- 1College of Economics and Management, Nanjing Forestry University, Nanjing, China
- 2School of Economics and Management, Chuzhou University, Chuzhou, China
- 3School of Finance, Nanjing University of Finance and Economics, Nanjing, China
- 4Institute of Industrial Economics, Chinese Academy of Social Sciences, Beijing, China
- 5College of Economics and Management, China Jiliang University, Hangzhou, China
This manuscript applies the GML model with unexpected output to measure agricultural green total factor productivity (GTFP) in 30 provinces in China from 2011 to 2019. We explore the effect and mechanism of digital inclusive finance (DIF) on agricultural green total factor productivity. Our empirical results show that during the sample period, China’s agricultural green total factor productivity has shown an increasing trend. Digital inclusive finance mainly promotes agricultural GTFP by improving green technology level. The coverage rate, the application rate and the digitalization rate of digital inclusive finance all generate positive effects on agricultural green total factor productivity, among which the coverage rate contributes the most. Besides, the positive effect of digital inclusive finance in the eastern coastal areas is more significant than in other areas. The analysis of the mechanism shows that digital inclusive finance can indirectly help improve agricultural green total factor productivity through motivating agricultural technology innovation and industrial structure optimization. The research results of this manuscript are extremely meaningful for better implement DIF-related policies, and promote the green development of agriculture.
Introduction
Since the Economic Reform and opening-up, China’s agricultural economy has been rapidly growing, which is now called the world-known “China Miracle”. During 1978 to 2020, the average annual growth of China’s agricultural gross domestic product (GDP) is at 4.6%. During the same period 2003 to 2021, China’s total grain output has increased from 430 million tons to 680 million tons, maintaining a continuous growth for 18 years. However, China’s high speed agricultural economic growth at the cost of high investment and heavy pollution inevitably lead to excessive waste of resources and deterioration of the biological environment (Su et al., 2020). Taking carbon emissions as an example, China’s agricultural carbon emissions account for 17% of world’s carbon emissions, but this share for the U.S. and the world’s average level is only at 7 and 11%, respectively (Huang et al., 2019). In fact, China has become the largest source of agricultural carbon emissions in the world. Agricultural development faces dual pressures of low production efficiency and heavy environmental pollution (Fang et al., 2021).
In this context, the report of the 19th National Congress of the Communist Party of China proposed to promote the development of environmentally friendly agriculture and improve the total factor productivity. In 2022, China’s “No. 1 Document” further emphasizes the importance of development of environmental efficient agriculture. Previous studies have confirmed that implementing sustainable development strategies and improving agricultural green total factor productivity is an effective way to achieve agricultural green development (Wu et al., 2020). Agricultural green total factor productivity, i.e., agricultural environmental efficiency and agricultural ecological efficiency, measures agricultural total factor productivity under the consideration of resources and environment. The acceleration of agricultural green total factor productivity growth is inseparable from supports provided by financial services (Zeng et al., 2021).
Under the traditional financial service framework, the cost and risk of providing financial services to agriculture and rural areas is high, therefore discouraging financial institutions from entering the industry, and by consequence creating obstacles for farmers to receive external financings. Zhang et al. (2015) shows that due to the constraints of cost, risk and technology, traditional finance fails to provide strong support for the green development of China’s economy. Therefore, it is urgent to accelerate the pace of financial model reform and innovation to improve the availability of agricultural financial services (Cao et al., 2021). Incorporating new technologies such as artificial intelligence and big data (Awan et al., 2021), digital financial inclusion emerges in responding the demand. Digital inclusive finance overcomes high costs and information asymmetry in traditional rural financial transactions, it is also able to improve the financial availability in remote areas and for vulnerable groups, thus playing an important role in alleviating the shortage of funds in the agricultural sector and promoting high-quality agricultural development (Xing, 2021). The 49th “Statistical Report on Internet Development in China” shows that the number of rural netizens in China has reached 284 million, and the Internet penetration rate in rural areas has reached to 57.6% up to December 2021. Through network terminals such as mobile phones, groups excluded from the formal financial system have access to financial services (Gomber et al., 2018). Practice shows that digital financial inclusion promotes the optimal allocation of financial resources, which has a significant positive impact on rural development and agricultural production (Ahmed and Huo, 2021; Cao et al., 2021). Hence, it is of great significance to explore the relationship between the digital inclusive finance and agricultural green total factor productivity for realizing the sustainable development of China’s agriculture.
Previous research has mainly focused on the effect of agricultural trade (Zhao et al., 2018), industrial agglomeration (Wu et al., 2020), agricultural insurance (Carter et al., 2016), and farmer household characteristics (Adnan et al., 2018) and other factors on agricultural green total factor productivity, nevertheless, little attention has been paid to the relationship between digital inclusive finance and agricultural green total factor productivity. Romer’s endogenous growth theory points out that financial development would affect total factor productivity through resource allocation and technological progression (Romer, 1986). Subsequently, some studies have shown that digital inclusive finance can promote green economic development (Siek and Sutanto, 2019) and green total factor productivity growth (Li et al., 2021). However, previous studies have controversial conclusions at the level of agricultural research. Hu et al. (2021) argue that inclusive finance supports the transformation of agricultural production by providing loans to improve agricultural total factor productivity. Li (2021) and Sun et al. (2022) found that digital inclusive finance increases the supply of financial services, thereby promoting changes in agricultural production methods, quality and efficiency, and leads to promoting agricultural green total factor productivity. However, Matthews (2019) pointed out that the short-term effect of digital financial inclusion on agricultural and rural economies is limited. In the absence of mature digital inclusive finance development systems and regulatory mechanisms, it may bring new risks to the development of the agricultural sector. Digital inclusive finance is profit-seeking like other types of capital. The narrow profit-seeking space in rural areas, technical threshold of digital inclusive finance, the lack of financial infrastructure in rural areas and financial knowledge of rural people make digital inclusive finance significantly less active in rural areas (Xing, 2021).
