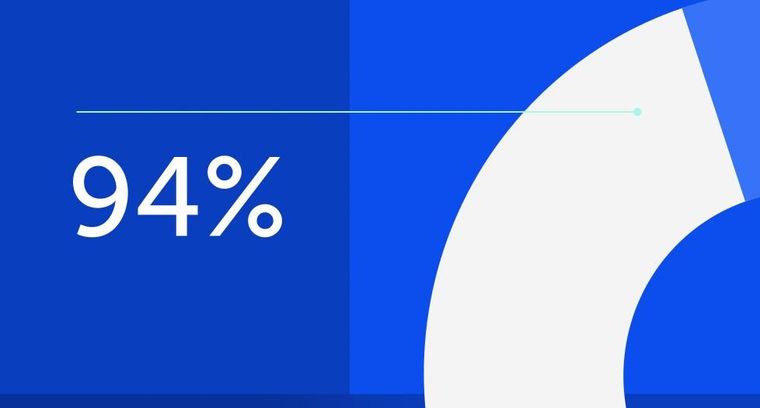
94% of researchers rate our articles as excellent or good
Learn more about the work of our research integrity team to safeguard the quality of each article we publish.
Find out more
BRIEF RESEARCH REPORT article
Front. Ecol. Evol., 28 October 2022
Sec. Behavioral and Evolutionary Ecology
Volume 10 - 2022 | https://doi.org/10.3389/fevo.2022.975687
Heritable genetic variation is a prerequisite for adaptive evolution; however, our knowledge about the heritability of plastic traits, such as behaviors, is scarce, especially in wild populations. In this study, we investigated the heritability of song traits in the collared flycatcher (Ficedula albicollis), a small oscine passerine with complex songs involved in sexual selection. We recorded the songs of 81 males in a natural population and obtained various measures describing the frequency, temporal organization, and complexity of each song. As we had multiple songs from each individual, we were able to statistically account for the first time for the effect of within-individual variance on the heritability of song. Heritability was calculated from the variance estimates of animal models relying on a genetic similarity matrix based on Single Nucleotide Polymorphism screening. Overall, we found small additive genetic variance and heritability values in all song traits, highlighting the role of environmental factors in shaping bird song.
Behaviors, such as social, explorative, or risky behaviors, frequently show consistent among-individual differences within and across populations (Verbeek et al., 1994; Sih et al., 2004a; Réale et al., 2007; Osborn and Briffa, 2017). Under the assumption of heritable variation, these consistent differences are probably caused by natural or sexual selection, as these behaviors are frequently associated with fitness (Réale and Festa-Bianchet, 2003; Dingemanse et al., 2004; Bergeron et al., 2013). However, animal behavior, that allows individuals to respond flexibly to environmental changes, often exhibits within-individual variability. Estimating the heritability in behavioral traits with large within-individual variance is challenging, but it is necessary to understand the evolution of behavior, as only selection on heritable variation results in the adaptive evolution of phenotypic traits (Fisher, 1930).
The underlying genetic structure of behavior and its heritability are widely studied (e.g., explorative, antipredator, or social behavior; Dingemanse et al., 2002; Stirling et al., 2002; Dochtermann et al., 2015). Accordingly, some genes associated with animal behavior have already been identified (Fidler et al., 2007; Garamszegi et al., 2014; Montag and Reuter, 2014) and the heritability of a few behaviors (such as antipredator behavior or exploration) has been estimated (van Oers et al., 2004; Blumstein et al., 2010; Ariyomo et al., 2013). Although a systematic review found that the heritability of behavior in wild populations was on average 0.5 (Postma, 2014), low estimates are frequently reported (Brommer et al., 2008; Araya-Ajoy and Dingemanse, 2017), possibly because behavioral traits are sensitive to environmental conditions (Dosmann and Mateo, 2014; Aplin, 2019; Royauté et al., 2019). Animal models capable of decomposing phenotypic variance into environmental and genetic components are therefore frequently used statistical methods in studies investigating the genetic background of behavior (Dochtermann and Roff, 2010; Wilson et al., 2010; Schielzeth et al., 2011). In plastic traits, such as behavioral traits, it is essential to make repeated measurements to separate among- and within-individual variation appropriately (Sih et al., 2004b; Allegue et al., 2017). Including repeated measurements into an animal model allows the differentiation of additive genetic and permanent environmental effects, the latter being fixed differences between individuals due to environmental and/or non-additive genetic effects (Kruuk, 2004; Wilson et al., 2010). However, it should be noted that the long-term evolutionary potential of a trait could be assessed by calculating evolvability instead of heritability (Visscher et al., 2008; Hansen et al., 2011; Hansen and Pelabon, 2021).
Despite the efforts invested into the research on the heritability of behavior, knowledge about the heritability of bird song is limited. Bird song is a very complex behavior that is assumed to be under sexual selection, playing a major role in mate choice and territory defense (Gil and Gahr, 2002; Marshall et al., 2003; Catchpole and Slater, 2008). Song shows individual consistency, but its repeatability (often considered the upper limit of heritability) is low, especially on longer timescales (Prùchová et al., 2017; Naguib et al., 2019; Osiejuk et al., 2019). Plasticity in the expression of sexual signals may affect signal reliability and the effect of sexual selection on them. Therefore, measuring the heritability of these traits considering within-individual variation is essential for assessing their evolutionary potential. The few studies—a handful in wild populations—on the heritability of song have found low values (Forstmeier et al., 2009; Labra and Lampe, 2018; Mets and Brainard, 2018; Lewis et al., 2021), probably because of the high plasticity of song and because social learning is an essential process in song development (Beecher and Brenowitz, 2005; Trösch et al., 2017; Lewis et al., 2021). The heritability may differ between song traits because song traits that are mostly learned should have lower heritability (although song learning itself can have heritable elements; Wheatcroft and Qvarnström, 2017), while, as morphological traits generally have high heritability (Stirling et al., 2002), traits related to morphology (e.g., song frequency, which is influenced by the morphology of the vocal tract) should have higher heritabilities (Forstmeier et al., 2009).
