- 1Key Laboratory of Genetics and Germplasm Innovation of Tropical Special Forest Trees and Ornamental Plants (Hainan University), Ministry of Education, School of Forestry, Hainan University, Haikou, China
- 2Key Laboratory of Vegetation Restoration and Management of Degraded Ecosystem, South China Botanical Garden, Chinese Academy of Sciences, Guangzhou, China
- 3Development Research Center of People’s Government of Sanya City, Sanya, China
Accurately predicting global drought-induced tree mortality remains a major challenge facing plant science and ecology. Stem hydraulic safety margin (HSM, the difference between water potential at the minimum value and the value that causes xylem vulnerability to embolism) performs as one of the best hydraulic traits in predicting global drought-induced tree mortality, however, HSM is time-consuming and very difficult to measure. We proposed to use leaf turgor loss point (TLP, the water potential at which leaves start to wilt) as a proxy for HSM because HSM may be highly correlated to TLP, as both of them are tightly linked with water potential changes after stomatal closure. Since TLP is more easy and rapid to measure than HSM, if we find strong HSM-TLP relationships at the global scale, TLP can be used in predicting global drought-induced tree mortality. However, no study has quantified the relationships between HSM and TLP at the global scale. Here we draw together published data on HSM and TLP for 1,773 species from 370 sites worldwide to check whether HSM and TLP are highly associated. We found that HSMs and TLPs are merely highly related in tropical forests, thus TLP can be a reliable surrogate of HSM to predict drought-induced tree mortality in tropical forest. Here we are certainly not advocating for the use of TLP instead of HSM to predict drought-induced tree mortality in tropical forests, but simply for predicting drought-induced tree mortality in tropical forests in supplementary of HSM in the future.
Introduction
Climate change is predicted to increase both the frequency and severity of droughts worldwide (Sheffield and Wood, 2008). When the severity of drought increases, water flow in the xylem conduits of trees may be irreversibly disrupted (hydraulic failure), leading to mortality of the roots or branches and even to tree death (Rice et al., 2004; Bréda et al., 2006; Choat et al., 2018). Rapid tree mortality events could convert the world’s forest from a net carbon sink into a large carbon source during this century. However, accurately predicting the impact of climate change on plant performance and survival remains a major challenge facing plant science and ecology (Grierson et al., 2011; Bartlett et al., 2014). Recent tree mortality under frequent extreme drought events have led to recognition of the importance of the physiological mechanisms associated with drought-induced tree mortality in different biomes (McDowell et al., 2011; Anderegg et al., 2012).
Stem hydraulic safety margin (HSM) which defines the difference between water potential at the minimum value and the value that causes xylem vulnerability to embolism, is widely assumed the key to understand drought-induced tree mortality (Brodribb and Holbrook, 2003; Choat et al., 2012; Martorell et al., 2014). A meta-analysis of drought-induced mortality rates across 475 species from 33 studies around the globe revealed that HSM is one of the best predictors of drought-induced forest mortality, with lower HSM indicating higher risk of mortality (Anderegg et al., 2016). Thus, quantifying the variations in global patterns of HSM can facilitate to predict how drought-induced forest mortality varies globally. However, it is time-consuming and hard to measure HSM (Zhu et al., 2018), which impeded the possibility to quantify the dynamics of global HSM. As a result, finding a reliable proxy of HSM can be an alternative way to solve this problem.
When facing drought stress, stomatal closure has been assumed to be the vital mechanism for avoiding xylem embolism as it stops transpiration and water loss in leaves and maintains negative Ψ in stems and roots (Brodribb and Holbrook, 2003). Although stomatal closure does not stop the decline of xylem pressure and hydraulic capacity (Choat et al., 2012), it is strongly associated with both TLP (Blackman, 2018) and HSM (Brodribb and Holbrook, 2003; Martorell et al., 2014), that measure plant’s ability to keep cell turgor pressure when facing drought stress. Compared to HSM, TLP is more easy and rapid to measure (Zhu et al., 2018) and there are largely available TLP dataset at global scale (Bartlett et al., 2012). Thus, if HSM and TLP are highly associated at the global scale, TLP may be a good proxy for HSM to predict drought-induced tree mortality. However, till now only Zhu et al. (2018) has found a strong relationship between TLP and HSM in China. Blackman (2018) also assumes this association is still uncertain, but emphasizes the high necessity to quantify the relationships between HSM and TLP at the global scale.
Here we draw together previous published data on stem HSM and TLP for 1,773 species at 370 sites worldwide. Aridity index (AI), the ratio of mean annual precipitation (MAP), and potential evapotranspiration (PET) were used to represent the water environment. It is highly possible that there is no strong HSM-TLP relationship at the global scale, as at the global scale, HSM does not vary significantly with water environment (Choat et al., 2012), whereas TLP is significantly positively associated with water availability (Bartlett et al., 2012). However, it is also possible that HSM-TLP may be highly correlated in some special water environment (i.e., moist tropical rainforest). Thus we first used traditional Pearson correlation method to quantify the relationship between HSM and TLP at the global scale. Finally, we applied a novel wavelet coherence method, which can compute how the relationship between stem HSM and TLP differs while considering water availability (indicated by AI) at local scales. Our goal was to quantify whether strong HSM-TLP relationship can also be found in some special water environment.
