Background and summary
Dispersal is a key process in ecology and evolution, as it contributes to shaping the spatial patterns of biological diversity at all levels of organization (Kokko and López-Sepulcre, 2006; Clobert et al., 2012). Growing evidence has revealed the role of phenotypic trait variation in affecting the dispersal dynamics of populations (Liedvogel et al., 2011). Moreover, spatial sorting of dispersal-related traits during range expansion events can lead to substantial, rapid, and directional changes in the phenotypic makeup of populations (Canestrelli et al., 2016a,b; Miller et al., 2020). Accordingly, selective pressures at the front of a range expansion wave would actively promote individuals with higher dispersal abilities (Lindström et al., 2013; Canestrelli et al., 2016a,b; Pizzatto et al., 2017). Although many studies have focused on phenotypic trait evolution during range expansion, its genomic underpinnings are almost unexplored, hampering a thorough understanding of the evolutionary processes involved in dispersal.
The Tyrrhenian tree frog Hyla sarda (De Betta, 1853) is an amphibian inhabiting the islands of Sardinia and Corsica and the Tuscan archipelago in the Tyrrhenian Sea (Western Mediterranean). According to previous phylogeographic studies, H. sarda underwent a northward range expansion from north of Sardinia (western Mediterranean Sea) during the last glacial phase (Spadavecchia et al., 2021). The colonization of Corsica Island was allowed by the temporary formation of a wide land bridge connecting Sardinia and Corsica, induced by the marine regression that occurred during the last glacial maximum (Bisconti et al., 2011a,b; Spadavecchia et al., 2021). The postglacial loss of this land bridge prevented subsequent gene flow between the Corsican and Sardinian populations. Interestingly, the genetic and phenotypic legacies of this range expansion event can still be detected in the existing populations of H. sarda (Liparoto, 2020; Canestrelli et al., 2021; Bisconti et al., 2022). Indeed, substantial personality trait variation has been observed among Corsica, Sardinia, and Elba populations, i.e., tree frogs from Corsica showed a shyer personality than tree frogs from Sardinia and Elba (Liparoto et al., 2020; Bisconti et al., 2022). Thus, H. sarda is an interesting candidate to study the genetic underpinnings of phenotypic trait evolution during range expansion.
Here, we contribute to the investigation of the genetic underpinning of phenotypic trait evolution during range expansion by generating the first brain de novo transcriptome of H. sarda. We focused on the brain transcriptome since brain gene expression patterns have been linked to a wide range of behavioral responses to environmental stimuli (Whitfield et al., 2003; Bendesky and Bargmann, 2011; Rey et al., 2013; Harris and Hofmann, 2014; Bell et al., 2016). The transcriptome presented here has been validated and annotated to provide a valuable genomic resource for further genetic, ecological, and behavioral studies.
Methods
Dataset generation
The sampling procedures were approved by the Institute for Environmental Protection and Research (“ISPRA”) (protocol #5944), Ministry of Environment “MATTM” (protocol #8275), Regione Sardegna (#12144), and Corsica (#2A20180206002 and #2B20180206001). The Local Health and Veterinary Centre provided permission to temporarily house amphibians with license code 050VT427. All handling procedures outlined in this study were performed under the approval of the Ethical Committee of the University of Tuscia for the use of live animals (D.R. n. 677/16 and D.R.644/17).
To generate a comprehensive de novo transcriptome, representative of the geographic and behavioral variation, we collected and sequenced nine Tyrrhenian tree frog individuals from distinct islands: four individuals from Sardinia, four from Corsica, and one from Elba (see Table 1). To test the boldness and shyness of individuals, two tests were performed: first to test the propensity of individuals to explore a novel environment and second to measure the latency to exit from a shelter as a proxy for willingness to take risks. Details of the sampling procedures and behavioral tests are described in Bisconti et al. (2022). The behavioral phenotypes of each individual are presented in Table 1.
