- 1UK Center for Ecology & Hydrology, Wallingford, United Kingdom
- 2Institute of Integrative Biology, University of Liverpool, Liverpool, United Kingdom
Landscapes have been drastically transformed by human activities, generally resulting in the loss of semi-natural habitat. In the United Kingdom, wildlife habitat mainly consists of small patches of semi-natural habitat that are poorly connected to each other. In May 2019 the United Kingdom Government published an outcome indicator framework for measuring progress against the goals and outcomes of the 25 Year Environment Plan (YEP) for England. The indicator of the Quantity, Quality and Connectivity of Habitats (D1) is one of seven indicators within the Wildlife theme and it follows the principle of making areas of semi-natural habitat “more, bigger, better and joined up.” In this study, we describe the process of co-designing the connectivity metric for indicator D1. In consultation with experts and stakeholders we selected three candidate landscape connectivity metrics to produce the indicator. The first metric comes from a suite of rules of thumb for practitioners and it is the proportion of habitat patches in the landscape that have a nearest neighbor ≤ 1 km away. The second metric is a habitat fragmentation index from the Natural England National Biodiversity Climate Change Vulnerability Assessment Tool (NBCCVAT). The third and final metric is from the software Condatis and it represents the ability of a species to move through a landscape. We tested each metric on a set of simulated landscapes representing different levels of habitat addition strategies and different spatial configurations. We asked if the metrics are able to detect changes in the connectivity of each of these landscapes after habitat addition. Two of the three metrics (NBCCVAT and Condatis) performed well and were sensitive to change. They both increased as the total extent of habitat increased and each showed particular sensitivity to one spatial arrangement over the other. Given these results, one or both of these metrics could be used to produce the indicator. We discuss the implications of using one or both of the metrics and highlight the fundamental choices that need to be made to produce the indicator.
Introduction
Almost everywhere on the planet, landscapes have been drastically transformed by human activities. In western Europe, agricultural intensification, urban development and afforestation have been identified as the three main drivers of habitat transformation in recent decades (Albon et al., 2011). Landscape changes driven by these human activities usually result in the loss of semi-natural habitat. A recent study found that the county of Dorset in England lost as much as 97% of its neutral grassland and 70% of calcareous grassland habitat since the 1930s (Ridding et al., 2020). The habitat patches that remain are usually small and isolated, surrounded by hostile environments such as urban or agricultural land. A study from 2015 (Haddad et al., 2015) has identified that more than 70% of the world’s forests are within 1 km from a forest edge, with 20% of forests being within 100 m from a forest edge and in close proximity to agricultural, urban, or other modified land cover. As a consequence, wildlife habitat across the world mainly consists of small patches of (semi-) natural habitat that are poorly connected to each other.
The theories of island biogeography (Wilson and MacArthur, 1967) and metapopulation dynamics (Hanski, 1998) show that the isolation of habitat patches, by reducing the movement of individuals between patches, can lead to reduced genetic diversity of local populations, lower rates of colonisation of remaining patches and, therefore, higher likelihood of local extinctions. These predictions have been confirmed by observational studies (Gibson et al., 2013; Morcatty et al., 2013) and fragmentation experiments (Haddad et al., 2015), which found that persistence, abundance and diversity of species are reduced by habitat fragmentation, leading to degradation of ecosystem functions. In addition, globally species are responding to climate change by shifting their geographic range (Chen et al., 2011). Successful shifting depends on the availability of habitat in areas of suitable climate and on the species being able to colonize these patches of suitable habitat (Hodgson et al., 2011; Parks et al., 2020). Habitat loss and climate change therefore pose a synergistic threat to biodiversity, because when availability of suitable habitat is decreased and when remaining habitat is more fragmented and surrounded by hostile environments, it is increasingly difficult for species to shift their ranges to track changing climate conditions. Increasing landscape connectivity is therefore crucial to improve species persistence in fragmented landscapes and facilitate species range shifts in a changing climate (Hodgson et al., 2012; Synes et al., 2020).
The importance of habitat connectivity is recognized in different international agreements, reports and conventions that seek to address the biodiversity and climate change crises. The “2030 Nature Compact” agreed by the 2021 G7 Leaders Summit advocates for “improved quality, effectiveness and connectivity of protected areas and other effective area-based conservation measures (OECMs).” The United Nations General Assembly in 2021 adopted Resolution 75/271, which encouraged member States to “maintain and enhance the connectivity of habitats […], including through increasing the establishment of transboundary protected areas, as appropriate, and ecological corridors based on the best available scientific data.” The International Union for the Conservation of Nature (IUCN) Policy Resolution 073 on “Ecological connectivity conservation in the post-2020 global biodiversity framework: from local to international levels” emphasizes the importance of ecological networks and corridors to sustaining biodiversity and nature’s contributions to people, and recommends that all IUCN Members work to conserve connectivity by documenting it across ecosystems, informing policies, laws, and plans, identifying key drivers and building synergies across institutions and borders to implement solutions.
In the 25 Year Environment Plan (YEP), the UK Government committed to creating a Nature Recovery Network, a network of interconnected wildlife-rich sites that will increase resilience to climate and environmental change. The Network builds on the recommendations in Professor Sir John Lawton’s report “Making Space for Nature” (Lawton et al., 2010), which concluded that England’s network of protected sites (the “ecological network”) was not sufficient to support wildlife populations in a changing climate. To make England’s network resilient to future change, areas of semi-natural habitat should be “more, bigger, better and joined up.” In May 2019 the United Kingdom Government published an outcome indicator framework (DEFRA, 2019) for measuring progress against the goals and outcomes of the 25 Year Environment Plan (YEP) for England. The indicator framework defines 66 indicators within ten themes. The indicator of the Quantity, Quality and Connectivity of Habitats (D1) is one of seven indicators within the Wildlife theme (theme D) and contributes to headline 7, “Changes in nature on land and water that support our lives and livelihoods.” Headline 7 pertains to the 25 YEP Goal “Thriving plants and wildlife.” So the ultimate aim of the indicators in this group is to measure progress towards species conservation.
In this paper we describe the process of co-designing the connectivity metric of indicator D1 on the Quantity, Quality and Connectivity of Habitats. A number of methods are available to measure connectivity: our aim here is to identify the most suitable for use in the indicator. We ran a stakeholder engagement workshop to define the desirable properties for metrics to create the indicator, and to evaluate candidate metrics against those properties. We then tested the performance of the top three candidates in detecting changes in landscape connectivity on simulated scenarios of habitat creation. Given the importance of habitat connectivity in international and national policies as a way to improve species local persistence and their resilience to climate change, indicators of landscape connectivity are a good tool to track initial progress toward the objectives of environmental policies. The steps taken in this study to co-design an indicator with experts, stakeholders and decision-makers provide a framework that can be applied to other countries or regions.
Materials and Methods
This study was co-designed with the Department for Environment Food & Rural Affairs (DEFRA) and Natural England. The design of the connectivity metric for Indicator D1 followed two main steps. First a review of connectivity metrics available from literature was carried out. Different metrics were scored according to a set of criteria that were deemed desirable by policy-makers, stakeholders and experts. This list of candidate metrics was then reviewed by a group of experts during a workshop, which produced a shorter list of top candidates. The second step in the indicator design process was to test the performance of the top candidate metrics on spatial data. The following sections describe these steps in detail.
Desirable Properties of Connectivity Metrics
From the early stages of reviewing possible candidate metrics for a connectivity indicator (or indicators), the following criteria were deemed essential:
• Transparency – the methods used to calculate the metrics are publicly available and can be reproduced
• Practicality – it needs to be practical to produce a new indicator every time new data is available
• Affordability – the connectivity indicator should not be prohibitively expensive to produce in terms of data collection and collation, staff resources and cost of software
• Flexibility - the data input required should be flexible
• Sensitivity – the metric needs to be able to detect changes in landscape connectivity that are biologically relevant to the successful conservation of species within the landscape
• Specificity - the metric should measure what it is expected to measure (connectivity) and not something else
• Representativeness - the metric should behave consistently across different habitats and regions
• Data availability - the data input required should be readily available and updated frequently to allow the indicator to be produced regularly
• Data quality - the data input required should be reliable and produced with a consistent methodology in the future so that the indicator is comparable across years
Ease of communication – the indicator should be easy to communicate to non-technical audiences
Many different connectivity metrics have been developed in the literature (Moilanen and Nieminen, 2002; Kindlmann and Burel, 2008), each with its own sets of strengths and weaknesses. These metrics range from very simple Euclidean distance between habitat patches, to complex, model-based assessment of species movement across landscapes (Bocedi et al., 2014b), or measures of population synchrony (Powney et al., 2011, 2012; UKBI - C2. Habitat connectivity). Generally, simple metrics of landscape structure are easy to calculate and communicate, but they have been criticized for being too simplistic and disregarding the interactions between species movement and the structure of the landscape (Moilanen and Nieminen, 2002). On the other hand, complex models of species movement are biologically more realistic, but can become too species-specific and thus less useful for a generalized indicator. These methods can also be difficult to parameterise due to lack of reliable data and to communicate due to the complexity of the models. We reviewed a selection of tools and metrics from the literature against the criteria above. The metrics and tools reviewed (listed in Supplementary Table 1) include some of the aforementioned approaches, for example simple indices of habitat fragmentation (e.g., Taylor et al., 2014), model-based tools such as RangeShifter (Bocedi et al., 2014a) and Condatis (Wallis and Hodgson, 2018) and metrics of population synchrony (Powney et al., 2012), as well as least-cost methods (Watts and Handley, 2010), network theory approaches (Albert et al., 2017) and conservation prioritization software (Moilanen et al., 2005; Ball et al., 2009).
