- 1Department of Wildlife, California State Polytechnic University, Arcata, CA, United States
- 2Wildlife Health Laboratory, California Department of Fish and Wildlife, Rancho Cordova, CA, United States
For mammalian mesopredators, human-dominated landscapes offer a mosaic of risk and reward. While the reward of anthropogenic food resources may attract mesopredators to human-dominated areas, increased mesopredator activity and abundance in these areas may cause interspecific conflict. For smaller-bodied mesopredators, the perceived risk of intraguild predation by larger mesopredators may be enough to drive spatial and temporal avoidance strategies to reduce risk while still benefiting from anthropogenic resources. We evaluated how the spatiotemporal activity of four non-canid mammalian mesopredators – raccoon (Procyon lotor), striped skunk (Mephitis mephitis), opossum (Didelphis virginiana), and domestic cat (Felis catus) – might change in the presence of an intraguild predator, the coyote (Canis latrans), and a “super predator,” humans. We quantified mesopredator activity by deploying camera traps at 110 sites across an urban gradient in the Sacramento Metropolitan Area in central California, USA. We hypothesized that mesopredators would likely change their spatiotemporal activity in response to urban intensity (H1), coyotes (H2), human presence (H3), and if urban intensity might mediate the response to humans and coyotes (H4). We used single-species occupancy models to test how mesopredators responded to different spatial scales of urbanization, as well as the temporal presence of coyotes and humans. Top single-species models then informed two-species conditional occupancy models to evaluate how mesopredators responded to “dominant” coyotes. Finally, we used temporal overlap analyzes to evaluate whether activity patterns of mesopredators changed in response to humans and coyotes across three levels of urban intensity. Mesopredators did not change their spatial or temporal activity across the urban gradient when coyotes were present. Additionally, coyotes did not influence mesopredator temporal activity at 1-3-day scales; however, raccoons and cats may avoid coyotes at finer scales. Humans influenced mesopredator detectability, as cats and coyotes avoided humans after 1 and 2 days respectively, and opossums avoided urban areas when humans were present within 3 days. Coyotes may play a limited role in altering smaller-bodied mesopredator activity, especially when humans are present. While the impacts of human presence and urban features are often linked, mesopredators may perceive the risk each poses differently, and adjust their activity accordingly.
Introduction
Human development and land use have made a patchwork of risk and reward for wildlife species globally (Venter et al., 2016). In the western United States, historic land conversion of natural areas into agricultural and residential lands have disturbed tropic food webs by removing apex predators from the landscape while simultaneously increasing food resources and habitat for opportunistic mesopredators (Prugh et al., 2009). Specifically, smaller-bodied mammalian mesopredators are a trophic guild that has benefited greatly from anthropogenic resources and are often highly tolerant and resilient to the highest levels of human disturbance and development (Bateman and Fleming, 2012).
While these generalist mammalian mesopredators are often ubiquitous across a wide range of habitats and levels of human disturbance, increased population densities may potentially put these species into competition with each other, especially if they are drawn to the same anthropogenic resources (Šálek et al., 2014; Theimer et al., 2015). Furthermore, as these mesopredators navigate landscapes dominated by humans, they may experience a higher risk of human-caused mortality (Baker et al., 2007). Therefore, in order to understand how mammalian mesopredators are able to thrive in human-dominated landscapes, ecologists must understand how these species may adapt and avoid potential interspecies stressors and mortality.
Identifying conflict caused by mammalian interspecies interactions can be complex. For instance, whereas competition while scavenging or seeking out anthropogenic food sources is hard to determine, conflict between species based on body size, such as intraguild predation, is often more apparent (Brown and Maurer, 1986). In the western United States, coyotes, (Canis latrans) may fill the role as de facto apex predators due to their larger body size compared to other mammalian mesopredators (Kitchen et al., 1999; Kozlowski et al., 2008; Grubbs and Krausman, 2009). This can lead them to being the dominant predator in a system, excluding other species from resource patches they inhabit (Thompson and Gese, 2007). However, the coyote’s role as an intraguild predator has largely been investigated in relation to other canids such as foxes, where body size and territorially may be the drivers of coyote aggression (Voigt and Earle, 1983; Lesmeister et al., 2015). Evidence for coyote influence over other species such as raccoons (Procyon lotor), striped skunks (Mephitis mephitis), opossums (Didelphis virginiana) is not as clear (Gipson et al., 2003; Gehrt, 2004; Gehrt and Prange, 2007). However, this may be a result of studies examining coyote and mesopredator interactions in or near urban areas where humans may play a larger role in affecting these mesopredators’ behavior.
Human presence can have profound impacts on mammalian mesopredator behavior and activity. For instance, mesopredators may view humans as threatening “super predators,” as humans disproportionately hunt and kill mammalian mesopredators at rates higher than other predators do (Darimont et al., 2015). As a result, mesopredators may increase their alertness and avoidance behaviors in response to humans more than other mammalian threats, such as domestic dogs (Clinchy et al., 2016). Humans may also influence mesopredators differently depending on the level of human activity in the area. In rural and agricultural areas, mesopredators may see humans as more of a threat due to activities such as hazing and lethal removal that may occur at lower rates in more urban areas (Prange et al., 2003). Thus, mesopredators may adjust their spatial or temporal activity to avoid humans depending on the density of humans.
Additionally, mammalian mesopredators may respond differently to features of urban areas compared to the direct impacts of humans themselves. For example, mesopredators living in urban areas have smaller home range sizes due to access to more readily available food. This can impact species movement and their ability to avoid each other, leading to increased competition and disease transmission compared to non-urban areas (Prange and Gehrt, 2004; Šálek et al., 2014; Murray et al., 2015). However, mesopredator species may also have different thresholds of how far into urban centers they are able or willing to traverse due to habitat requirements and the fragmentation of corridors (Tigas et al., 2002). Compared to smaller mesopredators, coyotes may be better able to move through and utilize more open agricultural land, but may be impeded by higher density of anthropogenetic structures (e.g., fences and buildings) in urban centers (Gehring and Swihart, 2003; Fidino et al., 2020). Thus, smaller species that have less impeded movement (e.g., more arboreal raccoons and opossums) may be able to access highly urbanized areas and gain a spatial refugia from their competitors/intraguild predators (Gosselink et al., 2003; Kozlowski et al., 2008; Mueller et al., 2018). Yet, if the ability to spatially avoid humans and other anthropogenic stressors becomes limited, mammalian mesopredators may rely on temporal partitioning (Wang et al., 2015).
The goal of our study was to evaluate whether coyote and human presence influence the spatial and temporal activity of three wild mammalian mesopredator species—raccoon, opossum, and striped skunk, and one domestic mesopredator, the domestic cat (Felis catus)—across a gradient of urban intensity. We used camera traps to quantify mesopredator activity throughout a variety of habitats and city centers in the Sacramento Metropolitan Area in central California. While many studies of mesopredators in urban areas use qualitative categorization of non-urban versus urban areas, there are limitations to these study designs, as wildlife may utilize different parts of urban areas seasonally, or move between categorically defined areas (Alberti et al., 2001). Additionally, urban studies using camera traps have typically focused on areas of relatively low levels of human development surrounded by more intact habitat in urban parks and nature preserves (Prange and Gehrt, 2004; Riley, 2006; Ordeñana et al., 2010). While these studies are important in addressing how species may react to low levels of intensity, they do not capture the full range of urbanization’s effects on mesopredators. Thus, we sought to quantify mesopredator activity across a continuous gradient of urban landcover types to fully assess mesopredator spatiotemporal responses to coyotes and humans.
We used occupancy and temporal overlap modeling to investigate four hypotheses addressing mesopredator response to urban intensity, coyotes and human presence. We tested whether mesopredator species varied their spatiotemporal activity due to urban intensity (H1: urban intensity hypothesis); avoided coyotes (H2: intraguild predation risk hypothesis); avoided humans (H3: human super predator hypothesis); and if they changed avoidance of coyotes and humans across urban intensities (H4: urban-mediated response to predators hypothesis). We predicted that the activity of each mesopredator species would vary depending on their tolerance to urbanization (H1) and thus, we would find more support for H4 compared to H2 and H3 alone. We first used single-species occupancy models to address a series of predictions. We predicted that mesopredators would have decreased detection when coyotes (H2) or humans were present (H3), and that detection of mesopredators would be influenced by an interaction between urban intensity and coyotes and/or humans (H4). We then used top covariates from single-species occupancy models to inform conditional two-species occupancy models to further evaluate coyotes’ role as a “dominant” intraguild predator. If mesopredators avoided coyotes, mesopredator occupancy and detection would be conditional on coyote presence (H2) and this may change due to urban intensity (H4). Finally, we used temporal overlap models to see if mesopredator temporal activity differed significantly when coyotes (H2) and humans (H3) were present and if urban intensity mediated these responses (H4).
Materials and Methods
Study Area
Our study was conducted in the California’s Central Valley, focusing on the Sacramento Metropolitan Area (SMA) and surrounding urban areas (Figure 1). More than two million people live in this area (U. S. Census Bureau, 2019), with populations expected to grow substantially due to increasing demand for housing, development, and economic growth (Soulard and Wilson, 2015). Interspersed between these urban centers are areas of varying levels of rural, exurban, suburban, and urban development, as well as highly productive agricultural land and several natural parks, refuges, and preserves (Wassmer, 2000).
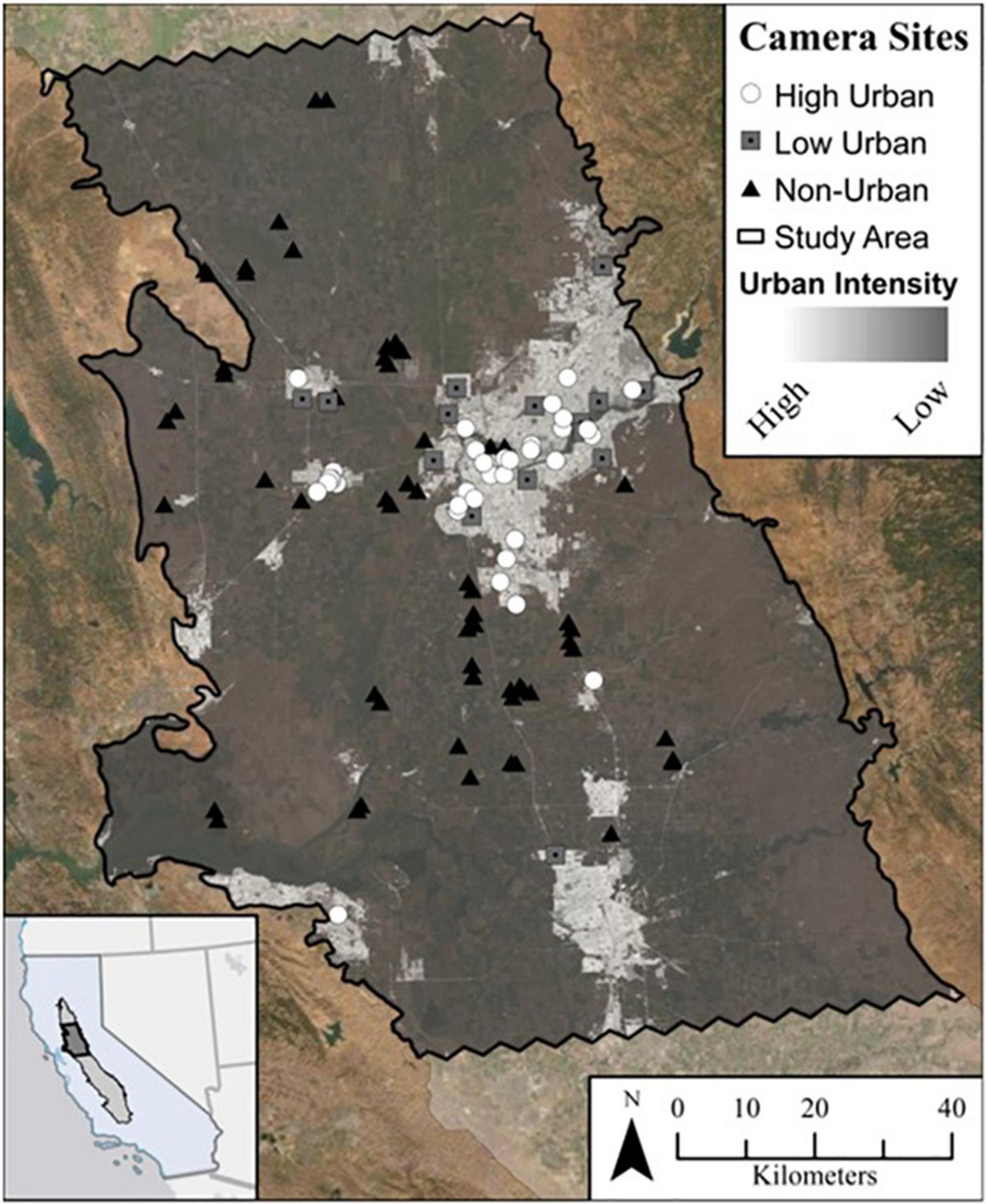
Figure 1. Camera sites (n = 110) across urban intensity in the Sacramento Metropolitan Area within California’s Central Valley. Urban intensity is based on a combination of imperviousness and building density within a 500 m kernel density search radius at 60 m2 resolution. Cameras are labeled here as either high intensity urban (white circles; n = 34), low intensity urban (gray squares; n = 16), or non-urban (black triangles; n = 60) based on k-means clustering.
