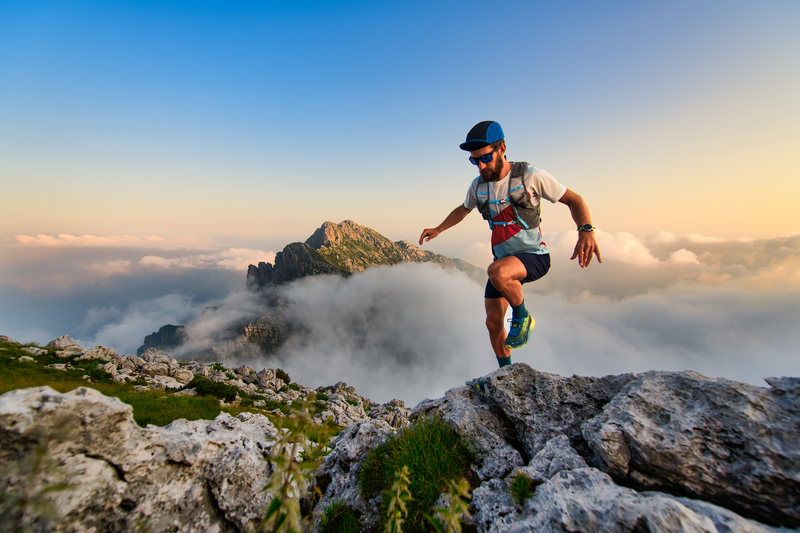
94% of researchers rate our articles as excellent or good
Learn more about the work of our research integrity team to safeguard the quality of each article we publish.
Find out more
ORIGINAL RESEARCH article
Front. Ecol. Evol. , 05 August 2022
Sec. Biogeography and Macroecology
Volume 10 - 2022 | https://doi.org/10.3389/fevo.2022.933424
This article is part of the Research Topic Rising Stars in Biogeography and Macroecology 2021 View all 4 articles
The effective conservation of highly migratory marine species is only possible if core areas of activity and critical habitat can be identified within the vast and dynamic oceanic environment and later on used to delineate marine protected areas (MPAs). However, gathering population-level data and identifying universal patterns within a species or population can be difficult when only a small sample size exists and individuals are not ecologically interchangeable. In addition, the open ocean beyond the Exclusive Economic Zone (EEZ) of a country is considered the high-seas and is not part of any jurisdiction and therefore challenging to govern by laws. Granting protection to species using these waters is sometimes virtually impossible. Another challenge is the dynamic nature of the oceanic environment. MPAs are usually based on spatially explicit and static areas, but migratory routes can shift following available food, currents, and temperatures or else, potentially rendering designated areas useless. The red-listed olive ridley turtle is known for its nomadic migratory and feeding behavior and a divergent nesting strategy among females. Our study used two approaches to identify critical habitats for the population nesting in Costa Rica and feeding in the Eastern Tropical Pacific. One was based on a static Kernel Density Approach to identify core areas. The other was a habitat preference model that took into account changing environmental variables such as sea surface temperature and chlorophyll-a concentrations. We were able to identify core areas at the population level by pooling two datasets and increasing our sample size. Our habitat preference model showed a high correlation of olive ridley presence with all tested environmental variables, except chlorophyll-a concentration. Our results reveal that olive ridleys use mainly regions that fall within EEZs and, therefore, the jurisdiction of six countries in Central America and provide an essential conservation tool.
Conservation planners are interested in implementing measures that will affect and protect the greatest number of individuals of a target species. To do this, it is essential to identify patterns that are truly reflective of the entire species or sets of populations and should consider the potentially high variability in behavior and resource use among populations, subpopulations, and even individuals. A crucial dimension of effective conservation and management is delineating critical habitat for an endangered or threatened species, which encompasses the specific geographic areas occupied by a species, containing the physical or biological features essential to their survival (US Fish and Wildlife Service, 2017).
Identification of critical habitat and how this relates to conservation strategies is particularly challenging for wide-ranging marine vertebrates, where populations use habitat that is both geographically expansive and that often spans multiple geopolitical boundaries (Block et al., 2011; Harrison et al., 2018). However, effective conservation across borders is only possible with coordinated international efforts in management, and clear scientific information about critical habitat of the species. The last decade has seen substantial progress in illuminating the movement ecology of large marine vertebrates, which has provided novel conservation opportunities (Shillinger et al., 2008; Block et al., 2011; Harrison et al., 2018). Satellite telemetry is still the most commonly used method for tracking wide-ranging marine animals (Block et al., 2011; Harrison et al., 2018). However, satellite telemetry is expensive, and often only small sample sizes inform policies and other conservation measures. This might not be a problem when most individuals of a population exhibit the same or very similar spatial patterns (Shillinger et al., 2008), and a small sample already reveals the general population patterns.
Identification of patterns in ecology typically depends on analyses of subsamples from a population, usually based upon a mean-field approach. Analyses of animal movements are no exception, especially when studying wide-ranging species that are followed with costly telemetry technology. It has been argued that only a small sample size is needed to deduce general spatial patterns for the entire population (Sequeira et al., 2019). However, that is only true for species and populations in which individuals are similar, almost ecologically interchangeable, in their migratory behavior. For instance, the mean-field approach might work well for species that migrate in large groups from one spatially explicit area to another using the same migratory corridors, such as herds of large mammals [e.g., wildebeest (Williamson et al., 1988), caribou (Fancy et al., 1989)], migratory birds that fly in large flocks between summer and winter grounds (Berthold, 2001), or fish [e.g., salmon (Thorpe, 1988), freshwater eels (Todd, 1981; van den Thillart et al., 2009)]. By contrast, for species where tracking is initiated in an area in which different populations with different patterns mingle, or where individuals exhibit high variation in behavior, small sample sizes can bias or hide overarching patterns. Even when a mean-field approach is used, parametric estimates are sensitive to the underlying density and dispersal (Bernardo, 1998).
For example, the International Union for Conservation of Nature (IUCN) typically focuses on species-level assessments (IUCN, 2019). The Endangered Species Act [ESA, United States (1983)] goes a step further, providing an explicit mechanism to categorize evolutionarily significant units (ESU) of conservation importance, which are considered distinct for conservation purposes because they are geographically separated, genetically different at neutral markers, or exhibit locally adapted phenotypic traits caused by differences in selection (Crandall et al., 2000). Another perspective was added by Crandall et al. (2000), who introduced the concept of ecological non-exchangeability and its implications for conservation. A further consideration that receives little attention and recognition is the individual variation in behavior which is also highly relevant to conservation (Sutherland, 1998). This relative lack of attention reflects the typological thinking that often characterizes ecological research (Bolnick et al., 2003; Violle et al., 2012).