Experts and Stakeholders Workshop
We organized a workshop with experts and stakeholders to discuss each tool and reduce the list down to fewer candidates. After introducing the indicator and each tool in the review (Supplementary Table 1), the experts triaged each option against the criteria above and discarded unsuitable metrics. Some of the tools were deemed to be more useful as a source of data (Edwards et al., 2018) or as planning tools for intervention (Moilanen et al., 2005; Ball et al., 2009) as opposed to producing the indicator. Other metrics were considered that were not present in the initial review and a rule-of-thumb metric (Crick et al., 2020), described in detail below, was taken forward for consideration as stakeholders felt this was already part of their toolkit and as such it would be simple to produce and interpret.
A second discussion session highlighted the pros and cons of each of the remaining metrics as well as knowledge and data gaps. Some methods (e.g., RangeShifter, network theory approach, least-cost path methods and population synchrony metrics) were considered to be too complex to implement and interpret, as well as requiring data that were unlikely to be available at the desired spatial and temporal resolution and extent. The experts then considered complementarity between the metrics in the context of the policy needs. There are two different policy issues for which connectivity may be relevant. Persistence of local metapopulations is usually facilitated by spatial aggregation of habitat (Hanski and Ovaskainen, 2003), while more even distribution of habitat and stepping stones primarily benefit species shifting their range to respond to climate change (Hodgson et al., 2011). It is therefore desirable that candidate metrics be assessed in light of both policy objectives. It’s possible that the indicator might display or combine two metrics: one that specifically measures the aggregation of habitat patches and another that measures the degree to which species are able to move across the landscape in response to environmental change.
Following these discussions with workshop participants, we reduced the list of candidate tools to three options (Figure 1).
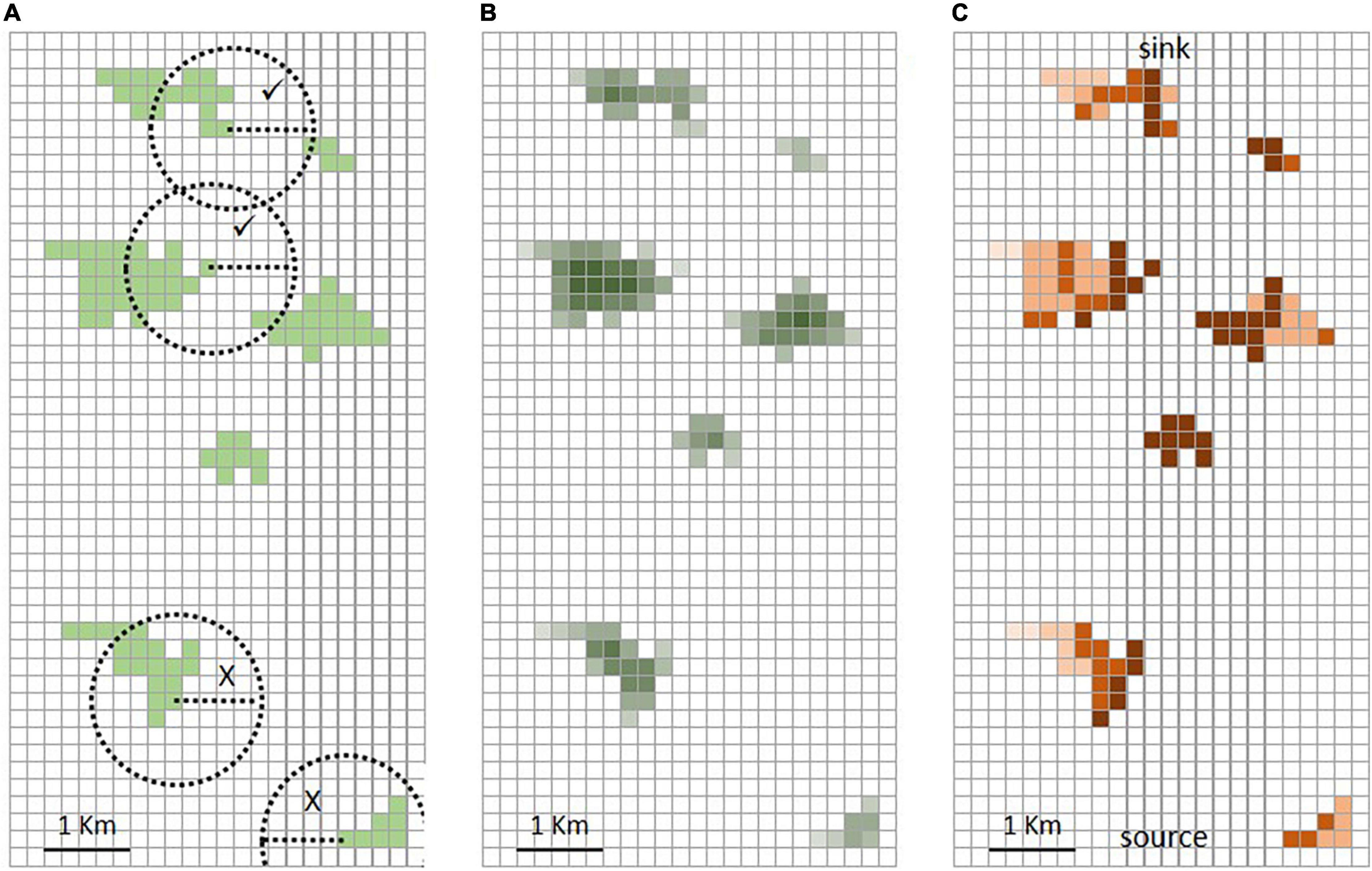
Figure 1. Graphic representation of the three tools tested. (A) The rule of thumb (RT) metric is based on Euclidean distance between patches; we used a moving window to determine if each patch of focal habitat had a nearest neighbor within a 1 km radius. (B) The National Biodiversity Climate Change Vulnerability Assessment Tool (NBCCVAT) tool calculates an index of habitat fragmentation, by scoring each cell of focal habitat according to the number of habitat or non-habitat cells surrounding it. (C) Condatis represents movement across the landscape as current flowing through an electrical circuit; it scores each habitat cell according to its contribution to the overall flow.
• Rule of thumb (RT) – This first metric comes from a suite of rules of thumb aimed at helping practitioners in the design of nature networks (Crick et al., 2020). The rules of thumb follow Lawton’s principles of more, bigger, better and more joined up sites (Lawton et al., 2010) and were based on a review of literature, particularly of those studies relevant to England. For example one rule of thumb is to enlarge protected sites to > 40 ha, while to make the nature network more joined up for poorly dispersing species, sites should be ≤ 1 km from each other. We focus on this last rule, which involves calculating the nearest neighbor distances of all habitat patches and the metric produced is the proportion of patches that have a nearest neighbor ≤ 1 km away.
• Natural England National Biodiversity Climate Change Vulnerability Assessment Tool (NBCCVAT) – NBCCVAT (Taylor et al., 2014) is a flexible GIS-based tool that provides an assessment of the vulnerability of habitats to climate change, using different metrics including a measure of habitat fragmentation. The habitat fragmentation module uses a kernel-based approach to calculate a habitat fragmentation index. The tool computes the habitat fragmentation index for each 200 × 200 m cell in the landscape. From this we can derive an average measure across all cells in the landscape.
• Condatis – Developed to facilitate the planning of habitat networks to enhance long distance connectivity, Condatis (Hodgson et al., 2016; Wallis and Hodgson, 2018) represents species’ movements through the landscape as an electric circuit. In this analogy, the electrical current flowing through the wires and resistors (habitat patches) represents the ability of a species to move through a landscape from a source to a destination. The metric, overall flow speed, is a measure of the connectivity of the entire landscape.
Testing the Metrics
In this section, we describe a simulation study to compare the performance of the three candidate metrics. To do this, we simulated the creation of habitat in three counties of England as well as at the national scale. Both the amount of habitat added and the spatial arrangement of new habitat patches was varied to create 6 habitat creation scenarios for each habitat type and study area. We calculated the three metrics for each of the simulated, as well as for the baseline, landscapes and finally we evaluated each metric in terms of its change from the baseline and in proportion to the area of habitat created.
