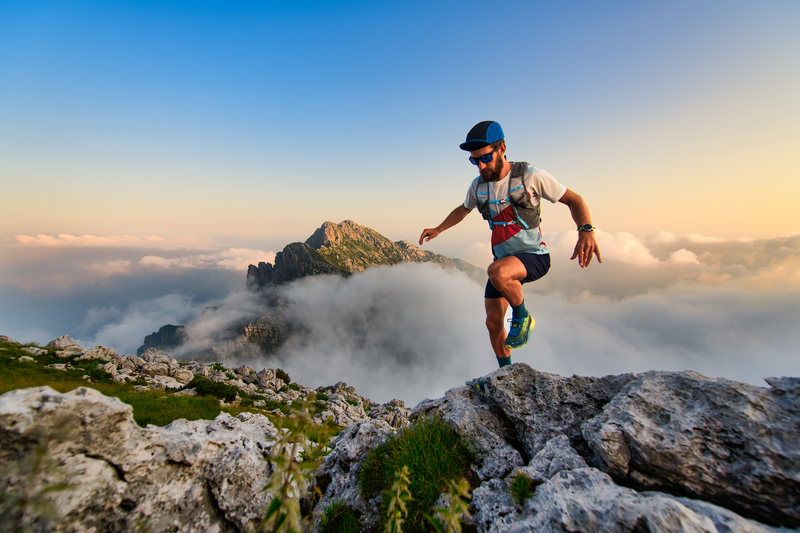
94% of researchers rate our articles as excellent or good
Learn more about the work of our research integrity team to safeguard the quality of each article we publish.
Find out more
ORIGINAL RESEARCH article
Front. Ecol. Evol. , 03 August 2022
Sec. Environmental Informatics and Remote Sensing
Volume 10 - 2022 | https://doi.org/10.3389/fevo.2022.935621
This article is part of the Research Topic Social Media, Artificial Intelligence and Carbon Neutrality View all 10 articles
Environmental regulations have been intensified across the country under the pressure of the national “double carbon” strategy, the constraints of energy-saving and emission-reduction targets by enterprises, and public opinion. The tightening of environmental regulations is bound to impact the innovation behavior of heavily polluting enterprises; however, it remains uncertain whether the impact is positive or negative. Using a differences-in-differences approach of data from listed companies in China’s heavily-polluting industries between 2010 and 2016, this paper examines the changes in their innovation behavior under the tightening environmental regulations after the “smog explosion” event as a “quasi-natural experiment” at the end of 2011. The study found that the “smog event” had a significant net negative effect on the innovation behavior of heavily polluting firms, with a significant decrease in their innovation inputs. The quantile regression results show that the R&D intensity of enterprises is related to the haze treatment effect in a U-shaped curve. Further research found that the decline in innovation investment was more significant for state-owned heavily-polluting firms compared to private heavy polluting firms. Robustness tests indicate that the empirical results of this paper are somewhat robust. This paper aims to identify the contradictory roots of the “Porter hypothesis” debate by analyzing the differences in innovation behavior of enterprises with different R&D intensity and different property rights.
Since the political reform and opening-up of trade, the Chinese economy developed rapidly to become the second-largest economy in the world. However, with this rapid economic development, industrial pollution, especially air pollution, has become increasingly prominent. Given that the traditional soot pollution has not been effectively controlled, the regional complex air pollution characterized by ozone, smog (PM2.5), and acid rain has grown in prominence. At the end of October 2011, a “PM2.5 explosion”1 incident resulted in a rise in smog, showcased as a new type of air pollution source to the public. On September 22, 2020, during the general debate of the 75th session of the United Nations General Assembly, President Xi Jinping declared to the world that carbon emissions would peak by 2030 and carbon neutrality would be achieved by 2060. The state increased its environmental regulation and therefore increased the risk of environmental liability for heavy polluters. Reducing PM2.5 and carbon sequestration are now included in government reports, and the government has strengthened environmental controls on heavily-polluting industries and introduced several legal policies, resulting in a change in the policy environment for heavily-polluting industries. This has led to a strong ‘stress response’ from companies in the heavily polluting industries, attracting the attention of many scholars. Liu Yanguo and Liu Mengning examined the impact of political costs on the surplus management of heavy polluters using the “PM2.5 explosion” and found that heavy polluters engaged in downward surplus management after the smog (Liu and Liu, 2015). Sheng et al. (2017) examined the impact of the “smog event” on the financing ability of heavily polluting firms and explored the mechanism of this impact. Firms reacted differently to environmental degradation (Sheng et al., 2017). There is no conclusive evidence on the impact of environmental regulation on firm innovation, and neither the “Pollution Paradise Hypothesis” nor the “Porter Hypothesis” has been able to clarify the matter via empirical study. In this study, using the exogenous impact of the “PM2.5 explosion,” the net effect of the “smog event” on the innovation behavior of heavily-polluting enterprises is analyzed by the differences-in-differences (DID) method. Moreover, using a “quasi-natural experiment,” endogenous problems can be avoided, which increases the credibility of our research conclusion. Concurrently, it also enriches the research perspective of the impact of environmental regulation on enterprise innovation behavior.
