costa, I. R., et al. (2016). Pleistocene radiation of coastal species of Pilosocereus (Cactaceae) in eastern Brazil. J. Arid Environ. 135, 22–32. doi: 10.1016/j.jaridenv.2016.08.006
Miranda, E. A., Batalha-Filho, H., Congrains, C., Carvalho, A. F., Ferreira, K. M., and Del Lama, M. A. (2016). Phylogeography of Partamona rustica (Hymenoptera, Apidae), an endemic stingless bee from the Neotropical dry forest diagonal. PLoS One 11:e0164441. doi: 10.1371/journal.pone.0164441
Moraes, L. J. C. L., Pavan, D., Barros, M. C., and Ribas, C. C. (2016). The combined influence of riverine barriers and flooding gradients on biogeographical patterns for amphibians and squamates in south-eastern Amazonia. J. Biogeogr. 43, 2113–2124. doi: 10.1111/jbi.12756
Moraes, L. J. C. L., Ribas, C. C., Pavan, D., and Werneck, F. P. (2020). “Biotic and landscape evolution in an Amazonian contact zone: Insights from the herpetofauna of the Tapajos River basin,” in Neotropical diversification: Patterns and processes, eds V. Rull and A. C. Carnaval (Cham: Springer), 683–712.
Naka, L. N., and Brumfield, R. T. (2018). The dual role of Amazonian rivers in the generation and maintenance of avian diversity. Sci. Adv. 4:eaar8575. doi: 10.1126/sciadv.aar8575
Naka, L. N., and Pil, M. W. (2020). Moving beyond the riverine barrier vicariant paradigm. Mol. Ecol. 29, 129–2132. doi: 10.1111/mec.15465
Nascimento, F. F., Lazar, A., Menezes, A. N., Durans, A. M., Moreira, J. C., Salazar-Bravo, J., et al. (2013). The role of historical barriers in the diversification processes in open vegetation formations during the Miocene/Pliocene using an ancient rodent lineage as a model. PLoS One 8:e61924. doi: 10.1371/journal.pone.0061924
Nascimento, F. F., Pereira, L. G., Geise, L., Bezerra, A. M. R., D’Andrea, P. S., and Bonvicino, C. R. (2011). Colonization process of the Brazilian common vesper mouse, Calomys expulsus (Cricetidae, Sigmodontinae): A biogeographic hypothesis. J. Hered. 102, 260–268. doi: 10.1093/jhered/esr012
Oliveira, E. F., Gehara, M., São-Pedro, V. A., Chen, X., Myers, E. A., Burbrink, F. T., et al. (2015). Speciation with gene flow in whiptail lizards from a Neotropical xeric biome. Mol. Ecol. 24, 5957–5975. doi: 10.1111/mec.13433
Oliveira, E. F., Gehara, M., São-Pedro, V. A., Costa, G. C., Burbrink, F. T., Colli, G. R., et al. (2018a). Phylogeography of Muller’s termite frog suggests the vicariant role of the Central Brazilian Plateau. J. Biogeogr. 45, 2508–2519. doi: 10.1111/jbi.13427
Oliveira, E. F., Martinez, P. A., São-Pedro, V. A., Gehara, M., Burbrink, F. T., Mesquita, D. O., et al. (2018b). Climatic suitability, isolation by distance and river resistance explain genetic variation in a Brazilian whiptail lizard. Heredity 120, 251–265. doi: 10.1038/s41437-017-0017-2
Paradis, E., and Schliep, K. (2019). ape 5.0: An environment for modern phylogenetics and evolutionary analyses in R. Bioinformatics 35, 526–528. doi: 10.1093/bioinformatics/bty633
Passoni, J. C., Benozzati, M. L., and Rodrigues, M. T. (2008). Phylogeny, species limits, and biogeography of the Brazilian lizards of the genus Eurolophosaurus (Squamata: Tropiduridae) as inferred from mitochondrial DNA sequences. Mol. Phylogenet. Evol. 46, 403–414. doi: 10.1016/j.ympev.2007.10.022