Data
We used the gridded Land Cover Map 2015 at 25 m resolution as a baseline (Rowland et al., 2017). We restricted the analysis to a range of habitats which would have been more connected in England in the past: woodland, semi-natural grassland, heathland and semi-natural habitat overall (Figure 2).
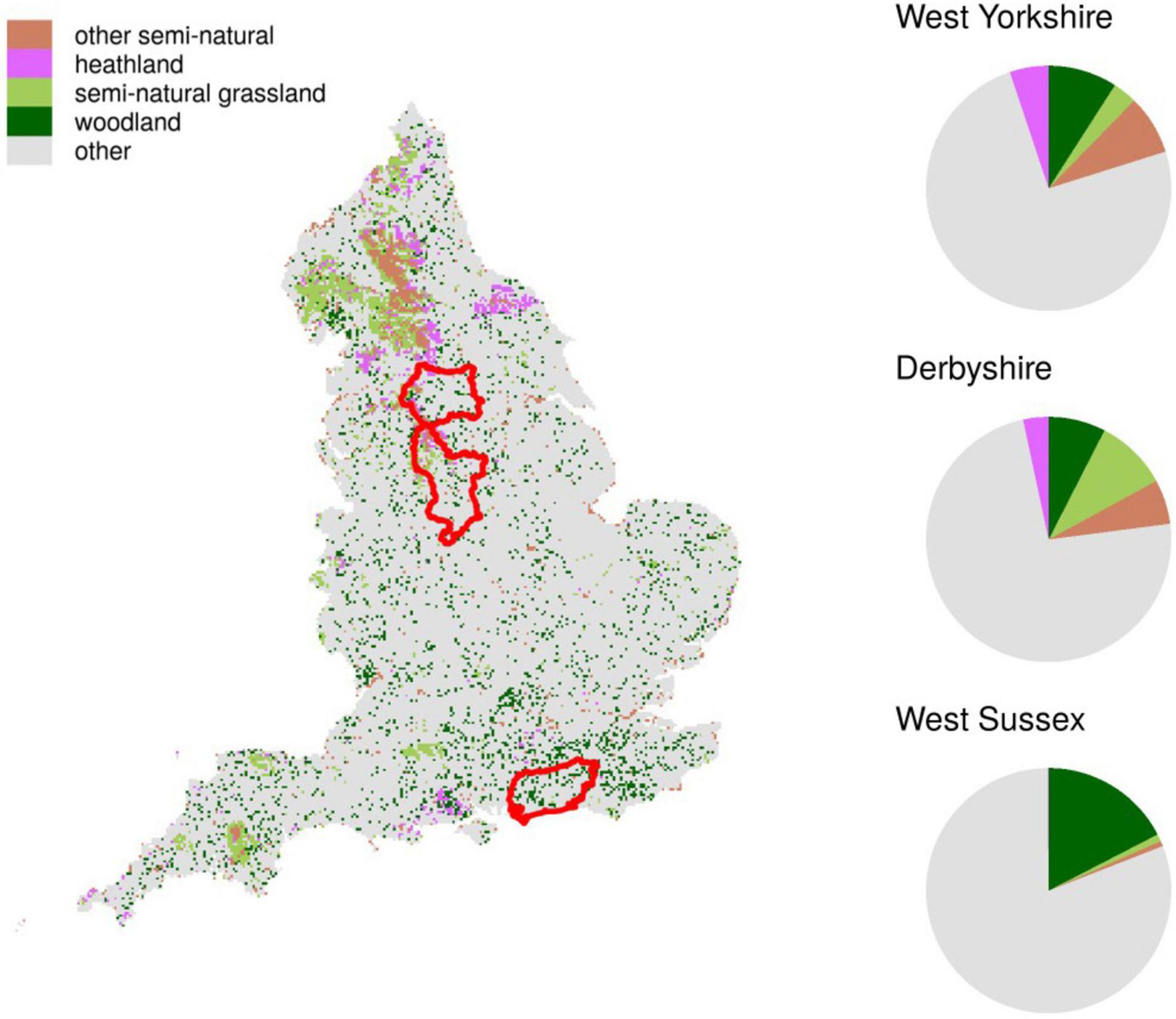
Figure 2. Map of the study area. The red polygons show the three English counties selected in this study: West Yorkshire, Derbyshire and West Sussex (from North to South). Different color squares are 25 m grid cells with aggregated land cover classes as used in this study. Pie charts on the right show the proportion of 25 m cells in each land cover class for the three counties.
The Simulated Landscapes
Habitat restoration and creation are two strategies to increase the resilience of ecological networks, by improving the quality of available habitat patches and creating new ones. New habitat can be created following different spatial arrangements: existing patches can be made bigger, by creating or restoring habitat directly adjacent to the existing patches, or new habitat can be created with the aim of connecting distant and isolated patches (e.g., corridors and stepping stones). These strategies promote the persistence of populations (and metapopulations) in fragmented landscapes, whilst also facilitating species range shifts in a changing climate. However, the optimal solution for habitat creation may be different for the two objectives (promoting persistence vs. range-shifting), because of the different spatial scale at which these two processes operate.
We modified the baseline habitat raster at 25 m resolution from the Land Cover Map 2015 to simulate habitat creation or restoration strategies. For each habitat type, new habitat patches were added until one of three targets was reached: an increase in the focal habitat type by 1, 5 and 10% of the total habitat already present. This was based on “Outcome 1D” of the Biodiversity 2020 strategy (Defra, 2011), which aims to restore 15% of degraded ecosystems as a contribution to climate change mitigation and adaptation. We chose values below this target to test if the metrics would be able to detect an improvement in connectivity with smaller changes to the landscape. The location of the new habitat patches was chosen according to the two contrasting spatial arrangements mentioned above: aggregated and dispersed habitat.
In all cases the broad steps were as follows:
(1) Select a set of non-habitat cells from the baseline, which are destined to become the centers of new patches. The methods of selection to create the two different spatial patterns are described in more detail below. We always propose more patches than we need to reach the maximum target area (a 10% increase over the baseline area), so that the deletions in step 4 below do not create a problem.
(2) Assign desired areas to the new patches. The area to be assigned to each new patch was taken from a distribution of the area of existing patches of the same habitat type, up to a maximum size of 14 ha (Supplementary Table 2).
(3) Expand around each central cell to create a square shape of the desired area.
(4) Check for and remove any overlaps where parts of the new patches overlap existing habitat or other new patches.
(5) Impose cumulative area thresholds (of 1, 5 and 10% of the baseline area) on the list of candidate patches, and write new raster files where habitat is included up to each of these thresholds.
The following sections describe the two methods of new patch selection to create the two different spatial patterns.
Aggregated Habitat
The center of each new patch of habitat in this scenario was selected according to the Hanksi connectivity metric (Hanski, 1998), so that it was more likely to create new habitat patches right next to existing habitat. First we calculated the Hanski metric of “colonization potential” for all non-habitat areas. Specifically, we used the formula
Where cell i could be any cell (habitat or non-habitat), cells j are only those with habitat in the baseline, and dij is the Euclidian distance between cell i and cell j in meters. The mean dispersal distance over 2D space implied by this colonization kernel is 2/α. Tα is the total colonization potential that would occur with the given alpha in any cell in a landscape of 100% habitat – so this means Hα,i is normalized to a maximum of 1. The non-habitat pixels right next to clusters of existing habitat cells obtained the highest scores and the metric decreased with distance from a habitat cell – down to a computationally feasible minimum of approx 10–9.
To help represent the benefits experienced by different species, we calculated the Hanski metric with alpha = 2/500 m and alpha = 2/2500 m over the entire landscape.
To bias the selection of new patches towards existing clusters during the 5-step habitat addition process described above, we:
• Created 10 times as many patches as we needed in step 1, with random centers
• Scored the center cell of each patch with the score (H2/500,i + H2/2500,i)/2, and used this score as the probability of picking the patch in step 5.
Dispersed Habitat
New habitat patches were created at random – i.e., in step 1 above, the center of each patch was a random selection from all the non-habitat pixels in the study landscape.
This framework produced 6 combinations (2 spatial configurations * 3 habitat amounts) for each habitat type. We applied these scenarios to exemplar English landscapes of different spatial scales, therefore testing the ability of the metrics to detect landscape change at different scales. For the local scale scenarios, we picked three English counties with enough focal habitat to obtain a reasonable baseline scenario to which we could apply the 6 habitat creation strategies. We selected Derbyshire, West Sussex and West Yorkshire as each of them had a high coverage of one habitat type and average or below average coverage of the other two (Figure 2). In the end, for each of the 16 base landscapes (3 counties + England * 4 habitat types) we generated 6 scenarios of habitat creation, for a total of 96 simulated landscapes. The scenarios were implemented in R (R Core Team, 2018).