The Sacramento Metropolitan Area generally exhibits a Mediterranean climate, with winter rainfall from the Sierras feeding into the Sacramento Delta, which is heavily diverted to agriculture and urban areas along the way (Durand et al., 2020). The Sacramento and American Rivers come to a confluence in the study area, acting as major ecological corridors for a variety of species, while also creating a highly biodiverse and productive landscape for hydrological function and socio-economic activity.
Study Design
Our goal was to sample for mesopredators using camera traps across a diverse and continuous urban gradient. Our site selection process followed two steps, (1) select sites based on a diversity of urban areas and features and (2) define the urbanization gradient at sites based on quantitative continuous variables. We rotated 30 cameras through a total of 110 camera sites during the survey period from May to August 2019. To get a representative sample across the urban gradient, we targeted placing cameras across a variety of sites including natural preserves, agricultural areas, nature parks and trails, city parks, and exurban, suburban, and city residences. In order to get enough camera sites at high intensities of urbanization, we recruited volunteer homeowners and private residents in the SMA that would allow us to place a camera on their property. Volunteers were recruited through email listservs, advertisement at universities and wildlife professional conference, and through the word of mouth. To simplify access and permission to place cameras, a majority of our residential sites represented single-family households, rather than apartments or business/commercial buildings.
Camera sites were at least 1 km apart to reduce spatial autocorrelation. To avoid temporal autocorrelation, we ensured that sites within similar geographic areas (cities, communities, or of the same landowner) were not all sampled at the same time. To do this, we split the study area into three equal regions, and selected one site from each community type (city, town, or locality) within each region by latitude (running north and south) until we had 30 sites split evenly through the three regions. On our subsequent rotation of cameras, we would then sample sites adjacent to previously sampled sites within each grouping, until all sites had been sampled within the survey period. This reduced the chance of individual animals being detected on nearby cameras within the same sample period and of sampling similar sites within one camera rotation. Based on previous camera studies in the area, we used a 14-day survey period for each camera site to maximize camera detection while also reducing the risk of theft or damage to cameras in urban areas.
For open, less human dense areas, we generally followed the camera placement methods described in Rich et al. (2018), modifying by necessity in more urban areas. We attached cameras to T-posts in areas that were open, secure, and had explicit landowner permission; otherwise, we attached cameras to any available attachment point (e.g., buildings, fence-posts, trees, poles). We placed cameras at residential houses either within backyards or front yards depending on landowner preference. We positioned cameras in backyards and front yards toward areas where there were holes in the fence or suspected walkways for mesopredators to use. We tried to avoid placing cameras in areas with completely enclosed fencing when possible; however, this was sometimes unavoidable in residential backyards. We preferentially positioned cameras to face north in areas where direct sunlight could cause false triggers and glare on the camera lens; however, microsite characteristics such as attachment structure, landowner permission, and movement corridors also determined camera direction. We secured cameras with lockboxes, cable locks, and padlocks as well as placed cameras in inconspicuous locations to avoid human interference. We preferentially placed cameras in areas that would maximize mesopredator detection while also avoiding areas where humans might preferentially use (e.g., targeting game trails rather than human walkways). We baited each site with fishy cat food at the beginning of the sample period to maximize mesopredator detection. Cameras in public areas with high human density were checked one to two times for camera functionality, battery, and signs of vandalism. Three cameras experienced failure upon first deployment and needed to be redeployed, meaning these cameras experienced two rounds of bait; however, this did not alter expected camera detection rates compared to similar camera site detections.
We visually inspected all photos over three rounds of quality assurance and all wildlife observations were identified to species when possible. All species were recorded for every photo they appear in, including detections of humans and any field technicians. Species records were considered independent following 30 min of a previous detection. From all consolidated species records, we created independent records using the “assessTemporalIdenepdence” function in the package “camptrapR” using R statistical software (Niedballa et al., 2016). Species were included in the analysis given enough detections (n = 10) for comparison. All statistical analysis was done in R (R Core Team, 2014). All methods were approved by the Humboldt State University Institutional Animal Care and Use Committee (IACUC), protocol # 08 16.17.W.08-A and adhere to the animal care and use policy.
Defining the Urban Gradient
We used two continuous variables that represented the physical footprint and functionality of human dense areas – building density and imperviousness―to define our urban gradient. Building density is a measure of the number of built structures within a given area. Building density was derived from Microsoft Building Footprint1 (accessed 11 Nov 2020), which defines building polygons for the whole conterminous United States. Likewise, imperviousness is a measure of how much of an area is covered by impervious surfaces – often areas of concrete or other built surfaces associated with roads, buildings, and urban sprawl. Imperviousness was derived from US Geological Survey’s mapping of impervious surfaces across the conterminous United States at a 60 m resolution (Falcone, 2017). Imperviousness is calculated as the ratio of land cover within each 60 m2 pixel that is covered in impervious surfaces, where a completely concrete landscape is classified as a 1, and a natural area with some water seepage would be classified as a 0.
Since mesopredators may respond to urbanization at different spatial scales, we created several variations for each variable. For building density, we converted building footprint polygons within the study area into centroid point data, which was then used as an input into the “Kernel Density” function in ArcMap (version 10.1). We used the “Kernel Density” function with four different search radii (100, 200, 500, and 1,000-m) to create four 30 m × 30 m raster layers representing the weakening influence of buildings on mesopredators at increased distances. We then calculated the mean value of each kernel density raster at four buffer sizes around each camera site (500, 1,000, 2,000, and 5,000-m) using the “raster” and “rgdal” packages in R (Bivand et al., 2020; Hijmans et al., 2020). This created 16 permutations for building density at multiple search radii and buffer size scales for each camera site. Since imperviousness was already derived from raster data, we calculated the mean value of the imperviousness raster at the same four increasing buffer sizes (500, 1,000, 2,000, and 5,000-m) used for building density. We scored imperviousness from 0 to 100 in order to standardize imperviousness at a scale similar to building density. This created four permutations for imperviousness at each camera site. In general, the larger the search radius and buffer size, the broader the effect building density and imperviousness had on camera sites (Supplementary Material 1).
Data Analysis
We used a three-part analytic process in order to understand how mesopredators respond to the risk of humans and coyotes across the urbanization gradient. First, single-species occupancy models were used to inform whether mesopredator detection differed in urban areas when coyotes or humans were present within 1, 2, or 3 days. Next, conditional two-species occupancy models informed by the single-species models were used to determine whether mesopredator presence and detection was conditional on coyote presence and detection. Finally, a temporal overlap analysis was used to evaluate whether mesopredators changed their overall temporal activity patterns in response to coyotes, humans, and each other.
Single-Season, Single-Species Occupancy Modeling
We used single-season, single-species occupancy models to evaluate whether mammalian mesopredators spatiotemporally responded to changes in urban intensity (H1), coyote presence (H2), human presence (H3), and an interaction between urban intensity and humans and coyotes (H4). Occupancy models estimate the probability of species occurrence at sampled sites (psi or ψ) after controlling for the probability of detecting a species if it is present (p; MacKenzie et al., 2017). Since mammalian mesopredator species may have large home ranges and thus may be able to move between camera sites sampled, potentially beyond our 1-km buffer, occupancy can be viewed as species “use” of sampled areas. The sample period for single-species occupancy models was 14 days across all camera sites, with each occasion being 1 day. We created detection histories for all mesopredator species as well as humans using the package “camtrapR” in R (Niedballa et al., 2016).
Building density and imperviousness at created spatial scales were included to determine variation in mesopredator occupancy (use) and detection (H1). To test whether changing human and coyote presence across the urbanization gradient influenced mesopredator detection probability, interaction terms between the urbanization covariates and coyote/human presence were included in detection models (H4).
Smaller mesopredators may respond to areas where coyotes have recently passed through; thus, coyote “presence” may have a lingering effect as a result of scent marking or other sign or behavior not captured on camera (H2). We used coyote detection history gathered from cameras as a way to measure coyote presence as a detection covariate. Coyote presence was tested at three temporal scales—1, 2, or 3 days. Covariates for coyote presence after 2 or 3 days were created by adding an extra “detection” to the coyote detection history for 1and 2 days, respectively. A maximum of 3 days was used as variation in covariate levels beyond 3 days decreased substantially.
Mesopredators may also avoid camera sites as a result of humans being present (H3). Thus, we included a covariate representing the daily detection history of humans at each camera site across the sample period. Human presence was treated with the same sequential temporal scale as coyote presence where a covariate was created for 1, 2, and 3-day lingering effects of human presence.
We included several covariates pertaining to sample design to test for imperfect detection of mesopredators. We included a covariate for camera placement to test whether placing the camera in an open greenspace (i.e., agricultural area, city park, area with minimal fencing), or in a resident’s front yard (fencing, open to a street) or backyard (highest level of fencing) would change detection of mesopredator species. We recorded if anthropogenic food resource such as residential fruit and vegetables, open trash, dog or cat food left outside, or bird feeders were present within 20 m of the camera area. This “food” covariate was coded as binary covariate (two levels, 1 = food present, 0 = food absent) and was only noted at the beginning of the sample period and for the immediate area surrounding the camera. To quantify the potential drop-off in detection due to baiting, we included a bait decay covariate representing decay of bait over the 14-day study period. Finally, we included Julian date to test whether species detection changed as a result of sampling period. All continuous occupancy and detection covariates (building density, imperviousness, and Julian date) were standardized to make sure differences in variation and ranges of covariate units were all comparable.
We created candidate model sets for each of the five mesopredator species using the “occu” function in the package “unmarked” in R (Fiske and Chandler, 2011). Candidate model sets for each species were created by first testing building density and imperviousness at each spatial scale on species occupancy. Then, models were created to establish best imperfect detection covariates (placement, date, bait, and food) per species. Finally, the impact of coyote and human presence on species detection as well as interactions with building and imperviousness were tested. We compared models (∼45–50 models per species) using Akaike’s Information Criterion for small sample sizes (AICc; Anderson and Burnham, 2002). If the c-hat value of the global model was over 1, a quasi-AICc (QAICc) methodology was used to select for top models by correcting for overdispersion, otherwise, if the c-hat value was 1 or below, c-hat was set to 1 and AICc was used (MacKenzie and Bailey, 2004). The top model used for interpretation of results in each candidate model set was the model with the lowest AICc score. Models within delta 2 AICc were also inspected if they included other covariates not in the top model, however, these covariates were uninformative and not included in interpretation of results.
Goodness of fit for each model set was determined by creating a partial global model representing a combination of all detection covariates and occupancy covariates at the best scale. This global model was then used for a goodness of fit test using the package “AICmodavg” in R to determine the median c-hat value with 2,000 bootstraps (Mazerolle, 2020). The global model for opossums was the only model that failed the goodness of fit test due to overdispersion (c-hat > 2). The addition of a detection covariate representing a lag effect was included to correct this goodness of fit test, where including the detection history of opossums plus a lag of 3 days helped bring the c-hat value down to appropriate levels. This lag effect, opo3, was then included in all of the previous models as a detection covariate to correct for overdispersion.
Conditional Two-Species Occupancy Modeling
We used single-season, two-species occupancy modeling to capture how subordinate mesopredators responded to coyotes and whether mesopredators land use and detection were conditional on coyotes’ presence and detection (H2). Occupancy and detection variables can either be conditional (estimated separately) or unconditional (estimated together; no difference between variables) in order to predict the relationship between a dominant species (coyotes) and a subordinate species (all other mesopredators; Richmond et al., 2010).
Coyote occupancy/use can be estimated directly as in a single-species model (ψA), while coyote detection can be determined by the presence (pA) or absence (rA) of another species. Since we were interested only in whether subordinate species (species B) responded to coyotes as a dominant intraguild predator/intimidator (species A), we made coyote detection unconditional, setting pA = rA. For subordinate species, occupancy/use probability can be either conditional, depending on coyote presence (ψBA) and absence (ψBa); or unconditional, or unchanging depending on whether coyotes are present at a site or not (ψBA = ψBa). Subordinate species detection probabilities depend on whether coyotes are absent (ψB), whether both species are present and detected (rBA) and whether both species are present, but coyotes are not detected (rBa). If coyote detection does not influence subordinate mesopredator detection, then subordinate mesopredator detection is unconditional on coyote detection (rBA = rBa) but conditional on presence; while if coyote presence does not influence subordinate species detection at all, then subordinate mesopredator detection is fully unconditional on coyotes (pB = rBA = rBa).