The influence of environmental regulation on enterprise innovation capability has always been a controversial topic. Environmental regulation has both a positive compensation effect and a negative offset effect on enterprise innovation. However, the culminating effect of both can be drenched in uncertainty; hence, research on this issue remains controversial. The traditional view holds that the offset effect of environmental regulation is greater than its compensation effect. Although environmental protection has good positive externalities, environmental regulation will increase the cost of enterprises and reduce the innovation expenditure of enterprises (Barbera and Mcconnell, 1990; Jorgenson and Wilcoxen, 1990; Brännlund et al., 1998; de Miguel and Pazo, 2017). On the contrary, Porter’s hypothesis holds that the compensation effect of environmental regulation is greater than the offset effect. Moderate environmental regulation can encourage enterprises to perform technological innovation, improve the production efficiency of enterprises, and reduce the production cost, thus offsetting the increased cost of environmental regulation. Many scholars have verified these two opposing views from an empirical point of view. Amongst others, Gray and Shadbegian (1995); Arduini and Cesaroni (2001), Wagner (2007); Wang et al. (2017) maintain that environmental regulation has a significant offset effect on technological innovation. In response, Berman and Bui (2001); Hamamoto (2006), Lambertini (2017); Fabrizi et al. (2018) and other scholars have verified Porter’s hypothesis through empirical tests. Therefore, there is merit to suggest that environmental regulation has a significant compensation effect on technological innovation. On the other hand, Eiadat et al. (2008); Frondel et al. (2008), Lanoie et al. (2008); Stoever and Weche (2018) and other scholars have found that the relationship between environmental regulation and enterprise innovation is uncertain in related industries in Europe and America.
With the increasing strength of environmental protection legislation, Chinese scholars have also started to study the existence and applicability of Porter’s Hypothesis in China. Some scholars have made an empirical analysis of the relationship between environmental regulation and productivity in China’s manufacturing industry and indirectly proved that environmental regulation can promote technological innovation (Zhao, 2008; Li and Nie, 2010). Multiple theories have been reported as to the exact relationship between environmental regulation and technological innovation in China. For instance, Neng and Feng-chao (2012) found that the “Porter’s Hypothesis” only existed in the eastern part of China when they investigated the influence of environmental regulation on technological innovation in different regions. In addition, some scholars have found that the relationship between environmental regulation and technological innovation is not purely linear, but there is a U-shaped relationship or an inverted U-shaped relationship between them. On the other hand, Jiang et al. (2013) and Wang and Liu (2014) found that the relationship between environmental regulation and technological innovation is a polygonal shape, and the influence coefficients between them vary in different stages. However, scholars such as Bing et al. (2008) and Xie (2008) have found an unclear relationship between environmental regulation and technological innovation.
The above-mentioned local and global research examined the relationship between environmental regulation and technological innovation as a whole and did not distinguish the heterogeneity of industries. Factors such as resource endowment, factor structure, and pollution level will cause different relationships between environmental regulation and technological innovation in various industries. Yu and Hu (2016) found that environmental regulation has always had a negative impact on the technological innovation of heavily-polluting industries, whereas it had a significant promoting effect in moderately-polluting industries and a U-shaped relationship in lightly-polluting industries. Liu et al. (2017) also found a U-shaped relationship between the impact of environmental regulation and industrial technological innovation. At present, the intensity of environmental regulation in China is on the left side of the inflection point, and the U-shaped inflection point of pollution-intensive industries appears later than that of clean industries. However, literature analysis reveals that environmental regulation should have a negative effect on the technological innovation of China’s heavily-polluting industries. Compared with other industries, heavily-polluting industries produce a lot of pollutants in production, and environmental regulation seriously hinders the normal development of the industry, greatly reduces the profits of the industry, and repurposes investment in the enterprises toward technological innovation. This dilemma is the reality of heavily-polluting industries after the “smog event.” Public opinion greatly affects the reputations of enterprises which can intensify the financing constraints of enterprises. Concomitantly, the government has stepped up the control of heavily-polluting industries, and successively introduced a series of targeted control policies. In 2012, the Ambient Air Quality Standard and the Twelfth Five-Year Plan for the Prevention and Control of Air Pollution in Key Areas were issued, and “smog control” was written into the Environmental Protection Law of the People’s Republic of China on April 24, 2014. The deterioration of financing constraints, the strengthening of legislation, and the restriction of the nature of industries further weaken the innovation power of heavily-polluting industries which severely handicaps their productivity. Therefore, this paper puts forward the following assumption.
