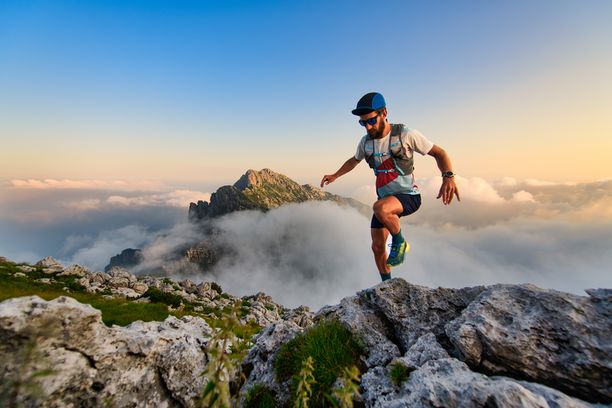
95% of researchers rate our articles as excellent or good
Learn more about the work of our research integrity team to safeguard the quality of each article we publish.
Find out more
ORIGINAL RESEARCH article
Front. Ecol. Evol., 06 September 2022
Sec. Biogeography and Macroecology
Volume 10 - 2022 | https://doi.org/10.3389/fevo.2022.934876
The peak frequency of bat echolocation is a species-specific functional trait linked to foraging ecology. It is tailored via evolution to suit conditions within the distribution range of each species, but the evolutionary drivers are not yet well-understood. Global patterns of humidity correlate with many aspects of bat ecology. We hypothesized that atmospheric absolute humidity could explain global peak frequency variation directly and indirectly via increasing species body size and bat species richness. These hypotheses were tested using Bayesian phylogenetic path analysis on 226 tropical and subtropical bat species. In line with our predictions, we found a positive total effect of humidity on peak frequency, which was dominated by the positive indirect effects via body size and bat species richness. We did not observe the negative direct effect of humidity on peak frequency, which was hypothesized based on atmospheric attenuation of sound. In line with our expectations, excluding the predominantly clutter foraging bat families from our dataset downplayed the importance of the richness-mediated route. To conclude, our findings suggest that indirect effects, owing to ecology and biogeography of bat taxa, play a major role in the global relationship between peak frequency and atmospheric humidity.
Most (87%) of the 1,401 extant bat species orient and locate their prey using echolocation (Wilson and Mittermeier, 2019). These species form an acoustic image of their surroundings by emitting ultrasonic repetitive acoustic pulses and interpreting the delay and structure of the returning echoes (Schnitzler and Kalko, 2001; Metzner and Müller, 2016). To be effective, the emitted signal must be adapted to the variable situations and surroundings experienced by the species in question. The observed similarity in pulses across many unrelated bat taxa is evidence of notable convergent evolution and attributed to similarity in their foraging ecology (Schnitzler and Kalko, 2001; Jones and Holderied, 2007; Metzner and Müller, 2016). The functional acoustics of bats are largely determined by the way they overcome sensory challenges in food acquisition like detecting small objects from afar, masking of prey echoes by the echoes from background, and avoiding collisions while commuting or chasing their prey (Schnitzler et al., 2003). The relative importance of these challenges in foraging is greatly affected by how close to the background clutter, such as vegetation or ground, the species tends to forage. Consequently, the bat taxa can be divided into feeding guilds such as aerial hawkers and clutter foragers. Aerial hawkers, further divided into open space and edge foragers, catch the airborne prey while flying away from or close to obstacles, respectively (Schnitzler and Kalko, 2001; Denzinger and Schnitzler, 2013). On the other hand, clutter foragers predominantly glean stationary food from surfaces, except for the constant frequency calling taxa. Some gleaners can locate and identify their prey solely by echolocation (Geipel et al., 2013), but usually prey-generated sounds or olfactory cues are also followed (Denzinger and Schnitzler, 2013). However, most species are flexible in their feeding habits, and therefore, the guild identity for some species is ambiguous (Denzinger and Schnitzler, 2013).
Research on bat echolocation often treats this feature as a tool for foraging and navigational tasks (Armstrong and Kerry, 2011; Pedro and Simonetti, 2014). For a bigger picture, however, it is important to understand the environmental conditions that create the set of challenges that require specific adaptations. As we hypothesize, atmospheric humidity, that is, the absolute amount of gaseous water in atmosphere, has potential to affect these challenges and therefore drive evolution of bat traits. From now on, we refer to this as specific humidity, which is the measure of atmospheric humidity used in this study. Unlike relative humidity, specific humidity is not directly affected by changes in temperature. This eliminates fluctuations arising from daily and seasonal temperature cycles (Encyclopedia Britannica, 1998).
The frequencies emitted by echolocating bats are generally in the ultrasonic range. Sufficiently high frequencies are essential for revealing details because sound reflects less effectively from an object of smaller dimensions than its wavelength. As a downside, high frequencies attenuate more in the air, thus compromising detection distances (Griffin, 1971; Lawrence and Simmons, 1982; Schnitzler and Kalko, 2001). For this study, we focus on peak frequency, which is the frequency of the bat call with the highest amplitude. Because attenuation depends on the frequency of the sound, the effects on peak frequency cannot be directly modeled in multiple bat taxa with variable peak frequencies. However, attenuation increases with sound frequency more rapidly in higher specific humidity (Figure 1) (National Physical Laboratory, 2018). This relationship is consistent within the range of ambient temperature and atmospheric pressure experienced by foraging bats (National Physical Laboratory, 2018) and potentially favors low peak frequencies in humid environments. Therefore, a negative association is expected between peak frequency and specific humidity across all echolocating bats (attenuation hypothesis, Figure 1). Even though attenuation is often proposed as an important driver of the evolution of call frequency (Griffin, 1971; Snell-Rood, 2012; Luo et al., 2014; Jacobs et al., 2017), the results have been mixed (Jiang et al., 2010; Luo et al., 2019), and supporting evidence has been limited to few studies with very little geographic and taxonomic coverage (Jiang et al., 2010; Armstrong and Kerry, 2011; Snell-Rood, 2012). To our knowledge, the current study is the first attempt to model the effect of specific humidity on global variation of bat peak frequencies.
Figure 1. Attenuation hypothesis: bat echolocation is subject to atmospheric absorption, which effectively decreases the detection range, especially in the higher end of the frequency spectrum. The slope of the relationship between absorption and frequency increases with specific humidity. Thus, humidity increases the cost of evolving higher echolocation frequency. Calculation of absorption is carried out with an ISO 9613-1-based online calculator (National Physical Laboratory, 2018).