To reduce the amount of potential candidate models, we used top single-species occupancy models to inform two-species models. Top occupancy covariates for coyotes were used for ψA, and top detection covariates were used for pA. For subordinate species, top occupancy covariates were used for ψBa and top detection covariates were used for pB. Continuous covariates were standardized in the same way as they were for single-species occupancy modeling.
We used two rounds of model selection and candidate model sets, the first for detection probability and the second for occupancy probability, to determine top two-species models. Detection covariates for subordinate mesopredators (pB) were checked using a backwards selection process, eliminating detection covariates until models had the least number of problematic covariates. Detection covariates for coyotes were inspected and remained untouched for each species pairing to keep coyote detection similar across all four candidate model sets. Once we selected top detection covariates for pB, we checked detection of subordinate species versus coyote detection for unconditionality (rBA = rBa), as well as subordinate species detection versus coyote presence (pB = rBA = rBa). The top detection model was selected using the same protocol as single-species model selection, as well as selecting for the top configuration of conditional/unconditional detection variables.
We then used the top detection model to decide the top occupancy model. Occupancy models were tested to see if subordinate species occupancy was unconditional on coyote presence (ψBA = ψBa). Top occupancy covariates were tested for ψBA and ψBa in both conditional and unconditional configurations. Additionally, if subordinate mesopredator occupancy was considered conditional (ψBA) on coyotes, building density and imperviousness covariates could be included to see if urbanization impacted the conditional relationship of this occupancy (H4). Top occupancy models were then selected using the same protocol as before. If subordinate mesopredator occupancy was considered conditional, a Species Interaction Factor (SIF) could be calculated (Richmond et al., 2010). The SIF is centered around 1 and tells us if subordinate species were more likely to be around the coyotes (SIF > 1) or if they avoided coyotes (SIF < 1).
If there was a discrepancy between whether a model was conditional or unconditional within the top two AICc ranking for both detection and occupancy models, we would choose the conditional model to see if the beta estimate 95% confidence intervals crossed zero. If the confidence intervals did cross zero, the beta estimate for the conditional variable would be considered uninformative. We chose this method as opposed to inspecting model-averaged results to avoid misinterpretation of model interpretation that may come from model averaging (Richmond et al., 2010; Cade, 2015).
All models were built using program PRESENCE2 (PRESENCE Version 2.12.43; accessed 11 Nov 2020) using condensed detection history formatting. As there are currently no goodness of fit tests for two-species occupancy global models, goodness of fit for each model is assumed based on single-species occupancy models for each species pairing.
Temporal Overlap
Times and dates from photo metadata were used to interpret the temporal activity patterns of mesopredators. We used the “overlap” package in R, which relies on a non-parametric kernel density analysis of species temporal data to estimate activity patterns and temporal overlap of each species (Meredith and Ridout, 2014). Temporal overlap is calculated as the coefficient of overlap ( or D-hat) between two species’ activity patterns. D-hat ranges from 0 to 1, where a value of 0 indicates no temporal overlap and a value of 1 indicates complete temporal overlap. As suggested by Ridout and Linkie (2009), we used two methods to estimate D-hat as provided by the overlap package; D-hat1 () for when at least one species had a small sample size (n < 50), and D-hat4 () for when both species had large sample sizes (n > 50). To account for changing daylight hours between surveys influencing species activity, we used the sunTime command in the “overlap” package to scale temporal activity to be between sunrise and sunset across survey periods (Nouvellet et al., 2012).
Unfortunately, temporal overlap analysis cannot determine overlap of species across a continuous urban gradient. Therefore, we used k-means clustering to group sites into three groups based a combination of building density and imperviousness. These variables were selected at the 500 m buffer scale based on preliminary occupancy analysis of mesopredators. Species detections were combined for the three “urban intensity” clusters: non-urban (n = 60), low intensity urban (n = 16), and high intensity urban (n = 34). We decided on three clusters in order to capture as much variation across the urban gradient while still having a large enough sample size for each species within each cluster. If the same species was detected at a site within 30 min of a previous detection, it was removed from the analysis.
We first compared within species overlap from urban intensity to urban intensity (intraspecies, H1) and then compared overlap of species pairs for each urban intensity (interspecies, H4). We used 95% confidence intervals for each D-hat estimate determined from 10,000 bootstrap samples to compare overlap estimates of species pairs between years. Coyotes had enough detections for comparisons between two of the urban intensity categories (non-urban and low urban intensity) which are included for analysis (H2); however, as there was only one coyote detection at a high intensity urban camera site, predictions could not be made for intraspecies or interspecies comparisons of coyotes at the highest urban intensity. Human detections were also analyzed to compare mesopredator overlap to changes in human presence across urban intensities (H3).
Results
Cameras were active for a total of 1,540 trap nights across the three-month survey season. A total of 3,133 detections were made of 51 species. Total mesopredator detections include 88 coyote, 139 raccoon, 181 opossum, 136 striped skunk, 552 domestic cat, and 698 human detections. Four other mammalian mesopredators were detected but had too few detections to be considered for analysis; these included the American mink (Neovison vison; n = 3), bobcat (Lynx rufus; n = 4), gray fox (Urocyon cinereoargenteus; n = 2), and red fox (Vulpes vulpes; n = 5).
Single-Season, Single-Species Occupancy Models
In general, global single-season, single-species occupancy models for each of the five mesopredator species all passed goodness of fit tests. Urbanization covariates had varying effects on occupancy (use) and detection probability for all mesopredator species (H1, Table 1, Figure 2). All mesopredator species were found throughout the urban gradient, with average naïve occupancy ranging from 0.288 (coyotes) to 0.400 (cats). Candidate model selection tables can be viewed in Supplementary Material 2.
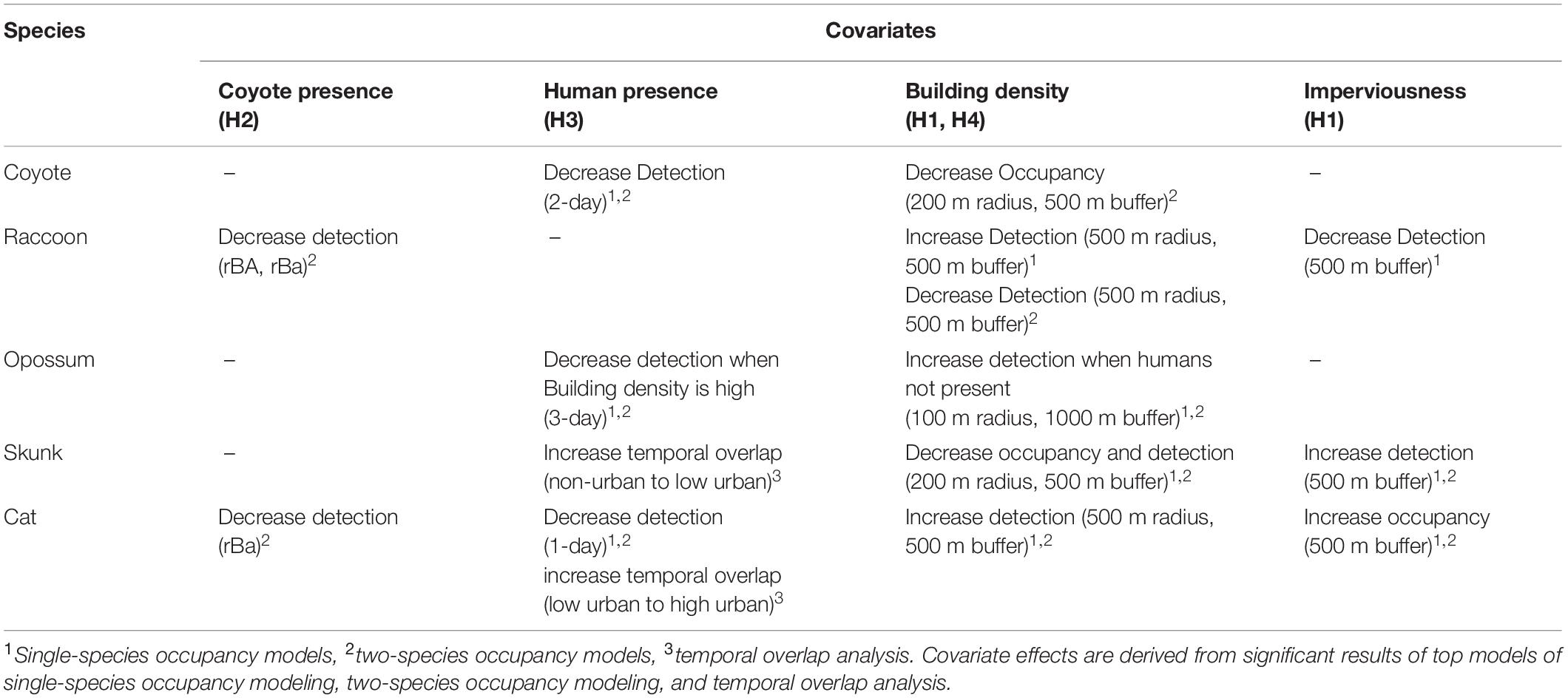
Table 1. Summary of important covariates effects and associated hypothesizes on mesopredator species.
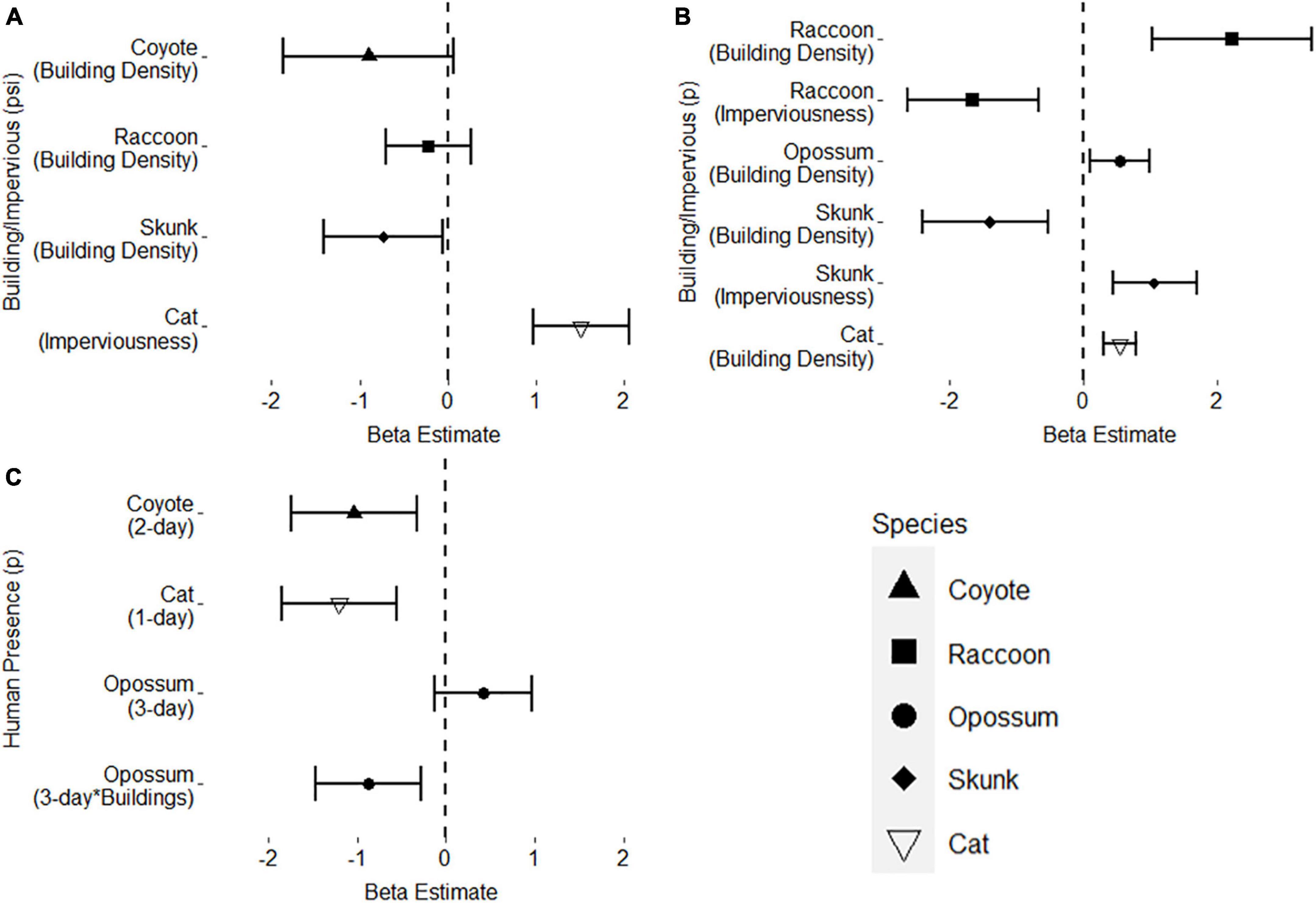
Figure 2. Beta estimates and 95% confidence intervals for top single-species mesopredator occupancy models. Beta estimates that cross the dashed line at 0 are not considered significant. (A) Top urbanization covariates, building density and imperviousness, for mesopredator occupancy. (B) Top urbanization covariates, building density and imperviousness, for mesopredator detection. (C) Top human presence covariates for mesopredator detection as well as an interaction term for human presence across building density for opossum detection.