Our study aimed to investigate the amount of additive genetic variation in a range of song traits in the collared flycatcher (Ficedula albicollis), a passerine with complex song and a well-studied model for sexual selection (Qvarnström, 1997; Garamszegi et al., 2008; Hegyi et al., 2010). We recorded the song of males as part of a long-term field study and calculated various song traits describing spectral and temporal characteristics. Our approach is unique in the sense that we included multiple measurements from the same individuals in the analyses instead of using the mean of the focal song traits, as done by previous studies on song. In this way, we could consider the within-individual variance of the traits, which is considerable in our study species (Zsebõk et al., 2017). We estimated relatedness among individuals based on Single Nucleotide Polymorphisms (SNPs), as a required input for the statistical assessment of additive genetic variance. Based on previous works (Labra and Lampe, 2018; Mets and Brainard, 2018; Lewis et al., 2021), we predicted that additive genetic variance and heritability will be low as the song traits investigated in this study are plastic and probably have learned elements (Eriksen et al., 2009, 2011). However, we expected higher heritability estimates for morphology-related song traits (e.g., song frequency).
The collared flycatcher is a hole-nesting, long-distance migratory passerine. In the spring, males arrive at the breeding grounds and occupy a small territory around a nest hole where the males sing intensively, especially in the morning hours, typically until pairing (Pärt, 1991; Garamszegi et al., 2004).
The song performance consists of sequences of songs that are 3–5 s long structures composed of syllables, the smallest units of song (Gelter, 1987). Males have a moderately high individual repertoire size of 20–100 syllables estimated from 20 songs (Garamszegi et al., 2006; Zsebõk et al., 2018b). Song traits typically have low-to-moderate repeatability (Zsebõk et al., 2017), and some of them may be important in sexual selection processes, as they are associated with estimates of mating success or the degree of male–male competition (Garamszegi et al., 2004; Hegyi et al., 2010).
Our knowledge about song learning in the collared flycatcher is scarce. The species is probably an open-ended learner, similar to its sister species, the pied flycatcher (Ficedula hypoleuca) (Eriksen et al., 2011). Likewise, males may learn song elements from their fathers (Labra and Lampe, 2018), however, this way of learning is probably limited as males rarely sing after pairing (Garamszegi et al., 2004). Additionally, there is some indication that song learning could have heritable elements in this species (Wheatcroft and Qvarnström, 2017).
The field work was done in an oak-dominated forest in the Pilis-Visegrádi Mountains, Hungary (47°43′N, 19°01′E). The research area belongs to the Duna-Ipoly National Park, and consists of about 800 nest boxes, where the collared flycatcher commonly breeds.
Song recordings were made from 2008 to 2018 during the courtship period of the study species, between 11 April and 7 May. In the morning, the most active singing period of the day (Pärt, 1991; personal observations), we monitored the study area daily for newly arrived, unpaired birds. When a displaying male was located near its occupied nest box, we either (1) presented him a social stimulus (a live male or female decoy) for 5–10 min, and after removing the stimulus, we recorded the song of the focal male or (2) recorded the song without presenting a social stimulus (these procedures are described in detail elsewhere; Garamszegi et al., 2008). The type of stimulus did not affect our results (see Supplementary Table 1).
Song recordings were made using a standard protocol (Garamszegi et al., 2006, 2007, 2012; Zsebõk et al., 2017). Briefly, we used parabolic microphone sets and digital recorders for recording. Recordings were only made in relatively good weather conditions without rain and wind, lasted at least 10 min, and included at least 20 songs. If there was a significant disturbance from other birds during the recording, such as direct contact with other conspecifics, the songs were not used in the analyses.
We captured the recorded males for ringing and morphological measurements within an hour after the song recordings using a spring trap in their nest boxes. Birds without rings were marked with individually numbered rings (Aranea, Poland, standard rings of the Hungarian Bird Ringing Centre). We determined the age of males (1-year-old or older) based on their plumage (Mullarney et al., 1999). Blood samples of a few microliters were obtained from the brachial vein of the birds after morphological measurements and stored in absolute ethanol.
All applicable international, national, and/or institutional guidelines for animal care and use were followed [e.g., the European Directive (EU 2010/63); Fair et al., 2010]. Field protocols were approved by the ethical committee of Eötvös Loránd University (ref. no. TTK/2203/3). Handling of decoy individuals, behavioral assays, capturing, measurements, and blood sampling were conducted as cautiously and efficiently as possible, in a way to minimize the welfare impact on the birds. Permissions for the fieldwork have been provided by the Government Office of Pest County National Inspectorate for Environment and Nature, reference numbers: DINP 2256-3/2002, DINP 1931-2/2003, DINP 2573/2/2004, KTVF/15951/2005, KTVF/22021/2006, KTVF 16360-2/2007, KTVF 30871-1/2008, KTVF 43355-1/2008, KTVF 45116-2/2011, KTVF 21664-3/2011, KTVF 12677-4/2012, KTVF 10949-8/2013, KTF 11978-5/2015, PEI/001/1053-6/2015, PE/EA/101-8/2018, PE-06/KTF/8550-4/2018, and PE-06/KTF/8550-5/2018.
We manually cut out the songs from the recordings using Adobe Audition 3.0 (Adobe Systems) software, choosing songs with clearly discernible syllables on the spectrograms. We used the Ficedula Toolbox (Zsebõk et al., 2018a) to extract two spectrographic features from segmented syllables: duration and mean frequency of the syllables (see details elsewhere; Zsebõk et al., 2017; Jablonszky et al., 2021). The latter variable was obtained by taking the peak frequency values in each spectrographic time window and calculating their averages at the syllable level (Garamszegi et al., 2012). We clustered the syllables manually into syllable types (see Zsebõk et al., 2018a for further details).
At the level of songs, we measured song length and tempo (the number of syllables within the song divided by song length in seconds, see also Figure 1). Short-term complexity (hereafter complexity) was calculated as the number of different syllable types divided by the total number of syllables in each song. Additionally, we calculated the mean frequency and the frequency bandwidth of each song based on the mean frequencies of the syllables of the song. We used these five song traits in the analyses.
Figure 1. Spectrogram of a collared flycatcher song depicting the song traits used in the analyses. Tempo was calculated by dividing the number of syllables in the song by song length, and complexity was calculated by dividing the number of syllable types (indicated by different letters) by the total number of syllables in the song. Fmax, Fmean, Fmin, maximum, mean, minimum frequency; Fbandwidth, frequency bandwidth.