The existing literature mainly focuses on the impact of digital financial inclusion on the agricultural economy, while few studies focus on the impact of agro-ecology, and fewer studies comprehensively consider the economic and ecological effects. Most of the existing literature examines and verifies the relationship between the two, and lacks sufficient analysis of the transmission path, so it is difficult to identify the internal mechanism of digital inclusive finance affecting agricultural green total factor productivity. In general, the impact of digital inclusive finance on agricultural green total factor productivity is still a relatively new topic. Can digital inclusive finance promote the improvement of agricultural green total factor productivity? If so, what is its mechanism of action? These questions require in-depth research by the academic community to give convincing answers. The main objective of this study is to measure agricultural green total factor productivity using the GML model that introduces undesired output based on the panel data of 30 provinces in China from 2011 to 2019. Then empirically investigate the effect of digital inclusive finance on agricultural green total factor productivity, as well as its internal mechanism.
The contributions of this study to the existing literature are as follows: First, this study incorporates both economic and ecological effects into the research framework to explore comprehensively the effect of digital inclusive finance. The existing literature focuses on the effect of digital inclusive finance on the agricultural economy, while little attention is paid to the effect on agroecology, and the simultaneous impact on economic and ecological effects. Second, this study uses the mediation effect model to explore the impact mechanism of digital inclusive finance on agricultural green total factor productivity. Previous studies are difficult to give a clear explanation on the internal affecting mechanism of digital inclusive finance on the agricultural green total factor productivity, for focusing on verifying the relationship between the two, rather than analyzing the transmission path. Third, this study examines the relationship between digital inclusive finance and agricultural green TFP from three perspectives: sub-index, sub-dimension, and sub-region, in order to investigate the heterogeneity of the impact of digital inclusive finance.
The rest of this article is organized as follows. Section “Theoretical Analysis and Research Hypothesis” introduces the theoretical framework. Section “Theoretical Analysis and Research Hypothesis” describes the data and models. Section “Materials and Methods” reports empirical results including heterogeneity analysis, endogeneity problem and mechanism analysis. Section “Conclusion and Policy Implications” concludes.
Theoretical Analysis and Research Hypothesis
The Direct Impact of Digital Inclusive Finance on Agricultural Green Total Factor Productivity
The small and scattered demand for agricultural capital, the difficulty in collecting credit information make it difficult for the agricultural sector to obtain support from traditional finance institutions. Digital inclusive finance relying on digital technology can greatly improve the efficiency of capital matching and the availability of financial services in the agricultural sector under the condition of low-cost, thereby promoting the improvement of agricultural green total factor productivity (Sun et al., 2022). Specifically, the impact of digital inclusive finance on agricultural green total factor productivity is mainly reflected in the following three aspects: First, digital inclusive finance lowers the entry difficulty to obtain financial services. Digital inclusive finance provides low-cost and high-efficiency access to funds for rural areas with relatively weak economic foundations, and helps alleviate insufficient financial supply and financial exclusion in rural areas. Adequate capital supply can improve the application of agricultural machineries and equipment, advanced technology, etc., which lays the foundation for large-scale and intensive development. Second, digital inclusive finance can facilitate the application of green technology. By leveraging its technological advantages, digital inclusive finance can accurately invest funds in green farming fields such as green farming, agricultural machinery and equipment, pollution prevention, which will improve the scale and efficiency of green agricultural output. Third, digital inclusive finance helps diversify risks. By building a risk control system based on big data, digital inclusive finance can effectively improve the financial literacy and risk awareness of farmers while reducing the systemic financial risks of financial institutions. At the same time, digital inclusive finance improves the resilience of the agricultural sector by promoting fintech and insurtech interconnectivity (such as “bank + insurance” loan), which in turn increases the acceptances of new technologies and green equipment (Visser et al., 2020), and can consequently improve agricultural green total factor productivity (Aung et al., 2019). Based on the above analysis, this study proposes the following hypotheses:
H1: Digital inclusive finance can promote the improvement of agricultural green total factor productivity.
The Indirect Impact of Digital Inclusive Finance on Agricultural Green Total Factor Productivity
The development of digital inclusive finance can improve the level of agricultural technological innovation and promote the optimization of industrial structure, which would improve agricultural green total factor productivity.
The GTFP improvement is inseparable from technological progress especially green technology innovation (Sun et al., 2020; Wang H. et al., 2021). The inclusive nature of digital inclusive finance has eased the financial constraints of agriculture-related entities, making it possible to invest more R&D funds in the agricultural sector, thereby improving the level of regional agricultural technology innovation. Digital inclusive finance establishes a reliable credit reporting system through digital technology (Du et al., 2021), which effectively reduces the credit risk faced by the financial system when it provides inclusive financial services to farmers. This fundamentally elevates the financing constraints of agricultural-related entities’ technology innovation (Gomber et al., 2018). Furthermore, the vigorous promotion of digital inclusive finance in China promotes the continuous improvement of rural digital infrastructure, which facilitates the promotion and application of advanced technologies and ultimately promotes the improvement of agricultural green total factor productivity (Zhang and Gao, 2018). Based on the above analysis, this study proposes the following hypotheses:
H2a: Agricultural technological innovation plays an intermediary role in the process of digital inclusive finance promoting the improvement of agricultural green total factor productivity.
As for the effect of industrial structure optimization. Digital inclusive finance reduces information asymmetry and transaction costs, thereby improving the efficiency of resource allocation and boosting the optimization and upgrading of the agricultural structure (Chava et al., 2013). The digital inclusive finance expands the coverage of financial services, enhances the application of financial services, promotes the green upgrade of the industrial structure, realizes the Pareto improvement of financial resources allocation, and finally achieves a win-win situation for output and for the environment (Liu and He, 2021; Zhou et al., 2021). The continuous optimization and upgrading of the agricultural structure have led to the expansion of the rural industries scale. The accompanying cluster effect and specialization effect not only reduce agricultural production costs and increase the additional value of products, but also bring huge structural and scale dividends, which will help improve agricultural green total factor productivity. Based on the above analysis, this study proposes the following hypotheses:
H2b: Industrial structural optimization plays an intermediary role in the process of digital inclusive finance promoting the improvement of agricultural green total factor productivity.