Building density was the top occupancy predictor for all wild mesopredators; however, striped skunks were the only species that had a significant decrease in occupancy due to building density. Domestic cats were the only species that included imperviousness as their top occupancy covariate, with cat occupancy increasing as imperviousness increased. Both building density and imperviousness were included as important detection covariates in the top models of all mesopredators except for coyotes. As building density increased, raccoon, opossum, and domestic cat detection also increased, while striped skunk detection decreased. On the other hand, imperviousness had the opposite effect of building density; increasing striped skunk detection and decreasing raccoon detection as imperviousness increased.
While small-bodied mesopredators’ detectability changed in response to the physical features of urbanization, the presence of coyotes and humans across the urban gradient had varying effects on mesopredator detection. In general, coyote presence within a 1, 2, or 3-day period did not explain or influence mesopredator detection, as it was not included in any top models for any of the smaller mesopredators (H2). Additionally, coyote presence and interaction terms with building density and imperviousness were tested for all subordinate mesopredators, with no significant improvement of AICc rankings in models that included these interaction terms (H4).
Human presence, on the other hand, influenced coyote, cat, and opossum detection at varying timescales (H3). Coyotes were less likely to be detected when humans were present at camera sites within a 2-day period, while domestic cat detection also decreased when humans were present during a 1-day period. Opossums were the only mesopredator to respond to humans differently across the urbanization gradient, with the top model including the interaction term between human presence and building density (H4). Thus, while opossums were more likely to be detected as building density increased, opossum detection would decrease in high building dense areas compared to low building dense areas when humans were present within a 3-day period.
Camera placement had varying effects on mesopredators (Table 2). Coyotes and raccoons were more likely to be detected in greenspaces, while cat detection decreased. Mesopredators showed no trend for detection within front yards; however, coyotes, raccoons, and domestic cats all were less likely to be detected within backyards. Camera placement had no impact on striped skunk or opossum detection. Julian date influenced coyote, raccoon detection. As the season progressed, coyote and skunks were more likely to be detected while raccoons were less likely to be detected. As bait decayed, there was a decrease in the detection of both coyotes and domestic cats, while anthropogenic food sources positively influenced cat detection.
Conditional Two-Species Occupancy Models
Coyote presence and detection had varying influence on smaller bodied, or subordinate, mesopredators (H2, Table 3). All two-species top models included top covariates for coyote detection from single-species occupancy modeling. These covariates included camera placement, Julian date, bait decay, and human presence in a 2-day period for estimating coyote detection with and without subordinate species presence and detection (pA = rA). All coyote detection covariate estimates followed similar trends to single-species modeling estimates with Julian date significantly increasing coyote detection while bait decay and human presence significantly decreased coyote detection. Camera placement also followed similar trends, with backyards negatively influencing coyote detection and greenspaces positively influencing coyote detection, while front yard estimations could not be calculated due to coyote not being detected in any front yard camera sites.

Table 3. Predicted two-species occupancy model conditionality for dominant coyotes versus subordinate mesopredator species based on top detection and occupancy model configurations.
Additionally, top models for all species pairings included building density at the 200-m kernel density and 500-m buffer size as a covariate for coyote occupancy (ψA). While single-species models estimated building density for coyotes to be trending negative, but non-significant; most two-species models (except for coyote-cat pairings) determined coyotes used lower building density areas compared to high density areas (Figure 3). Reporting of top models for species pairings below will focus on subordinate species parameters and responses to coyotes. Candidate model selection tables can be viewed in Supplementary Material 3.
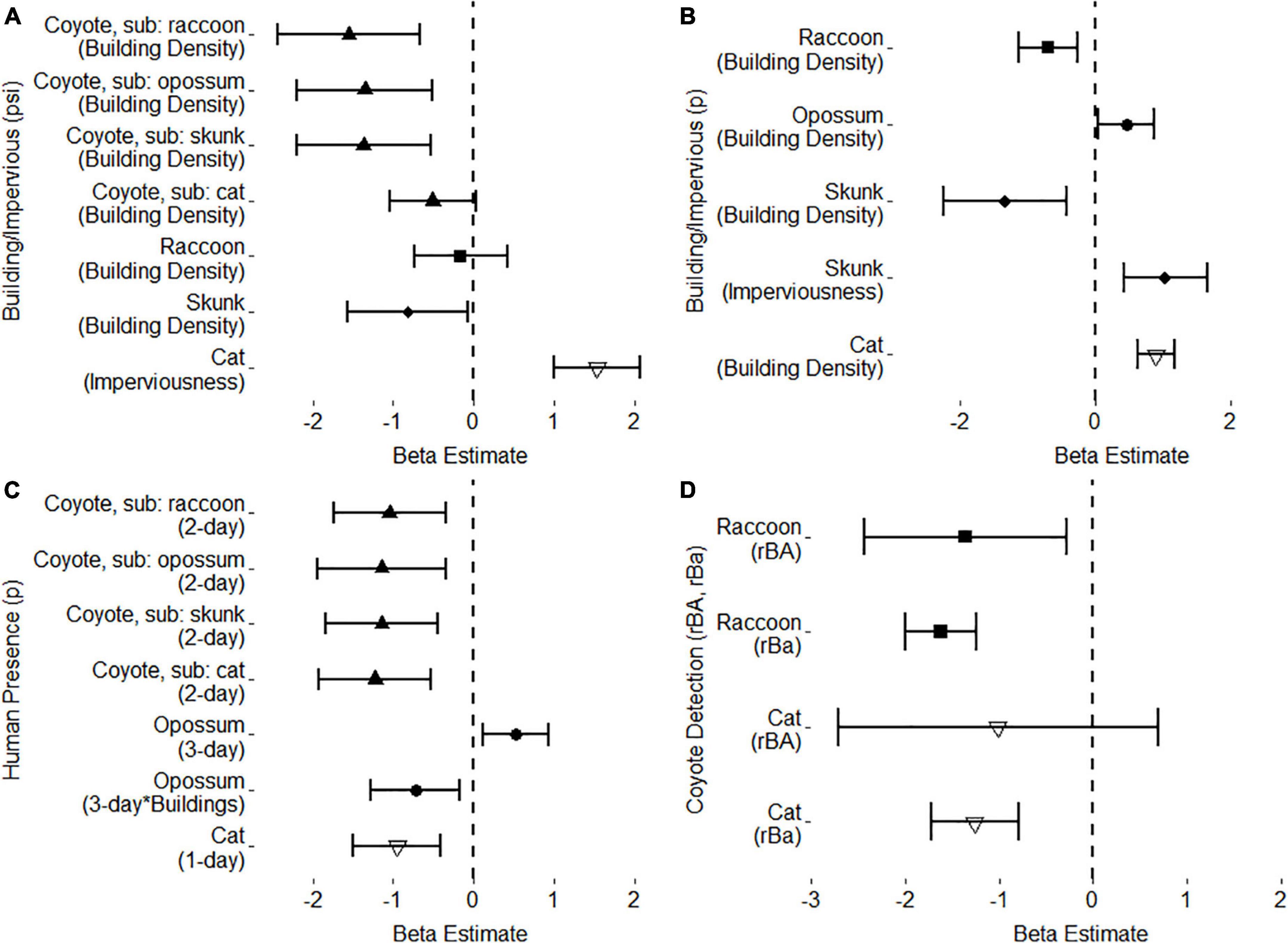
Figure 3. Beta estimates and 95% confidence intervals for top two-species occupancy models. Beta estimates that cross the dashed line at 0 are not considered significant. (A) Top urbanization covariates, building density and imperviousness, for mesopredator occupancy. (B) Top urbanization covariates, building density and imperviousness, for mesopredator detection. (C) Top human presence covariates for mesopredator detection as well as an interaction term for human presence across building density for opossum detection. (D) Raccoon and cat detection variables for when coyotes are present and detected (rBA) and present but not detected (rBa).
Coyotes and Raccoons
The top detection model for coyotes and raccoons determined that building density decreased raccoon detection in the absence of imperviousness. Additionally, Julian date still reduced raccoon detection as the survey season continued. Raccoon detection was considered to be conditional on coyote presence and detection (H2). When coyotes were present and detected (rBA), odds of raccoon detection decreased by 74.3% (β = –1.358, OR = 0.257, OR 95% CI = [0.087, 0.758]); while, when coyotes were present but not detected (rBa), odds of raccoon detection decreased by 80.3% (β = –1.625, OR = 0.197, OR 95% CI = [0.135, 0.287]).
The top occupancy model included building density for raccoon occupancy (ψBa), with raccoon occupancy being conditional on coyote presence (ψBA). However, building density had no impact on raccoon occupancy in the absence of coyotes, and no relationship could be estimated from raccoon occupancy when coyotes were presence (H4, ψBA). Additionally, the SIF estimates were non-conclusive.
Coyotes and Opossums
The top two-species detection model for coyotes and opossums included human presence within a 3-day period, building density at the 100 m kernel density and 1000 m buffer scale, and an interaction term between human presence and building density as covariates for opossum detection (pB), as well as the 3-day lag effect (opo3) to improve model estimation. Opossum detection was considered unconditional on both coyote presence and detection (pB = rBA = rBa). As with single-species occupancy modeling, two-species modeling found that opossum detection increased in response to building density, unless humans were present within a 3-day period; then opossum detection decreased as building density increases. However, at low building density, opossum detection increased when humans were present in a 3-day period.
The top occupancy model did not include any covariates for opossum occupancy (ψBa), and opossum occupancy was considered unconditional on coyote presence (ψBa = ψBA). Thus, opossum occupancy showed high variability and no trend when coyotes were present. As opossum and coyote occupancy was unconditional, no SIF could be estimated.
Coyotes and Striped Skunks
Two-species modeling provided similar estimation for skunk detection variables compared to single-species top models. Skunk detection decreased as a result of building density; however, imperviousness increased skunk detection. Skunk detection also increased as a result of Julian date. Skunk detection was considered unconditional on both coyote presence and detection (pB = rBA = rBa).
The top model for occupancy included building density for both coyote (ψA) and skunk occupancy (ψBa). Although single-species models did not find significant trends in coyote occupancy estimation, the two-species model showed building density negatively impacting both species at the same spatial scale. Skunk occupancy was considered conditional upon coyote presence (ψBA ≠ψBa). However, while the conditional top model was selected, parameter estimations for ψBA did not provide any meaningful trend in skunk occupancy when coyotes were present. As with coyotes and raccoons, coyote and skunk SIF showed no significant relationship.
Coyotes and Domestic Cats
The top two-species detection model for coyotes and domestic cats included camera placement, anthropogenic food, bait decay, human presence within a 1-day period and building density as covariates for cat detection (pB), and cat detection being conditional on coyote presence (rBA) and detection (rBa). Estimations of covariates for cat detection in the two-species model were similar to covariates estimates in the single-species model, with food and building density increasing detection while human presence, backyards, greenspaces, and bait decay all decreased cat detection. Although cat detection was considered conditional on coyote presence and detection (H2), the odds of cat detection were only seen to decrease significantly when coyotes were present but not detected (rBa; β = –1.264, OR = 0.282, OR 95% CI = [0.178, 0.449]). Yet, when coyotes were present and detected (rBA), cat detection did not show a significant relationship (β = –1.014, OR = 0.363, OR 95% CI = [0.066, 1.992]).
The top two-species occupancy model for coyotes and domestic cats included imperviousness as a covariate for cat occupancy, while cat occupancy was unconditional upon coyote presence (ψBA = ψBa). Cat occupancy was estimated to have a positive relationship with imperviousness as was shown in the single-species top model. Although coyote occupancy used the same building density at the 200 m kernel density and 500 m buffer size scale as used in previous two-species pairings, the top model for coyote-cat occupancy did not show a significant relationship between coyote occupancy and building density. As coyote-cat occupancy was determined to be unconditional, no SIF could be derived from the top model.