The practice of using a typological approach in conservation is also illustrated in marine turtle stock assessments. While most marine turtle species exhibit intraspecific (among populations) variation in resource use (Figgener et al., 2019a) and genetically defined subpopulations have long been recognized as management units (Wallace et al., 2010; Pearson et al., 2017), most species have only been assessed globally for the IUCN red list (IUCN, 2019). The exceptions are the leatherback turtle (Dermochelys coriacea), loggerhead turtle (Caretta caretta), and green turtle (Chelonia mydas), where regional subpopulations have recently been assessed independently.1 Surprisingly little attention has been given to the most abundant of all species, the olive ridley turtle (Lepidochelys olivacea), which is still one of the least studied (Plotkin, 2007; Figgener et al., 2019b). It is classified globally as “vulnerable” by the IUCN, with some populations being stable or increasing [Abreu-Grobois and Plotkin (IUCN SSC Marine Turtle Specialist Group), 2008]. Olive ridleys exhibit two nesting behaviors (synchronized mass nesting, also called arribada nesting, and solitary nesting) that are sometimes both present within the same population (Bernardo and Plotkin, 2007). Most data informing the IUCN report are derived from arribada beaches, but little is known about the ecology and migratory behavior of solitary nesting individuals and populations [Abreu-Grobois and Plotkin (IUCN SSC Marine Turtle Specialist Group), 2008], although these might differ substantially from arribada females.
Unlike other marine turtle species, olive ridleys do not have clearly outlined spatially explicit foraging areas as adults, and post-breeding migrations appear randomly nomadic rather than directed (Plotkin, 2010). This migratory behavior is likely the result of their broad and opportunistic diet (Figgener et al., 2019a) and the diffuse nature of resources in the oceanic realm (Pennington et al., 2006; Sigman and Hain, 2012). This makes delineating critical habitats and predicting occurrences for olive ridleys difficult. As a result, a large sample size would be needed to identify spatially explicit areas of high use at the population level. Here, an additional habitat preference model could help identify underlying patterns that direct movements (Drexler and Ainsworth, 2013).
Another challenge for conservation efforts is that olive ridleys occur in oceanic, pelagic waters (Plotkin, 2010). Most areas of our ocean are beyond the national jurisdiction of a specific county and are called international waters or the high seas (Corrigan and Kershaw, 2008). If the areas of highest occurrence of olive ridleys were to fall within international waters, future attempts to implement conservation measures or establish protected areas could be challenging. However, some oceanic-pelagic waters still fall within the jurisdiction of individual countries. These waters are the Exclusive Economic Zones (EEZs) which extend 200 nautical miles (370 km) from the coastline (Vallega, 2002; Corrigan and Kershaw, 2008; Leathwick et al., 2008; Game et al., 2009). They could help establish protected migratory corridors for marine species governed by individual countries’ laws.
We pooled two datasets of locations from arribada and solitary nesting olive ridleys derived from post-nesting satellite tracks. We then took two approaches to identify and characterize the critical habitat for the population of olive ridley turtles nesting in Costa Rica and foraging in the wider Eastern Tropical Pacific (ETP). First, we used a Utilization Distribution (UD) modeling approach to delineate core areas of occurrence and the overall observed range of adult olive ridleys (Worton, 1989). To examine whether differences exist between arribada and solitary females, we calculated separate UDs for the two groups. Second, we employed a Habitat Preference Model (HPM) to correlate the presence of olive ridleys within the ETP to a selection of environmental variables related to productivity and static topographic features. Our aim is to provide concrete spatial guidance to governments and stakeholders in planning and developing marine protected areas (MPAs), including migratory corridors in the ETP.
We hypothesized that our combined data and hence larger sample size would reveal a population-level spatial pattern for olive ridleys nesting in Costa Rica. Additionally, we hypothesized that migratory routes differ between the two groups of females showing divergent nesting behaviors (arribada versus solitary). We further hypothesized that movements of solitary olive ridleys would have an equal amount of variation in core areas as those of arribada females. This would support the initial findings of nomadic migratory behavior (Plotkin, 2010) and a high degree of individual variation in movement patterns observed first in arribada females. Lastly, we hypothesized that the presence of olive ridley within the ETP could best be explained by the limitations of water temperatures, given that marine turtles are ectotherm, and high levels of primary productivity, which indicates the availability of food.
Our goal was to understand the oceanic movement ecology of olive ridleys in the ETP region using satellite telemetry. The coastal waters of Central America harbor large numbers of nesting sites and individuals (Bernardo and Plotkin, 2007; Abreu-Grobois and Plotkin (IUCN SSC Marine Turtle Specialist Group), 2008). One arribada population in the region, at Nancite Beach, Costa Rica, has previously been studied extensively (Plotkin, 1994, 2010; Plotkin et al., 1995, 1996, 1997; Valverde, 1996; Fonseca et al., 2009), but as yet nothing is known about the movement ecology of individuals from the other arribada beach, Ostional, nor from the numerous solitary nesting sites in Costa Rica. Therefore, we studied individuals from five nesting beaches (Ostional and four solitary beaches) along the Pacific coast of Costa Rica (Figure 1) between August and September 2016 and 2017.
Figure 1. Map of Costa Rican nesting sites and respective samples sizes where satellite transmitters were attached to olive ridley females about to depart on their post-nesting migrations between 1990 and 1992 (*Plotkin, 2010) and in 2016 and 2017 (this study). Open circles indicate solitary nesting beaches, and stars indicate arribada nesting beaches.
Because olive ridleys lay multiple egg clutches in a single nesting season (Plotkin, 2007), we wanted to identify only post-nesting females that would be departing nearshore areas after their last nesting event to begin a new foraging cycle. To determine reproductive status, we used ultrasonography (SonoSite 180+) to identify females that had completed their nesting cycle. We scanned the inguinal cavity of females that had completed nesting to examine the ovaries for evidence of vitellogenic follicles and oviducts for shelled eggs (Rostal et al., 1998). If no shelled eggs and vitellogenic follicles were present in either oviduct or ovary, we inferred that the turtle was a suitable candidate for the attachment of a satellite transmitter. We attached satellite transmitters only to turtles that appeared in good health and had no apparent injuries.