Materials and Methods
Sample and Data Source
The data used in this manuscript consists of provincial statistics and the digital inclusive finance data. The statistical data of 30 provinces in China (excluding Hong Kong, Macau, Taiwan, and Tibet) are collected from the “China Statistical Yearbook”, “China Rural Statistical Yearbook”, “China Science and Technology Statistical Yearbook”, “China’s Population and Employment Statistical Yearbook”, “Monthly Statistical Report on Import and Export of China’s Agricultural Products” and Statistical Yearbooks of various provinces and cities. Data of digital inclusive finance comes from the China Digital inclusive finance Index released by the Digital Finance Research Center of Peking University (Guo et al., 2020). Therefore, our dataset is a balanced panel dataset of 30 provinces in China from 2011 to 2019.
Definition of Variables
Dependent Variables
The dependent variable in this manuscript is agricultural green total factor productivity (GTFP). Previous studies mainly apply two methods to measure green total factor productivity, first, the Malmquist-Luenberger (ML index) based on the directional distance function proposed by Chung and Fare (1997), second the Global Malmquist-Luenberger index (GML index) constructed by Oh (2010). However, compared with the ML index, the GML index can solve the defects of non-transitivity and no feasible solution perfectly. Therefore, this manuscript adopts the GML index to measure the agricultural green total productivity of various provinces of China. The specific model settings are as follows:
The GML values can be greater than 1, equal to 1 or less than 1, respectively, it represents the increase, the constant and the decrease of the level of agricultural green total factor productivity from the t to t + 1 period, respectively. As shown in equation (2), the GML index can be further decomposed into the product of Technical progress(GTC)and Technical efficiency(GEC), according to which we can examine the impact of GEC and GTC on productivity improvement.
According to Liu and Xin (2019), we took 2010 as the base period to convert the agricultural green total factor productivity index of each province into a cumulative productivity index. The variables in the measurement model of agricultural green total factor productivity include input, expected output and unexpected output. The specific indicators list as follows:
First, input indicators including labor, land and capital. Among them, we use the total number of agricultural employees to represent the labor factor. We choose the total sown area of crops to represent the land factor, for it can better reflect the situation of multiple cropping and intercropping, replanting and replanting, transplanting crops, comparing t with the arable land area index. The capital factor is represented by the amount of fertilizer use and the total mechanical power.
Second, the expected output indicators. The study selects the total agricultural output to represent the expected output. In order to exclude the impact of price factors, we converted the agricultural output value data into comparable data using 2010 as the base period.
Third, the unexpected output indicators. Non-point source pollution and carbon emissions are often used as proxies for undesired output in previous studies, and their measurement is still controversial (Sun et al., 2012). This manuscript selects carbon emissions as the undesired output because carbon emissions increase the global greenhouse effect, and the current agricultural carbon emission reduction play the essential part of environmentally friendly agriculture development. Carbon emissions are “real pollutants,” which do not contain any nutrients such as nitrogen and phosphorus. Liu et al. (2021) and IPCC (2007) argue that most of the polluting behaviors of agricultural production generate greenhouse gases, so it is reasonable to treat carbon emissions as an undesired output. Li et al. (2011) and IPCC (2007) measured the total amount of agricultural carbon emissions by chemical fertilizers, pesticides, agricultural film, diesel oil, tillage and agricultural irrigation in the agricultural production process. And the coefficient of each carbon emission source is accurately estimated: fertilizer 0.8956 kg⋅kg-1; Chemical pesticide 4.9341 kg⋅kg-1; agricultural film 5.18 kg⋅kg-1; diesel oil used in agriculture 0.5927 kg⋅kg-1; tillage 312.6 kg km-2; irrigation 20.476 kg Cha-1.
Explanatory Variables
The core explanatory variable of this research is the development level of digital inclusive finance. This study selects the Peking University Digital Financial Inclusion Index as a proxy variable for digital inclusive finance. The index measures the development of digital inclusive finance from three dimensions: breadth of coverage (BREA), depth of use (DEP) and degree of digital support services (DIG) according to the principles of comprehensiveness, balance and comparability. Among them, the breadth of coverage (BREA) is mainly reflected by the number of electronic accounts, the depth of usage (DEP) is measured by the actual use of Internet financial services, and the level of digitalization (DIG) is closely related to convenience and cost. For the convenience of analysis, the digital inclusive finance index scores in this manuscript are divided by 100 with their raw data.
Mediating Variables
According to the above analysis, digital inclusive finance can indirectly affect agricultural green total factor productivity through agricultural technology innovation and industrial structure upgrading. Specifically, agricultural technology innovation (TEC) can be measured from the perspective of both input and output. However, the use of input indicators may overestimate the level of agricultural technology innovation due to the difficulty of fully converting inputs into outputs. Therefore, this manuscript selects the total number of patent applications in the agricultural sector to measure the level of agricultural technology innovation, whose data can be obtained from the CNKI patent database. According to Wang M.-X. et al. (2021), we constructed an industrial structure optimization index to measure the industrial structure level of 30 provinces, and its calculation formula is . Where, Ii represents the proportion of the output of the i-th industry of a province to the total output of the corresponding province. The value range of isu is 1 ≤ isu ≤ 3. isu close to 1 indicates the current economic society is an agricultural society dominated by farming. On the contrary, isu close to 3 indicates that the economic society is entering a post-industrial knowledge economy society.
Control Variables
According to previous literatures (Li et al., 2020; Cao et al., 2021; Fang et al., 2021; Li, 2021), the control variables include the following: (1) Extent of disaster (EXT) measured by the proportion of the affected area of the sown area of crops. (2) Income structure (INS), measured by the ratio of urban and rural per capita disposable income. (3) Degree of economic openness (OPE), expressed by the ratio of the import and export volume of agricultural products to the total agricultural production value. (4) Average education level (EDU), expressed by the average years of education of rural residents. (5) Urbanization (URB), measured by the level of urbanization, which solves the dilemma of rural surplus labor and helps improve the efficiency of agricultural resource allocation (Yu et al., 2014), thereby promoting the growth of agricultural green total factor productivity. (6) Effective irrigation degree (WAT) and Industrialization (IND). The variable definitions and descriptive statistics of this study are shown in Table 1.
