- 1Biology Department, City College of New York, City University of New York, New York, NY, United States
- 2PhD Program in Biology, Graduate Center, City University of New York, New York, NY, United States
- 3McGuire Center for Lepidoptera and Biodiversity, Florida Museum of Natural History, University of Florida, Gainesville, FL, United States
- 4Entomology and Nematology Department, University of Florida, Gainesville, FL, United States
- 5Department of Biology, University of Florida, Gainesville, FL, United States
- 6Department of Organismic and Evolutionary Biology and Museum of Comparative Zoology, Harvard University, Cambridge, MA, United States
- 7Entomology Section, National Museum of Natural History, Manila, Philippines
Next-generation sequencing (NGS) technologies have revolutionized phylogenomics by decreasing the cost and time required to generate sequence data from multiple markers or whole genomes. Further, the fragmented DNA of biological specimens collected decades ago can be sequenced with NGS, reducing the need for collecting fresh specimens. Sequence capture, also known as anchored hybrid enrichment, is a method to produce reduced representation libraries for NGS sequencing. The technique uses single-stranded oligonucleotide probes that hybridize with pre-selected regions of the genome that are sequenced via NGS, culminating in a dataset of numerous orthologous loci from multiple taxa. Phylogenetic analyses using these sequences have the potential to resolve deep and shallow phylogenetic relationships. Identifying the factors that affect sequence capture success could save time, money, and valuable specimens that might be destructively sampled despite low likelihood of sequencing success. We investigated the impacts of specimen age, preservation method, and DNA concentration on sequence capture (number of captured sequences and sequence quality) while accounting for taxonomy and extracted tissue type in a large-scale butterfly phylogenomics project. This project used two probe sets to extract 391 loci or a subset of 13 loci from over 6,000 butterfly specimens. We found that sequence capture is a resilient method capable of amplifying loci in samples of varying age (0–111 years), preservation method (alcohol, papered, pinned), and DNA concentration (0.020 ng/μl - 316 ng/ul). Regression analyses demonstrate that sequence capture is positively correlated with DNA concentration. However, sequence capture and DNA concentration are negatively correlated with sample age and preservation method. Our findings suggest that sequence capture projects should prioritize the use of alcohol-preserved samples younger than 20 years old when available. In the absence of such specimens, dried samples of any age can yield sequence data, albeit with returns that diminish with increasing age.
Introduction
Next-generation sequencing (NGS) has revolutionized phylogenomics by drastically decreasing the cost and time required to generate large datasets of genome-wide genetic markers. However, while NGS technologies were developed to sequence whole genomes, entire assemblies are generally not preferred for systematics because the surfeit of data is unwieldy. Data files are large, requiring high performance computer clusters and much time for bioinformatics and phylogenetic analysis. In addition, gene duplication and chromosomal arrangements complicate assessment of homology between species and make alignment of whole assemblies difficult (Armstrong et al., 2019). Low-coverage whole genome sequencing is an alternative to traditional high-coverage genome sequencing that shows promise for use in phylogenomics and population genetics (Zhang et al., 2019a; Lou et al., 2021). This method can be used in both model and non-model organisms and for species with relatively small genomes it can be a powerful and cost-effective approach (Zhang et al., 2019b). Low-coverage whole genome sequencing has been used to study evolution of the butterfly family Papilionidae by extracting loci with BLAST-based orthology searches (Allio et al., 2020). There is also potential for combining low-coverage whole genome data with other methods to increase genetic and taxonomic sampling in phylogenetic studies (Ribeiro et al., 2021; Talavera et al., 2021). Despite this, low-coverage whole genome sequencing still retains some limitations of whole genome sequencing, including dependency on existing reference genomes and genomic resources. To overcome these limitations, several reduced representation methods have been developed to target and sequence only homologous loci (Davey et al., 2011). These methods still require high performance computers, but the computational power needed is lower than for assembly of whole genomes. The most common reduced representation methods used in phylogenetics might be divided into three categories: enzymatic digestion methods such as RADseq (Baird et al., 2008); sequence capture including capture and sequencing of ultraconserved elements (UCEs; Faircloth et al., 2012; McCormack et al., 2012), which targets a specific category of genomic areas; and transcriptomics. Transcriptomes, another source of genome-wide markers from protein-coding genes that can be used for phylogenomic reconstruction (Grabherr et al., 2011; Kawahara and Breinholt, 2014; Kawahara et al., 2019). There are costs and benefits of each method (Table 1).

Table 1. Advantages and disadvantages of several reduced representation methods for obtaining phylogenomic datasets.
Reduced representation methods
Complete taxon sampling is desirable to provide accurate estimates of diversification through time and other questions in macroecology and evolution (Morlon et al., 2011; Jetz et al., 2012). Increased taxon sampling also increases the accuracy of phylogenetic inference by breaking up long branches and minimizing the effects of coalescent stochasticity (Zwickl and Hillis, 2002; Huang et al., 2010). Comprehensive phylogenetic studies that aim to include samples from all described taxa within a group or samples from a geographically broad area are frequently hampered by lack of samples with high quality DNA. Many species are rare, have limited geographic distributions, are protected from collecting by legislation, or live in a part of the world where research permission is difficult to obtain (Rabinowitz, 1981; Prathapan et al., 2018; Wells et al., 2019). Thus, more comprehensive sampling can be achieved by incorporating existing genetic data, such as DNA barcodes or other Sanger data. These pre-existing data cannot usually be combined with UCEs or RADseq data because they rarely have any homologous loci in common (Table 1; Harvey et al., 2016; Toussaint et al., 2021c). However, loci with ample pre-existing data can be targeted by sequence capture. In addition, DNA can be sequenced from museum or herbarium specimens that were not collected specifically for genetic research (Bi et al., 2013; Staats et al., 2013). Following recent usage, we refer to DNA extracted from such specimens as historical DNA or hDNA (Billerman and Walsh, 2019; Raxworthy and Smith, 2021). Historical DNA is typically degraded and fragmented after years of storage at ambient temperatures. Prior to NGS, specimens collected within a few decades could sometimes yield sequence data by labor-intensive means: designing taxon-specific primers to amplify short, overlapping DNA segments usually under 200 bp (Eastwood and Hughes, 2003; Lohman et al., 2008). Fortuitously, preparation of DNA for short-read NGS requires that it be fragmented into short pieces, so specimens collected in the 20th century frequently yield NGS sequence data.
RADseq and allied methods use enzymes to cut high molecular weight genomic DNA into fragments that are then selected based on their size. If the only sample available for a particular taxon is from a decades-old museum specimen with degraded hDNA, the technique will likely not work because the DNA has already been fragmented randomly over time before digestion with site-specific enzymes. Thus, fragments of a given length may not be homologous among samples, and sequence quality may be poor (Graham et al., 2015). While it is possible to map short NGS reads of hDNA to existing RADseq loci or develop sequence capture probes matching the RAD fragments (Tin et al., 2014; Ali et al., 2016; Hoffberg et al., 2016; Suchan et al., 2016; Lang et al., 2020), these methods are more expensive and complex. In addition, it is difficult to distinguish orthologs from paralogs and assess potential linkage disequilibrium with RADseq data (Rubin et al., 2012).
Both UCEs and target capture can use short-read NGS and are thus amenable to sequencing hDNA from museum specimens (Bailey et al., 2016; Blaimer et al., 2016; McCormack et al., 2016). However, target capture has a few advantages over UCEs: Sanger sequences are available for a greater diversity of species because the techniques have been around longer (Table 1). In addition, the function of UCEs and the evolutionary mechanism for their invariance among distantly related taxa are poorly understood (Dermitzakis et al., 2005; Ahituv et al., 2007). Some researchers are therefore reluctant to apply evolutionary models to stretches of DNA flanking the UCE sites, which may evolve in an atypical fashion. With target capture, loci with known evolutionary rates can be targeted to resolve either deep or shallow relationships (Leaché and Rannala, 2011; Townsend and Leuenberger, 2011; Grover et al., 2012; Hamilton et al., 2016). A possible disadvantage of target capture is the time and money that needs to be invested in identifying target loci and developing probes for them (Faircloth, 2017), but probe sets for numerous taxa already exist (Andermann et al., 2020), or can be designed with the help of software packages including MrBait and others (Chamala et al., 2015; Mayer et al., 2016; Faircloth, 2017; Campana, 2018; Chafin et al., 2018). Thus, target capture is frequently the method of choice for phylogenomics projects, especially those that incorporate hDNA from museum and herbarium samples (Jones and Good, 2016). The method has been used to investigate relationships among many taxa including bats (Bailey et al., 2016), birds (Prum et al., 2015), frogs (Hime et al., 2021), spiders (Hamilton et al., 2016; Wood et al., 2018), harvestmen (Derkarabetian et al., 2019), odonates (Bybee et al., 2021), butterflies (Breinholt et al., 2018; Espeland et al., 2018; Kawahara et al., 2018; Ma et al., 2020), moths (Hamilton et al., 2019; Homziak et al., 2019; Dowdy et al., 2020; Zhang et al., 2020), and a variety of plants (Johnson et al., 2019; Eserman et al., 2021; Acha and Majure, 2022).
Sequence capture: How it works
Sequence capture, also known as target capture, target sequence capture, target enrichment, or anchored hybrid enrichment, is an in vitro process that separates pre-selected loci of interest from other genomic regions (Lemmon et al., 2012). First, genomic regions are selected and single-stranded, oligonucleotide probes complementary to the target sequences are designed using existing genomes (Gnirke et al., 2009). If the probes target exons, the process is sometimes called exon capture (Bragg et al., 2016), and if all of the protein-coding loci in the genome are sequenced, the end result is called an exome. The probes are only ca. 100–200 bp in length, but longer genomic regions can be targeted by overlapping or “tiling” multiple probes to span the desired probe region (Bertone et al., 2006). The success of sequence capture depends on the similarity of the probe sequence to the target sequence, which declines with decreasing relatedness between the taxon used to design the probes and the taxon being enriched. Tiling probes from more than one species’ genome can increase the taxonomic breadth with which the probes can be used.
Probes can be synthesized commercially or be made from the modified PCR products of high-quality genomic DNA (Maricic et al., 2010; Peñalba et al., 2014; Knyshov et al., 2019; Zhang et al., 2019a, 2019b). One advantage of PCR-generated probes is that a reference genome is not required to design the probes, and sequence capture may therefore be used in taxa that lack genomic resources (Jones and Good, 2016). The probes are then biotinylated and combined with streptavidin-coated magnetic beads. Ratios of different probes should be carefully controlled so that sequencing coverage will be equal for all loci, which requires reducing the concentration of probes for organellar DNA in relation to nuclear DNA because it is more abundant in DNA extracts (Peñalba et al., 2014).
To prepare specimens for sequence capture, genomic DNA is extracted from each sample and transmogrified into a “library” by chopping it into short pieces with ultrasound or enzymes, then ligating sequencing adapters and sample-specific indexes (a.k.a. barcodes) to the ends of the DNA fragments (Bronner and Quail, 2019). At this stage, multiple libraries can be multiplexed by combining them and sequencing them together (Meyer and Kircher, 2010). Next, the probes and libraries are combined in a solution hot enough to denature double-stranded library fragments, and the temperature is lowered so that target sequences anneal to their complementary probes. The biotin within the probe then irreversibly binds to the streptavidin on the magnetic beads. A neodymium magnet is placed near the tube, causing the targeted fragments, now bound to the magnetic beads, to adhere to the sides (Paijmans et al., 2015). The fluid is then removed from the tube along with non-target DNA in solution. After a purification step, the tube is re-filled with buffer, heated so the hydrogen bonds binding the target DNA to the probes break, thus releasing the targeted library fragments from the probes and into solution, and—with the magnet still in place—the buffer perfused with DNA fragments from targeted regions is removed and sequenced on a short-read NGS platform such as Illumina. Libraries can be PCR amplified before and/or after the hybridization step. The resulting short reads are bioinformatically demultiplexed, quality-controlled, and assembled.