We attached satellite transmitters to the second vertebral scute of the carapace using the fiberglass-resin method (Plotkin, 1998) in 2016 and cold-setting two-phase epoxy (Pure50 +, POWERS ™) in 2017 (Mansfield et al., 2009; Arendt et al., 2012). Turtles were restrained on the nesting beach by hand during the attachment procedure (approximately 4 h in 2016, 2 h in 2017). We used several types of transmitters: SeaTagTT in 2016 (n = 7, Deserts Star LLC), SeaTrkr-4370-4 in 2017 (n = 2, Telonics, Inc.), and TAM-4310-3 in 2017 (n = 17, Telonics, Inc.). After transmitter attachment, we marked each turtle with two metal tags (Inconel 601, National Band and Tag Company, Inc.), one on the trailing edge of each front flipper. We then released turtles from restraint so they could return to the water. Our transmitters in 2016 were solar-powered and consistently turned on. The transmitters in 2017 were programmed with a transmission (duty) cycle of alternatingly 6 h on/50 h off and 6 h on/58 h off, resulting in transmissions alternating between UTC 11:00–17:00 and UTC 19:00–01:00 (early morning and late afternoon local time). We chose these times by combining the knowledge of peak times for surface sun-basking behavior in olive ridleys (CF personal observations) and the predicted satellite overflights for the region (ARGOS website).
We obtained locations from the satellite transmitters via the Argos Satellite System (Maryland, United States), excepting the two SeaTrkr transmitters, which utilize the IRIDIUM Satellite System (Maine et al., 1995; Figgener et al., 2018). Argos supplies an accuracy estimate via location classes (LC) for each calculated latitude and longitude; these include LC 3, 2, 1, 0, A, B, or Z (Douglas et al., 2012). Argos has estimated that accuracy in latitude and longitude for LC 3 is < 150 m, from 150 to 350 m for LC 2, from 350 to 1,000 m for LC 1, and > 1,000 m for LC 0 (Argos, 1996). Locations from class Z were rejected because they constitute invalid locations. Argos provides no estimation of location accuracy for LC A, and LC B. Hays et al. (2019) found that the accuracy of LC A was comparable to that of LC 1 and LC B had less accuracy than LC A. However, the lowest level of accuracy was found in LC 0. IRIDIUM provides coordinates based on GPS, and the accuracy estimations for each location are < 11 m (Figgener et al., 2018). Data were filtered using a maximum speed of 5 km d–1, and only one daily location (in the case of ARGOS locations, the most accurate based on location class) was retained.
In our first approach to delineate critical habitat, we used a Utilization Distribution (UD) based on Kernel Density Estimates (KDEs). These estimates address the space use of populations and individuals (Burt, 1943) via location probability density estimates (Worton, 1989; Fleming and Calabrese, 2017) focused on the identification of high-density regions (HDRs, Hyndman, 1996). More specifically, the use of areas within the ETP by females was characterized by the number of locations per grid cell. The UD was calculated by first determining the number of positions per grid cell and then normalized to the proportion of total locations per grid cell by dividing by the total number of locations used in the analyses. These proportions were sorted from largest to smallest and the cumulative proportion of locations per grid cell was determined to create UDs. This was done using ArcGIS (Version 10.6, ESRI). We used Percent Volume Contours to depict the areas that were used most intensely, PVC50 (core areas, which are simply the 50% HDR of the location distribution), as well as the overall range, PVC95 (95% HDR of the location distribution). For these analyses, we combined the data from the newly tracked turtles in this study with previously published data from Nancite beach (Plotkin, 1994, 2010).
To determine differences in critical habitat between arribada and solitary females, as well as among individuals, we calculated separate UDs at two hierarchical levels. The first comparison was arribada (18 tracks, 658 locations) versus solitary (16 tracks, 479 locations) female tracks. Arribada female tracks were derived mainly from the Plotkin dataset with two additional tracks from this study. The second comparison contrasted the tracks of 10 solitary females (n = 10) from 2017. The solitary female data included tracks with more than 30 locations (after filtering) from all five beaches. ArcGIS v. 10.6.1 was used to calculate overlaps and generate maps.
In our second approach, we attempted to identify which environmental variables best predicted the occurrence of olive ridley females in the ETP in 2016 and 2017. Because data on the true absence of olive ridleys were unavailable, we first created a presence-absence raster in ArcGIS 10.6.1 based on our satellite locations (Barbet-Massin et al., 2012). To do this, we randomly generated a raster of an equal amount of pseudo-absence locations compared to our present locations from cells of no presence records (Barbet-Massin et al., 2012). While we only know the locations visited by our tagged turtles, all the turtle movements represent habitat choices, presumably driven by habitat preferences and the niches occupied by the species. Therefore, any locations our turtles could have feasibly visited in our study area but chose not to visit, represent potentially true absences for the purposes of understanding habitat preferences. Next, we overlaid our locations (presence and absence) with remote sensed environmental data using the automated annotation service EnvData on MOVEBANK.2 All of the subsequent data layers for our environmental variables are derived from MOVEBANK: bathymetry data at a spatial resolution of 0.017° were provided by the global relief model (ETOPO1); distances to the nearest coast at a spatial solution of 0.04° (NASA); 8-day composites of chlorophyll-a (as an index of phytoplankton biomass and proxy of primary production), particulate organic carbon, particulate inorganic carbon, and sea surface temperature at a spatial resolution of 4 km (MODIS Ocean); 8-day composites of euphotic depth as another proxy of primary productivity [the depth at which 1% of the sea surface photosynthetically active radiation remains (Kirk, 1994)] at a spatial resolution of 4 km (Suomi-NPP VIIRS Ocean). We used R (R Core Team, 2019) to test for covariance between the environmental variables. Last, we used the annotated locations in a general additive modeling (GAM) approach to explain the observed presence-absence pattern of olive ridleys in the ETP (MacLeod and Zuur, 2005). We chose the GAM approach because many environmental variables that define the niches occupied by marine species have non-linear relationships. GAMs can cope with non-linear relationships without making any a priori assumptions as to their shape, which is crucial when investigating habitat preferences. We fitted the GAM using the gam function in the R package mgcv (Wood, 2017) and ran a total of 23 models which we compared to the full model with all environmental predictor variables. The best models were chosen using the Akaike information criterion (AIC). To better understand the results of our GAM models, we created a classification tree (De’ath and Fabricius, 2000; MacLeod and Zuur, 2005) using the rpart R-software package (Therneau et al., 2013).
In total, 23 olive ridley females were fitted with satellite transmitters on five nesting beaches along the Pacific coast of Costa Rica (Figure 1) between August and September 2016 and 2017. We also used previously published data from 19 females (20 tracks) departing from the arribada beach Nancite in Costa Rica (Plotkin, 1994, 2010). The UDs based on a sample size of all 43 tracks (1,553 locations) revealed a population-level spatial pattern for the occurrence of olive ridleys in the ETP (Figure 2). The collective core areas (PVC50) of all locations fell within 400 km of the coasts of Panama, Costa Rica, Nicaragua, El Salvador, Guatemala, and Mexico. Further, it is important to note that core areas fell within the EEZs of these countries (Figure 2B).