Model
The non-negative cumulative value of agricultural green total factor productivity and its decomposition index should be attributed to the restricted dependent variable. Therefore, the OLS estimation method will lead to large deviations in the results, thus this manuscript adopts the Tobit model setting as follows:
Where, Yitis the accumulated GTFP, GTC and GEC for the i-th province in year t, DIFTit is the development level of digital inclusive finance, Xitare control variables, and εit is random disturbance term with distribution μi∼(0,σ2).
Empirical Analysis
Agricultural Green Total Factor Productivity and Decomposition
Table 2 show China’s agricultural green total factor productivity and its decomposition index calculated by GML index. Overall, the average annual growth rate of China’s Agricultural green total factor productivity (GTFP), Technical progression (GTC) and Technical efficiency (GEC) are 2.0, 1.8, and 0.2% from 2011 to 2019, respectively. GTFP and GTC are both greater than 1, and generally exhibit an upward trend. Although the efficiency of green technology is generally greater than 1, it has been less than 1 in 5 years, and the overall trend is declining. The above analysis implies that the green technology progress is the main driving force of agricultural green TFP, while green technology efficiency is counterproductive.
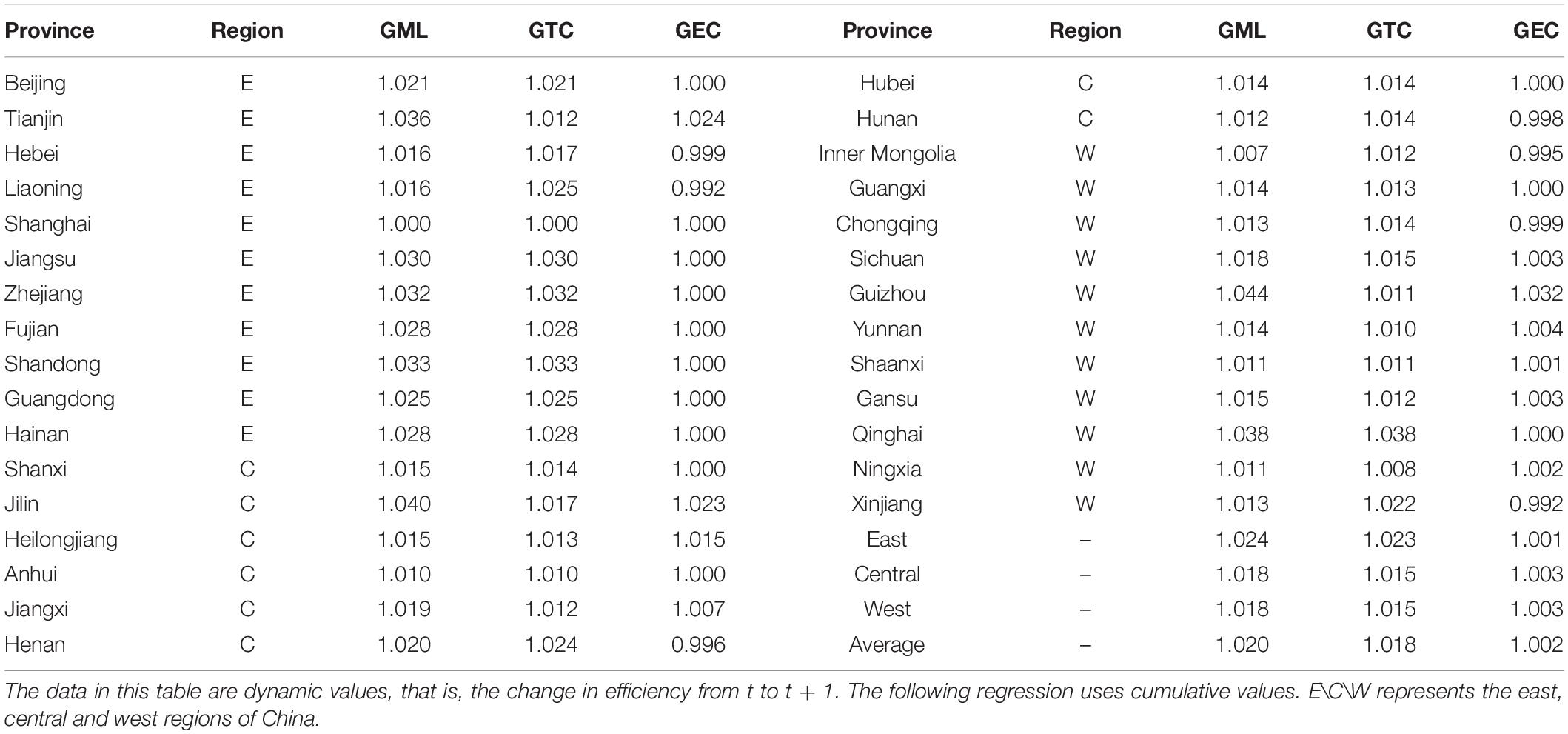
Table 2. Provincial agricultural Green total factor productivity and its decomposition of China from 2011 to 2019.
From a geographical perspective, the average annual growth rates of agricultural green total factor productivity in the eastern, central and western regions are 2.4, 1.8, and 1.8%, respectively. The reason for the high growth of agricultural green total factor productivity in the eastern region is that the eastern regions have abundant resources, good economic foundation, preferential policies and relatively advanced technologies, which have a stronger impetus to promote agricultural transformation and upgrading, thereby promoting agricultural Green total factor productivity grew at a relatively rapid rate. As for the central and western regions, although agricultural development is relatively slower, with the increasingly application of technology introduction and policy support, agricultural green total factor productivity has also maintained growing.
The Impact of Digital Inclusive Finance on Agricultural Green Total Factor Productivity
Benchmark Regression
Table 3 reports the estimated results of the impact of DIF on Agricultural GTFP. We run the LR test and the Wald test to verify the model’s fitness and the results show that our model fits well. Columns (1) to (3) of Table 3 report the estimated results of the benchmark models without control variables, while columns (4) to (6) show the estimated results of the models with control variables. The results of the benchmark models manifest that DIF has a positive impact on agricultural GTFP. The results in columns (4) to (6) manifest that the coefficient of DIF is significantly positive, indicating that DIF still has a positive impact on agricultural GTFP after controlling for other variables. Thus, the hypothesis H1 is initially verified.
