- 1International Science and Technology Cooperation Base of Hebei Province, Hebei International Joint Research Center for Remote Sensing of Agricultural Drought Monitoring, Hebei GEO University, Shijiazhuang, China
- 2Natural Resource Asset Capital Research Center, Hebei GEO University, Shijiazhuang, China
- 3Hebei Provincial Climate Centre, Hebei Province Meteorological and Ecological Environment Laboratory, Shijiazhuang, China
The surface net radiation as an important component of the surface radiation budget has attracted wide attention; however, it is still an enormous challenge to carry out an accurate estimation of the surface net radiation in areas with complex terrain due to the scarcity of radiation observation sites and high-spatial heterogeneity of the influencing factors of the surface net radiation. Taking the Haihe River Basin as the study area, this study estimated the surface net radiation under clear-sky conditions from 2001∼2019 based on an improved algorithm of the net long-wave radiation, and the solar short-wave radiation in terms of direct radiation, diffuse sky radiation, and reflected radiation from the surrounding terrain. In this study, the regional meteorological factors were inverted based on remote sensing data to make up for the deficiency of meteorological factor interpolation. The solar short-wave radiation was corrected by considering the comprehensive influence of the atmosphere, underlying surface, and terrain, and the net long-wave radiation was optimized by localizing the algorithm coefficients. The results showed the correlation coefficient between the estimated and observed surface net radiation reached approximately 0.9, indicating the accuracy of this improved method is acceptable. Besides, the results suggested the surface net radiation was significantly influenced by the terrain, the highest value of which occurred on the south slope, followed by that on the southwest slope, west or southeast slopes, and the lowest value occurred on the north slope. In addition, there was the highest surface net radiation in summer, and there was the lowest and most frequently negative surface net radiation in winter. This study makes up for the shortcomings of the traditional spatial interpolation of meteorological factors and previous empirical formulas, and can therefore provide an important methodological foundation for the research on the surface radiation, climate, and hydrology in the areas with complex terrain.
Introduction
The surface net radiation as an important component of the surface radiation balance serves as an important driver of regional and even global climate change (Gui et al., 2010; Gharekhan et al., 2022). The climate change in recent decades has inevitably affected the structure and function of ecosystems on the earth, leading to a series of ecological problems, such as the ecosystem imbalance, increased droughts, decreased plant productivity, and species population reduction (Li et al., 2022). The surface net radiation plays an important role in maintaining the surface water–heat balance, which is of great importance to understanding the evolution, structure, and spatial distribution of ecosystems, and it has raised considerable attention in the fields of ecology, agriculture, and water conservation (Jia et al., 2018). A series of surface radiation observation networks and sites have been globally established since the 1950s, but still failed to meet the need for relevant research in the fields of climate, hydrology, and ecology due to the uneven distribution of surface radiation observation sites, especially in complex terrain areas (Gui, 2010). The development of remote sensing technology since the 1960s has provided an innovative approach for the estimation of regional net surface radiation (Hallikainen and Kirimoto, 2008). The remote sensing technology can obtain the large-area and continuous observation data, which can effectively overcome the difficulty of assimilating data from sparse ground stations to a large spatial area (Zhang et al., 2019). There have been a variety of global surface radiation budget products based on remote sensing data (Pinker et al., 2003; Zhang et al., 2004; Tang et al., 2019), which are generally based on the atmospheric radiative transfer models that are not easy to implement and have higher uncertainties resulting from the requirement of more parameters. More importantly, there is generally low spatial resolution of the existing products based on the remote sensing inversion, which cannot reflect the spatial heterogeneity of areas with complex terrain and cannot meet the strict requirements of regional climatic and hydrological studies.
The remote sensing inversion of the net surface radiation on the regional scale, which mainly involves the estimation of solar incident radiation and net long-wave radiation, generally relies on the meteorological data from ground-based observations (Guo and Shen, 2015; Liu et al., 2017; Wu et al., 2017). Specifically, there are many commonly used inversion models of the solar incident radiation, e.g., the Zuo-Dakang Equation, Tong-Hong-Liang Equation, FAO56 Equation, Weng-Du-Ming Equation, YIN Equation, and Yang Equation (Allen et al., 1998; Yang et al., 2006; Yin et al., 2008; Cao et al., 2014). The inversion of the net long-wave radiation is generally carried out with multiple methods such as the Penman method, Brunt method, and Deng Genyun method, and the net long-wave radiation algorithm recommended by the FAO, which have the same structure but different empirical coefficients (Allen et al., 1998; Ren et al., 2006). However, the applicability of these various methods varies greatly in different regions, it is, therefore, necessary to carry out localization and verification of these methods according to the specific conditions, and to take into account the influence of the complex terrain in order to improve the applicability of these methods (Matsui and Osawa, 2015).
A number of scholars have tried to explore solar radiation in complex terrain with the help of the digital elevation model (DEM), geographic information system (GIS), and other technologies (Dozier and Frew, 1990; Dubayah and Rich, 1995; Sultan et al., 2014; Zhang et al., 2020, 2022). These previous studies suggested that the solar zenith angle, altitude, atmospheric transmittance, and surface albedo have a significant influence on the solar incident radiation in complex terrain, and the combination of the remote sensing technology and DEM can more accurately reveal the spatial distribution of solar incident radiation in complex terrain (Chen et al., 2012). However, in these relevant studies based on the remote sensing data and DEM data, scholars have generally focused more on the influence of either the atmosphere or the underlying surface, and they are more concentrated on the study of instantaneous radiation with a short-time series due to the limitation of remote sensing data (Roupioz et al., 2016; Zhang and Zhao, 2016; Hao et al., 2019). Additionally, there are scarcely meteorological stations in mountainous areas, which cannot meet the requirements of the large-scale estimation of the surface net radiation, and scholars generally have to obtain the spatial explicit information of the regional meteorological factors with spatial interpolation, which inevitably leads to some errors in the estimation of the surface net radiation (Long et al., 2010; Wu et al., 2017). Moreover, some previous studies have considered the influence of elevation (Long et al., 2010; Guo and Shen, 2015; Liu et al., 2017), but it is still difficult to distinguish the difference between shaded and sunny slopes and to accurately reflect the influence of the terrain.
The Haihe River Basin is one of the most important national grain production bases in China, where the average annual temperature has increased significantly in recent decades, and the relevant ecological issues under the background of global warming have received increasing attention from scholars. This region is located in a temperate continental monsoon climate zone, with very limited water resources, accounting for less than 1.3% of the national total water resource amount, but this region also accounted for 11% of the national arable land area, and therefore there is an outstanding contradiction between water supply and demand under climate change (Guo and Shen, 2015). More importantly, the water resources are very unevenly distributed in time and space, and the mountainous areas as the main water-producing areas are very sensitive to the changes in the surface radiation budget caused by climate change (Lei et al., 2014). It is therefore of great significance to explore the net surface radiation variation to the relevant research on agricultural, ecological, and hydrological problems of the Haihe River Basin. However, the scarcity of observation data on mountainous areas with complex terrain greatly limits the accurate acquisition of the regional net surface radiation in the Haihe River Basin. This study inverted the meteorological factors based on the remote sensing data to make up for the shortage of traditional spatial interpolation of meteorological factors, taking into account the impacts of the atmosphere, underlying surface, and complex terrain. This study further improved the key parameters of the algorithm to achieve a more accurate inversion of the net surface radiation under clear-sky conditions in the Haihe River Basin from 2001∼2019, aiming to provide a methodological reference for the estimation of net surface radiation in areas with complex terrain and relevant research in the fields of climate, agriculture, ecology, hydrology, and so on.
Materials and Methods
The daily net surface radiation was calculated as the difference between the incoming daily short-wave net radiation and the outgoing daily long-wave net radiation. This study first estimated the direct radiation, diffuse sky radiation, and reflected radiation from the surrounding terrain in the Haihe River Basin based on the remote sensing data and elevation data. Then, this study estimated the clear-sky solar incident radiation on the surface. Thereafter, the daily short-wave net radiation was estimated based on the surface reflectance from the remote sensing inversion. Finally, the net daily long-wave radiation of the Haihe River Basin was corrected based on the meteorological data from the remote sensing inversion, with the coefficients algorithm recommended by the FAO (Figure 1).