First, low quality reads and sequence contaminants including adapters are removed. Next, the filtered reads are assembled in one of several ways: de novo, with reference sequences, or via reference-guided assembly (Allen et al., 2017). Paralogs are then removed, and consensus sequences are extracted (Andermann et al., 2020). Several bioinformatic pipelines for assembling short of target loci are available (Faircloth, 2016; Johnson et al., 2016; Allen et al., 2017; Andermann et al., 2018). The final product is a set of homologous sequences for a group of taxa.
Sample preservation and DNA quality
Decades of research have identified best practices for preserving tissues for genetic and other molecular research. The high molecular weight nucleic acids present in the nuclei of living tissues quickly degrade into ever-smaller fragments as the post-mortem interval increases (Ludes et al., 1993; Camacho-Sanchez et al., 2013). When genetic data became more commonplace in evolutionary and systematic studies in the late 1980s, it was apparent that standard methods of specimen preservation, such as pinning insects and preparing vertebrate skins, was not ideal for preserving DNA. Conventional wisdom held that thin insect legs dried quickly and often yielded DNA suitable for PCR, but drying, relaxing, spreading, and re-drying Lepidoptera specimens accelerated DNA fragmentation. Experiments to find the best DNA preservation methods ensued (Arctander, 1988; Pyle and Adams, 1989; Post et al., 1993) and continue to be tested as new preservatives are developed (Dillon et al., 1996; Dawson et al., 1998; Camacho-Sanchez et al., 2013; Moreau et al., 2013). The current consensus affirms that cryopreservation in liquid nitrogen or −80°C storage is the preservation method of choice for animal tissues because it preserves DNA, RNA, and proteins indefinitely if the cold chain remains unbroken (Prendini et al., 2002). However, it is often not feasible to lug a nitrogen vapor shipper into the field, keep it charged with liquid nitrogen, and convince airline staff that the bomb-shaped container is safe to bring on an airplane. Thus, fieldwork-friendly alternatives are required. Comparative studies on vertebrate tissues find that some buffers can preserve RNA and DNA at room temperature for long periods of time (Camacho-Sanchez et al., 2013), while a dimethylsulfoxide-sodium solution works well for marine invertebrates (Dawson et al., 1998). Strong (95–100%) ethanol is the favored preservative for insects (Quicke et al., 1999; King and Porter, 2004; Moreau et al., 2013), and drying specimens quickly using silica gel also works well for preserving insect DNA (Post et al., 1993; Dillon et al., 1996). Other types of alcohol, such as methanol and propanol, are not as effective as ethanol for DNA preservation (Post et al., 1993). Killing insects with ethyl acetate seems to degrade DNA (Dillon et al., 1996), and should therefore be avoided. Since the scaly wings of Lepidoptera would be disfigured if immersed in ethanol, making them difficult to identify, one or both forewing-hindwing pairs are removed and placed in a glassine envelope or coin holder before the body is placed in a tube of ethanol (Supplementary Figure S1; Cho et al., 2016). With their cell walls and enzyme-inhibiting secondary metabolites, preservation conditions differ for plants. Early research suggested that ethanol is a poor preservative of plant DNA (Doyle and Dickson, 1987), and drying leaf tissue rapidly in silica gel is generally the preferred method (Pyle and Adams, 1989; Chase and Hills, 1991).
Sample preservation and sequence capture success
As studies incorporating hDNA become increasingly common (Colella et al., 2020; Toussaint et al., 2021c; Garg et al., 2022), researchers will be faced with decisions regarding sample selection. Should an ethanol-preserved specimen always be extracted if a museum specimen is available? If an irreplaceable specimen is destructively sampled to extract DNA, how likely is sequence capture success? What body parts are most likely to yield high quality DNA? We took advantage of sample metadata collected from a large-scale sequence capture project aimed at investigating the evolutionary history of butterflies to identify relationships among several measures of sequencing success and sample age, preservation method, and extracted tissue type. Our results are summarized to provide a decision tree to aid sample selection. While our results are derived exclusively from butterfly samples, they will apply to other insects and dried specimens stored at ambient temperatures.
Materials and methods
Samples
We analyzed metadata associated with 6,146 butterfly specimens from six families that were subjected to sequence capture for several phylogenetic studies undertaken as part of ButterflyNet (Espeland et al., 2018; Kawahara et al., 2018; Toussaint et al., 2018; Toussaint et al., 2019; Braby et al., 2020; Carvalho et al., 2020; Valencia-Montoya et al., 2021; Toussaint et al., 2021a; Toussaint et al., 2021b; Kawahara et al., 2022). This NSF-funded collaborative network aims to infer the phylogeny of butterflies and aggregate data on species distributions (Pinkert et al., 2022) and traits (Shirey et al., 2022; butterflynet.org). The phylogenomic component of the project used two sequence capture probe sets. The first of these, BUTTERFLY1.0, targets 390 single-copy, protein-coding nuclear loci and a single mitochondrial locus: the DNA barcoding fragment of cytochrome c oxidase I (COI; Breinholt et al., 2018; Espeland et al., 2018). We refer to this as the “391-locus probe set”. We aimed to sequence at least one species from each of the ca. 1900 valid butterfly genera (Lamas, 2015) with the BUTTERFLY1.0 probe set (Kawahara et al., 2022); the type species of each genus was sequenced if available. Sequences from the remaining specimens were captured with the BUTTERFLY2.0 probe set (Kawahara et al., 2018), which targets 13 loci found in BUTTERFLY1.0 that are often used in butterfly phylogenetics, (Wahlberg and Wheat, 2008) including COI. We call this the “13-locus probe set”.
The 13-locus probe set and the 391-locus probe set have successfully generated data to resolve evolutionary relationships at varying taxonomic levels. The BUTTERFLY 2.0 13-locus dataset has resolved relationships within the family Hedylidae providing robust support for 80% of nodes (Kawahara et al., 2018). Data generated with this probe set has also been used to recover tribal level relationships in the Acraeini (Carvalho et al., 2020), Baorini (Toussaint et al., 2019), and Candalidini (Braby et al., 2020). The larger BUTTERFLY 1.0 probe set has most notably been used in creating comprehensive and dated phylogenies of the superfamily Papilionoidea (butterflies) including 98% of all tribes (Espeland et al., 2018) and 84% of all genera (Kawahara et al., 2022). The loci in this set have also been use to generate phylogenetic backbones for the subtribe Euptychiina (Espeland et al., 2019) and the tribe Eumaeini (Valencia-Montoya et al., 2021). Some studies have even combined both sets to further increase phylogenetic resolution in the subfamily Coeliadinae (Toussaint et al., 2021a, 2021b, 2021c) and in the subfamily Heteropterinae (Toussaint et al., 2021a, 2021b, 2021c). Data generated with these sets also have applications beyond systematics and have been applied to study butterfly phylogenetic diversity (Earl et al., 2021).
We recorded specimen variables that might predict sequencing success: DNA concentration; type of tissue extracted; preservation method; sample age; and family. We refer to these variables as Concentration, Tissue, Preservation, Age, and Family, respectively (Table 2). Values for Preservation were “ethanol” for samples in which wingless bodies were preserved in a tube of 95–100% ethanol specifically for genetic research, “papered” for specimens that were dried with their wings folded and stored in a paper envelope—a common method of preservation in the field, and “pinned” to indicate specimens that had been skewered on a pin and prepared for a dry specimen collection (Supplementary Figure S1). Most pinned samples were likely dried and papered in the field, then relaxed in a sealed, humid container for ca. 3–24 h before being pinned and spread. The length of time between collection and relaxing/spreading/pinning is unknown and likely varies among samples. Pinned and papered specimens were obtained from the Museum of Comparative Zoology at Harvard University, the McGuire Center for Lepidoptera and Biodiversity at the University of Florida, the City College of New York, and the American Museum of Natural History. Pinned and papered specimens are common in museum collections and were not preserved with the intention of using the samples for genetic research (Kassambara, 2020). There were 654 samples sequenced with the 391-locus probe set and 2,645 samples sequenced with the 13-locus probe set that had complete metadata. Thousands of other samples had some but not all metadata. Missing metadata meant that analyses were conducted with different numbers of samples (Table 2).
We used these predictor variables to assess several measures of sequence capture success: DNA concentration (which is a response variable in some analyses); the fragment length of extracted DNA before library preparation; the probe set used; the number of loci captured with each probe set; and the sequence quality (Table 3). Average DNA fragment length after extraction but prior to library preparation was assessed by running ca. 3 μl of each extracted DNA sample on a 2% agarose gel. This index of DNA quality, which we called “Fragmentation,” was scored in a binary manner depending on whether most fragments were greater than or less than 1,000 bp in relation to a standard DNA ladder. After the raw reads for each sample were processed in accordance with uniform quality control measures described below, we assessed sequencing success as the number of loci captured (variable names: LociCaptured13 and LociCaptured391), depending on the probe set (13 or 391) and assessed sequence quality by calculating the number of IUPAC ambiguities in the 657 bp sequence of COI from each specimen (variable name: Quality). This mitochondrial gene is maternally inherited and should be wholly homozygous within a single individual. Any ambiguities therefore represent uncertainty in the assembly associated with poor sequence quality. Ambiguous bases might represent truly heterozygous sites in nuclear genes, but not in mitochondrial genes, which is why we only used COI.
DNA extraction
DNA was extracted with OmniPrep™ Genomic DNA Purification Kits for Tissue.1 Tissue samples were not weighed before extraction. Ethanol preserved specimens were extracted following the methods in Espeland et al. (2018), while papered and pinned specimens were extracted following methods described in St Laurent et al. (2018). Genitalia at the tip of the abdomen were never extracted. If abdominal tissue from a pinned specimen was extracted non-destructively by macerating it in extraction buffer, the distal end of the abdomen was placed in a clear gelatin capsule that was then pierced with the specimen pin (Supplementary Figure S1). DNA extracts were quantified using a Qubit 3 Fluorometer using dsDNA HS and BR Assay kits.2 To minimize sequencing failure, samples with a DNA concentration less than 4 ng/μl were rarely subjected to capture and sequencing, and overly concentrated extracts were often diluted to be less than 150 ng/μl to prevent problems with multiplexing.
Library preparation, target enrichment, and sequencing
Quantified extracts were submitted to RAPiD Genomics3 for library preparation, hybrid enrichment, and sequencing. Libraries were generated by first mechanically shearing DNA to a size of 300 bp. Once sheared, adenine residues were ligated to the 3′ end of the blunt-end fragments to allow for the ligation of barcoded adapters and the PCR-amplification of the library (Breinholt et al., 2018; Espeland et al., 2018; Kawahara et al., 2018). Agilent SureSelect probes4 were then used for solution-based target enrichment of pools containing 16 libraries. Enrichment of these libraries followed the SureSelectXT Target Enrichment System for Illumina Paired-End Multiplexed Sequencing Library protocol (Breinholt et al., 2018; Espeland et al., 2018; Kawahara et al., 2018). These enriched libraries were then multiplexed and sequenced with an Illumina HiSeq 3,000 producing paired-end 100-bp reads (Espeland et al., 2018; Kawahara et al., 2018).
Locus assembly
An existing pipeline for anchored phylogenomics was used to assemble raw Illumina reads (Breinholt et al., 2018). First, paired-end Illumina data were cleaned, and adapters were removed using Trim Galore! 0.4.0.4 Selected reads had a minimum read size of 30 bp and bases with a Phred score above 20 (Breinholt et al., 2018). Loci were then assembled using an iterative baited assembly (IBA) process that used reads with a forward and reverse read that passed prior filtering (Breinholt et al., 2018; Espeland et al., 2018; Kawahara et al., 2018). The assembly process uses the custom python script IBA.py available on Dryad (Breinholt et al., 2017), which uses USEARCH v7.0 (Edgar, 2010) to find raw reads that matches the probe region of the reference taxa. These assembled reads were then filtered using the python script s_hit_checker.py available on Dryad (Breinholt et al., 2017). This script searched assembled reads against a Danaus plexippus reference genome and these results were used for single hit and orthology filtering with a bit score threshold of 0.90 (Breinholt et al., 2018; Espeland et al., 2018; Kawahara et al., 2018). Orthologs were then screened for contamination by identifying and removing sequences that were identical or nearly identical at different taxonomic levels (Breinholt et al., 2018; Espeland et al., 2018; Kawahara et al., 2018).