As all individuals were blood sampled, we performed genetic screening based initially on 503,325 SNPs obtained by DNA genotyping. A genetic similarity matrix based on SNPs is appropriate for calculating additive genetic variance when a large number of SNPs are used (usually > 10,000; Purcell et al., 2007; Bérénos et al., 2014; Lee and Chow, 2014; Widmer et al., 2014).
A paired-end library (2 μg of genomic DNA per sample, digested with PstI) was prepared following the manufacturer’s specifications at CNAG-CRG (National Genome Analyses Centre, Barcelona, Spain) and sequenced on an Illumina HiSeq2000 v4 with 2 × 125 bp reads at a depth of approximately 10×. To evaluate the reliability of the sequencing process and optimize loci assembly, 20 individuals were included as duplicates.
Raw sequences were inspected with FASTQC (Andrews, 2010) for quality control, demultiplexed and Illumina adapters as well as poor-quality sequences were removed. A reference genome for the species (Ellegren et al., 2012) was used during assembly.
SNP calling was performed using bcftools (Danecek et al., 2016) and filtered using PLINK 1.07 (Purcell et al., 2007). We removed loci that were not in Hardy–Weinberg equilibrium, were located on sex chromosomes, and those with a minor allele frequency below 0.05 or a maximum per-SNP missing of 0.1. Individuals considered in further analyses had no more than 5% of missing data. PLINK 1.07 was also used to build a matrix of relatedness based on IBS (Identity by State) segments. The final matrix was based on 188.231 SNPs.
Both song and genetic data were available for 81 individuals between 2008 and 2018 (3,582 songs, except for complexity, for which 2,277 songs were available from 57 birds, and we used 9–248 songs per individual). Only one song recording (the first) per bird was used in the analyses, as the number of repeated recordings (a total of 95 recordings from 81 individuals) was insufficient for including this additional hierarchical level into the models.
We carefully chose five focal song traits (song length, mean frequency, frequency bandwidth, tempo, complexity) that show generally low phenotypic correlation i.e., they could be considered independent and likely have different biological meanings (see Zsebõk et al., 2017), thus minimizing any potential bias.
As a preliminary step, we calculated adjusted within-recording repeatability for the song traits using the rptR package (Stoffel et al., 2017). Adjusted repeatability of the song traits was calculated by dividing their among-individual variance with their total phenotypic variance obtained from mixed models (Lessells and Boag, 1987; Nakagawa and Schielzeth, 2010). The song traits used as response variables were normally distributed. All continuous variables were z-transformed before the analyses. In the models, we considered potentially confounding effects such as the age of the bird, date of measurement, and order of the song in the given recording (Garamszegi et al., 2007; Jablonszky et al., 2021). The order of the song was included to control for time-related changes in the environment during the song recording, such as the effect of the removal of stimulus birds. We did not add further fixed effects to avoid overly complex models and because we made an effort to record songs under standard conditions (see Field procedures). Year and individual identity were included as random factors. Confidence intervals were calculated using parametric bootstrapping.
We calculated the narrow-sense heritability of song traits using the animal model framework, thus decomposing the phenotypic variance into genetic and environmental components (Kruuk, 2004; Wilson et al., 2010). We used the “brms” R package, a package to fit Bayesian regression models (Bürkner, 2017, 2018). The details of model specification and the diagnostics used are presented in Supplementary Text 1. Narrow-sense heritability was calculated as the posterior mean of the ratio of additive genetic variance and the sum of all the variance components, with 95% CIs. In the case of variance components and heritability, we did not rely on CIs when assessing significance, as these could not include 0 due to the prior specification for the models.
We built two models for each song trait (song length, mean frequency, frequency bandwidth, tempo, complexity), one with no fixed effects (minimum models) and one with fixed effects (full models) considered to influence the song traits. The full models contained the age of the birds, date of measurement, and order of the song in the recording as fixed effects. We included two random effects for individual identity: one for estimating between-individual variance due to permanent environmental effects and one (connected to the genetic similarity matrix between the individuals) for estimating additive genetic variance. We also included the year as a random effect in the set of full models (i.e., those containing control effects). We interpreted the two sets of models (minimum and full models) complementary to each other, as control variables are of biological importance and should be therefore considered statistically, but their inclusion into the models may bias the heritability estimates upwards (de Villemereuil et al., 2018).
We compared additive genetic variance estimates from full and minimum models and we also compared estimates for the different song traits. Since the values from minimum and full models were very similar, the comparison of the estimates for the different song traits was carried out only for the estimates of full models. The comparisons were based on CIs and probability of direction (p–, p+) values calculated for the difference of the posterior distributions of the specific estimates (Makowski et al., 2019a). The probability of direction is the proportion of posterior samples of the median sign and can be compared to the frequentist p-value according to the formula ptwo−sided = 2*(1−p−/+) (Makowski et al., 2019a).
The variance of relatedness associated with the genetic similarity matrix was low (0.00034), though comparable to estimates from other wild populations (Bérénos et al., 2014). For this reason and to assess the reliability of our results, we reran the full models again with fully randomized response variables and compared the heritability estimates of these models to that of our original models. Thus, we could confirm whether our results differed from those arising from a situation created by chance. These models were run only 3–4 times due to the high computation time, but the results of all models were nearly identical.
All statistical analyses were performed in the R 3.6.1 statistical environment (R Core Team, 2019). Posterior estimates were compared using functions from the “bayestestR” package (Makowski et al., 2019b).
Between-individual relatedness was rather low in our dataset (mean: 0.036, range: 0–0.378, 25% quantile: 0.025, median: 0.034, 75% quantile: 0.045).
Adjusted within-recording repeatability was moderate but significant for all song traits (see Table 1).
We found low heritability values for the song traits based on genetic similarity (h2 = 0.06–0.10, Figure 2). The variance estimates from the animal models are presented in Table 2. Among the investigated fixed effects, age [estimate: −0.37, 95% credible interval (CI): −0.61, −0.12] and order of the song (−0.11, CI: −0.15, −0.07) had significant relationship with mean frequency. Order also had a significant relationship with tempo (−0.08, CI: −0.12, −0.05) and complexity (0.09, CI: 0.05, 0.13).