Tools Implementation
All the tools were implemented in R (R Core Team, 2018), making particular use of the raster package (Hijmans, 2020) which helps to make most calculations on rasters very fast. R is open-source software so this contributes to the transparency and affordability of all the indicators.
Our code is very flexible to work with different input data, only requiring: a raster of habitat (habitat type identified by numeric codes); a lookup table defining the particular habitats of interest; a polygon shapefile of the study area (which is used to “cookie cut” the habitat as well as to define the edges of the landscape needed by Condatis).
For every scenario, as well as for the baseline landscapes, we calculated the 3 connectivity metrics as well as simple summary statistics, such as the area of focal habitat coverage, number of habitat patches and average size of habitat patches.
Rule of Thumb (RT)
In order to calculate the proportion of habitat patches with a nearest neighbor at 1 km (or less) distance, we first identified contiguous patches across the raster’s habitat cells – assigning each patch a unique ID. We then used a moving-window algorithm to pinpoint cells that sat in one patch but had a patch with a non-identical ID within 1 km. This method is more transparent and repeatable than using patches defined by an input polygon shapefile, in which minute changes in digitisation could lead to vastly different results.
Once patches were defined across the raster, we calculated the RT, the proportion of patches with a nearest neighbor ≤ 1 km away, and the size of each patch.
National Biodiversity Climate Change Vulnerability Assessment Tool (NBCCVAT)
This tool uses a resolution of 200 m (5 × 5 200 m cells in each 1 km square neighborhood contributing to the proximity analysis). Therefore to run this tool we aggregated the 25 m land cover raster to 200 m, calculating the percentage cover of the focal habitat type in each cell. To calculate the NBCCVAT habitat fragmentation metric, each habitat cell in the landscape is scored according to the number of cells in the surrounding 1 km square that contains the same habitat and their level of aggregation around that central cell. Each habitat cell (in this instance, 200 m × 200 m cells with over 20% habitat coverage) is scored a 1 while non-habitat cells are scored a 0. Then each cell in the 1 km square takes the value of the sum of its adjacent cells. At this step, the score can take values from 0 (non-habitat cell with no adjacent habitat cells) to 9 (habitat cell with all 8 adjacent cells containing the focal habitat). This step is repeated again with the values calculated in step 1. The value is then scaled between 0 (no habitat in the neighborhood) and 1 (100% coverage).
The overall connectivity summary statistic across the entire landscape is taken as the mean of score x habitat presence. This statistic can reach a maximum of p (the proportion of habitat in the landscape) if all present habitat is well connected, and can fall to zero if all habitat cells are isolated by more than 500 m.
Condatis
We calculated Condatis’ “conductance” metric (AKA “speed,” (Hodgson et al., 2016)) in four different directions across each study landscape. The directions were defined by splitting the boundary line of the study landscape into eight segments, with the first segment centered on the most southerly point. In turn, the “source” and “target” for the Condatis analysis were taken to be (a) the south to the north segment, (b) south-west to north-east, (c) west to east, and (d) south-east to north-west. In Condatis, reversing the polarity of the source and target makes no difference to the conductance, so there was no need to run the opposites of these four directions.
Analyzes at county level were performed at 1 ha resolution with a mean dispersal distance of 2.5 km, and those at national level at 5 km × 5 km resolution with a mean dispersal distance of 10 km.
To give a single summary statistic, the geometric mean of conductance in the four directions was taken.
Evaluation
For each simulated landscape, we calculated the percentage change in each metric from the baseline landscape as: (metric - metricbaseline) / metricbaseline.
In the context of an indicator, a useful metric would:
• increase from the baseline with the amount of habitat added
• present extra information besides the amount of habitat added, by reaching higher values when habitat is particularly well placed in the landscape, for example the percentage increase in the metric should be more than the percentage increase in habitat area
We expected different tools to be preferentially sensitive to different landscape spatial arrangements. NBCCVAT is a metric of habitat aggregation, therefore we expect it to be more sensitive (increase proportionally more) in scenarios where habitat was added around high connectivity patches. On the other hand, Condatis was designed to model long-distance dispersal of species through the landscape, therefore we expect it to be more sensitive to the dispersed habitat scenarios. The RT could also be more sensitive to the dispersed habitat scenarios, since it is based on distance between patches and not aggregation of habitat cells.
Results
Landscapes
We created 6 landscapes for each county and habitat type combination and the same number of landscapes for England. Each landscape had a different combination of amount of habitat added and spatial configuration. The woodland 10% aggregation scenario did not add the expected amount of habitat to the baseline landscape so the results for this scenario are not shown.
Tools Comparison
Rule of Thumb (RT)
The RT metric cannot go above 1, which means that no improvement is detected in the metric when the original landscape already has 100% of the patches with a nearest neighbor within a radius of 1 km. In some dispersed habitat scenarios, the metric decreased when adding habitat (Figure 3). For example in the dispersed scenario for England heathland the metric went from 0.88 for the original landscape, to 0.84, 0.72 and 0.66 in the 1, 5 and 10% addition scenarios, respectively (Figure 3). This result is explained by the fact that, by adding more habitat pixels, we are increasing the number of patches in the landscape. Although these additional patches connect distant patches of habitat, this increase in long-distance connectivity is not reflected in the metric because these new patches do not have a nearest neighbor within a 1 km radius. The same was true for the county scale landscapes (Supplementary Figure 1).
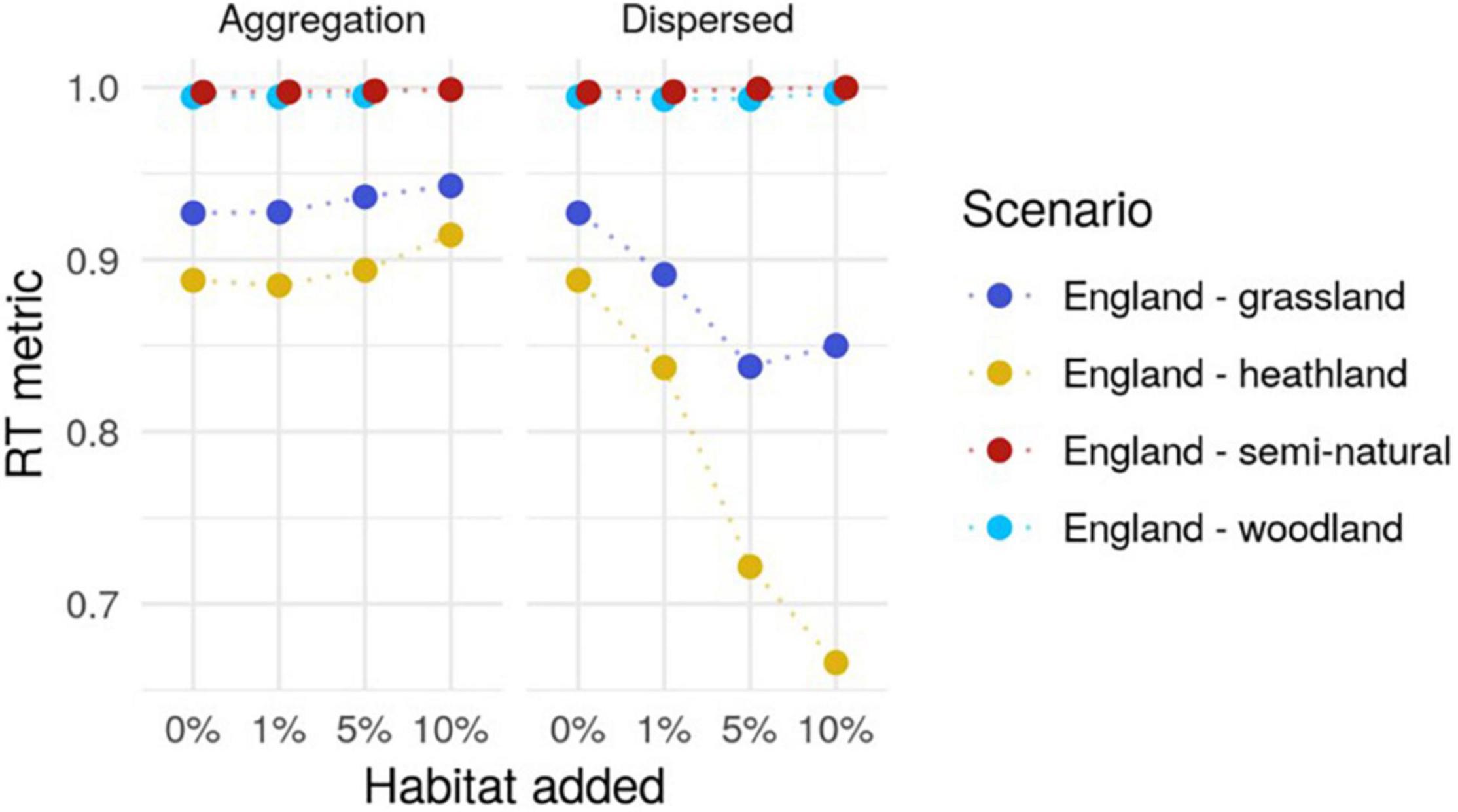
Figure 3. The rule of thumb (RT) metric for each of the simulated landscapes at the England scale. The points are the metric calculated for each scenario, with dashed lines connecting the baseline and three levels of habitat addition.