Hypothesis 1: After the “smog event”, the innovation investment of heavily-polluting enterprises will decrease significantly compared with non-polluting enterprises.
In addition, although both state-owned enterprises and private enterprises are the products of the market-oriented reform in China, there remain huge differences between them (Wu and Qian, 2011). State-owned enterprises, as the embodiment of national interests, consider both economic and social goals, while private enterprises take survival and profit as their main goals. Different strategic objectives of enterprises will also lead to different innovative behaviors in enterprises. Xiao-Qing et al. (2014) and other studies have found that the innovation efficiency of state-owned enterprises is generally lower than that of private enterprises. Zhao et al. (2014) and other studies have found that there is a big difference in the innovative spirit between state-owned enterprises and private enterprises—with the innovative power of private enterprises stronger than that of state-owned enterprises. Given these differences, it is worth asking how will environmental regulation affect enterprise innovation? Under the pressure of environmental protection, some large heavily-polluting state-owned enterprises often need higher investment in environmental protection, and environmental regulation weakens the scale and capital advantages of state-owned enterprises, thus affecting the innovation of enterprises (Jiang et al., 2013). In addition, compared with private enterprises, state-owned enterprises often bear huge social responsibilities because of their unique political status. Under strict environmental regulation, state-owned enterprises tend to follow end-of-pipe pollution treatment to create the perception of “environmentally friendly” practices. As a result, pollution control costs increase, corporate profits decrease, and some R&D investments are suspended. As for private enterprises that strive to maximize profit, when faced with strict environmental regulations they tend to improve their production technology and production efficiency to offset the increased environmental costs. After the “smog event”, it can be expected that both state-owned and private heavily-polluting enterprises faced more severe environmental pressure. Under the strong pressure of environmental protection, the innovative behavior of state-owned and private heavily-polluting enterprises will inevitably form a great contrast. Based on this, this study puts forward the following assumption:
Hypothesis 2: After the “smog event,” compared with private heavily-polluting enterprises, the innovation investment of state-owned heavily-polluting enterprises decreased more significantly.
The initial sampling period for this study is between 2009 and 2016. The initial samples include A-share companies in Shanghai and Shenzhen Stock Exchanges as of December 31, 2008. The key point of this paper is to choose the appropriate control group and experimental group, i.e., to reasonably distinguish the heavily-polluting listed companies from the non-heavily-polluting listed companies. According to official documents, such as the “Twelfth Five-Year Plan” on Air Pollution Prevention and Control in Key Regions and the Announcement on the Implementation of Special Emission Limits for Air Pollutants, we manually selected companies belonging to heavily-polluting industries (as defined in the documents) as the experimental group of this study2. As for the control group, referring to the matching strategy used by Liu and Liu (2015) and Sheng et al. (2017): Guidelines for the Industry Classification of Listed Companies (2012 Revision), and defined other listed companies in the same category of heavily-polluting enterprises as non-heavily-polluting enterprises, i.e., the control group of this study3. Then, the sample data were screened as follows: (1) companies with multiple missing values were removed; (2) listed companies in the ST, *ST, and S*ST categories during the sample period with abnormal financial conditions were removed. Among them, there were many missing data concerning the R&D investment of the listed companies. The main reason is that on February 15th, 2006, the Ministry of Finance issued the Accounting Standards for Business Enterprises No. 6-Intangible Assets, which greatly revised the accounting treatment of the original standard stating that the R&D expenses of enterprises should be included in reporting current profits and losses. However, many companies did not disclose R&D investment data in their financial statements from 2008 to 2009. Therefore, the final sample interval of this study was from 2010 to 2016, and the final sample observation value was 3,066. The enterprise R&D data of this study were retrieved from the CCER database, and the financial and property rights data were obtained from the CSMAR and RESSET databases.