Figure 2. Heritability estimates with 95% credible intervals for the song traits. Solid line and solid black points represent estimates based on genetic similarity and empty diamonds and dashed lines represent results from the models using randomized response variables (N of individuals = 81, except for complexity, for which N of individuals = 57).
Table 2. Variance estimates of random effects with 95% credible intervals from the animal models for each song traits.
When comparing the additive genetic variance estimates of different models, we did not find statistically significant differences between the estimates calculated with full or minimum models nor between the estimates for the different song traits (see Supplementary Tables 2, 3).
The models with randomized response variables yielded heritability values of at most 0.01 (CIs: < 0.01, 0.01, or 0.02) for all the song traits, and the differences between these and the heritability estimates from the original models were marginally significant (Figure 2 and Supplementary Table 4). These results indicate that our original estimates, although small, indeed represent heritable variance.
We have comprehensively investigated, taking into account within-individual variation in behavior, the heritability of several song traits in a migratory passerine, the collared flycatcher. All song traits analyzed showed low heritability values. Although heritability estimates were not due to random noise, these results suggest that most phenotypic variation in the studied song traits is not due to genotypic variation.
Our low heritability estimates highlight the critical role of the environment in shaping bird song through multiple mutually non-exclusive mechanisms. First, collared flycatchers probably learn (partly) their songs (Garamszegi et al., 2007; Zsebõk et al., 2020), and thus, the song of the tutors may play a major role in determining the realized song performance of an individual (see: Price, 1979; Chaiken et al., 1993; Beecher and Brenowitz, 2005; Trösch et al., 2017). Although this topic needs to be studied further in our study species, we can suppose that collared flycatchers do not learn songs from their social fathers, because (i) males rarely sing after the hatching of their chicks in this species, and (ii) in birds in general the dispersal of recruits is higher than the parents (Greenwood and Harvey, 1982), thus they are unlikely to breed close to their fathers. Breeding birds might learn from other males in their vicinity, thus creating spatial patterns in song. However, this does not seem to be the case in our population as a previous study found that birds singing in close proximity to each other did not sing similarly (Garamszegi et al., 2012). Additionally, the birds in our dataset generally were spatially or temporally isolated from each other at the time of the recording, thus potential spatial patterns due to learning could not cause serious bias in our analyses. Second, it is possible that the immediate acoustic environment greatly influences the song of the study species, as supported by the finding of low repeatability values (Zsebõk et al., 2017). Environmental factors relevant for song, e.g., presence of conspecifics and predators (Schmidt and Belinsky, 2013; Kipper et al., 2015; Gersick and White, 2018), could change on the scale of our short song recording and these factors may have triggered variability in song. Third, it is known that birds in general may adjust their songs to their immune state (York et al., 2016), breeding stage (Ballentine et al., 2003; Lattin and Ritchison, 2009; Naguib et al., 2016), the current social environment (Kroodsma et al., 1989; Gersick and White, 2018) and predation risk (Schmidt and Belinsky, 2013), among other factors. Thus, the song of many passerine species may function as a signal of the immediate state and environment (e.g., audience composition) of the signaler, as well as displaying some information about individual quality.
The main role of the environment in song expression is supported by the low heritability of learned song found in a wild bird population of the sister species, the pied flycatcher (Labra and Lampe, 2018), and in laboratory studies on zebra (Forstmeier et al., 2009) and Java finches (Lonchura oryzivora, Lewis et al., 2021). Other laboratory studies with Bengalese finches (Lonchura striata domestica) and canaries (Serinus canaria), though finding high heritability for some song traits, also emphasized the role of individual quality and the social environment during learning in the expression of song (Trösch et al., 2017; Mets and Brainard, 2018; Lewis et al., 2021). It should be noted that the differences between the cited results and ours may arise because only a mean value for each individual was used in previous work, which could have led to an underestimation of the residual variance. Furthermore, birds held in the laboratory are exposed to fewer external stimuli that could affect their song, thus the additive genetic component of song traits may increase (but see Lewis et al., 2021). However, low heritability values reported here should be interpreted with caution due to the combination of the modest sample size in terms of individuals and two factors inherent to wild open populations and the nature of the studied traits: the low relatedness between individuals and the moderate repeatability of behavior in general and the song traits in particular (Morrissey et al., 2007; de Villemereuil et al., 2013). It is also worth bearing in mind that even if a song trait has low heritability, it can have high evolvability, i.e., response to selection (Hansen et al., 2011; Houle et al., 2011; Hansen and Pelabon, 2021).
We found similar additive genetic variance estimates for all the song traits analyzed. These findings contrast with previous studies wherein the genetically determined component of bird song depended on the specific song trait considered (Forstmeier et al., 2009; Trösch et al., 2017; Labra and Lampe, 2018). For example in a laboratory study on zebra finches (Taeniopygia guttata), higher and significant heritability values were found for song traits connected to some morphological characteristics, such as mean frequency of calls, compared to the low values reported for other traits, such as repertoire size and song length (Forstmeier et al., 2009). However, we did not find such differences between the heritability of the song traits in our data. It is possible that in the collared flycatcher song traits are not closely related to morphological traits, but this issue should be studied further.
In summary, the heritability of song traits of collared flycatchers was low, indicating the great capacity of birds to adjust their song to their current environment or condition. Low heritability values may also result from the critical role of social learning in the expression of song (Mets and Brainard, 2018; but see: Wheatcroft and Qvarnström, 2017).
The datasets presented in this study can be found in online repositories. The name of the repository and link to the data can be found below: Figshare; https://doi.org/10.6084/m9.figshare.21213896.v1.
The animal study was reviewed and approved by the Ethical Committee of Eötvös Loránd University.
LZG designed the study. All authors except DC and OL participated in the field work. LZG, ML, GN, and SZ collected and analyzed song recordings. DC and OL performed genetic analyses and obtained the genetic relatedness matrix of individuals. MJ carried out the statistical analysis with inputs from LZG and wrote the first draft of the manuscript. All authors contributed to the revisions of the manuscript.
This work was supported by the Hungarian National Research, Development and Innovation Office (Grant Nos. K120249, K124443, and K139992). DC was supported by the Hungarian Academy of Sciences (MTA Premium Postdoctoral Research Program; Grant No. 2019-353).