National Biodiversity Climate Change Vulnerability Assessment Tool (NBCCVAT)
The NBCCVAT metric increased with increasing amounts of habitat both in the county and the England scenarios (Figure 4). The NBCCVAT indicator increased proportionally more in landscapes where the habitat was more aggregated compared to the dispersed habitat scenarios (Figure 4). In the dispersed habitat scenarios the metric increased proportionally less than the percentage amount of habitat added, on average by 0.4, 2 and 5% for the three county scale habitat addition scenarios and always by a percentage that was less than the percentage of habitat added. On the other hand, in the aggregated scenarios the metric increased on average by a percentage that was the same as the amount of habitat added and in cases where the spatial distribution of habitat cells was optimal, for example West Sussex semi-natural, the metric increased by 1.2, 6 and 13%. However, at the England scale the metric increased by the same amount or less than the percentage of habitat added to the landscape.
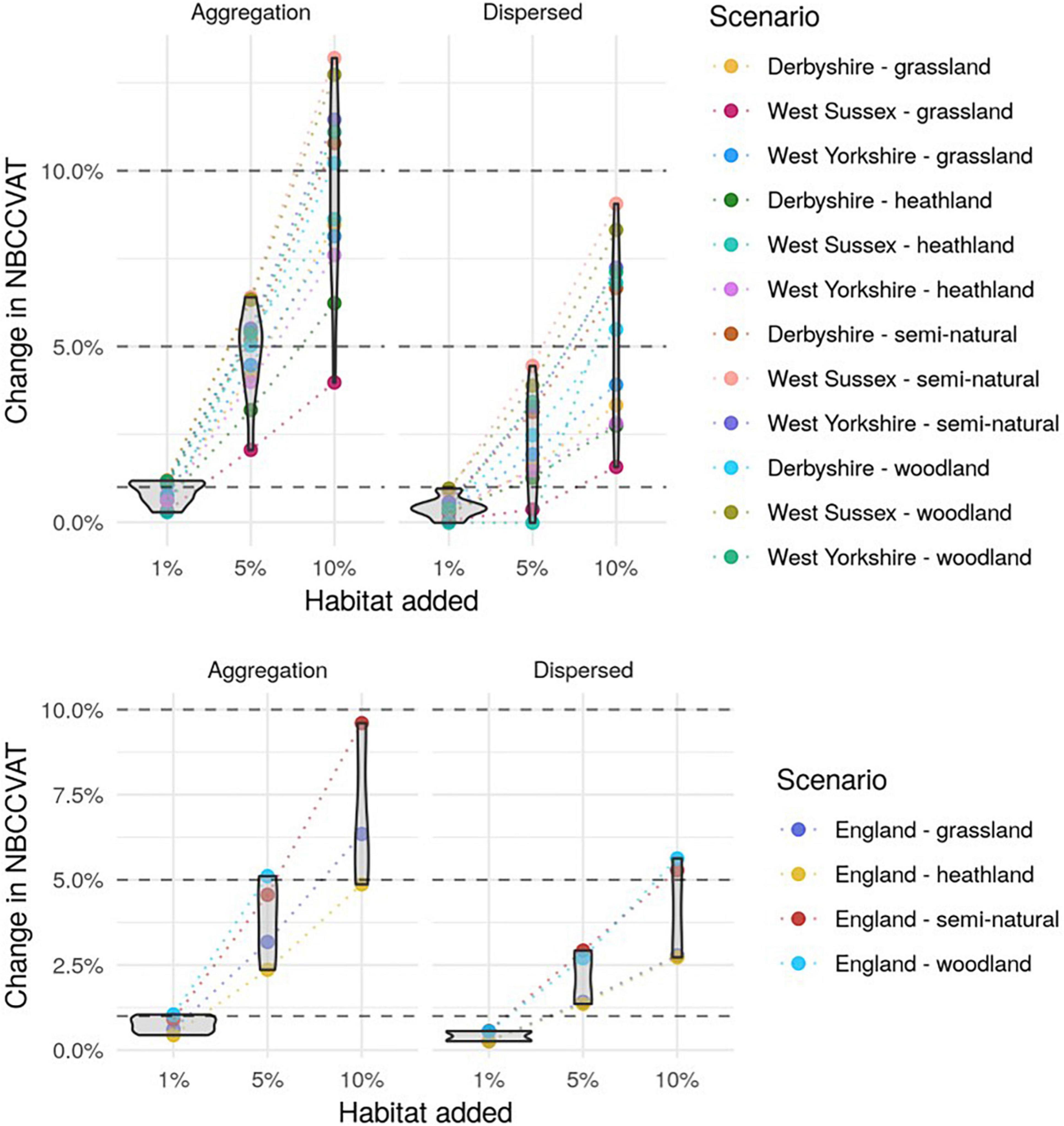
Figure 4. Proportional increase in the National Biodiversity Climate Change Vulnerability Assessment Tool (NBCCVAT) metric for each of the simulated landscapes at the county scale (top) and England scale (bottom). The points are the proportional change in the metric from the baseline for each scenario, with dashed lines connecting the three levels of habitat addition. Violin plots show the distribution of the metric’s proportional change across habitats (for England) and habitat-counties combinations for each of the habitat addition amounts and spatial arrangements. The width of the violin represents the approximate frequency of data points in each region. Dashed horizontal lines indicate a 1, 5, and 10% change in the metric.
Condatis
The Condatis speed metric also showed an increase with increasing amounts of habitat added to the landscapes (Figure 5) and was generally much more sensitive than the NBCCVAT metric, consistently increasing more than the percentage of habitat added (Figures 4, 5). Speed increased by a greater amount in the dispersed habitat scenarios compared to the aggregated scenarios. On average, the speed metric increased by 130, 1418 and 4804% in the three county scale dispersed habitat addition scenarios, while it increased by 1, 13 and 34% in the county scale aggregation scenarios. This was expected and it is due to the nature of the metric. Condatis was designed to measure long-distance connectivity of habitat patches to determine how easily individuals can move across the landscape to expand their range, therefore the speed metric will be more sensitive to scenarios in which distant and isolated patches are connected through stepping stones or corridors.
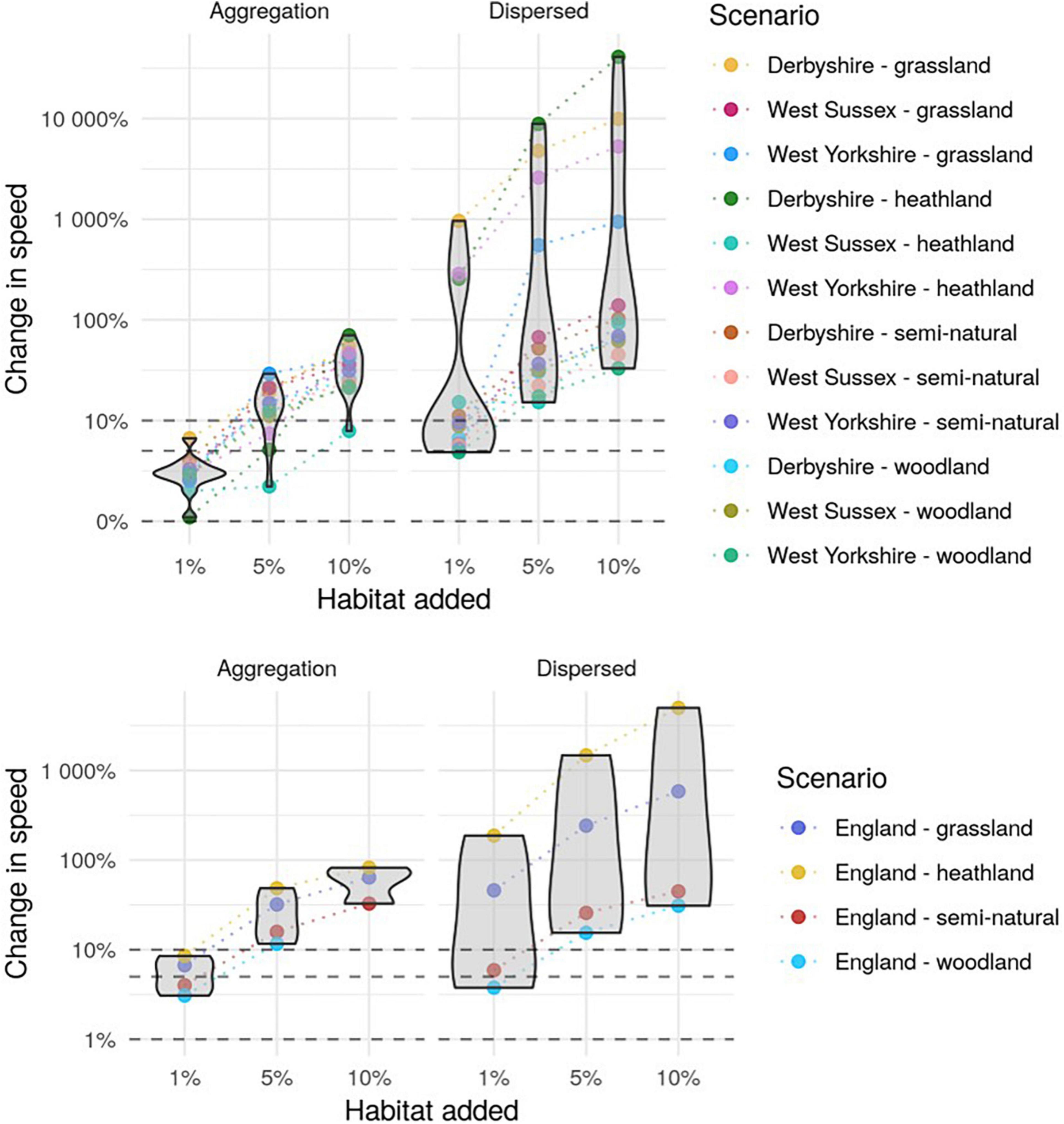
Figure 5. Proportional increase in the speed metric for each of the simulated landscapes at the county scale (top) and England scale (bottom). The points are the proportional change in the metric from the baseline for each scenario, with dashed lines connecting the three levels of habitat addition. Violin plots show the distribution of the metric’s proportional change across habitats (for England) and habitat-counties combinations for each of the habitat addition amounts and spatial arrangement. The width of the violin represents the approximate frequency of data points in each region. Dashed lines indicate a 1, 5, and 10% change in the metric. The y axis is on the log10 scale.
