- 1School of Economics, Fujian Normal University, Fuzhou, China
- 2School of Management, Huazhong University of Science and Technology, Wuhan, China
The research analyzes the impact of environmental regulation on industrial green development using panel data from 30 provinces in China from 2006 to 2018. We employ the Super-slack-based measuring (SBM) model to measure the level of domestic industrial green development and use the ordinary panel model, the panel threshold model, and the spatial panel model for empirical estimation. The results reveal that the environmental regulation index plays a significant role in promoting such development. Environmental regulation index, command-and-control environmental regulation, market-incentive environmental regulation, and public-participation environmental regulation all have only a single threshold of technological progress and fiscal decentralization. Further analysis shows that China’s industrial green development presents obvious spatial agglomeration characteristics, and there is a significantly positive spatial correlation between different environmental regulation indicators and industrial green development. Our findings provide useful policy recommendations for promoting industrial green development in China.
Introduction
China’s industrial development process in recent years has been accelerating, and great achievements have been made. In 2020 its total industrial output value was 31.3 trillion yuan, or an increase of nearly 90% over 2010. However, for a long time, domestic industrial development has been excessively dependent on the input of resources and energy factors, emphasizing the expansion of output scale. Although this extensive development pattern has promoted rapid economic development, it has also led to serious environmental pollution problems (Zhu et al., 2019; Chen et al., 2020a,b; Zhao et al., 2022a,b). In 2019 China’s industry consumed about 66% of its energy and generated more than 85% of sulfur dioxide and dust. It can be seen that the realization of industrial growth is accompanied by huge environmental costs, and the deepening of its industrialization undoubtedly brings new challenges to the construction of ecological civilization.
The ninth sustainable development goal in the 2030 Agenda for Sustainable Development issued by the United Nations, which is to “Build resilient infrastructure, promote inclusive and sustainable industrialization and foster innovation,” points out the direction and presents arduous tasks for the future industrial development of countries. In fact, the China government attaches great importance to resource and environmental pollution issues and is committed to promoting sustainable industrial development. The country has proposed five development concepts of innovation, coordination, greenness, openness, and sharing and has issued a series of relevant laws and regulations to support industrial green development. In addition, the goal of peak carbon emissions by 2030 and achieving carbon neutrality by 2060 has also strengthened China’s industrial commitment to green and low-carbon development (Zhao et al., 2022c,d). In this context, the domestic industry urgently needs to transform to green production, reduce excessive resource consumption and pollutant emissions, and contribute to global pollution control and the realization of sustainable development goals (Yang et al., 2022; Zhang et al., 2022). However, effectively coordinating the relationship between industrial development and environmental protection is a major problem to be solved urgently.
Due to the negative externality of environmental pollution, it is difficult to achieve effective regulation of pollution emissions only based on spontaneous market regulation (Sun et al., 2021; Chen et al., 2022). Therefore, government intervention in pollution control and environmental protection is particularly important (Wang and Liu, 2019). Environmental regulation, as the main policy tool for the government to prevent pollution emissions, is of great significance to realize sustainable development of economy and environment (Ma and Xu, 2022). On the one hand, the implementation of environmental regulations will increase the production cost of enterprises by levying pollutant discharge fees, prompting enterprises to reduce the use of high-polluting production factors and adopt clean energy, thus achieving the goal of reducing pollution emissions (Zhang et al., 2019). On the other hand, environmental regulation will promote enterprises to carry out technological research and development and improve the level of green technology and production efficiency (Porter and Van der Linde, 1995). In addition, strict environmental regulations will not only squeeze the profit space of highly polluting enterprises and force them to withdraw from the market, but also strengthen the development of environmentally friendly enterprises and contribute to the upgrading of industrial structure. Most scholars point out that environmental regulation has been effective in improving energy efficiency and addressing the externalities of environmental pollution (Mandal, 2010; Neves et al., 2020; Chen et al., 2022).
The Chinese government in recent years has issued a series of environmental regulatory measures aimed at reducing industrial pollution emissions and achieving sustainable development through environmental regulation (Zhang et al., 2019). However, it can be found that China’s environmental quality seems to continue to deteriorate, and industrial emissions are still the main cause of environmental problems. Moreover, through in-depth research, especially after the green paradox theory was put forward, scholars are questioning the necessity and effectiveness of environmental regulation at improving environmental quality (Sinn, 2008; Van der Werf and Di Maria, 2012). Due to the imbalance of its industrial development, there are great differences in the degree of pollution emissions, which in turn result in different effects of environmental policies. In addition, the implementation of environmental regulation policies may also lead to the relocation of industries in different regions, further complicating the industrial pollution situation in China. So can environmental regulation effectively promote China’s industrial green transformation? This question has not been adequately answered. Therefore, it is necessary to clearly identify the role and influence mechanism of environmental regulation in industrial green transformation, which is of great significance for China to take further policy measures to promote industrial green transformation.
At present, scholars have conducted extensive discussions on environmental issues and provided useful evidence. However, the existing research still has the following shortcomings. First, there is no consensus on the impact of environmental regulation on environmental performance, and the existing literature on environmental performance focuses on the fields of agriculture and manufacturing (Chen et al., 2021; Chen and Zhu, 2022). There is still a lack of research on green development in the industrial sector. The development of industry is an important factor leading to environmental problems, so it is necessary to expand research on the industrial field. Second, although relevant literatures have investigated the nonlinear characteristics of environmental regulation on green innovation and pollution emission (Chen et al., 2019; Song et al., 2020), these studies seldom consider the interference of external factors and cannot identify the inflection point values. The influence of environmental regulation on industrial green development is a dynamic and complex process, which is restricted by technical conditions and institutional environment. This makes it possible that the effect has threshold characteristics. Third, most studies assume that regions are independent of each other, while ignoring the spatial correlation between economic variables in different regions, making it difficult to comprehensively analyze the spatial effect of environmental regulation on industrial green development.
We therefore adopt the threshold model and spatial econometric model to explore the relationship between environmental regulation and industrial green development in China. The main contributions of this study can be summarized as follows. First, we subdivide the types of environmental regulations, and deeply explore the differences in the impact of various environmental regulations on industrial green development, so as to provide useful supplements to existing research. Second, our study takes technological progress and fiscal decentralization as threshold variables to analyze the nonlinear effects of different types of environmental regulations on industrial green development. Third, considering the spatial dependence characteristics of regional industrial green development, this study further examines the spatial effects of different types of environmental regulations on industrial green development, thus providing a reference for the government to effectively implement joint governance policies for regional pollution.
The remaining contents of this study are arranged as follows. The second part reviews the relevant literature. The third part involves model setting, variable measurement and data description. The fourth part analyzes the empirical results. The fifth part summarizes the research results and puts forward policy suggestions. The sixth part is to clarify the limitations of the research and future research directions.
Literature review
Existing research views on the relationship between environmental regulation and environmental performance have not yet reached a consensus, but mainly offer three viewpoints.
First, most studies in the literature have noted that environmental regulation has a positive effect on environmental performance. Shapiro and Walker (2018) pointed out that environmental regulation promotes the adoption of emission reduction technologies, which is the main reason to explain the reduction of manufacturing pollution emissions. Hashmi and Alam (2019) examined the impact of environmental technologies and regulations on carbon emissions, and found that environmental regulations were more effective in reducing carbon dioxide emissions than environmental technologies, with a 1% increase in per capita environmental taxation and a 0.03% reduction in carbon dioxide emissions. Ulucak et al. (2020) took Brazil, India, China, Russia, and South Africa as research objects, and confirmed the positive role of environmental regulations in mitigating carbon emissions – that is, current environmental regulations are effective in achieving pollution reduction goals in these countries. Sun et al. (2021) recognized that environmental regulation not only increases the number of innovative products in high-tech industries, but also helps to improve the quality of innovative products, thus achieving a win-win situation for economic development and environmental governance. Cai et al. (2020) clarified that direct environmental regulation significantly stimulates green technology innovation in heavily polluting industries, and this impact is heterogeneous – that is, direct environmental regulation has a more obvious effect on green technology innovation of state-owned listed companies in heavy pollution industries and technology-capital-intensive industries.