We thank the Behavioural Ecology Group of ELTE Eötvös Loránd University, especially György Blázi, Nóra Boross, Rita Fõzõ, Dorottya Kiss, Dóra Kötél, Katalin Krenhardt, Eszter Szöllõsi, and Éva Vaskuti for their help in the fieldwork. We are grateful to the Pilis Park Forestry. We thank for the usage of ELKH Cloud (https://science-cloud.hu/) that significantly helped us in achieving the results published in this manuscript. We would also like to thank the three reviewers.
The authors declare that the research was conducted in the absence of any commercial or financial relationships that could be construed as a potential conflict of interest.
All claims expressed in this article are solely those of the authors and do not necessarily represent those of their affiliated organizations, or those of the publisher, the editors and the reviewers. Any product that may be evaluated in this article, or claim that may be made by its manufacturer, is not guaranteed or endorsed by the publisher.
The Supplementary Material for this article can be found online at: https://www.frontiersin.org/articles/10.3389/fevo.2022.975687/full#supplementary-material
Allegue, H., Araya-Ajoy, Y. G., Dingemanse, N. J., Dochtermann, N. A., Garamszegi, L. Z., Nakagawa, S., et al. (2017). Statistical Quantification of Individual Differences (SQuID): An educational and statistical tool for understanding multilevel phenotypic data in linear mixed models. Methods Ecol. Evol. 8, 257–267. doi: 10.1111/2041-210x.12659
Andrews, S. (2010). FastQC: A Quality Control Tool For High Throughput Sequence Data. Available online at: http://www.bioinformatics.babraham.ac.uk/projects/fastqc/ (accessed March 26, 2021).
Aplin, L. M. (2019). Culture and cultural evolution in birds: A review of the evidence. Anim. Behav. 147, 179–187. doi: 10.1016/j.anbehav.2018.05.001
Araya-Ajoy, Y. G., and Dingemanse, N. J. (2017). Repeatability, heritability, and age-dependence of seasonal plasticity in aggressiveness in a wild passerine bird. J. Anim. Ecol. 86, 227–238. doi: 10.1111/1365-2656.12621
Ariyomo, T. O., Carter, M., and Watt, P. J. (2013). Heritability of boldness and aggressiveness in the zebrafish. Behav. Genet. 43, 161–167. doi: 10.1007/s10519-013-9585-y
Ballentine, B., Badyaev, A., and Hill, G. E. (2003). Changes in song complexity correspond to periods of female fertility in blue grosbeaks (Guiraca caerulea). Ethology 109, 55–66. doi: 10.1046/j.1439-0310.2003.00852.x
Beecher, M. D., and Brenowitz, E. A. (2005). Functional aspects of song learning in songbirds. Trends Ecol. Evol. 20, 143–149. doi: 10.1016/j.tree.2005.01.004
Bérénos, C., Ellis, P. A., Pilkington, J. G., and Pemberton, J. M. (2014). Estimating quantitative genetic parameters in wild populations: A comparison of pedigree and genomic approaches. Mol. Ecol. 23, 3434–3451. doi: 10.1111/mec.12827
Bergeron, P., Montiglio, P. O., Réale, D., Humphries, M. M., Gimenez, O., and Garant, D. (2013). Disruptive viability selection on adult exploratory behaviour in eastern chipmunks. J. Evol. Biol. 26, 766–774. doi: 10.1111/jeb.12081
Blumstein, D. T., Lea, A. J., Olson, L. E., and Martin, J. G. A. (2010). Heritability of anti-predatory traits: Vigilance and locomotor performance in marmots. J. Evol. Biol. 23, 879–887. doi: 10.1111/j.1420-9101.2010.01967.x
Brommer, J. E., Rattiste, K., and Wilson, A. J. (2008). Exploring plasticity in the wild: Laying date-temperature reaction norms in the common gull Larus canus. Proc. R. Soc. B Biol. Sci. 275, 687–693. doi: 10.1098/rspb.2007.0951
Bürkner, P.-C. (2017). brms: An R package for Bayesian multilevel models using Stan. J. Stat. Softw. 80, 1–28. doi: 10.18637/jss.v080.i01
Bürkner, P.-C. (2018). Advanced bayesian multilevel modeling with the R package brms. R J. 10, 395–411. doi: 10.32614/RJ-2018-017
Catchpole, C. K., and Slater, P. J. B. (2008). Bird Song: Biological Themes and Variations, 2nd Edn. New York, NY: Cambridge University Press.
Chaiken, M., Böhner, J., and Marler, P. (1993). Song acquisition in European starlings, Sturnus vulgaris: A comparison of the songs of live-tutored, tape-tutored, untutored, and wild-caught males. Anim. Behav. 46, 1079–1090. doi: 10.1006/anbe.1993.1298
Danecek, P., Schiffels, S., and Durbin, R. (2016). Multiallelic Calling Model in Bcftools (-m). Available online at: https://samtools.github.io/bcftools/call-m.pdf (accessed March 26, 2021).
de Villemereuil, P., Gimenez, O., and Doligez, B. (2013). Comparing parent-offspring regression with frequentist and Bayesian animal models to estimate heritability in wild populations: A simulation study for Gaussian and binary traits. Methods Ecol. Evol. 4, 260–275. doi: 10.1111/2041-210x.12011
de Villemereuil, P., Morrissey, M. B., Nakagawa, S., and Schielzeth, H. (2018). Fixed-effect variance and the estimation of repeatabilities and heritabilities: Issues and solutions. J. Evol. Biol. 31, 621–632. doi: 10.1111/jeb.13232
Dingemanse, N. J., Both, C., Drent, P. J., and Tinbergen, J. M. (2004). Fitness consequences of avian personalities in a fluctuating environment. Proc. R. Soc. B Biol. Sci. 271, 847–852. doi: 10.1098/rspb.2004.2680
Dingemanse, N. J., Both, C., Drent, P. J., Van Oers, K., and Van Noordwijk, A. J. (2002). Repeatability and heritability of exploratory behaviour in great tits from the wild. Anim. Behav. 64, 929–938. doi: 10.1006/anbe.2002.2006
Dochtermann, N. A., and Roff, D. A. (2010). Applying a quantitative genetics framework to behavioural syndrome research. Philos. Trans. R. Soc. B Biol. Sci. 365, 4013–4020.