Methods and methods
Data collection
Three categories of data were collected for each site, including plant hydraulic traits (stem HSM and TLP), AI and biome type. The detailed information is shown below:
(1) plant stem HSM and leaf TLP: We obtained stem HSM and leaf TLP for as many woody species and study sites as possible. Datasets we collected were from the TRY Plant Traits Database (Kattge et al., 2011) and published papers (see Supplementary Table 1). We also tried to collect leaf P50, but the largest dataset available was only about 60 species from three different forest types (Zhu et al., 2019), which is not enough for a meta-analysis. Because leaf TLP could be used as a convenient proxy of leaf HSM, we decided to use more available TLP data (298 species in our dataset) to represent leaf drought tolerance. Collected data were confined to measurements taken on the terminal branches of mature plants. For multiple measures on the same species from the same site, we used average values. In total, our collected dataset included 1,116 stem HSM (198 sites) and 703 TLP (172 sites) values for 1,773 species at 370 sites worldwide. There were matched HSM and TLP data in 105 sites.
(2) Aridity index (AI). AI is defined as the ratio of MAP and PET. AI directly reflects a site’s water environment and includes more climatic factors than just precipitation (Maestre et al., 2015). We used AI as a proxy for water availability or drought stress at each site. We extracted AI values for each study site based on the Global Aridity Index database at a 30 arc-s resolution1 (Trabucco and Zomer, 2009). In this study AI values range from 0.1 to 2.5; we discretized them at intervals of 0.1. The distribution of each site and its corresponding AI value are shown in Figure 1.
(3) Biome type for each site. We followed the biome type classification of the geological database of Terrestrial Ecoregions of the World (Olson et al., 2001). The biome type for each site was classified into seven biome types, i.e., deserts, woodland/shrubland, boreal forest, temperate seasonal forest, temperate rain forest, tropical seasonal forest (including savanna), and tropical rain forest. Supplementary Figure 1 shows the distribution of biome type for each study site in our study.
Statistical methods
Pearson correlation analysis
Pearson correlation is a measure of the strength and direction of the linear relationship between two random variables. We considered correlations strong if the correlation coefficient was greater than 0.8 and weak if the correlation coefficient was less than 0.5 (Bolboacǎ and Jǎntschi, 2006). A student t-test was used to determine if the value of the Pearson correlation coefficient was statistically significant. We used a significance level of 5%; a p-value less than 0.05 represents evidence to reject the null hypothesis in favor of the alternative hypothesis that there is a linear relationship between the variables. The data length of HSM and TLP are not the same in this study (1,116 stem HSM and 703 TLP values), thus we quantified the HSM-TLP relationship varies with specific water availability levels (classified by AI values). Here we calculated the mean value for stem HSM and TLP at each specific AI interval. The data series relating stem HSM and TLP to AI at a global scale are shown in Supplementary Figure 2. We then applied Pearson correlation analysis on the two data series (mean HSM-TLP vs. AI).
Wavelet coherence analysis
The wavelet transform is a useful tool for analyzing localized variations of a data series. It can transform a data series into frequency space where oscillations can be seen in an intuitive way and can show localized intermittent periodicities or relationships (Grinsted et al., 2014). Wavelet coherence can analyze two data series together. It can be thought of as the local correlation between two data series. Following Torrence and Webster (1999), the wavelet coherence of two data series can define as
where S is a smoothing operator, and can be written as
where Sscale denotes smoothing along the wavelet scale axis and Stime smoothing in time. For the Morlet wavelet used in this study, a suitable smoothing operator is given by Torrence and Webster (1999):
where c1 and c2 are normalization constants and Π() is the rectangle function. The factor of 0.6 is the empirically determined scale decorrelation length for the Morlet wavelet (Torrence and Compo, 1998; Grinsted et al., 2014). The coefficient of wavelet coherence ranges from zero to one and the closer the value approximates one, the more correlated the two series are. The statistical significance levels of the wavelet coherence, which show confidence levels against red noise, were estimated using Monte Carlo methods. The detailed process of calculation and the MATLAB codes for calculating wavelet coherence are provided in Grinsted et al. (2014). Wavelet coherence is most used for time series. Nevertheless, more recently, applications of wavelet coherence have been extended to other data series analysis (He, 2014; Guo et al., 2015; Hu and Si, 2016). Consequently, here we use it to investigate localized intermittent relationships between stem HSM and TLP values in different water environments reflected by AI. Specifically wavelet coherence analysis requires continuous time or spatial variables, so we first calculate the mean HSM and TLP for all species at each of the continuous AI variables (ranging from 0.1 to 2.5 at interval of 0.1). Then we used wavelet coherence analysis to quantify whether strong HSM-TLP relationship can be found in some special AI ranges. Previous studies have found that TLP can be easily affected by whether the species being sampled are deciduous or evergreen (Bartlett et al., 2012). Moreover, tropical rain forests have the largest proportions of evergreen species, thus we further tested if HSM-TLP relationships might vary when merely considering evergreen species, using both Pearson correlation and wavelet coherence analysis.