You et al. (2019) concluded that without the influence of the government’s political system, environmental regulation can significantly facilitate the ecological investment and ecological planning innovation of industrial enterprises, which add great significance to the sustainable development of China’s economy. Liao and Shi (2018) discussed the positive effect between public appeal and green investment and showed that public appeal encourages local governments to adopt stricter environmental regulation measures, which are conducive to guiding enterprises to increase the research and development of clean technologies and green products. Wang et al. (2021) found that formal environmental regulation alleviates local air pollution by transferring polluting industries, while informal environmental regulation indirectly suppresses air pollution by improving formal environmental regulation measures. Wang et al. (2022) showed that all three types of environmental regulations have effectively contributed to the upgrading of China’s industrial structure, among which the market-incentivized environmental regulation has a more significant role in promoting the industrial structure. Yu and Wang (2021) suggested that environmental regulation policy accelerates the change of regional industrial structure, and the legislative supervision and economic incentive of environmental regulation play a stronger role in explaining the upgrading of industrial structure.
Second, some studies have also suggested that environmental regulation may negatively affect environmental performance. The enhancement of environmental regulation increases the production cost of enterprises, which may eventually inhibit the upgrading of industrial structure (Jaffe and Palmer, 1997; Wang et al., 2022). Millimet et al. (2009) explored the economic impact of environmental regulation on different aspects of the market structure and acknowledged that environmental regulation increases enterprises’ production cost, thus squeezing their profit margins and reducing their production efficiency. This will affect the entry and exit behavior of enterprises and ultimately have a negative impact on the industrial structure. Sinn (2008) noted that if fossil fuel suppliers feel a potential threat from the gradual implementation of national environmental policies, then they will extract fossil fuel reserves at a faster rate, thereby accelerating global warming. Van der Werf and Di Maria (2012) showed that imperfect environmental policies may give rise to the “green paradox” – that is, the well-intended policies encourage resource owners to increase resource extraction due to insufficient subsidies for alternative energy sources and a lag in implementation, resulting in an increase in current pollution emissions rather than a decrease.
He et al. (2022) pointed out that under the influence of fiscal decentralization, in order to maximize their own interests, local governments engage in “race to the bottom” when formulating and implementing environmental regulation policies, which is not conducive to reducing agricultural carbon emission intensity. Zhang et al. (2021) noticed that local governments in China have diversified competitive behaviors in the implementation of environmental regulations, which lead to the transfer of pollution to nearby areas and increase local carbon dioxide emissions. Moreover, this study also proves that China’s current environmental regulation is still in the stage of “green paradox”. Millimet and Roy (2016) emphasized that due to the differences in environmental standards between different regions, polluting enterprises move from areas with strict environmental requirements to areas with lax environmental regulations, leading to continuous deterioration of environmental quality in the transferred areas. Kheder and Zugravu (2012) provided evidence for the pollution haven hypothesis by analyzing the impact of environmental regulations on the site selection of French manufacturing firms. They argued that manufacturing in France is more likely to locate in other countries with looser environmental regulations, making them potentially pollution havens. The effect of environmental regulation is also disturbed by external factors. You et al. (2019) believed under the influence of the fiscal decentralization system and political promotion championships that environmental regulation has a significant inhibitory effect on ecological innovation, ecological planning innovation, and ecological investment.
Third, different from the above two viewpoints, some studies pointed out that the relationship between environmental regulation and environmental performance is uncertain or exhibits nonlinear characteristics. Hao et al. (2018) mentioned that the current environmental regulation methods implemented in China have not achieved the expected results and proved that environmental regulation is only effective in curbing pollution emissions when foreign direct investment is controlled. Ren et al. (2018) used the STIRPAT model to examine the impact of environmental regulation on eco-efficiency and found heterogeneity in the influence of different types of environmental regulation on eco-efficiency. Xie et al. (2017) proved a non-linear relationship between command-and-control and market-based environmental regulations and green productivity, and the growth effect of green productivity driven by market-based environmental regulation is much stronger than that of command- and-control regulation. Du et al. (2021) believed that when the level of economic development is low, environmental regulation has no significant impact on the upgrading of industrial structure and also inhibits green technology innovation. Only when the level of economic development is relatively high will environmental regulation significantly promote green technology innovation and industrial structure upgrading, thereby accelerating the process of economic green transformation (Chen et al., 2020a,b; Zou et al., 2022).
The research of Song et al. (2020) confirmed the U-shaped relationship between environmental regulation and green product innovation. As the intensity of environmental regulation increases, its effect on green product innovation shifts from inhibition to promotion. Zhang et al. (2020) believed that environmental regulation has a non-linear impact on carbon emissions. The improvement of environmental regulation makes the reduction effect of the total amount and intensity of carbon emissions more obvious, and foreign direct investment under the constraints of environmental regulation also inhibits carbon emissions. Chen et al. (2019) noted that environmental regulation and industrial structure have obvious non-linear effects on carbon dioxide emissions – that is, the impact of environmental regulation on carbon emissions changes with the rationalization of industrial structure. Wu et al. (2020a) confirmed a U-shape relationship between environmental regulation and green total factor energy efficiency, which means that the expansion of environmental governance decentralization has effectively improved local governments’ autonomous choices for pollution control. Chen and Qian (2020) found that various types of marine environmental regulation have a positive U-shape relationship with the upgrading of the manufacturing industry structure and the transfer of polluting industries, in which the inflection point of industrial structure upgrading occurs later than the transfer of polluting industries.
Materials and methods
Model setting
Baseline regression model
Considering the volatility of green development level, this study draws on the research of Li and Wu (2017), and firstly constructs an ordinary panel data model to explore the impact of environmental regulations on the level of industrial green development as follows.
where i denotes province and t denotes year; yit denotes industrial green development level; xit denotes environmental regulation; kit denotes a series of control variables; β1 and β2 denotes regression coefficients of core explanatory variables and control variables, respectively; μi and λt denotes individual effects and time effects, respectively; and εit is a random disturbance term.
Threshold regression model
As the impact of environmental regulation on industrial green development is a complex and dynamic process, which is easily disturbed by external factors such as technologies and policies. On the one hand, most scholars have confirmed that technological progress is a key link in achieving green development (Kang et al., 2018; Xie et al., 2020), and the effects of environmental regulation are closely related to the level of green technologies in enterprises (Ren and Ji, 2021). Therefore, it is necessary to analyze the role of technological progress in the impact of environmental regulation on industrial green development. On the other hand, the impact of environmental regulation on environmental quality is inseparable from institutional constraints (Chen and Chang, 2020; Wu et al., 2020b). Wu et al. (2020a) believe that the effect of environmental regulation on energy efficiency is closely related to environmental decentralization, and there are significant differences in the role of different types of environmental management decentralization. It can be seen that environmental regulation may have threshold characteristics in the process of acting on industrial green development. When technological progress or fiscal decentralization are on both sides of the threshold, the effect may jump or even reverse.
Therefore, we refer to the research of Wang and Shao (2019) and Wu et al. (2020a) to analyze the nonlinear characteristics of environmental regulation affecting industrial green development from the perspective of technological progress and fiscal decentralization. On this basis, drawing on relevant studies by Hansen (2000), the following threshold regression model is constructed.
where I(⋅) denotes the indicator function; qit denotes the threshold variable; and γi denotes the threshold value.
Spatial econometric model
The panel model constructed above assumes that regions are independent of each other, while in fact any economic variable in one region is often influenced by neighboring regions. Spatial autocorrelation is a common phenomenon in ecological data that affects the estimation and inference of statistical models (Legendre, 1993; Kissling and Carl, 2008). Hu and Wang (2020) emphasized that environmental regulation and environmental performance have obvious spatial attributes, and the results that ignore spatial correlation may be biased. Some scholars have conducted a spatial econometric analysis of the relationship between environmental regulation and pollution emissions, indirectly confirming the existence of this spatial correlation (Feng et al., 2020; Liu et al., 2022). Therefore, we further construct a spatial panel model to investigate the spatial effect of environmental regulation on industrial green development. The spatial correlation test is a prerequisite for spatial model regression. Referring to the study of Feng et al. (2020), we select global Moran’s I index to test whether the impact of heterogeneous environmental regulation on industrial green development is spatially dependent. The specific formula is as follows.
where yi and yj are the variable values of province i and province j, respectively; N represents the total number of regions; represents the sample mean; and wij is the adjacency space weight matrix. The values of Moran’s I index range from [–1, 1], indicating positive spatial correlation when it is greater than 0, negative spatial correlation when it is less than 0, and no spatial correlation when it is equal to 0.