Patton, J. L., Silva, M. N. F., and Malcolm, J. R. (1994). Gene genealogy and differentiation among arboreal spiny rats (Rodentia: Echimyidae) of the Amazon basin: A test of the riverine barrier hypothesis. Evolution 48, 1314–1323. doi: 10.1111/j.1558-5646.1994.tb05315.x
Pavlidis, P., Laurent, S., and Stephan, W. (2010). msABC: A modification of Hudson’s ms to facilitate multi-locus ABC analysis. Mol. Ecol. Resour. 10, 723–727. doi: 10.1111/j.1755-0998.2010.02832.x
Pirani, R. M., Werneck, F. P., Thomaz, A. T., Kenney, M. L., Sturaro, M. J., Avila-Pires, T. C. S., et al. (2019). Testing main Amazonian rivers as barriers across time and space within widespread taxa. J. Biogeogr. 46, 2444–2456. doi: 10.1111/jbi.13676
Potter, P. E. (1997). The Mesozoic and Cenozoic paleodrainage of South America: A natural history. J. S. Am. Earth Sci. 10, 331–344. doi: 10.1016/S0895-9811(97)00031-X
Puida, D. B. C., and Paglia, A. P. (2015). Primary productivity and the demography of Gracilinanus agilis, a small semelparous marsupial. J. Mammal. 96, 221–229. doi: 10.1093/jmammal/gyu030
Qu, Y., Zhang, R., Quan, Q., Song, G., Hsien, S., and Lei, F. (2012). Incomplete lineage sorting or secondary admixture: Disentangling historical divergence from recent gene flow in the Vinous-throated parrotbill (Paradoxornis webbianus). Mol. Ecol. 21, 6117–6133. doi: 10.1111/mec.12080
Reid, B. N., Naro-Maciel, E., Hahn, A. T., FitzSimmons, N. N., and Gehara, M. (2019). Geography best explains global patterns of genetic diversity and postglacial co-expansion in marine turtles. Mol. Ecol. 28, 3358–3370. doi: 10.1111/mec.15165
Ribeiro, R. F., Dominguez, J. M. L., Santos, A. D., and Rangel, A. G. A. N. (2021). Continuous canyon-river connection on a passive margin: The case of São Francisco Canyon (eastern Brazil). Geomorphology 375:107549. doi: 10.1016/j.geomorph.2020.107549
Rodrigues, M. T. (1986). Um novo Tropidurus com crista dorsal do Brasil com comentários sobre suas relações, distribuição e origem (Sauria, Iguanidae). Papéis Avulsos Zool. 36, 171–179.
Rodrigues, M. T. (1996). Lizards, snakes, and amphisbaenians from the Quaternary sand dunes of the middle Rio São Francisco, Bahia, Brazil. J. Herpetol. 30, 513–523. doi: 10.2307/1565694
Rodrigues, M. T., and Juncá, F. A. (2002). Herpetofauna of the Quaternary sand dunes of the middle Rio São Francisco: Bahia: Brazil. VII.: Typhlops amoipira sp. nov., a possible relative of Typhlops yonenagae (Serpentes, Typhlopidae). Papéis Avulsos Zool. 42, 325–333. doi: 10.1590/S0031-10492002001300001
Salgado, E. V., Andrade, E. M., Hevia, J. N., Nunes, E. P., and Rodrigues, M. M. A. (2015). Rainfall patterns and the contribution of litter in the Caatinga dry tropical forest. Rev. Ciênc. Agron. 46, 299–309. doi: 10.5935/1806-6690.20150009
Santorelli, S. Jr., Magnusson, W. E., and Deus, C. P. (2018). Most species are not limited by an Amazonian river postulated to be a border between endemism areas. Sci. Rep. 8:2294. doi: 10.1038/s41598-018-20596-7
Siedchlag, A. C., Benozzati, M. L., Passoni, J. C., and Rodrigues, M. T. (2010). Genetic structure, phylogeny, and biogeography of Brazilian eyelid-less lizards of genera Calyptommatus and Nothobachia (Squamata, Gymnophthalmidae) as inferred from mitochondrial DNA sequences. Mol. Phylogenet. Evol. 56, 622–630. doi: 10.1016/j.ympev.2010.04.027
Skutch, A. F. (1969). A study of the Rufous-fronted thornbird and associated birds. Wilson Bull. 81, 5–43.