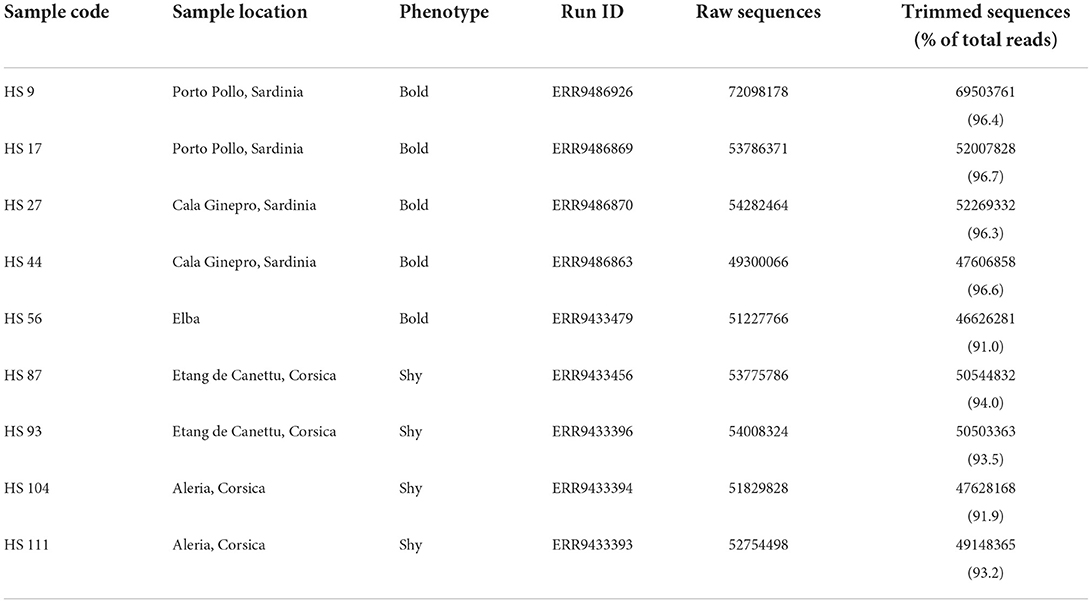
Table 1. Summary of the nine samples and of the associated data deposited in the ENA database (European Nucleotide Archive, Study Accession ID PRJEB51203) in terms of the number of raw and trimmed reads per sample.
We sequenced the transcriptome of the brain tissue. For each individual, the head was cut and the brain was quickly dissected (~10 min). The whole brain was used, from the olfactory bulbs to the caudal hindbrain, excluding the spinal cord; cranial nerves and glands were removed. The brain tissue was immediately transferred into RNAprotect Tissue Reagent (Qiagen) and then stored at−20°C until RNA extraction. The RNeasy Plus Kit (Qiagen) was used to extract total RNA, following the manufacturer's instructions. RNA quality and concentration were evaluated using an Agilent Cary60 UV-vis and a Bioanalyzer Agilent 2100 (Agilent Technologies, Santa Clara, CA, USA).
Library preparation and RNA sequencing were performed using NOVOGENE (UK) COMPANY LIMITED, using the Illumina NovaSeq platform. Library construction was carried out using the NEBNext Ultra RNA Library Prep Kit for Illumina, according to the manufacturer's instructions. Briefly, mRNA present in the total RNA sample was isolated using magnetic beads made of oligo d(T)25 (polyA-tailed mRNA enrichment). Subsequently, mRNA was randomly fragmented, and cDNA was synthesized using random hexamers and reverse transcriptase. Once the synthesis of the first chain was completed, the second chain was synthesized with the addition of Illumina buffer, dNTPs, RNase H, and polymerase I of Escherichia coli using the Nick translation method. The resulting products were purified, repaired, A-tailed, and subjected to adapter ligation. Subsequently, fragments of appropriate size were enriched by PCR, indexed P5 and P7 primers were introduced, and the final products were purified.
The libraries were sequenced using the Illumina Novaseq6000 sequencing system using a paired-end 150bp (PE150) strategy. An average of 54.78 million reads were obtained for each library. Sequencing data are available at the European Nucleotide Archive (ENA, study ID PRJEB51203).