Figure 2. Utilization Distributions (UD) of olive ridleys within the Eastern Tropical Pacific (ETP) based on KDEs from 43 individual females tracked during their post-nesting migrations from Costa Rican nesting grounds (Data: Plotkin, 2010 and this study). (A) Identified high use areas using locations and a UD in the ETP based on 43 tracks. Red polygons demark PVC50 (=core areas) and sand colored polygons demark PVC95 based on KDEs. (B) Locations and core areas of high use overlaid onto the outlines of the Exclusive Economic Zones (Flanders Marine Institute, 2014) within the ETP.
The comparison between UDs of arribada and solitary females revealed substantial differences (Figures 3, 4 and Table 1). Only 19.7% of arribada females’ core areas (PVC50) overlapped with the core areas of solitary females. Only 14.3% of solitary females’ core areas overlapped with the core areas of arribada females (Table 1). The core area of arribada females was one large connected area that was overall smaller (Table 1) and in general closer to the coast stretching along the coast of northern Costa Rica, Nicaragua, El Salvador, and Guatemala than the solitary female core areas (Figures 3, 4 and Table 1). By contrast, the core areas of solitary females were four disconnected areas that were overall larger (Table 1) than the core area of arribada females. Three solitary core areas were close to the coast whereas one area was farther from the closest coastline. In general, arribada females had no core areas south of their nesting beaches, whereas solitary females had two large areas.
Figure 3. Comparison of UDs from arribada and solitary olive ridley females. Red polygons demark PVC50 (=core areas) and sand-colored polygons demark PVC95 based on KDEs. (A) Locations and UD of arribada females. (B) Locations and UD of solitary females.
Figure 4. Overlap of UDs from arribada and solitary olive ridley females. Dark red polygons demark PVC50 (=core areas) for arribada females, light red polygons PVC50 for solitary females. Dark sand-colored polygons demark PVC95 for arribada females and light sand-colored polygons PVC95 for solitary females.
Table 1. Comparison of UDs (PVC50 and PVC95) within the ETP between arribada and solitary olive ridley females.
The comparison of tracks among ten solitary females from 2017 and their respective core areas (PVC50) showed little overlap (Figure 5).
Figure 5. Comparison of tracks from ten solitary olive ridley turtle females and their respective core areas of high use (PVC50) in the Eastern Tropical Pacific.
Our habitat preference model aimed to correlate the observed presence of olive ridley presence based on seven environmental predictor variables: water depth (depth), distance to coast (dist_coast), particulate organic carbon (POC), particulate inorganic carbon (PIC), euphotic depth (euph_depth), chlorophyll-a concentrations (chlo-a), and sea surface temperature (SST). The only strong covariance existed between POC and chlo-a, which we considered in our model choices and interpretations. The best two models, identified by the AIC, were the full model and the model that did not contain chlorophyll-a as a variable [AIC 515.1 and 514.0, respectively (Table 2)]. Both models explained the same amount of variation in olive ridley presence-absence (deviance of 71.8% and had an adjusted R2 of 0.76) (Figure 6 and Tables 2, 3). All predictor variables showed a significant relationship to olive ridley presence (excepting chlorophyll-a) with p < 0.005. Our CART identified euphotic depth, SST, and distance to coast, as the most important variables, followed by POC. In general, presence was lowest in shallow waters through depths of 4,000 m where presence increases. The relationship between olive ridley presence and distance to the nearest coast is negative, with presence decreasing farther away from land. The relationship between euphotic depth and presence was similar, with presence decreasing with increasing depth. The maximum presence is at a euphotic depth of 20 m or less. Presence increases with increasing water temperature and plateaus at values of approximately > 25°C. Both PIC and POC have a curved relationship with presence. However, PIC has an inverted relationship, with presence decreasing with increasing PIC values until a minimum at 0.0005 and then increasing again. For POC, presence increases with increasing values until a maximum of 150 before decreasing.
Table 2. General additive model selection for habitat preference model of olive ridley turtles in the Eastern Tropical Pacific using different environmental variables as predictors for presence.
Figure 6. Summary plot for the GAM with the highest explanatory power for the presence-absence pattern of olive ridley turtles observed in the Eastern Tropical Pacific (see also Tables 2, 3). The separate panels depict how each environmental variable (x-axis) interacts with the dependent variable presence (y-axis): (A) water depth; (B) distance to the nearest coastline; (C) particulate inorganic carbon (PIC); (D) particulate organic carbon (POC); (E) euphotic depths; and (F) sea surface temperature (SST).
Table 3. Summary of the explanatory power of the six environmental variables used to predict olive ridley turtle presence in the ETP based on the best general additive model (GAM16, see Table 2); EDF are the estimated degrees of freedom.
The differences in the tracks among the 10 solitary females that were compared are also evident in the large range in mean values for depth, distance to coast, and size of core areas (Table 4). By contrast, little variation existed in mean SST (Table 4). Most core areas were north of Costa Rica except those of two individuals whose core areas were far South [off southern Colombia and northern Ecuador, and off southern Costa Rica and northern Panama (Figure 5)].
Table 4. Mean values, coefficient of variation (CV), and range in values for water depth, distance to coast, core area size, and sea surface temperature (SST) in ten individual tracks of solitary olive ridley females in the Eastern Tropical Pacific.
Our first approach to delineate critical habitat for olive ridleys pooled two datasets to increase sample size and used UDs to estimate core areas of high use for the population and describe its overall range in the ETP. This revealed a population-level spatial pattern and indicates areas of conservation importance (Figure 2) within the EEZs of six countries: Mexico, Guatemala, El Salvador, Nicaragua, Costa Rica, and Panama.
However, given the divergent nesting strategies within the Costa Rican population, we also compared the UDs between arribada and solitary females (Figure 3). It showed that core areas differed substantially from each other in their spatial distribution, as well as in size. This aligns with the findings of previous studies comparing other aspects of arribada and solitary females documenting differences in life history, physiology, and ecology between the two groups. For instance, the inter-nesting interval of arribada females is 28 days whereas solitary females re-nest in intervals of 14–20 days (Kalb, 1999; Bernardo and Plotkin, 2007; Figgener et al., 2018). A recent study also demonstrated that arribada females have an enhanced capacity of maintaining an extended embryonic arrest in their eggs compared to solitary females (Williamson et al., 2019). Further, arribada females show a higher level (90%) of multiple paternity in their clutches than solitary females (30%) (Jensen et al., 2006). Our quantitative characterization of differences in the spatially explicit foraging patterns between arribada and solitary females lends further support to the argument that the two groups should be assessed separately for conservation purposes.