Statistical analyses
Data were cleaned in the tidyverse (Wickham et al., 2019) and visualized with ggplot (Wickham 2016; Kassambara, 2020). First, we modeled Concentration as a response variable with Age, Preservation, Tissue, and Family as the explanatory variables (Table 2). We considered interactions between Age and Preservation to determine whether Preservation had age-dependent effects on DNA concentration. We log-transformed Concentration and generated generalized linear models (GLM) in R (RStudio Team, 2020; R Core Team, 2021) using the lme4 package (Bates et al., 2015).
Next, we modeled LociCaptured13 and LociCaptured391 (Table 3) with Age, Preservation, and Tissue as explanatory variables (Table 2). Family was initially used as an explanatory variable but was removed from the final model due to its lack of significance. We considered interactions between Age and Preservation to determine whether Preservation had age-dependent effects on locus capture. We generated GLMs in R using the MASS package (Venables and Ripley, 2002) with a quasi-Poisson distribution to model LociCaptured13 and LociCaptured391 while accounting for overdispersion. To determine whether the proportion of loci captured was different between probe sets, we calculated the proportion of loci captured as the ratio of loci captured over the targeted number of loci. We used a nonparametric Kruskal-Wallis test to determine if the proportion of loci captured was significantly different between probe sets.
To understand how sequence capture and concentration varied in relation to age for each combination of Preservation and Tissue, we calculated Spearman rank correlations between LociCaptured13, LociCaptured391 and Concentration versus sample age across the 9 unique combinations of Preservation and Tissue type possible. To explore the relationship between sequence capture and butterfly family we plotted LociCaptured13 and LociCaptured391 versus sample age across the unique combinations of family and preservation method. We also calculated Spearman rank correlations between the numerical variables in our dataset for each probe set, which included combinations of Age:Concentration, Age:LociCaptured, Age:LociCaptured13, Age:LociCaptured391 and Concentration:LociCaptured (Table 3). Spearman rank correlations were calculated in R using the correlation package (Makowski et al., 2020).
To determine whether some Preservation methods or Tissue types led to higher LociCaptured13, higher LociCaptured391, or longer DNA fragment lengths, we used Pearson chi-square tests. We compared the number of ethanol, papered, and pinned samples that failed or succeeded to capture 50% or more of the loci targeted by the probe set, which is how we coded “successful” locus capture. We performed a similar analysis comparing numbers of samples with average DNA Fragment sizes over 1,000 bp vs. under 1,000 bp in relation to their method of Preservation. We then assessed failed vs. successful sequence capture as a function of the Tissue that was extracted: legs, thorax, or abdomen. Since the majority of samples that we analyzed were ethanol samples, we suspected that these might drive the result, so we excluded them and repeated the analysis with data from papered and pinned specimens only
Results
Determinants of DNA concentration
Age, Preservation, Tissue, and Family were significant predictors of DNA Concentration. Additionally, there were significant interactions between Age and Preservation suggesting that Age impacted Concentration differently depending on the Preservation method (Supplementary Figure S2). The concentration of extracted DNA declines with specimen age when data from all sample preservation types are aggregated (ρ = −0.071, p = 3.07e-07; Table 3). Throughout this paper ρ = the Greek letter rho, which is the Spearman rank correlation test statistic, and p, an abbreviation for probability, is the Latin lowercase letter P. However, the effect is only significant in papered (ρ = −0.1, p = 0.00012) and pinned specimens (ρ = −0.26, p = 1e-06), which were not preserved for molecular research (Figure 1A; Supplementary Figure S2). There was no relationship between age and DNA concentration in ethanol preserved tissues (ρ = 0.022, p = 0.37), but the oldest such sample that we included was 26.83 years old because preservation of Lepidoptera in ethanol for genetic research began only around three decades ago. The type of tissue extracted had a strong effect on DNA concentration. For papered and pinned specimens, the rank order from highest to lowest concentration was abdomen > thorax > legs, while for ethanol-preserved specimens, the order was thorax > abdomen > legs (Figure 2A). Within each tissue type, the rank order of DNA concentration was always ethanol > papered > pinned, though the differences were negligible when legs were extracted (Figure 2A).
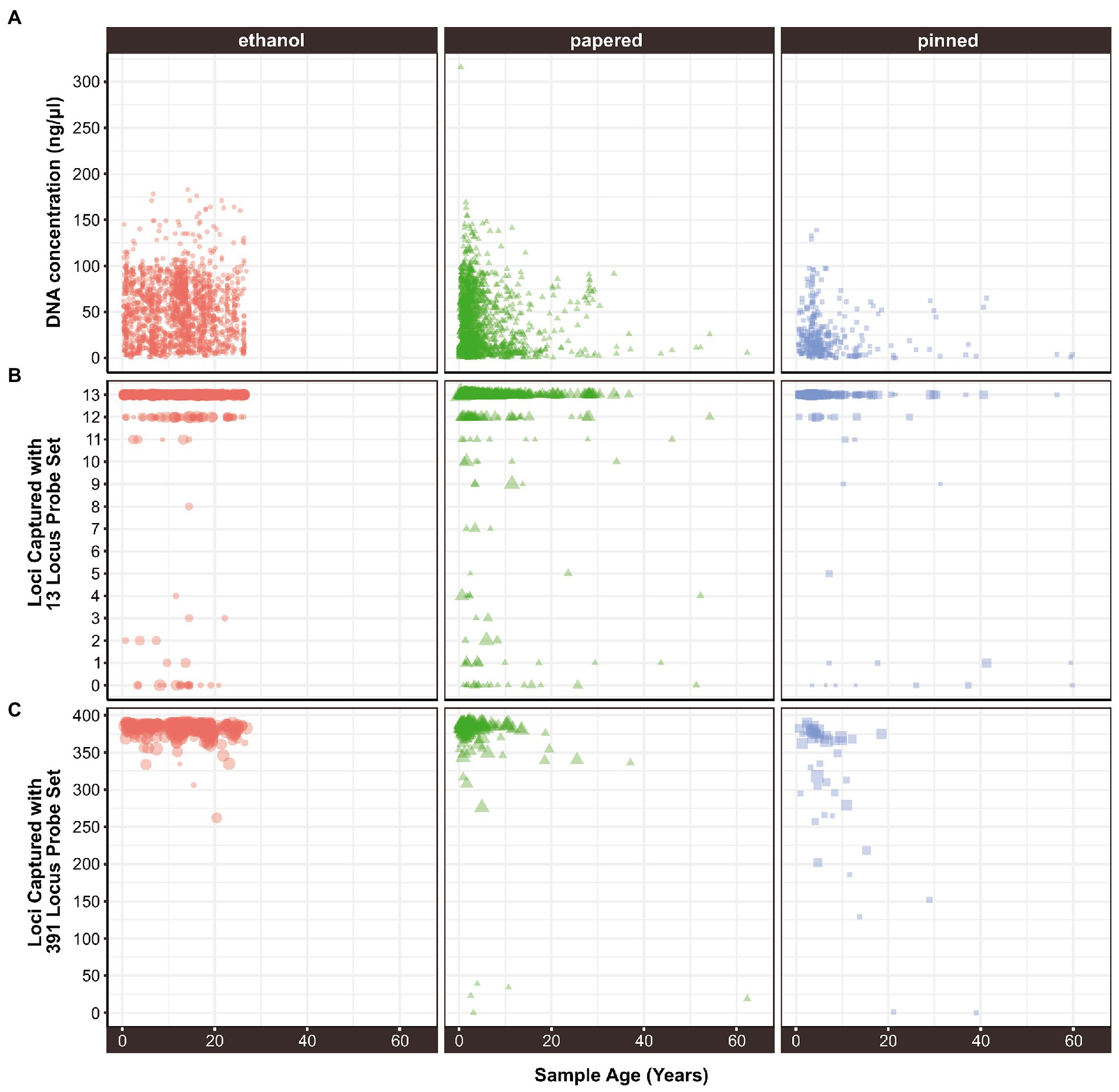
Figure 1. The relationship between sample age and (A) extracted DNA concentration, (B) locus capture with a 13-locus probe set, and (C) locus capture with a 391-locus probe set. In (B,C) the size of each point is proportional to its concentration.
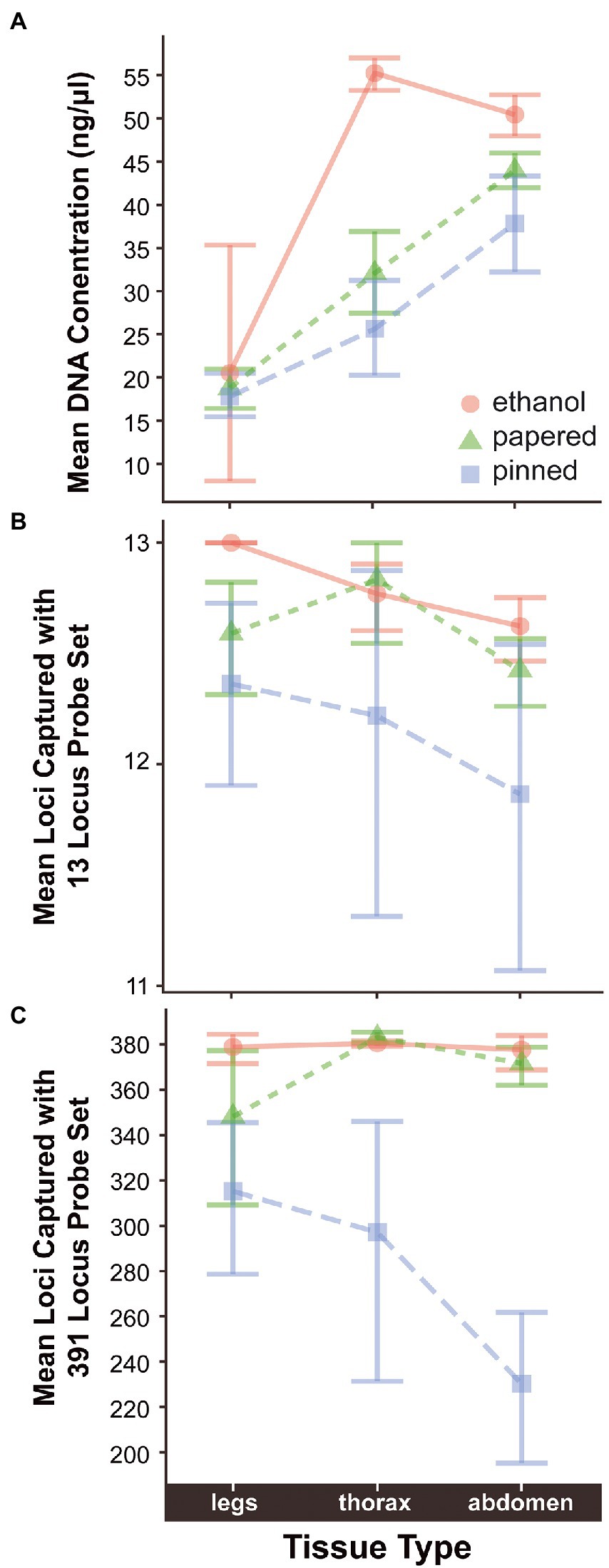
Figure 2. Differences in (A) mean DNA concentration and (B) mean number of loci captured with 13-locus and (C) 391-locus probe sets in relation to preservation method and type of tissue extracted. Jitter has been added to points in (A) so their distribution can be better assessed visually.