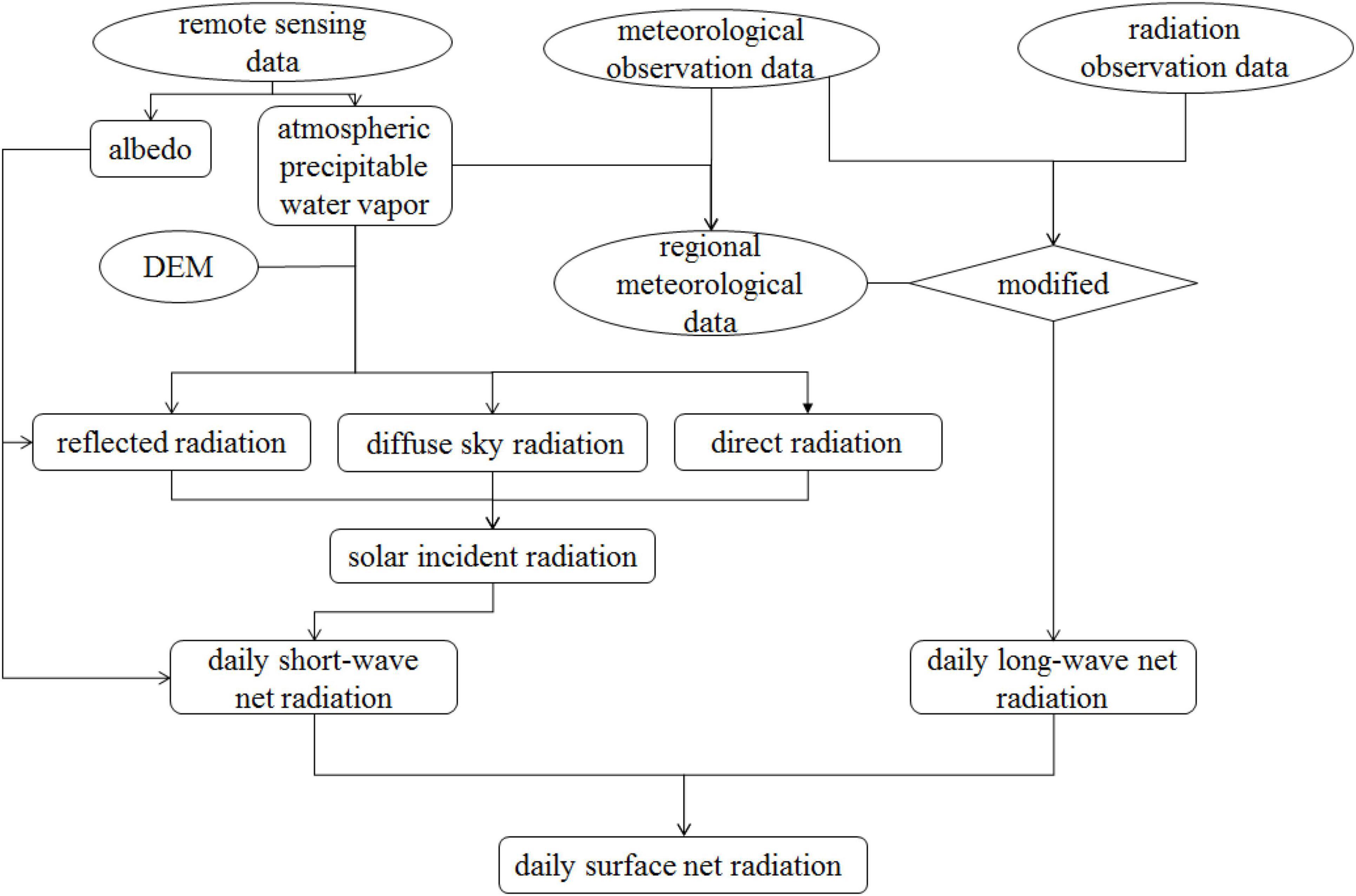
Figure 1. Flow diagram of the estimation of the surface net radiation under clear-sky conditions in this study.
The data used in the study included the remote sensing data, elevation data, and meteorological data used to optimize the parameters and validate the results. Specifically, the remote sensing data included the MODIS surface reflectance product MOD09GA and the MODIS atmospheric precipitable water product MOD05L2 from 2001∼2019, which were downloaded at https://search.earthdata.nasa.gov/and https://ladsweb.modaps.eosdis.nasa.gov/. The meteorological data of multiple meteorological stations included the relative humidity, daily maximum temperature, and daily minimum temperature from 2001∼2019 in the daily meteorological dataset of basic meteorological elements of China National Surface Weather Station, and the daily global and net radiation in the daily radiation dataset of China, which were downloaded from the China Meteorological Data Network1 (Figure 2). The elevation data were SRTM DEM data with a spatial resolution of 90 m, which were downloaded from the Geospatial Data Cloud2. All the data were finally processed into the spatial resolution of 0.01 degree in view of the difference in the resolution between the remote sensing and DEM data.
Estimation of Solar Incident Radiation
The solar incident radiation under complex terrain conditions consists of direct radiation, diffuse sky radiation, and reflected radiation from the surrounding terrain, as shown in Equation (1). This study estimated the instantaneous solar incident radiation amount and then extended it to the daily solar incident radiation amount by accumulating it over the sunrise and sunset time angles since the solar incident radiation varies with time during the day.
where Rs is the solar incident radiation, Rdir is the direct radiation, Rdif is the diffuse sky radiation, and Rref is the reflected radiation from the surrounding terrain.
Estimation of Direct Radiation
Direct radiation is affected by the incidence angle on slope I (i.e., the angle between the solar ray and the normal slope surface). Under the condition of I ≥ 90°, Rdir is 0 as the target point is completely in shadow; under the condition of 0° ≤ I < 90°, the algorithm of Rdir is as follows (Wang, 2011):
where Vs is the shading coefficient, τ is the direct radiation transmissivity, Gsc is the solar constant (1367 W⋅m–2), is the correction coefficient of the Earth-Sun distance, and cosI represents the influence of the incident angle on the slope surface.
The shading coefficient Vs is the area proportion of solar radiation received by the target point, and the value of 1 indicates there is no shading of the surrounding terrain, and the value of 0 indicates the target point is completely shaded. This coefficient was calculated with the algorithm of the Hillshade function using the ArcGIS software as follows:
where z is the solar zenith angle (radians); s is the slope gradient (radians); α is the solar azimuth angle (radians), with 0 (2π), π, π/2, and 3π/2 representing the due east, due west, due north, and due south, respectively; γ is the slope direction (radians), with the range of [0–2π]. Specifically, s and γ were calculated with the methods in the algorithm of the Hillshade function using the ArcGIS software; z and α were calculated with the algorithms of Wang (1999) and Allen et al. (2007) as follows:
where δ is the solar declination (radians); φ is the geographical latitude (radians); ω is the solar time angle (radians); h is the solar altitude (radians), which is reciprocal with z; DOY is the annual cumulative day; and t is the local time. Notably, the value of α obtained with this method is 0 (2π) for the due south, π/2 for the due west, π for the due north, and 3π/2 for the due east, so these directions should be converted when they are substituted into Equation (3).
The clear-sky direct radiation transmissivity was estimated with the empirical equation proposed by Kreith and Kreider (1978) for calculating the atmospheric transparency coefficient as follows (Kreith and Kreider, 1978):
where Mh is the atmospheric volume below a certain elevation. M0 is the atmospheric volume at the sea level, and is the atmospheric pressure correction factor, which were calculated as follows (Kreith and Kreider, 1978; List, 1984):
where dem is the altitude (m).
Additionally, this study corrected τ during November and February into kτ since τ is affected by the season (Chen et al., 2009), and k was set to be 1.8198 according to the previous research (Liu et al., 2021).
The correction coefficient of the Earth–Sun distance was calculated with the algorithm of Wang (1999) as follows:
where Year is the concerned year, and INT denotes the integer function.
The influence of the incident angle on the slope surface was calculated as follows (Duffie and Beckman, 1991):
where the slope direction γ ranges between [−π, π] and 0, −π/2, π/2, and ± π, which represent the south, east, west, and north directions, respectively.
Estimation of the Diffuse Sky Radiation
The surface cannot receive all the diffuse radiation from the space hemisphere under the influence of the terrain under conditions of arbitrary terrain, and this study introduced the sky view factor to determine the sky range where the diffuse radiation can be received based on the algorithm of Allen et al. (2006) as follows:
where fi is the sky view factor under conditions of isotropic scattering; KD is the transmissivity coefficient of scattered radiation, which is related to the broad-band atmospheric transmissivity KB (Asce-Ewri, 2005; Allen et al., 2010), when KB ≥ 0.15, KD = 0.35−0.36 × KB, and when KB < 0.15, KD = 0.18 + 0.82 × KB.
where Kt is the atmospheric turbidity coefficient, the value of which is 1 under clear-sky conditions; pre is the atmospheric precipitable water vapor (mm); Pair is atmospheric pressure (kPa).
Estimation of the Reflected Radiation From the Surrounding Terrain
The land surface receives solar radiation reflected from the surrounding terrain under conditions of arbitrary terrain, especially in complex terrain areas. The reflected radiation from the surrounding terrain is influenced by the surface albedo of the surrounding terrain, slope direction, and the sky view factor, and it can be calculated as follows (Allen et al., 2010):
where albedo is the ground albedo of the adjacent pixel corresponding to the slope direction of the target point.
Estimation of the Net Surface Radiation
According to the algorithm of daily net radiation recommended in FAO56 (Allen et al., 1998), the daily net radiation is calculated as the difference between the daily short-wave net radiation and daily long-wave net radiation as follows:
where Rn, Rns, and Rnl are the net daily radiation, net daily short-wave radiation, and net daily long-wave radiation (MJ⋅m–2⋅day–1), respectively.
where Rs is the solar incident radiation (MJ⋅m–2⋅day–1) and albedo is the surface albedo, which can be extracted from the 7-band reflectance of the MOD09GA product as follows (Tasumi et al., 2008):
where αi is the 7-band reflectance of the MOD09GA product, and i = 1, 2,., 7.
Rnl was corrected based on the algorithm recommended by the FAO as follows:
where σ is the Stefan–Boltzmann constant, which is 4.903 × 10–9 MJ⋅K–4⋅m–2⋅day–1; Tmax, Tmin, and ea are the maximum temperature, minimum temperature (K), and the actual water vapor pressure (kPa), respectively, which were inversed from the MOD05L2 product. Rso is the clear-sky solar short-wave radiation (MJ⋅m–2⋅day–1). is 1 under clear-sky conditions; k and b are coefficients obtained by fitting the station observations.
Correction of the Daily Solar Long-Wave Net Radiation Coefficient
According to the surface net radiation calculation [Equations (20)–(23)], the relationship among the radiation, air temperature, and air–water vapor pressure under clear-sky conditions is as follows:
assuming , , then y = −kx + b, where ea was estimated with the algorithm in the FAO56. The linear relationship between y and x was then fitted with the albedo data inverted from the MOD09GA product, the daily solar incident radiation and daily net radiation data observed at radiation stations on clear-sky days, and the maximum temperature, minimum temperature, and relative humidity data observed at meteorological stations at corresponding locations, based on which the values of k and b were thereafter determined.