Metrics Sensitivity
There was great variation among the landscapes in the degree of habitat fragmentation as measured by the NBCCVAT (Supplementary Figure 2). For example in the West Sussex heathland aggregated scenarios, the metric went from a baseline of 0.0005 to 0.0006 in the highest habitat addition scenario. On the other hand, according to this metric, the most connected landscapes were the Derbyshire semi-natural habitat aggregated scenarios, where the metric increased from 0.22 to 0.24. This is most likely to be explained by the total area of habitat in the two examples, with heathland in West Sussex only covering around 2 km2, while semi-natural habitat in Derbyshire covers around 691 km2. This metric is highly dependent on the cover of focal habitat in the baseline landscape, increasing proportionally more in landscapes where the focal habitat covers more of the region’s area (Figure 6 left). The same is not true of the speed metric, which is not sensitive to the cover of focal habitat in the baseline landscape (Figure 6 right). However, there is considerable variation in the speed metric across the landscapes. Both at the England and county scale, grassland and heathland are the least connected landscapes according to the speed metric (Supplementary Figure 3), while woodland and semi-natural habitat reached higher values of speed, especially in the dispersed habitat scenarios. For example, the West Sussex semi-natural dispersed habitat scenario saw an increase in speed from 34.6 to 49.9 from the baseline landscape to the 10% habitat addition scenario. On the other hand, West Sussex heathland started from a very small value of speed, around 0.00001, in the baseline scenario and only increased to 0.00003. This low value of speed is most likely driven by the spatial configuration of heathland in the landscape, which tends to be clustered in patches isolated from each other (Figure 2), while woodland exists in smaller patches distributed across the landscape. However, for West Sussex heathland, the value of speed doubled from the baseline to the highest habitat addition scenario, demonstrating how small changes can still be detected with this metric (Figure 5).
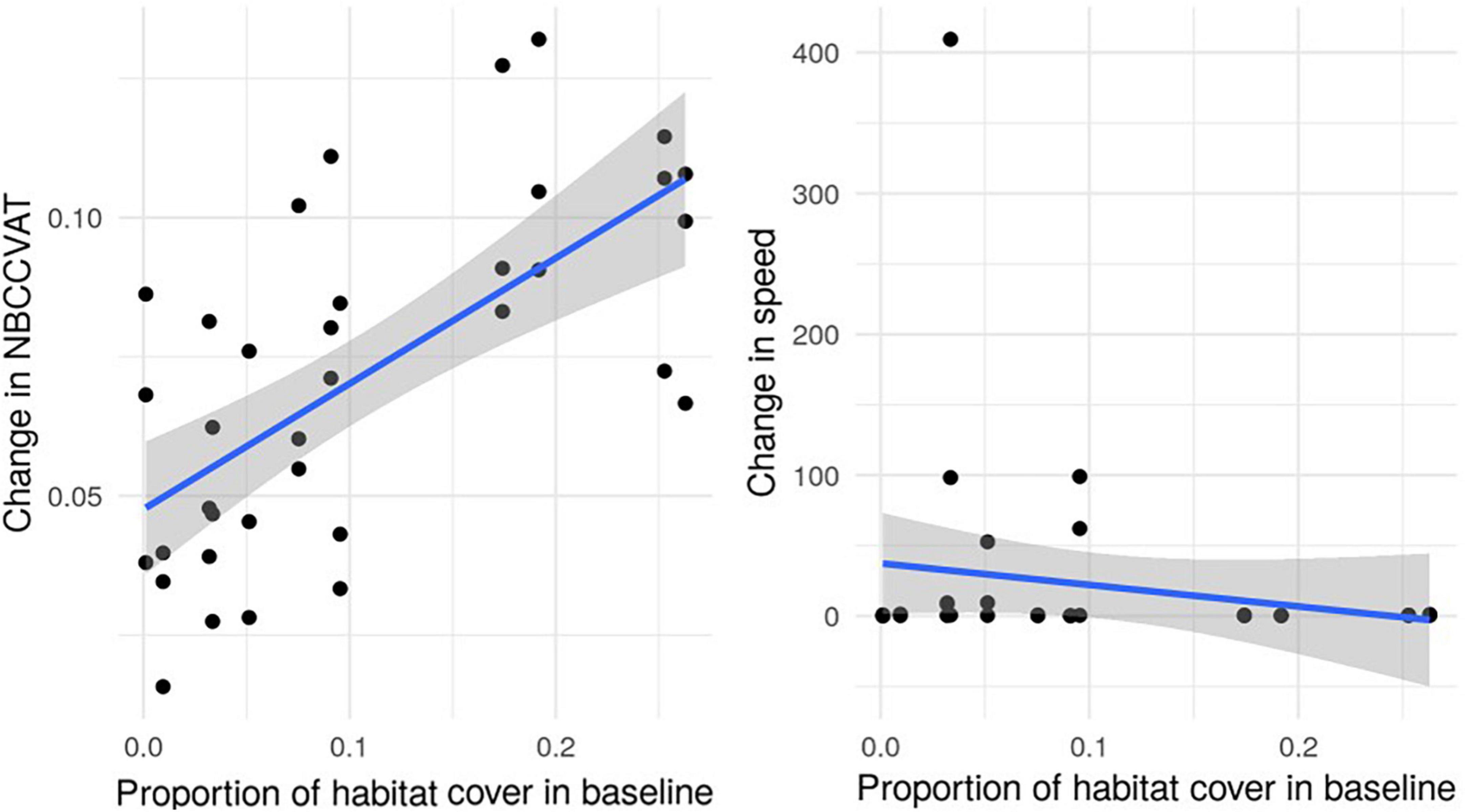
Figure 6. Change in connectivity metrics from baseline in relation to proportion of focal habitat cover. Every point represents the proportional change in the National Biodiversity Climate Change Vulnerability Assessment Tool (NBCCVAT) (left) and speed (right) metric from the baseline for each 10% habitat addition scenario at the county scale against the proportion of focal habitat cover in the baseline landscape. Blue line is the regression line and the gray shared areas are confidence intervals.
Discussion
We have presented our workflow to co-design the connectivity indicator for Indicator D1 of Quantity, Quality and Connectivity of Habitats for England. In collaboration with DEFRA and Natural England and in consultation with field experts, we co-produced a list of selection criteria for the connectivity metrics that would form the indicator and a list of candidate metrics to take forward. We tested three metrics as potential candidates. Two of the three metrics, the NBCCVAT and the Condatis speed metric, showed reliable responses to these landscape changes. Both metrics increased with the addition of new habitat and showed proportionally greater gains in connectivity when the new habitat was placed in optimal spatial configurations. The NBCCVAT and Condatis speed metric also meet the other indicator selection criteria.