Data processing and transcriptome analyses
High-quality RNA-Seq sequence reads were used as the starting input for de novo transcriptome assembly. All described bioinformatics procedures were performed on the high-performance computing system provided by ELIXIR-IT HPC@CINECA (Castrignanò et al., 2020). As a first step in the raw data analysis, adapters and low-quality sequences were removed using Trimmomatic (v. 0.39) (Bolger et al., 2014) with predefined parameters. After the removal of the low-quality reads, 456,838,788 clean reads (93% of raw reads) were maintained for building the de novo transcriptome assembly. A summary of the sequencing statistics (total number of reads before and after trimming) is presented in Table 1. The FastQC tool (https://www.bioinformatics.babra-ham.ac.uk/projects/fastqc/) was used to evaluate RNA-Seq read quality before and after trimming. The FastQC results were gathered by launching MultiQC (Ewels et al., 2016) and are presented in Supplementary Figure 1. As there is no reference genome for H. sarda, two workflows were examined to choose the optimal transcriptome assembly strategy (Supplementary Figure 2). To construct an optimized de novo transcriptome to avoid chimeric transcripts, we adopted a strategy to launch two de novo assembly tools using a multi-kmer approach. The two assemblers, Trinity (trinityrnaseq-v2.11.0) and SPAdes (version 3.11.1), used in rnaSPAdes mode (Bushmanova et al., 2019), were launched to improve the reliability of the final assembly. The results of these analyses using both assemblers are shown in Supplementary Table 1. The outputs obtained by the two assemblers were merged by launching Trans-AbySS (v. 2.0.1) (Jackman et al., 2017) to provide the best contigs. They were then subjected to a validation step (see “Transcriptome Quality Check” in Supplementary Figure 2), using both Denotate (v. 1.11) (Li et al., 2014) and TransRate (v. 1.0.3) (Smith-Unna et al., 2016). To assess the sources of error in the assembly procedure, several metrics produced by these tools serve as guides for estimating the quality of the assembled transcriptome. TransRate is an assembly validation software based on four quality metrics based on alignments to obtain an overall quality criterion applied to each contig individually and for the complete set of contigs. BUSCO, Benchmarking Universal Single-Copy Orthologs (Simão et al., 2015), calculates the gene content based on the evolutionary expectations mined from the near-universal, ultra-conserved eukaryotic proteins database (eukaryota_odb9). This estimation was used to build a quantitative measure of transcriptome quality and completeness (Supplementary Table 1). The outputs obtained after the validation was merged with Trans-ABySS (v2.0.1). After, the assembly result was the input for the CD-HIT-est program (Fu et al., 2012) v4.8.1, a hierarchical clustering tool used to avoid redundant or fragmented transcripts and provide unique contigs, with default parameters corresponding to a similarity of 95%. CD-HIT-est sorts contigs by length and removes contigs beyond a selected identity and coverage threshold. The results are presented in Supplementary Table 1. TransDecoder (Tang et al., 2015), a current standard tool for detecting long open read frames (ORFs) in nucleotide sequences, was run using predefined parameters on the CD-HIT-est output file. By default, TransDecoder predicts ORFs on both strands of assembled contigs. By controlling several rules (such as ORFs completeness, the incompleteness of the 5 'end, and looking for any length of peptides beginning with a start codon (M) and ending without a stop codon), TransDecoder uses BLAST to align the ORFs to the UniProt database for homology annotation with known proteins and searches in the Pfam-A database for protein signatures. In the last step, the database information was also used from the program to predict the coding sequences.