DNA fragmentation and sequence quality
Fragment length depends on Preservation method (χ2 = 19.12; p = 7.05E-05). Ethanol-preserved specimens had more samples with fragment lengths over 1,000 bp (93%), followed by papered (72%) and pinned (56%) samples. Ethanol-preserved and papered specimens had significantly more samples with fragment lengths over 1,000 bp than would be expected by chance (p = 5.75E-163 and p = 3.67E-36; Supplementary Figure S3A). Remarkably, there were no significant relationships between age and fragment length in any Preservation method (Figure 3A). Out of 3,586 COI mitochondrial sequences, only 210 (~6%) had at least one ambiguity. The modal number of ambiguities per sequence was 2 (68 samples), and the highest number of ambiguities per sequence was 144. When disaggregated by Preservation method and plotted against sample Age, there were no apparent relationships (Figure 3B).
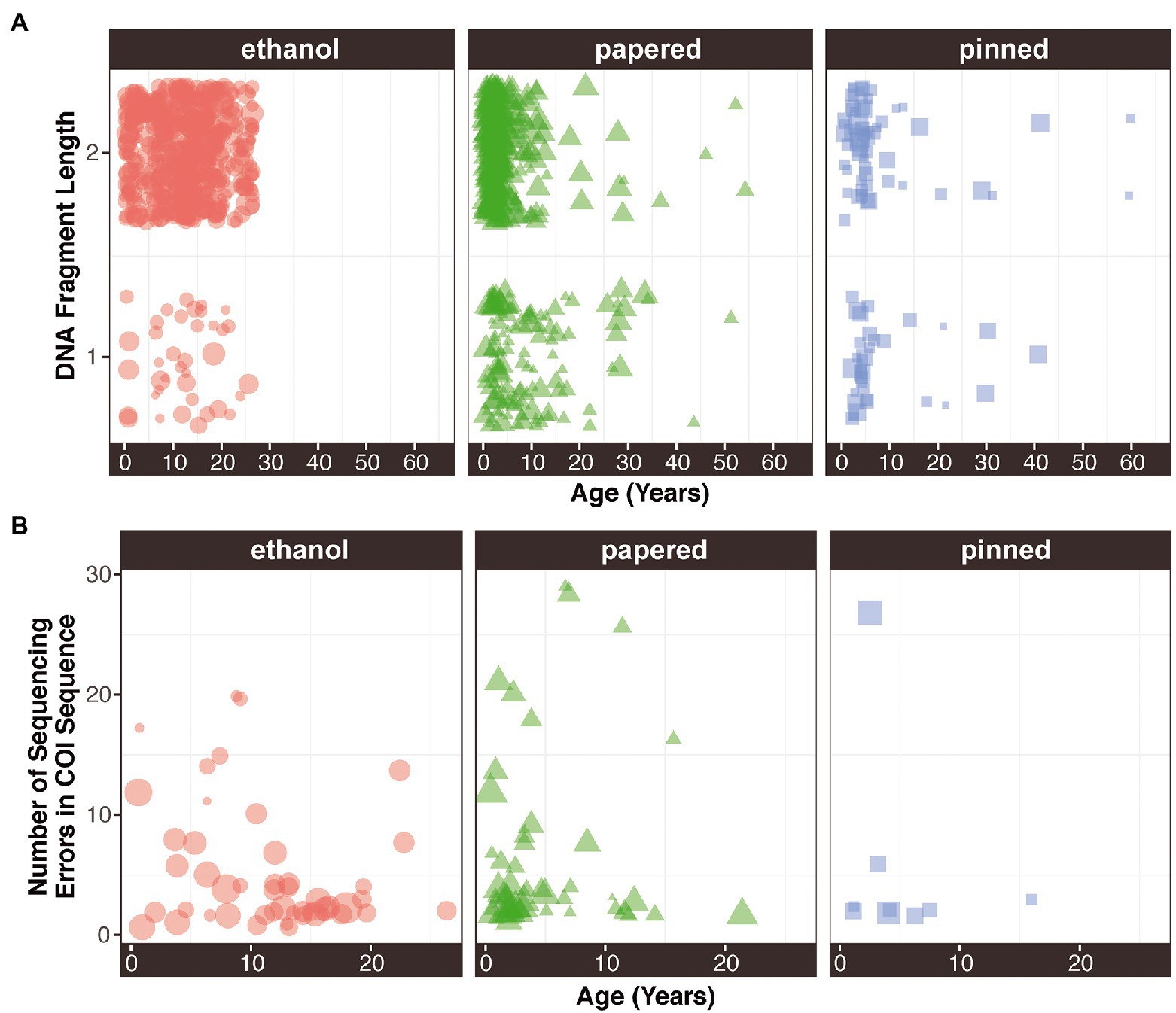
Figure 3. (A) DNA fragment length and (B) sequence quality as a function of age preservation method. The size of each point is proportional to its concentration.
Determinants of sequence capture success
The 13-locus and 391-locus probe sets successfully captured loci from samples of varying Age, Concentration, Preservation, Tissue, and Family. Family had no significant effect on LociCaptured13 or LociCaptured391, but all other variables did. There are no significant relationships between Family and LociCaptured with either ProbeSet or any Preservation method (Supplementary Figures S4, S5). The variable “Family” was therefore removed from the models. Age, Concentration, and Preservation were significant predictors of both LociCaptured13 and LociCaptured391. However, while Tissue was not a significant predictor of LociCaptured13, it was a significant predictor of LociCaptured391. Interactions between Age:Preservation were significant, suggesting that Age impacts locus capture differently depending on the sample preservation method (Supplementary Figures S6, S7). Ethanol preserved specimens have higher average locus capture as Age increases when the other predictors are held constant, followed by papered specimens, and then pinned specimens.
The BUTTERFLY1.0 probe set recovered 100% of 391 targeted loci in some samples, with a mean of 352.68 loci (mode = 385) and the BUTTERFLY2.0 probe set captured a mean of 12.53 loci (median and mode = 13; Table 3). Remarkably, this probe set captured 100% of the 13 targeted loci from the oldest sample in our dataset (111 years). Across all 6,146 samples, we recovered more than 50% of targeted loci in 5879 samples (391-locus probe set = 1888 samples; 13-locus probe set = 3,991 samples), and less than 50% of targeted loci from 267 samples (391-locus probe set = 137 samples; 13-locus probe set = 130 samples), including at least 82 samples that failed to recover any loci (391-locus probe set = 9 samples, 13-locus probe set = 73 samples). The median proportion of locus capture (ratio of loci captured over the number of loci targeted) of the 13-locus probe set was significantly higher than the median proportion of locus capture of the 391-locus probe set (H = 3561.3, df = 1, p = <2.2e–16; Supplementary Figure S8).
LociCaptured13, LociCaptured391, and Concentration are negatively correlated with sample Age, and, while the direction of the correlations is consistent between the probe sets, the strength of the correlations varies (Figures 1B,C; Supplementary Figures S6, S7). The number of loci captured is negatively correlated with Age, and this effect is stronger for the 391-locus probe set (LociCaptured391) than the 13-locus probe set (LociCaptured13; ρ391 = −0.25, p = 8.12E-24; ρ13 = −0.13, p = 9.24E-15; Table 4). There was an exception to this pattern when looking at the unique combinations of Preservation and Tissue: LociCaptured391 was not affected by the Age of papered specimens, as there were several young and old specimens that failed to capture (Supplementary Figure S7). Across all sample tissues and preservation methods, a negative trend between locus capture and age is apparent although not always significant. The strength of the relationship between sample age and loci captured was weak for ethanol-preserved samples (ρ391 = −0.19; p = 3.4e-05; ρ13 = −0.07, p = 0.013), strongest for pinned samples (ρ391 = −0.64; p = 1.2e-06; ρ13 = −0.31, p = 1.4e-06), and intermediate for papered samples (ρ391 = −0.024; p = 0.74; ρ13 = −0.12, p = 3.1e-0.5). Age and LociCaptured for papered and pinned specimens generally had significant negative correlation coefficients (Supplementary Figures S6, S7). Age-dependent capture was strongly affected by tissue type and ProbeSet (Supplementary Figures S6, S7). This trend of decreasing locus capture with age is more clearly seen with both probe sets in pinned samples regardless of Tissue extracted, although the decrease in LociCaptured vs. Age is more apparent in the 391-locus probe set.
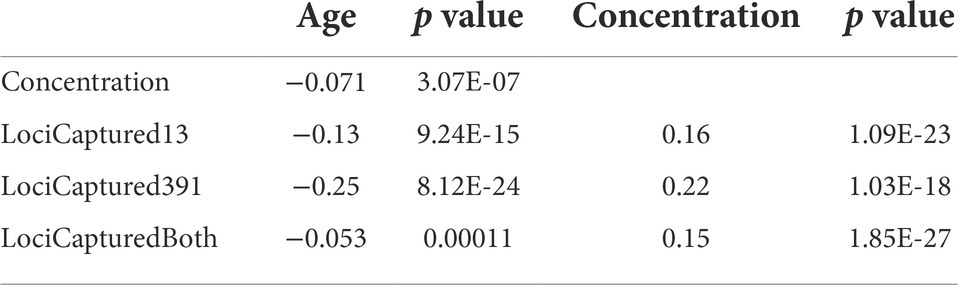
Table 4. Spearman rank correlations between sample age, DNA extract concentration, and the number of loci captured with two probe sets targeting 13 or 391 loci.
LociCaptured is positively correlated with Concentration, and this effect is stronger for the 391-locus than the 13-locus probe set (ρ391 = 0.22, p = 1.03E-18; ρ13 = 0.16, p = 1.09E-23). When including LociCaptured for both probe sets, Age is negatively correlated with LociCaptured (ρ = −0.053, p = 0.00011); Concentration and LociCaptured are positively correlated (ρ = 0.150, p = 1.85E-27; Table 4).
The incidence of sequence capture failure was low, but there was again a clear rank order of success. Ethanol samples had the highest capture rate (98%) followed by papered (96%), then pinned specimens (94%; Supplementary Figure S3B). The type of tissue extracted had a similarly negligible effect on capture success. Extractions from abdominal tissue were most successful (98%), followed by thorax tissue (97%), followed by legs (96%). These values were lower by 1–2% when ethanol samples were excluded from the analysis (Supplementary Figures S3C,D).
Discussion
Sample preservation
Of the three methods we analyzed, immersion in absolute ethanol is the best way to preserve sample DNA for sequencing. If ethanol preserved samples are not available, dry papered specimens generally have better results than pinned specimens. The concentration of DNA extracted from ethanol-preserved specimens did not decline with sample age (Figures 1A, 2A; Supplementary Figure S2), as it did with papered and pinned specimens. The fragment length of extracted DNA was also generally longer (Figure 3A; Supplementary Figure S3A). While this is not crucial for sequence capture, which requires fragmented DNA for short-read sequencing, it is essential for other sequencing platforms such as PacBio HiFi and Oxford Nanopore Technologies long-read sequencing (Whibley et al., 2021; Lawniczak et al., 2022). Thus, preserving samples in ethanol allows them to be used with a broader range of genetic/genomic techniques.
We found no relationship between Preservation type and sequence quality. Although we found a non-significant trend for declining sequence quality with sample age in ethanol-preserved samples but no other sample types (Figure 3B), this might have been an artifact of how we plotted these data. We removed samples with perfect sequence quality (no ambiguities in COI), which comprised most samples, prior to plotting the data. There were thousands more ethanol samples than other sample types (Table 2), so the true impact of age on sequence quality is likely negligible. A greater proportion of loci were captured from ethanol preserved samples than from papered or pinned samples (Table 4; Figures 1B,C, 2B,C). The labs that provided the ethanol-preserved specimens sequenced for this study follow best practices that may improve DNA preservation: 1) Specimens are immersed in 100% ethanol immediately after being killed by pinching the thorax and having their wings removed. No chemical killing agents are used that could compromise DNA quality, and dead specimens are not allowed to air dry (and potentially decay) before ethanol preservation. 2) Several weeks after returning from the field, the ethanol in each tube is discarded and replaced with fresh 100% ethanol. Water in the specimen leaches into the ethanol and dilutes its concentration over time. 3) Ethanol samples are stored in ultracold −80° C freezers.