Results
Stem HSM and TLP values were not significantly correlated when we averaged them according to AI intervals (r = 0.2296, p = 0.2696) (Figure 2), indicating that stem HSM and TLP were not significantly correlated at the global scale. It was possible that the correlation between HSM and TLP was significant only for some particular range of AI. Results of wavelet coherence analysis supported this assertion. The correlation between stem HSM and TLP was significant only for AI values between 1.3 and 1.5 (Figure 3A), which represented the environment of tropical seasonal and rain forests (Supplementary Figure 3). When repeating the wavelet coherence analysis for just evergreen species, we found further evidence for the strongest relationships between stem HSM and TLP values in tropical woody species (Figure 3B).
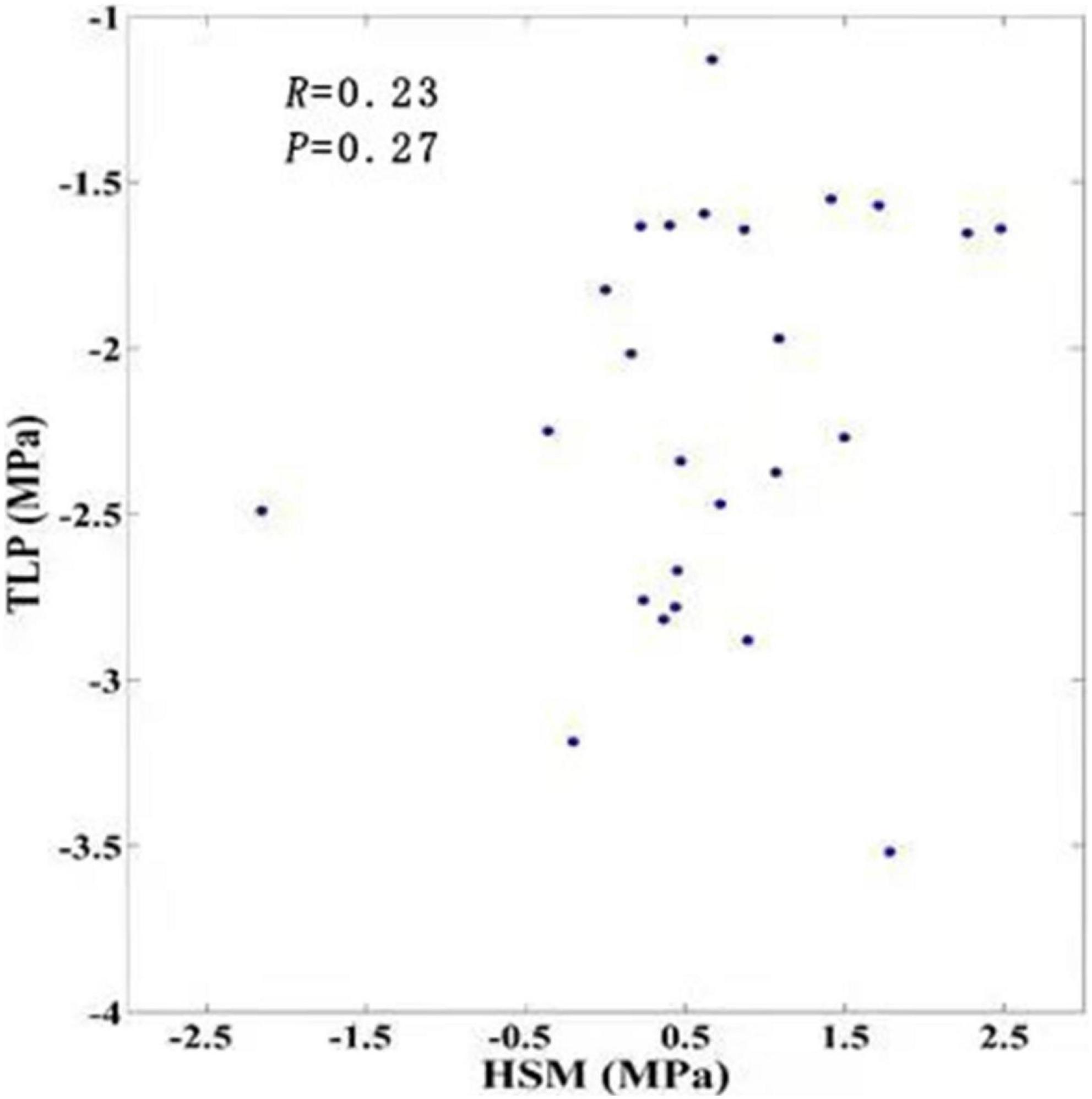
Figure 2. Relationship between stem HSM and TLP paired according to AI levels (n = 25). The Pearson correlation coefficient (R) and its corresponding p-value are presented in the upper left.