A negative association between body size and environmental heat is observed in homeothermic animals due to thermoregulation constraints but can also be expected to apply to heterothermic mammals, particularly because of the elevated body temperature during foraging (James, 1970; Thomas et al., 1991; Meiri and Dayan, 2003; Voigt and Lewanzik, 2011; Conenna et al., 2021; Rubalcaba et al., 2022). Specific humidity represents a combined effect of temperature and relative humidity, both of which increase heat stress by affecting body temperature directly and impeding evaporative cooling, respectively (James, 1970). In addition, a negative association between body size and peak frequency is well-demonstrated in bats (Barclay, 1991; Bogdanowicz et al., 1999; Jones, 1999; Thiagavel et al., 2017; Luo et al., 2019; López-Cuamatzi et al., 2020). Considering this together with the aforementioned association between specific humidity and body size, body size could mediate the indirect effect of specific humidity on peak frequency, as previously suggested by Mutumi et al. (2016) (body size constraint hypothesis, Figure 2).
Figure 2. Mechanisms leading to a lower average peak frequency in bat communities occurring in low-humidity area, and vice versa. According to the body mass constraint (a) and diversity (b) hypotheses: blue bats represent taxa with open space aerial hawking traits (low peak frequency), purple bats represent taxa with edge space aerial hawking traits, and red bats represent taxa with clutter foraging traits (high peak frequency).
In addition, specific humidity could indirectly affect bat echolocation via bat species richness. Specific humidity and bat species richness are positively correlated in the global tropics and subtropics (Supplementary Figure 1). This could be due to humidity governing global patterns of vegetation biomass (Sankaran et al., 2004; Malhi et al., 2009), and habitats with complex vegetation providing niches for a more diverse bat community. However, complex vegetation could be more beneficial to clutter foragers than aerial hawkers. While the former group is restricted to sites with sufficient vegetation complexity to provide their tightly framed foraging habitat, suitable habitat for the latter does not necessarily require structural complexity to the same degree (Mtsetfwa et al., 2018; Conenna et al., 2021). On average, clutter foragers use higher peak frequencies than edge space foragers (Denzinger and Schnitzler, 2013), which potentially creates a positive association between species richness and peak frequency. In addition, this association could also result from acoustic resource partitioning, according to which bat species adapt to using echolocation frequency bands that are less utilized by other sympatric bat species (Jones and Siemers, 2011; Russo et al., 2018) and are consequently utilizing higher frequencies in areas with high species richness. Considering these aspects, specific humidity could be predicted to have an indirect effect on peak frequency via bat species richness (Figure 2). From now on, we refer to this as the diversity hypothesis.
We tested these three hypotheses simultaneously by contrasting the direct effect of specific humidity on peak frequency with its indirect effects via body mass and bat species richness using a global sample of 226 tropical and subtropical bat species. We predict that part of the peak frequency variation must be explained by a negative direct effect of specific humidity for the attenuation hypothesis to be supported. For body size constraint and diversity hypotheses, we expect positive indirect effects on peak frequency mediated by body size or species richness, respectively. Furthermore, the mass-mediated indirect effect needs to consist of two negative direct effects (i.e., from humidity to body mass and from body mass to peak frequency) for the body mass hypothesis to be supported. By contrast, two positive direct effects (i.e., from humidity to species richness and from species richness to peak frequency) in the species richness-mediated route are expected according to the diversity hypothesis (Figure 3). We test our hypotheses using a phylogenetic path analysis, which accommodates both direct and indirect effects while accounting for phylogenetic non-independence between the species (von Hardenberg and Gonzalez-Voyer, 2013).
Figure 3. A schematic path diagram showing the variables and direct and indirect effects in the model: The effects corresponding to each of the three hypotheses are shown in different colors.
Because the ecology of a bat species most likely affects the evolutionary drivers of its sensory ecology (Fenton, 1990; Denzinger et al., 2018), we repeat the analyses with a subset of data consisting of predominantly aerial hawking families or subfamilies of bats (n = 158 species). We predict that attenuation is a more severe issue for aerial hawking bats because their airborne prey items are scattered in vast three-dimensional space, and sufficient detection distance significantly increases the volume scanned with echolocation. Furthermore, these bats rely solely on their echolocation, unlike gleaners which often complement detection with olfaction and passive hearing (Schnitzler and Kalko, 2001; Denzinger and Schnitzler, 2013). We thus expect that the indirect effect predicted by the diversity hypothesis will appear stronger if the clutter foraging bats are involved.
We used echolocation peak frequency, the frequency with the highest intensity in the echolocation pulse, as our as our species-specific variable. It is a widely adopted parameter in bioacoustics studies because its variation is connected to many ecological and morphological factors (Schnitzler and Kalko, 2001; Denzinger and Schnitzler, 2013).
We extracted information on species-specific average peak frequencies from various literature sources (see Supplementary Data). Only the bat taxa using laryngeal frequency-modulated echolocation were used in fitting the model. In other words, we excluded the tongue clicking Rousettus spp. among the non-echolocating pteropodid bats, and also the constant frequency calling bats (superfamily Rhinolophoidea and Pteronotus spp. in the subgenus Phyllodia), which have a highly specialized acoustic method for localization of airborne insects within the clutter (Schnitzler and Denzinger, 2011). Regardless of the ecological importance of these bat taxa in many ecosystems, their inclusion in the modeling would significantly widen the definition of the study system and hinder interpretability of the model results.
An average echolocation peak frequency value was calculated for every species. When multiple references for a species were available, the final average was obtained by first calculating averages within these sources and finally between the sources. In the calculation of the within-source averages, the recordings from different contexts (location, situation, call type) were first averaged within the context and then between the contexts. Some references reported calls separately characterized by different strongest harmonics, but these call types were not considered as different contexts.
We gathered body mass data primarily from the Phylacine trait database (Faurby et al., 2018) to represent body size of the bats. Some records in these data did not have measurements so were assumed allometrically based on forearm length. Where possible, we replaced these data with measured values from other literature sources; however, the assumed values were retained in the absence of reported values for 12 of 226 species (detailed source information in Supplementary Data).
The allometric non-linear relationship between bat peak frequency and body mass has been demonstrated (Heller, 1996; López-Cuamatzi et al., 2020). However, we use the path model that assumes linear relationships between variables and therefore performed natural log (ln) transformation on these variables to achieve linearity. Using linear regression to fit the individual paths also makes interpretations of indirect effects in a straightforward manner.
A species-level phylogenetic supertree for mammals was obtained from the Phylogenetic Atlas of Mammal Macroecology (Faurby et al., 2018). This atlas provided a posterior sample of 1,000 trees, from which 500 random trees were drawn to be used in all analyses. This reduction was made to reduce the processing time in the following steps. Species distribution data were downloaded from the IUCN website (IUCN Red List of Threatened Species, 2019).