Metrics Performance
Rule of Thumb (RT)
The metrics tested differed in their ability to detect change in the simulated landscapes. The RT metric didn’t always increase when habitat was added to the landscape and it sometimes showed a decline (Figure 3). This happens because this metric relies on a threshold value of 1 km to determine a landscape’s connectivity. When stepping stones are added to the landscape to increase long distance connectivity, this metric will not show an improvement unless the new habitat patches are within 1 km of the existing habitat. Moreover, as the RT metric is a simple proportion, it can decline when the newly added habitat creates an isolated patch (>1 km from any other), or where it joins together two existing patches that were previously close together. Also, for most counties and half of the England landscapes, the baseline already had nearly 100% of habitat patches with a nearest neighbor within a 1 km radius, leaving little room for improvement. For these reasons, the RT is not a suitable metric for this connectivity indicator.
National Biodiversity Climate Change Vulnerability Assessment Tool (NBCCVAT)
The NBCCVAT metric is a measure of habitat fragmentation and its value depends on both the coverage of the habitat and its spatial arrangement. The value of this metric would be 1 only when the whole area is covered by the focal habitat. A low value of this metric could result from a landscape with a single continuous patch of habitat that only covers a small proportion of the area or a very fragmented landscape but a higher overall focal habitat coverage. Because of this reliance on the total amount of habitat coverage, the NBCCVAT metric does not increase as fast as, for example, the Condatis speed metric. Despite this, the NBCCVAT metric does show a reliable behavior, increasing proportionally with the amount of habitat added and showing greater increases when placement of new habitat is optimized to reduce local fragmentation, in our case in the aggregation scenarios (Figure 4). It is also possible to decouple the NBCCVAT metric from changes in habitat area, by dividing the metric by the maximum value the metric could take with that amount of habitat. Further work would be required to consider whether the metric should be presented “as is” or scaled for the amount of habitat.
The NBCCVAT metric uses a distance-weighting that was originally chosen for coding ease rather than to represent a species’ dispersal kernel. It is relatively “myopic” in that it assumes habitat more than 500 m from the focal cell has no benefit. We note that, with up-to-date computers and the availability of the R raster package (Hijmans, 2020), a more biologically-based distance weighting is possible (and is being discussed in the context of an update to the NBCCVAT tool), as is performing analyzes at better than 200 m resolution.
Condatis
The Condatis speed metric is complementary to the NBCCVAT metric, being more sensitive to the dispersed compared to the aggregation scenarios (Figure 5). This metric is a measure of the ability of a species to move through a landscape and therefore it increases proportionally more when new habitat is added to reduce the distance between isolated patches. It is important to note that we ran Condatis using exemplar, fairly long dispersal distances (2.5 km at the county scale and 10 km at the England scale). The choice of dispersal distance can have a large effect on the absolute speed, but a lesser effect on the relative impact of different addition patterns (in particular, buffering existing patches will always be sub-optimal and mitigating pinch-points will always be beneficial, regardless of the dispersal distance assumed – Supplementary Figure 4).
Metrics Interpretation
It is important to consider how much the baseline landscape influences both the absolute value of the metrics and their relative change. As Figure 6 shows, change in NBCCVAT metric is positively correlated with the amount of focal habitat already present in the landscape. So any comparison in the progress shown by the indicator between regions will need to be scaled by the initial amount of habitat present. Speed does not seem to be as affected by the initial amount of habitat (Figure 6, right) and more dependent on the spatial distribution of habitat patches in the baseline landscape. For habitats that tend to be clustered into larger and more isolated patches (heathland and semi-natural grassland) the absolute value of speed will be lower in comparison with other habitat types that exist in more dispersed patches. Heathland and grassland landscapes showed the largest relative changes in speed compared to other habitat types, suggesting that an indicator that uses this metric might show diminishing improvements as the landscape gets more and more connected, while the indicator for the less connected landscapes will show the greatest improvements. So again, comparisons must be made with caution and both absolute value and relative change in the indicator should be considered when assessing progress and to inform habitat restoration or creation on the ground.
The NBCCVAT fragmentation metric is less sensitive compared with the speed metric. This is partly because it is bounded between 0 and 1 and the metric can only be 1 when the habitat covers the whole study area. For this reason, the metric does not show the same magnitude of change as speed. However, this change is still informative, as it signifies that, according to the metric’s criteria, the landscape is still fragmented. If only one metric is brought forward to make the indicator, the Condatis speed metric would be the better choice given its greater flexibility in specifying parameter values (resolution and dispersal) and its greater sensitivity to changes in the landscape. However, speed and NBCCVAT metric measure different attributes of the landscape, with speed providing information on the long-distance connectivity of the landscape and NBCCVAT estimating the degree of fragmentation that might be impacting species at a local level. For this reason it might be desirable to retain both metrics in an indicator framework, but comparisons between the two will be tricky because of the difference in their sensitivities. Moreover, presenting two metrics might present a confusing message about how the landscape is changing.
Recommendations for Indicator Development
Finally, several decisions need to be made when developing an indicator from these candidate metrics. First the choice of the spatial resolution to use to derive the metrics. This will be in part dependent on the resolution of the data available. Data at a finer resolution might increase the computational time to calculate the metrics. On the other hand, smaller patches of habitat, such as linear features, are more likely to be captured at a smaller resolution and therefore the metrics might have a higher baseline value. The choice of dispersal distance can have a large impact on the metric of speed (Supplementary Figure 4). As an indicator needs to be general and represent many species dispersal abilities, a possible strategy would be to use an average dispersal distance across all species found in each focal habitat. Another option would be to generate an indicator for each species group according to their dispersal abilities (i.e., one indicator for short-distance dispersers, one for long-distance dispersers and another for average-distance dispersers). The trade-off here is between multiple indicators that are biologically realistic for different species (especially short-distance dispersers that have more stringent requirements) and a concise indicator that can broadly represent all species in a landscape.
Conclusion
It is important to track progress towards environmental targets and using succinct indicators has many benefits, including ease of presentation, impact and clarity of the message. It is very difficult to monitor biodiversity and the impact that local or national scale policies and conservation interventions are having on species. There is often a significant lag between a change in the environment and a response in species richness or abundance/distribution and for this reason setting intermediate milestones and associated targets is necessary to avoid erroneously concluding that a conservation intervention has not been successful (Watts et al., 2020). Improvements in habitat quality, quantity and connectivity can represent such intermediate milestones, forgoing the colonization of the restored habitat by different species. Such indicators are useful for evaluating the success of conservation actions and provide incentives for further interventions.
Data Availability Statement
The original contributions presented in the study are included in the article/Supplementary Material, further inquiries can be directed to the corresponding author.
Author Contributions
FM conducted the tool review. NI and FM organized and ran the workshop. JH and FM conducted the simulations, implemented the tools and analyzed the results. All authors contributed to the writing of the manuscript.
Funding
This work was funded through a research partnership agreement between Defra, Natural England and the Centre for Ecology & Hydrology (CEH) number ECM_54322, which builds upon work supported by the Natural Environment Research Council award number NE/R016429/1 as part of the UK-SCAPE program delivering National Capability.
Conflict of Interest
The authors declare that the research was conducted in the absence of any commercial or financial relationships that could be construed as a potential conflict of interest.
Publisher’s Note
All claims expressed in this article are solely those of the authors and do not necessarily represent those of their affiliated organizations, or those of the publisher, the editors and the reviewers. Any product that may be evaluated in this article, or claim that may be made by its manufacturer, is not guaranteed or endorsed by the publisher.
Acknowledgments
The authors would like to acknowledge all the experts and stakeholders that attended the workshop for their input and feedback. We are particularly grateful to Kevin Watts and Humphrey Crick for feedback provided during the first stages of this work and on earlier drafts. Many thanks to all the members of the D1 Indicator Development Steering Group for their guidance and feedback. Finally we are grateful for the support from the research partnership agreement between Defra, Natural England and the Centre for Ecology & Hydrology (CEH) number ECM_54322, which builds upon work supported by the Natural Environment Research Council award number NE/R016429/1 as part of the UK-SCAPE program delivering National Capability.
Supplementary Material
The Supplementary Material for this article can be found online at: https://www.frontiersin.org/articles/10.3389/fevo.2022.892987/full#supplementary-material
References
Albert, C. H., Rayfield, B., Dumitru, M., and Gonzalez, A. (2017). Applying network theory to prioritize multispecies habitat networks that are robust to climate and land-use change. Conserv. Biol. 31, 1383–1396. doi: 10.1111/cobi.12943
Albon, S., Turner, K., Watson, R., Anger, A., Baker, J., Bateman, I., et al. (2011). UK National Ecosystem Assessment: Synthesis of the Key Findings. Cambridge: Cambridge University Press.