There are other insect preservation methods not evaluated in this study. For example, we had no access to tissues stored at −140°C in liquid nitrogen vapor. While it is an excellent method for preserving biological molecules, it is impractical to use in many field situations. We extracted ca. ten samples preserved in RNAlater, but these rarely yielded DNA that was sufficiently concentrated for sequencing (>4 ng/μl). These samples were immersed in the preservative immediately after specimens were killed and torn into pieces because aqueous solutions such as RNAlater cannot easily penetrate the hydrophobic cuticle of insects, and thus can fail to preserve tissues suspended in preservative unless the cuticle is ruptured (Evans et al., 2013). In accordance with the manufacturer’s instructions, these specimens were kept as cold as possible in a thermos with ice in the field, and frozen upon return to the lab. There were too few RNAlater preserved specimens to include in our statistical analyses, but we anecdotally conclude that RNAlater is a poor DNA preservative, consistent with the findings of others (Moreau et al., 2013). A study comparing nucleic acid preservation methods for mammal tissues stored at room temperature found that nucleic acid preservation (NAP) buffer was better than 100% ethanol and cryopreservation for preserving DNA and better than RNAlater for preserving RNA after several months of storage (Camacho-Sanchez et al., 2013) at ambient temperatures. Future comparative work should investigate preservation of insect tissues with NAP buffer under ambient conditions, as this buffer has additional advantages of being inexpensive, non-flammable, and stable at ambient temperatures.
Sample age
Sample age has miniscule effects on DNA concentration (Supplementary Figure S2) and sequence capture (Supplementary Figures S6, S7) of ethanol-preserved tissues, regardless of tissue type. The concentration of DNA extracts declines with sample age in papered and pinned specimens, but the type of tissue extracted affects this pattern. The negative correlation is strongest and most significant in abdominal tissues, but weak and not significant (or marginally significant) in extracts from legs or thoraxes. However, extracts from abdomens are generally more concentrated than extracts from other tissues (Supplementary Figure S2). The relationships between Age and LociRecovered13 and LociRecovered391 are significantly negative for pinned specimens, but the relationship is weak for papered specimens (Supplementary Figures S6, S7). In sum, ethanol preserved specimens do not degrade over time, but if one must use papered or pinned specimens, younger specimens yield better results—especially for pinned specimens.
These results bolster results from other research taxa, demonstrating that plant specimens up to 204 years old are amenable to hybrid capture (Brewer et al., 2019). While McGaughran (2020) found that older moth samples have the poorest capture success, Toussaint et al. (2021c) found that sequence coverage was not linked to the age of beetle specimens.
DNA concentration
Hybrid capture requires more DNA than PCR (Chung et al., 2016). While PCR can proceed if there are just a few strands of DNA that are not fragmented between the binding sites of the two primers, the commercial laboratory that we contracted to perform sequence capture and sequencing (see footnote 3) recommends a minimum of ca. 132 ng of DNA per sample (4 ng/μl x 33 μl), though we successfully sequenced samples with less DNA. Since DNA concentration generally decreases with age in pinned and papered specimens (Figure 1A; Supplementary Figure S2), it is best to select the youngest available specimens if there are several of varying ages. The small size of many insects constrains the amount of DNA that can be extracted from them. The amount of DNA that can be extracted is further diminished as papered and pinned specimens age at ambient temperatures (Supplementary Figure S2).
DNA concentration can affect sequence capture below a threshold concentration that is difficult to estimate (perhaps ca. 2–5 ng/μl), but above that, it has a negligible impact on the number of loci captured. We captured 100% of loci from samples with DNA concentrations as low as 0.020 ng/μl and 10.60 ng/μl (13-locus and 391-locus probe sets, respectively), and large numbers of loci were captured with the 391-locus probe set from samples with much lower concentrations, including a sample with a DNA concentration of 2.4 ng/μl that captured 386 loci. These results demonstrate that high sequence capture success can be achieved with surprising small amounts of DNA, albeit not consistently. Conversely, samples with high DNA concentrations do not always guarantee sequence capture. Samples with concentrations of 144 ng/μl and 167 ng/μl failed to recover any loci with the 13-locus and 391-locus probe sets, respectively. Higher DNA concentrations do not guarantee locus capture or higher numbers of captured loci. Further, high DNA concentrations can adversely affect the sequencing depth of other samples multiplexed in the same run by using a disproportionately large number of sequencing reads.
While tissue type is a significant determinant of DNA Concentration, it has little impact on the number of loci captured (Supplementary Figures S6, S7). Therefore, destructively sampling a specimen’s thorax or abdomen only needs to be undertaken when the minimum DNA concentration threshold cannot be met by extracting legs. The value of this threshold will likely depend on the requirements of the PCR hybridization and amplification steps employed in the sequence capture protocol. We used a standard number of PCR cycles during the hybridization step for every sample, but increasing the number of PCR cycles might increase locus capture success of samples with low DNA concentrations. This strategy might increase the likelihood of successful sequence capture of rare or endangered species that can only be obtained as old museum samples.
Degradation
Preservation method seems to be an important determinant of both DNA concentration and locus capture since alcohol preserved specimens had consistently high average concentrations and locus capture regardless of age, while papered and pinned samples had gradual decreases in concentration and loci capture versus sample Age. This is likely due to the ability of different preservation methods to stabilize DNA and prevent degradation.
Short-read next-generation sequencing methods require short fragments of DNA and can sequence DNA from old specimens. Thus, NGS has become a common alternative to PCR and Sanger sequencing, enabling incorporation of museum and herbarium samples in projects that require DNA sequencing (McGaughran, 2020; Mayer et al., 2021; Raxworthy and Smith, 2021). However, severe degradation that produces fragment lengths below the target length of the sequencing method will likely prevent a sample from being captured. The magnitude of these effects depends on the probe length and sequence target length of the library preparation step. Increasing the probe tiling depth and length of the probed region will likely aid capture of degraded samples.
Stochastic variation
We analyzed thousands of samples—one to two orders of magnitude more than similar comparative studies investigating the relationship between sample type and sequencing success (McGaughran, 2020; Mayer et al., 2021). Several samples that were expected to perform well failed to recover many (or any) loci. Given our large sample size, outliers are likely, and may have resulted from unrecorded sample properties that would be important for determining the amount of DNA degradation such as storage temperature, humidity, sample history (specimens shipped as loans, extractions being repeatedly frozen/thawed, extracts kept at ambient temperature for too long, etc.). Additionally, this could also be the result of human or laboratory error. Competition for sequencing within pooled runs could also explain some of this variation, but we did not have information to include that factor in our models.
The sequence quality metadata in this study are a byproduct of multiple phylogenetics studies and many steps were taken to maximize the likelihood of successful locus capture. Therefore, our dataset has a disproportionate number of younger samples, meaning that the smaller number of older samples that happen to have been successful have a strong effect on the relationships that we explore. We excluded no outliers in our analyses. Including old samples that captured successfully sometimes created weakly positive relationships between locus capture and sample age, when this relationship is expected to be negative. However, removal of these outlier samples could erroneously create models that confirm a priori assumptions about locus capture.
Conclusion
Sequence capture is a remarkably resilient method for obtaining sequence data for phylogenomic analysis. We find that DNA from insect specimens stored under less-than-ideal conditions and over a century old can be sequenced successfully. However, success is more likely under certain conditions, and we use our results to provide recommendations for sample selection and preservation (Figure 4). We find higher DNA concentrations are correlated with greater locus capture, but the difference between loci captured is small across samples with low and high concentrations. Sample age is negatively correlated with locus capture, although many or all loci can be captured from older samples. Sample preservation type plays an important role for determining locus capture, with ethanol-preserved samples performing better than papered and pinned samples in our models and correlation analyses. However, samples preserved with any of the methods we investigated can capture a large proportion of targeted loci. The effect that age has on locus capture appears to depend on preservation method, and pinned samples have the steepest decline in locus capture vs. age. By comparing the proportion of loci captured with the number of targeted loci for each probe set, we find that the probe set with fewer targeted loci not only performs better, it also appears to be resistant to decreases in locus capture associated with Age, Concentration, Preservation, and Tissue. We conclude that sequence capture is a robust method that can be used to include historical samples in contemporary phylogenetic and population genetic studies with relatively low risk of failure and marginally diminishing returns when using older and non-ethanol-preserved samples, regardless of the tissue type used for DNA extraction.
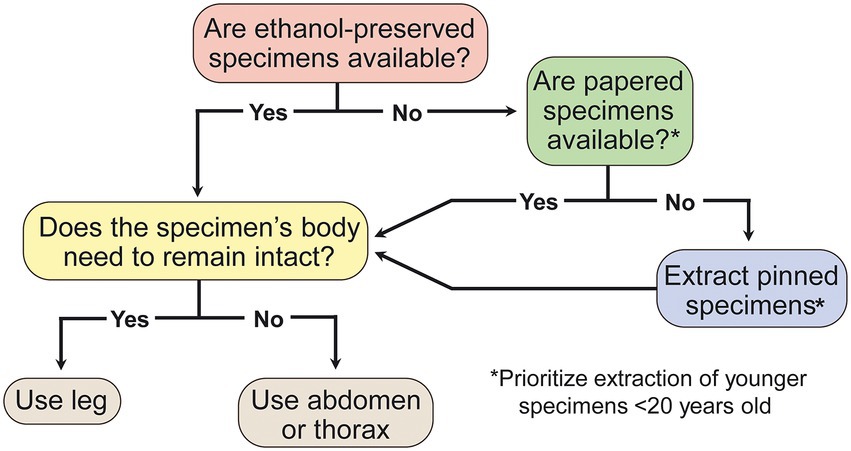
Figure 4. Decision tree for selection of insect specimens most likely to yield optimal sequence capture results.
Data availability statement
Supplementary figures and the dataset analyzed in this paper are provided in the Supplementary material. Further inquiries can be directed to the corresponding author.
Author contributions
DL conceived of this project. RN performed statistical analyses. CS and TD performed lab work. CS undertook bioinformatic analyses of NGS data. AK and NP provided samples for analysis and conceived of the ButterflyNet project with DL. RN and DL wrote the first draft of the manuscript and prepared the figures. All authors contributed to the article and approved the submitted version.
Funding
This project was supported by an NSF GoLife collaborative grant “ButterflyNet”: DEB-1541500 to AK and Robert Guralnick; DEB-1541560 to NP; and DEB-1541557 to DL. Published by a grant from the Wetmore Colles Fund of the Museum of Comparative Zoology.
Acknowledgments
We thank Y-Lan Nguyen and Kelly M. Dexter for performing lab work, and are grateful to David Grimaldi, Andrew D. Warren, Crystal A. Maier, and Rachel L. Hawkins Sipe for facilitating access to specimens under their care. We are grateful for the improvements suggested by two reviewers.
Conflict of interest
The authors declare that the research was conducted in the absence of any commercial or financial relationships that could be construed as a potential conflict of interest.
Publisher’s note
All claims expressed in this article are solely those of the authors and do not necessarily represent those of their affiliated organizations, or those of the publisher, the editors, or the reviewers. Any product that may be evaluated in this article, or claim that may be made by its manufacturer, is not guaranteed or endorsed by the publisher.