In addition, according to Table 3, we find that DIF had a significant positive effect on GTC, which is enhanced to a certain extent after introduce control variables. While we did not find evidence for the impact of DIF on the GEC. Therefore, we can conclude that the improvement of agricultural GTFP by DIF is mainly through GTC. The specific reason is that digital inclusive finance has lowered the entry difficulty to obtain financings and provided sufficient financial support for the advancement of agricultural technology. The possible reason for the weak effect of digital inclusive finance on technical efficiency is the insufficient coverage and depth of digital inclusive finance in rural areas.
Heterogeneity Analysis
The Impact of Different Dimensions of Digital Inclusive Finance
In order to further clarify the internal mechanism of DIF affecting agricultural GTFP, we constructed regression models of the three dimensions of DIF and agricultural GTFP, the estimated results are shown in Table 4. Where columns (1), (3), and (5) are the estimated results of the benchmark models without control variables for breadth of coverage (BREA), depth of usage (DEP) and digital support services (DIG), respectively, while columns (2), (4) and Column (6) are the estimated results of the corresponding models with control variables. From the columns (1), (3), and (5) of Table 4, the Breadth of coverage, Depth of usage and Level of digitalization all have a significant positive impact on agricultural green total productivity at the 1% significant level. The results in columns (2), (4), and (6) of Table 4 show that Breadth of coverage, Depth of usage and Level of digitalization all have a significant positive impact on agricultural green total productivity at the 1% significant level. Therefore, regardless of the introduction of control variables, the coefficients of Breadth of coverage, Depth of usage and Level of digitalization are significantly positive at the 1% significant level, indicating that the results in Table 4 are highly stable. It shows that Breadth of coverage, Depth of usage and Level of digitalization all have a significant improvement effect on agricultural green total productivity.
The above results show that: First, the wider the coverage of digital financial inclusion, the more plausible it covers the “long tail” customer group, i.e., digital financial inclusion can solve financing problems for rural areas with relatively lagging economies on a larger scale, thereby promoting the agricultural green total factor productivity. Second, continuous enhancement of the depth of the usage of digital inclusive finance has improved the financing of agricultural operators by providing more diverse financial products and services. Third, with the help of digital technologies such as big data and cloud computing, digital inclusive finance can effectively solve the problems of credit fragmentation and information asymmetry, laying a financial foundation for expanding financial coverage and utilization, and improving agricultural green total factor productivity.
Distinguishing Regional Development Levels
China’s provinces have different characteristics in resource endowment, agricultural technology level, business scale and labor quality, in turn, it generates heterogeneity between regions in terms of effect of digital inclusive finance on agricultural green total factor productivity. Therefore, this manuscript first sets two regional division standards, the first one divides all provinces into eastern, central and western regions, the second divides all provinces into coastal areas and inland areas. We then investigate the heterogeneity of the effect of digital inclusive finance on agricultural GTFP according to the two types of regional division methods. Columns (1) ∼ (3) and (4) ∼ (5) of Table 5 report the effects of DIF on agricultural GTFP under the first and second regional divisions, respectively.
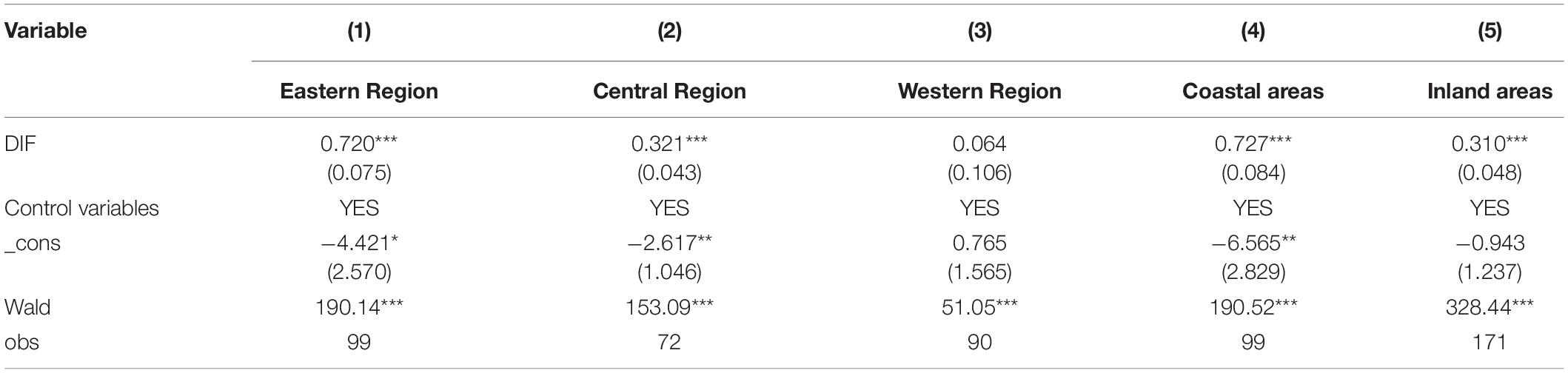
Table 5. Regional differences in the impact of digital inclusive finance on agricultural green total factor productivity.
The results in Table 5 show that, except for the western region, digital inclusive finance in the eastern, central, coastal and interior regions all have a significant positive impact on agricultural green total factor productivity at the 1% significance level. Nevertheless, the effect of digital inclusive finance on agricultural total factor productivity in the eastern region is much larger than that in the central and western regions, and the effect of digital inclusive finance in the coastal region on improving GTFP is also much greater than that in the inland region. It can be explained by the fact that, on the one hand, high level of marketization in the eastern coastal areas help improve the efficiency of digital inclusive finance in the agricultural sector. Capital investment in the agricultural sector guided by digital inclusive finance can realize benefits through a profound market mechanism. On the other hand, the digital technology level in the eastern coastal areas is relatively more advanced, thereby an easier access to financing provided by a more developed digital inclusive finance is more likely to promote the progression of agricultural technology.