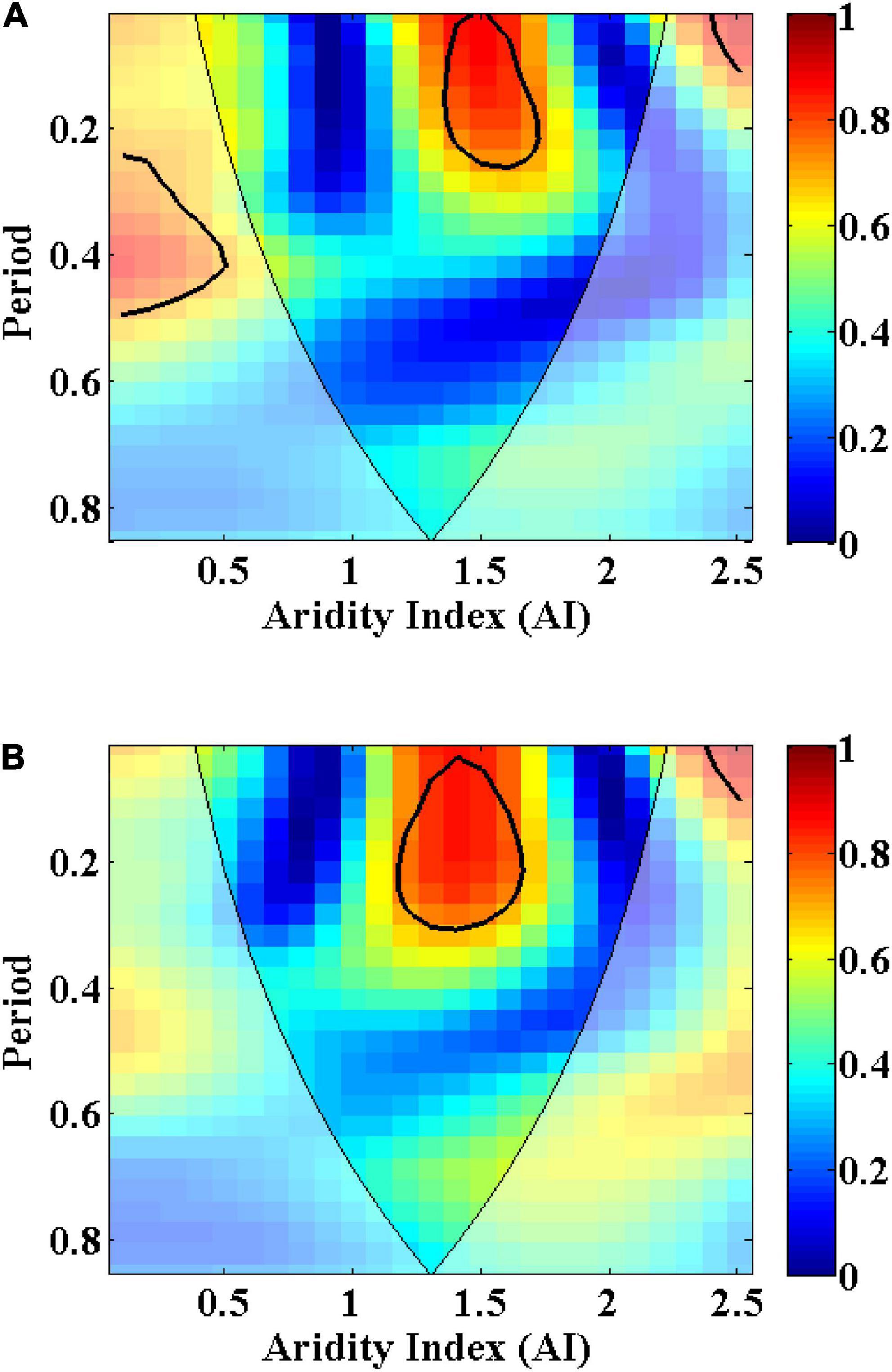
Figure 3. Wavelet coherence analysis between stem HSM and TLP for (A) all woody species and (B) evergreen woody species paired according to AI intervals at global scale. Red reflects perfect correlation while blue reflects a lack of correlation. The thick black contour represents the 95% significance level against red noise. The y label means the period of strong local correlation. In this study, the strong correlation only for AI between 1.3 and 1.5, and the corresponding y label value is about 0.2. It means that the period of strong local correlation is 0.2 (just the interval between 1.3 and 1.5). Because the edge effects cannot be ignored, we only focus on the data inside the Cone of Influence (COI) (darker shade).