As for the measurement of the innovation capability of an enterprise, the research of Wen and Feng (2012) was used as the reference, and the R&D investment intensity of enterprises was used to measure their innovation capability. Among them, R&D investment intensity is equal to the ratio of total R&D expenditure to business income. To improve the accuracy of the model, this study builds on previous studies and adds company characteristic variables that may affect the innovation investment of enterprises (Huihua et al., 2008; Wu, 2012; Wu and Tang, 2016). The control variables of company characteristics include: the asset-liability ratio (lev), the growth rate of main business income, the net profit rate of total assets (roa), intangible asset rate (int), company size, and company age. The specific definitions are shown in Table 1.
The main purpose of this study is to investigate the net effect of the exogenous impact of the “smog event” on the innovation behavior of heavily-polluting enterprises. Therefore, we constructed the following DID estimation model:
Among them, i represents the enterprise, t represents the observation year, treati represents whether the sample belongs to the dummy variable of the experimental group, and aftert represents whether the sample is in the period after the “smog event”; yit is the proxy variable of the innovation behavior of the enterprise, which is expressed by the R&D intensity of the enterprise in this study; control represents the control variable that affects enterprise innovation; εit represents the random interference term. The object of concern is the coefficient of interaction β between treati and aftert. The net effect of the “smog event” on the innovation behavior of heavily-polluting enterprises is measured by β. If its value is negative, the hypothesis of this study is proved, i.e., the innovation investment of heavily-polluting enterprises dropped more obviously than that of non-heavily-polluting enterprises.
Before DID estimation, the distribution of the experimental group and the control group are first assessed to determine the rationality of sample selection. Table 2 lists the industrial distribution of 105 heavily-polluting enterprises in the experimental group. As shown in Table 2, there was uneven industrial distribution in the experimental group, which closely resembled the actual distribution of listed companies in the heavily-polluting industries of China and could depict the whole to a certain extent. In addition, the sample companies of the experimental group included nine major industries, accounting for 10% of the 90 major industries classified by the China Securities Regulatory Commission, indicating good representation by the samples.
Table 3 lists the descriptive statistics of the sample data in this study. For financial data, outliers were extremely easy to occur. To prevent the influence of outliers on model estimation, this study truncated all sample data at the 1% level. As seen in Table 3, the standard deviation of R&D of the explained variable was the largest, indicating great differences in R&D for each sample, with some of the differences potentially caused by the impact of the “smog event.” This also reflects the rationality of the inference drawn in this study.
The common trend assumption is the most critical assumption of the DID method, which requires the experimental group and the control group to have the same change trend before the external impact. The common trend hypothesis sometimes becomes a constant bias hypothesis, i.e., the experimental group and the control group may be dissimilar, allowing them to have certain differences. These differences are mainly caused by unobserved confounding factors that do not change with time. If there is no external impact, the differences between the experimental group and the control group before and after the impact point should be constant. Based on the above analysis, Table 4 compares and analyzes the differences in innovative behaviors between the experimental group and the control group before and after smog. As seen in Table 4, before the “smog event,” there was a significant difference in R&D intensity between the experimental group and the control group, whether using the mean or median tests, and the difference was mainly caused by the different nature of the industries. After the “smog event,” the difference in the R&D intensity between the experimental group and the control group further widened. This shows that the “smog event” had a certain impact on enterprise innovation, but the specific impact still needs to be follow-up with an empirical test.
Table 5 lists the full sample DID estimation results of the influence of the “smog event” on enterprise innovation. This study used R&D intensity as the proxy variable of enterprise innovation, and mainly investigated the changes in enterprise innovation behavior before and after the “smog event,” i.e., the coefficient of treat × after. If its coefficient is significantly negative, it means that the “smog event” had a significant negative effect on the innovation behavior of heavily-polluting enterprises, and the expected hypothesis of this study is proved. Models (1) and (2) represent benchmark regression, and only examined the relationship between the DID variable and the explained variable. Among them, model (2) controls the influence of the year and industry. To improve the reliability of model estimation, model (3) and model (4) added enterprise characteristic variables at the company level as control variables of the model.
In both models (1) and (2), the coefficient of treat × after was significantly negative at the 1% level. However, the goodness of fit of model (2) was improved after controlling for the year and industry characteristics. After adding the control variables that reflect the characteristics of the enterprise, the treat × after coefficients in models (3) and (4) were still significantly negative at the 1% level. The goodness of fit of models (3) and (4) was further improved, and the overall explanatory power of the model was stronger. The regression results of models (1)–(4) show that the “smog event” does have an impact on the innovation behavior of heavily-polluting enterprises. After the “smog event,” the innovation investment of heavily-polluting enterprises dropped significantly, which verifies the previous deduction noted in this study. Therefore, hypothesis 1 was proven in this study.