Only the species represented in both the species distribution dataset and the phylogeny were included in the data. We further discarded any species with a median of annual average temperatures within its distribution range <15°C because many of these species go through an annual prolonged period of hibernation when there is no foraging activity. In such cases, annual means of specific humidity would be biased by the cold period, which bats spend in hibernation. However, bat hibernation behavior is a gradient, and consequently, any hard limit is rather arbitrary (Humphries et al., 2002). We consider an annual average of 15°C as a limit providing a satisfying trade-off between cutting the most problematic species out and retaining the sample size while roughly including the subtropical climate zones of the world. A list of the discarded species is provided as a separate sheet in the Supplementary Data. The temperature data were downloaded from the CHELSA climatology dataset (Karger et al., 2017) as a raster. The workflow to obtain these medians was similar to that described for the other raster data given later.
With 226 species in the final full dataset, we further selected a subset of 158 species from the families and subfamilies that predominantly rely on aerial hawking as the foraging strategy. These taxa included families Emballonuridae, Cistugidae, Craseonycteridae, Miniopteridae, Molossidae, Mormoopidae, Rhinopomatidae, and vespertilionid subfamilies Vespertilioninae and Myotinae. The final dataset is provided as Supplementary Data. Table 1 shows the key statistics of both datasets.
Table 1. Descriptive statistics for the variables used in this study: both raw scale and natural logarithm (ln) transformations are shown for peak frequency and body mass.
We use mean annual specific humidity to describe the humidity within the distribution range of each species. In the frequency range used by bats, specific humidity is by far the largest determinant of absorption of sound, which makes up a major part of attenuation for ultrasonic frequencies (Griffin, 1971; National Physical Laboratory, 2018). Specific humidity also works well in representing heat stress because it combines the effect of both temperature and humidity (James, 1970). The specific humidity data were obtained from the NASA Langley Research Center (LaRC) POWER Project funded through the NASA Earth Science/Applied Science Program. The global data surface consists of annual averages of daily average values from 1984 to 2020 at 2 m height from the ground averaged over half a degree grid or 55 km by cosϕ 55 km, where ϕ is the latitude (NASA, 2020).
We loaded the species distribution dataset as a shapefile, and species richness and specific humidity as global raster surfaces into R version 4.0.4 (R Core Team, 2015) workspace. The species richness raster for all extant bats, including the taxa removed from the modeling dataset, was downloaded from https://biodiversitymapping.org/ (Jenkins et al., 2013; Pimm et al., 2014) and re-projected to match its resolution to the humidity raster (0.5 degrees). Each global raster layer was masked separately with species-specific distribution polygons, cutting out the cells where the species were absent. Then, cell values in the resulting raster layer were summarized, and the resulting median value was saved to represent environmental conditions within the distribution range of that species. These operations were performed using the raster package version 3.4-5 (Hijmans, 2019).
We conducted a phylogenetic path analysis (von Hardenberg and Gonzalez-Voyer, 2013) that describes the variation of the species-specific average peak frequency with prevailing average specific humidity via direct and indirect (i.e., multiplication of direct effects along a given route) effects through species richness and body mass. Instead of fitting phylogenetic path analysis using generalized least squares (GLS) (von Hardenberg and Gonzalez-Voyer, 2013), we applied the Bayesian framework using the R package brms version 2.14.4 (Bürkner, 2018) for Bayesian multilevel modeling using Stan. This was done because GLS is inherently biased (Hadfield and Nakagawa, 2010), and this bias is likely to be exacerbated; when the sample size is not large, multivariate normality is not met and the model is misspecified (Olsson et al., 2000). Moreover, the Bayesian approach does not depend on the large sample theory and is thus more appropriate for small to moderate sample sizes (Depaoli, 2021). Finally, Bayesian estimation is not limited by similar identification constraints as frequentist approaches (Depaoli, 2021), allowing, for example, the possibility to perform robustness checks for omitted confounding (see Robustness Checks). We did not perform model selection because our aim was to contrast a priori selected hypotheses.
A phylogenetic correlation matrix was compiled using the package ape 5.4-1 (Paradis and Schliep, 2019). This matrix was then used to specify the correlation structure in an intercept-only group-level effects (equivalent to random effects in frequentist approaches) in the phylogenetic path models. Default uninformative Student's t-test (degrees of freedom = 3, location = 0, scale = 2.5) priors were used for standard deviations of group-level effects and residual standard deviations. We set weakly informative normally distributed (mean = 0, SD = 2) priors for the population-level effects (equivalent to fixed effects of frequentist approaches) to rule out unrealistic parameter draws during the Markov chain. We performed a sensitivity analysis to confirm that our choice of priors for population-level effects does not affect the results in an unexpected way. The results were insensitive as both uninformative and more informative priors yielded virtually the same results than the weakly informative priors (see Supplementary Table 2 for more details).
The model was run for 10,000 iterations with a warm-up of 2,000 iterations using two chains, which was repeated for 500 random trees from the posterior sample of the phylogeny. The posterior samples were thinned with a factor of 4 to avoid autocorrelation (Depaoli, 2021). This resulted in 500 individual model fits with 4,000 posterior samples each.
Convergence of chains was inspected by checking the potential scale reduction factor (Gelman and Rubin, 1992). This was 1.0 (full convergence) for every parameter in >90% of model fits and always below 1.08. Before calculating the following statistics, the posterior distributions of the 500 fitted models were combined by relevant parameters into the final distributions with 2,000,000 posterior samples. Medians, probabilities of direction (Makowski et al., 2019), and 95% credibility intervals were extracted for the parameters of interest from the corresponding posterior distributions. The values for indirect effects were calculated by multiplying the posterior distributions of the two associated path coefficients (Miočević et al., 2018). Distributions for extracting values of phylogenetic signals for each response variable were calculated by dividing squared group-level standard deviations by the sum of themselves and the residual standard deviation [i.e., /(SDres)]. Model fitting was performed separately with the same specifications for both the aerial hawking subset and the full data. An example of R code for fitting the brms model and calculating the descriptive statistics from the results is available at https://github.com/mhkoti/path_analysis.
We consider a hypothesis supported if the fitted path coefficients are in line with the predicted direction and result in a non-zero direct or indirect effect of humidity on peak frequency. Because we test directional (constrained) hypotheses, we define non-zero here as >95% probability of direction (pd), which indicates the proportion of posterior mass located in the median side of zero (Makowski et al., 2019).