Remote Sensing Inversion of the Meteorological Factors
The atmospheric precipitable water vapor data at the corresponding locations of meteorological stations in the Haihe River Basin were extracted from the MOD05L2 product. Then the mathematical relationships among the maximum temperature, minimum temperatures, and the atmospheric precipitable water were established separately for the spring (March–May), summer (June–August), autumn (September–November), and winter (December–February) [Equations (25) and (26)]. Thereafter, the spatially explicit regional meteorological data can be inferred according to the atmospheric precipitable water vapor data based on these mathematical relationships.
where Tmin and Tmax are the daily minimum temperature and maximum temperature (°C), respectively, and pre is the atmospheric precipitable water vapor (cm).
There is generally a close mathematical relationship between the actual water vapor pressure and atmospheric precipitable water, and it is feasible to establish the functional relationship between the actual water vapor pressure observation data at meteorological stations and the atmospheric precipitable water data in the MOD05L2 product, based on which the spatially explicit regional water vapor pressure can be inverted. In this study, the actual water vapor pressure data at meteorological stations in the Haihe River Basin from 2001∼2019 were estimated with the data of relative humidity, maximum temperature, and minimum temperature available at meteorological stations as follows:
where ea is the actual water vapor pressure (kPa), RH is the relative humidity (%); T, Tmin, and Tmax are the temperature, minimum temperature, and maximum temperature (°C), respectively.
Evaluation of the Estimation Accuracy
This study evaluated the estimation accuracy by comparing the estimated results with the observation data from radiation stations in the Haihe River Basin. Specifically, the correlation coefficient and the root mean square error were used to represent the estimation accuracy of the daily incident solar radiation and daily net radiation under clear-sky conditions from 2001∼2019 as follows:
where R is the correlation coefficient, RMSE is the root mean square error, n is the total number of estimated radiation data to be verified, xi is the site observation value, yi is the estimated value, is the mean of the site observation values, and is the mean of the estimated values.
Results and Discussion
Relationship Between the Meteorological Factors and the Remote Sensing Data
Figure 3 suggests that there was a remarkable seasonal variation of the atmospheric precipitable water vapor and temperature in the Haihe River Basin, which showed a significant correlation. Specifically, there was a very significant seasonal variation of the atmospheric precipitable water, which reached the maximum value above 8 cm in summer, the minimum value generally below 1 cm in winter, and the medium value below 4 cm in spring or autumn. The magnitude of temperature variation with atmospheric precipitation varied significantly in different seasons, specifically, the temperature variation was stronger when the atmospheric precipitable water was very low, but it gradually tended to level off when the atmospheric precipitable water exceeded 1 cm.
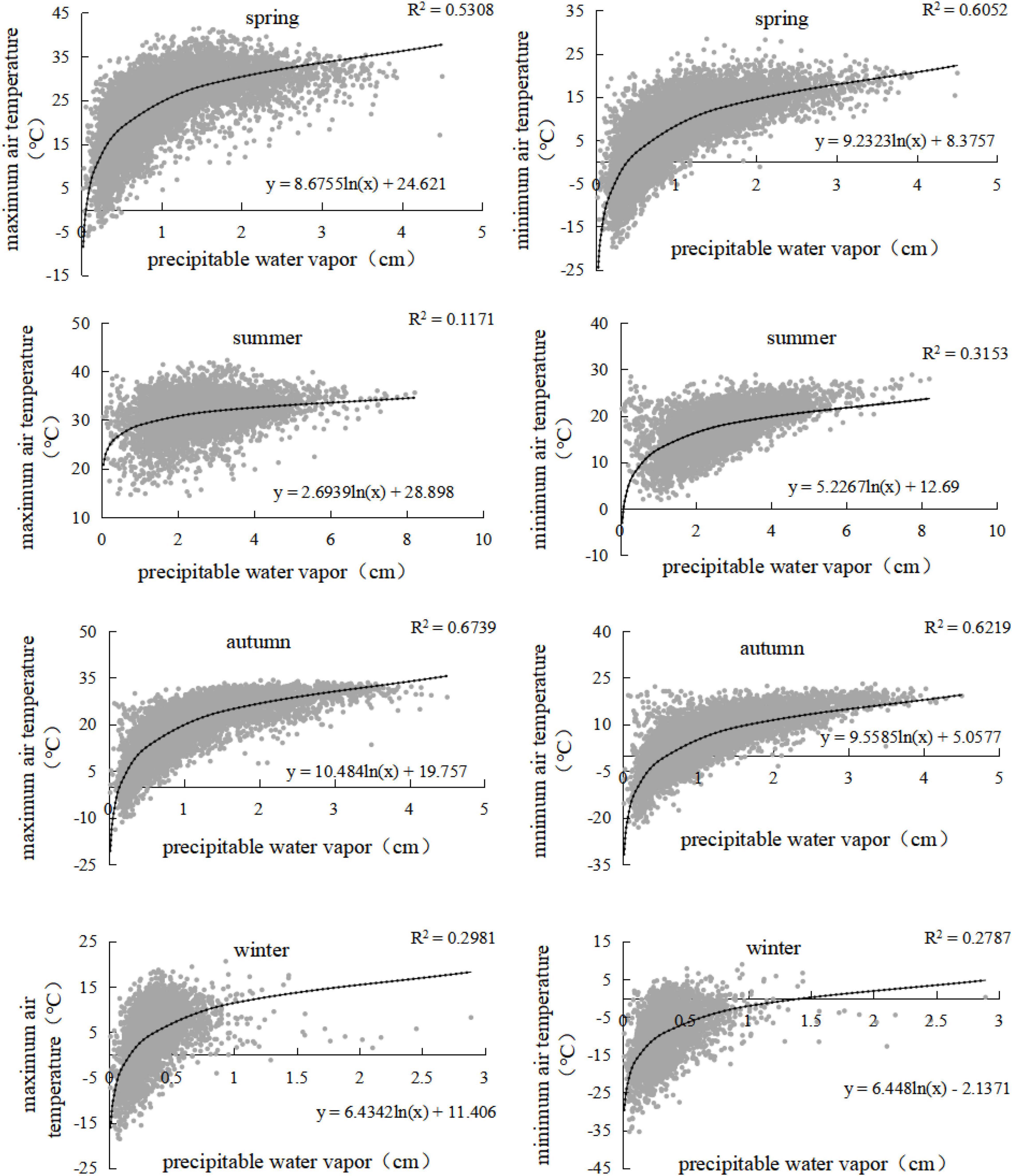
Figure 3. Relationship between the temperature and atmospheric precipitable water by season in Haihe River Basin.
It is more accurate to reveal the mathematical relationship between temperature and atmospheric precipitable water vapor by fitting them by season, and the fitting results based on the atmospheric precipitable water vapor data and the MOD05L2 product at the location of meteorological stations in the Haihe River Basin from 2001∼2019 suggested that there was an obvious logarithmic relationship between the air temperature and atmospheric precipitable water vapor as shown in Equations (31) and (32). In particular, the significant correlation between air temperature and atmospheric precipitable water vapor varied with seasons. Specifically, the correlation between the air temperature and atmospheric precipitable water vapor was high in spring and autumn and low in summer and winter, with the correlation coefficient between 0.7 and 0.8 and 0.3 and 0.6, respectively.
where a1, b1, a2, and b2 are the fitted coefficients. Specifically, in spring, a1 = 9.2323, b1 = 8.3757, a2 = 8.6755, and b2 = 24.621; in summer, a1 = 5.2267, b1 = 12.69, a2 = 2.6939, and b2 = 28.898; in autumn, a1 = 9.5585, b1 = 5.0577, a2 = 10.484, and b2 = 19.757; and in winter, a1 = 6.448, b1 = −2.1371, a2 = 6.4342, and b2 = 11.406.
The relationship between the actual water vapor pressure and atmospheric precipitable data is shown in Equation (33) and Figure 4, which was revealed on the basis of the actual water vapor pressure data and the atmospheric precipitable data extracted from the MOD05L2 product at the location of meteorological stations from 2001∼2019.