Endogeneity Discussion
Although previous discussion has been made from multiple perspectives such as sub-index, sub-dimension, and sub-region to ensure the robustness of the results, there may still be endogenous biases caused by reverse causality in the model. That is, when the level of GTFP increases, the agricultural sector takes the initiative to raise funds to achieve intensive operation and efficient use of resources, which will instead promote the development of digital inclusive finance. According to Cao et al. (2021), this study selects Internet penetration rate as an instrumental variable for DIF to conduct an endogeneity test. First the Internet penetration rate, as an infrastructure variable reflecting digital finance, is closely related to the development of digital inclusive finance and meets the correlation requirements of instrumental variables. Second, agricultural green total factor productivity mainly reflects the status of agricultural production and operation, so there is no direct correlation between the Internet penetration rate and agricultural green total factor productivity. Therefore, the instrumental variables selected in this manuscript satisfy the correlation and exogenous assumptions. The test results are shown in Table 6.
According to the results of IV-Tobit estimation, the Wald test rejects the null hypothesis of “α = 0”, suggesting that there are endogenous variables in the model. The estimation results of the first stage show that the coefficient of Internet penetration rate (INT) is significantly positive at the 1% significance level, and the F-value is 55.96, indicating no weak instrumental variable problem, i.e., the instrumental variable selection in this manuscript is effective. The second-stage Wald test results again reject the null hypothesis. The above analysis shows that the estimated value of the two-step method is close to the IV-Tobit, indicating the IV-Tobit regression is effective. After solving the endogenous problem, DIF still has a significant positive impact on agricultural GTFP, which indicates that the analysis results of the above regression are robust. Hypothesis H1 is verified again.
Analysis of the Mechanism
The empirical results have confirmed the development of digital inclusive finance can significantly promote agricultural green total factor productivity, however, its function mechanism is still unclear. In order to clarify the effect path of digital inclusive finance on agricultural green total factor productivity, we adopt the mediation effect model based on Baron and Kenny (1986) and Gomber et al. (2018) to analyze the mechanism effect of digital inclusive finance on agricultural green total factor productivity from the mediating role of agricultural technology innovation and upgrading of industrial structure. The results of the mediation effect test are shown in Table 7.
The Mediating Effect of Agricultural Technology Innovation
Table 7 (1) ∼ (2) shows the stepwise regression test results with agricultural technological innovation as the mediating variable. Column (1) shows that the coefficient of DIF is 0.384, which is significant at the 1% level, indicating that DIF has a promoting effect on technology innovation (TEC). The results in column (2) show that after incorporating TEC into the model, the coefficients of TEC and DIF are significantly positive, and the coefficient of DIF is smaller than that of the benchmark model, indicating that TEC only produces partial mediation effect. This also indicates that there is a mediating effect of DIF promotes agricultural GTFP through TEC, thus confirming the hypothesis H2a. Further calculations show that the mediating effect of TEC accounts for 9.34% of the total effect.
The Intermediary Effect of Optimization of Industrial Structure
The coefficient of DIF in column (3) of Table 7 is significantly positive at the level of 1%, indicating that DIF has significantly improved the industrial structure (ISU). Column (4) in Table 7 shows that after incorporating ISU into the model, the coefficient of ISU is 0.486 at the 5% significance level. In other words, DIF can have a significant positive impact on agricultural GTFP by promoting the ISU, indicating the existence of the intermediary effect of ISU. Further calculation shows that the mediating effect of industrial structure upgrading accounts for 24.88% of the total effect. Thus, the hypothesis H2b is verified.
Conclusion and Policy Implications
Based on China’s provincial panel data from 2011 to 2019, this manuscript applies the GML index to measure agricultural green total factor productivity considering undesired output. Furthermore, this study systematically investigates the impact and mechanism of digital inclusive finance on agricultural green total factor productivity. The main fundings are as follows: First, agricultural green total factor productivity mainly drove by green technology progress has shown a positive growth trend. Second, digital inclusive finance mainly promotes the improvement of agricultural green total factor productivity by promoting the progress of green technology. Third, the Breadth of coverage, Depth of usage, and Level of digitalization of digital financial inclusion have a positive effect on agricultural green total factor productivity, among which the Breadth of coverage has the strongest impellent effect. In addition, compared with other regions, the eastern coastal region has the greatest improvement effect by digital financial inclusion. Fourth, digital inclusive finance can indirectly promote the improvement of agricultural green total factor productivity by motivating Agricultural technology innovation and optimizing the industrial structure. The mediating effects of TEC and ISU on agricultural green total factor productivity are 9.34 and 24.88%, respectively.
The implications of this manuscript are as follows: First, the government should encourage and support private capital to participate in the rural financial field while increasing investments in digital inclusive finance in rural areas, strengthening the construction of digital inclusive financial infrastructure in rural areas, and effectively increasing the scale of financial development in rural areas. Second, local governments should accurately and orderly improve the development of digital inclusive finance according to their own resource endowments, agricultural economic development level and target positioning, while avoiding the “digital divide.” Third, the government should establish a profound green development incentive mechanism to help integrate the development of digital inclusive finance and the agricultural industry, so that digital inclusive finance can comprehensively motivate technological innovation and structure optimization in agriculture.
We hope to expand this research in the future from the following aspects: First, with the development of database resources, more adequate empirical analysis can be carried out on the basis of larger-scale data in the future. Secondly, when examining the effect of digital inclusive finance on agricultural green total factor productivity, this manuscript’s selection remains relatively narrow, which may contain latent variables that cause bias and insufficiency in the analysis of the mechanism. In the future, more dimensions should be taking into account. Finally, this manuscript mainly conducts empirical analysis from a macro perspective, and empirical analysis can be carried out from a micro perspective in the future.
Data Availability Statement
The original contributions presented in the study are included in the article/supplementary material, further inquiries can be directed to the corresponding author.
Author Contributions
QG: responsible for the conception and structural design of the article. CC and GS: responsible for writing and revising the first draft of the manuscript. JL: responsible for the later revision of the manuscript. All authors contributed to the article and approved the submitted version.