To determine whether the nomadic nature of arribada females is a universal pattern within the studied population of olive ridleys, we compared the tracks of 10 solitary females and their respective core areas (Figure 5). It showed that little overlap exists among areas and that tracks appear undirected, similar to the individual tracks of arribada females previously described (Plotkin, 2010). While marine turtles are not social animals and do not move in aggregations, in other marine turtle species, individuals or subgroups of the same population share migratory corridors because their breeding grounds and foraging grounds are the same spatially explicit areas (Shillinger et al., 2008). This is not the case for olive ridleys. This interindividual variation presents a challenge for the delineation of spatially explicit critical habitat for olive ridleys in the ETP and indicates that a typological approximation is not the most robust approach for this population.
Our second approach to characterize the critical habitat for olive ridleys in the ETP used a habitat preference model based on environmental variables. This approach might correlate the presence more accurately as it takes into account the dynamic nature of the oceanic environment and might better explain the apparently undirected migratory routes not leading to specific locations, and the high degree of individual variation in migratory behavior. Our habitat preference model indicated that all variables, except chlorophyll-a, had a significant relationship with the dependent variable (presence) and the model containing all of these variables (not including chlorophyll-a) had the lowest AIC and explained the highest proportion of deviance (71.8%) (Tables 2, 3). During model selection, we were able to identify SST as one of the variables with the highest explanatory power (Figure 6), which indicates that temperature might be a restricting factor for occurrence. Marine turtles are ectotherms, and their core body temperature generally ranges from 26 to 30°C (Mrosovsky, 1980). The associated limitations in the thermal regime are reflected in the summary of our model, which shows that the species presence is highest in water temperatures above ∼25°C. Additional evidence of the importance of water temperatures for the prediction of occurrence can be found in our comparison of mean SST values among individual tracks. SST was the variable that showed the least variation. The model summary also reveals that occurrence peaks in waters within 400 m to the next coastline. Productivity is usually highest close to the coasts where estuarine run-off provides many nutrients (Kirk, 1994; Pennington et al., 2006; Sigman and Hain, 2012). The northeastern tropical waters close to the coast are especially productive because they harbor the Middle American Trench running close to the Central American coastline (Pennington et al., 2006), as well as the Costa Rican Thermic Dome (Jimenez, 2017). Both features enhance upwelling close to the coast. The preference for highly productive waters is also reflected in our model as the highest presence was in waters with euphotic depths of 30 m, as well as high levels of PIC and POC. We have to point out that the habitat preferences only apply to the specific individuals we tagged, but we have no reason to believe that they may not be representative of different populations or the species as a whole.
In order to implement adequate protective measures, practitioners need to be able to identify migratory corridors and areas of high use at the population level of a species. Money is often in short supply in conservation (Plotkin and Bernardo, 2014), and expensive technologies to gather data are often used on only a small sample (Sequeira et al., 2019). The population of olive ridleys in the ETP exhibits a high level of individual variation and, therefore, identifying critical habitat for the entire population based on a small subsample is not the correct approach to inform conservation measures for the entire population. This individual variation in movement patterns presents indeed a challenge for conservation as protecting a static spatially explicit area will not guarantee the protection of the entire population.
An important observation from our study is that the core areas of space use of the females examined (based on 43 tracks) fall within the EEZs of six countries. While the high seas are, in general, international waters outside of the jurisdiction of one country, countries do have authority over their EEZs and can implement protective measures (Vallega, 2002; Corrigan and Kershaw, 2008; Leathwick et al., 2008; Game et al., 2009). For instance, in Gabon, Central Africa, satellite tracking data obtained for leatherback turtles (Dermochelys coriacea) and olive ridleys were used to help delineate critical habitats and identify overlap with human activities, such as fisheries (Maxwell et al., 2011; Casale et al., 2017; Dawson et al., 2017; Pikesley et al., 2018; Hays et al., 2019). This information was then mobilized to extend a marine protected area network, which now encompasses 27% of the nation’s EEZ (Hays et al., 2019).
Similarly, our study identifies new opportunities for conservation. For instance, olive ridleys, like most other marine turtle species, have a high vulnerability to fisheries bycatch. One study estimated that 699,600 olive ridleys had been incidentally caught in fishing lines between 1999 and 2010 within the EEZ of Costa Rica and Panama (Dapp et al., 2013). Those numbers are likely conservative estimates based on reported and observed bycatch rates only in Costa Rican and Panamanian waters. However, the density of fishing boats is high in the EEZs of Nicaragua, El Salvador, Guatemala, and Mexico and the numbers might be a magnitude higher. The delineation of the spatially explicit critical habitat provides the opportunity to regulate fisheries in core areas and maybe even to establish no-take zones.
Recently, ecosystem-based management approaches are helpful to integrate the satellite tracking data into more dynamic ocean management. These approaches combine presence data derived from satellite tracking with real-time oceanographic data to adaptively predict the presence of a species and protect it (Maxwell et al., 2015; Hays et al., 2019). The results of our study suggest that in the case of olive ridleys, an approach based on real-time oceanographic data might yield better results in predicting the presence of individuals within the ETP than classic static critical habitat approaches.
Our study shows that individual differences in migratory patterns and the resulting ecological non-interchangeability paired with the dynamic nature of the oceanic environment pose a true challenge to conservation planning. But, it also provides a material basis to inform conservation measures in the ETP. The identified areas of high use of olive ridleys departing from nesting sites in Costa Rica are falling within jurisdictions of specific countries which should be used in the implementation of conservation measures. However, our study demonstrates that a typological description of the critical habitat of olive ridleys in the ETP is not enough to delineate occurrence and we suggest that for more effective conservation measures, spatially explicit data should be combined with a more dynamic species modeling approach based on environmental variables to predict presence.
The raw data supporting the conclusions of this article will be made available by the authors, without undue reservation.
The satellite transmitter deployment was performed following the Animal Use Protocol of the Animal Care and Use Committee of Texas A&M University, United States (IACUC-2016-0213), and was permitted, reviewed and approved by the Costa Rican Government (MINAE/SINAC # ACT-OR-DR-074-16, ACTOR- DR-109-16, ACG-PI-026-2016, ACOPAC-INV019-16, INV-ACOSA-030-16, SINAC-SE-CUSBE-PI-R-0095-2017, and SINAC-SE-CUS-PI-R-0106-2017).
CF designed the study, carried out the field work, collected primary data and mined data, carried out the statistical analyses, and drafted the manuscript. JB participated in the design of the study and co-drafted the manuscript. PP provided primary data, participated in the design of the study, and critically revised the manuscript. All authors gave final approval for publication and agreed to be held accountable for the work performed therein.