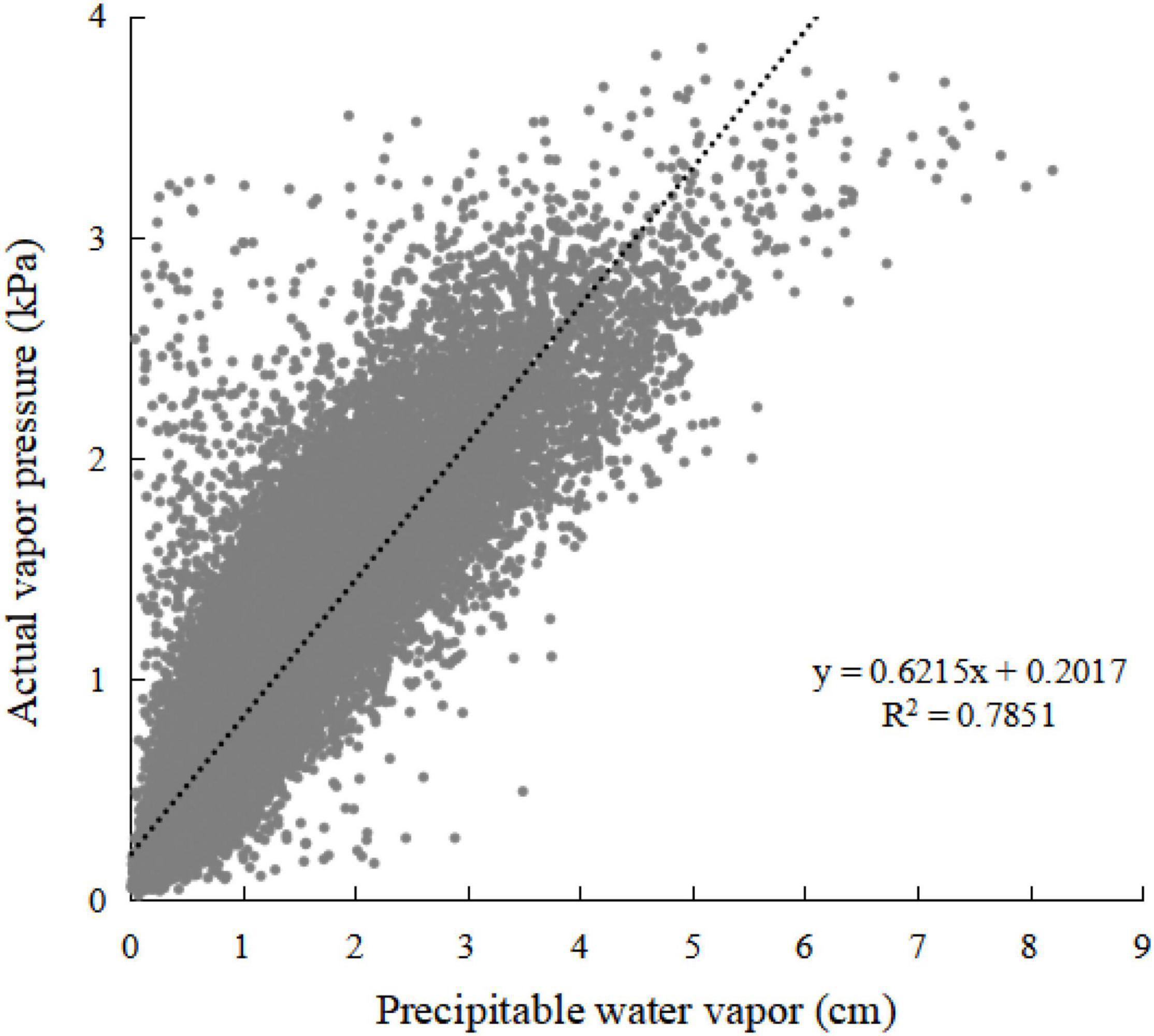
Figure 4. Relationship between the actual vapor pressure and atmospheric precipitable water in Haihe River Basin.
Verification of the Solar Radiation Inversion
Correction Coefficients of the Daily Long-Wave Net Radiation
The correction coefficients k and b in the daily long-wave net radiation algorithm were obtained by fitting the meteorological data and radiation data observed at the stations and the albedo data extracted from the remote sensing data. The results shown in Figure 5 suggested k and b were 0.1042 and 0.3821, respectively. The long-wave net radiation algorithm recommended by the FAO was localized with the correction coefficients k and b, making it more applicable to the Haihe River Basin.
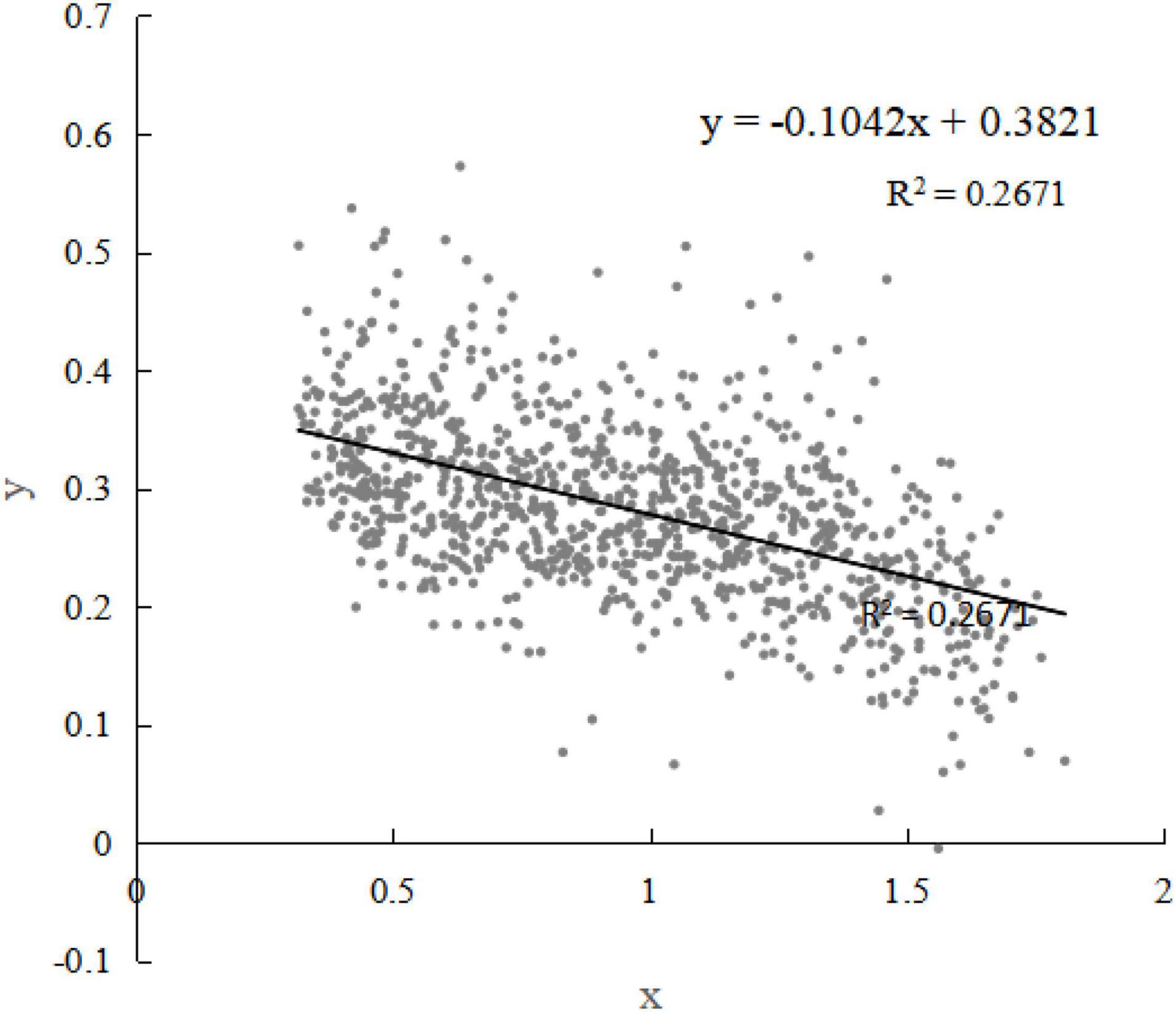
Figure 5. Fitting curve of the correction coefficients k and b in the daily long-wave net radiation algorithm.
Verification of the Estimated Solar Radiation
There are four radiation observation stations in the Haihe River Basin (Figure 2), i.e., Beijing station (39.48°N, 116.28°E, altitude 31.3 m), Datong station (40.06°N, 113.2°E, altitude 1,067.2 m), Laoting station (39.26°N, 118.53°E, altitude 10.5 m), and Tianjin station (39.05°N, 117.04°E, altitude 2.5 m). Specifically, Beijing, Laoting, and Tianjin stations provided both the daily solar radiation and net radiation observation data, while Datong station provided only the daily solar radiation observation data. Meanwhile, the Beijing station provided more comprehensive observation available for the full period of 2001∼2019, while the other three stations only provided observation data available from 2001 to 2010.
The estimated results of the solar incident radiation and daily net radiation under clear-sky conditions from 2001∼2019 were verified with the available observation data of these radiation stations (Figures 6, 7). The correlation coefficients between the estimated and observed daily solar incident radiation at Beijing, Datong, Laoting, and Tianjin stations reached 0.90, 0.91, 0.91, and 0.90, respectively, with the root mean square errors of 3.39 MJ/(m2⋅day), 3.55 MJ/(m2⋅day), 3.18 MJ/(m2⋅day), and 3.38 MJ/(m2⋅day), respectively. The correlation coefficients between the estimated and observed daily net radiation at Beijing, Laoting, and Tianjin stations were 0.90, 0.90, and 0.89, respectively, with the root mean square errors of 2.80 MJ/(m2⋅day), 3.08 MJ/(m2⋅day), and MJ/(m2⋅day), respectively. Overall, the estimated incident solar radiation and net daily radiations were highly consistent with the observed ones, with the estimation accuracy within the acceptable range.