The commonly used spatial econometric models mainly include the spatial lag model (SAR), spatial error model (SEM), and spatial Durbin model (SDM). Since SDM is the most general and widely used form, we adopt SDM for empirical testing based on the research of LeSage and Pace (2009). The specific form is as follows.
where denotes the spatial lagged explanatory variables of neighboring regions; N denotes the total number of regions; ρ denotes the spatial autoregressive coefficients; β and δ denote the parameters to be estimated; and other variables have the same meanings as above.
Variable description
Calculation of industrial green development level
Given that data envelopment analysis (DEA) can deal with multiple input and multiple output problems, this study uses a Super-slack-based measuring model (Super-SBM model) model containing undesirable outputs to measure the industrial green development level by referring to the relevant research of Tone (2002). The specific form is as follows.
where ρ* denotes the efficiency value; n denotes the number of decision units; m, s1, and s2 denote the number of input, desirable output, and undesirable output indicators, respectively; xij, yij, and zij denote the input, desirable output, and undesirable output variables of the evaluated units, respectively; and , , and denote the slack variables of input, desirable output, and undesirable output, respectively.
Combining with related studies, we choose capital stock, total number of employees at the end of the year, and total energy consumption as input indicators, industrial value added as desirable output, and industrial wastewater emissions, industrial solid waste emissions, industrial sulfur dioxide emissions, and industrial carbon dioxide emissions as undesirable outputs.
Calculation of environmental regulation
According to the different subjects of implementing environmental regulation policies, environmental regulation is subdivided into command-and-control environmental regulation (ERC), market-incentive environmental regulation (ERM), and public-participation environmental regulation (ERP). Among them, the command-and-control environmental regulation is measured by the amount of completed investment in industrial pollution control, the market-incentive environmental regulation is represented by pollutant discharge fees and environmental taxes, and the public-participation environmental regulation is measured by the number of proposals made by the National People’s Congress. On this basis, the overall environmental regulation index (ER) is obtained by using the entropy method and taken as a proxy variable for environmental regulation. A higher value of environmental regulation index means a higher intensity of environmental regulation.
Control variables
The control variables selected in this study include the following. Total actual utilized foreign investment is chosen to measure the foreign direct investment (FDI), so as to examine the influence of foreign investment on the level of industrial green development. Referring to the work of Shan and Zhang (2018), the coordination coefficient between industry and employment structure is measured as a proxy variable of industrial coordination degree (IC), and the indicators used involve the ratio of the added value of the tertiary industry to the total output value, as well as the proportional relationship between the employment of the tertiary industry and the total employment. Energy structure (ES) is captured by the share of coal consumption in total energy consumption. The comprehensive utilization rate of industrial solid waste is taken to measure the resource recycling level of industrial enterprises (RC). The ratio of total urban population at the end of the year to land area is selected to evaluate population density (PD). Technological progress (TI) is measured by the number of patent applications in the region. Fiscal decentralization (FD) is measured by the ratio of per capita local fiscal expenditure to per capita central fiscal expenditure.
Data sources
Considering data availability, this study selects panel data of 30 provinces in China from 2006 to 2018 (These provinces refer to provincial administrative units, including provinces, municipalities and ethnic minority autonomous regions, Tibet, Taiwan, Hong Kong, and Macao are not included in the scope of this analysis). The data of each indicator are obtained from China Statistical Yearbook, China Industrial Economic Statistical Yearbook, China Environmental Statistical Yearbook, China Energy Statistical Yearbook, the statistical yearbooks of each province, and the EPS database. To alleviate and eliminate the possible heteroscedasticity without changing the time-varying characteristics of the original data, we perform logarithmic processing on all variables. The descriptive statistical results of variables appear in Table 1.
Empirical results and analysis
Baseline regression results
The random effects model and fixed effects model are respectively used for the empirical test, and Table 2 lists the results. From the results of the Hausman test, the P-statistic values are 0.6861 and 0.1061, respectively, indicating that the research model does not reject the original hypothesis of using random effects. Therefore, we focus on the estimation results of the random effects model.
Before the inclusion of control variables, the coefficients for the effect of environmental regulation on industrial green development in model (1) and model (2) are 0.1850 and 0.1926, respectively, and both are significant at the 1% level. This indicates that environmental regulation has a significant contribution to industrial green development when the influence of other factors is not considered, and every 1% increase in environmental regulation causes at least a 0.1850% increase in industrial green development. From the regression results of model (3) and model (4), after adding the control variables, the coefficients of environmental regulation on industrial green development become 0.0840 and 0.0844, and both of them pass the 5% significance level test – that is, every 1% increase in environmental regulation raises the level of industrial green development by at least 0.0840%. Although the influence coefficient of environmental regulation decrease, its significant contribution does not change. When the intensity of environmental regulation is strengthened, industrial enterprises face considerable environmental penalty costs, which motivate them to increase investment in energy-saving equipment and clean technology R&D, thus promoting industry’s green development. As we know, the formulation and implementation of environmental regulation have a cost effect, which may squeeze out the funds needed for R&D by industrial enterprises. At the same time, there is also an innovation compensation effect, which forces enterprises to improve resource utilization and expected output through technological innovation. Therefore, the effect of environmental regulation is the result of the game of two opposing forces. From the baseline regression results, it is clear that the innovation compensation effect of environmental regulation is greater than the compliance cost effect, thereby significantly promoting China’s industrial green development.
In terms of the control variables, the coefficient of industrial coordination is significantly positive at the 5% level, suggesting that the higher the industrial coordination is, the more conducive it is to the industrial green development. This is because a reasonable industrial structure and employment structure help optimize factor allocation and promote green development efficiency through the technological linkage between industries (Zhao et al., 2016). The coefficient of resource recycling is significantly positive at the 5% level, which indicates that the improvement of resource recycling efficiency is conducive to reducing undesired outputs such as industrial waste and convert them into desired outputs, which in turn promote the development of industrial green transformation. This is also an important reason for the long-term implementation of circular economy development in China. The coefficient of technological progress is also significantly positive, meaning that technological progress contributes to industrial green development. As the core driving force of industrial transformation and upgrading, technological progress implies the transformation of traditional production methods and the improvement of enterprise production efficiency, thus promoting the green transformation and development of the entire industry. In addition, the effects of fiscal decentralization, population density, foreign direct investment, and energy structure on industrial green development fail to pass the significance test.
Robustness test
To verify the robustness of the above findings, this study re-tests the research model by subdividing regions and environmental regulation indicators. The results appear in Tables 3, 4. Table 3 reports the results of the subregional robustness test. On the one hand, due to the regional differences in China’s economic development level and resource endowment, we divide China into the eastern regions and the central and western regions, and examine the impact of environmental regulations on industrial green development in different regions. On the other hand, we calculate the average value of industrial added value in each province during the sample period, and divide the sample data into strong industrial provinces and weak industrial provinces according to the median. From the regression results of models (1)–(4) in Table 3, environmental regulation has shown a significant role in promoting industrial green development in different regions, which means that the above findings are robust.
Table 4 reports the robustness test results of the sub-indicators and replacement methods. On the one hand, environmental regulation is subdivided into command-and-control environmental regulation, market-incentive environmental regulation, and public-participation environmental regulation. We then examine the impact of the three types of environmental regulations on industrial green development. The results of models (1)–(3) in Table 4 show that the coefficients of all three types of environmental regulations are significantly positive at least at the 5% level – that is, they all significantly contribute to industrial green development. This finding is consistent with the baseline regression.