To check robustness of the previous results to external model misspecification (i.e., omitted confounders), we fitted the path model by estimating the error correlations among all the response variables. Such error correlations model all the unmodeled associations among the variables in question. Note that such a model would not be identified in the frequentist framework owing to the negative degrees of freedom but can be estimated using the Bayesian approach (Depaoli, 2021). If there are important unobserved confounders, we expect clear changes in our estimates of direct and indirect effects (Pearl, 2009). In addition, fitting such error correlations provides an opportunity to test model fit to the data using conditional independences, given by d-separation criterion (Shipley, 2016). For our model, there was just one conditional independency statement (i.e., basis set), which would indicate a good model fit to the data: species richness should be independent of body mass when conditioned on humidity if the model was correctly specified. Given the model structure examined here, such a test is statistically equivalent to looking at whether the errors of species richness and body mass are correlated (Shipley, 2016). The default uninformative LKJ correlation prior for Cholesky factors with a default eta parameter value of 1 was used for error correlations among the response variables. The specifications described earlier for the main model apply for the correlated error model.
We further inspected the fit of the main models with graphical posterior predictive checks (PPCs) using the bayesplot R package version 1.8.0 (Gabry and Mahr, 2019) through a wrapper function provided by the brms package (Bürkner, 2018). In PPCs, new datasets are generated according to the model. These sets are then visually compared with the actual data used in fitting the model. If the model has performed well, generated and actual data will resemble each other. A PPC was performed from a combined output of 50 randomly selected model fits to account for phylogenetic uncertainty, while combined posterior distributions of 500 model fits were used to extract all other parameters.
Finally, we examined the influence of intraspecific variation in peak frequency measurements on our results. Such a variation is documented in the literature since geographical area, season, chosen methodology, and possibly other unknown parameters could together affect the measured species-specific average (Russo et al., 2018). However, the available data for most species are insufficient in this regard. We fitted a quadratic function between average peak frequency and its standard deviation using all the species in the data with more than one study as source information (see Supplementary Data and Supplementary Figure 3). Using this fitted function, we estimated standard deviation of intraspecific variability for the range of peak frequencies. Then, we created simulated datasets drawing random samples for each species from a normal distribution with a species-specific mean (i.e., the average of the reported peak frequency values, see Supplementary Data) and standard deviation derived using the quadratic function. To account for phylogenetic uncertainty, we used 20 different trees, for each of which we ran 10 samples; that is, a total of 200 models were estimated, and the posterior distributions of these models were combined into the final simulated error model. Furthermore, to evaluate the consequences of more severe measurement errors in peak frequency, we repeated the aforementioned procedure by using a larger intercept for the function fitted (severe error model, Supplementary Figure 3). The other specifications were used in the main model. Separate models were again fitted for the full data and aerial hawking subset. Should the main model be sensitive to measurement error in peak frequency, the estimates or their credibility intervals in the simulated error models deviate from those in the main model.
We found no evidence for the attenuation hypothesis as the direct effect of humidity on peak frequency did not statistically differ from zero in the full dataset (Figure 4 and Table 2). This was also true for the aerial hawking subset of the data, for which we predicted a stronger negative direct effect of humidity on peak frequency (Figure 4 and Table 2).
Figure 4. Posterior distributions for the direct and indirect effects of humidity on peak frequency from the path analysis for the aerial hawking subset and full data: Plotted distributions consist of 200,000 posterior samples each because thinning factor of 10 was applied prior to plotting.
Table 2. Path coefficients (coef.) and probabilities of direction (Pd) for each individual path and the corresponding indirect effects (bolded) by dataset: all parameters are calculated from the full posterior distributions with 2,000,000 samples each.
Instead, our results support the body size constraint hypothesis as the associated path coefficients were positive and differed from zero in both datasets (Table 2). The resulting body mass-mediated indirect effect of humidity on peak frequency was nearly 40% higher in the aerial hawking subset (0.0078 with 98.15% pd) than in the full data (0.0049 with 96.39% pd) (Figure 4 and Table 2). Due to ln-transforming peak frequency, indirect effects contribute to a 0.78 and 0.49 percent increase in peak frequency for a one-unit (a gram of water vapor per kilogram of air) increase in specific humidity in the aerial hawking subset and in full data, respectively. In the aerial hawking subset, for example, a standard deviation increase (3.58, Table 1) in humidity is associated with a 1.13-kHz increase in 40-kHz peak frequency.
The paths associated with the diversity hypothesis were positive in both datasets as predicted (Table 2). In the full data, the indirect effect via bat species richness was non-zero with an estimate of 0.0123 and 99.85% pd (Figure 4 and Table 2), supporting the diversity hypothesis. This indirect effect on peak frequency equals a 1.24 percent change per one unit change in humidity. Thus, 40-kHz sound would increase 1.76 kHz per standard deviation increase (3.51, Table 1) in humidity. The indirect effect was weaker in the aerial hawking subset, as expected, and did not exceed the limit of 95% pd (Figure 4 and Table 2).
We also calculated a total effect of humidity on peak frequency, which is a sum of its direct and indirect effects. The total effect of humidity was 0.0132 in the aerial hawking subset and 0.0198 in the full data, contributing to 1.32 and 1.99 percent changes per one unit change in humidity in the aerial hawking subset and full data, respectively. Therefore, a standard deviation increase in humidity (Table 1) would be associated with 1.94 and 2.88 kHz increases in 40-kHz peak frequency in the aerial hawking subset and full data, respectively. Path- and route-specific estimates, phylogenetic signals, and their corresponding credibility limits are presented in Supplementary Table 4.
Allowing correlated error terms resulted in minor changes in the estimates of direct and indirect effects (Supplementary Table 5) suggesting that our results are relatively robust to omitted confounders. Note that the inclusion of error term correlations somewhat widened the posterior distributions, but this is likely due to added complexity in the model (i.e., a bias variance trade-off in estimated effects and their uncertainty, Supplementary Figure 6). Moreover, the errors of body mass and species richness were not associated in either of the datasets [aerial hawking: posterior median = 0.055 (95% credibility limits = −0.166, 0.280); full dataset: posterior median = 0.003 (95% credibility limits = −0.199, and 0.215)], showing that the main models fitted the data well. Moreover, graphical posterior predictive checking indicated that the data generated by the primary model is relatively close to the actual data, again suggesting a reasonable fit (Supplementary Figures 7, 8). In addition, simulated error models also did not indicate high sensitivity to error in the peak frequency because regardless of the slight changes in the estimated effects, our biological conclusions were not affected (Supplementary Figure 9 and Supplementary Table 10).
Our results demonstrate that the positive covariance (i.e., total effect) between specific humidity and peak frequency is likely explained by its indirect effects via body mass and bat species richness. This finding thus supports both the body mass constraint and diversity hypotheses. No direct effect between specific humidity and peak frequency was observed. The robustness checks performed indicate that the results are reasonably robust to model misspecification and non-systematic error in the reported peak frequency values.