Supplementary material
The Supplementary material for this article can be found online at: https://www.frontiersin.org/articles/10.3389/fevo.2022.943361/full#supplementary-material
Footnotes
References
Acha, S., and Majure, L. C. (2022). A new approach using targeted sequence capture for phylogenomic studies across Cactaceae. Genes 13:350. doi: 10.3390/genes13020350
Ahituv, N., Zhu, Y., Visel, A., Holt, A., Afzal, V., Pennacchio, L. A., et al. (2007). Deletion of ultraconserved elements yields viable mice. PLoS Biol. 5:e234. doi: 10.1371/journal.pbio.0050234
Ali, O. A., O’Rourke, S. M., Amish, S. J., Meek, M. H., Luikart, G., Jeffres, C., et al. (2016). RAD capture (rapture): flexible and efficient sequence-based genotyping. Genetics 202, 389–400. doi: 10.1534/genetics.115.183665
Allen, J. M., Boyd, B., Nguyen, N.-P., Vachaspati, P., Warnow, T., Huang, D. I., et al. (2017). Phylogenomics from whole genome sequences using aTRAM. Syst. Biol. 66, syw105–syw798. doi: 10.1093/sysbio/syw105
Allio, R., Scornavacca, C., Nabholz, B., Clamens, A.-L., Sperling, F. A., and Condamine, F. L. (2020). Whole genome shotgun phylogenomics resolves the pattern and timing of swallowtail butterfly evolution. Syst. Biol. 69, 38–60. doi: 10.1093/sysbio/syz030
Andermann, T., Cano, Á., Zizka, A., Bacon, C., and Antonelli, A. (2018). SECAPR—a bioinformatics pipeline for the rapid and user-friendly processing of targeted enriched Illumina sequences, from raw reads to alignments. PeerJ 6:e5175. doi: 10.7717/peerj.5175
Andermann, T., Torres Jiménez, M. F., Matos-Maraví, P., Batista, R., Blanco-Pastor, J. L., Gustafsson, A. L. S., et al. (2020). A guide to carrying out a phylogenomic target sequence capture project. Front. Genet. 10:1407. doi: 10.3389/fgene.2019.01407
Arctander, P. (1988). Comparative studies of avian DNA by restriction fragment length polymorphism analysis: convenient procedures based on blood samples from live birds. J. Ornithol. 129, 205–216. doi: 10.1007/BF01647289
Armstrong, J., Fiddes, I. T., Diekhans, M., and Paten, B. (2019). Whole-genome alignment and comparative annotation. Annu. Rev. Anim. Biosci. 7, 41–64. doi: 10.1146/annurev-animal-020518-115005
Bailey, S. E., Mao, X., Struebig, M., Tsagkogeorga, G., Csorba, G., Heaney, L. R., et al. (2016). The use of museum samples for large-scale sequence capture: a study of congeneric horseshoe bats (family Rhinolophidae). Biol. J. Linn. Soc. 117, 58–70. doi: 10.1111/bij.12620
Baird, N. A., Etter, P. D., Atwood, T. S., Currey, M. C., Shiver, A. L., Lewis, Z. A., et al. (2008). Rapid SNP discovery and genetic mapping using sequenced RAD markers. PLoS One 3:e3376. doi: 10.1371/journal.pone.0003376
Bates, D., Mächler, M., Bolker, B., and Walker, S. (2015). Fitting linear mixed-effects models using lme4. J. Stat. Softw. 67, 1–48. doi: 10.18637/jss.v067.i01
Bertone, P., Trifonov, V., Rozowsky, J. S., Schubert, F., Emanuelsson, O., Karro, J., et al. (2006). Design optimization methods for genomic DNA tiling arrays. Genome Res. 16, 271–281. doi: 10.1101/gr.4452906
Bi, K., Linderoth, T., Vanderpool, D., Good, J. M., Nielsen, R., and Moritz, C. (2013). Unlocking the vault: next-generation museum population genomics. Mol. Ecol. 22, 6018–6032. doi: 10.1111/mec.12516
Billerman, S. M., and Walsh, J. (2019). Historical DNA as a tool to address key questions in avian biology and evolution: a review of methods, challenges, applications, and future directions. Mol. Ecol. Resour. 19, 1115–1130. doi: 10.1111/1755-0998.13066
Blaimer, B. B., Lloyd, M. W., Guillory, W. X., and Brady, S. G. (2016). Sequence capture and phylogenetic utility of genomic ultraconserved elements obtained from pinned insect specimens. PLoS One 11:e0161531. doi: 10.1371/journal.pone.0161531
Braby, M. F., Espeland, M., Müller, C. J., Eastwood, R., Lohman, D. J., Kawahara, A. Y., et al. (2020). Molecular phylogeny of the tribe Candalidini (Lepidoptera: Lycaenidae): systematics, diversification and evolutionary history. Syst. Entomol. 45, 703–722. doi: 10.1111/syen.12432
Bragg, J. G., Potter, S., Bi, K., and Moritz, C. (2016). Exon capture phylogenomics: efficacy across scales of divergence. Mol. Ecol. Resour. 16, 1059–1068. doi: 10.1111/1755-0998.12449
Breinholt, J. W., Earl, C., Lemmon, A. R., Lemmon, E. M., Xiao, L., and Kawahara, A. Y. (2017). Data from: resolving relationships among the megadiverse butterflies and moths with a novel pipeline for anchored phylogenomics. Syst. Biol. 67, 78–93. doi: 10.5061/DRYAD.RF7G5
Breinholt, J. W., Earl, C., Lemmon, A. R., Lemmon, E. M., Xiao, L., and Kawahara, A. Y. (2018). Resolving relationships among the megadiverse butterflies and moths with a novel pipeline for anchored phylogenomics. Syst. Biol. 67, 78–93. doi: 10.1093/sysbio/syx048
Brewer, G. E., Clarkson, J. J., Maurin, O., Zuntini, A. R., Barber, V., Bellot, S., et al. (2019). Factors affecting targeted sequencing of 353 nuclear genes from herbarium specimens spanning the diversity of angiosperms. Front. Plant Sci. 10:1102. doi: 10.3389/fpls.2019.01102
Bronner, I. F., and Quail, M. A. (2019). Best practices for Illumina library preparation. Curr. Protoc. Hum. Genet. 102:e86. doi: 10.1002/cphg.86
Bybee, S. M., Kalkman, V. J., Erickson, R. J., Frandsen, P. B., Breinholt, J. W., Suvorov, A., et al. (2021). Phylogeny and classification of Odonata using targeted genomics. Mol. Phylogenet. Evol. 160:107115. doi: 10.1016/j.ympev.2021.107115
Camacho-Sanchez, M., Burraco, P., Gomez-Mestre, I., and Leonard, J. A. (2013). Preservation of RNA and DNA from mammal samples under field conditions. Mol. Ecol. Resour. 13, 663–673. doi: 10.1111/1755-0998.12108
Campana, M. G. (2018). BaitsTools: software for hybridization capture bait design. Mol. Ecol. Resour. 18, 356–361. doi: 10.1111/1755-0998.12721
Carvalho, A. P. S., St Laurent, R. A., Toussaint, E. F. A., Storer, C., Dexter, K. M., Aduse-Poku, K., et al. (2020). Is sexual conflict a driver of speciation? A case study with a tribe of brush-footed butterflies. Syst. Biol. 70, 413–420. doi: 10.1093/sysbio/syaa070
Chafin, T. K., Douglas, M. R., and Douglas, M. E. (2018). MrBait: universal identification and design of targeted-enrichment capture probes. Bioinformatics 34, 4293–4296. doi: 10.1093/bioinformatics/bty548
Chamala, S., García, N., Godden, G. T., Krishnakumar, V., Jordon-Thaden, I. E., De Smet, R., et al. (2015). MarkerMiner 1.0: a new application for phylogenetic marker development using angiosperm transcriptomes. Appl. Plant Sci. 3:1400115. doi: 10.3732/apps.1400115
Chase, M. W., and Hills, H. H. (1991). Silica gel: an ideal material for field preservation of leaf samples for DNA studies. Taxon 40, 215–220. doi: 10.2307/1222975
Cho, S., Epstein, S. W., Mitter, K., Hamilton, C. A., Plotkin, D., Mitter, C., et al. (2016). Preserving and vouchering butterflies and moths for large-scale museum-based molecular research. PeerJ 4:e2160. doi: 10.7717/peerj.2160
Chung, J., Son, D.-S., Jeon, H.-J., Kim, K.-M., Park, G., Ryu, G. H., et al. (2016). The minimal amount of starting DNA for Agilent’s hybrid capture-based targeted massively parallel sequencing. Sci. Rep. 6:26732. doi: 10.1038/srep26732
Colella, J. P., Tigano, A., and MacManes, M. D. (2020). A linked-read approach to museomics: higher quality de novo genome assemblies from degraded tissues. Mol. Ecol. Resour. 20, 856–870. doi: 10.1111/1755-0998.13155
Davey, J. W., Hohenlohe, P. A., Etter, P. D., Boone, J. Q., Catchen, J. M., and Blaxter, M. L. (2011). Genome-wide genetic marker discovery and genotyping using next-generation sequencing. Nat. Rev. Genet. 12, 499–510. doi: 10.1038/nrg3012
Dawson, M. N., Raskoff, K. A., and Jacobs, D. K. (1998). Field preservation of marine invertebrate tissue for DNA analyses. Mol. Mar. Biol. Biotechnol. 7, 145–152.