Transcriptome annotation
All downstream analyses included homology and functional annotations. The predicted Open Reading Frames (ORF) were aligned with DIAMOND (calling the function blastx and blatp, respectively) on the three databases, NCBI NR, Swiss-Prot and TrEMBL, to retrieve the corresponding annotations (Buchfink et al., 2015). Six annotation output files were uploaded on figshare (https://figshare.com/articles/online_resource/Annotation_HS_/17005198). Parsing the analysis results of BLASTX against NCBI Nr, Swiss-Prot, and TrEMBL, we obtained, respectively: 179944 sequences (46.99%), 133455 (34.85%), and 179978 (47.00%). The results obtained following the analysis with blastp against NCBI Nr, Swiss-Prot, and TrEMBL are 128401 (33.53%), 88996 (23.24%), and 130165 (33.99%). Information on the homology annotation results for the three databases is presented in Supplementary Table 2. A Venn diagram was created to show the redundancy of the annotations in different databases. The diagrams were constructed for both Diamond blastx (Supplementary Figure 3A) and Diamond blastp (Supplementary Figure 3B) results, showing 132635 and 88671 unigenes commonly annotated from the three databases. To provide an up-to-date exhaustive homology annotation file accessing more detailed information on the best hits, we employed Uniprot API “https://www.uniprot.org/help/api_queries” to mine information about the protein IDs matching with the predicted ORF in Trembl database. Entrez Bio-python libraries (v. 1.79) (https://biopython.org/docs/1.79/api/Bio.Entrez.html) were used to obtain more information about the protein IDs matching the predicted ORF in both the Nr and SwissProt databases. The result of the queries was stored in a file (in xlsx format) available in figshare (Hylasarda_bestHits_final_results.xlsx: https://figshare.com/articles/online_resource/Annotation_HS_/17005198?file=36810237) and contained more detailed information than the diamond output for matched proteins: database, locus name, sequence description, sequence length, division, gene name, organism, protein name, and product name.
In the second step, the predicted ORF was also processed using InterProScan (Hunter et al., 2009) to scan InterProScan signatures. The “signatures” or predictive models of protein domains, families, and functional sites, retrieved from several resources, are combined in the InterPro database (http://www.ebi.ac.uk/interpro/). The InterProScan output results are also available in figshare (https://figshare.com/articles/online_resource/Annotation_HS_/17005198).
Technical validation
In Figure 1, the values of “raw reads”, “paired reads-after quality trimming”, and “trimmed paired-reads” mapped against H. sarda de novo transcriptome are expressed as million and always higher than 90%.
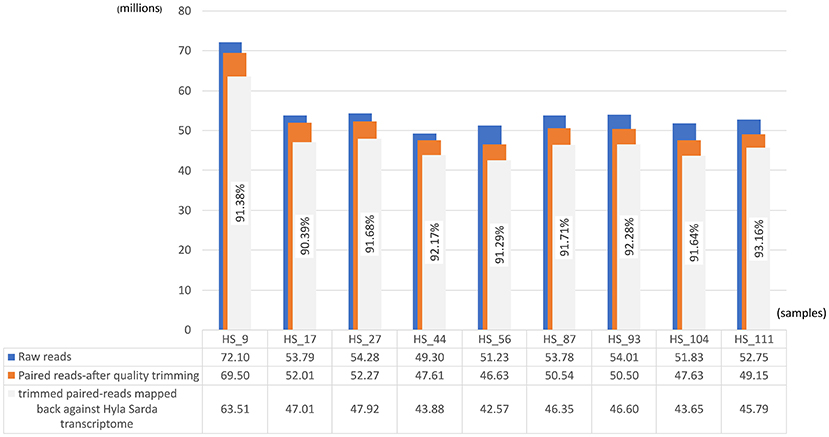
Figure 1. Paired-reads mapped back against the Hyla sarda de novo transcriptome. For each sample, we have in blue, the representation of total paired-reads, in orange, the total paired-reads after the adapter removal and quality trimming, and in gray we have the trimmed paired-reads mapped back against the Hyla sarda assembled de novo transcriptome.
The TransRate assessment showed high values for the “TransRate Optimal Score” item, remaining around 0.09. The value of “good contigs” decreased after CD-HIT-est (due to redundancy removal), but with a value of 0.85 for the final assembly. TransRate also reported a value of GC of approximately 44%. The final assembled transcriptome included 1295741 transcripts with an N50 of 914 bp.