As for other coefficients, the treat variable depicts the differences between the experimental group and the control group, and its coefficients are significant in the four models, indicating that there are significant differences in innovation behaviors between heavily-polluting enterprises and non-heavily-polluting enterprises, which is consistent with the results of the mean and median tests mentioned above. After capturing the variables responsible for the possible impact of other events on the innovation behavior of enterprises in the same period after the “smog event”, the most likely one was found to be the impact of time. In the model, the coefficient of after was significantly positive, indicating that with the passage of events, enterprises are constantly strengthening their R&D investment, which was consistent with the development law of enterprises. In addition, the coefficient symbols of control variables at the enterprise level are also in good agreement with previous literature.
The results of full sample regression proved that the “smog event” had a negative impact on the innovation behavior of heavily-polluting enterprises. However, for enterprises with different R&D intensities, the impact of the “smog event” on innovation behavior may have varied. Therefore, the difference in the smog treatment effect under different R&D intensities was further estimated by quantile regression.
A total of four quantiles (0.2, 0.4, 0.6, and 0.8) were selected for estimation. Table 6 lists the estimation results of the treatment effect in each quantile. To improve the explanatory ability of the model, the year and industry characteristics were still controlled in the model, and the control variable reflecting the enterprise characteristics was added. The explained variable of the model remained the R&D intensity. The results of the quantile regression showed that for each quantile, the coefficient of treat × after was significantly negative at the 1% level, i.e., the “smog event” had a negative effect on the R&D investment of heavily-polluting enterprises. Further analysis showed that the processing effect of the “smog event” was different in each quantile. The treatment effects in the four quartiles were 6.3, 5.9, 5.32, and 11.07%, respectively. As the R&D intensity increased, the treatment effects first decreased and then increased. This indicates that the R&D intensity of the enterprise had a U-shaped curve relationship with the “smog event.”
The unique institutional environment of China determines the environmental differences between state-owned enterprises and private enterprises. For these, the political pressure and environmental pressure caused by the “smog event” were quite different. After the “smog event,” the awareness of environmental protection returned to the people, and the heavily-polluting state-owned enterprises became the “culprits” of pollution. Under the strong pressure of environmental protection, the heavily-polluting state-owned enterprises could only adopt short and quick end-off-pipe treatment methods to reflect so that the government and the public can see their “environmental performance.” Private enterprises, after the “smog event” faced stricter environmental regulations. To survive and develop, they had to increase R&D investment to meet the national environmental protection requirements.
Therefore, this study expects that, under the “smog event” and a consequential series of environmental pressures, the innovation behaviors of state-owned and private heavily-polluting enterprises would be different. State-owned enterprises had weak innovation motivation to fulfill their social responsibilities, while private enterprises had strong innovation motivation due to survival pressure. Table 7 lists the results of the regression of property rights DID grouping. As discussed above, this part is still concerned with the coefficient of treat × after. For conciseness, the estimation results of control variables are not listed in this study. Models (1) and (2) are the results of property right grouping regression without introducing control variables. The model results show that the coefficient of the state-owned group treat × after was significantly negative at the 1% level, while the coefficient of the private group treat × after was not significant. Models (3) and (4) grouped regression results after introducing control variables. The sign and significance of the two groups of the treat × after coefficients did not change but the values did. The results of sub-property rights DID regression show that after the “smog event,” compared with private heavily-polluting enterprises, the innovation investment of the state-owned heavily-polluting enterprises decreased more significantly, whereas the negative effect of the “smog event” on the private heavily-polluting enterprises was not significant. Therefore, hypothesis two is proven from these results. Among them, a possible explanation for the non-significant negative effect of the “smog event” on the heavily-polluting private enterprises is that under the greater pressure of environmental protection, although private enterprises tend to increase R&D investment, the “smog event” caused greater financing constraints (Sheng et al., 2017). The interaction between innovation tendency and financial constraints means the impact of the “smog event” on the technological innovation of heavily-polluting private enterprises is full of uncertainty.