Derkarabetian, S., Benavides, L. R., and Giribet, G. (2019). Sequence capture phylogenomics of historical ethanol-preserved museum specimens: unlocking the rest of the vault. Mol. Ecol. Resour. 19, 1531–1544. doi: 10.1111/1755-0998.13072
Dermitzakis, E. T., Reymond, A., and Antonarakis, S. E. (2005). Conserved non-genic sequences — an unexpected feature of mammalian genomes. Nat. Rev. Genet. 6, 151–157. doi: 10.1038/nrg1527
Dillon, N., Austin, A. D., and Bartowsky, E. (1996). Comparison of preservation techniques for DNA extraction from hymenopterous insects. Insect Mol. Biol. 5, 21–24. doi: 10.1111/j.1365-2583.1996.tb00036.x
Dowdy, N. J., Keating, S., Lemmon, A. R., Lemmon, E. M., Conner, W. E., Scott Chialvo, C. H., et al. (2020). A deeper meaning for shallow-level phylogenomic studies: nested anchored hybrid enrichment offers great promise for resolving the tiger moth tree of life (Lepidoptera: Erebidae: Arctiinae). Syst. Entomol. 45, 874–893. doi: 10.1111/syen.12433
Doyle, J. J., and Dickson, E. E. (1987). Preservation of plant samples for DNA restriction endonuclease analysis. Taxon 36, 715–722. doi: 10.2307/1221122
Earl, C., Belitz, M. W., Laffan, S. W., Barve, V., Barve, N., Soltis, D. E., et al. (2021). Spatial phylogenetics of butterflies in relation to environmental drivers and angiosperm diversity across North America. iScience 24:102239. doi: 10.1016/j.isci.2021.102239
Eastwood, R., and Hughes, J. (2003). Molecular phylogeny and evolutionary biology of Acrodipsas (Lepidoptera: Lycaenidae). Mol. Phylogenet. Evol. 27, 93–102. doi: 10.1016/s1055-7903(02)00370-6
Edgar, R. C. (2010). Search and clustering orders of magnitude faster than BLAST. Bioinformatics 26, 2460–2461. doi: 10.1093/bioinformatics/btq461
Eserman, L. A., Thomas, S. K., Coffey, E. E. D., and Leebens-Mack, J. H. (2021). Target sequence capture in orchids: developing a kit to sequence hundreds of single-copy loci. Appl. Plant Sci. 9:e11416. doi: 10.1002/aps3.11416
Espeland, M., Breinholt, J. W., Barbosa, E. P., Casagrande, M. M., Huertas, B., Lamas, G., et al. (2019). Four hundred shades of brown: higher level phylogeny of the problematic Euptychiina (Lepidoptera, Nymphalidae, Satyrinae) based on hybrid enrichment data. Mol. Phyloget. Evol. 131, 116–124. doi: 10.1016/j.ympev.2018.10.039
Espeland, M., Breinholt, J., Willmott, K. R., Warren, A. D., Vila, R., Toussaint, E. F. A., et al. (2018). A comprehensive and dated phylogenomic analysis of butterflies. Curr. Biol. 28, 770–778.e5. doi: 10.1016/j.cub.2018.01.061
Evans, J. D., Schwarz, R. S., Chen, Y. P., Budge, G., Cornman, R. S., De La Rua, P., et al. (2013). Standard methods for molecular research in Apis mellifera. J. Apic. Res. 52, 1–54. doi: 10.3896/ibra.1.52.4.11
Faircloth, B. C. (2016). PHYLUCE is a software package for the analysis of conserved genomic loci. Bioinformatics 32, 786–788. doi: 10.1093/bioinformatics/btv646
Faircloth, B. C. (2017). Identifying conserved genomic elements and designing universal bait sets to enrich them. Methods Ecol. Evol. 8, 1103–1112. doi: 10.1111/2041-210X.12754
Faircloth, B. C., McCormack, J. E., Crawford, N. G., Harvey, M. G., Brumfield, R. T., and Glenn, T. C. (2012). Ultraconserved elements anchor thousands of genetic markers spanning multiple evolutionary timescales. Syst. Biol. 61, 717–726. doi: 10.1093/sysbio/sys004
Garg, K. M., Chattopadhyay, B., Cros, E., Tomassi, S., Benedick, S., Edwards, D. P., et al. (2022). Island biogeography revisited: museomics reveals affinities of shelf island birds determined by bathymetry and paleo-rivers, not by distance to mainland. Mol. Biol. Evol. 39:msab340. doi: 10.1093/molbev/msab340
Gnirke, A., Melnikov, A., Maguire, J., Rogov, P., Leproust, E. M., Brockman, W., et al. (2009). Solution hybrid selection with ultra-long oligonucleotides for massively parallel targeted sequencing. Nat. Biotechnol. 27, 182–189. doi: 10.1038/nbt.1523
Grabherr, M. G., Haas, B. J., Yassour, M., Levin, J., Thompson, D. A., Amit, I., et al. (2011). Full-length transcriptome assembly from RNA-Seq data without a reference genome. Nat. Biotechnol. 29, 644–652. doi: 10.1038/nbt.1883
Graham, C. F., Glenn, T. C., McArthur, A. G., Boreham, D. R., Kieran, T., Lance, S., et al. (2015). Impacts of degraded DNA on restriction enzyme associated DNA sequencing (RADSeq). Mol. Ecol. Resour. 15, 1304–1315. doi: 10.1111/1755-0998.12404
Grover, C. E., Salmon, A., and Wendel, J. F. (2012). Targeted sequence capture as a powerful tool for evolutionary analysis. Am. J. Bot. 99, 312–319. doi: 10.3732/ajb.1100323
Hamilton, C. A., Lemmon, A. R., Lemmon, E. M., and Bond, J. E. (2016). Expanding anchored hybrid enrichment to resolve both deep and shallow relationships within the spider tree of life. BMC Evol. Biol. 16:212. doi: 10.1186/s12862-016-0769-y
Hamilton, C. A., St Laurent, R. A., Dexter, K., Kitching, I. J., Breinholt, J. W., Zwick, A., et al. (2019). Phylogenomics resolves major relationships and reveals significant diversification rate shifts in the evolution of silk moths and relatives. BMC Evol. Biol. 19:182. doi: 10.1186/s12862-019-1505-1
Harvey, M. G., Smith, B. T., Glenn, T. C., Faircloth, B. C., and Brumfield, R. T. (2016). Sequence capture versus restriction site associated DNA sequencing for shallow systematics. Syst. Biol. 65, 910–924. doi: 10.1093/sysbio/syw036
Hime, P. M., Lemmon, A. R., Lemmon, E. C. M., Prendini, E., Brown, J. M., Thomson, R. C., et al. (2021). Phylogenomics reveals ancient gene tree discordance in the amphibian tree of life. Syst. Biol. 70, 49–66. doi: 10.1093/sysbio/syaa034
Hoffberg, S. L., Kieran, T. J., Catchen, J. M., Devault, A., Faircloth, B. C., Mauricio, R., et al. (2016). RADcap: sequence capture of dual-digest RADseq libraries with identifiable duplicates and reduced missing data. Mol. Ecol. Resour. 16, 1264–1278. doi: 10.1111/1755-0998.12566
Homziak, N. T., Breinholt, J. W., Branham, M. A., Storer, C. G., and Kawahara, A. Y. (2019). Anchored hybrid enrichment phylogenomics resolves the backbone of erebine moths. Mol. Phylogenet. Evol. 131, 99–105. doi: 10.1016/j.ympev.2018.10.038
Huang, H., He, Q., Kubatko, L. S., and Knowles, L. L. (2010). Sources of error inherent in species-tree estimation: impact of mutational and coalescent effects on accuracy and implications for choosing among different methods. Syst. Biol. 59, 573–583. doi: 10.1093/sysbio/syq047
Jetz, W., Thomas, G. H., Joy, J. B., Hartmann, K., and Mooers, A. O. (2012). The global diversity of birds in space and time. Nature 491, 444–448. doi: 10.1038/nature11631
Johnson, M. G., Gardner, E. M., Liu, Y., Medina, R., Goffinet, B., Shaw, A. J., et al. (2016). HybPiper: extracting coding sequence and introns for phylogenetics from high-throughput sequencing reads using target enrichment. Appl. Plant Sci. 4:1600016. doi: 10.3732/apps.1600016
Johnson, M. G., Pokorny, L., Dodsworth, S., Botigué, L. R., Cowan, R. S., Devault, A., et al. (2019). A universal probe set for targeted sequencing of 353 nuclear genes from any flowering plant designed using k-medoids clustering. Syst. Biol. 68, 594–606. doi: 10.1093/sysbio/syy086
Jones, M. R., and Good, J. M. (2016). Targeted capture in evolutionary and ecological genomics. Mol. Ecol. 25, 185–202. doi: 10.1111/mec.13304
Kassambara, A. (2020). ggpubr: ‘ggplot2’ bvsed publication ready plots. R package version 0.4.0. Available at: https://CRAN.R-project.org/package=ggpubr (Accessed May 13, 2022).
Kawahara, A. Y., and Breinholt, J. W. (2014). Phylogenomics provides strong evidence for relationships of butterflies and moths. Proc. R. Soc. B 281:20140970. doi: 10.1098/rspb.2014.0970
Kawahara, A. Y., Breinholt, J. W., Espeland, M., Storer, C., Plotkin, D., Dexter, K. M., et al. (2018). Phylogenetics of moth-like butterflies (Papilionoidea: Hedylidae) based on a new 13-locus target capture probe set. Mol. Phylogenet. Evol. 127, 600–605. doi: 10.1016/j.ympev.2018.06.002
Kawahara, A. Y., Plotkin, D., Espeland, M., Meusemann, K., Toussaint, E. F. A., Donath, A., et al. (2019). Phylogenomics reveals the evolutionary timing and pattern of butterflies and moths. Proc. Natl. Acad. Sci. 116, 22657–22663. doi: 10.1073/pnas.1907847116
Kawahara, A. Y., Storer, C., Carvalho, A. P. S., Plotkin, D. M., Condamine, F., Braga, M. P., et al. (2022). Evolution and diversification dynamics of butterflies. BioRxiv. 1–27. doi: 10.1101/2022.05.17.491528
King, J. R., and Porter, S. D. (2004). Recommendations on the use of alcohols for preservation of ant specimens (Hymenoptera, Formicidae). Insect. Soc. 51, 197–202. doi: 10.1007/s00040-003-0709-x
Knyshov, A., Gordon, E. R. L., and Weirauch, C. (2019). Cost-efficient high throughput capture of museum arthropod specimen DNA using PCR-generated baits. Methods Ecol. Evol. 10, 841–852. doi: 10.1111/2041-210x.13169
Lang, P. L. M., Weiß, C. L., Kersten, S., Latorre, S. M., Nagel, S., Nickel, B., et al. (2020). Hybridization ddRAD-sequencing for population genomics of non-model plants using highly degraded historical specimen DNA. Mol. Ecol. Resour. 20, 1228–1247. doi: 10.1111/1755-0998.13168
Lawniczak, M. K. N., Durbin, R., Flicek, P., Lindblad-Toh, K., Wei, X., Archibald, J. M., et al. (2022). Standards recommendations for the earth BioGenome project. Proc. Natl. Acad. Sci. 119:e2115639118. doi: 10.1073/pnas.2115639118
Leaché, A. D., and Rannala, B. (2011). The accuracy of species tree estimation under simulation: a comparison of methods. Syst. Biol. 60, 126–137. doi: 10.1093/sysbio/syq073
Lemmon, A. R., Emme, S. A., and Lemmon, E. M. (2012). Anchored hybrid enrichment for massively high-throughput phylogenomics. Syst. Biol. 61, 727–744. doi: 10.1093/sysbio/sys049
Lohman, D. J., Peggie, D., Pierce, N. E., and Meier, R. (2008). Phylogeography and genetic diversity of a widespread Old World butterfly, Lampides boeticus (Lepidoptera: Lycaenidae). BMC Evol. Biol. 8:301. doi: 10.1186/1471-2148-8-301
Lou, R. N., Jacobs, A., Wilder, A. P., and Therkildsen, N. O. (2021). A beginner’s guide to low-coverage whole genome sequencing for population genomics. Mol. Ecol. 30, 5966–5993. doi: 10.1111/mec.16077
Ludes, B., Pfitzinger, H., and Mangin, P. (1993). DNA fingerprinting from tissues after variable postmortem periods. J. Forensic Sci. 38, 686–690. doi: 10.1520/JFS13456J
Ma, L., Zhang, Y., Lohman, D. J., Wahlberg, N., Ma, F., Nylin, S., et al. (2020). A phylogenomic tree inferred with an inexpensive PCR-generated probe kit resolves higher-level relationships among Neptis butterflies (Nymphalidae: Limenitidinae). Syst. Entomol. 45, 924–934. doi: 10.1111/syen.12435
Makowski, D., Ben-Shachar, M., Patil, I., and Lüdecke, D. (2020). Methods and algorithms for correlation analysis in R. J. Open Source Softw. 5:2306. doi: 10.21105/joss.02306
Maricic, T., Whitten, M., and Pääbo, S. (2010). Multiplexed DNA sequence capture of mitochondrial genomes using PCR products. PLoS One 5:e14004. doi: 10.1371/journal.pone.0014004
Mayer, C., Dietz, L., Call, E., Kukowka, S., Martin, S., and Espeland, M. (2021). Adding leaves to the Lepidoptera tree: capturing hundreds of nuclear genes from old museum specimens. Syst. Entomol. 46, 649–671. doi: 10.1111/syen.12481
Mayer, C., Sann, M., Donath, A., Meixner, M., Podsiadlowski, L., Peters, R. S., et al. (2016). BaitFisher: a software package for multispecies target DNA enrichment probe design. Mol. Biol. Evol. 33, 1875–1886. doi: 10.1093/molbev/msw056
McCormack, J. E., Faircloth, B. C., Crawford, N. G., Gowaty, P. A., Brumfield, B. T., and Glenn, T. C. (2012). Ultraconserved elements are novel phylogenomic markers that resolve placental mammal phylogeny when combined with species tree analysis. Genome Res. 22, 746–754. doi: 10.1101/gr.125864.111
McCormack, J. E., Tsai, W. L. E., and Faircloth, B. C. (2016). Sequence capture of ultraconserved elements from bird museum specimens. Mol. Ecol. Resour. 16, 1189–1203. doi: 10.1111/1755-0998.12466
McGaughran, A. (2020). Effects of sample age on data quality from targeted sequencing of museum specimens: what are we capturing in time? BMC Genomics 21:188. doi: 10.1186/s12864-020-6594-0
Meyer, M., and Kircher, M. (2010). Illumina sequencing library preparation for highly multiplexed target capture and sequencing. Cold Spring Harb. Protoc. 2010:pdb.prot5448. doi: 10.1101/pdb.prot5448
Moreau, C. S., Wray, B. D., Czekanski-Moir, J. E., and Rubin, B. E. R. (2013). DNA preservation: a test of commonly used preservatives for insects. Invertebr. Syst. 27, 81–86. doi: 10.1071/IS12067
Morlon, H., Parsons, T. L., and Plotkin, J. B. (2011). Reconciling molecular phylogenies with the fossil record. Proc. Natl. Acad. Sci. 108, 16327–16332. doi: 10.1073/pnas.1102543108
Paijmans, J. L. A., Fickel, J., Courtiol, A., Hofreiter, M., and Förster, D. W. (2015). Impact of enrichment conditions on cross-species capture of fresh and degraded DNA. Mol. Ecol. Resour. 16, 42–55. doi: 10.1111/1755-0998.12420
Peñalba, J. V., Smith, L. L., Tonione, M. A., Sass, C., Hykin, S. M., Skipwith, P. L., et al. (2014). Sequence capture using PCR-generated probes: a cost-effective method of targeted high-throughput sequencing for nonmodel organisms. Mol. Ecol. Resour. 14, 1000–1010. doi: 10.1111/1755-0998.12249
Pinkert, S., Barve, V., Guralnick, R., and Jetz, W. (2022). Global geographical and latitudinal variation in butterfly species richness captured through a comprehensive country-level occurrence database. Glob. Ecol. Biogeogr. 31, 830–839. doi: 10.1111/geb.13475
Post, R. J., Flook, P. K., and Millest, A. L. (1993). Methods for the preservation of insects for DNA studies. Biochem. Syst. Ecol. 21, 85–92. doi: 10.1016/0305-1978(93)90012-g
Prathapan, K. D., Pethiyagoda, R., Bawa, K. S., Raven, P. H., and Rajan, P. D. (2018). When the cure kills—CBD limits biodiversity research. Science 360, 1405–1406. doi: 10.1126/science.aat9844
Prendini, L., Hanner, R., and Desalle, R. (2002). “Obtaining, storing and archiving specimens and tissue samples for use in molecular studies,” in Techniques in molecular systematics and evolution (Basel: Birkhäuser), 176–248.