Stephens, M., and Donnelly, P. (2003). A comparison of Bayesian methods for haplotype reconstruction from population genotype data. Am. J. Hum. Genet. 73, 1162–1169. doi: 10.1086/379378
Stephens, M., Smith, N., and Donnelly, P. (2001). A new statistical method for haplotype reconstruction from population data. Am. J. Hum. Genet. 68, 978–989. doi: 10.1086/319501
Thomé, M. T. C., Carstens, B. C., Rodrigues, M. T., Galetti, P. M., Alexandrino, J., and Haddad, C. F. B. (2021a). A role of asynchrony of seasons in explaining genetic differentiation in a Neotropical toad. Heredity 127, 363–372. doi: 10.1038/s41437-021-00460-7
Thomé, M. T. C., Carstens, B. C., Rodrigues, M. T., Alexandrino, J., and Haddad, C. F. B. (2021b). Genomic data from the Brazilian sibilator frog reveal contrasting Pleistocene dynamics and regionalism in two South American dry biomes. J. Biogeogr. 48, 1112–1123. doi: 10.1111/jbi.14064
Tricart, J. (1974). Existence de périodes sèches au Quaternaire en Amazonie et dans les régions voisines. Rev. Géomorphol. Dyn. 23, 145–158.
Valadão, R. C. (1998). Evolução de longo-termo do relevo do Brasil Oriental (desnudação, superfiìcies de aplanamento e soerguimentos crustais). Ph. D. Dissertation. Salvador: Universidade Federal Da Bahia, Instituto de Geociencias.
Wallace, A. R. (1854). On the monkeys of the Amazon. J. Nat. Hist. 14, 451–454. doi: 10.1080/037454809494374
Werneck, F. P., Gamble, T., Colli, G. R., Rodrigues, M. T., and Sites, J. K. W. Jr. (2012). Deep diversification and long-term persistence in the South American ‘dry diagonal’: Integrating continent-wide phylogeography and distribution modeling of geckos. Evolution 66, 3014–3034. doi: 10.1111/j.1558-5646.2012.01682.x
Werneck, F. P., Leite, R. N., Geurgas, S. R., and Rodrigues, M. T. (2015). Biogeographic history and cryptic diversity of saxicolous Tropiduridae lizards endemic to the semiarid Caatinga. BMC Evol. Biol. 15:94. doi: 10.1186/s12862-015-0368-3
Wollenberg, K. C., Vieites, D. R., Glaw, F., and Vences, M. (2011). Speciation in little: The role of range and body size in the diversification of Malagasy mantellid frogs. BMC Evol. Biol. 11:217. doi: 10.1186/1471-2148-11-217
Wright, S. (1931). Evolution in Mendelian populations. Genetics 16, 97–159. doi: 10.1093/genetics/16.2.97
Keywords: approximate Bayesian computation, birds, Caatinga, reptiles, amphibians, mammals, riverine barrier hypothesis, supervised machine learning
Citation: Coelho FEA, Guillory WX and Gehara M (2022) Coalescent simulations indicate that the São Francisco River is a biogeographic barrier for six vertebrates in a seasonally dry South American forest. Front. Ecol. Evol. 10:983134. doi: 10.3389/fevo.2022.983134
Received: 30 June 2022; Accepted: 22 August 2022;
Published: 23 September 2022.
Edited by:
Isabel Bonatelli, Federal University of São Paulo, BrazilReviewed by:
Bárbara Simões Santos Leal, State University of Campinas, BrazilPedro Amorim, Federal University of Rio de Janeiro, Brazil
José Maria Landim Dominguez, Federal University of Bahia, Brazil
Copyright © 2022 Coelho, Guillory and Gehara. This is an open-access article distributed under the terms of the Creative Commons Attribution License (CC BY). The use, distribution or reproduction in other forums is permitted, provided the original author(s) and the copyright owner(s) are credited and that the original publication in this journal is cited, in accordance with accepted academic practice. No use, distribution or reproduction is permitted which does not comply with these terms.
*Correspondence: Felipe Eduardo Alves Coelho, fcoelho220@gmail.com