On the other hand, considering the possible endogeneity issue, the two-stage least squares regression is performed by selecting one lag period (Z1) and two lag periods (Z2) of the core explanatory variables as instrumental variables. Table 4’s models (4)–(7) report the relevant regression results. From the results of the first stage, the instrumental variables highly correlate with the endogenous variables, and environmental regulation shows a tendency to strengthen from year to year. From the results of the second stage, the values of KP rk LM-statistic are 81.410 and 67.988, respectively, and the P-values of the LM test are both 0.0000, which reject the original hypothesis and indicate that the choice of instrumental variables is reasonable. The values of KP rk wald F-statistic are 348.156 and 174.573, respectively, which are much larger than the empirical statistics value of 10, indicating that both Z1 and Z2 pass the weak instrumental variable test. Therefore, it can be considered that the selection of the two instrumental variables satisfies the necessary conditions. Specifically, the coefficients of the two instrumental variables are 0.1186 and 0.2607, respectively, and are significant at least at the 10% level, which means that environmental regulation still significantly promotes industrial green development after replacing the regression method. The above results once again confirm the robustness of the findings herein.
Threshold test
We further select technological progress and fiscal decentralization as threshold variables and apply a panel threshold model to explore the nonlinear characteristics of heterogeneous environmental regulations affecting industrial green development. The premise for conducting the threshold model test is that a threshold effect must exist. Therefore, this study uses the bootstrap self-sampling method to examine the significance level and the specific threshold value of the threshold effect.
Threshold effect of technological progress
Table 5 reports the results of the threshold effect of technological progress for each variable. From the environmental regulation index, the F-statistic for its single threshold of technological progress is significant, while the F-statistic for the double threshold is not significant, indicating that there is only a single technological progress threshold for the impact of environmental regulation on industrial green development with a threshold value of 8.7494. From the perspective of the three types of environmental regulation, the single technological progress thresholds of command-and-control environmental regulation, market-incentive environmental regulation, and public-participation environmental regulation all exist, and none of them pass the double-threshold test. The single threshold values are 8.7494, 8.7494, and 10.9373, respectively. It can be seen that the technological progress threshold values of command-and-control environmental regulation and market-incentive environmental regulation are the same as that of the environmental regulation index, while the threshold value of public-participation environmental regulation is higher. Possible explanations for this result are as follows. Currently, environmental regulation is dominated by command-and-control environmental regulation and market-incentive environmental regulation, while public-participation environmental regulation shows a great difference from the other two kinds of environmental regulation. Thus, the impact of technological progress is inconsistent.
On the basis of the above analysis, the threshold model regression is performed for a single threshold of technological progress, and the results are in Table 6. Table 6’s model (1) presents the technological progress threshold effect of the environmental regulation index. When the technological progress is in the low threshold range, the effect of environmental regulation on industrial green development is small, and its value is only 0.0679. When technological progress continues to rise to the high threshold range, the regression coefficient of environmental regulation on industrial green development increases significantly to 0.1183. The reason is that the implementation of environmental regulations squeezes out the R&D investment of industrial enterprises, while technological innovation is characterized by high investment cost, long cycle time and high risk. When the level of technological progress is low, most enterprises can only manage from the pollution side of things due to constraints of capital and technology. Although the total amount of industrial pollution emissions is controlled to a certain extent, the technological progress of the whole industry is hindered, resulting in a slow process of industrial green development. When technological progress reaches a high level, the implementation of environmental regulations encourages enterprises to shift from pollution-end governance to production-end governance – that is, to reduce undesired output by using clean technologies and energy-saving equipment, thereby vigorously promoting industrial green development.
Models (2)–(4) report the threshold effects of technological progress for three types of environmental regulations. Command-and-control environmental regulation and market-incentive environmental regulation have an upward jump after crossing the threshold value. In other words, when the level of technological progress changes from low to high, the promotion effect of environmental regulation on industrial green development is enhanced, but the reasons for the improvement of the two effects are not completely consistent. Among them, the command-and-control environmental regulation restrains the enterprises’ pollution emissions by issuing punitive and preventive regulation, which leads to an excessive cost burden placed on enterprises and limits technological progress and industrial green development. Only when the level of technological progress is raised to a certain level can environmental regulation promote the green production process of enterprises and thus improve the quality of production and industrial green development (Shen et al., 2018).
Market-incentive environmental regulation is, to the contrary, more flexible, and industrial enterprises have greater autonomy of choose. When the level of technological progress is low, the cost of pollution emission can be compensated by market means such as subsidies and deposit-return systems, so as to promote industrial green development. At higher levels of technological progress, high-tech enterprises profit from environmental regulation policies through the emissions trading market, and small- and medium-sized enterprises (SMEs) can also imitate and learn green technology processes at a lower cost, speeding up the green development of the entire industry (Wang and Xu, 2015). It is noteworthy that public-participation environmental regulation plays a significant facilitating role only when technological progress is in the low threshold range, and its effect becomes less significant as technological progress increases. One possible reason is that in the low-tech stage, the pollution emissions of enterprises are relatively greater, causing certain damage to the life safety of surrounding residents. At this stage, the polluting behavior of enterprises is more likely to be detected by the public, and they will get punished. Therefore, public-participation environmental regulation has a significantly positive effect on industrial green development. With the continuous advancement of technology, the total amount of pollution emissions decreases, and the harm to the public is alleviated. Thus, the role of public participation in environmental supervision gradually decreases at this time.
Threshold effect of fiscal decentralization
Table 7 displays the results of the fiscal decentralization threshold effect for each variable. In terms of the environmental regulation index, it has only a single fiscal decentralization threshold with a threshold value of 0.1017.1 From the three types of environmental regulation, the single threshold of fiscal decentralization exists for command-and-control environmental regulation, market-incentive environmental regulation, and public-participation environmental regulation. All have a threshold value of 0.1017, but none of them pass the double threshold test. It can be seen that the threshold value of fiscal decentralization is the same for both the environmental regulation index and different types of environmental regulation, indicating that various environmental regulation instruments reflect fiscal decentralization to a similar extent.
We further conduct a threshold model regression on the single threshold of fiscal decentralization, and the results are in Table 8. Model (1) in the table reports the fiscal decentralization threshold effect of the environmental regulation index. When the level of fiscal decentralization is below the threshold, the promotion effect of environmental regulation on industrial green development is not significant. After the fiscal decentralization crosses the threshold value, environmental regulation significantly promotes industrial green development. At this point, a 1% increase in the environmental regulation index raises the level of industrial green development by 0.0855%. This is because the expansion of fiscal decentralization helps to improve public sector efficiency and promotes government attention to environmental governance issues, which in turn increase green total factor productivity (Adam et al., 2014; Ma et al., 2021; Shi et al., 2022). When the level of fiscal decentralization is low, local governments have less autonomy to promote industrial green development through proactive environmental management. Conversely, when the level of fiscal decentralization rises to a certain level, local governments are able to improve the efficiency of environmental regulation tools based on their own information advantages to stimulate the introduction of technology and green development of enterprises.
Models (2)–(4) show the threshold effects of fiscal decentralization for three types of environmental regulations. Similar to the technological progress threshold, command-and-control environmental regulation and market-incentive environmental regulation jump upward after crossing the threshold - that is, as the degree of fiscal decentralization increases from the low threshold range to the high threshold range, the role of environmental regulation in promoting industrial green development is enhanced. This indicates that fiscal decentralization influences both command-and-control environmental regulation with technical coercion and market-incentive environmental regulation with market flexibility. Appropriate fiscal decentralization effectively mobilizes the enthusiasm of local governments and provides more innovations in public services, which guarantee the smooth implementation of environmental regulations and improve the quality of industrial green development.
The role of public-participation environmental regulation by contrast is not significant at lower levels of fiscal decentralization and only exerts a significant positive effect in the high fiscal decentralization threshold interval. The reason may be that when the degree of fiscal decentralization is low, the local government lacks enthusiasm and initiative and ignores the local public-participation environmental regulation. As a result, the environmental problems as reflected by the public cannot be solved in time, and the role of environmental regulation is not obvious. As the degree of decentralization increases, local governments have certain discretionary power, and the public has more opportunities to directly participate in local governments’ decisions on key environmental projects, so as to better take a positive role of environmental regulation on industrial green development.