The empirical results of this study have verified that the research logic of this study is reasonable to some extent. However, to ensure the robustness of the empirical results, the robustness test of the empirical results is needed. The logic of the robustness test is as follows: First, the explained variable was changed. In our previous article, R&D investment intensity was used as the proxy variable of enterprise innovation. Here, the total R&D expenditure of enterprises was used as the explained variable to test whether the “smog event” had a negative processing effect on enterprise innovation. Second, using the research method of Hou et al. (2015), this study used the method of the placebo test to set a pseudo-smog event as the placebo before the smog event to test the robustness of the model. Theoretically speaking, if there is a causal relationship between the “smog event” and the innovative behavior of heavily-polluting enterprises, then the processing effect of the “smog event” should not be significant if the event does not happen. The specific design of the placebo test was as follows: 2010 was set as the occurrence point of the “smog event,” and the research samples were taken from 2009 to 2016. In 2009–2010, the value of the after variable was 0, and after 2010, the after variable was 1. The treat variable was the same as above, with 1 for heavily-polluting enterprises and 0 for non-heavily-polluting enterprises.
Table 8 lists the results of the robustness test. Models (1) and (2) represent the test results of replacing the explained variables, and models (3) and (4) the results of the placebo test. The empirical results of models (1) and (2) showed that after changing the explained variables, the “smog event” still had a negative impact on the innovation behavior of heavily-polluting enterprises. Furthermore, whether the control variables are introduced or not, the treat × after coefficient remained significantly negative. The conclusion of changing the explanatory variables was consistent with that in Table 5. The empirical results of models (3) and (4) showed that whether control variables were introduced or not, the pseudo “smog event” had a negative impact on the innovation behavior of heavily-polluting enterprises. This result was contrary to our expectation of a placebo test and almost overturned the previous research conclusion. However, further analysis showed that the placebo test needed multiple periods of historical data for support. Nevertheless, due to the data limitation, this study could only advance the placebo time by one period, which caused the virtual “smog event” time to be too close to the actual “smog event” time, failing the criteria of the placebo test. The results of the placebo test showed that, although the pseudo “smog event” had a negative impact on the innovation behavior of the enterprises, the significance of the treatment effect was lower and the degree smaller compared with the actual “smog event.” This result proved the robustness of this conclusion from the side, because with approaching authenticity, the possibility of a significant treat × after coefficient is greater, which reveals that the concept of “green development” is a deeply rooted sentiment for people. In this regard, the influence of environmental regulation on enterprise behavior cannot be achieved overnight but requires a process of continuous evolution.
In this paper, under the national “double carbon” strategy, using the exogenous shock of “PM2.5 explosion” in many regions of China at the end of 2011 as well as the DID method, it was found that the R&D intensity of heavily-polluting enterprises decreased significantly compared to non-heavily polluting enterprises after the “smog event.” The effect of the “smog event” on firms with variable R&D intensities differed significantly, with a U-shaped curve relationship between the two. The regression results of the property grouping showed that the negative effect of the “smog event” on private heavy polluters was not significant, while the decrease in innovation investment of state-owned heavy polluters was more significant. After the variable test and placebo test, the model results were still robust.
This study only discussed the innovation behavior of enterprises from the aspect of R&D investment without discussing the influence of the “smog event” on the innovation output of enterprises. In addition, the innovation behavior of enterprises is only one aspect of many behaviors of enterprises. In this regard, the influence of environmental regulation on enterprise behavior should be discussed from multiple angles concerning the exogenous impact of the “smog event”. Mechanisms to enhance the innovation ability of heavily-polluting enterprises via advances in science and technology should also be elucidated to consolidate energy saving and emission reduction. Lastly, the existence and applicability of Porter’s Hypothesis in China should be discussed from the micro-enterprise level.
The original contributions presented in the study are included in the article/supplementary material, further inquiries can be directed to the corresponding author.
NY, LY, XJ, and WD were responsible for designing the framework of the entire manuscript from topic selection to solution to experimental verification. All authors contributed to the article and approved the submitted version.
This study was supported by Special Project of Philosophy and Social Sciences in Hainan Province Topic Title: Innovation Research on the Evolution of Island Ecological Civilization and the Circular Division of Labor in Green Industry Project number: HNSK (ZX) 18-118.
The authors declare that the research was conducted in the absence of any commercial or financial relationships that could be construed as a potential conflict of interest.
All claims expressed in this article are solely those of the authors and do not necessarily represent those of their affiliated organizations, or those of the publisher, the editors and the reviewers. Any product that may be evaluated in this article, or claim that may be made by its manufacturer, is not guaranteed or endorsed by the publisher.
Arduini, R., and Cesaroni, F. (2001). Environmental Technologies in the European Chemical Industry. Montgomeryville, PA: LEM Papers.