Funding
This work was supported by the National Natural Science Foundation of China (Grant No. 72003086).
Conflict of Interest
The authors declare that the research was conducted in the absence of any commercial or financial relationships that could be construed as a potential conflict of interest.
Publisher’s Note
All claims expressed in this article are solely those of the authors and do not necessarily represent those of their affiliated organizations, or those of the publisher, the editors and the reviewers. Any product that may be evaluated in this article, or claim that may be made by its manufacturer, is not guaranteed or endorsed by the publisher.
References
Adnan, N., Nordin, S. M., and Ali, M. (2018). A solution for the sunset industry: adoption of Green Fertiliser Technology amongst Malaysian paddy farmers. Land Use Policy 79, 575–584. doi: 10.1016/j.landusepol.2018.08.033
Ahmed, A. D., and Huo, R. (2021). Volatility transmissions across international oil market, commodity futures and stock markets: empirical evidence from China. Energ. Econ. 93:104741. doi: 10.1016/j.eneco.2020.104741
Aung, N., Nguyen, H., and Sparrow, R. (2019). The Impact of Credit Policy on Rice Production in Myanmar. J. Agr. Econ. 70, 426–451. doi: 10.1111/1477-9552.12299
Awan, U., Shamim, S., Khan, Z., Zia, N. U., Shariq, S. M., and Khan, M. N. (2021). Big data analytics capability and decision-making: the role of data-driven insight on circular economy performance. Technol. Forecast. Soc. 168:120766. doi: 10.1016/j.techfore.2021.120766
Baron, R. M., and Kenny, D. A. (1986). The moderator-mediator variable distinction in social psychological research: conceptual, strategic, and statistical considerations. J. Pers. Soc. Psychol. 51, 1173–1182. doi: 10.1037/0022-3514.51.6.1173
Cao, J. H., Law, S. H., Samad, A. R. B., Mohamad, W., Wang, J. L., and Yang, X. D. (2021). Impact of financial development and technological innovation on the volatility of green growth-evidence from China. Environ. Sci. Pollut. R. 28, 48053–48069. doi: 10.1007/s11356-021-13828-3
Carter, M. R., Cheng, L., and Sarris, A. (2016). Where and how index insurance can boost the adoption of improved agricultural technologies. J. Dev. Econ. 118, 59–71. doi: 10.1016/j.jdeveco.2015.08.008
Chava, S., Oettl, A., Subramanian, A., and Subramanian, K. V. (2013). Banking deregulation and innovation. J. Financ. Econ. 109, 759–774. doi: 10.1016/j.jfineco.2013.03.015
Chung, Y., and Fare, R. (1997). Productivity and Undesirable Outputs: A Directional Distance Function Approach. Microeconomics 51, 229–240. doi: 10.1006/jema.1997.0146
Du, G.-S., Liu, Z.-X., and Lu, H.-F. (2021). Application of innovative risk early warning mode under big data technology in Internet credit financial risk assessment. J. Comput. Appl. Math. 386:113260. doi: 10.1016/j.cam.2020.113260
Fang, L., Hu, R., Mao, H., and Chen, S. J. (2021). How crop insurance influences agricultural green total factor productivity: evidence from Chinese farmers. J. Clean. Prod. 321:128977. doi: 10.1016/j.jclepro.2021.128977
Gomber, P., Kauffman, R. J., Parker, C., and Weber, B. W. (2018). On the Fintech Revolution: interpreting the Forces of Innovation, Disruption, and Transformation in Financial Services. J. Manag. Inform. Syst. 35, 220–265. doi: 10.1080/07421222.2018.1440766
Guo, F., Wang, J.-Y., Wang, F., Kong, T., Zhang, X., and Cheng, Z.-Y. (2020). Measuring China’s digital financial inclusion; index compilation and spatial characteristics. CHIN. Econ. Q. 19, 1401–1418. doi: 10.13821/j.cnki.ceq.2020.03.12
Hu, Y., Liu, C., and Peng, J.-G. (2021). Financial inclusion and agricultural total factor productivity growth in China. Econ. Model. 96, 68–82. doi: 10.1016/j.econmod.2020.12.021
Huang, X.-Q., Xu, X.-C., Wang, Q.-Q., Zhang, L., Gao, X., and Chen, L.-H. (2019). Assessment of Agricultural Carbon Emissions and Their Spatiotemporal Changes in China, 1997–2016. Int. J. Env. Res. Pub. He. 16:3105. doi: 10.3390/ijerph16173105
IPCC (2007). Climate Change 2007: mitigation. Contribution of Working Group III to the Fourth Assessment Report of the Intergovernmental Panel on Climate Change. Comp. Geom. 18, 95–123.
Li, B., Zhang, J.-B., and Li, H.-P. (2011). Research on spatial-temporal characteristics and affecting factors decomposition of agricultural carbon emission in China. CHIN. Popul. Resour. Environ. 21, 80–86. doi: 10.3969/j.issn.1002-2104.2011.08.013
Li, G.-Q., Fang, X.-B., and Liu, M.-T. (2021). Will Digital Inclusive Finance Make Economic Development Greener? Evidence From China. Front. Env. Sci. 9:762231. doi: 10.3389/fenvs.2021.762231
Li, J.-X. (2021). Rural financial development and the improvement of agricultural green total factor productivity. Manag. Rev. 33, 84–95. doi: 10.14120/j.cnki.cn11-5057/f.20201223.008
Li, M.-Y., Wang, J.-J., Zhao, P.-J., Chen, K., and Wu, L.-B. (2020). Factors affecting the willingness of agricultural green production from the perspective of farmers’ perceptions. Sci. Total. Environ. 738:140289. doi: 10.1016/j.scitotenv.2020.140289
Liu, D.-D., Zhu, X.-Y., and Wang, Y.-F. (2021). China’s agricultural green total factor productivity based on carbon emission: an analysis of evolution trend and influencing factors. J. Clean. Prod. 278:123692. doi: 10.1016/j.jclepro.2020.123692
Liu, L., and He, L.-Y. (2021). Output and welfare effect of green credit in China: evidence from an estimated DSGE model. J. Clean. Prod. 294:126326. doi: 10.1016/j.jclepro.2021.126326
Liu, Z.-K., and Xin, L. (2019). Has China’s Belt and Road Initiative promoted its green total factor productivity ? Evidence from primary provinces along the route. Energy Policy 129, 360–369. doi: 10.1016/j.enpol.2019.02.045
Matthews, B. H. (2019). Hidden constraints to digital financial inclusion: the oral-literate divide. Dev. Pract. 29, 1014–1028. doi: 10.1080/09614524.2019.1654979
Oh, D. H. (2010). A global Malmquist-Luenberger productivity index. J. Prod. Anal. 34, 183–197. doi: 10.1007/s11123-010-0178-y
Romer, P. M. (1986). Increasing Returns and Long-Run Growth. J. Polit. Econ. 94, 1002–1037. doi: 10.1086/261420
Siek, M., and Sutanto, A. (2019). “Impact Analysis of Fintech on Banking Industry,” in Paper Presented at the 2019 International Conference on Information Management and Technology (ICIMTech). (Piscataway: IEEE).