Temporal Overlap
Wild mesopredator species had more detections in non-urban camera sites than low and high intensity urban areas. Mesopredators displayed mainly crepuscular and nocturnal activity across the urbanization gradient with slight variability (Figure 4). Domestic cats were the only mesopredator to vary significantly from non-urban to urban camera sites, both in number of detections and activity patterns (H1). Humans remained mostly diurnal across the urbanization gradient; however, shifts in both range and peaks of activity occurred as urbanization intensity increased. This shift in human activity between non-urban and urban areas is apparent as temporal overlap significantly decreased between non-urban and low intensity sites (D-hat4 = 0.852, 95% CI = [0.776, 0.922]) and non-urban and high intensity urban sites (D-hat4 = 0.696, 95% CI = [0.630, 0.760]). Intraspecies temporal overlap was consistently high for wild mesopredators across the urban gradient, with no significant change in overlap between urban gradients (H1).
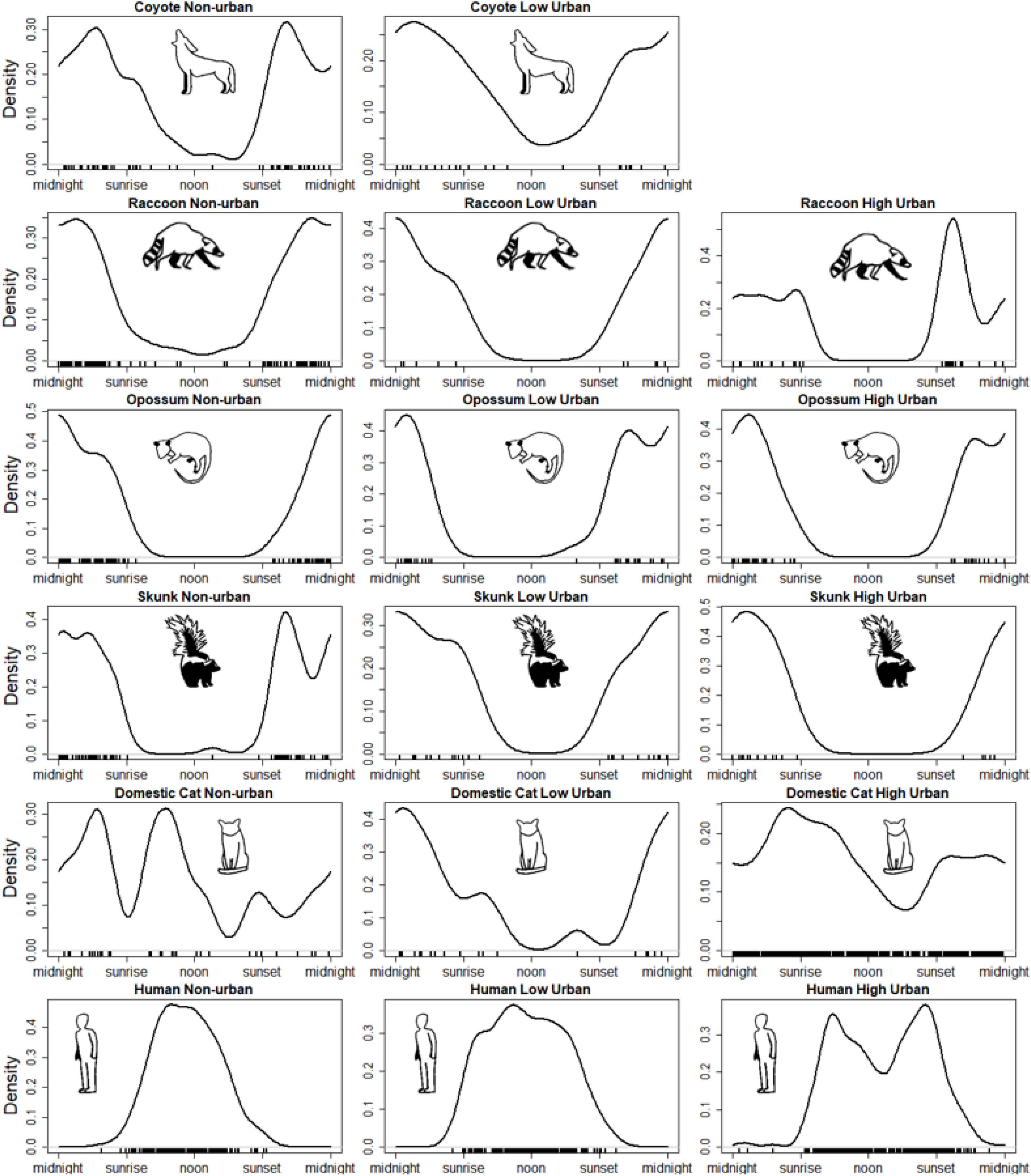
Figure 4. Temporal activity patterns for species across urban intensity groups (non-urban, low intensity urban, high intensity urban). Plots are scaled from a 24-h clock to sun-time to account for daylight from sunrise to sunset. Black tick marks at the bottom of each plot indicate when species were detected.
Coyotes were only detected once at a high intensity urban camera (a mother and juvenile pair at 10:33PM in an urban resident’s backyard) and thus their temporal activity could not be compared for the high intensity urban areas. Coyote activity did not change substantially between non-urban areas and low intensity urban areas, and temporal overlap between coyotes in these two areas remained relatively high. Although coyotes displayed some increased diurnal activity compared to other mesopredators in low intensity urban areas, temporal overlap with all mesopredator species across non-urban and low intensity urban sites remained high (H2).
Raccoons had a majority of their detections in non-urban and high intensity urban areas, while having the fewest detections in low intensity urban areas. Raccoon activity, while exhibiting more diurnal detections in non-urban areas, did not change significantly across the urbanization gradient. Raccoon overlap with wild mesopredator species was consistently high across the urbanization gradient and did not change significantly. Alternatively, raccoons had consistently low overlap values with humans across all three urbanization levels (H3).
Like raccoons, opossums had the highest detections in non-urban and high intensity urban areas compared to low intensity urban areas. Opossum were the only wild mesopredator to have their activity patterns differ between non-urban and high intensity urban areas (AD = 2.786, T.AD = 2.376, p = 0.034, α = 0.05), likely due to a shift to more nocturnal activity in high intensity urban areas. While opossum activity did shift, intraspecies temporal overlap remained high between all three pairings of urban intensities. Opossum overlap with humans remained low across urbanization levels (H3).
Striped skunks had the most detections in non-urban, followed by low intensity urban areas, and the least detection in high intensity urban areas. Skunk activity was consistently crepuscular and nocturnal across the urbanization gradient. Because of this, skunk intraspecies temporal overlap was consistently high across the urbanization gradient. Skunks did significantly increase temporal overlap with humans between non-urban (D-hat4 = 0.060, 95% CI = [0.018, 0.108]) and low intensity urban areas (D-hat1 = 0.227, 95% CI = [0.117, 0.338]; H4, Table 1, Figure 5).
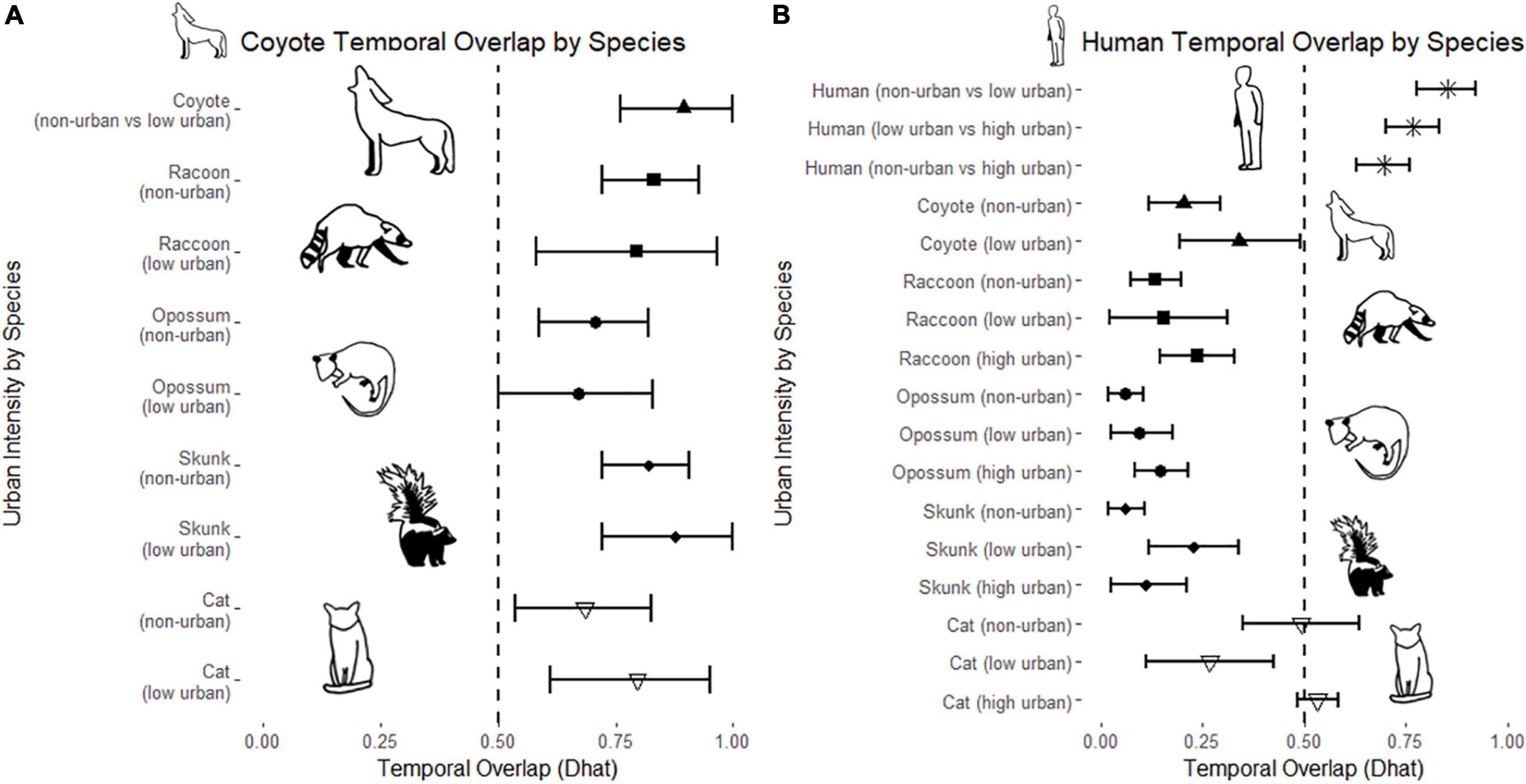
Figure 5. Temporal overlap (Dhat) estimates for (A) coyotes and all subordinate mesopredators and (B) humans and all mesopredators across non-urban, low urban, and high urban intensities. 95% confidence intervals are derived from 10,000 bootstrap analyzes. Dashed line at 0.50 represents a visual marker for temporal overlap estimates where species share at least 50% temporal activity.
Domestic cat detections increased substantially in the high intensity urban areas compared to non-urban and low intensity urban sites. Domestic cats had highly variable activity patterns across each of the three urban intensities (H1); having peaks of nocturnal, diurnal, and crepuscular activity in non-urban areas, mainly nocturnal activity in low intensity urban areas, and a strong shift from early morning to afternoon activity followed by a nocturnal peak following sunset in high intensity urban areas. Because of this, cat activity in non-urban areas was considered significantly different from activity in both low intensity (AD = 5.313, T.AD = 5.737, p = 0.002, α = 0.05) and high intensity urban areas (AD = 2.772, T.AD = 2.332, p = 0.036, α = 0.05). While activity patterns differed between non-urban and urban areas, intraspecies temporal overlap for cats remained relatively high for all mesopredator species. Additionally, cat temporal overlap with humans was significantly lower at low intensity urban sites (D-hat1 = 0.266, 95% CI = [0.112, 0.426]) versus high intensity urban sites (D-hat4 = 0.533, 95% CI = [0.485, 0.583]; H4, Table 1, Figure 5). All model estimates can be viewed in Supplementary datasheet 1.
Discussion
While mammalian mesopredators were observed across the Sacramento Metropolitan Area, their responses to urban intensity, human presence, and coyote presence were complex (Figure 6). We found that urban intensity (H1) and human presence (H3) had more of an impact on small mesopredator activity than coyote presence (H2), similar to previous studies (Lesmeister et al., 2015; Moll et al., 2018; Nickel et al., 2020). We found that in general, coyotes, raccoons, and striped skunks all had some evidence of avoidance of high urban intensity while opossums and domestic cats were more likely to be attracted to urban areas. Raccoons and cats were the only mesopredators to display some evidence for avoidance of coyotes (H2). Coyotes, cats, and opossums were all found to avoid humans (H3). Opossums displayed the most evidence of urban intensity mediating the risk of humans (H4); although, skunks and cats displayed some increase in temporal overlap with humans across urban intensities as well. Finally, urban intensity did not impact mesopredators’ relationships with coyotes (H4).