The BUSCO assessment also showed a high value for the “Complete and single-copy” equal to 124 (48.6%) after the CD-HIT-est processing. As shown in Supplementary Table 1, CD-HIT-est improved the assembled transcriptome (“Complete and single-copy” higher than single rnaSPAdes and Trinity de-novo assemblies; see Supplementary Table 1), removing redundancy, reducing the number of transcripts generated by the two assemblers, and improving the quality scores.
The transcriptome was functionally annotated using the DIAMOND and InterProScan software. The six annotation files produced by Diamond provided 88671 and 132635 contigs annotated in at least one of the three databases.
InterProScan integrates different protein signature recognition methods retrieved from the InterPro member databases into a unique resource. As a result, it produced several accession numbers: the corresponding InterPro and among other accession IDs, the GO and KEGG annotations.
Code availability
All software programs used in this study (de novo transcriptome assembly and annotation steps) are listed in the Methods section. When no details on the parameters were available, the programs were used with default settings.
Data availability statement
All datasets generated in this project were stored in the European Nucleotide Archive (ENA) under project accession number PRJEB51203 (ENA accessions are listed in Table 1). Datasets containing the raw Trinity and rnaSPAdes transcriptome assemblies, unigenes, and functional annotation files were uploaded to the figshare archive (https://figshare.com/; links to pipeline outcomes are listed in Supplementary Table 3).
Ethics statement
The animal study was reviewed and approved by Ethical Committee of the University of Tuscia.
Author contributions
DC conceived and financed the study. DC, AC, and RB designed the experiment. AC, RB, and GS performed sample collection and preparation. AC coordinated the RNA extraction and sequencing. TC designed and coordinated the bioinformatic analysis. PL and TC performed read quality assessment, read alignment on the transcriptome, transcriptome annotation, and validation. PL, TC, RB, and AC drafted the manuscript. All authors reviewed and approved the manuscript.
Funding
This study was supported by grants from the Italian Ministry for Education, University and Research (Prin project: 2017KLZ3MA; PI: DC) and by ELIXIR-ITA HPC@CINECA [call ELIXIR-ITA CINECA (2020–2021)], Project name: ELIX4_chiocchi (PI: AC), and Project name: ELIX4_castrign2 (PI: TC).
Acknowledgments
We are grateful to Michela Paoletti for her support during laboratory procedures and Jessica Di Martino for bioinformatics analysis.
Conflict of interest
The authors declare that the research was conducted in the absence of any commercial or financial relationships that could be construed as a potential conflict of interest.
Publisher's note
All claims expressed in this article are solely those of the authors and do not necessarily represent those of their affiliated organizations, or those of the publisher, the editors and the reviewers. Any product that may be evaluated in this article, or claim that may be made by its manufacturer, is not guaranteed or endorsed by the publisher.
Supplementary material
The Supplementary Material for this article can be found online at: https://www.frontiersin.org/articles/10.3389/fevo.2022.947186/full#supplementary-material
References
Bell, A. M., Bukhari, S. A., and Sanogo, Y. O. (2016). Natural variation in brain gene expression profiles of aggressive and nonaggressive individual sticklebacks. Behaviour 153, 1723–1743. doi: 10.1163/1568539X-00003393
Bendesky, A., and Bargmann, C. I. (2011). Genetic contributions to behavioural diversity at the gene–environment interface. Nat. Rev. Genet. 12, 809–820. doi: 10.1038/nrg3065
Bisconti, R., Canestrelli, D., Colangelo, P., and Nascetti, G. (2011a). Multiple lines of evidence for demographic and range expansion of a temperate species (Hyla sarda) during the last glaciation. Mol. Ecol. 20, 5313–5327. doi: 10.1111/j.1365-294X.2011.05363.x