The lack of support for the attenuation hypothesis is interesting because atmospheric attenuation of sound is perhaps the most discussed candidate for a climatic driver of bat bioacoustics (Griffin, 1971; Lawrence and Simmons, 1982). Attenuation could simply be a less relevant issue than previously suggested. However, the following possible reasons behind the lack of support should be considered. The effects of attenuation on the acoustic signals of different species could be highly variable and not always manifest in the peak frequency. Thus, the alternative strategies among the studied bat taxa could create enough noise to mask the effect of attenuation. In addition to decreasing peak frequency, bat species could increase the echolocation pulse duration and signal amplitude to increase the detection range (Schnitzler and Kalko, 2001). Furthermore, attenuation could be mitigated by optimizing, for example, timing and location of the foraging activity, and foraging patterns in relation to clutter. In this case, the macroecological scale might not be fine enough to catch the effect of attenuation.
Contrary to the attenuation hypothesis, the diversity hypothesis can only be held true when a range of feeding guilds is considered. According to our predictions, the effect mediated by bat species richness decreased after excluding clutter foraging bat taxa. As shown by the posterior predictive check, the model did not perfectly predict bat species richness values. Presumably, this is due to the oversimplification of the humidity–richness–peak frequency–route; that is, our model predicts the number of sympatric bat species solely based on specific humidity, even though we know that there are many other factors involved. As visualized in Supplementary Figure 1, bat diversity on islands is often relatively low because of isolation. Also, neotropics appear richer in bat species than the old world. These and other unmodeled processes probably affect the humidity richness regression and result in the predictive error, increasing with specific humidity, which we observed in the posterior predictive check (Supplementary Figure 8). As discussed by Conenna et al. (2021), bats inhabiting areas with extremely low humidity, such as the Arabian Peninsula, may also deviate from the general trend by exhibiting clutter forager traits. In such areas, the scarce food items are centered around the few patches of vegetation. Moreover, considering the abundance of different sympatric species would be beneficial if frequency band partitioning is a major component for the path between richness and peak frequency. Nevertheless, the indirect effect predicted by the diversity hypothesis in the full dataset was the strongest link between humidity and peak frequency, indicating its important role in the evolution of peak frequency.
As expected, the indirect effect mediated by body mass persisted in both the aerial hawking subset and full data. However, the aerial hawking subset had an almost 40% larger estimate and slightly higher probability of direction, regardless of smaller sample size. Both paths in the body mass-mediated route had a larger estimate in the aerial hawking subset (Table 2). This could simply result from the presence of phyllostomids and other non-insectivorous bat taxa in the full data. Compared to the insectivorous bat taxa, which make up nearly 100% of the aerial hawking subset, there is indeed a weaker association between body mass and peak frequency in phyllostomid and non-insectivorous bats (Jones, 1999; López-Cuamatzi et al., 2020).
Along with body size, the wing surface area contributes to thermoregulation because a larger wing surface dissipates more heat (Rubalcaba et al., 2022). Therefore, focusing on body mass only could be problematic if surface area-to-body mass ratio (S-MR) varies within data. This could be the case in the full dataset because high S-MR associates with clutter foraging (Nordberg and Rayner, 1987). On the other hand, S-MR could have less variance in the aerial hawking subset, which lacks most clutter foraging taxa. The weaker correlation between humidity and body mass in the full data set compared to the aerial hawking subset could therefore result from a problem in model specification and not biological difference.
In addition, the difference between the two sets of data could be explained with high metabolic heat generation in flight (Thomas and Suthers, 1972; Thomas et al., 1991; Voigt and Lewanzik, 2011). Although completely speculative, aerial hawking bats may be spending more time aloft than clutter foraging bat species, with the latter often utilizing alternative feeding strategies such as perch hunting, catching vertebrates, and visiting known stationary food sources such as fruiting trees. Hence, large body size could be more of a burden in aerial hawking bat families. Flight is indeed considered an important constraint for body size in bats due to its biomechanical requirements (Nordberg and Rayner, 1987; Arévalo et al., 2020). However, heat conservation has been recently suggested as the main thermoregulatory cost in flying bats (Rubalcaba et al., 2022), contrasting the ideas of James (1970), and therefore the premise of the body size constraint hypothesis.
Recently, Conenna et al. (2021) found a negative correlation between aridity and bat peak frequency. The result is analogous with the positive total effect of humidity on peak frequency observed in this study. Moreover, Conenna et al. (2021) found a positive correlation between aridity and body size, which is in agreement with the negative correlation that we found between humidity and body size. However, our result suggests that the positive indirect effect of body size also contributes to the positive total effect of humidity on peak frequency, adding a novel dimension to the understanding of the topic. Other studies on the effects of climate on bat echolocation characteristics are not widely applicable in large scale because they have been hypothetical (Griffin, 1971; Lawrence and Simmons, 1982; Luo et al., 2014), focused on only one or two species (Guillén and Ibáñez, 2000; Armstrong and Kerry, 2011; Mutumi et al., 2016) or lacked phylogenetic approaches to correct for relatedness among taxa (Snell-Rood, 2012).
While interpreting the results of this study, the spatial and the temporal scale should be considered (Teng et al., 2020). The spatial scale in our dataset might be too coarse to accurately describe the species-specific foraging conditions because there are usually variable habitats and microclimates for a bat species to exploit within one 0.5-degree raster cell. Moreover, the distribution polygons only specify the general extent of distribution for each species, and not all cells within that area are guaranteed to be occupied by the species. Similarly, annual average conditions might not accurately summarize the relevant periods of foraging activity for all species (Vasko et al., 2020). For the aforementioned reasons, our results should be interpreted as the correlation of the general conditions and the selection of different habitats within the geographic area occupied by a species. Unfortunately, the effect of the preferred foraging habitat on bat traits is too fine-scaled for the used data.
However, error in the predictor variables could result in causally inconsistent estimates regarding those predictor variables (Shipley, 2016). This is a potential pitfall for our study because the used body mass data do not consider intraspecific variation, the distribution ranges might have errors, and current environmental conditions are used, instead of historical ones. Therefore, we do not want to interpret the observed correlations as proven causal estimates, which are inherently unknown.