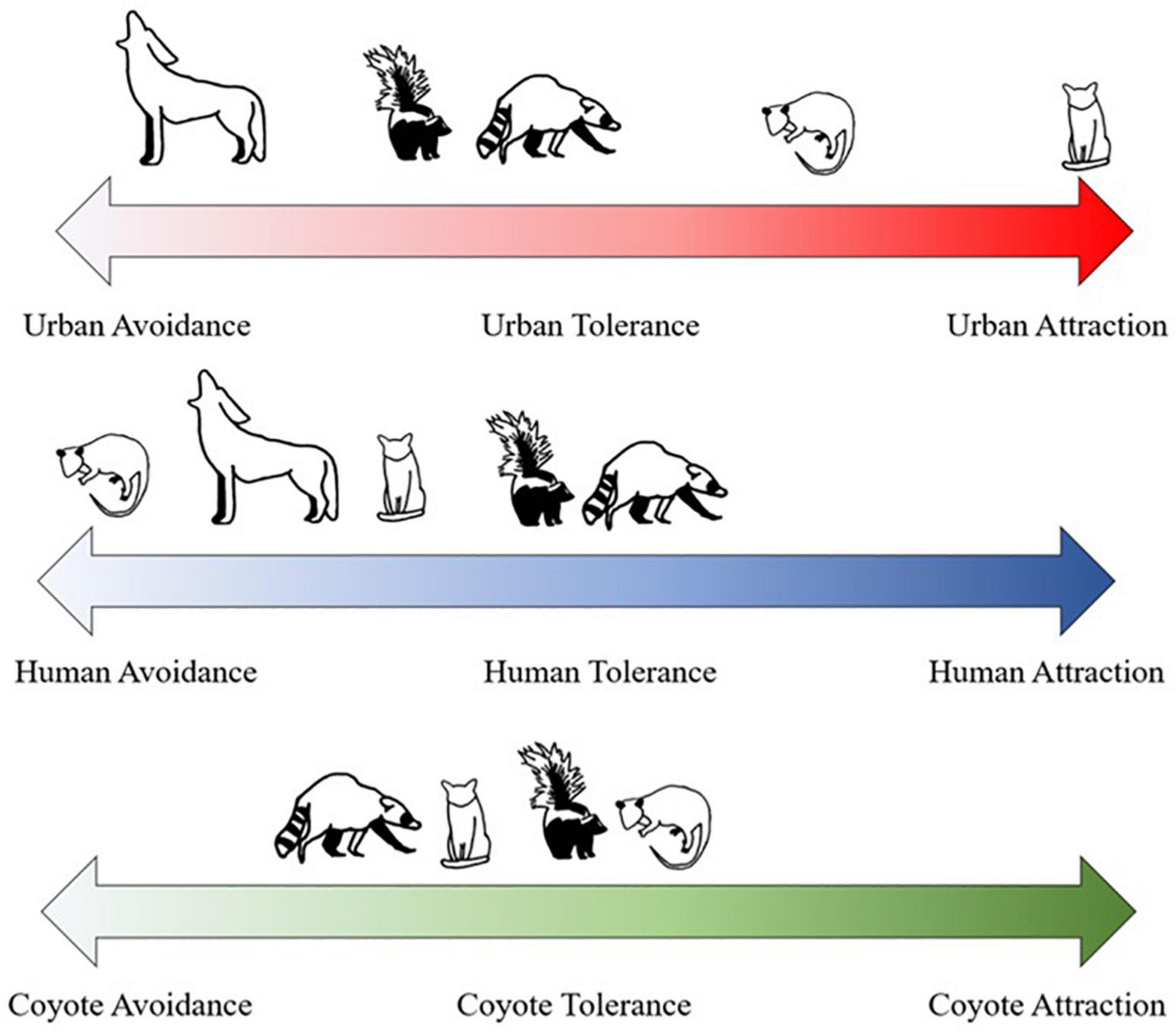
Figure 6. Mammalian mesopredator (coyote, raccoon, opossum, striped skunk, and domestic cat) responses to urban areas, humans, and coyotes. Species fall along a spectrum of either avoidance, or attraction for each stressor, with area in the middle representing a general tolerance.
We found that small mesopredators did not respond to coyote presence at the 1-day to 3-day scale and showed high interspecific temporal overlap with coyotes across the urban gradient. Instead, evidence of direct coyote avoidance was limited to predictions based on two-species conditional models for two mesopredators, raccoon, and domestic cat. Even so, raccoons and cats may only spatiotemporally partition from coyotes at a limited scale, as top models for these species predicted negative trends in species detection rather than area use.
Evidence from top occupancy models indicate that some mesopredators avoid humans across the urban gradient, which is consistent with humans being considered influential super predators (Ditchkoff et al., 2006; Darimont et al., 2015). In single and two-species models, we found that coyotes and domestic cats avoided humans within a one to 2-day period, while opossums were more likely to avoid humans within a 3-day period as building density increased. However, temporal overlap showed that wild mesopredators were primarily nocturnal, rarely overlapping with humans across the urban gradient. Skunks were the only wild mesopredator to overlap more with humans in low intensity urban areas compared to non-urban areas, yet overlap fell again in high intensity urban areas. It may be that skunk and other wild mesopredators are not making substantial changes to their overall nocturnal activity in respond to humans, but instead alter the timing and frequency of using areas depending on human activity (Suraci et al., 2019). In contrast, mesopredators may not perceive coyotes as a threat worth altering their activity (Gipson et al., 2003; Prange and Gehrt, 2007; Chitwood et al., 2020).
Mesopredator urban land use, detection, and overall activity levels were sensitive to changes in urban features at varying spatial scales, which is consistent with current research of urban mammals (Fidino et al., 2020; Moll et al., 2020). For wild mesopredators, building density was a better predictor of urban intensity than imperviousness. Building density was consistently in the top models for all mesopredators, and was more consistent in its estimation between model types than imperviousness. Building density had mostly negative effects on mesopredator occupancy and detection, except for opossums and cats, which saw positive influences on detection. Interestingly, while these two species were attracted to higher building density, they both had negative associations with human presence. Potentially, mesopredators might be responding to human activity and resources near residences in urban areas, as areas of high density buildings and housing may offer greater risk and reward to mesopredators rather than apparent concrete cover (Fidino et al., 2020). Furthermore, only two mesopredators responded positively to imperviousness, the striped skunk and domestic cat. Indeed, mesopredators may have different thresholds of tolerance for urban areas and features at, and our study supports findings that using multiple predictors at multiple spatial scales enhances overall model performance and interpretation (Moll et al., 2019).
Additionally, mesopredator detection varied due to camera placement and study design elements. Seasonality had some effect on our mesopredator detection, where raccoon and opossum detection decreased and coyote and skunk detection increased as the survey period progressed. This may be due to general trends in dispersal and maternal care of young as well as increases in temperature that may have limited detections of scavenging raccoons and opossums (DeVault et al., 2011). Interestingly, bait decay had minimal effects on reducing coyote and domestic cat detection, potentially due to coyote having larger home ranges to search for food resources, while cats may have been drawn to a known food cue. Domestic cats were also the only mesopredator to respond to anthropogenic food on site; however, this result may be conflated due to the presence of feeding stations specifically for feral cat colonies at urban parks or pet cats near homes. Finally, coyote, raccoon, and cat detection were all impacted by camera placement. Unfortunately, fencing in backyards may have been impermeable or a deterrence to mesopredators, especially coyotes, which were only observed in large backyards (n = 3) that shared fencing with connected greenspace. Yet, coyotes were surprisingly not detected by any front yard cameras, even with relatively low fencing or access to greenspace nearby. While the features of backyard and front yard sites such as size, landscaping, and fencing were highly variable, mesopredators activity may be more representative of the surrounding landscape features and level of urbanization in which the yards occurred (Parsons et al., 2019; Grade et al., 2022).
Coyotes as Intraguild Predators
Coyotes’ role as intraguild predators may be limited for non-canid species, especially in human-dominated landscapes (Gehrt and Clark, 2003). This may be the result of urban and working lands providing a plethora of food resources, decreasing the potential for competition over shared prey or food (Holt and Huxel, 2007; Morey et al., 2007). For example, previous studies of sympatric coyote and raccoon interactions suggests that intraguild predation is rare between raccoons and coyotes and that raccoons do not spatiotemporally avoid coyotes when foraging (Gehrt and Prange, 2007; Chitwood et al., 2020). Yet, we found a negative response in raccoon detection due to coyote presence, indicating some degree of avoidance. This relationship may be due to seasonal risks of juvenile raccoons leaving their natal dens, as this period may be their biggest risk in mortality (Cowan, 1973). However, while raccoons may avoid coyotes during this time, other mesopredators, such as skunks and opossums may avoid coyote conflict through either defensive or deterrence behaviors (Gabrielsen and Smith, 1985; Larivière and Messier, 1996). Coyotes may instead be limiting the distribution of two smaller canid mesopredators, the gray and red fox. Foxes were only found at three camera sites (two urban parks, and one working farm) where coyotes were absent. Potentially urban areas, and human presence, may facilitate small populations of these foxes in absence of coyotes (Lewis et al., 1999; Lombardi et al., 2017; Mueller et al., 2018).
While raccoons, skunks, and opossum are rare prey for coyotes, domestic cats are more likely to be depredated on by coyotes (Grubbs and Krausman, 2009). However, we found little support to conclude that domestic cats are altering their spatiotemporal activity due to coyotes. For instance, we found cat detection was lower when coyotes were using an area, but not detected (rBa) compared to when coyotes were both present and detected (rBA). This may be as result of low sample size, as both species were detected together at only four camera sites and only twice within the same day. Of those shared sites, two were in non-urban and low intensity urban areas where cats returned four to 5 days following coyotes, while at the other two sites (an exurban and high intensity urban site) cats returned within 1 day of coyote detection. Thus, urban cats may be less vigilant or fearful of coyotes and more focused on humans, which has been shown with other coyote sympatric prey species such as deer and rabbits in urban areas (Gallo et al., 2019). Thus, we suspected that coyotes could be disproportionately impacting the spatiotemporal activity of cats in non-urban and low human density areas, as other studies suggest (Gehrt et al., 2013; Breck et al., 2019).
Human Presence and Risk Across the Urban Gradient
The influence of humans was variable across the mesopredator community. To start, coyotes and domestic cats had the clearest avoidance of areas when human were present, regardless of urban influences. Coyote avoidance of humans is unsurprising, as humans often haze, trap, or kill coyotes due to being viewed as dangerous predators of domestic animals and children (Kitchen et al., 2000; Fox, 2006). However, coyote avoidance of humans was most influential at the 2-day period rather than the maximum 3-day period, suggesting that coyotes will return to areas humans use relatively quickly. Domestic cats, on the other hand, avoided areas when humans were present within a day. This may be a result of both pet cats avoiding humans when outside to mark and survey their territory momentarily before returning home and feral cats avoiding humans while still accepting regular food subsidies. The differences between pet and feral cat activity may have influenced overall temporal overlap with humans, especially in high intensity urban areas. Feral cat nocturnal activity may be driving temporally avoidance of humans, while pet cats increase apparent temporal overlap due to diurnal outdoor activity (Gehrt et al., 2013; Loyd et al., 2013).
Opossums represented the only small wild mesopredator to respond to human presence. However, unlike coyotes and cats, opossum detection decreased with human presence across a gradient of building density. Surprisingly, opossums also had the greatest intolerance for human presence, showing a decrease in activity when humans were present within the maximum 3-day period. Thus, while opossums may benefit from high intensity urban areas as scavenging and food resources increases, increased human density and presence may represent a threat to these often slow moving, shy omnivores (Beatty et al., 2013).
While we were only able to investigate how human presence impacted mesopredators temporally due to complete occupancy of humans at camera sites, this is representative of our study area representing high human impact, even in natural areas (Leu et al., 2008). However, we were not able to quantify the density of humans beyond the camera’s view, especially as our camera placement was biased toward secretive placement rather than being out in the open. Therefore, a negative response of striped skunk and raccoons may not have been captured by the scale of our human presence variable even though studies have shown that areas with high human presence may still impact mesopredators, such as striped skunks (Nickel et al., 2020). Additionally, while temporal overlap analyzes did not capture significant differences between mesopredator and human overlap across the urban gradient, we did see a general trend in reduced crepuscular and diurnal detections as urban intensity increased. Indeed, even in our non-urban areas, human activity and density was at elevated levels compared to many other non-urban areas throughout our mesopredators range (Leu et al., 2008). Likely, increased human presence and density may alter mesopredator temporal activity to be more nocturnal, especially for more non-urban populations. (Wang et al., 2015; Reilly et al., 2017; Gaynor et al., 2018).