Su, Y., He, S., Wang, K., Shahtahmassebi, A. R., Zhang, L.-P., Zhang, J., et al. (2020). Quantifying the sustainability of three types of agricultural production in China: an emergy analysis with the integration of environmental pollution. J. Clean. Prod. 252:119650. doi: 10.1016/j.jclepro.2019.119650
Sun, B., Zhang, L.-X., Yang, L.-Z., Zhang, F.-S., Norse, D., and Zhu, Z.-L. (2012). Agricultural Non-Point Source Pollution in China: causes and Mitigation Measures. Ambio 41, 370–379. doi: 10.1007/s13280-012-0249-6
Sun, H., Kporsu, A. K., Taghizadeh-Hesary, F., and Edziah, B. K. (2020). Estimating environmental efficiency and convergence: 1980 to 2016. Energy 208:118224. doi: 10.1016/j.energy.2020.118224
Sun, X.-T., Yu, T., and Yu, F.-W. (2022). The impact of digital finance on agricultural mechanization: evidence from 1869 counties in China. CHIN. Rural Econ. 2, 76–93.
Visser, M., Jumare, H., and Brick, K. (2020). Risk preferences and poverty traps in the uptake of credit and insurance amongst small-scale farmers in South Africa. J. Econ. Behav. Organ. 180, 826–836. doi: 10.1016/j.jebo.2019.05.007
Wang, H., Cui, H., and Zhao, Q.-Z. (2021). Effect of green technology innovationon green total factor productivity in China: evidence from spatial durbin model analysis. J. Clean. Prod. 288:125624. doi: 10.1016/j.jclepro.2020.125624
Wang, M.-X., Li, Y.-L., and Liao, G.-K. (2021). Research on the Impact of Green Technology Innovation on Energy Total Factor Productivity, Based on Provincial Data of China. Front. Env. Sci. 9:710931. doi: 10.3389/fenvs.2021.710931
Wu, J.-Z., Ge, Z.-M., Han, S.-Q., Xing, L.-W., Zhu, M.-S., Zhang, J., et al. (2020). Impacts of agricultural industrial agglomeration on China’s agricultural energy efficiency: A spatial econometrics analysis. J. Clean. Prod. 260:121011. doi: 10.1016/j.jclepro.2020.121011
Xing, Y. (2021). “Dividend” and “Gap” of Rural Digital Inclusive Finance. Economist 2, 102–111. doi: 10.16158/j.cnki.51-1312/f.2021.02.011
Yu, A., Wu, Y.-Z., Zheng, B.-B., Zhang, X.-L., and Shen, L.-Y. (2014). Identifying risk factors of urban-rural conflict in urbanization: a case of china. Habitat. Int. 44, 177–185. doi: 10.1016/j.habitatint.2014.06.007
Zeng, J. J., Han, J.-Y., Qu, J.-S., Maraseni, T. N., Xu, L., Li, H.-J., et al. (2021). Ecoefficiency of China’s agricultural sector: what are the spatiotemporal characteristics and how are they determined? J. Clean. Prod. 325:129346. doi: 10.1016/j.jclepro.2021.129346
Zhang, R.-J., and Gao, M. (2018). New technology adoption behaviors and differences in technology efficiency: A comparative analysis of small and large grain producers. CHIN. Rural Econ. 5, 85–97.
Zhang, W., Jin, Y. G., and Wang, J. P. (2015). Greenization of venture capital and green innovation of chinese entity industry. Ecol. Indic. 51, 31–41. doi: 10.1016/j.ecolind.2014.10.025
Zhao, X.-M., Liu, C.-J., and Yang, M. (2018). The effects of environmental regulation on China’s total factor productivity: an empirical study of carbon-intensive industries. J. Clean. Prod. 179, 325–334. doi: 10.1016/j.jclepro.2018.01.100
Keywords: agricultural green total factor productivity, digital inclusive finance, Tobit model, mechanism, China
Citation: Gao Q, Cheng C, Sun G and Li J (2022) The Impact of Digital Inclusive Finance on Agricultural Green Total Factor Productivity: Evidence From China. Front. Ecol. Evol. 10:905644. doi: 10.3389/fevo.2022.905644
Received: 27 March 2022; Accepted: 07 April 2022;
Published: 28 April 2022.
Edited by:
Dingde Xu, Sichuan Agricultural University, ChinaReviewed by:
Jing Lan, Henan University of Economics and Law, ChinaHairong Zheng, Fujian Agriculture and Forestry University, China
Peng Jiquan, Jiangxi University of Finance and Economics, China
Copyright © 2022 Gao, Cheng, Sun and Li. This is an open-access article distributed under the terms of the Creative Commons Attribution License (CC BY). The use, distribution or reproduction in other forums is permitted, provided the original author(s) and the copyright owner(s) are credited and that the original publication in this journal is cited, in accordance with accepted academic practice. No use, distribution or reproduction is permitted which does not comply with these terms.
*Correspondence: Jianfeng Li, fengzhongfeifan@foxmail.com