Analysis of spatial effects
Too strict environmental regulation may restrict economic development, while too loose environmental regulation may turn the local area into a polluting paradise. Therefore, when local governments formulate and implement environmental regulation policies, there is often strategic interaction between regions (Zhang, 2016), which makes the impact of environmental regulation have a spatial effect. This study further incorporates spatial factors into the empirical analysis framework and uses a spatial panel model to focus on the spatial effects of heterogeneous environmental regulations on industrial green development.
Spatial autocorrelation test
Before conducting the spatial model regression, the spatial correlation of variables needs to be examined. Table 9 reports the results of the global Moran’s I index test for the level of industrial green development. The results in the table show that the univariate Moran’s I index of industrial green development is positive and passes the 5% significance test except for 2011 and 2018. Overall, the level of industrial green development in China has a strong positive spatial correlation, and industrial green development among adjacent provinces presents an obvious spatial clustering and dependence characteristics.
Since this part explores the spatial influence of environmental regulation on industrial green development, it is necessary to further investigate the spatial correlation between the two – that is, to measure the bivariate global Moran’s I index. Figure 1 portrays the bivariate Moran’s I index of environmental regulation and industrial green development. As a whole, Moran’s I index for different environmental regulation indicators and its index for industrial green development are positive and significant. Although the spatial correlation between environmental regulation and industrial green development fluctuates in different years, it does not change the positive spatial correlation between them. In conclusion, both univariate and bivariate global Moran’s I indices indicate that environmental regulation and industrial green development are influenced by spatial factors. Therefore, it is necessary to use spatial econometric methods for an in-depth discussion.
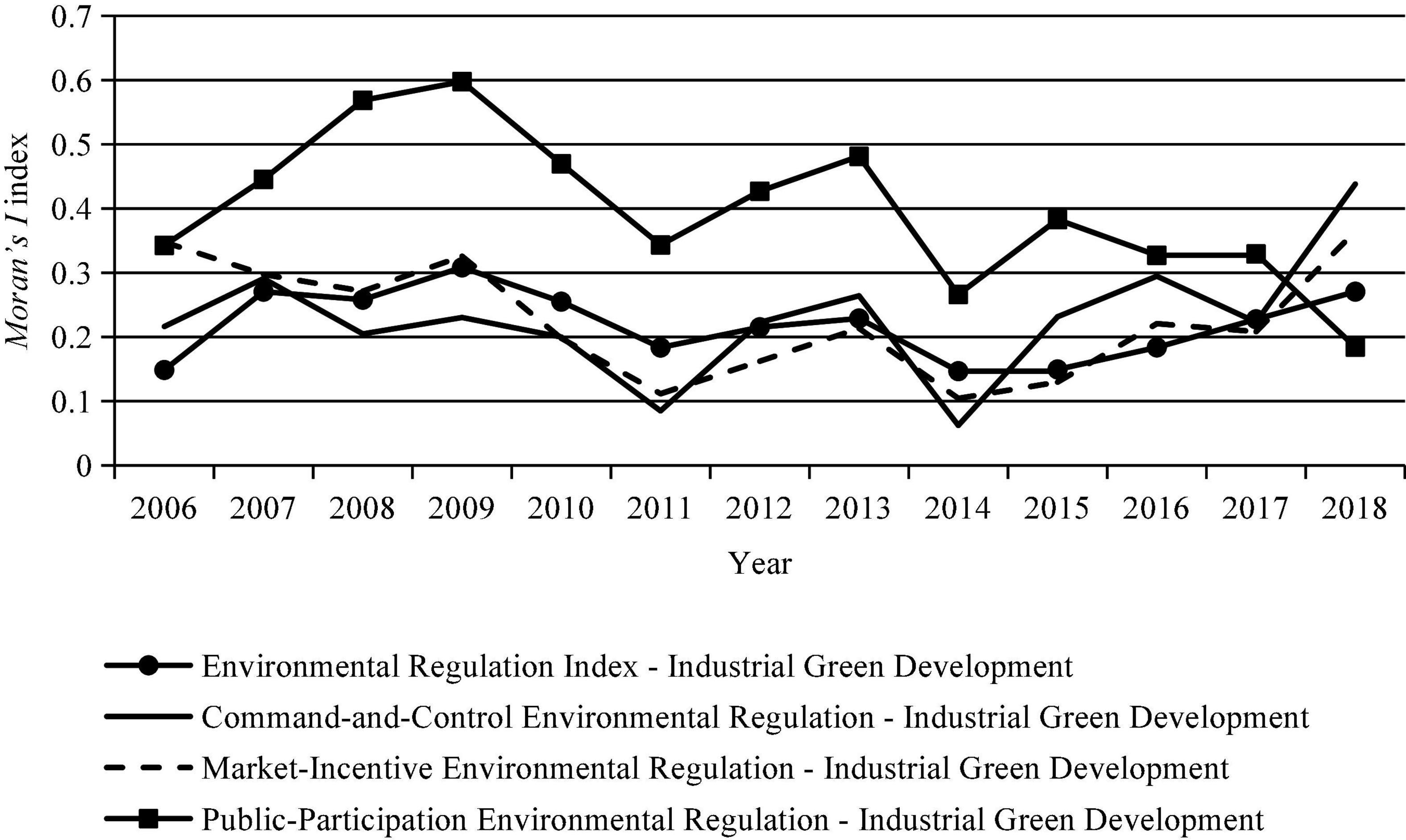
Figure 1. Bivariate Moran’s I index of heterogeneous environmental regulation and industrial green development.
Selection and regression of spatial econometric model
Before model estimation, the spatial econometric model needs to be identified and tested, and Table 10 lists the results. On the one hand, the LM test is used to explore whether a SAR or a SEM should be selected. From the results of the LM test and the robust LM test, the null hypothesis of no spatial lag or spatial error is rejected at the 1% level for the environmental regulation index and the three types of environmental regulation instruments, meaning that a spatial model needs to be selected for regression. On the other hand, we also examine which spatial model should be chosen specifically. Both the LR test and the Wald test pass the 1% significance test, indicating that SDM cannot be simplified into SAR or SEM. In addition, the Hausman test results both reject the null hypothesis of using random effects. Therefore, we choose the fixed-effect SDM to explore the spatial effect of heterogeneous environmental regulations on industrial green development.
Table 11 shows the regression results of SDM. In terms of the lagged term spatial coefficient (rho), the estimated coefficients of the environmental regulation index and three types of environmental regulation are all significant at the 10% level, suggesting a strong spatial spillover effect of industrial green development, which again confirms the conclusion drawn from the spatial correlation test. In terms of the main effect of environmental regulation, the estimated coefficients of the environmental regulation index and the three types of environmental regulations are all smaller than the coefficient values when spatial factors are not considered, representing that the promotion of environmental regulation is affected by the combined force of environmental regulation in the entire region. The actual effect of environmental regulation does not fully meet the expectation due to the superposition of many influencing factors, such as inter-regional environmental regulation strategy interaction and spatial clustering of industrial green development. Hence, the use of environmental regulation instruments should be scientifically combined from the regional level rather than limited to the local area. At the same time, the coefficients of environmental regulation index, market-incentive environmental regulation, and public-participation environmental regulation are significantly positive after considering the spatial factor, while the coefficient of command-and-control environmental regulation does not pass the significance level test, which proves that there may be competition to the bottom in the formulation of environmental regulation policies by local governments in order to develop the regional economy, leading to the failure of environmental regulation.