Discussion
At the global scale, stem HSM values are not significantly correlated to TLP values. This indicates leaf and stem hydraulic failure are decoupled at the global scale, which supports a strong hydraulic segmentation between leaves and stems in hydraulic pathways (Bucci et al., 2013; Pivovaroff et al., 2014). However, when using wavelet coherence to analyze the regional patterns of stem HSM and TLP, we found strong stem HSM-TLP relationships at AI values indicative of tropical seasonal and rain forests. This indicates that stem and leaf hydraulic properties are well coupled only in tropical forests, as has been found in three tropical forest species (Nolf et al., 2015). This finding suggests that TLP is a good proxy of HSM to predict drought-induced tree mortality only in tropical forests.
It is reasonable that we observed strong correlations between stem HSM and TLP values only in tropical forests, given that plants tend to develop different leaf and stem drought adaptation strategies in different water environments. In wet areas, such as tropical rain and seasonal forests, stem and leaf hydraulic connections can be coupled in the absence of selection pressure from drought (Nolf et al., 2015; Zhu et al., 2016). However, other mechanisms may also save plant species from drought stress in non-tropical forest types (Klein et al., 2014). For example, in extremely dry areas, such as deserts, some plants are adapted to long-term drought stress and can survive under large stem HSM, because xylem cavitation is not always fatal (Fisher et al., 2006; Klein et al., 2011). On the other hand, leaf TLP is not closely related with tree mortality across the globe (Anderegg et al., 2016), thus it has more flexibility in drought tolerance. In deserts (roughly AI < 1.3), species show both very negative stem HSM and leaf TLP values (through different drought tolerance strategies) and no coordination between the two. Furthermore, in biomes with seasonal drought, plants tend to develop drought avoidance strategies, such as dropping leaves to prevent fatal xylem cavitation (Volaire, 2018). Similar deciduousness strategies are also used by shrub species to avoid drought stress in shrubland biomes (Hacke et al., 2000). In our data, if only considering evergreen woody species, comparable correlations between stem HSM and leaf TLP values are evident (see Figure 3B). This confirms that drought avoidance, but not drought stress resistance, prevails in temperate forest and woodland biomes, thereby causing weak HSM-TLP relationships. As a result, except for tropical forests, TLP can act as a safety valve to prevent xylem embolism (low HSM) for global forests.
Our observed HSM-TLP relationships may also explain why HSM does not vary with water environment at the global scale (Choat et al., 2012). In very wet environments, such as tropical rain and seasonal forests, although TLP cannot act as safety valve to prevent xylem embolism, HSM can still persist at safe values to avoid xylem embolism due to the lower probability of drought stress (Nolf et al., 2015) and cavitation-resistant leaves and effective hydraulic compensatory strategies (Zhu et al., 2016). In other biomes, TLP can serve as a safety valve (e.g., shed leaves) to prevent stem xylem embolism even in extreme arid environments such as desert. Thus, HSM can remain at safe values for all biomes. However, there is a possibility that HSM in tropical rain forest could drop under extreme drought stress, as is suggested by high drought-induced tree mortality in tropical rain forests. We noted that our HSM data were not measured during extreme drought stress, which explains why we observed globally similar HSM values (Choat et al., 2012). Future studies should examine HSM during extreme drought stress to better understand how global HSMs response to drought stress.
There are still some gaps in our understanding of the HSM-TLP relationships at the global scale. First, currently, there is a large innovation on the measurements of HSM and TLP and also some difference among them (see Sergent et al., 2020, doi.org/10.1016/j.foreco.2020.118175). Moreover, one single water potential measurement can hardly be considered an estimate of minimum leaf water potential which is the basis for calculating HSM. That is because a proper estimate of minimum water potential is likely to be substantially lower than the values you report for a single date, even if during the dry season. Here for collecting as many HSM and TLP data as possible to perform reliable wavelet coherence analysis, we cannot consider these two potential limitations for our collected HSM and TLP data. Thus, future quantifying the HSM-TLP relationships are still needed to be performed, when many and reliable HSM and TLP data are available. The fifth intergovernmental panel on climate change assessment report (IPCC AR5) indicates that, by the end of the twenty-first century, it is very likely that extreme drought events will be more intense and frequent over the tropical regions (Ipcc, 2013). Indeed, drought has occurred more frequently in tropical regions recently. Examples include the dry extremes over the Amazon in 2005 and 2010 and Northeast Brazil in 2012 (Marengo et al., 2008, 2013; Coelho et al., 2012). Models predict that extreme drought will result in ∼223-million-hectare canopy cover losses by 2050, with the vast majority of losses occurring in the tropics (Cox et al., 2000; Bastin et al., 2019), which in turn could result in large carbon emissions. Given the strong relationship between HSM and TLP in tropical forests, TLP should possibly be used to predict drought induced tree mortality in tropics. Moreover, given the largely available TLP data in tropics, TLP can also be directly incorporated into models forecasting the influence of increased drought-induced tree mortality on carbon emissions in tropical rain forests. Here we are certainly not advocating for the use of TLP instead of HSM to predict drought-induced tree mortality in tropical forests, but simply for the addition of HSM in predicting drought-induced tree mortality in tropical forests in the future.