Bisconti, R., Canestrelli, D., and Nascetti, G. (2011b). Genetic diversity and evolutionary history of the Tyrrhenian treefrog Hyla sarda (Anura: Hylidae): adding pieces to the puzzle of Corsica–Sardinia biota. Biol. J. Linnean Soc. 103, 159–167. doi: 10.1111/j.1095-8312.2011.01643.x
Bisconti, R., Carere, C., Costantini, D., Liparoto, A., Chiocchio, A., Canestrelli, D., et al. (2022). Evolution of personality and locomotory performance traits during a late Pleistocene island colonization in a treefrog. Curr. Zool. zoac062. doi: 10.1101/2022.05.15.491936
Bolger, A. M., Lohse, M., and Usadel, B. (2014). Trimmomatic: a flexible trimmer for Illumina sequence data. Bioinformatics 30, 2114–2120. doi: 10.1093/bioinformatics/btu170
Buchfink, B., Xie, C., and Huson, D. H. (2015). Fast and sensitive protein alignment using DIAMOND. Nat. Methods 12, 59–60. doi: 10.1038/nmeth.3176
Bushmanova, E., Antipov, D., Lapidus, A., and Prjibelski, A. D. (2019). rnaSPAdes: a de novo transcriptome assembler and its application to RNA-Seq data. GigaScience 8, giz100. doi: 10.1093/gigascience/giz100
Canestrelli, D., Bisconti, R., and Carere, C. (2016a). Bolder takes all? The behavioral dimension of biogeography. Trends Ecol Evol. 31, 35–43. doi: 10.1016/j.tree.2015.11.004
Canestrelli, D., Bisconti, R., Liparoto, A., Angelier, F., Ribout, C., Carere, C., et al. (2021). Biogeography of telomere dynamics in a vertebrate. Ecography 44, 453–455. doi: 10.1111/ecog.05286
Canestrelli, D., Porretta, D., Lowe, W. H., Bisconti, R., Carere, C., Nascetti, G., et al. (2016b). The tangled evolutionary legacies of range expansion and hybridization. Trends Ecol. Evol. 31, 677–688. doi: 10.1016/j.tree.2016.06.010
Castrignanò, T., Gioiosa, S., Flati, T., Cestari, M., Picardi, E., Chiara, M., et al. (2020). ELIXIR-IT HPC@CINECA: high performance computing resources for the bioinformatics community. BMC Bioinform. 21, 352. doi: 10.1186/s12859-020-03565-8
Clobert, J., Baguette, M., Benton, T. G., and Bullock, J. M. (2012). Dispersal Ecology and Evolution. Oxford: Oxford University Press.
Ewels, P., Magnusson, M., Lundin, S., and Käller, M. (2016). MultiQC: summarize analysis results for multiple tools and samples in a single report. Bioinformatics 32, 3047–3048. doi: 10.1093/bioinformatics/btw354
Fu, L., Niu, B., Zhu, Z., Wu, S., and Li, W. (2012). CD-HIT: accelerated for clustering the next-generation sequencing data. Bioinformatics 28, 3150–3152. doi: 10.1093/bioinformatics/bts565
Harris, R. M., and Hofmann, H. A. (2014). Neurogenomics of behavioral plasticity. Ecol. Genom. 781, 149–168. doi: 10.1007/978-94-007-7347-9_8
Hunter, S., Apweiler, R., Attwood, T. K., Bairoch, A., Bateman, A., Binns, D., et al. (2009). InterPro: the integrative protein signature database. Nucleic |Acids Res. 37, D211–D215. doi: 10.1093/nar/gkn785
Jackman, S. D., Vandervalk, B. P., Mohamadi, H., Chu, J., Yeo, S., Hammond, S. A., et al. (2017). ABySS 2, 0. resource-efficient assembly of large genomes using a Bloom filter. Genome Res. 27, 768–777. doi: 10.1101/gr.214346.116
Kokko, H., and López-Sepulcre, A. (2006). From individual dispersal to species ranges: perspectives for a changing world. Science 313, 789–791. doi: 10.1126/science.1128566
Li, B., Fillmore, N., Bai, Y., Collins, M., Thomson, J. A., Stewart, R., et al. (2014). Evaluation of de novo transcriptome assemblies from RNA-Seq data. Genome Biol. 15, 1–21. doi: 10.1186/s13059-014-0553-5
Liedvogel, M., Åkesson, S., and Bensch, S. (2011). The genetics of migration on the move. Trends Ecol. Evol. 26, 561–569. doi: 10.1016/j.tree.2011.07.009
Lindström, T., Brown, G. P., Sisson, S. A., Phillips, B. L., and Shine, R. (2013). Rapid shifts in dispersal behavior on an expanding range edge. Proc Natl. Acad. Sci. 110, 13452–13456. doi: 10.1073/pnas.1303157110
Liparoto, A. (2020). Behavioural ecology of a late Quaternary range expansion (Doctoral dissertation). Università degli Studi della Tuscia, Viterbo, Italy.