Management Implications
Due to the potentially limited role of coyotes as an intraguild predator in urban areas, managers should not rely on coyote presence as an indicator of reduced mesopredator presence or impacts, especially for those trying to reduce depredation of endangered or threatened species by mesopredators. For managers looking to reduce mesopredator activity in sensitive areas, eliminating or fencing areas where mesopredators may easily access food or denning sites may be the best ways to mitigate against the negative impacts of small urban mesopredators (Gehrt et al., 2010; Beatty et al., 2013; Theimer et al., 2015). We found that high building density and residential yards generally reduced mesopredator detection, while greenspaces increased detection especially for wild mesopredators. For urban land managers, enhancing backyard habitat in low intensity urban areas may facilitate movement of mammalian mesopredators, including coyotes, increasing gene flow and biodiversity of mammal species (Rudd et al., 2002; Adducci et al., 2020; Grade et al., 2022).
We found that human presence may negatively impact some mesopredators’ detectability, especially for coyotes. Managers seeking to reduce negative human-coyote interactions should target their efforts to greenspaces in close proximity to urban areas and less building dense communities around wildland-urban interface boundaries where coyotes are more likely to be active (Poessel et al., 2013; Lombardi et al., 2017). While we found that coyotes may avoid humans within a 2-day period, we cannot account for the individual behaviors and risk factors that some coyotes may take. Coyotes in urban areas may be more likely to go after riskier food sources, such as pets, especially if they are suffering from debilitating health impacts and diseases such as mange (Murray et al., 2015). Therefore, enhancing wildlife habitat around and through urban and agricultural areas where conflict is greatest may allow coyotes better forage opportunities, increasing time spent in these areas rather than near humans (Magle et al., 2014).
Conclusion
Small mesopredators display high spatiotemporal overlap with coyotes, suggesting that intraguild predation risk from coyotes does not greatly influence mesopredator activity across human-dominated landscapes. Meanwhile, urbanization both attracts mesopredators while also being a constant source of risk due to increased human presence (Tigas et al., 2002; Welch et al., 2017; Gaynor et al., 2018). As studies on urban mesopredators are becoming more common, our results suggest that research should more closely address how fine-scale movement and microhabitat use facilitate mesopredator access and connectivity across different levels of urban intensities. Additionally, urban ecologists should investigate the role behavioral plasticity plays in mesopredators risk assessment, especially for different types of human activities. Human presence may influence even the most “urban adapted” species and play more of a role in shaping species interactions than potential intraguild predators.
Data Availability Statement
The raw data supporting the conclusions of this article will be made available by the authors, without undue reservation.
Ethics Statement
The animal study was reviewed and approved by the Humboldt State University Institutional Animal Care and Use Committee (IACUC), protocol # 08 16.17.W.08-A.
Author Contributions
CM contributed to the study design, field work, analysis, and writing of the research and final manuscript. BC contributed to the study design, coordination, guidance, grant acquisition, editing, comments, and final approval of the research and manuscript. BF contributed to the guidance, grant acquisition, editing, comments, and statistical support for the research and manuscript. All authors contributed to the article and approved the submitted version.
Funding
Funding for this project was provided by the California Department of Fish and Wildlife, and Humboldt State University (now California State Polytechnic University, Humboldt).
Conflict of Interest
The authors declare that the research was conducted in the absence of any commercial or financial relationships that could be construed as a potential conflict of interest.
Publisher’s Note
All claims expressed in this article are solely those of the authors and do not necessarily represent those of their affiliated organizations, or those of the publisher, the editors and the reviewers. Any product that may be evaluated in this article, or claim that may be made by its manufacturer, is not guaranteed or endorsed by the publisher.
Acknowledgments
We would like to acknowledge the generosity of all of the residents and landowners who made this study possible. Additionally, we greatly appreciate the time and work Misty Nelson placed into coordinating the access to geospatial and landowner contact information that was paramount to making this study a reality. We would like to thank Angela Baker and Daniel Barton for contributions to insight and comments while this study was still in its development phase. Much gratitude is owed to the technicians who helped set-up, collect, sort, and analyze data, including Anjelica Yee, Trevor McDowell, Sara Moriarty-Graves, Ximena Gil, and Chan Saeteurn.
Supplementary Material
The Supplementary Material for this article can be found online at: https://www.frontiersin.org/articles/10.3389/fevo.2022.867188/full#supplementary-material
Footnotes
- ^ https://github.com/Microsoft/USBuildingFootprints
- ^ https://www.mbr-pwrc.usgs.gov/software/presence.html
References
Alberti, M., Botsford, E., and Cohen, A. (2001). “Quantifying the urban gradient: linking urban planning and ecology,” in Avian Ecology and Conservation in an Urbanizing World, eds J. M. Marzluff, R. Bowman, and R. Donnelly (Boston, MA: Springer US), 89–115. doi: 10.1007/978-1-4615-1531-9_5
Adducci, A., Jasperse, J., Riley, S., Brown, J., Honeycutt, R., and Monzón, J. (2020). Urban coyotes are genetically distinct from coyotes in natural habitats. J. Urban Ecol. 6:juaa010. doi: 10.1093/jue/juaa010
Anderson, D. R., and Burnham, K. P. (2002). Avoiding pitfalls when using information-theoretic methods. J. Wildl. Manag. 66, 912–918. doi: 10.2307/3803155
Baker, P. J., Dowding, C. V., Molony, S. E., White, P. C. L., and Harris, S. (2007). Activity patterns of urban red foxes (Vulpes vulpes) reduce the risk of traffic-induced mortality. Behav. Ecol. 18, 716–724. doi: 10.1093/beheco/arm035
Bateman, P. W., and Fleming, P. A. (2012). Big city life: carnivores in urban environments. J. Zool. 287, 1–23. doi: 10.1111/j.1469-7998.2011.00887.x
Beatty, W. S., Beasley, J. C., and Rhodes, O. E. (2013). Habitat selection by a generalist mesopredator near its historical range boundary. Can. J. Zool. 92, 41–48. doi: 10.1139/cjz-2013-0225
Bivand, R., Keitt, T., Rowlingson, B., Pebesma, E., Sumner, M., Baston, D., et al. (2020). Package “rgdal” Bindings for the Geospatial Data Abstraction Library. Available online at: http://rgdal.r-forge.r-project.org
Breck, S. W., Poessel, S. A., Mahoney, P., and Young, J. K. (2019). The intrepid urban coyote: a comparison of bold and exploratory behavior in coyotes from urban and rural environments. Sci. Rep. 9:2104. doi: 10.1038/s41598-019-38543-5
Brown, J. H., and Maurer, B. A. (1986). Body size, ecological dominance and Cope’s rule. Nature 324, 248–250. doi: 10.1038/324248a0
Cade, B. S. (2015). Model averaging and muddled multimodel inferences. Ecology 96, 2370–2382. doi: 10.1890/14-1639.1
U. S. Census Bureau (2019). City and Town Population Totals: 2010-2019. Available online at: https://www.census.gov/data/tables/time-series/demo/popest/2010s-total-cities-and-towns.html (Accessed November 28, 2020).
Chitwood, M. C., Lashley, M. A., Higdon, S. D., DePerno, C. S., and Moorman, C. E. (2020). Raccoon vigilance and activity patterns when sympatric with coyotes. Diversity 12:341. doi: 10.3390/d12090341
Clinchy, M., Zanette, L. Y., Roberts, D., Suraci, J. P., Buesching, C. D., Newman, C., et al. (2016). Fear of the human “super predator” far exceeds the fear of large carnivores in a model mesocarnivore. Behav. Ecol. 27, 1826–1832. doi: 10.1093/beheco/arw117
Cowan, W. F. (1973). Ecology and Life History of the Raccoon (Procyon lotor hirtus Nelson and Goldman) in the Northern Part of Its Range. (Ph.D. thesis). Grand Forks, ND: The University of North Dakota.
Darimont, C. T., Fox, C. H., Bryan, H. M., and Reimchen, T. E. (2015). The unique ecology of human predators. Science 349, 858–860. doi: 10.1126/science.aac4249
DeVault, T. L., Olson, Z. H., Beasley, J. C., and Rhodes, O. E. (2011). Mesopredators dominate competition for carrion in an agricultural landscape. Basic Appl. Ecol. 12, 268–274. doi: 10.1016/j.baae.2011.02.008
Ditchkoff, S. S., Saalfeld, S. T., and Gibson, C. J. (2006). Animal behavior in urban ecosystems: modifications due to human-induced stress. Urban Ecosyst. 9, 5–12. doi: 10.1007/s11252-006-3262-3
Durand, J. R., Bombardelli, F., Fleenor, W. E., Henneberry, Y., Herman, J., Jeffres, C., et al. (2020). Drought and the Sacramento-San Joaquin Delta, 2012–2016: environmental review and lessons. San Franc. Estuary Watershed Sci. 18, 1–26. doi: 10.15447/sfews.2020v18iss2art2
Falcone, J. A. (2017). Coefficient-Based Consistent Mapping of Imperviousness in the Conterminous U.S. at 60-m Resolution for 1974, 1982, 1992, 2002, and 2012. U.S. Geological Survey Data Release. Reston, VA: U.S. Geological Survey. doi: 10.5066/F72F7KMG
Fidino, M., Gallo, T., Lehrer, E. W., Murray, M. H., Kay, C., Sander, H. A., et al. (2020). Landscape-scale differences among cities alter common species’ responses to urbanization. Ecol. Appl. 31:e02253. doi: 10.1002/eap.2253
Fiske, I., and Chandler, R. (2011). Unmarked: an R package for fitting hierarchical models of wildlife occurrence and abundance. J. Stat. Softw. 43, 1–23. doi: 10.18637/jss.v043.i10
Fox, C. H. (2006). “Coyotes and humans: can we coexist?,” in Proceedings of the 22nd Vertebrate Pest Conference, eds R. M. Timm and J. M. O’Brien (Davis, CA: University of California), doi: 10.5070/V422110104
Gabrielsen, G. W., and Smith, E. N. (1985). Physiological responses associated with feigned death in the American opossum. Acta Physiol. Scand. 123, 393–398. doi: 10.1111/j.1748-1716.1985.tb07605.x
Gallo, T., Fidino, M., Lehrer, E. W., and Magle, S. (2019). Urbanization alters predator-avoidance behaviours. J. Anim. Ecol. 88, 793–803. doi: 10.1111/1365-2656.12967
Gaynor, K. M., Hojnowski, C. E., Carter, N. H., and Brashares, J. S. (2018). The influence of human disturbance on wildlife nocturnality. Science 360, 1232–1235. doi: 10.1126/science.aar7121
Gehring, T. M., and Swihart, R. K. (2003). Body size, niche breadth, and ecologically scaled responses to habitat fragmentation: mammalian predators in an agricultural landscape. Biol. Conserv. 109, 283–295. doi: 10.1016/S0006-3207(02)00156-8
Gehrt, S. D. (2004). “Ecology and management of striped skunks, raccoons, and coyotes in urban landscapes,” in People and Predators: From Conflict to Coexistence, eds N. Fascione, A. Delach, and M. Smith (Washington, DC: Island Press), 81–104.
Gehrt, S. D., and Clark, W. R. (2003). Raccoons, coyotes, and reflections on the mesopredator release hypothesis. Wildl. Soc. Bull. 31, 836–842.
Gehrt, S. D., and Prange, S. (2007). Interference competition between coyotes and raccoons: a test of the mesopredator release hypothesis. Behav. Ecol. 18, 204–214. doi: 10.1093/beheco/arl075
Gehrt, S. D., Riley, S. P. D., and Cypher, B. L. (2010). Urban Carnivores: Ecology, Conflict, and Conservation. Baltimore, MD: JHU Press.
Gehrt, S. D., Wilson, E. C., Brown, J. L., and Anchor, C. (2013). Population ecology of free-roaming cats and interference competition by coyotes in urban parks. PLoS One 8:e75718. doi: 10.1371/journal.pone.0075718
Gipson, P. S., Livingston, T. R., Zuercher, G. L., and Howard, M. E. (2003). Responses of opossums and raccoons to bobcat and coyote feces. West. North Am. Nat. 63, 538–540.
Grade, A. M., Warren, P. S., and Lerman, S. B. (2022). Managing yards for mammals: mammal species richness peaks in the suburbs. Landsc. Urban Plan. 220:104337. doi: 10.1016/j.landurbplan.2021.104337
Gosselink, T. E., Van Deelen, T. R., Warner, R. E., and Joselyn, M. G. (2003). Temporal habitat partitioning and spatial use of coyotes and red foxes in East-Central Illinois. J. Wildl. Manag. 67, 90–103. doi: 10.2307/3803065
Grubbs, S. E., and Krausman, P. R. (2009). Observations of coyote-cat interactions. J. Wildl. Manag. 73, 683–685. doi: 10.2193/2008-033
Hijmans, R., van Etten, J., Sumner, M., Chang, J., Baston, D., Bevan, A., et al. (2020). Package “raster” Geographic Data Analysis and Modeling. Available online at: https://rspatial.org/raster
Holt, R. D., and Huxel, G. R. (2007). Alternative prey and the dynamics of intraguild predation: theoretical Perspectives. Ecology 88, 2706–2712. doi: 10.1890/06-1525.1
Kitchen, A. M., Gese, E. M., and Schauster, E. R. (1999). Resource partitioning between coyotes and swift foxes: space, time, and diet. Can. J. Zool. 77, 1645–1656. doi: 10.1139/z99-143
Kitchen, A. M., Gese, E. M., and Schauster, E. R. (2000). Changes in coyote activity patterns due to reduced exposure to human persecution. Can. J. Zool. 78, 853–857. doi: 10.1139/z00-003
Kozlowski, A. J., Gese, E. M., and Arjo, W. M. (2008). Niche overlap and resource partitioning between sympatric kit foxes and coyotes in the Great Basin Desert of western Utah. Am. Midl. Nat. 160, 191–208.