Data availability statement
The original contributions presented in the study are included in the article/Supplementary material, further inquiries can be directed to the corresponding author/s.
Author contributions
RS: conceptualization, methodology, writing—original draft, and review and editing. HL: methodology, data curation, and writing—review and editing. CW: writing—review and editing. HZ and JC: conceptualization, methodology, data curation, and writing—original draft. All authors contributed to the article and approved the submitted version.
Funding
This work was funded by the Hainan Provincial Natural Science Foundation of China (2019RC161 and 422CXTD508), the specific research fund of the Innovation Platform for Academicians of Hainan Province, Research project of Hainan academician innovation platform (YSPTZX202017), the Scientific Research Project of Ecological Restoration of Baopoling (Sanya), the Pearl River S&T Nova Program of Guangzhou (201806010083), and the Youth Innovation Promotion Association of the Chinese Academy of Sciences (2019339).
Conflict of interest
The authors declare that the research was conducted in the absence of any commercial or financial relationships that could be construed as a potential conflict of interest.
Publisher’s note
All claims expressed in this article are solely those of the authors and do not necessarily represent those of their affiliated organizations, or those of the publisher, the editors and the reviewers. Any product that may be evaluated in this article, or claim that may be made by its manufacturer, is not guaranteed or endorsed by the publisher.
Supplementary material
The Supplementary Material for this article can be found online at: https://www.frontiersin.org/articles/10.3389/fevo.2022.974004/full#supplementary-material
Footnotes
References
Anderegg, W. R., Berry, J. A., Smith, D. D., Sperry, J. S., Anderegg, L. D., and Field, C. B. (2012). The roles of hydraulic and carbon stress in a widespread climate-induced forest die-off. Proceedings of the National Academy of Sciences. 109, 233–237. doi: 10.1073/pnas.1107891109
Anderegg, W. R., Klein, T., Bartlett, M., Sack, L., Pellegrini, A. F., Choat, B., et al. (2016). Meta-analysis reveals that hydraulic traits explain cross-species patterns of drought-induced tree mortality across the globe. Proceedings of the National Academy of Sciences. 113, 5024–5029. doi: 10.1073/pnas.1525678113
Bartlett, M. K., Scoffoni, C., and Sack, L. (2012). The determinants of leaf turgor loss point and prediction of drought tolerance of species and biomes: a global meta-analysis. Ecology Letters. 15, 393–405. doi: 10.1111/j.1461-0248.2012.01751.x
Bartlett, M. K., Zhang, Y., Kreidler, N., Sun, S., Ardy, R., Cao, K., et al. (2014). Global analysis of plasticity in turgor loss point, a key drought tolerance trait. Ecol. Lett. 17, 1580–1590. doi: 10.1111/ele.12374
Bastin, J. F., Finegold, Y., Garcia, C., Mollicone, D., Rezende, M., Routh, D., et al. (2019). The global tree restoration potential. Science 365, 76–79. doi: 10.1126/science.aax0848
Blackman, C. J. (2018). Leaf turgor loss as a predictor of plant drought response strategies. Tree Physiology. 38, 655–657. doi: 10.1093/treephys/tpy047
Bolboacǎ, S. D., and Jǎntschi, L. (2006). Pearson versus Spearman, Kendall’s tau correlation analysis on structure-activity relationships of biologic active compounds. Leonardo J. Sci. 5, 179–200.