Dochtermann, N. A., Schwab, T., and Sih, A. (2015). The contribution of additive genetic variation to personality variation: Heritability of personality. Philos. Trans. R. Soc. B Biol. Sci. 282:20142201. doi: 10.1098/rspb.2014.2201
Dosmann, A., and Mateo, J. M. (2014). Food, sex and predators: Animal personality persists with multidimensional plasticity across complex environments. Anim. Behav. 90, 109–116. doi: 10.1016/j.anbehav.2014.01.011
Ellegren, H., Smeds, L., Burri, R., Olason, P. I., Backström, N., Kawakami, T., et al. (2012). The genomic landscape of species divergence in Ficedula flycatchers. Nature 491, 756–760. doi: 10.1038/nature11584
Eriksen, A., Lampe, H. M., and Slagsvold, T. (2009). Interspecific cross-fostering affects song acquisition but not mate choice in pied flycatchers, Ficedula hypoleuca. Anim. Behav. 78, 857–863. doi: 10.1016/j.anbehav.2009.07.005
Eriksen, A., Slagsvold, T., and Lampe, H. M. (2011). Vocal plasticity - are pied flycatchers, Ficedula hypoleuca, open-ended learners? Ethology 117, 188–198. doi: 10.1111/j.1439-0310.2010.01864.x
Fair, J., Paul, E., and Jones, J. (eds) (2010). Guidelines to the Use of Wild Birds in Research. Washington, DC: Ornithological Council.
Fidler, A. E., van Oers, K., Drent, P. J., Kuhn, S., Mueller, J. C., and Kempenaers, B. (2007). Drd4 gene polymorphisms are associated with personality variation in a passerine bird. Proc. R. Soc. B Biol. Sci. 274, 1685–1691. doi: 10.1098/rspb.2007.0337
Forstmeier, W., Burger, C., Temnow, K., and Deregnaucourt, S. (2009). The genetic basis of zebra finch vocalizations. Evolution 63, 2114–2130. doi: 10.1111/j.1558-5646.2009.00688.x
Garamszegi, L. Z., Eens, M., and Török, J. (2008). Birds reveal their personality when singing. PLoS One 3:e2647. doi: 10.1371/journal.pone.0002647
Garamszegi, L. Z., Merino, S., Török, J., Eens, M., and Martinez, J. (2006). Indicators of physiological stress and the elaboration of sexual traits in the Collared flycatcher. Behav. Ecol. 17, 399–404. doi: 10.1093/beheco/arj042
Garamszegi, L. Z., Møller, A. P., Török, J., Michl, G., Péczely, P., and Richard, M. (2004). Immune challenge mediates vocal communication in a passerine bird: An experiment. Behav. Ecol. 15, 148–157. doi: 10.1093/beheco/arg108
Garamszegi, L. Z., Mueller, J. C., Markó, G., Szász, E., Zsebõk, S., Herczeg, G., et al. (2014). The relationship between DRD4 polymorphisms and phenotypic correlations of behaviors in the Collared flycatcher. Ecol. Evol. 4, 1466–1479. doi: 10.1002/ece3.1041
Garamszegi, L. Z., Török, J., Hegyi, G., Szöllõsi, E., Rosivall, B., and Eens, M. (2007). Age-dependent expression of song in the Collared flycatcher, Ficedula albicollis. Ethology 113, 246–256. doi: 10.1111/j.1439-0310.2007.01337.x
Garamszegi, L. Z., Zsebõk, S., and Török, J. (2012). The relationship between syllable repertoire similarity and pairing success in a passerine bird species with complex song. J. Theor. Biol. 295, 68–76. doi: 10.1016/j.jtbi.2011.11.011
Gelter, H. P. (1987). Song differences between the pied flycatcher Ficedula hypoleuca, the Collared flycatcher F. albicollis, and their hybrids. Ornis Scand. 18, 205–215. doi: 10.2307/3676768
Gersick, A. S., and White, D. J. (2018). Male cowbirds vary the attractiveness of courtship songs with changes in the social context. Behaviour 155, 1–25. doi: 10.1163/1568539x-00003475
Gil, D., and Gahr, M. (2002). The honesty of bird song: Multiple constrains for multiple traits. Trends Ecol. Evol. 17, 133–140. doi: 10.1016/S0169-5347(02)02410-2
Greenwood, P. J., and Harvey, P. H. (1982). The natal and breeding dispersal of birds. Annu. Rev. Ecol. Syst. 13, 1–21. doi: 10.1146/annurev.es.13.110182.000245
Hansen, T. F., and Pelabon, C. (2021). “Evolvability: A quantitative-genetics perspective. Annu. Rev. Ecol. Evol. Syst. 52, 153–175.
Hansen, T. F., Pelabon, C., and Houle, D. (2011). Heritability is not evolvability. Evol. Biol. 38, 258–277. doi: 10.1007/s11692-011-9127-6
Hegyi, G., Szöllõsi, E., Jenni-Eiermann, S., Török, J., Eens, M., and Garamszegi, L. Z. (2010). Nutritional correlates and mate acquisition role of multiple sexual traits in male Collared flycatchers. Naturwissenschaften 97, 567–576. doi: 10.1007/s00114-010-0672-0
Houle, D., Pelabon, C., Wagner, G. P., and Hansen, T. F. (2011). Measurement and meaning in biology. Q. Rev. Biol. 86, 3–34.
Jablonszky, M., Zsebõk, S., Laczi, M., Nagy, G., Vaskuti, É, and Garamszegi, L. Z. (2021). The effect of social environment on bird song: Listener-specific expression of a sexual signal. Behav. Ecol. 32, 395–406. doi: 10.1093/beheco/araa132
Kipper, S., Kiefer, S., Bartsch, C., and Weiss, M. (2015). Female calling? Song responses to conspecific call playbacks in nightingales, Luscinia megarhynchos. Anim. Behav. 100, 60–66. doi: 10.1016/j.anbehav.2014.11.011
Kroodsma, D. E., Bereson, R. C., Byers, B. E., and Minear, E. (1989). Use of song types by the chestnut-sided warbler - Evidence for both intra-sexual and inter-sexual functions. Can. J. Zool. 67, 447–456. doi: 10.1139/z89-065
Kruuk, L. E. B. (2004). Estimating genetic parameters in natural populations using the ‘animal model’. Philos. Trans. R. Soc. B Biol. Sci. 359, 873–890. doi: 10.1098/rstb.2003.1437
Labra, A., and Lampe, H. M. (2018). The songs of male pied flycatchers: Exploring the legacy of the fathers. PeerJ 6:e5397. doi: 10.7717/peerj.5397
Lattin, C., and Ritchison, G. (2009). Intra- and intersexual functions of singing by male blue grosbeaks: The role of within-song variation. Wilson J. Ornithol. 121, 714–721. doi: 10.1676/09-026.1
Lee, J. J., and Chow, C. C. (2014). Conditions for the validity of SNP-based heritability estimation. Hum. Genet. 133, 1011–1022. doi: 10.1007/s00439-014-1441-5
Lessells, C. M., and Boag, P. T. (1987). Unrepeatable repeatabilities - A common mistake. Auk 104, 116–121.