Despite the high probability of the direction of hypothesized effects, the indirect effects predicted by the body mass constraint and diversity hypotheses are quite small compared with the range of peak frequency. Therefore, our results do not explain a large portion of peak frequency variation, which is mostly determined by other factors. The largest effect size for a single indirect effect was for the richness-mediated route in the full data and has a value of 0.0123 (1.24% change per 1‰ change in specific humidity), while the total effect of humidity in the full data was 0.0197 (1.99% change per 1‰ change in specific humidity). According to these numbers, the effect of humidity on 40-kHz peak frequency would be roughly 12.5 kHz between the two extremes of the humidity range in our data and only 2.9 kHz between environments with one standard deviation (3.51) difference in humidity. For context, many bat species can change their peak frequency 5 kHz or more on average when moving between open and confined environments (Mora et al., 2011; Pedro and Simonetti, 2014). However, the frequently observed situation-dependent peak frequency switches of <5 kHz suggest that such differences are ecologically relevant. Even though error in the predictor variables could influence our results, the observed trends in our data suggest evolutionary pressure and could therefore provide important considerations for future research. Moreover, we believe that these trends can be significantly more pronounced or even dominant drivers of acoustic evolution in some of the bat groups included in our analysis. Carefully separating these groups in the analyses could provide a yet more comprehensive understanding of these effects and reveal even more relevant group-wise effect sizes.
Specific humidity within the distribution range correlated with species-specific average peak frequency of tropical and subtropical bats via two distinct routes. These routes were mediated by average body mass and sympatric bat species richness, as predicted by the body mass constraint hypothesis and the diversity hypothesis, respectively. Even though the causal interpretation of the results could be affected by measurement error in the predictor variables, the model specification appears robust after vigorous testing, and the effect size can be considered ecologically meaningful. This suggests that the global variation of bat echolocation could be affected by specific humidity and highlights the potential of indirect effects in explaining contemporary variation of functional traits.
The data used in this study and source information are available in Supplementary material. R-code for replicating the analysis is available at https://github.com/mhkoti/path_analysis. Further inquiries can be directed to the corresponding authors.
MK, EV, DR, HL, and TL conceptualized the idea. MK curated the data. MK and SH performed statistical analysis. MK prepared the first draft of the manuscript. All authors contributed to the final draft. All authors contributed to the article and approved the submitted version.
MK was funded by the Maj and Tor Nessling Foundation (project 201900354). TL was funded by the Academy of Finland (project 331515). DR was partially funded through project 020101070 from Pontificia Universidad Javeriana Cali.
The authors wish to acknowledge CSC – IT Center for Science, Finland, for computational resources. We thank Melissa Meierhofer, Katarina Meramo, and Annina Kantelinen for commenting on the manuscript.
The authors declare that the research was conducted in the absence of any commercial or financial relationships that could be construed as a potential conflict of interest.
All claims expressed in this article are solely those of the authors and do not necessarily represent those of their affiliated organizations, or those of the publisher, the editors and the reviewers. Any product that may be evaluated in this article, or claim that may be made by its manufacturer, is not guaranteed or endorsed by the publisher.
The Supplementary Material for this article can be found online at: https://www.frontiersin.org/articles/10.3389/fevo.2022.934876/full#supplementary-material
Arévalo, R. L., Amador, L. I., Almeida, F. C., and Giannini, N. P. (2020). Evolution of body mass in bats: insights from a large supermatrix phylogeny. J. Mammal. Evol. 27, 123–138. doi: 10.1007/s10914-018-9447-8
Armstrong, K. N., and Kerry, L. J. (2011). Modelling the prey detection performance of Rhinonicteris aurantia (Chiroptera: Hipposideridae) in different atmospheric conditions discounts the notional role of relative humidity in adaptive evolution. J. Theor. Biol. 278, 44–54. doi: 10.1016/j.jtbi.2011.02.026
Barclay, R. M. R. (1991). Population structure of temperate zone insectivorous bats in relation to foraging behaviour and energy demand. J. Anim. Ecol. 60, 165–178. doi: 10.2307/5452
Bogdanowicz, W., Fenton, M. B., and Daleszczyk, K. (1999). The relationships between echolocation calls, morphology and diet in insectivorous bats. J. Zool. 247, 381–393. doi: 10.1111/j.1469-7998.1999.tb01001.x
Bürkner, P.-C. (2018). Advanced Bayesian multilevel modeling with the R package brms. R J. 10, 395–411. doi: 10.32614/RJ-2018-017
Conenna, I., Santini, L., Rocha, R., Monadjem, A., Cabeza, M., and Russo, D. (2021). Global patterns of functional trait variation along aridity gradients in bats. Glob. Ecol. Biogeogr. 30, 1014–1029. doi: 10.1111/geb.13278
Denzinger, A., and Schnitzler, H.-U. (2013). Bat guilds, a concept to classify the highly diverse foraging and echolocation behaviors of microchiropteran bats. Front. Physiol. 4, 164. doi: 10.3389/fphys.2013.00164
Denzinger, A., Tschapka, M., and Schnitzler, H.-U. (2018). The role of echolocation strategies for niche differentiation in bats. Can. J. Zool. 96, 171–181. doi: 10.1139/cjz-2017-0161
Encyclopedia Britannica (1998). Specific Humidity. Available online at: https://www.britannica.com/science/specific-humidity.
Faurby, S., Davis, M., Pedersen, R. Ø., Schowanek, S. D., Antonelli, A., and Svenning, J.-C. (2018). PHYLACINE 1.2: the phylogenetic atlas of mammal macroecology. Ecology 99, 2626. doi: 10.1002/ecy.2443
Fenton, M. B. (1990). The foraging behaviour and ecology of animal-eating bats. Can. J. Zool. 68, 411–422. doi: 10.1139/z90-061
Gabry, J., and Mahr, T. (2019). bayesplot: Plotting for Bayesian Models. R package version 1.7.1. Available online at: https://mc-stan.org/bayesplot.
Geipel, I., Jung, K., and Kalko, E. K. V. (2013). Perception of silent and motionless prey on vegetation by echolocation in the gleaning bat Micronycteris microtis. Proc. R. Soc. B Biol. Sci. 280, 20122830. doi: 10.1098/rspb.2012.2830
Gelman, A., and Rubin, D. B. (1992). Inference from iterative simulation using multiple sequences. Stat. Sci. 7, 457–472. doi: 10.1214/ss/1177011136
Griffin, D. R. (1971). The importance of atmospheric attenuation for the echolocation of bats (Chiroptera). Anim. Behav. 19, 55–61. doi: 10.1016/S0003-3472(71)80134-3
Guillén, B.J, and Ibáñez (2000). Variation in the frequency of the echolocation calls of Hipposideros ruber in the Gulf of Guinea: an exploration of the adaptive meaning of the constant frequency value in rhinolophoid CF bats. J. Evol. Biol. 13, 70–80. doi: 10.1046/j.1420-9101.2000.00155.x
Hadfield, J. D., and Nakagawa, S. (2010). General quantitative genetic methods for comparative biology: phylogenies, taxonomies and multi-trait models for continuous and categorical characters. J. Evol. Biol. 23, 494–508. doi: 10.1111/j.1420-9101.2009.01915.x
Heller, K.-G. (1996). Echolocation and body size in insectivorous bats: the case of the giant naked bat Cheiromeles torquatus (Molossidae). Le Rhinolophe 11, 27–38.