Bréda, N., Huc, R., Granier, A., and Dreyer, E. (2006). Temperate forest trees and stands under severe drought: A review of ecophysiological responses, adaptation processes and long-term consequences. Ann. For. Sci. 63, 625–644. doi: 10.1051/forest:2006042
Brodribb, T. J., and Holbrook, N. M. (2003). Stomatal closure during leaf dehydration, correlation with other leaf physiological traits. Plant Physiology 132, 2166–2173. doi: 10.1104/pp.103.023879
Bucci, S. J., Scholz, F. G., Peschiutta, M. L., Arias, N. S., Meinzer, F. C., and Goldstein, G. (2013). The stem xylem of Patagonian shrubs operates far from the point of catastrophic dysfunction and is additionally protected from drought-induced embolism by leaves and roots. Plant, Cell & Environment. 36, 2163–2174. doi: 10.1111/pce.12126
Choat, B., Jansen, S., Brodribb, T. J., Cochard, H., Delzon, S., Bhaskar, R., et al. (2012). Global convergence in the vulnerability of forests to drought. Nature 491, 752–755. doi: 10.1038/nature11688
Choat, B., Brodribb, T. J., Brodersen, C. R., Duursma, R. A., López, R., and Medlyn, B. E. (2018). Triggers of tree mortality under drought. Nature 558, 531–539. doi: 10.1038/s41586-018-0240-x
Coelho, C. A. S., Cavalcanti, I. A., Costa, S., Freitas, S. R., Ito, E. R., Luz, G., et al. (2012). Climate diagnostics of three major drought events in the Amazon and illustrations of their seasonal precipitation predictions. Meteorol. Appl. 19, 237–255. doi: 10.1002/met.1324
Cox, P. M., Betts, R. A., Jones, C. D., Spall, S. A., and Totterdell, I. J. (2000). Acceleration of global warming due to carbon-cycle feedbacks in a coupled climate model. Nature 408, 184–187. doi: 10.1038/35041539
Fisher, R. A., Williams, M., Do, V., Lobo, R., Costa, A. L. D. A., and Meir, P. (2006). Evidence from Amazonian forests is consistent with isohydric control of leaf water potential. Plant, Cell & Environment. 29, 151–165. doi: 10.1111/j.1365-3040.2005.01407.x
Grierson, C. S., Barnes, S. R., Chase, M. W., Clarke, M., Grierson, D., Edwards, K. J., et al. (2011). One hundred important questions facing plant science research. New Phytol. 192, 6–12. doi: 10.1111/j.1469-8137.2011.03859.x
Grinsted, A., Moore, J. C., and Jevrejeva, S. (2014). Application of the cross wavelet transform and wavelet coherence to geophysical time series. Nonlinear Processes in Geophysics. 11, 561–566. doi: 10.5194/npg-11-561-2004
Guo, J., Wang, L., Liu, X., Kong, Q., Zhao, C., and Guo, B. (2015). Temporal-spatial variation of global gps-derived total electron content, 1999–2013. PLoS One. 10:e0133378. doi: 10.1371/journal.pone.0133378
Hacke, U. G., Sperry, J. S., and Pittermann, J. (2000). Drought experience and cavitation resistance in six shrubs from the Great Basin. Utah. Basic and Applied Ecology. 1, 31–41. doi: 10.1078/1439-1791-00006
He, Y. (2014). The relationship between an invasive shrub and soil moisture: seasonal interactions and spatially covarying relations. ISPRS International Journal of Geo-Information. 3, 1139–1153. doi: 10.3390/ijgi3031139
Hu, W., and Si, B. C. (2016). Technical note: multiple wavelet coherence for untangling scale-specific and localized multivariate relationships in geosciences. Hydrology & Earth System Sciences. 20, 3183–3191. doi: 10.5194/hess-20-3183-2016
Ipcc. (2013). “IPCC, 2013,” in climate change 2013: the physical science basis. Contribution of working group I to the fifth assessment report of the intergovernmental panel on climate change, eds T. F. Stocker, D. Qin, G.-K. Plattner, M. Tignor, S. K. Allen, J. Boschung, et al. (Cambridge: Cambridge University Press), 1535.
Kattge, J., Diaz, S., Lavorel, S. I, Prentice, C., Leadley, P., and Bönisch, G. (2011). TRY–a global database of plant traits. Global Change Biology. 17, 2905–2935. doi: 10.1111/j.1365-2486.2011.02451.x
Klein, T., Cohen, S., and Yakir, D. (2011). Hydraulic adjustments underlying drought resistance of Pinus halepensis. Tree physiology. 31, 637–648. doi: 10.1093/treephys/tpr047
Klein, T., Yakir, D., Buchmann, N., and Grünzweig, J. M. (2014). Towards an advanced assessment of the hydrological vulnerability of forests to climate change-induced drought. New Phytologist. 201, 712–716. doi: 10.1111/nph.12548
Maestre, F. T., Delgado-Baquerizo, M., Jeffries, T. C., Eldridge, D. J., Ochoa, V., Gozalo, B., et al. (2015). Increasing aridity reduces soil microbial diversity and abundance in global drylands. Proceedings of the National Academy of Sciences. 112, 15684–15689. doi: 10.1073/pnas.1516684112
Marengo, J. A., Alves, L. M., Soares, W. R., Rodriguez, D. A., Camargo, H., Riveros, M. P., et al. (2013). Two contrasting severe seasonal extremes in tropical South America in (2012). flood in Amazonia and drought in northeast Brazil. Journal of Climate. 26, 9137–9154. doi: 10.1175/JCLI-D-12-00642.1