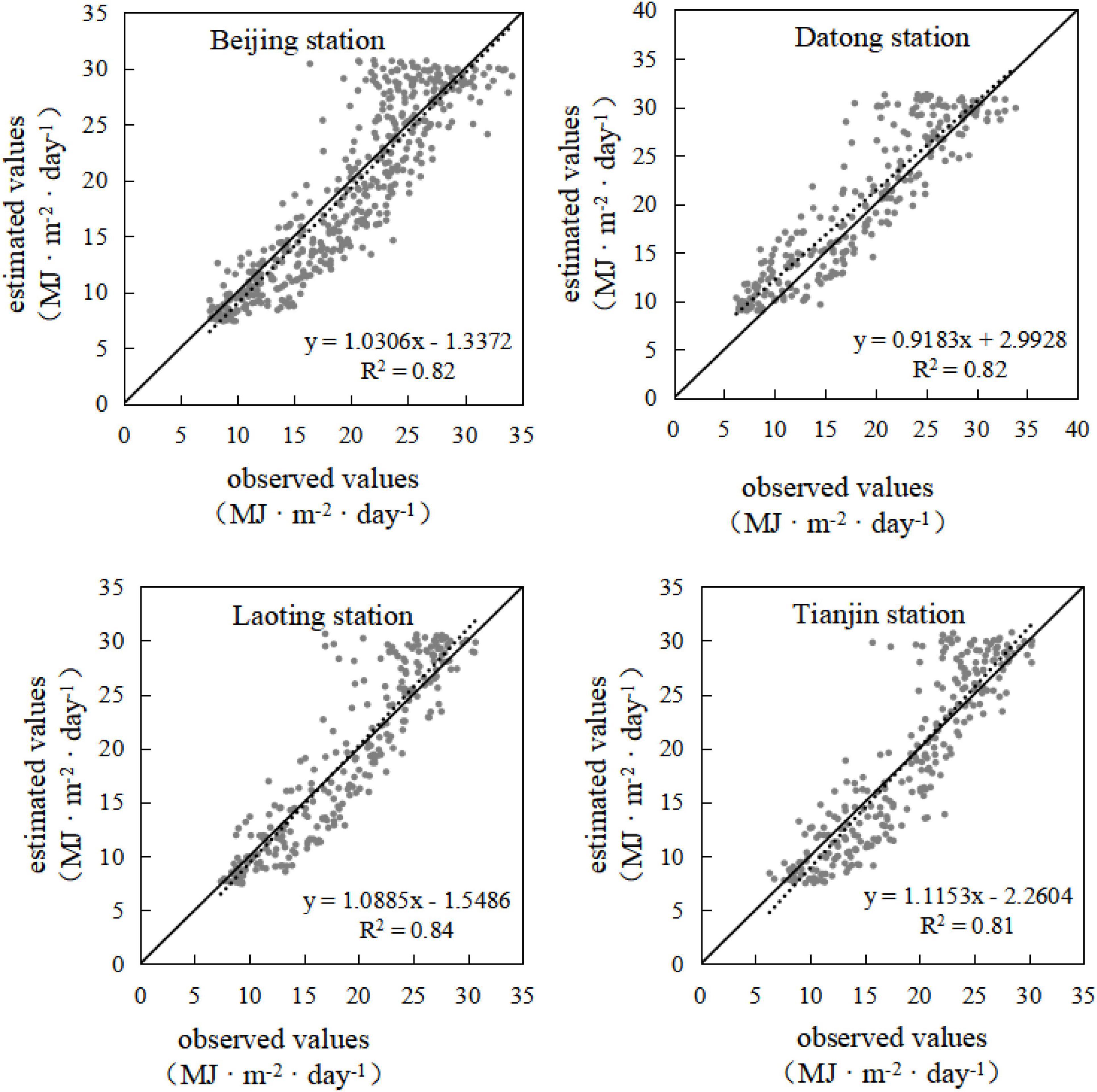
Figure 6. Comparison of the estimated and observed daily solar incident radiation of the Haihe River Basin.
Spatiotemporal Variation of Solar Radiation in the Haihe River Basin
Temporal Variation of Solar Radiation
The average solar radiation of the Haihe River Basin showed no significant inter-annual changing trends but remarkable intra-annual fluctuation during 2001∼2019 (Figure 8). Specifically, the annual average values of the daily solar incident radiation, daily long-wave net radiation, and daily net radiation under clear-sky conditions in the Haihe River Basin from 2001∼2019 ranged between 16.6 and 20.9 MJ/(m2⋅day), 9.1 and 9.5 MJ/(m2⋅day), 4.8 and 8.2 MJ/(m2⋅day), respectively. Overall, the solar incident radiation and net radiation under clear-sky conditions showed significant inter-annual variation, but their annual average values showed no obvious changing trends, which only increased slightly, with the variation slopes of 0.08 and 0.07, respectively.
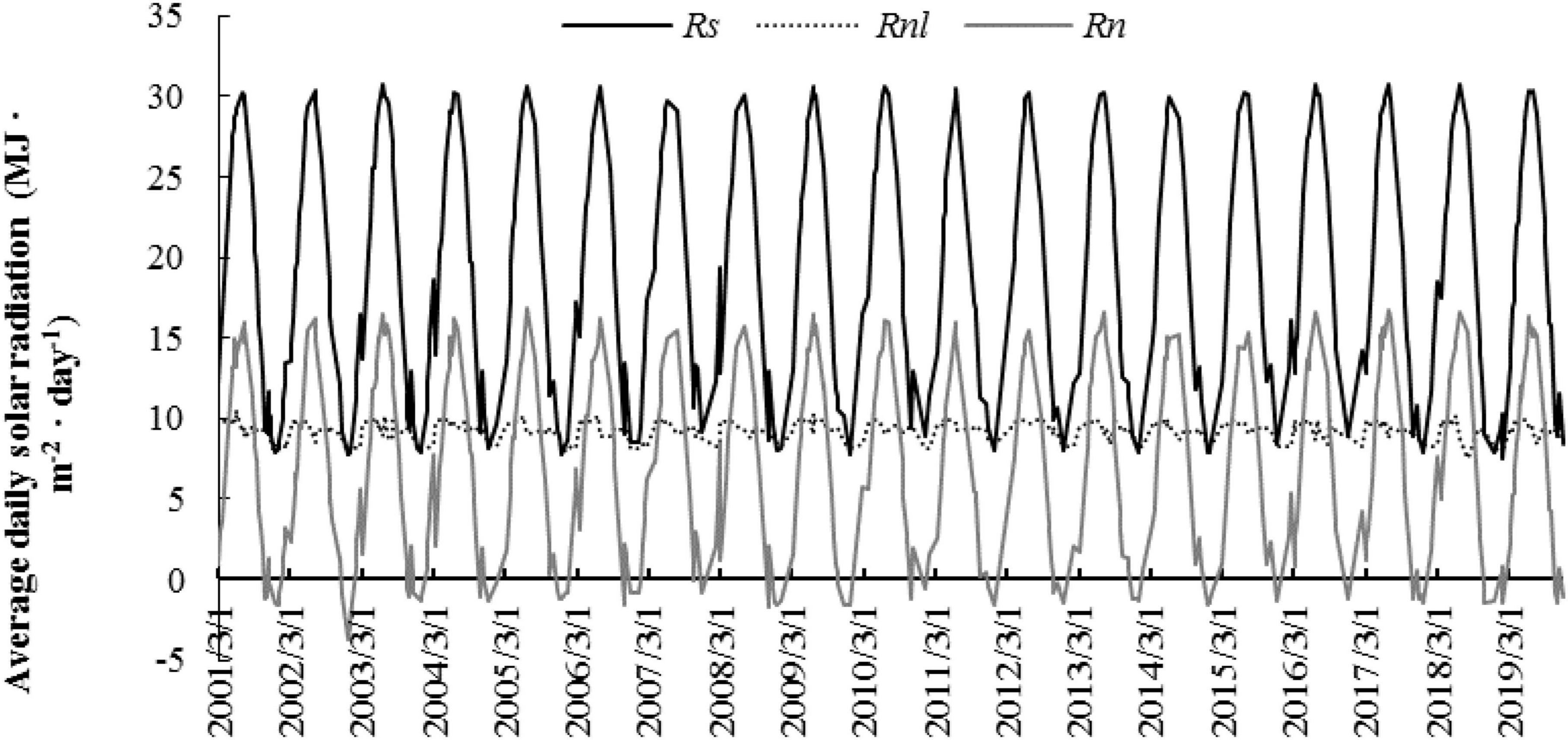
Figure 8. The average values of daily solar incident radiation (Rs), daily long-wave net radiation (Rnl), and daily net radiation (Rn) under clear-sky conditions from 2001∼2019 in the Haihe River Basin.
There was a very significant seasonal variation in the solar radiation of the Haihe River Basin, and the average daily solar incident radiation and daily net radiation under clear-sky conditions were the highest in summer and the lowest in winter, basically showing a single-peak pattern. Specifically, the seasonal average values of daily solar incident radiation, daily net radiation, and daily long-wave net radiation ranged between 7.5 and 30.8 MJ/(m2⋅day), −3.8 and 16.9 MJ/(m2⋅day), and 7.5 and 10.6 MJ/(m2⋅day), respectively. More specifically, the average values of daily solar incident radiation, daily net long-wave radiation, and daily net radiation in spring from 2001∼2019 ranged between 20.4 and 25.4 MJ/(m2⋅day), 9.6 and 9.8 MJ/(m2⋅day), and 7.4 and 11.5 MJ/(m2⋅day), respectively. In summer, the solar incident radiation and daily net radiation increased to some extent, with the daily average value ranging between 26.6 and 30.3 MJ/(m2⋅day) and 13.1 and 16.0 MJ/(m2⋅day), respectively, while the daily long-wave net radiation decreased to some extent, with the average value ranging between 8.8 and 9.8 MJ/(m2⋅day). In contrast, in autumn, the solar incident radiation and daily net radiation were relatively lower, with average daily values fluctuating between 12.1 and 16.0 MJ/(m2⋅day) and 1.2 and 4.7 MJ/(m2⋅day), respectively, while the daily long-wave net radiation fluctuates between 9.1 and 9.3 MJ/(m2⋅day). The lowest values of solar incident radiation, net daily longwave radiation, and net daily radiation all occurred in winter, with the average daily values ranging between 8.0 and 16.5 MJ/(m2⋅day), 8.1 and 8.4 MJ/(m2⋅day), and −1.5 and 5.7 MJ/(m2⋅day), respectively. Moreover, the seasonal solar radiation showed no significant changing trends during the study period, and the solar incident radiation in spring and the net daily radiation in summer and winter increased most remarkably, but with the slopes of growth reaching only 0.05 and 0.03, respectively.
Spatial Heterogeneity of Solar Radiation in the Haihe River Basin
The solar incident radiation at the surface differed greatly among regions in the Haihe River Basin, and the net surface radiation in the Haihe River Basin showed complex spatial heterogeneity under the influence of multiple factors such as location and terrain (Figure 9). Taking the solar radiation under clear-sky conditions in different seasons of 2017 as an example (Figure 9), this study suggested there was an obvious difference in solar incident radiation and net surface radiation in mountainous areas, where the difference between the highest and lowest solar incident radiation exceeded 11 MJ/(m2⋅day) and the difference between the highest and lowest net surface radiation even exceeded 16 MJ/(m2⋅day). On the one hand, the solar incident radiation is closely related to the latitude. In general, the lower the latitude is, the larger the solar altitude angle is, and the more the solar radiation reaching the ground is, and vice versa, and this study also suggested the solar incident radiation in the Haihe River Basin showed an overall decreasing trend from south to north (Figure 9). On the other hand, the daily outgoing long-wave net radiation is positively correlated with the temperature, which is also closely related to the latitude. Generally, the lower the latitude is, the higher the temperature is, and vice versa, which can offset the influence of solar incident radiation on the net surface radiation in latitudinal zones to a certain extent. As a result, this study suggested the spatial difference in the net surface radiation between latitudinal zones from south to north was not as significant as that of the solar incident radiation in the Haihe River Basin (Figure 9).