This research was supported by grants from Texas A&M University, Texas Sea Grant Grants-in-Aid of Graduate Research, PADI Grant #21778, and Lerner-Gray-Fund for Marine Research. The dissertation research that collected the data of the older data sets was supported by the US National Science Foundation (grants BNS-9000075 and BNS-8819940 to D. Owens).
We thank our field research assistants Andrey Castillo MacCarthy, Erick Beck, Derek Dunlop, Cody Glasbrenner, Hunter Holly, Brie Myre, Candice Oliver, Kim Lato, and Brittney Traynor for their help deploying the satellite transmitters. We would also like to thank the Area de Conservación Guanacaste for allowing fieldwork at the Nancite Beach Research Station and R. Blanco, who facilitated field logistics and permits. We thank Mike Arndt for his insights on alternative attachment methods and Andrew DiMatteo and Nathan Putman for help with spatial data analyses. We would like to thank our two reviewers for improving our manuscript, as well as Colin MacLeod for advising us on our habitat preference modeling approach. Lastly, we would also like to thank Duncan MacKenzie and Lee Fitzgerald for improving earlier versions of this manuscript.
The authors declare that the research was conducted in the absence of any commercial or financial relationships that could be construed as a potential conflict of interest.
All claims expressed in this article are solely those of the authors and do not necessarily represent those of their affiliated organizations, or those of the publisher, the editors and the reviewers. Any product that may be evaluated in this article, or claim that may be made by its manufacturer, is not guaranteed or endorsed by the publisher.
Abreu-Grobois, F. A., and Plotkin (IUCN SSC Marine Turtle Specialist Group), P. T. (2008). Lepidochelys olivacea. The IUCN Red List of Threatened Species 2008. Gland: IUCN.
Arendt, M. D., Segars, A. L., Byrd, J. I., Boynton, J., Whitaker, J. D., Parker, L., et al. (2012). Distributional patterns of adult male loggerhead sea turtles (Caretta caretta) in the vicinity of Cape Canaveral, Florida, USA during and after a major annual breeding aggregation. Mar. Biol. 159, 101–112. doi: 10.1007/s00227-011-1793-5
Barbet-Massin, M., Jiguet, F., Albert, C. H., and Thuiller, W. (2012). Selecting pseudo-absences for species distribution models: How, where and how many? Methods Ecol. Evol. 3, 327–338. doi: 10.1111/j.2041-210X.2011.00172.x
Bernardo, J. (1998). “The Logic, Value, and Necessity of Grounding Experiments in Reality: An Essential Link in the Inferential Chain Back to Nature,” in Experimental Ecology: Issues and Perspectives, eds W. J. Resetarits and Jr. J. Bernardo (New York, NY: Oxford University Press), 370–393.
Bernardo, J., and Plotkin, P. T. (2007). “An evolutionary perspective on the arribada phenomenon and reproductive behavioral polymorphism of olive ridley sea turtles (Lepidochelys olivacea),” in Biology and conservation of ridley sea turtles, ed. P. T. Plotkin (Baltimore, MA: Johns Hopkins University Press), 59–87.
Block, B. A., Jonsen, I. D., Jorgensen, S. J., Winship, A. J., Shaffer, S. A., Bograd, S. J., et al. (2011). Tracking apex marine predator movements in a dynamic ocean. Nature 475:86. doi: 10.1038/nature10082
Bolnick, D. I., Svanbäck, R., Fordyce, J. A., Yang, L. H., Davis, J. M., Hulsey, C. D., et al. (2003). The ecology of individuals: incidence and implications of individual specialization. Am. Nat. 161, 1–28. doi: 10.1086/343878
Burt, W. H. (1943). Territoriality and home range concepts as applied to mammals. J. Mammal. 24, 346–352.
Casale, P., Abitsi, G., Aboro, M. P., Agamboue, P. D., Agbode, L., Allela, N. L., et al. (2017). A first estimate of sea turtle bycatch in the industrial trawling fishery of Gabon. Biodivers. Conserv. 26, 2421–2433. doi: 10.1007/s10531-017-1367-z
Corrigan, C., and Kershaw, F. (2008). Working Toward High Seas Marine Protected Areas: An Assessment of Progress Made and Recommendations for Collaborations. Cambridge: Cambridge University.
Crandall, K. A., Bininda-Emonds, O. R. P., Mace, G. M., and Wayne, R. K. (2000). Considering evolutionary processes in conservation biology. Trends Ecol. Evol. 15, 290–295. doi: 10.1016/S0169-5347(00)01876-0
Dapp, D., Arauz, R., Spotila, J. R., and O’Connor, M. P. (2013). Impact of Costa Rican longline fishery on its bycatch of sharks, stingrays, bony fish and olive ridley turtles (Lepidochelys olivacea). J. Exp. Mar. Biol. Ecol. 448, 228–239. doi: 10.1016/j.jembe.2013.07.014
Dawson, T. M., Formia, A., Agamboué, P. D., Asseko, G. M., Boussamba, F., Cardiec, F., et al. (2017). Informing marine protected area designation and management for nesting olive ridley sea turtles using satellite tracking. Front. Mar. Sci. 4:312. doi: 10.3389/fmars.2017.00312
De’ath, G., and Fabricius, K. E. (2000). Classification and regression trees: a powerful yet simple technique for ecological data analysis. Ecology 81, 3178–3192. doi: 10.1890/0012-9658(2000)081[3178:CARTAP]2.0.CO;2
Douglas, D. C., Weinzierl, R. C., Davidson, S., Kays, R., Wikelski, M., and Bohrer, G. (2012). Moderating Argos location errors in animal tracking data. Methods Ecol. Evol. 3, 999–1007. doi: 10.1111/j.2041-210X.2012.00245.x
Drexler, M., and Ainsworth, C. H. (2013). Generalized additive models used to predict species abundance in the Gulf of Mexico: an ecosystem modeling tool. PLoS One 8:e64458. doi: 10.1371/journal.pone.0064458
Fancy, S. G., Pank, L. F., Whitten, K. R., and Regelin, W. L. (1989). Seasonal movements of caribou in arctic Alaska as determined by satellite. Can. J. Zool. 67, 644–650. doi: 10.1139/z89-093
Figgener, C., Bernardo, J., and Plotkin, P. T. (2018). “Successful use of the IRIDIUM satellite system to study the fine-scale movements of internesting marine turtles,” in Poster at the 38th International Symposium on Sea Turtle Biology and Conservation, Kobe.