Marengo, J. A., Nobre, C. A., Tomasella, J., Oyama, M. D., de Oliveira, G. Sampaio, Oliveira, R. De, et al. (2008). The drought of Amazonia in 2005. Journal of Climate 21, 495–516. doi: 10.1175/2007JCLI1600.1
Martorell, S., Diaz-Espejo, A., Medrano, H., Ball, M. C., and Choat, B. (2014). Rapid hydraulic recovery in Eucalyptus pauciflora after drought: linkages between stem hydraulics and leaf gas exchange. Plant, Cell and Environment 37, 617–626. doi: 10.1111/pce.12182
McDowell, N. G., Beerling, D. J., Breshears, D. D., Fisher, R. A., Raffa, K. F., and Stitt, M. (2011). The interdependence of mechanisms underlying climate-driven vegetation mortality. Trends in Ecology & Evolution 26, 523–532. doi: 10.1016/j.tree.2011.06.003
Nolf, M., Creek, D., Duursma, R., Holtum, J., Mayr, S., and Choat, B. (2015). Stem and leaf hydraulic properties are finely coordinated in three tropical rain forest tree species. Plant, Cell & Environment. 38, 2652–2661. doi: 10.1111/pce.12581
Olson, D. M., Dinerstein, E., Wikramanayake, E. D., Burgess, N. D., Powell, G. V., Underwood, E. C., et al. (2001). Terrestrial eco-regions of the world: A new map of life on earth: A new global map of terrestrial ecoregions provides an innovative tool for conserving biodiversity. BioScience 51, 933–938. doi: 10.1641/0006-3568(2001)051[0933:TEOTWA]2.0.CO;2
Pivovaroff, A. L., Sack, L., and Santiago, L. S. (2014). Coordination of stem and leaf hydraulic conductance in southern California shrubs: a test of the hydraulic segmentation hypothesis. New Phytologist. 203, 842–850. doi: 10.1111/nph.12850
Rice, K. J., Matzner, S. L., Byer, W., and Brown, J. R. (2004). Patterns of tree dieback in Queensland, Australia: The importance of drought stress and the role of resistance to cavitation. Oecologia 139, 190–198. doi: 10.1007/s00442-004-1503-9
Sergent, A. S., Varela, S. A., Barigah, T. S., Badel, E., Cochard, H., Dalla-Salda, G., et al. (2020). A comparison of five methods to assess embolism resistance in trees. For. Ecol. Manage. 468:118175. doi: 10.1016/j.foreco.2020.118175
Sheffield, J., and Wood, E. F. (2008). Global trends and variability in soil moisture and drought characteristics, 1950-2000, from observation-driven simulations of the terrestrial hydrologic cycle. J. Clim. 21, 432–458. doi: 10.1175/2007JCLI1822.1
Torrence, C., and Compo, G. P. (1998). A practical guide to wavelet analysis. Bulletin of the American Meteorological Society. 79, 61–78. doi: 10.1175/1520-0477(1998)079<0061:APGTWA>2.0.CO;2
Torrence, C., and Webster, P. J. (1999). Interdecadal changes in the enso–monsoon system. Journal of Climate. 12, 2679–2690. doi: 10.1175/1520-0442(1999)012<2679:ICITEM>2.0.CO;2
Trabucco, A., and Zomer, R. (2009). Global aridity index (global-aridity) and global potential evapo-transpiration (global-PET) geospatial database. CGIAR Consortium for Spatial Information 89, 1–2.
Volaire, F. (2018). A unified framework of plant adaptive strategies to drought: crossing scales and disciplines. Global change biology 24, 2929–2938. doi: 10.1111/gcb.14062
Zhu, S. D., Chen, Y. J., Ye, Q., He, P. C., Siddiq, Z., and Cao, K. F. (2018). Leaf turgor loss point is correlated with drought tolerance and leaf carbon economics traits. Tree Physiology 38, 658–663. doi: 10.1093/treephys/tpy013
Zhu, S. D., Li, R. H., He, P. C., Siddiq, Z., Cao, K. F., and Ye, Q. (2019). Large branch and leaf hydraulic safety margins in subtropical evergreen broadleaved forest. Tree Physiology. 39, 1405–1415. doi: 10.1093/treephys/tpz028
Keywords: drought-induced tree mortality, hydraulic failure, physiological trait, stem hydraulic safety margin, turgor loss point, wavelet coherence
Citation: Su R, Liu H, Wang C, Zhang H and Cui J (2022) Leaf turgor loss point is one of the best predictors of drought-induced tree mortality in tropical forest. Front. Ecol. Evol. 10:974004. doi: 10.3389/fevo.2022.974004
Received: 20 June 2022; Accepted: 28 July 2022;
Published: 15 August 2022.
Edited by:
Xiang Liu, Lanzhou University, ChinaReviewed by:
Yunquan Wang, Zhejiang Normal University, ChinaYao Guangqian, Lanzhou University, China
Clément Stahl, INRA Centre Antilles-Guyane, France
Copyright © 2022 Su, Liu, Wang, Zhang and Cui. This is an open-access article distributed under the terms of the Creative Commons Attribution License (CC BY). The use, distribution or reproduction in other forums is permitted, provided the original author(s) and the copyright owner(s) are credited and that the original publication in this journal is cited, in accordance with accepted academic practice. No use, distribution or reproduction is permitted which does not comply with these terms.
*Correspondence: Jie Cui, 443464199@qq.com; Hui Zhang, zhanghuitianxia@126.com
†These authors have contributed equally to this work