To examine the marginal effects of heterogeneous environmental regulations on industrial green development, the spatial effects need to be decomposed, and the results are reported in Table 12. The three effect coefficients of environmental regulation index, market-incentive environmental regulation, and public-participation environmental regulation are significantly positive, and the indirect and total effects of command-and-control environmental regulation are significantly positive, while the direct effect is not significant. This means that market-incentive environmental regulation and public-participation environmental regulation are beneficial to industrial green development of local and neighboring provinces, while command-and-control environmental regulation mainly is manifested in promoting industrial green development in neighboring provinces. In recent years, with the gradual improvement of an environmental performance assessment system, inter-provincial environmental regulation competition behavior has improved and formed a “ruler effect” (Zhang et al., 2010). As a result, the environmental regulations in adjacent areas have a certain similarity, and the increase in the intensity of environmental regulation in one place will inevitably lead to the corresponding adjustment of environmental regulations in adjacent areas, thereby driving industry’s green development. The direct effect of command-and-control environmental regulation is not significant, which also indicates that the current environmental regulation tools characterized by government coercion measures are not effective means to promote industrial green development. It is often better to make full use of diversified tools such as market-incentive environmental regulation and public-participation environmental regulation.
Conclusion and policy recommendations
In the context of the increasingly severe industrial pollution problem, this study aims to explore the relationship between environmental regulation and industrial green development, to provide a theoretical basis for further identifying the effectiveness of environmental regulation, and to make up for the lack of research on industrial green development, so as to find a sustainable development path that balances industrial development and environmental protection.
Therefore, based on panel data of 30 provinces in China from 2006 to 2018, this study constructs a panel threshold model to empirically test the nonlinear characteristics of different types of environmental regulations on industrial green development from the perspective of technological progress and fiscal decentralization. We further use the spatial panel model to analyze the spatial effects of different environmental regulations on industrial green development. The main conclusions of this study are as follows: (1) The environmental regulation index has a significant role in promoting industrial green development. For every 1% increase in the intensity of environmental regulation, the level of industrial green development rises by at least 0.0840%. (2) Environmental regulation index, command-and-control environmental regulation, market-incentive environmental regulation, and public-participation environmental regulation all have only a single threshold of technological progress and fiscal decentralization. (3) There is a significantly positive spatial correlation between different environmental regulation indicators and industrial green development. (4) The results of spatial effect analysis show that, except for command-and-control environmental regulation, the environmental regulation index and the other two types of environmental regulation have significantly positive impacts on industrial green development.
Based on the above research conclusions, we propose the following policy recommendations: (1) Since different types of environmental regulations have different impacts on industrial green development, it is necessary to use heterogeneous environmental regulation tools flexibly. For enterprises with serious industrial pollution, local governments should mainly adopt command-and-control environmental regulations and strictly supervise the pollution discharge behavior of enterprises. At the same time, the government needs to fully stimulate the vitality of market-incentive environmental regulation such as carbon emissions trading and constantly improve their trading market and systems. It should build a channel for public participation in environmental regulation and expand the coverage of education and publicity. (2) When technological progress crosses the threshold, the positive role of environmental regulation in promoting industrial green development is greatly enhanced, which means that local governments should further improve the technological innovation capabilities of industrial enterprises. The government must encourage industrial enterprises to step up R&D of clean technologies through tax incentives and financial subsidies and introduce foreign advanced environmental protection technologies to promote the upgrading of industrial enterprises. In addition, great importance must be attached to the patent protection of clean technology innovation and process efficiency improvement, providing institutional guarantee for enterprises to carry out technological R&D activities. (3) Since fiscal decentralization plays an important role in the process of environmental regulation promoting green industrial development, it is necessary to appropriately decentralize the government’s environmental governance power. The central government should further expand the authority of such departments in personnel arrangement and use of environmental governance funds to ensure the smooth implementation of environmental management power. At the same time, the proportion of environmental governance in the assessment of local governments must be strengthened, so as to encourage local governments to focus on improving environmental issues. (4) The spatial dependence of environmental problems should not be ignored, and the government needs to pay attention to the joint prevention and control of regional pollution. Local governments should improve the inter-regional cooperation mechanisms and establish regional sharing models of green technologies to jointly promote the coordinated management of environmental pollution.
Limitations and future research directions
Although we have expanded the related research from both theoretical and practical aspects, there are still the following shortcomings. First, our research focuses on provincial administrative units and fails to cover data on prefecture-level cities and enterprises. Subsequent research should further analyze the data of prefecture-level cities or enterprises, and conduct detailed research according to the industrial layout of urban agglomerations and the nature of enterprises. Second, this study lacks an examination of different types of industries. Future research should divide specific industries and further investigate the role of factor allocation ratios between different industries in the impact of environmental regulation on industrial green development. Third, we only test the influence of technological progress and fiscal decentralization, while the green development effect of environmental regulation may also be affected by other factors, especially the role of government behavior and its results. Subsequent research should be expanded from other perspectives such as government competition, market segmentation, and factor distortion.
Data availability statement
The original contributions presented in this study are included in the article/supplementary material, further inquiries can be directed to the corresponding author.
Author contributions
YY and HC: conceptualization and writing–original draft preparation, review and editing. HC: methodology, project administration, funding acquisition, and formal analysis. YY: software and resources. HC, YY, and HH: validation and data curation. MY: investigation. YY and HH: visualization. HH: supervision. All authors have read and agreed to the published version of the manuscript.
Funding
This research was funded by the National Social Science Fund General Project of China (No. 19BGL092), Innovation Strategy Research Project of Fujian Province (Nos. 2021R0156 and 2022R0030), and GF Securities Social Welfare Foundation Teaching and Research Fund for National Finance and Mesoeconomics.
Acknowledgments
The authors are grateful to the editor and the reviewers of this manuscript.
Conflict of interest
The authors declare that the research was conducted in the absence of any commercial or financial relationships that could be construed as a potential conflict of interest.
Publisher’s note
All claims expressed in this article are solely those of the authors and do not necessarily represent those of their affiliated organizations, or those of the publisher, the editors and the reviewers. Any product that may be evaluated in this article, or claim that may be made by its manufacturer, is not guaranteed or endorsed by the publisher.
Footnotes
- ^ Due to the logarithmic processing of fiscal decentralization, its level has a negative value, but it does not affect the conclusions of the empirical analysis.