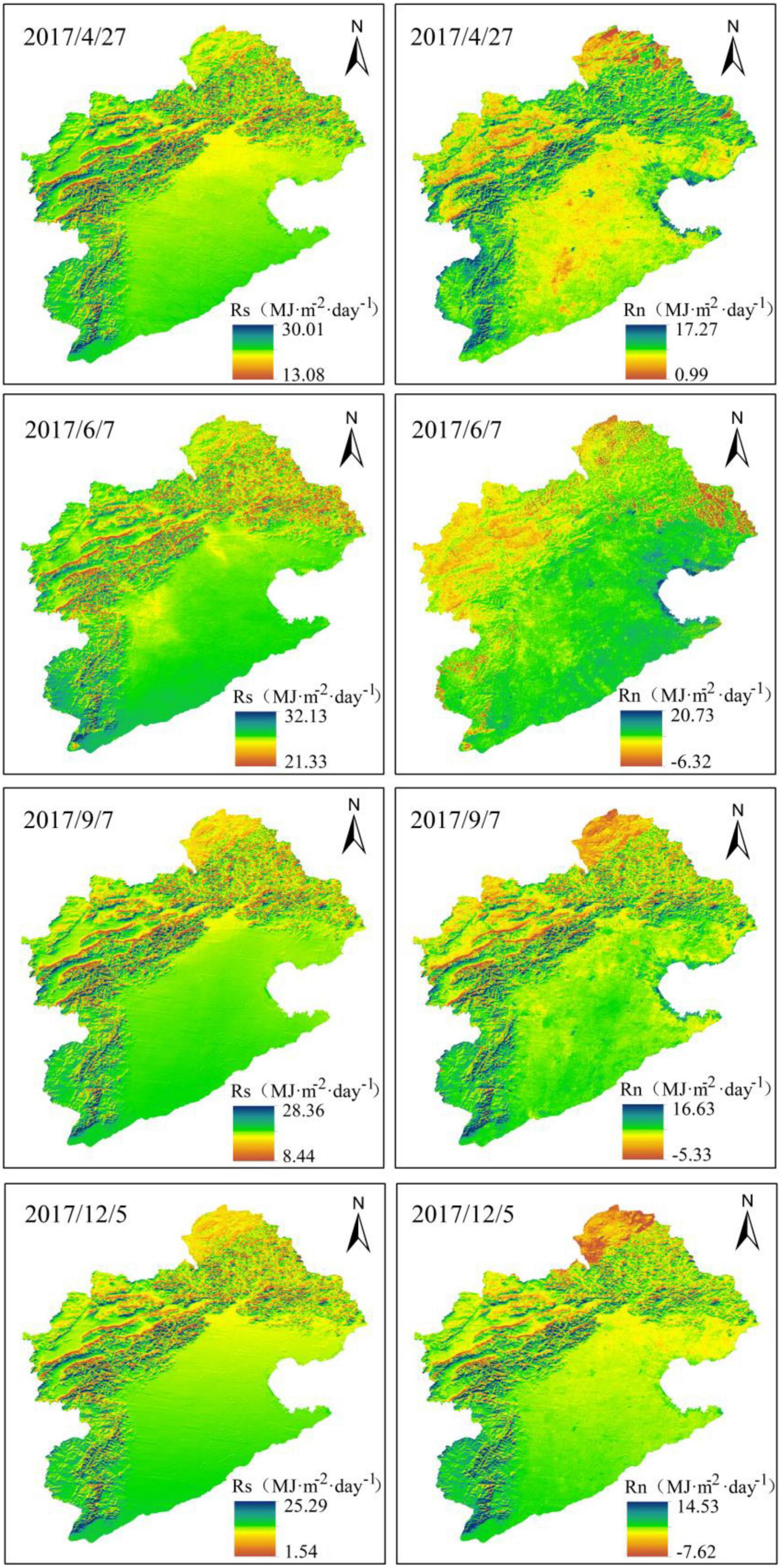
Figure 9. Spatial heterogeneity of the daily solar incident radiation (Rs) and the surface net radiation (Rn) in Haihe River Basin.
There is very significant spatial heterogeneity of terrain in the Haihe River Basin, which had a very important influence on the solar radiation. Specifically, there are mainly plains with low elevation in the eastern and southern parts of the Haihe River Basin, where the simple terrain has very limited influence on both the solar incident radiation and net surface radiation. By contrast, there are the Yanshan-Taihang Mountains in the western and northern part of the Haihe River Basin, with a high altitude and very complex terrain variation, and the lowest and highest values of solar incident radiation both occurred in these mountainous areas under the influence of terrain shading and slope direction. On the one hand, there is a limited weakening effect of the atmosphere on the solar incident radiation in these mountainous areas with the high altitude, long sunshine hours, and thin air, and therefore the solar incident radiation in mountainous areas is slightly higher than that in plain areas at the same latitude. On the other hand, the sunshine hours and intensity of received solar radiation varied greatly among different slope gradients and directions. The closer to 90° the solar altitude angle on the slope is, the more the solar incident radiation is. The less the influence of the terrain shading on different slopes is, the longer the sunshine hour is, and the more solar incident radiation is. The variation of solar incident radiation is therefore much more significant in areas with strong terrain variation in these mountainous areas than the plain areas. Additionally, the daily net long-wave radiation is affected by both the temperature and water vapor pressure. In mountainous areas with high altitudes, the temperature is relatively lower and the actual water vapor pressure is also lower due to the lower water vapor content under clear-sky conditions, which jointly lead to a higher daily net long-wave radiation and slightly lower daily net radiation in the mountainous areas with little terrain variation than the plain areas. Nevertheless, the solar incident radiation on the sunny slopes of areas with strong terrain variation in mountainous areas is still much higher than that on the plains, and the highest daily surface net radiation still occurred on these sunny slopes of areas with strong terrain variation in mountainous areas.
Variation of Daily Solar Radiation on Different Slope Directions
There is a remarkable difference in the solar incident radiation received by the ground surface on different slopes in the Haihe River Basin under the influence of terrain. There is a more remarkable difference in the sunshine hour and the percentage of solar incident radiation received on a unit area on different slopes under the influence of terrain shading. This study generated the slope direction map of Haihe River Basin with the slope direction tool in the ARCGIS software to clarify the surface radiation budget of different slope directions, according to which the study area was divided into eight directions, i.e., N (0-π/8, 15π/8-2π), NE (π/8-3π/8), E (3π/8-5π/8), SE (5π/8), and SE (5π/8). 8), SE (5π/8-7π/8), S (7π/8-9π/8), SW (9π/8-11π/8), W (11π/8-13π/8), and NW (13π/8-15π/8), and thereafter this study extracted the solar radiation under the clear-sky condition on different slope directions.
The results based on the average daily solar radiation under clear-sky conditions from 2001∼2019 suggested that there was a similar descending rank order of the solar incident radiation and the surface net radiation on different slope directions, i.e., south, southwest, west or southeast, northwest, east, northeast, and north (Figure 10). Specifically, the south slope received the highest solar radiation, followed by the southwest slope, but the difference between them was very slight, reaching only about 0.03 MJ/(m2⋅day). There was similar solar incident radiation on the west and southeast slopes, which was about 0.13 MJ/(m2⋅day) lower than that on the north slope. The solar incident radiation on the northwest slope was about 0.1–0.2 MJ/(m2⋅day) higher than that on the east slope and about 0.1 MJ/(m2⋅day) lower than that on the southeast slope. The solar incident radiation on the northeast slope was about 0.6–0.7 MJ/(m2⋅day) lower than that on the south slope, but it was about 0.3MJ/(m2⋅day) higher than that on the east slope. The north slope received the least solar incident radiation, which was about 1 MJ/(m2⋅day) lower than that on the south slope.