Hijmans, R. J. (2019). raster: Geographic data analysis and modeling. R package version 3.0-7. Available online at: https://CRAN.R-project.org/package=raster
Humphries, M. M., Thomas, D. W., and Speakman, J. R. (2002). Climate-mediated energetic constraints on the distribution of hibernating mammals. Nature 418, 313–316. doi: 10.1038/nature00828
I. U. C. N Red List of Threatened Species (2019). Digital Distribution Maps (Version 6.2). Available online at: https://www.iucnredlist.org/resources/spatial-data-download
Jacobs, D. S., Catto, S., Mutumi, G. L., Finger, N., and Webala, P. W. (2017). Testing the sensory drive hypothesis: geographic variation in echolocation frequencies of Geoffroy's horseshoe bat (Rhinolophidae: Rhinolophus clivosus). PLoS ONE 12, e0187769. doi: 10.1371/journal.pone.0187769
James, F. C. (1970). Geographic size variation in birds and its relationship to climate. Ecology 51, 365–390. doi: 10.2307/1935374
Jenkins, C. N., Pimm, S. L., and Joppa, L. N. (2013). Global patterns of terrestrial vertebrate diversity and conservation. PNAS 110, E2602–E2610. doi: 10.1073/pnas.1302251110
Jiang, T., Liu, R., Metzner, W., You, Y., Li, S., Liu, S., et al. (2010). Geographical and individual variation in echolocation calls of the intermediate leaf-nosed bat, Hipposideros larvatus. Ethology 116, 691–703. doi: 10.1111/j.1439-0310.2010.01785.x
Jones, G. (1999). Scaling of echolocation call parameters in bats. J. Exp. Biol. 202, 3359–3367. doi: 10.1242/jeb.202.23.3359
Jones, G., and Holderied, M. W. (2007). Bat echolocation calls: adaptation and convergent evolution. Proc. R. Soc. B. 274, 905–912. doi: 10.1098/rspb.2006.0200
Jones, G., and Siemers, B. M. (2011). The communicative potential of bat echolocation pulses. J. Comp. Physiol. A 197, 447–457. doi: 10.1007/s00359-010-0565-x
Karger, D. N., Conrad, O., Böhner, J., Kawohl, T., Kreft, H., Soria-Auza, R. W., et al. (2017). Climatologies at high resolution for the earth's land surface areas. Sci. Data 4, 170122. doi: 10.1038/sdata.2017.122
Lawrence, B. D., and Simmons, J. A. (1982). Measurements of atmospheric attenuation at ultrasonic frequencies and the significance for echolocation by bats. J. Acoust. Soc. Am. 71, 585–590. doi: 10.1121/1.387529
López-Cuamatzi, I. L., Vega-Gutiérrez, V. H., Cabrera-Campos, I., Ruiz-Sanchez, E., Ayala-Berdon, J., and Saldaña-Vázquez, R. A. (2020). Does body mass restrict call peak frequency in echolocating bats? Mam. Rev. 50, 304–313. doi: 10.1111/mam.12196
Luo, B., Leiser-Miller, L., Santana, S. E., Zhang, L., Liu, T., Xiao, Y., et al. (2019). Echolocation call divergence in bats: a comparative analysis. Behav. Ecol. Sociobiol. 73, 154. doi: 10.1007/s00265-019-2766-9
Luo, J., Koselj, K., Zsebok, S., Siemers, B. M., and Goerlitz, H. R. (2014). Global warming alters sound transmission: differential impact on the prey detection ability of echolocating bats. J. R. Soc. Interface 11, 20130961. doi: 10.1098/rsif.2013.0961
Makowski, D., Ben-Shachar, M. S., Chen, S. H. A., and Lüdecke, D. (2019). Indices of effect existence and significance in the Bayesian framework. Front. Psychol. 10, 2767. doi: 10.3389/fpsyg.2019.02767
Malhi, Y., Aragão, L. E. O. C., Galbraith, D., Huntingford, C., Fisher, R., Zelazowski, P., et al. (2009). Exploring the likelihood and mechanism of a climate-change-induced dieback of the Amazon rainforest. PNAS 106, 20610–20615. doi: 10.1073/pnas.0804619106
Meiri, S., and Dayan, T. (2003). On the validity of Bergmann's rule. J. Biogeogr. 30, 331–351. doi: 10.1046/j.1365-2699.2003.00837.x
Metzner, W., and Müller, R. (2016). “Ultrasound production, emission, and reception.,” in Bat Bioacoustics, eds. M. B. Fenton, A. D. Grinnell, A. N. Popper, and R. R. Fay (New York, NY: Springer), 55–91. doi: 10.1007/978-1-4939-3527-7_3
Miočević, M., Gonzalez, O., Valente, M. J., and MacKinnon, D. P. (2018). A tutorial in bayesian potential outcomes mediation analysis. Struct. Equation Model. Multidisciplinary J. 25, 121–136. doi: 10.1080/10705511.2017.1342541
Mora, E. C., Ibáñez, C., Macías, S., Juste, J., López, I., and Torres, L. (2011). Plasticity in the echolocation inventory of Mormopterus minutus (Chiroptera, Molossidae). Acta 13, 179–187. doi: 10.3161/150811011X578723
Mtsetfwa, F., McCleery, R. A., and Monadjem, A. (2018). Changes in bat community composition and activity patterns across a conservation-agriculture boundary. Afr. Zool. 53, 99–106. doi: 10.1080/15627020.2018.1531726
Mutumi, G. L., Jacobs, D. S., and Winker, H. (2016). Sensory drive mediated by climatic gradients partially explains divergence in acoustic signals in two Horseshoe bat species, Rhinolophus swinnyi and Rhinolophus simulator. PLoS ONE 11, e0148053. doi: 10.1371/journal.pone.0148053
NASA (2020). Data from: The POWER Project. Available online at: https://power.larc.nasa.gov/
National Physical Laboratory (2018). Calculation of Absorption of Sound by the Atmosphere. Available online at: http://www.resource.npl.co.uk/acoustics/techguides/absorption/
Nordberg, U. M., and Rayner, J. M. V. (1987). Ecological morphology and flight in bats (Mammalia; Chiroptera): wing adaptations, flight performance, foraging strategy and echolocation. Phil. Trans. R. Soc. Lond. B 316, 335–427. doi: 10.1098/rstb.1987.0030
Olsson, U. H., Foss, T., Troye, S. V., and Howell, R. D. (2000). The performance of ML, GLS, and WLS estimation in structural equation modeling under conditions of misspecification and nonnormality. Struct. Equation Model. Multidisciplinary J. 7, 557–595. doi: 10.1207/S15328007SEM0704_3
Paradis, E., and Schliep, K. (2019). ape 5.0: an environment for modern phylogenetics and evolutionary analyses in R. Bioinformatics 35, 526–528. doi: 10.1093/bioinformatics/bty633
Pearl, J. (2009). Causality: Models, Reasoning and Inference. 2nd Edn. Cambridge: Cambridge University Press.