References
Adam, A., Delis, M. D., and Kammas, P. (2014). Fiscal decentralization and public sector efficiency: evidence from OECD countries. Econ. Gov. 15, 17–49. doi: 10.1007/s10101-013-0131-4
Cai, X., Zhu, B., Zhang, H., Li, L., and Xie, M. (2020). Can direct environmental regulation promote green technology innovation in heavily polluting industries? Evidence from Chinese listed companies. Sci. Total Environ. 746:140810. doi: 10.1016/j.scitotenv.2020.140810
Chen, H., Lin, H., and Zou, W. (2020a). Research on the regional differences and influencing factors of the innovation efficiency of China’s high-tech industries: based on a shared inputs two-stage network DEA. Sustainability 12:3284. doi: 10.3390/su12083284
Chen, H., Zhang, L., Zou, W., Gao, Q., and Zhao, H. (2020b). Regional differences of air pollution in China: comparison of clustering analysis and systematic clustering methods of panel data based on gray relational analysis. Air Qual. Atmos. Health 13, 1257–1269. doi: 10.1007/s11869-020-00880-0
Chen, H., Shi, Y., and Zhao, X. (2022). Investment in renewable energy resources, sustainable financial inclusion and energy efficiency: a case of US economy. Resour. Policy. 77:102680. doi: 10.1016/j.resourpol.2022.102680
Chen, X., and Chang, C. P. (2020). Fiscal decentralization, environmental regulation, and pollution: a spatial investigation. Environ. Sci. Pollut. Res. 27, 31946–31968. doi: 10.1007/s11356-020-09522-5
Chen, X., Chen, Y. E., and Chang, C. P. (2019). The effects of environmental regulation and industrial structure on carbon dioxide emission: a non-linear investigation. Environ. Sci. Pollut. Res. 26, 30252–30267. doi: 10.1007/s11356-019-06150-6
Chen, X., and Qian, W. (2020). Effect of marine environmental regulation on the industrial structure adjustment of manufacturing industry: an empirical analysis of China’s eleven coastal provinces. Mar. Policy 113:103797. doi: 10.1016/j.marpol.2019.103797
Chen, Y., Miao, J., and Zhu, Z. (2021). Measuring green total factor productivity of China’s agricultural sector: a three-stage SBM-DEA model with non-point source pollution and CO2 emissions. J. Clean. Prod. 318:128543. doi: 10.1016/j.jclepro.2021.128543
Chen, Y., and Zhu, Z. (2022). Liability Structure and Carbon Emissions Abatement: evidence from Chinese Manufacturing Enterprises. Environ. Resour. Econ. 1–27. doi: 10.1007/s10640-022-00649-2
Du, K., Cheng, Y., and Yao, X. (2021). Environmental regulation, green technology innovation, and industrial structure upgrading: the road to the green transformation of Chinese cities. Energy Econ. 98:105247. doi: 10.1016/j.eneco.2021.105247
Feng, T., Du, H., Lin, Z., and Zuo, J. (2020). Spatial spillover effects of environmental regulations on air pollution: evidence from urban agglomerations in China. J. Environ. Manag. 272:110998. doi: 10.1016/j.jenvman.2020.110998
Hansen, B. E. (2000). Sample splitting and threshold estimation. Econometrica 68, 575–603. doi: 10.1111/1468-0262.00124
Hao, Y. U., Deng, Y., Lu, Z. N., and Chen, H. (2018). Is environmental regulation effective in China? Evidence from city-level panel data. J. Clean. Prod. 188, 966–976. doi: 10.1016/j.jclepro.2018.04.003
Hashmi, R., and Alam, K. (2019). Dynamic relationship among environmental regulation, innovation, CO2 emissions, population, and economic growth in OECD countries: a panel investigation. J. Clean. Prod. 231, 1100–1109. doi: 10.1016/j.jclepro.2019.05.325
He, Q., Deng, X., Li, C., Yan, Z., Kong, F., and Qi, Y. (2022). The green paradox puzzle: fiscal decentralisation, environmental regulation, and agricultural carbon intensity in China. Environ. Sci. Pollut. Res. [Epub ahead of print]. doi: 10.1007/s11356-022-21149-2
Hu, W., and Wang, D. (2020). How does environmental regulation influence China’s carbon productivity? An empirical analysis based on the spatial spillover effect. J. Clean. Prod. 257:120484. doi: 10.1016/j.jclepro.2020.120484
Jaffe, A. B., and Palmer, K. (1997). Environmental regulation and innovation: a panel data study. Rev. Econ. Stat. 79, 610–619. doi: 10.1162/003465397557196
Kang, Z. Y., Li, K., and Qu, J. (2018). The path of technological progress for China’s low-carbon development: evidence from three urban agglomerations. J. Clean. Prod. 178, 644–654. doi: 10.1016/j.jclepro.2018.01.027
Kheder, S. B., and Zugravu, N. (2012). Environmental regulation and French firms location abroad: an economic geography model in an international comparative study. Ecol. Econ. 77, 48–61. doi: 10.1016/j.ecolecon.2011.10.005
Kissling, W. D., and Carl, G. (2008). Spatial autocorrelation and the selection of simultaneous autoregressive models. Glob. Ecol. Biogeogr. 17, 59–71. doi: 10.1111/j.1466-8238.2007.00334.x
Legendre, P. (1993). Spatial autocorrelation: trouble or new paradigm? Ecology 74, 1659–1673. doi: 10.2307/1939924
LeSage, J., and Pace, R. K. (2009). Introduction To Spatial Econometrics. London: Chapman and Hall/CRC.
Li, B., and Wu, S. (2017). Effects of local and civil environmental regulation on green total factor productivity in China: a spatial Durbin econometric analysis. J. Clean. Prod. 153, 342–353. doi: 10.1016/j.jclepro.2016.10.042
Liao, X. C., and Shi, X. P. (2018). Public appeal, environmental regulation and green investment: evidence from China. Energy Policy 119, 554–562. doi: 10.1016/j.enpol.2018.05.020
Liu, C., Xin, L., and Li, J. (2022). Environmental regulation and manufacturing carbon emissions in China: a new perspective on local government competition. Environ. Sci. Pollut. Res. 29, 36351–36375. doi: 10.1007/s11356-021-18041-w
Ma, X., and Xu, J. (2022). Impact of Environmental Regulation on High-Quality Economic Development. Front. Environ. Sci. 10:896892. doi: 10.3389/fenvs.2022.896892
Ma, X., Zhao, X., Zhang, L., Zhou, Y., and Chen, H. (2021). Spatial-temporal characteristics and influencing factors of atmospheric environmental efficiency in China. Environ. Sci. Pollut. Res. 28, 12428–12440. doi: 10.1007/s11356-020-11128-w
Mandal, S. K. (2010). Do undesirable output and environmental regulation matter in energy efficiency analysis? Evidence from Indian cement industry. Energy Policy 38, 6076–6083. doi: 10.1016/j.enpol.2010.05.063
Millimet, D. L., and Roy, J. (2016). Empirical tests of the pollution haven hypothesis when environmental regulation is endogenous. J. Appl. Econom. 31, 652–677. doi: 10.1002/jae.2451
Millimet, D. L., Roy, S., and Sengupta, A. (2009). Environmental regulations and economic activity: influence on market structure. Ann. Rev. Resour. Econ. 1, 99–118. doi: 10.1146/annurev.resource.050708.144100
Neves, S. A., Marques, A. C., and Patrício, M. (2020). Determinants of CO2 emissions in European Union countries: does environmental regulation reduce environmental pollution? Econ. Anal. Policy 68, 114–125. doi: 10.1016/j.eap.2020.09.005
Porter, M. E., and Van der Linde, C. (1995). Toward a new conception of the environment-competitiveness relationship. J. Econ. Perspect. 9, 97–118. doi: 10.1257/jep.9.4.97
Ren, S., Li, X., Yuan, B., Li, D., and Chen, X. (2018). The effects of three types of environmental regulation on eco-efficiency: a cross-region analysis in China. J. Clean. Prod. 173, 245–255. doi: 10.1016/j.jclepro.2016.08.113
Ren, W., and Ji, J. (2021). How do environmental regulation and technological innovation affect the sustainable development of marine economy: new evidence from China’s coastal provinces and cities. Mar. Policy 128:104468. doi: 10.1016/j.marpol.2021.104468
Shan, L., and Zhang, T. (2018). Study on the Spatiotemporal Evolvement of Coordination between Industrial Structure and Employment Structure in China. Chin. J. Popul. Sci. 2, 39–49.