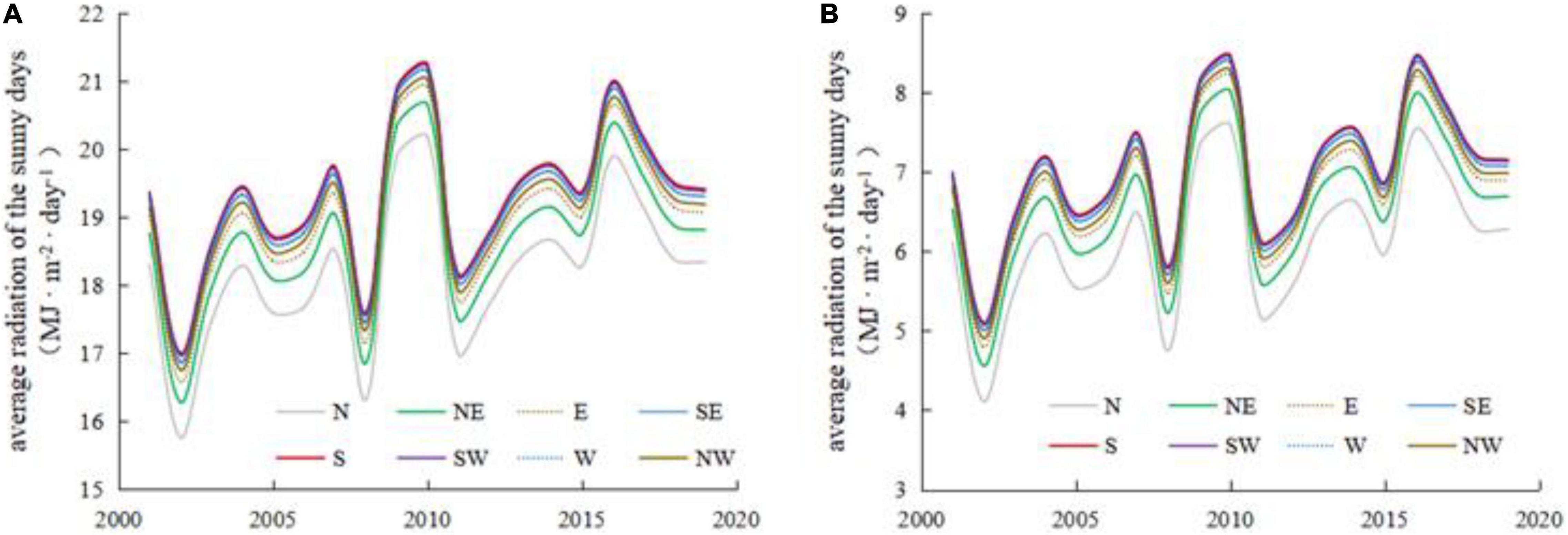
Figure 10. Average daily solar radiation under clear-sky conditions on different slopes from 2001∼2019 in the Haihe River Basin. (A) Solar incident radiation; (B) Surface net radiation.
Notably, the difference in the average surface net radiation under clear-sky conditions on different slopes varied remarkably from year to year due to the variation in the total number of clear-sky days and their distribution in different seasons in different years. Specifically, the difference between the surface net radiation in the north and south directions was about 0.8–1.1 MJ/(m2⋅day) in different years. While the differences in net surface radiation on the southwest, west or southeast, northwest, east, and northeast slopes compared to the south slope reached about 0.02–0.03 MJ/(m2⋅day), 0.07–0.11 MJ/(m2⋅day), 0.16–0.21 MJ/(m2⋅day), 0.24–0.34 MJ/(m2⋅day), and 0.43–0.59 MJ/(m2⋅day), respectively. Additionally, Figure 10 shows that the annual average solar incident radiation and the surface net radiation under clear-sky conditions were higher in 2009, 2010, 2016, and 2017 and lower in 2002, 2008, and 2011. Specifically, the annual average solar radiation was the highest in 2010 and the lowest in 2002, and the difference between the solar incident radiation and the surface net radiation on different slopes reached about 4.2–4.4 MJ/(m2⋅day) and 3.4–3.5 MJ/(m2⋅day), respectively. This is mainly due to the fact that the sunny days in 2010 were mainly concentrated in May–October, while the sunny days in 2002 were mostly concentrated in January–May and September–December.
Discussion
The method used in this study can more accurately reveal the spatial heterogeneity of solar radiation under complex terrain conditions than the methods in previous studies. The regional meteorological data in previous studies were mostly obtained with the spatial interpolation methods, and some scholars took into account the influence of the elevation but generally failed to reflect the influence of the shaded slope. In contrast, this study improved the estimation accuracy of the meteorological factors in the Haihe River Basin by inverting the maximum and minimum temperatures and the actual water vapor pressure based on the remote sensing data, the MOD05L2 product, which can better reflect the spatial distribution of these meteorological factors. Compared with the traditional spatial interpolation method of meteorological factors used by previous scholars and even the GIDW method, which is optimized by considering the elevation factor, the method used in this study can better reflect the influence of terrain variation on the meteorological factors in areas with complex terrain, especially the meteorological difference between shaded and sunny slopes resulting from the complex terrain. Besides, this study significantly improves the estimation accuracy of the solar incident radiation in complex terrain areas by fully considering the solar incident radiation from three aspects, i.e., direct radiation, diffuse sky radiation, and reflected radiation from the surrounding terrain, amending the influence of the cosine value of the incident angle on the slope based on multiple factors, e.g., the slope gradient, slope direction and latitude, and introducing the shading coefficients to amend the influence of shading caused by the shadow of the surrounding terrain. In addition, this study has localized some key parameter values in the daily net long-wave radiation algorithm recommended by the FAO, which further improves the estimation accuracy. More importantly, this study has carried out the verification of the estimated solar radiation with the long time series observation data, which is more convincing than previous studies with the short time data.
There are still some uncertainties in this study due to the data limitation, etc. First, there is a limited number of meteorological stations available for the model verification, especially in the mountainous areas with complex terrain. Besides, there are some uncertainties resulting from the inherent error of the remote sensing data and the problems of mixed pixels due to the limited spatial resolution of the remote sensing data. In particular, there may be a remarkable difference in the quality of the remote sensing data under clear-sky conditions. In addition, the method used in this study is only applicable to the clear-sky conditions due to the limitation of the remote sensing data availability and the transmittance coefficient algorithm, and it is necessary to carry out further research for the cloudy and overcast conditions. It is therefore the next agenda of this study to explore how to extend the daily-scale surface radiation data to monthly and annual scales. Nevertheless, the results of this study can still provide important methodological support for the estimation of the surface radiation budget and relevant studies of agriculture, ecology, hydrology, and meteorology in mountainous areas with complex terrain.
Conclusion
This study estimated the surface net radiation under clear-sky conditions in the Haihe River Basin as an area with complex terrain from 2001∼2019 based on the topographic data, the remote sensing data, and the temperature and water vapor pressure data inverted with the remote sensing data. The main conclusions are as follows: (1) This study effectively improved the estimation accuracy of the surface net radiation algorithm by comprehensively considering the impacts of complex terrain, underlying surface and atmosphere on the surface net radiation, and localizing the parameter values in the algorithm of net long-wave radiation recommended by the FAO, with the correlation coefficient between the estimated surface net radiation and the observed value reaching approximately 0.9 and the root mean square error reaching about 3 MJ/(m2⋅day). (2) The average daily solar incident radiation and daily net radiation under clear-sky conditions in the Haihe basin were the highest in summer and the lowest in winter, showing an overall single-peak pattern, and the annual average solar radiation was the highest in 2010 and the lowest in 2002. (3) The solar incident radiation and net surface radiation in the Haihe River Basin showed complex spatial heterogeneity. On the one hand, the lowest and highest values of solar incident radiation both occurred in the mountainous areas in the western and northern part of the Haihe River Basin. On the other hand, the solar radiation varied significantly on different slopes, and there was a similar descending rank order of the solar incident radiation and the surface net radiation on different slope directions in the Haihe River Basin, i.e., south, southwest, west or southeast, northwest, east, northeast, and north. (4) The method used in this study is only applicable to the clear-sky conditions due to the limitation of the remote sensing data availability and the transmittance coefficient algorithm, and it is the next agenda of this study to carry out further research for the cloudy and overcast conditions and explore how to extend the daily-scale surface radiation data to monthly and annual scales. Overall, it is necessary to further improve the algorithm used in this study, but this study can still provide important methodological support for the estimation of solar radiation in areas with complex terrain in the relevant fields.
Data Availability Statement
The original contributions presented in this study are included in the article/supplementary material, further inquiries can be directed to the corresponding author/s.
Author Contributions
XL and HaY: conceptualization, data curation, investigation, resources, funding acquisition, project administration, and supervision. XL and JZ: methodology, software, validation, visualization, and writing—original draft. JZ and HuY: writing—review and editing and formal analysis. All authors contributed to the article and approved the submitted version.
Funding
This research was funded by the National Natural Science Foundation of China (42101040 and 51909052); the Natural Science Foundation of Hebei Province (D2019403022); the Science and Technology Project of Hebei Education Department (BJ2019045); and the Scientific and Technological Innovation Team Project of Hebei GEO University in 2021 (KJCXTD-2021-10).
Conflict of Interest
The authors declare that the research was conducted in the absence of any commercial or financial relationships that could be construed as a potential conflict of interest.
Publisher’s Note
All claims expressed in this article are solely those of the authors and do not necessarily represent those of their affiliated organizations, or those of the publisher, the editors and the reviewers. Any product that may be evaluated in this article, or claim that may be made by its manufacturer, is not guaranteed or endorsed by the publisher.
Footnotes
References
Allen, R. G., Pereira, L. S., Raes, D., and Smith, M. (1998). FAO Irrigation and drainage paper No.56. Rome: Food and Agriculture Organization of the United Nations.
Allen, R. G., Tasumi, M., and Trezza, R. (2007). Satellite-based energy balance for mapping evapotranspiration with internalized calibration (METRIC)-Model. J. Irrig. Drain. Eng. 133, 380–394. doi: 10.1061/(ASCE)0733-9437(2007)133:4(380)
Allen, R. G., Tasumi, M., Trezza, R., and Kjaersgaard, J. (2010). METRICtm: Mapping Evapotranspiration at High Resolution–Applications Manual. Kimberly: University of Idaho.
Allen, R. G., Trezza, R., and Tasumi, M. (2006). Analytical integrated functions for daily solar radiation on slopes. Agr. Forest Meteorol. 139, 55–73. doi: 10.1016/j.agrformet.2006.05.012
Asce-Ewri (2005). The ASCE Standardized Reference Evapotranspiration Equation. ASCE Reston, VA: ASCE-EWRI Task Committee Report.
Cao, W., Xu, Y., and Duan, C. F. (2014). Research on Applicability of Solar Radiation Parametric Model in Anhui Province. Chin. Agric. Sci. Bull. 30, 207–212.