Figgener, C., Bernardo, J., and Plotkin, P. T. (2019a). Beyond trophic morphology: stable isotopes reveal ubiquitous versatility in marine turtle trophic ecology. Biol. Rev. 94, 1947–1973. doi: 10.1111/brv.12543
Figgener, C., Bernardo, J., and Plotkin, P. T. (2019b). MarTurtSI, a global database of stable isotope analyses of marine turtles. Sci. Data 6:16. doi: 10.1038/s41597-019-0030-9
Flanders Marine Institute (2014). Union of the ESRI Country shapefile and the Exclusive Economic Zones (version 2). Available online at: http://www.marineregions.org (accessed Sep. 18, 2019).
Fleming, C. H., and Calabrese, J. M. (2017). A new kernel density estimator for accurate home-range and species-range area estimation. Methods Ecol. Evol. 8, 571–579.
Fonseca, L. G., Murillo, G. A., Guadamuz, L., Spinola, R. M., and Valverde, R. A. (2009). Downward but stable trend in the abundance of arribada olive ridley sea turtles (Lepidochelys olivacea) at Nancite Beach, Costa Rica (1971-2007). Chelon. Conserv. Biol. 8, 19–27. doi: 10.2744/CCB-0739.1
Game, E. T., Grantham, H. S., Hobday, A. J., Pressey, R. L., Lombard, A. T., Beckley, L. E., et al. (2009). Pelagic protected areas: the missing dimension in ocean conservation. Trends Ecol. Evol. 24, 360–369. doi: 10.1016/j.tree.2009.01.011
Harrison, A.-L., Costa, D. P., Winship, A. J., Benson, S. R., Bograd, S. J., Antolos, M., et al. (2018). The political biogeography of migratory marine predators. Nat. Ecol. Evol. 2, 1571–1578. doi: 10.1038/s41559-018-0646-8
Hays, G. C., Bailey, H., Bograd, S. J., Bowen, W. D., Campagna, C., Carmichael, R. H., et al. (2019). Translating marine animal tracking data into conservation policy and management. Trends Ecol. Evol. 34, 459–473. doi: 10.1016/j.tree.2019.01.009
Jensen, M. P., Abreu-Grobois, F. A., Frydenberg, J., and Loeschcke, V. (2006). Microsatellites provide insight into contrasting mating patterns in arribada vs. non-arribada olive ridley sea turtle rookeries. Mol. Ecol. 15, 2567–2575. doi: 10.1111/j.1365-294X.2006.02951.x
Jimenez, J. A. (2017). The Thermal Dome of Costa Rica: An oasis of productivity at the Pacific coast of Central America. Costa Rica: San Jose.
Kalb, H. (1999). Behavior and Physiology of Solitary and Arribada Nesting Olive Ridley Sea Turtles (Lepidochelys olivacea) During the Internesting Period. Ph.D. thesis. Texas: Texas A&M University.
Kirk, J. T. O. (1994). Light and Photosynthesis in Aquatic Ecosystems. Cambridge: Cambridge University Press. doi: 10.1017/CBO9780511623370
Leathwick, J., Moilanen, A., Francis, M., Elith, J., Taylor, P., Julian, K., et al. (2008). Novel methods for the design and evaluation of marine protected areas in offshore waters. Conserv. Lett. 1, 91–102. doi: 10.1111/j.1755-263X.2008.00012.x
MacLeod, C. D., and Zuur, A. F. (2005). Habitat utilization by Blainville’s beaked whales off Great Abaco, northern Bahamas, in relation to seabed topography. Mar. Biol. 147, 1–11. doi: 10.1007/s00227-004-1546-9
Maine, K., Devieux, C., and Swan, P. (1995). “Overview of IRIDIUM® Satellite Network,” in Proceedings of the WESCON/’95. Conference Record. ‘Microelectronics Communications Technology Producing Quality Products Mobile and Portable Power Emerging Technologies’, Chandler, AZ.
Mansfield, K. L., Saba, V. S., Keinath, J. A., and Musick, J. A. (2009). Satellite tracking reveals a dichotomy in migration strategies among juvenile loggerhead turtles in the Northwest Atlantic. Mar. Biol. 156, 2555–2570. doi: 10.1007/s00227-009-1279-x
Maxwell, S. M., Breed, G. A., Nickel, B. A., Makanga-Bahouna, J., Pemo-Makaya, E., Parnell, R. J., et al. (2011). Using satellite tracking to optimize protection of long-lived marine species: olive ridley sea turtle conservation in Central Africa. PLoS One 6:e19905. doi: 10.1371/journal.pone.0019905
Maxwell, S. M., Hazen, E. L., Lewison, R. L., Dunn, D. C., Bailey, H., Bograd, S. J., et al. (2015). Dynamic ocean management: defining and conceptualizing real-time management of the ocean. Mar. Policy 58, 42–50. doi: 10.1016/j.marpol.2015.03.014
Mrosovsky, N. (1980). Thermal biology of sea turtles. Am. Zool. 20, 531–547. doi: 10.1093/icb/20.3.531
Pearson, R. M., van de Merwe, J. P., Limpus, C. J., and Connolly, R. M. (2017). Realignment of sea turtle isotope studies needed to match conservation priorities. Mar. Ecol. Progress Ser. 583, 259–271. doi: 10.3354/meps12353
Pennington, J. T., Mahoney, K. L., Kuwahara, V. S., Kolber, D. D., Calienes, R., and Chavez, F. P. (2006). Primary production in the eastern tropical Pacific: a review. Progress Oceanogr. 69, 285–317. doi: 10.1016/j.pocean.2006.03.012
Pikesley, S. K., Agamboue, P. D., Bayet, J. P., Bibang, J. N., Bonguno, E. A., Boussamba, F., et al. (2018). A novel approach to estimate the distribution, density and at-sea risks of a centrally-placed mobile marine vertebrate. Biol. Conserv. 221, 246–256. doi: 10.1016/j.biocon.2018.03.011
Plotkin, P., and Bernardo, J. (2014). Sea turtle funding dries up. Science 343, 484. doi: 10.1126/science.343.6170.484-a
Plotkin, P. T. (1994). Migratory and reproductive behavior of the olive ridley turtle, Lepidochelys olivacea (Eschscholtz, 1829), in the eastern Pacific Ocean. Ph.D. thesis. Texas: Texas A&M University.
Plotkin, P. T. (1998). Interaction between behavior of marine organisms and the performance of satellite transmitters: a marine turtle case study. Mar. Technol. Soc. J. 32, 5–10.