Shapiro, J. S., and Walker, R. (2018). Why is pollution from US manufacturing declining? The roles of environmental regulation, productivity, and trade. Am. Econ. Rev. 108, 3814–3854. doi: 10.1257/aer.20151272
Shen, C., Li, S., and Huang, L. (2018). Different Types of Environmental Regulation and the Green Transformation of Chinese Industry: path Selection and Mechanism Analysis. Nankai Econ. Stud. 5, 95–114. doi: 10.14116/j.nkes.2018.05.006
Shi, Y., Xie, Y., Chen, H., and Zou, W. (2022). Spatial and Temporal Differences in the Health Expenditure Efficiency of China: reflections Based on the Background of the COVID-19 Pandemic. Front. Public Health 10:879698. doi: 10.3389/fpubh.2022.879698
Sinn, H. W. (2008). Public policies against global warming: a supply side approach. Int. Tax Public Financ. 15, 360–394. doi: 10.1007/s10797-008-9082-z
Song, M., Wang, S., and Zhang, H. (2020). Could environmental regulation and R&D tax incentives affect green product innovation? J. Clean. Prod. 258:120849. doi: 10.1016/j.jclepro.2020.120849
Sun, Z., Wang, X., Liang, C., Cao, F., and Wang, L. (2021). The impact of heterogeneous environmental regulation on innovation of high-tech enterprises in China: mediating and interaction effect. Environ. Sci. Pollut. Res. 28, 8323–8336. doi: 10.1007/s11356-020-11225-w
Tone, K. (2002). A slacks-based measure of super-efficiency in data envelopment analysis. Eur. J. Operat. Res. 143, 32–41. doi: 10.1016/S0377-2217(01)00324-1
Ulucak, R., Khan, S. U. D., Baloch, M. A., and Li, N. (2020). Mitigation pathways toward sustainable development: is there any trade-off between environmental regulation and carbon emissions reduction? Sustain. Dev. 28, 813–822. doi: 10.1002/sd.2032
Van der Werf, E., and Di Maria, C. (2012). Imperfect environmental policy and polluting emissions: the green paradox and beyond. Int. Rev. Environ. Resour. Econ. 6, 153–194. doi: 10.1561/101.00000050
Wang, H., and Liu, H. (2019). Foreign direct investment, environmental regulation, and environmental pollution: an empirical study based on threshold effects for different Chinese regions. Environ. Sci. Pollut. Res. 26, 5394–5409. doi: 10.1007/s11356-018-3969-8
Wang, L., Wang, Z., and Ma, Y. (2022). Heterogeneous environmental regulation and industrial structure upgrading: evidence from China. Environ. Sci. Pollut. Res. 29, 13369–13385. doi: 10.1007/s11356-021-16591-7
Wang, S., and Xu, Y. (2015). Environmental regulation and haze pollution decoupling effect: based on the perspective of enterprise investment preferences. China Ind. Econ. 4, 18–30. doi: 10.19581/j.cnki.ciejournal.2015.04.003
Wang, T., Peng, J., and Wu, L. (2021). Heterogeneous effects of environmental regulation on air pollution: evidence from China’s prefecture-level cities. Environ. Sci. Pollut. Res. 28, 25782–25797. doi: 10.1007/s11356-021-12434-7
Wang, X., and Shao, Q. (2019). Non-linear effects of heterogeneous environmental regulations on green growth in G20 countries: evidence from panel threshold regression. Sci. Total Environ. 660, 1346–1354. doi: 10.1016/j.scitotenv.2019.01.094
Wu, H., Hao, Y., and Ren, S. (2020a). How do environmental regulation and environmental decentralization affect green total factor energy efficiency: evidence from China. Energy Econ. 91:104880. doi: 10.1016/j.eneco.2020.104880
Wu, H., Li, Y., Hao, Y., Ren, S., and Zhang, P. (2020b). Environmental decentralization, local government competition, and regional green development: evidence from China. Sci. Total Environ. 708:135085. doi: 10.1016/j.scitotenv.2019.135085
Xie, R. H., Yuan, Y. J., and Huang, J. J. (2017). Different types of environmental regulations and heterogeneous influence on “green” productivity: evidence from China. Ecol. Econ. 132, 104–112. doi: 10.1016/j.ecolecon.2016.10.019
Xie, W., Yan, T., Xia, S., and Chen, F. (2020). Innovation or introduction? The impact of technological progress sources on industrial green transformation of resource-based cities in China. Front. Energy Res. 8:598141. doi: 10.3389/fenrg.2020.598141
Yang, Y., Wu, D., Xu, M., Yang, M., and Zou, W. (2022). Capital misallocation, technological innovation, and green development efficiency: empirical analysis based on China provincial panel data. Environ. Sci. Pollut. Res. [Epub ahead of print]. doi: 10.1007/s11356-022-20364-1
You, D., Zhang, Y., and Yuan, B. (2019). Environmental regulation and firm eco-innovation: evidence of moderating effects of fiscal decentralization and political competition from listed Chinese industrial companies. J. Clean. Prod. 207, 1072–1083. doi: 10.1016/j.jclepro.2018.10.106
Yu, X., and Wang, P. (2021). Economic effects analysis of environmental regulation policy in the process of industrial structure upgrading: evidence from Chinese provincial panel data. Sci. Total Environ. 753:142004. doi: 10.1016/j.scitotenv.2020.142004
Zhang, H. (2016). Strategic Interaction of Regional Environmental Regulation: an Explanation on the Universality of Incomplete Enforcement of Environmental Regulation. China Ind. Econ. 7, 74–90. doi: 10.19581/j.cnki.ciejournal.2016.07.006
Zhang, L., Wang, Q., and Zhang, M. (2021). Environmental regulation and CO2 emissions: based on strategic interaction of environmental governance. Ecol. Complex. 45:100893. doi: 10.1016/j.ecocom.2020.100893
Zhang, L., Xu, M., Chen, H., Li, Y., and Chen, S. (2022). Globalization, Green Economy and Environmental Challenges: state of the Art Review for Practical Implications. Front. Environ. Sci. 10:870271. doi: 10.3389/fenvs.2022.870271
Zhang, M., Liu, X., Ding, Y., and Wang, W. (2019). How does environmental regulation affect haze pollution governance?—An empirical test based on Chinese provincial panel data. Sci. Total Environ. 695:133905. doi: 10.1016/j.scitotenv.2019.133905
Zhang, W., Li, G., Uddin, M. K., and Guo, S. (2020). Environmental regulation, foreign investment behavior, and carbon emissions for 30 provinces in China. J. Clean. Prod. 248:119208. doi: 10.1016/j.jclepro.2019.119208
Zhang, W., Zhang, L., and Zhang, K. (2010). The pattern and evolution of inter-provincial competition in China’s environmental regulation intensity: an analysis based on the two-regional spatial Durbin fixed-effects model. J. Manag. World 12, 34–44.
Zhao, L., Zhang, L., Xu, L., and Hu, M. (2016). Mechanism of human capital, industrial structure adjustment and green development efficiency. China Popul. Resour. Environ. 26, 106–114. doi: 10.3969/j.issn.1002-2104.2016.11.014
Zhao, X., Mahendru, M., Ma, X., Rao, A., and Shang, Y. (2022a). Impacts of environmental regulations on green economic growth in China: new guidelines regarding renewable energy and energy efficiency. Renew. Energy 187, 728–742. doi: 10.1016/j.renene.2022.01.076
Zhao, X., Ramzan, M., Sengupta, T., Deep Sharma, G., Shahzad, U., and Cui, L. (2022b). Impacts of bilateral trade on energy affordability and accessibility across Europe: does economic globalization reduce energy poverty? Energy Build. 262:112023. doi: 10.1016/j.enbuild.2022.112023
Zhao, X., Ma, X., Chen, B., Shang, Y., and Song, M. (2022c). Challenges toward carbon neutrality in China: strategies and countermeasures. Resour. Conserv. Recycl. 176:105959. doi: 10.1016/j.resconrec.2021.105959
Zhao, X., Ma, X., Shang, Y., Yang, Z., and Shahzad, U. (2022d). Green economic growth and its inherent driving factors in Chinese cities: based on the Metafrontier-global-SBM super-efficiency DEA model. Gondwana Res. 106, 315–328. doi: 10.1016/j.gr.2022.01.013
Zhu, L., Hao, Y., Lu, Z. N., Wu, H., and Ran, Q. (2019). Do economic activities cause air pollution? Evidence from China’s major cities. Sustain. Cities Soc. 49:101593. doi: 10.1016/j.scs.2019.101593
Keywords: environmental regulation, industrial green development, technological progress, fiscal decentralization, heterogeneity
Citation: Chen H, Yang Y, Yang M and Huang H (2022) The impact of environmental regulation on China’s industrial green development and its heterogeneity. Front. Ecol. Evol. 10:967550. doi: 10.3389/fevo.2022.967550
Received: 13 June 2022; Accepted: 04 July 2022;
Published: 29 July 2022.
Edited by:
Piotr Toczyski, The Maria Grzegorzewska University, PolandReviewed by:
Haitao Wu, Beijing Institute of Technology, ChinaYufeng Chen, Zhejiang Gongshang University, China
Copyright © 2022 Chen, Yang, Yang and Huang. This is an open-access article distributed under the terms of the Creative Commons Attribution License (CC BY). The use, distribution or reproduction in other forums is permitted, provided the original author(s) and the copyright owner(s) are credited and that the original publication in this journal is cited, in accordance with accepted academic practice. No use, distribution or reproduction is permitted which does not comply with these terms.
*Correspondence: Hao Huang, aHVhbmdoYW8yMTU2QGh1c3QuZWR1LmNu