Lewis, R. N., Soma, M., de Kort, S. R., and Gilman, R. T. (2021). Like father like son: Cultural and genetic contributions to song inheritance in an Estrildid finch. Front. Psychol. 12:2030. doi: 10.3389/fpsyg.2021.654198
Makowski, D., Ben-Shachar, M. S., Chen, S. H. A., and Lüdecke, D. (2019a). Indices of effect existence and significance in the bayesian framework. Front. Psychol. 10:2767. doi: 10.3389/fpsyg.2019.02767
Makowski, D., Ben-Shachar, M. S., and Lüdecke, D. (2019b). Bayestestr: Describing effects and their uncertainty, existence and significance within the bayesian framework. J. Open Source Softw. 4:1541. doi: 10.21105/joss.01541
Marshall, R. C., Buchanan, K. L., and Catchpole, C. K. (2003). Sexual selection and individual genetic diversity in a songbird. Proc. R. Soc. B Biol. Sci. 270:S248–S250. doi: 10.1098/rsbl.2003.0081
Mets, D. G., and Brainard, M. S. (2018). Genetic variation interacts with experience to determine interindividual differences in learned song. Proc. Natl. Acad. Sci. U.S.A. 115, 421–426. doi: 10.1073/pnas.1713031115
Montag, C., and Reuter, M. (2014). Disentangling the molecular genetic basis of personality: From monoamines to neuropeptides. Neurosci. Biobehav. Rev. 43, 228–239. doi: 10.1016/j.neubiorev.2014.04.006
Morrissey, M. B., Wilson, A. J., Pemberton, J. M., and Ferguson, M. M. (2007). A framework for power and sensitivity analyses for quantitative genetic studies of natural populations, and case studies in Soay sheep (Ovis aries). J. Evol. Biol. 20, 2309–2321. doi: 10.1111/j.1420-9101.2007.01412.x
Mullarney, K., Svensson, L., Zetterström, D., and Grant, P. J. (1999). Bird Guide, the Most Complete Field Guide to the Birds of Britain and Europe. London: HarperCollins.
Naguib, M., Diehl, J., van Oers, K., and Snijders, L. (2019). Repeatability of signalling traits in the avian dawn chorus. Front. Zool. 16:27. doi: 10.1186/s12983-019-0328-7
Naguib, M., van Rooij, E. P., Snijders, L., and van Oers, K. (2016). To sing or not to sing: Seasonal changes in singing vary with personality in wild great tits. Behav. Ecol. 27, 932–938. doi: 10.1093/beheco/arv235
Nakagawa, S., and Schielzeth, H. (2010). Repeatability for gaussian and non-gaussian data: A practical guide for biologists. Biol. Rev. Camb. Philos. Soc. 85, 935–956. doi: 10.1111/j.1469-185X.2010.00141.x
Osborn, A., and Briffa, M. (2017). Does repeatable behaviour in the laboratory represent behaviour under natural conditions? A formal comparison in sea anemones. Anim. Behav. 123, 197–206. doi: 10.1016/j.anbehav.2016.10.036
Osiejuk, T. S., Zbikowski, B., Wheeldon, A., and Budka, M. (2019). Hey mister Tambourine Dove, sing a song for me: Simple but individually specific songs of Turtur tympanistria from Cameroon. Avian Res. 10:14. doi: 10.1186/s40657-019-0153-x
Pärt, T. (1991). Is dawn singing related to paternity insurance - the case of the Collared flycatcher. Anim. Behav. 41, 451–456. doi: 10.1016/s0003-3472(05)80847-4
Postma, E. (2014). “Four decades of estimating heritabilities in wild vertebrate populations: Improved methods, more data, better estimates?,” in Quantitative Genetics in the Wild, eds A. Charmantier, D. Garant, and L. E. B. Kruuk (Oxford: Oxford University Press), 16–33.
Price, P. H. (1979). Developmental determinants of structure in zebra finch song. J. Comp. Physiol. Psychol. 93, 260–277. doi: 10.1037/h0077553
Prùchová, A., Jaška, P., and Linhart, P. (2017). Cues to individual identity in songs of songbirds: Testing general song characteristics in Chiffchaffs Phylloscopus collybita. J. Ornithol. 158, 911–924. doi: 10.1007/s10336-017-1455-6
Purcell, S., Neale, B., Todd-Brown, K., Thomas, L., Ferreira, M. A. R., Bender, D., et al. (2007). PLINK: A tool set for whole-genome association and population-based linkage analyses. Am. J. Hum. Genet. 81, 559–575. doi: 10.1086/519795
Qvarnström, A. (1997). Experimentally increased badge size increases male competition and reduces male parental care in the Collared flycatcher. Proc. R. Soc. B Biol. Sci. 264, 1225–1231. doi: 10.1098/rspb.1997.0169
R Core Team (2019). R: A Language and Environment for Statistical Computing. Vienna: R Foundation for Statistical Computing.