Plotkin, P. T. (2010). Nomadic behaviour of the highly migratory olive ridley sea turtle Lepidochelys olivacea in the Eastern Tropical Pacific Ocean. Endanger. Spec. Res. 13, 33–40. doi: 10.3354/esr00314
Plotkin, P. T., Byles, R. A., Rostal, D. C., and Owens, D. W. (1995). Independent versus socially facilitated oceanic migrations of the olive ridley, Lepidochelys olivacea. Mar. Biol. 122, 137–143. doi: 10.1007/bf00349287
Plotkin, P. T. (ed.) (2007). Biology and Conservation of Ridley Sea Turtles. Baltimore, MA: Johns Hopkins University Press.
Plotkin, P. T., Owens, D. W., Byles, R. A., and Patterson, R. (1996). Departure of male olive ridley turtles (Lepidochelys olivacea) from a nearshore breeding ground. Herpetologica 52, 1–7.
Plotkin, P. T., Rostal, D. C., Byles, R. A., and Owens, D. W. (1997). Reproductive and developmental synchrony in female Lepidochelys olivacea. J. Herpetol. 31, 17–22. doi: 10.2307/1565323
R Core Team (2019). R: A Language and Environment for Statistical Computing. Vienna: R Foundation for Statistical Computing.
Rostal, D. C., Owens, D. W., Grumbles, J. S., MacKenzie, D. S., and Amoss, M. S. Jr. (1998). Seasonal reproductive cycle of the Kemp’s ridley sea turtle (Lepidochelys kempi). Gen. Comp. Endocrinol. 109, 232–243. doi: 10.1006/gcen.1997.7026
Sequeira, A. M. M., Heupel, M. R., Lea, M. A., Eguíluz, V. M., Duarte, C. M., Meekan, M. G., et al. (2019). The importance of sample size in marine megafauna tagging studies. Ecol. Appl. 29:e01947. doi: 10.1002/eap.1947
Shillinger, G. L., Palacios, D. M., Bailey, H., Bograd, S. J., Swithenbank, A. M., Gaspar, P., et al. (2008). Persistent leatherback turtle migrations present opportunities for conservation. PLoS Biol. 6:e171. doi: 10.1371/journal.pbio.0060171
Sigman, D. M., and Hain, M. P. (2012). The biological productivity of the ocean. Nat. Educ. Knowledge 3:21.
Sutherland, W. J. (1998). The importance of behavioural studies in conservation biology. Anim. Behav. 56, 801–809. doi: 10.1006/anbe.1998.0896
Therneau, T., Atkinson, B., and Ripley, B. (2013). Rpart: Recursive Partitioning. R Package Version 4.1-3. Available online at: http://CRAN.R-project.org/package=rpart (accessed June 25, 2022).
Todd, P. R. (1981). Timing and periodicity of migrating New Zealand freshwater eels (Anguilla spp.). N.Z. J. Mar. Freshw. Res. 15, 225–235. doi: 10.1111/j.1095-8649.2009.02241.x
United States (1983). The Endangered Species Act of 1973 as amended by Public Law 97-304 (the Endangered Species Act amendments of 1982)”. Washington, DC: US GPO.
US Fish and Wildlife Service (2017). Critical Habitat - What is it? Falls Church, VA: Endangered Species Program.
Vallega, A. (2002). Sustainable ocean governance: a geographical perspective. Milton Park: Routledge. doi: 10.4324/9780203209721
Valverde, R. A. (1996). Corticosteroid dynamics in a free-ranging population of olive ridley sea turtles (Lepidochelys olivacea Eschscholtz, 1829) at Playa Nancite, Costa Rica as a function of their reproductive behavior. Ph.D. thesis. Texas: Texas A&M University.
van den Thillart, G., Dufour, S., and Rankin, J. C. (2009). Spawning Migration of the European Eel: Reproduction Index, a Useful Tool for Conservation Management. Dordrecht: Springer. doi: 10.1007/978-1-4020-9095-0
Violle, C., Enquist, B. J., McGill, B. J., Jiang, L., Albert, C. H., Hulshof, C., et al. (2012). The return of the variance: intraspecific variability in community ecology. Trends Ecol. Evol. 27, 244–252. doi: 10.1016/j.tree.2011.11.014
Wallace, B. P., DiMatteo, A. D., Hurley, B. J., Finkbeiner, E. M., Bolten, A. B., Chaloupka, M. Y., et al. (2010). Regional management units for marine turtles: a novel framework for prioritizing conservation and research across multiple scales. PLoS One 5:e15465. doi: 10.1371/journal.pone.0015465
Williamson, D., Williamson, J., and Ngwamotsoko, K. T. (1988). Wildebeest migration in the Kalahari. Afr. J. Ecol. 26, 269–280. doi: 10.1111/j.1365-2028.1988.tb00979.x
Williamson, S. A., Evans, R. G., Robinson, N. J., and Reina, R. D. (2019). Synchronised nesting aggregations are associated with enhanced capacity for extended embryonic arrest in olive ridley sea turtles. Sci. Rep. 9:9783. doi: 10.1038/s41598-019-46162-3
Wood, S. (2017). Generalized Additive Models: An Introduction with R. Orange, CA: Chapman and Hall. doi: 10.1201/9781315370279
Keywords: olive ridley turtle, Lepidochelys olivacea, conservation, marine protected areas, migration, Eastern Tropical Pacific, satellite tagging, habitat preference model
Citation: Figgener C, Bernardo J and Plotkin PT (2022) Delineating and characterizing critical habitat for the Eastern Pacific olive ridley turtle (Lepidochelys olivacea): Individual differences in migratory routes present challenges for conservation measures. Front. Ecol. Evol. 10:933424. doi: 10.3389/fevo.2022.933424
Received: 30 April 2022; Accepted: 07 July 2022;
Published: 05 August 2022.
Edited by:
Blanca Figuerola, Institute of Marine Sciences (CSIC), SpainReviewed by:
Shiang-Lin Huang, Guangxi Academy of Sciences, ChinaCopyright © 2022 Figgener, Bernardo and Plotkin. This is an open-access article distributed under the terms of the Creative Commons Attribution License (CC BY). The use, distribution or reproduction in other forums is permitted, provided the original author(s) and the copyright owner(s) are credited and that the original publication in this journal is cited, in accordance with accepted academic practice. No use, distribution or reproduction is permitted which does not comply with these terms.
*Correspondence: Christine Figgener, Y2hyaXN0aW5lLmZpZ2dlbmVyQHlhaG9vLmRl
Disclaimer: All claims expressed in this article are solely those of the authors and do not necessarily represent those of their affiliated organizations, or those of the publisher, the editors and the reviewers. Any product that may be evaluated in this article or claim that may be made by its manufacturer is not guaranteed or endorsed by the publisher.
Research integrity at Frontiers
Learn more about the work of our research integrity team to safeguard the quality of each article we publish.