Ball, I. R., Possingham, H. P., and Watts, M. (2009). “Marxan and relatives: Software for spatial conservation prioritisation,” in Spatial Conservation Prioritisation: Quantitative Methods and Computational Tools, eds A. Moilanen, K. A. Wilson, and H. P. Possingham (Oxford: Oxford University Press), 185–195. doi: 10.1371/journal.pone.0182396
Bocedi, G., Zurell, D., Reineking, B., and Travis, J. M. J. (2014b). Mechanistic modelling of animal dispersal offers new insights into range expansion dynamics across fragmented landscapes. Ecography 37, 1240–1253. doi: 10.1111/ecog.01041
Bocedi, G., Palmer, S. C. F., Pe’er, G., Heikkinen, R. K., Matsinos, Y. G., Watts, K., et al. (2014a). RangeShifter: A platform for modelling spatial eco-evolutionary dynamics and species’ responses to environmental changes. Methods Ecol. Evol. 5, 388–396. doi: 10.1111/2041-210X.12162
Chen, I. C., Hill, J. K., Ohlemüller, R., Roy, D. B., and Thomas, C. D. (2011). Rapid range shifts of species associated with high levels of climate warming. Science 333, 1024–1026. doi: 10.1126/science.1206432
Crick, H. Q. P., Crosher, I., Mainstone, C., Taylor, S., Wharton, A., Langford, P., et al. (2020). Nature Networks Evidence Handbook. Natural England Research Report NERR081. York: Natural England Research.
Defra (2011). Biodiversity 2020: A strategy for England’s wildlife and ecosystem services. Available online at: https://www.gov.uk/government/uploads/system/uploads/attachment_data/file/69446/pb13583-biodiversity-strategy-2020-111111.pdf (accessed May 27, 2022).
DEFRA (2019). Measuring environmental change: outcome indicator framework for the 25 Year Environment Plan. London: DEFRA.
Edwards, J., Knight, M., Taylor, S., and Crosher, I. (2018). National Habitat Network Mapping Project - Combined Habitats Networks Map User Guidance Document v.1. Available online at: https://s3-eu-west-1.amazonaws.com/data.defra.gov.uk/Natural_England/Habitat_Species/Habitats/Habitat_Network_England_NE/Habitat+Network+Mapping+Guidance+November2018.pdf (accessed September 7, 2021).
Gibson, L., Lynam, A. J., Bradshaw, C. J. A., He, F., Bickford, D. P., Woodruff, D. S., et al. (2013). Near-complete extinction of native small mammal fauna 25 years after forest fragmentation. Science 341, 1508–1510. doi: 10.1126/SCIENCE.1240495/SUPPL_FILE/GIBSON.SM.PDF
Haddad, N. M., Brudvig, L. A., Clobert, J., Davies, K. F., Gonzalez, A., Holt, R. D., et al. (2015). Habitat fragmentation and its lasting impact on Earth’s ecosystems. Sci. Adv. 1:e1500052. doi: 10.1126/sciadv.1500052
Hanski, I., and Ovaskainen, O. (2003). Metapopulation theory for fragmented landscapes. Theor. Popul. Biol. 64, 119–127. doi: 10.1016/S0040-5809(03)00022-4
Hijmans, R. J. (2020). raster: Geographic Data Analysis and Modeling. Available online at: https://cran.r-project.org/package=raster (accessed June 9, 2020).
Hodgson, J. A., Thomas, C. D., Cinderby, S., Cambridge, H., Evans, P., and Hill, J. K. (2011). Habitat re-creation strategies for promoting adaptation of species to climate change. Conserv. Lett. 4, 289–297. doi: 10.1111/j.1755-263X.2011.00177.x
Hodgson, J. A., Thomas, C. D., Dytham, C., Travis, J. M. J., and Cornell, S. J. (2012). The Speed of Range Shifts in Fragmented Landscapes. PLoS One 7:e47141. doi: 10.1371/journal.pone.0047141
Hodgson, J. A., Wallis, D. W., Krishna, R., and Cornell, S. J. (2016). How to manipulate landscapes to improve the potential for range expansion. Methods Ecol. Evol. 7, 1558–1566. doi: 10.1111/2041-210X.12614
Kindlmann, P., and Burel, F. (2008). Connectivity measures: A review. Landsc. Ecol. 23, 879–890. doi: 10.1007/s10980-008-9245-4
Lawton, J., Brown, V., Elphick, C., Fitter, A., Forshaw, J., Haddow, R., et al. (2010). Making Space for Nature: A review of England’s Wildlife Sites and Ecological Network. London: Defra.
Moilanen, A., Franco, A. M. A., Early, R. I., Fox, R., Wintle, B., and Thomas, C. D. (2005). Prioritizing multiple-use landscapes for conservation: Methods for large multi-species planning problems. Proc. R. Soc. B Biol. Sci. 272, 1885–1891. doi: 10.1098/rspb.2005.3164
Moilanen, A., and Nieminen, M. (2002). Simple connectivity measures in spatial ecology. Ecology 83, 1131–1145.
Morcatty, T. Q., El Bizri, H. R., Carneiro, H. C. S., Biasizzo, R. L., de Oliveira Alméri, C. R., da Silva, E. S., et al. (2013). Habitat loss and mammalian extinction patterns: are the reserves in the Quadrilátero Ferrífero, southeastern Brazil, effective in conserving mammals? Ecol. Res. 28, 935–947. doi: 10.1007/S11284-013-1076-3
Parks, S. A., Carroll, C., Dobrowski, S. Z., and Allred, B. W. (2020). Human land uses reduce climate connectivity across North America. Glob. Chang. Biol. 26, 2944–2955. doi: 10.1111/gcb.15009
Powney, G. D., Broaders, L. K., and Oliver, T. H. (2012). Towards a measure of functional connectivity: Local synchrony matches small scale movements in a woodland edge butterfly. Landsc. Ecol. 27, 1109–1120. doi: 10.1007/s10980-012-9771-y
Powney, G. D., Roy, D. B., Chapman, D., Brereton, T., and Oliver, T. H. (2011). Measuring functional connectivity using long-term monitoring data. Methods Ecol. Evol. 2, 527–533. doi: 10.1111/j.2041-210X.2011.00098.x
R Core Team (2018). R: A Language and Environment for Statistical Computing. Vienna: R Foundation for Statistical Computing.
Ridding, L. E., Watson, S. C. L., Newton, A. C., Rowland, C. S., and Bullock, J. M. (2020). Ongoing, but slowing, habitat loss in a rural landscape over 85 years. Landsc. Ecol. 35, 257–273. doi: 10.1007/s10980-019-00944-2
Rowland, C. S., Morton, R. D., Carrasco, L., McShane, G., O’Neil, A. W., and Wood, C. M. (2017). Land Cover Map 2015 (25m raster, GB). Atlanta, GA: NERC.
Synes, N. W., Ponchon, A., Palmer, S. C. F., Osborne, P. E., Bocedi, G., Travis, J. M. J., et al. (2020). Prioritising conservation actions for biodiversity: Lessening the impact from habitat fragmentation and climate change. Biol. Conserv. 252, 108819. doi: 10.1016/j.biocon.2020.108819
Taylor, S., Knight, M., and Harfoot, A. (2014). “National biodiversity climate change vulnerability model”. Natural England Research. Report NERR054. York: Natural England Research.
Wallis, D. W., and Hodgson, J. A. (2018). Condatis; software to assist with the planning of habitat restoration. Available online at: http://wordpress.condatis.org.uk (accessed June 12, 2019).
Watts, K., and Handley, P. (2010). Developing a functional connectivity indicator to detect change in fragmented landscapes. Ecol. Indic. 10, 552–557. doi: 10.1016/j.ecolind.2009.07.009
Watts, K., Whytock, R. C., Park, K. J., Fuentes-Montemayor, E., Macgregor, N. A., Duffield, S., et al. (2020). Ecological time lags and the journey towards conservation success. Nat. Ecol. Evol. 4, 304–311. doi: 10.1038/s41559-019-1087-8
Keywords: habitat connectivity, environmental indicators, co-design, habitat restoration, environmental policy
Citation: Mancini F, Hodgson JA and Isaac NJB (2022) Co-designing an Indicator of Habitat Connectivity for England. Front. Ecol. Evol. 10:892987. doi: 10.3389/fevo.2022.892987
Received: 09 March 2022; Accepted: 22 June 2022;
Published: 08 July 2022.
Edited by:
Andrew Gregory, University of North Texas, United StatesReviewed by:
Pedro Cardoso, University of Helsinki, FinlandPhillip Staniczenko, Brooklyn College (CUNY), United States
Copyright © 2022 Mancini, Hodgson and Isaac. This is an open-access article distributed under the terms of the Creative Commons Attribution License (CC BY). The use, distribution or reproduction in other forums is permitted, provided the original author(s) and the copyright owner(s) are credited and that the original publication in this journal is cited, in accordance with accepted academic practice. No use, distribution or reproduction is permitted which does not comply with these terms.
*Correspondence: Francesca Mancini, ZnJhbWFuQGNlaC5hYy51aw==
†These authors have contributed equally to this work and share first authorship