Chen, L., Yan, G., Wang, T., Ren, H., Calbó, J., Zhao, J., et al. (2012). Estimation of surface short-wave radiation components under all sky conditions: modeling and sensitivity analysis. Remote Sens. Environ. 123, 457–469. doi: 10.1016/j.rse.2012.04.006
Chen, Y. Y., Qiu, X. F., Gao, Y. H., You, Y. S., and Liao, Q. L. (2009). A study on calculation of direct solar radiation transmission rate. Chinese J. Agrometeorol. 30, 492–495.
Dozier, J., and Frew, J. (1990). Rapid calculation of terrain parameters for radiation modeling from digital elevation data. IEEE T. Geosci. Remote 28, 963–969. doi: 10.1109/36.58986
Dubayah, R., and Rich, P. M. (1995). Topographic solar radiation models for GIS. Int. J. Geogr. Inf. Sci. 9, 405–419. doi: 10.1080/02693799508902046
Gharekhan, D., Nigam, R., Bhattacharya, B. K., Desai, D., and Patel, P. (2022). Estimating regional-scale daytime net surface radiation in cloudless skies from GEO-LEO satellite observations using data fusion approach. J. Earth. Syst. Sci. 131:73. doi: 10.1007/s12040-021-01806-9
Gui, S. (2010). Satellite Remote Sensing of Surface Net Radiation. Ph.D.thesis. Wuhan, China: Wuhan University.
Gui, S., Liang, S. L., Wang, K. C., Li, L., and Zhang, X. T. (2010). Assessment of Three Satellite-Estimated Land Surface Downwelling Shortwave Irradiance Data Sets. IEEE Geosci. Remote. S. 7, 776–780. doi: 10.1109/LGRS.2010.2048196
Guo, Y., and Shen, Y. J. (2015). Quantifying water and energy budgets and the impacts of climatic and human factors in the Haihe River Basin, China: 1. Model and validation. J. Hydrol. 528, 206–216. doi: 10.1016/j.jhydrol.2015.06.039
Hallikainen, M., and Kirimoto, T. (2008). Microwave Radiometry for Remote Sensing of the Earth Surfaces. Chofu, Japan: SICE Annual Conference, 20–22. doi: 10.1109/SICE.2008.4654601
Hao, D. L., Wen, J. G., Xiao, Q., Wu, S. B., Lin, X. W., You, D. Q., et al. (2019). Impacts of DEM geolocation bias on downward surface shortwave radiation estimation over clear-sky rugged terrain: a case study in Dayekou Basin. China. IEEE Geosci. Remote S. 16, 10–14. doi: 10.1109/LGRS.2018.2868563
Jia, A. L., Liang, S. L., Jiang, B., Zhang, X. T., and Wang, G. X. (2018). Comprehensive Assessment of Global Surface Net Radiation Products and Uncertainty Analysis. J. Geophys. Res. 123, 1970–1989. doi: 10.1002/2017JD027903
Kreith, F., and Kreider, J. F. (1978). Principles of Solar Engineering. New York: Taylor & Francis Inc.
Lei, H. M., Yang, D. W., and Huang, M. Y. (2014). Impacts of climate change and vegetation dynamics on runoff in the mountainous region of the Haihe River basin in the past five decades. J. Hydrol. 511, 786–799. doi: 10.1016/j.jhydrol.2014.02.029
Li, Y. Q., Chen, Y., Cao, W. J., Wang, X. Y., and Niu, Y. Y. (2022). Theoretical basis of ecology for the influence of global change on resources, environment, and eco-systems. J. Appl. Ecol. 33, 603–612. doi: 10.13287/j.1001-9332.202203.019
List, R. J. (1984). Smithsonian Meteorological Tables (6th reversion). Washington: Smithsonian Institution press.
Liu, X. R., Shen, Y. J., Li, H. J., Guo, Y., Pei, H. W., and Dong, W. (2017). Estimation of land surface evapotranspiration over complex terrain based on multi-spectral remote sensing data. Hydrol. Process. 31, 446–461. doi: 10.1002/hyp.11042
Liu, X. R., Zhang, H. Y., Yan, H. M., and Luo, J. M. (2021). Estimation of regional solar radiation over complex terrain. Acta Energ. Sol. Sin. doi: 10.19912/j.0254-0096.tynxb.2021-0127
Long, D., Gao, Y. C., and Singh, V. P. (2010). Estimation of daily average net radiation from MODIS data and DEM over the Baiyangdian watershed in North China for clear sky days. J. Hydrol. 388, 217–233. doi: 10.1016/j.jhydrol.2010.04.042
Matsui, H., and Osawa, K. (2015). Calibration effects of the net longwave radiation equation in Penman-type methods at Tateno, Japan. Hydrol. Res. Lett. 9, 113–117. doi: 10.3178/hrl.9.113
Pinker, R. T., Dan Tarpley, J., Laszlo, I., Mitchell, K. E., Houser, P. R., Wood, E. F., et al. (2003). Surface radiation budgets in support of the GEWEX Continental-Scale International Project (GCIP) and the GEWEX Americas Prediction Project (GAPP), including the North American Land Data Assimilation System (NLDAS) project. J. Geophys. Res. 108:8844. doi: 10.1029/2002JD003301
Ren, H. R., Luo, Y., and Xie, X. Q. (2006). Evaluation of application of several net radiation calculation methods in Huanghuaihai Plain. Trans. Chin. Soc. Agric. Eng. 22, 140–146.
Roupioz, L., Jia, L., Nerry, F., and Menenti, M. (2016). Estimation of daily solar radiation budget at kilometer resolution over the Tibetan Plateau by integrating MODIS data products and a DEM. Remote Sens. 8:504. doi: 10.3390/rs8060504
Sultan, S., Wu, R. G., Ahmad, I., and Ahmad, M. F. (2014). Modeling of diffuse solar radiation and impact of complex terrain over Pakistan using RS/GIS. J. Geogr. Inf. Syst. 6, 404–413. doi: 10.4236/jgis.2014.64035
Tang, W. J., Yang, K., Qin, J., Li, X., and Niu, X. L. (2019). A 16-year dataset (2000–2015) of high-resolution (3 h, 10 km) global surface solar radiation. Earth Syst. Sci. Data 11, 1905–1915. doi: 10.5194/essd-11-1905-2019
Tasumi, M., Allen, R. G., and Trezza, R. (2008). At-surface reflectance and albedo from satellite for operational calculation of land surface energy balance. J. Hydrol. Eng. 13, 51–63. doi: 10.1061/(ASCE)1084-0699(2008)13:2(51)
Wang, B. Z. (1999). Lecture of solar radiation calculation: part1. The calculation of astronomical parameters in solar energy. Sol. Energy 2, 8–10.
Wang, W. T. (2011). Research on Regional Land Surface Evapotranspiration Estimation based on Remote Sensing Technology-a Case of Yiluo River Basin. Ph.D.thesis. Kaifeng, China: Henan University.
Wu, B. F., Liu, S. F., Zhu, W. W., Yan, N. N., Xing, Q., and Tan, S. (2017). An improved approach for estimating daily net radiation over the Heihe River Basin. Sensors 17:86. doi: 10.3390/s17010086
Yang, K., Koike, T., and Ye, B. S. (2006). Improving estimation of hourly, daily, and monthly solar radiation by importing global data sets. Agr. Forest Meteorol. 137, 43–55. doi: 10.1016/j.agrformet.2006.02.001
Yin, Y. H., Wu, S. H., Du, Z., and Yang, Q. Y. (2008). Radiation calibration of FAO56 Penman-Monteith model to estimate reference crop evapotranspiration in China. Agr. Water Manage. 95, 77–84. doi: 10.1016/j.agwat.2007.09.002
Zhang, C. H., Guo, Y. B., He, Z. W., He, L., and Xu, H. X. (2022). Analysis of influence mechanism of spatial distribution of incoming solar radiation based on DEM. Earth Sci. Inform. 15, 635–648. doi: 10.1007/s12145-021-00740-0
Zhang, S. H., Li, X. G., She, J. F., and Peng, X. M. (2019). Assimilating remote sensing data into GIS-based all sky solar radiation modeling for mountain terrain. Remote Sens. Environ. 231:111239. doi: 10.1016/j.rse.2019.111239
Zhang, Y. C., Rossow, W. B., Lacis, A. A., Oinas, V., and Mishchenko, M. I. (2004). Calculation of radiative fluxes from the surface to top of atmosphere based on ISCCP and other global data sets: Refinements of the radiative transfer model and the input data. J. Geophys. Res. 109:D19105. doi: 10.1029/2003JD004457
Zhang, Y. L., and Zhao, J. (2016). Sensitivity Analysis of Estimating Shortwave Solar Radiation to the DEM Spatial Scale. Beijing, China: IEEE International Geoscience and Remote Sensing Symposium, 10–15. doi: 10.1109/IGARSS.2016.7730140
Keywords: complex terrain, net radiation, solar radiation, remote sensing, Haihe River Basin
Citation: Liu X, Zhang J, Yan H and Yang H (2022) Estimation of the Surface Net Radiation Under Clear-Sky Conditions in Areas With Complex Terrain: A Case Study in Haihe River Basin. Front. Ecol. Evol. 10:935250. doi: 10.3389/fevo.2022.935250
Received: 04 May 2022; Accepted: 23 May 2022;
Published: 14 June 2022.
Edited by:
Jinyan Zhan, Beijing Normal University, ChinaReviewed by:
Chunhong Zhao, Northeast Normal University, ChinaShaohua Zhao, Ministry of Ecology and Environment Center for Satellite Application on Ecology and Environment, China
Copyright © 2022 Liu, Zhang, Yan and Yang. This is an open-access article distributed under the terms of the Creative Commons Attribution License (CC BY). The use, distribution or reproduction in other forums is permitted, provided the original author(s) and the copyright owner(s) are credited and that the original publication in this journal is cited, in accordance with accepted academic practice. No use, distribution or reproduction is permitted which does not comply with these terms.
*Correspondence: Haiming Yan, aGFpbWluZy55YW5AaGd1LmVkdS5jbg==