Larivière, S., and Messier, F. (1996). Aposematic behaviour in the striped skunk, Mephitis mephitis. Ethology 102, 986–992. doi: 10.1111/j.1439-0310.1996.tb01176.x
Lesmeister, D. B., Nielsen, C. K., Schauber, E. M., and Hellgren, E. C. (2015). Spatial and temporal structure of a mesocarnivore guild in midwestern north America. Wildl. Monogr. 191, 1–61. doi: 10.1002/wmon.1015
Leu, M., Hanser, S. E., and Knick, S. T. (2008). The human footprint in the west: a large-scale analysis of anthropogenic impacts. Ecol. Appl. 18, 1119–1139. doi: 10.1890/07-0480.1
Lewis, J. C., Sallee, K. L., and Golightly, R. T. (1999). Introduction and range expansion of nonnative red foxes (Vulpes vulpes) in California. Am. Midl. Nat. 142, 372–381.
Lombardi, J. V., Comer, C. E., Scognamillo, D. G., and Conway, W. C. (2017). Coyote, fox, and bobcat response to anthropogenic and natural landscape features in a small urban area. Urban Ecosyst. 20, 1239–1248. doi: 10.1007/s11252-017-0676-z
Loyd, K. A. T., Hernandez, S. M., Carroll, J. P., Abernathy, K. J., and Marshall, G. J. (2013). Quantifying free-roaming domestic cat predation using animal-borne video cameras. Biol. Conserv. 160, 183–189. doi: 10.1016/j.biocon.2013.01.008
MacKenzie, D. I., and Bailey, L. L. (2004). Assessing the fit of site-occupancy models. J. Agric. Biol. Environ. Stat. 9, 300–318. doi: 10.1198/108571104X3361
MacKenzie, D. I., Nichols, J. D., Royle, J. A., Pollock, K. H., Bailey, L., and Hines, J. E. (2017). Occupancy Estimation and Modeling: Inferring Patterns and Dynamics of Species Occurrence. Amsterdam: Elsevier.
Magle, S. B., Poessel, S. A., Crooks, K. R., and Breck, S. W. (2014). More dogs less bite: the relationship between human–coyote conflict and prairie dog colonies in an urban landscape. Landsc. Urban Plan. 127, 146–153. doi: 10.1016/j.landurbplan.2014.04.013
Mazerolle, M. (2020). Package “AICmodavg” Model Selection and Multimodel Inference Based on (Q)AIC(c). Available online at: https://cran.r-project.org/package=AICcmodavg
Meredith, M., and Ridout, M. (2014). Overview of the Overlap Package. Available online at: https://cran.r-project.org/web/packages/overlap/index.html
Moll, R. J., Cepek, J. D., Lorch, P. D., Dennis, P. M., Robison, T., Millspaugh, J. J., et al. (2018). Humans and urban development mediate the sympatry of competing carnivores. Urban Ecosyst. 21, 765–778. doi: 10.1007/s11252-018-0758-6
Moll, R. J., Cepek, J. D., Lorch, P. D., Dennis, P. M., Robison, T., and Montgomery, R. A. (2020). At what spatial scale(s) do mammals respond to urbanization? Ecography 43, 171–183. doi: 10.1111/ecog.04762
Moll, R. J., Cepek, J. D., Lorch, P. D., Dennis, P. M., Tans, E., Robison, T., et al. (2019). What does urbanization actually mean? A framework for urban metrics in wildlife research. J. Appl. Ecol. 56, 1289–1300. doi: 10.1111/1365-2664.13358
Morey, P. S., Gese, E. M., and Gehrt, S. (2007). Spatial and temporal variation in the diet of coyotes in the Chicago Metropolitan Area. Am. Midl. Nat. 158, 147–161.
Mueller, M. A., Drake, D., and Allen, M. L. (2018). Coexistence of coyotes (Canis latrans) and red foxes (Vulpes vulpes) in an urban landscape. PLoS One 13:e0190971. doi: 10.1371/journal.pone.0190971
Murray, M., Edwards, M. A., Abercrombie, B., and Clair, C. C. S. (2015). Poor health is associated with use of anthropogenic resources in an urban carnivore. Proc. Biol. Sci. 282:20150009. doi: 10.1098/rspb.2015.0009
Nickel, B. A., Suraci, J. P., Allen, M. L., and Wilmers, C. C. (2020). Human presence and human footprint have non-equivalent effects on wildlife spatiotemporal habitat use. Biol. Conserv. 241:108383. doi: 10.1016/j.biocon.2019.108383
Niedballa, J., Sollmann, R., Courtiol, A., and Wilting, A. (2016). camtrapR: an R package for efficient camera trap data management. Methods Ecol. Evol. 7, 1457–1462. doi: 10.1111/2041-210X.12600
Nouvellet, P., Rasmussen, G. S. A., Macdonald, D. W., and Courchamp, F. (2012). Noisy clocks and silent sunrises: measurement methods of daily activity pattern. J. Zool. 286, 179–184. doi: 10.1111/j.1469-7998.2011.00864.x
Ordeñana, M. A., Crooks, K. R., Boydston, E. E., Fisher, R. N., Lyren, L. M., Siudyla, S., et al. (2010). Effects of urbanization on carnivore species distribution and richness. J. Mammal. 91, 1322–1331. doi: 10.1644/09-MAMM-A-312.1
Parsons, A. W., Rota, C. T., Forrester, T., Baker-Whatton, M. C., McShea, W. J., Schuttler, S. G., et al. (2019). Urbanization focuses carnivore activity in remaining natural habitats, increasing species interactions. J. Appl. Ecol. 56, 1894–1904. doi: 10.1111/1365-2664.13385
Poessel, S. A., Breck, S. W., Teel, T. L., Shwiff, S., Crooks, K. R., and Angeloni, L. (2013). Patterns of human–coyote conflicts in the Denver Metropolitan Area. J. Wildl. Manag. 77, 297–305. doi: 10.1002/jwmg.454
Prange, S., and Gehrt, S. D. (2004). Changes in mesopredator-community structure in response to urbanization. Can. J. Zool. 82, 1804–1817. doi: 10.1139/z04-179
Prange, S., and Gehrt, S. D. (2007). Response of skunks to a simulated increase in coyote activity. J. Mammal. 88, 1040–1049. doi: 10.1644/06-MAMM-A-236R1.1
Prange, S., Gehrt, S. D., and Wiggers, E. P. (2003). Demographic factors contributing to high raccoon densities in urban landscapes. J. Wildl. Manag. 67:324. doi: 10.2307/3802774
Prugh, L. R., Stoner, C. J., Epps, C. W., Bean, W. T., Ripple, W. J., Laliberte, A. S., et al. (2009). The Rise of the Mesopredator. BioScience 59, 779–791. doi: 10.1525/bio.2009.59.9.9
R Core Team (2014). R: A Language and Environment for Statistical Computing. Vienna: R Foundation for Statistical Computing.
Reilly, M. L., Tobler, M. W., Sonderegger, D. L., and Beier, P. (2017). Spatial and temporal response of wildlife to recreational activities in the San Francisco Bay ecoregion. Biol. Conserv. 207, 117–126. doi: 10.1016/j.biocon.2016.11.003
Rich, L., Furnas, B., Brashares, J., and Beissinger, S. (2018). Evaluating Mammalian Diversity in the Mojave Desert and Great Valley Ecoregions of California Using Camera Trap Surveys. doi: 10.13140/RG.2.2.12861.51688
Richmond, O. M. W., Hines, J. E., and Beissinger, S. R. (2010). Two-species occupancy models: a new parameterization applied to co-occurrence of secretive rails. Ecol. Appl. 20, 2036–2046. doi: 10.1890/09-0470.1
Ridout, M. S., and Linkie, M. (2009). Estimating overlap of daily activity patterns from camera trap data. J. Agric. Biol. Environ. Stat. 14, 322–337. doi: 10.1198/jabes.2009.08038
Riley, S. P. D. (2006). Spatial ecology of bobcats and gray foxes in urban and rural zones of a national park. J. Wildl. Manag. 70, 1425–1435. doi: 10.7589/0090-3558-40.1.11
Rudd, H., Vala, J., and Schaefer, V. (2002). Importance of backyard habitat in a comprehensive biodiversity conservation strategy: a connectivity analysis of urban green spaces. Restor. Ecol. 10, 368–375. doi: 10.1046/j.1526-100X.2002.02041.x
Šálek, M., Drahníková, L., and Tkadlec, E. (2014). Changes in home range sizes and population densities of carnivore species along the natural to urban habitat gradient: carnivores along the natural-urban habitat gradient. Mammal Rev. 45, 1–14. doi: 10.1111/mam.12027
Soulard, C. E., and Wilson, T. S. (2015). Recent Land-Use/Land-Cover Change in the Central California Valley. Available online at: https://pubag.nal.usda.gov/catalog/1229956 (Accessed November 11, 2020).
Suraci, J. P., Smith, J. A., Clinchy, M., Zanette, L. Y., and Wilmers, C. C. (2019). Humans, but not their dogs, displace pumas from their kills: an experimental approach. Sci. Rep. 9:12214. doi: 10.1038/s41598-019-48742-9
Theimer, T. C., Clayton, A. C., Martinez, A., Peterson, D. L., and Bergman, D. L. (2015). Visitation rate and behavior of urban mesocarnivores differs in the presence of two common anthropogenic food sources. Urban Ecosyst. 18, 895–906. doi: 10.1007/s11252-015-0436-x
Thompson, C. M., and Gese, E. M. (2007). Food webs and intraguild predation: community interactions of a native mesocarnivore. Ecology 88, 334–346. doi: 10.1890/0012-9658(2007)88[334:fwaipc]2.0.co;2
Tigas, L. A., Van Vuren, D. H., and Sauvajot, R. M. (2002). Behavioral responses of bobcats and coyotes to habitat fragmentation and corridors in an urban environment. Biol. Conserv. 108, 299–306. doi: 10.1016/S0006-3207(02)00120-9
Venter, O., Sanderson, E. W., Magrach, A., Allan, J. R., Beher, J., Jones, K. R., et al. (2016). Sixteen years of change in the global terrestrial human footprint and implications for biodiversity conservation. Nat. Commun. 7:12558. doi: 10.1038/ncomms12558
Voigt, D. R., and Earle, B. D. (1983). Avoidance of coyotes by red fox families. J. Wildl. Manage. 47, 852–857. doi: 10.2307/3808625
Wang, Y., Allen, M. L., and Wilmers, C. C. (2015). Mesopredator spatial and temporal responses to large predators and human development in the Santa Cruz Mountains of California. Biol. Conserv. 190, 23–33. doi: 10.1016/j.biocon.2015.05.007
Wassmer, R. W. (2000). Urban Sprawl in a U.S. Metropolitan Area: Ways to Measure and A Comparison of The Sacramento Area to Similar Metropolitan Areas in California and The U.S. Available online at: https://papers.ssrn.com/abstract=241975 (Accessed October 22, 2018).
Keywords: human presence, mesopredators, overlap, spatiotemporal activity, urban gradient
Citation: Moura CW, Clucas B and Furnas BJ (2022) Humans Are More Influential Than Coyotes on Mammalian Mesopredator Spatiotemporal Activity Across an Urban Gradient. Front. Ecol. Evol. 10:867188. doi: 10.3389/fevo.2022.867188
Received: 31 January 2022; Accepted: 06 June 2022;
Published: 23 June 2022.
Edited by:
Ryan Jensen, Brigham Young University, United StatesReviewed by:
Tom Langen, Clarkson University, United StatesInger Suzanne Prange, Appalachian Wildlife Research Institute, United States
Seth Magle, Lincoln Park Zoo, United States
Copyright © 2022 Moura, Clucas and Furnas. This is an open-access article distributed under the terms of the Creative Commons Attribution License (CC BY). The use, distribution or reproduction in other forums is permitted, provided the original author(s) and the copyright owner(s) are credited and that the original publication in this journal is cited, in accordance with accepted academic practice. No use, distribution or reproduction is permitted which does not comply with these terms.
*Correspondence: Chad W. Moura, Y2hhZC5tb3VyYUBodW1ib2xkdC5lZHU=