Prum, R. O., Berv, J. S., Dornburg, A., Field, D. J., Townsend, J. P., Lemmon, E. M., et al. (2015). A comprehensive phylogeny of birds (Aves) using targeted next-generation DNA sequencing. Nature 526, 569–573. doi: 10.1038/nature15697
Pyle, M. M., and Adams, R. P. (1989). In situ preservation of DNA in plant specimens. Taxon 38, 576–581. doi: 10.2307/1222632
Quicke, D. L. J., Belshaw, R., and Lopez-Vaamonde, C. (1999). Preservation of hymenopteran specimens for subsequent molecular and morphological study. Zool. Scr. 28, 261–267. doi: 10.1046/j.1463-6409.1999.00004.x
R Core Team (2021). R: A language and environment for statistical computing. R foundation for statistical computing, Vienna, Austria. Available at: https://www.R-project.org (Accessed May 13, 2022).
RStudio Team (2020). RStudio: Integrated development for R. PBC, Boston, MA: RStudio. Available at: http://www.rstudio.com/. (Accessed May 13, 2022).
Rabinowitz, D. (1981). “Seven forms of rarity,” in The biological aspects of rare plant conservation. ed. H. Synge (Chichester: John Wiley & Sons Ltd.), 205–217.
Raxworthy, C. J., and Smith, B. T. (2021). Mining museums for historical DNA: advances and challenges in museomics. Trends Ecol. Evol. 36, 1049–1060. doi: 10.1016/j.tree.2021.07.009
Ribeiro, P., Torres Jiménez, M. F., Andermann, T., Antonelli, A., Bacon, C. D., and Matos-Maraví, P. (2021). A bioinformatic platform to integrate target capture and whole genome sequences of various read depths for phylogenomics. Mol. Ecol. 30, 6021–6035. doi: 10.1111/mec.16240
Rubin, B. E. R., Ree, R. H., and Moreau, C. S. (2012). Inferring phylogenies from RAD sequence data. PLoS One 7:e33394. doi: 10.1371/journal.pone.0033394
Shirey, V., Larsen, E., Doherty, A., Kim, C. A., Al-Sulaiman, F. T., Hinolan, J. D., et al. (2022). LepTraits 1.0: a globally comprehensive dataset of butterfly traits. Sci. Data 9:382. doi: 10.1038/s41597-022-01473-5
St Laurent, R. A., Hamilton, C. A., and Kawahara, A. Y. (2018). Museum specimens provide phylogenomic data to resolve relationships of sack-bearer moths (Lepidoptera, Mimallonoidea, Mimallonidae). Syst. Entomol. 43, 729–761. doi: 10.1111/syen.12301
Staats, M., Erkens, R. H. J., van de Vossenberg, B., Wieringa, J. J., Kraaijeveld, K., Stielow, B., et al. (2013). Genomic treasure troves: complete genome sequencing of herbarium and insect museum specimens. PLoS One 8:e69189. doi: 10.1371/journal.pone.0069189
Suchan, T., Pitteloud, C., Gerasimova, N. S., Kostikova, A., Schmid, S., Arrigo, N., et al. (2016). Hybridization capture using RAD probes (hyRAD), a new tool for performing genomic analyses on collection specimens. PLoS One 11:e0151651. doi: 10.1371/journal.pone.0151651
Talavera, G., Lukhtanov, V., Pierce, N. E., and Vila, R. (2021). DNA barcodes combined with multi-locus data of representative taxa can generate reliable higher-level phylogenies. Syst. Biol. 71, 382–395. doi: 10.1093/sysbio/syab038
Tin, M. M.-Y., Economo, E. P., and Mikheyev, A. S. (2014). Sequencing degraded DNA from non-destructively sampled museum specimens for RAD-tagging and low-coverage shotgun phylogenetics. PLoS One 9:e96793. doi: 10.1371/journal.pone.0096793
Toussaint, E. F. A., Breinholt, J. W., Earl, C., Warren, A. D., Brower, A. V. Z., Yago, M., et al. (2018). Anchored phylogenomics illuminates the skipper butterfly tree of life. BMC Evol. Biol. 18:101. doi: 10.1186/s12862-018-1216-z
Toussaint, E. F. A., Chiba, H., Yago, M., Dexter, K. M., Warren, A. D., Storer, C., et al. (2021a). Afrotropics on the wing: phylogenomics and historical biogeography of awl and policeman skippers. Syst. Entomol. 46, 172–185. doi: 10.1111/syen.12455
Toussaint, E. F. A., Ellis, E. A., Gott, R. J., Warren, A. D., Dexter, K. M., Storer, C., et al. (2021b). Historical biogeography of Heteropterinae skippers via Beringian and post-Tethyan corridors. Zool. Scr. 50, 100–111. doi: 10.1111/zsc.12457
Toussaint, E. F. A., Gauthier, J., Bilat, J., Gillett, C. P. D. T., Gough, H. M., Lundkvist, H., et al. (2021c). HyRAD-X exome capture museomics unravels giant ground beetle evolution. Genome Biol. Evol. 13, 13:evab112. doi: 10.1093/gbe/evab112
Toussaint, E. F. A., Vila, R., Yago, M., Chiba, H., Warren, A. D., Aduse-Poku, K., et al. (2019). Out-of-orient: post-Tethyan transoceanic and trans-Arabian routes fostered the spread of Baorini skippers in the Afrotropics. Syst. Entomol. 44, 926–938. doi: 10.1111/syen.12365
Townsend, J. P., and Leuenberger, C. (2011). Taxon sampling and the optimal rates of evolution for phylogenetic inference. Syst. Biol. 60, 358–365. doi: 10.1093/sysbio/syq097
Valencia-Montoya, W. A., Quental, T. B., Tonini, J. F. R., Talavera, G., Crall, J. D., Lamas, G., et al. (2021). Evolutionary trade-offs between male secondary sexual traits revealed by a phylogeny of the hyperdiverse tribe Eumaeini (Lepidoptera: Lycaenidae). Proc. R. Soc. B 288:20202512. doi: 10.1098/rspb.2020.2512
Venables, W.N., and Ripley, B.D. (2002). Modern applied statistics with S. New York: Springer, doi: 10.1007/978-0-387-21706-2.
Wahlberg, N., and Wheat, C. W. (2008). Genomic outposts serve the phylogenomic pioneers: designing novel nuclear markers for genomic DNA extractions of Lepidoptera. Syst. Biol. 57, 231–242. doi: 10.1080/10635150802033006
Wells, A., Johanson, K. A., and Dostine, P. (2019). Why are so many species based on a single specimen? Zoosymposia 14, 32–38. doi: 10.11646/zoosymposia.14.1.5
Whibley, A., Kelley, J., and Narum, S. (2021). The changing face of genome assemblies: guidance on achieving high-quality reference genomes. Mol. Ecol. Resour. 21, 641–652. doi: 10.1111/1755-0998.13312
Wickham, H. (2016). ggplot2: Elegant graphics for data analysis. New York: Springer-Verlag. ISBN 978-3-319-24277-4. Available at: https://ggplot2.tidyverse.org. (Accessed May 13, 2022).
Wickham, H., Averick, M., Bryan, J., Chang, W., McGowan, L. D., Françoi, R., et al. (2019). Welcome to the tidyverse. J. Open Source Softw. 4:1686. doi: 10.21105/joss.01686
Wood, H. M., González, V. L., Lloyd, M., Coddington, J., and Scharff, N. (2018). Next-generation museum genomics: phylogenetic relationships among palpimanoid spiders using sequence capture techniques (Araneae: Palpimanoidea). Mol. Phylogenet. Evol. 127, 907–918. doi: 10.1016/j.ympev.2018.06.038
Zhang, Y., Deng, S., Liang, D., and Zhang, P. (2019a). Sequence capture across large phylogenetic scales by using pooled PCR-generated baits: a case study of Lepidoptera. Mol. Ecol. Resour. 19, 1037–1051. doi: 10.1111/1755-0998.13026
Zhang, F., Ding, Y., Zhu, C.-D., Zhou, X., Orr, M. C., Scheu, S., et al. (2019b). Phylogenomics from low-coverage whole-genome sequencing. Methods Ecol. Evol. 10, 507–517. doi: 10.1111/2041-210X.13145
Zhang, Y., Huang, S., Liang, D., Wang, H., and Zhang, P. (2020). A multilocus analysis of Epicopeiidae (Lepidoptera, Geometroidea) provides new insights into their relationships and the evolutionary history of mimicry. Mol. Phylogenet. Evol. 149:106847. doi: 10.1016/j.ympev.2020.106847
Keywords: anchored hybrid enrichment, historical DNA, hybrid capture, Lepidoptera, museomics, archival DNA, Papilionoidea, phylogenomics
Citation: Nunes R, Storer C, Doleck T, Kawahara AY, Pierce NE and Lohman DJ (2022) Predictors of sequence capture in a large-scale anchored phylogenomics project. Front. Ecol. Evol. 10:943361. doi: 10.3389/fevo.2022.943361
Edited by:
Jonathan J. Fong, Lingnan University, ChinaReviewed by:
Pedro Ribeiro, Academy of Sciences of the Czech Republic (ASCR), CzechiaLauren Eserman-Campbell, Atlanta Botanical Garden, United States
Copyright © 2022 Nunes, Storer, Doleck, Kawahara, Pierce and Lohman. This is an open-access article distributed under the terms of the Creative Commons Attribution License (CC BY). The use, distribution or reproduction in other forums is permitted, provided the original author(s) and the copyright owner(s) are credited and that the original publication in this journal is cited, in accordance with accepted academic practice. No use, distribution or reproduction is permitted which does not comply with these terms.
*Correspondence: David J. Lohman, ZGxvaG1hbkBjY255LmN1bnkuZWR1
†Present address: Caroline Storer, Pacific Biosciences, Menlo Park, CA, United States