Réale, D., and Festa-Bianchet, M. (2003). Predator-induced natural selection on temperament in bighorn ewes. Anim. Behav. 65, 463–470. doi: 10.1006/anbe.2003.2100
Réale, D., Reader, S. M., Sol, D., McDougall, P. T., and Dingemanse, N. J. (2007). Integrating animal temperament within ecology and evolution. Biol. Rev. 82, 291–318. doi: 10.1111/j.1469-185X.2007.00010.x
Royauté, R., Garrison, C., Dalos, J., Berdal, M. A., and Dochtermann, N. A. (2019). Current energy state interacts with the developmental environment to influence behavioural plasticity. Anim. Behav. 148, 39–51. doi: 10.1016/j.anbehav.2018.11.013
Schielzeth, H., Bolund, E., Kempenaers, B., and Forstmeier, W. (2011). Quantitative genetics and fitness consequences of neophilia in zebra finches. Behav. Ecol. 22, 126–134. doi: 10.1093/beheco/arq184
Schmidt, K. A., and Belinsky, K. L. (2013). Voices in the dark: Predation risk by owls influences dusk singing in a diurnal passerine. Behav. Ecol. Sociobiol. 67, 1837–1843. doi: 10.1007/s00265-013-1593-7
Sih, A., Bell, A., and Johnson, J. C. (2004a). Behavioral syndromes: An ecological and evolutionary overview. Trends Ecol. Evol. 19, 372–378. doi: 10.1016/j.tree.2004.04.009
Sih, A., Bell, A. M., Johnson, J. C., and Ziemba, R. E. (2004b). Behavioral syndromes: An intergrative overiew. Q. Rev. Biol. 79, 241–277. doi: 10.1086/422893
Stirling, D. G., Réale, D., and Roff, D. A. (2002). Selection, structure and the heritability of behaviour. J. Evol. Biol. 15, 277–289. doi: 10.1046/j.1420-9101.2002.00389.x
Stoffel, M. A., Nakagawa, S., and Schielzeth, H. (2017). rptR: Repeatability estimation and variance decomposition by generalized linear mixed-effects models. Methods Ecol. Evol. 8, 1639–1644. doi: 10.1111/2041-210X.12797
Trösch, M., Müller, W., Eens, M., and Iserbyt, A. (2017). Genes, environments and their interaction: Song and mate choice in canaries. Anim. Behav. 126, 261–269. doi: 10.1016/j.anbehav.2017.02.006
van Oers, K., Drent, P. J., de Jong, G., and van Noordwijk, A. J. (2004). Additive and nonadditive genetic variation in avian personality traits. Heredity 93, 496–503. doi: 10.1038/sj.hdy.6800530
Verbeek, M. E. M., Drent, P. J., and Wiepkema, P. R. (1994). Consistent individual-differences in early exploratory-behavior of male great tits. Anim. Behav. 48, 1113–1121. doi: 10.1006/anbe.1994.1344
Visscher, P. M., Hill, W. G., and Wray, N. R. (2008). Heritability in the genomics era - concepts and misconceptions. Nat. Rev. Genet. 9, 255–266. doi: 10.1038/nrg2322
Wheatcroft, D., and Qvarnström, A. (2017). Genetic divergence of early song discrimination between two young songbird species. Nat. Ecol. Evol. 1:0192. doi: 10.1038/s41559-017-0192
Widmer, C., Lippert, C., Weissbrod, O., Fusi, N., Kadie, C., Davidson, R., et al. (2014). Further improvements to linear mixed models for genome-wide association studies. Sci. Rep. 4:6874. doi: 10.1038/srep06874
Wilson, A. J., Réale, D., Clements, M. N., Morrissey, M. M., Postma, E., Walling, C. A., et al. (2010). An ecologist’s guide to the animal model. J. Anim. Ecol. 79, 13–26. doi: 10.1111/j.1365-2656.2009.01639.x
York, J. E., Radford, A. N., Groothuis, T. G., and Young, A. J. (2016). Dominant male song performance reflects current immune state in a cooperatively breeding songbird. Ecol. Evol. 6, 1008–1015. doi: 10.1002/ece3.1938
Zsebõk, S., Herczeg, G., Blázi, G., Laczi, M., Nagy, G., Török, J., et al. (2018b). Minimum spanning tree as a new, robust repertoire size comparison method: Simulation and test on birdsong. Behav. Ecol. Sociobiol. 72:48. doi: 10.1007/s00265-018-2467-9
Zsebõk, S., Blázi, G., Laczi, M., Nagy, G., Vaskuti, É, and Garamszegi, L. Z. (2018a). “Ficedula”: An open-source MATLAB toolbox for cutting, segmenting and computer-aided clustering of bird song. J. Ornithol. 159, 1105–1111. doi: 10.1007/s10336-018-1581-9
Zsebõk, S., Herczeg, G., Blázi, G., Laczi, M., Nagy, G., Szász, E., et al. (2017). Short- and long-term repeatability and pseudo-repeatability of bird song: Sensitivity of signals to varying environments. Behav. Ecol. Sociobiol. 71:154. doi: 10.1007/s00265-017-2379-0
Keywords: acoustic communication, animal model, behavioral variance, heritability, SNPs
Citation: Jablonszky M, Canal D, Hegyi G, Herényi M, Laczi M, Lao O, Markó G, Nagy G, Rosivall B, Szász E, Török J, Zsebõk S and Garamszegi LZ (2022) Estimating heritability of song considering within-individual variance in a wild songbird: The collared flycatcher. Front. Ecol. Evol. 10:975687. doi: 10.3389/fevo.2022.975687
Received: 22 June 2022; Accepted: 04 October 2022;
Published: 28 October 2022.
Edited by:
Michael Sheriff, University of Massachusetts Dartmouth, United StatesReviewed by:
Roi Dor, Open University of Israel, IsraelCopyright © 2022 Jablonszky, Canal, Hegyi, Herényi, Laczi, Lao, Markó, Nagy, Rosivall, Szász, Török, Zsebõk and Garamszegi. This is an open-access article distributed under the terms of the Creative Commons Attribution License (CC BY). The use, distribution or reproduction in other forums is permitted, provided the original author(s) and the copyright owner(s) are credited and that the original publication in this journal is cited, in accordance with accepted academic practice. No use, distribution or reproduction is permitted which does not comply with these terms.
*Correspondence: Mónika Jablonszky, amFibG9uc3preS5tb25pa2FAZ21haWwuY29t
Disclaimer: All claims expressed in this article are solely those of the authors and do not necessarily represent those of their affiliated organizations, or those of the publisher, the editors and the reviewers. Any product that may be evaluated in this article or claim that may be made by its manufacturer is not guaranteed or endorsed by the publisher.
Research integrity at Frontiers
Learn more about the work of our research integrity team to safeguard the quality of each article we publish.