Barbera, A. J., and Mcconnell, V. D. (1990). The impact of environmental regulations on industry productivity: direct and indirect effects. J. Environ. Econ. Manag. 18, 50–65. doi: 10.1016/0095-0696(90)90051-Y
Berman, E., and Bui, L. T. M. (2001). Environmental regulation and productivity: evidence from oil refineries. Rev. Econ. Stat. 83, 498–510. doi: 10.1162/00346530152480144
Bing, W., Yanrui, W., and Pengfei, Y. (2008). environmental regulation and total factor productivity growth: an empirical study of the APEC. Econ. Res. J. 5, 19–32.
Brännlund, R., Chung, Y., Färe, R., and Grosskopf, S. (1998). Emissions trading and profitability: the Swedish pulp and paper industry. Environ. Resour. Econ. 12, 345–356. doi: 10.1023/A:1008285813997
de Miguel, C., and Pazo, C. (2017). Environmental protection, innovation and price-setting behavior in Spanish manufacturing firms. Energy Econ. 68, 116–124. doi: 10.1016/j.eneco.2017.10.030
Eiadat, Y., Kelly, A., Roche, F., and Eyadat, H. (2008). Green and competitive? An empirical test of the mediating role of environmental innovation strategy. J. World Bus. 43, 131–145. doi: 10.1016/j.jwb.2007.11.012
Fabrizi, A., Guarini, G., and Meliciani, V. (2018). Green patents, regulatory policies and research network policies. Res. Policy 47, 1018–1031. doi: 10.1016/j.respol.2018.03.005
Faff, R. W., Shao, X., Alqahtani, F., Atif, M., Białek-Jaworska, A., Chen, A., et al. (2017). Increasing the discoverability of non-English language research papers: a reverse-engineering application of the pitching research template. J. Account. Manag. Inform. Syst. 57. doi: 10.2139/ssrn.2948707
Frondel, M., Horbach, J., and Rennings, K. (2008). What triggers environmental management and innovation? Empirical evidence for Germany. Ecol. Econ. 66, 153–160. doi: 10.1016/j.ecolecon.2007.08.016
Gray, W. B., and Shadbegian, R. J. (1995). Pollution Abatement Costs, Regulation, and Plant-Level Productivity. Cambridge, MA: NBER Working Paper. doi: 10.3386/w4994
Hamamoto, M. (2006). Environmental regulation and the productivity of Japanese manufacturing industries. Resour. Energy Econ. 28, 299–312. doi: 10.1016/j.reseneeco.2005.11.001
Hou, Q., Yuan, J., and Liu, Q. (2015). Launching of high speed rail, information environment and enterprise innovation ——quasi – natural experiment based on the opening of high speed railways. Int. Symp. Account. Res. China
Huihua, N. I. E., Tan, S., and Wang, Y. (2008). Innovation, firm size and market competition: panel data analysis based on China’s enterprises. J. World Econ. 31, 57–66.
Jiang, F., Wang, Z., and Bai, J. (2013). The dual effect of environmental regulations’ impact on innovation——an empirical study based on dynamic panel data of Jiangsu manufacturing. China Ind. Econ. 7, 44–55.
Jorgenson, D. W., and Wilcoxen, P. J. (1990). Environmental regulation and U.S. economic growth. Rand J. Econ. 21, 314–340. doi: 10.2307/2555426
Lambertini, L. (2017). Green innovation and market power. Annu. Rev. Resour. Econ. 9, 231–252. doi: 10.1146/annurev-resource-100516-053508
Lanoie, P., Patry, M., and Lajeunesse, R. (2008). Environmental regulation and productivity: testing the porter hypothesis. J. Product. Anal. 30, 121–128. doi: 10.1007/s11123-008-0108-4
Li, Q., and Nie, R. (2010). Environmental regulation and China’s industrial productivity of large and medium-sized enterprises——empirical study based on porter hypothesis. J. China Univ. Geosci. 10, 55–59.
Liu, W., Tong, J., and Xue, J. (2017). Industrial heterogeneity, environmental regulation and technological innovation in the industrial sector. Sci. Res. Manag. 38, 1–11.
Liu, Y. G., and Liu, M. N. (2015). Have smog affected earning management of heavy-polluting enterprises?——based on the political-cost hypothesis. Account. Res. 3, 26–33.
Neng, S., and Feng-chao, L. (2012). can intensive environmental regulation promote technological innovation? Porter hypothesis reexamined. China Soft Sci. 4, 49–59.
Shao, X. F., Gouliamos, K., Luo, B. N. F., Hamori, S., Satchell, S., Yue, X.-G., et al. (2020). Diversification and desynchronicity: an organizational portfolio perspective on corporate risk reduction. Risks 8:51. doi: 10.3390/risks8020051
Sheng, M. Q., Wang, S., and Zhang, C. Q. (2017). Smog and corporate financing: empirical evidence from heavily polluting listed industries. Econ. Rev. 5, 28–39.