Pedro, A. R.-S., and Simonetti, J. A. (2014). Variation in search-phase calls of Lasiurus varius (Chiroptera: Vespertilionidae) in response to different foraging habitats. J. Mammal. 95, 1004–1010. doi: 10.1644/13-MAMM-A-327
Pimm, S. L., Jenkins, C. N., Abell, R., Brooks, T. M., Gittleman, J. L., Joppa, L. N., et al. (2014). The biodiversity of species and their rates of extinction, distribution, and protection. Science 344, 1246752. doi: 10.1126/science.1246752
R Core Team (2015). R: a language and environment for statistical computing. R Foundation for Statistical Computing, Vienna, Austria. Available online at: http://www.R-project.org/
Rubalcaba, J. G., Gouveia, S. F., Villalobos, F., Cruz-Neto, A. P., Castro, M. G., Amado, T. F., et al. (2022). Physical constraints on thermoregulation and flight drive morphological evolution in bats. Proc. Natl. Acad. Sci. U.S.A. 119, e2103745119. doi: 10.1073/pnas.2103745119
Russo, D., Ancillotto, L., and Jones, G. (2018). Bats are still not birds in the digital era: echolocation call variation and why it matters for bat species identification. Can. J. Zool. 96, 63–78. doi: 10.1139/cjz-2017-0089
Sankaran, M., Ratnam, J., and Hanan, N. P. (2004). Tree–grass coexistence in savannas revisited – insights from an examination of assumptions and mechanisms invoked in existing models. Ecol. Lett. 7, 480–490. doi: 10.1111/j.1461-0248.2004.00596.x
Schnitzler, H.-U., and Denzinger, A. (2011). Auditory fovea and Doppler shift compensation: adaptations for flutter detection in echolocating bats using CF-FM signals. J. Comp. Physiol. A Neuroethol. Sens. Neural Behav. Physiol. 197, 541–559. doi: 10.1007/s00359-010-0569-6
Schnitzler, H.-U., and Kalko, E. K. V. (2001). Echolocation by insect-eating bats. Bioscience 51, 557–569. doi: 10.1641/0006-3568(2001)0510557:EBIEB2.0.CO;2
Schnitzler, H.-U., Moss, C. F., and Denzinger, A. (2003). From spatial orientation to food acquisition in echolocating bats. Trends Ecol. Evol. 18, 386–394. doi: 10.1016/S0169-5347(03)00185-X
Shipley, B. (2016). Cause and Correlation in Biology: A User's Guide to Path Analysis, Structural Equations and Causal Inference With R. Cambridge: Cambridge University Press. doi: 10.1017/CBO9781139979573
Snell-Rood, E. C. (2012). The effect of climate on acoustic signals: does atmospheric sound absorption matter for bird song and bat echolocation? J. Acoust. Soc. Am. 131, 1650–1658. doi: 10.1121/1.3672695
Teng, S. N., Svenning, J.-C., Santana, J., Reino, L., Abades, S., and Xu, C. (2020). Linking landscape ecology and macroecology by scaling biodiversity in space and time. Curr. Landsc. Ecol. Rep. 5, 25–34. doi: 10.1007/s40823-020-00050-z
Thiagavel, J., Santana, S. E., and Ratcliffe, J. M. (2017). Body size predicts echolocation call peak frequency better than gape height in Vespertilionid bats. Sci. Rep. 7, 828. doi: 10.1038/s41598-017-00959-2
Thomas, S. P., Follette, D. B., and Farabaugh, A. T. (1991). Influence of air temperature on ventilation rates and thermoregulation of a flying bat. Am. J. Physiol. 260, R960–R968. doi: 10.1152/ajpregu.1991.260.5.R960
Thomas, S. P., and Suthers, R. A. (1972). The physiology and energetics of bat flight. J. Exp. Biol. 57, 317–335. doi: 10.1242/jeb.57.2.317
Vasko, V., Blomberg, A. S., Vesterinen, E. J., Suominen, K. M., Ruokolainen, L., Brommer, J. E., et al. (2020). Within-season changes in habitat use of forest-dwelling boreal bats. Ecol. Evol. 10, 4164–4174. doi: 10.1002/ece3.6253
Voigt, C. C., and Lewanzik, D. (2011). Trapped in the darkness of the night: thermal and energetic constraints of daylight flight in bats. Proc. R. Soc. B Biol. Sci. 278, 2311–2317. doi: 10.1098/rspb.2010.2290
von Hardenberg, A., and Gonzalez-Voyer, A. (2013). Disentangling evolutionary cause-effect relationships with phylogenetic confirmatory path analysis. Evolution 67, 378–387. doi: 10.1111/j.1558-5646.2012.01790.x
Keywords: bats, echolocation, adaptation, climate, biogeography, functional traits, bioacoustics, indirect effects
Citation: Kotila M, Helle S, Lehto HJ, Rojas D, Vesterinen EJ and Lilley TM (2022) Atmospheric humidity affects global variation of bat echolocation via indirect effects. Front. Ecol. Evol. 10:934876. doi: 10.3389/fevo.2022.934876
Received: 03 May 2022; Accepted: 09 August 2022;
Published: 06 September 2022.
Edited by:
Sidney F. Gouveia, Federal University of Sergipe, BrazilReviewed by:
Tinglei Jiang, Northeast Normal University, ChinaCopyright © 2022 Kotila, Helle, Lehto, Rojas, Vesterinen and Lilley. This is an open-access article distributed under the terms of the Creative Commons Attribution License (CC BY). The use, distribution or reproduction in other forums is permitted, provided the original author(s) and the copyright owner(s) are credited and that the original publication in this journal is cited, in accordance with accepted academic practice. No use, distribution or reproduction is permitted which does not comply with these terms.
*Correspondence: Thomas M. Lilley, dGhvbWFzLmxpbGxleUBoZWxzaW5raS5maQ==; Miika Kotila, bWhrb3RpQHV0dS5maQ==
Disclaimer: All claims expressed in this article are solely those of the authors and do not necessarily represent those of their affiliated organizations, or those of the publisher, the editors and the reviewers. Any product that may be evaluated in this article or claim that may be made by its manufacturer is not guaranteed or endorsed by the publisher.
Research integrity at Frontiers
Learn more about the work of our research integrity team to safeguard the quality of each article we publish.