Liparoto, A., Canestrelli, D., Bisconti, R., Carere, C., and Costantini, D. (2020). Biogeographic history moulds population differentiation in ageing of oxidative status in an amphibian. J. Exp. Biol. 223:jeb235002. doi: 10.1242/jeb.235002
Miller, T. E., Angert, A. L., Brown, C. D., Lee-Yaw, J. A., Lewis, M., Lutscher, F., et al. (2020). Eco-evolutionary dynamics of range expansion. Ecology 101, e03139. doi: 10.1002/ecy.3139
Pizzatto, L., Both, C., Brown, G., and Shine, R. (2017). The accelerating invasion: dispersal rates of cane toads at an invasion front compared to an already-colonized location. Evol. Ecol. 31, 533–545. doi: 10.1007/s10682-017-9896-1
Rey, S., Boltana, S., Vargas, R., Roher, N., and MacKenzie, S. (2013). Combining animal personalities with transcriptomics resolves individual variation within a wild-type zebrafish population and identifies underpinning molecular differences in brain function. Mol. Ecol. 22, 6100–6115. doi: 10.1111/mec.12556
Simão, F. A., Waterhouse, R. M., Ioannidis, P., Kriventseva, E. V., and Zdobnov, E. M. (2015). BUSCO: assessing genome assembly and annotation completeness with single-copy orthologs. Bioinformatics 31, 3210–3212. doi: 10.1093/bioinformatics/btv351
Smith-Unna, R., Boursnell, C., Patro, R., Hibberd, J. M., and Kelly, S. (2016). TransRate: reference-free quality assessment of de novo transcriptome assemblies. Genome Res. 26, 1134–1144. doi: 10.1101/gr.196469.115
Spadavecchia, G., Chiocchio, A., Bisconti, R., and Canestrelli, D. (2021). Paso doble: a two-step Late Pleistocene range expansion in the Tyrrhenian tree frog Hyla sarda. Gene 780, 145489. doi: 10.1016/j.gene.2021.145489
Tang, S., Lomsadze, A., and Borodovsky, M. (2015). Identification of protein coding regions in RNA transcripts. Nucleic Acids Res. 43, e78–e78. doi: 10.1093/nar/gkv227
Keywords: bioinformatics and computational biology, behavior, dispersal, de novo transcriptome, phenotypic trait variation, RNA-Seq
Citation: Libro P, Bisconti R, Chiocchio A, Spadavecchia G, Castrignanò T and Canestrelli D (2022) First brain de novo transcriptome of the Tyrrhenian tree frog, Hyla sarda, for the study of dispersal behavior. Front. Ecol. Evol. 10:947186. doi: 10.3389/fevo.2022.947186
Received: 18 May 2022; Accepted: 20 September 2022;
Published: 10 October 2022.
Edited by:
Claudio Casola, Texas A&M University, United StatesReviewed by:
Wenqiao Fan, Chongqing University of Arts and Sciences, ChinaLaurent M. Sachs, Muséum National d'Histoire Naturelle, France
Copyright © 2022 Libro, Bisconti, Chiocchio, Spadavecchia, Castrignanò and Canestrelli. This is an open-access article distributed under the terms of the Creative Commons Attribution License (CC BY). The use, distribution or reproduction in other forums is permitted, provided the original author(s) and the copyright owner(s) are credited and that the original publication in this journal is cited, in accordance with accepted academic practice. No use, distribution or reproduction is permitted which does not comply with these terms.
*Correspondence: Tiziana Castrignanò, dGl6aWFuYS5jYXN0cmlnbmFub0B1bml0dXMuaXQ=