Stoever, J., and Weche, J. P. (2018). Environmental regulation and sustainable competitiveness: evaluating the role of firm level green investments in the context of the porter hypothesis. Environ. Resour. Econ. 70, 429–455. doi: 10.1007/s10640-017-0128-5
Wagner, M. (2007). On the relationship between environmental management, environmental innovation and patenting: evidence from German manufacturing firms. Res. Policy 36, 1587–1602. doi: 10.1016/j.respol.2007.08.004
Wang, J., and Liu, B. (2014). Environmental regulation and enterprises’ TFP——an empirical analysis based on China’s industrial enterprises data. China Ind. Econ. 3, 44–56.
Wang, X., Ge, J., Li, J., and Han, A. (2017). Market impacts of environmental regulations on the production of rare earths: a computable general equilibrium analysis for China. J. Clean Prod. 154, 614–620. doi: 10.1016/j.jclepro.2017.03.200
Wen, J., and Feng, G. F. (2012). Heterogeneous institutional investor, nature of firm and independent innovation. Econ. Res. J. 3, 53–64.
Wu, C., and Qian, T. (2011). Business group affiliation and the governance of state-owned enterprises. Econ. Res. J. 6, 93–104.
Wu, C., and Tang, D. (2016). Intellectual property rights enforcement, corporate innovation and operating performance: evidence from China’s listed companies. Econ. Res. J. 11, 125–139.
Wu, Y. (2012). What kind of ownership of Chinese enterprises is the most innovative? J. World Econ. 6, 3–29.
Xiao-Qing, D., Jian, Z., and Pengwei, Y. (2014). Research on innovation efficiency loss of state-owned enterprises. China Ind. Econ. 2, 97–108.
Xie, E. (2008). Environmental regulationand industrial productivity Growthin China. Ind. Econ. Res. 1, 19–25.
Yu, D., and Hu, Y. (2016). Does tightening environmental regulation impede technological innovation upgrading of manufacturing industries in China?——an empirical re-examination on porter hypothesis. Ind. Econ. Res. 2, 11–20.
Yue, X. G., Cao, Y., Duarte, N., Shao, X. F., and Manta, O. (2019). Social and financial inclusion through nonbanking institutions: a model for rural Romania. J. Risk Financ. Manag. 12:166. doi: 10.3390/jrfm12040166
Yue, X. G., Liao, Y., Zheng, S., Shao, X., and Gao, J. (2021). The role of green innovation and tourism towards carbon neutrality in Thailand: evidence from bootstrap ADRL approach. J. Environ. Manag. 292:112778. doi: 10.1016/j.jenvman.2021.112778
Zhao, H. (2008). Empirical study impact of environmental regulationon enterprises’ technological innovation——take Chinese 30 provincial largeand medium-sized enterpriseasan example. Soft Sci. 22, 121–125.
Keywords: smog, environmental regulations, heavily-polluting enterprises, innovative behaviors, differences-in-differences
Citation: Ying N, Yao L, Jihong X and Daqing W (2022) Environmental Regulation and Corporate Innovation Behavior Based on the “Double Carbon” Strategy – Empirical Analysis Based on A-Share Listed Companies of Heavy Pollution Industries on the Shanghai and Shenzhen Stock Exchanges. Front. Ecol. Evol. 10:935621. doi: 10.3389/fevo.2022.935621
Received: 04 May 2022; Accepted: 06 June 2022;
Published: 03 August 2022.
Edited by:
Xuefeng Shao, The University of Newcastle, AustraliaReviewed by:
Marwan Alim, University of Jedda, Saudi ArabiaCopyright © 2022 Ying, Yao, Jihong and Daqing. This is an open-access article distributed under the terms of the Creative Commons Attribution License (CC BY). The use, distribution or reproduction in other forums is permitted, provided the original author(s) and the copyright owner(s) are credited and that the original publication in this journal is cited, in accordance with accepted academic practice. No use, distribution or reproduction is permitted which does not comply with these terms.
*Correspondence: Wang Daqing, d2FuZ2RhcWluZzUxMUAxNjMuY29t
Disclaimer: All claims expressed in this article are solely those of the authors and do not necessarily represent those of their affiliated organizations, or those of the publisher, the editors and the reviewers. Any product that may be evaluated in this article or claim that may be made by its manufacturer is not guaranteed or endorsed by the publisher.
Research integrity at Frontiers
Learn more about the work of our research integrity team to safeguard the quality of each article we publish.