- 1Centro de Investigaciones Tropicales, Universidad Veracruzana, Xalapa, Mexico
- 2Instituto de Investigaciones en Comportamiento Alimentario y Nutrición, Universidad de Guadalajara, Ciudad Guzmán, Mexico
- 3Department of Anthropology, Northwestern University, Evanston, IL, United States
- 4Vanderbilt Microbiome Innovation Center, Vanderbilt University, Nashville, TN, United States
- 5Unit of Physiology, Pathophysiology and Experimental Endocrinology, Department of Biomedical Sciences, University of Veterinary Medicine, Vienna, Austria
Animals living in anthropogenically disturbed habitats are exposed to environmental stressors which can trigger physiological reactions, such as chronic elevations of glucocorticoid hormones. Physiological responses to stressors may induce changes in the gut microbiome, most likely, facilitated by the gut–brain communication. Although these effects have been observed in humans and animal models, elucidating gut bacterial changes in wild animals under natural stressful conditions is still an ongoing task. Here we analyzed the association between physiological stress related to anthropogenic forest disturbance and changes in gut bacterial communities of black howler monkeys (Alouatta pigra) living in forest fragments in Mexico. We measured individuals’ fecal glucocorticoid metabolites (fGCMs) as an index of physiological stress and created inventories of fecal bacterial communities sequencing the 16S rRNA gene to assess gut microbiome change. We evaluated environmental stress by estimating differences in food availability – feeding tree diversity and biomass – in each group’s habitat. We found that both fGCMs and food availability indices were related to gut bacterial community shifts in black howler monkeys. Furthermore, using structural equation modeling, we found that a decrease in food availability, estimated through reductions in feeding tree basal area, increased fGCMs, which in turn induced increases in bacterial richness. Our findings show that the activation of the hypothalamic–pituitary–adrenal (HPA)-axis, which is a physiological response sensitive to environmental stressors such as the ecological disturbance of a habitat, contributes to structure the gut microbiome of arboreal primates in disturbed forests.
Introduction
Wild animals are frequently exposed to adverse conditions in nature which trigger stress responses of individuals (Wingfield, 2005; Messina et al., 2018). Environmental stressors can be acute (e.g., predation) or chronic (e.g., habitat degradation), and predictable (e.g., seasonality) or unpredictable (e.g., sudden storms, deforestation) (Wingfield, 2005; Boonstra, 2013), affecting differently the degree of the animal stress response. While acute stress responses occur on a short-term scale (e.g., within minutes) and are characterized by the rapid rise and fall of hormones, suppressing functions that are not immediately needed like digestion or reproduction, a chronic stress response develops over a longer-term scale (e.g., weeks or months) with negative consequences for individuals, such as immunosuppression or reduced growth (Romero, 2004; Romero et al., 2009; Sapolsky, 2021). The reaction of the hypothalamic–pituitary–adrenal (HPA) axis and the resulting release of glucocorticoids are often measured to assess the physiological response related to stressful environmental stimuli (Sheriff et al., 2011), and there is plenty of evidence indicating that animals inhabiting anthropogenically disturbed habitats tend to have increased glucocorticoid concentrations possibly reflecting higher stress levels (Creel et al., 2002; Martínez-Mota et al., 2007; Balestri et al., 2014; Rehnus et al., 2014; Formenti et al., 2018; Kleist et al., 2018; Messina et al., 2018; Boyle et al., 2021). However, determining the effects of stress due to anthropogenic impact on animal-symbiont associations is still a research area that needs to be explored in wildlife (Trevelline et al., 2019).
The gut microbiome of vertebrates is shaped by a combination of intrinsic (e.g., host genetics) and extrinsic (e.g., ecology, climate) factors which determine the structure, stability, and dynamics of bacterial communities, including diversity and function (Douglas, 2018). The host’s physiological stress response, i.e., physiological reactions to noxious or threatening physical or psychological stimuli (Romero, 2004; Reeder and Kramer, 2005), has been recognized as a contributing factor of microbiome change, mediated in part by interactions within the gut–brain axis (Foster et al., 2017; Benavidez et al., 2019). That is, the complex communication between the gastrointestinal tract and the central nervous system modulates microbiome responses to stressful stimuli (Foster and McVey Neufeld, 2013; Moloney et al., 2014).
Traditional models of stress indicate that hosts experiencing stress-related conditions, such as anxiety or depression triggered by psychosocial stressful stimuli, frequently suffer gut microbial dysbiosis (Pierce and Alviña, 2019; Meyyappan et al., 2020). For example, in rodents in which dominance–subordination relationships are tested, socially stressed individuals show lower gut bacterial diversity and alterations in microbial metabolism related to short-chain fatty acids (SCFAs) compared to controls (Bharwani et al., 2016). On the other hand, a depauperate gut microbiome has been linked to exacerbated stress-related behaviors and physiological stress reactions (Sudo et al., 2004; Sun et al., 2017), but restoration of microbial communities alleviates stress perception. This is supported by rodent models which show reductions in anxiety-like behaviors after receiving fecal transplants from hosts with an intact gut microbiome (De Palma et al., 2017; Zhang et al., 2019). Despite the evidence of the relationship between stress and the microbiome in humans and animal models, elucidating gut bacterial changes of wild animals under natural stressful conditions is still an ongoing task in the scientific community.
The relationship between changes in glucocorticoids and shifts in microbial communities has been explored in a limited number of wild animal systems. While minimal effects of glucocorticoids on bacterial communities have been found in lowland gorillas (Vlčková et al., 2018), in other mammals, such as eastern gray and red squirrels, baseline and stress-induced glucocorticoids were associated with the structure of fecal bacterial communities (Stothart et al., 2016, 2019). Moreover, an experimental study in which yellow-legged gulls received corticosterone implants provided strong support to the premise that increased glucocorticoids may induce shifts in the gut microbiome of wildlife (Noguera et al., 2018).
Wild primates that endure in anthropogenically disturbed forest fragments are a suitable model to assess the relative effects of physiological stress on changes in gut bacterial communities. Canopy dwelling primates rely on trees for daily activities such as foraging, feeding, traveling, and resting (Hopkins, 2011; Cartmill, 2017), but forest disturbance is a persistent threat for primate survival (Estrada et al., 2017). Forest loss and fragmentation associated with anthropogenic activities tend to modify the vegetation structure (e.g., tree species composition and biomass) in forest remnants, reducing the availability of primary food sources that are energetically and nutritionally important for frugivorous–folivorous arboreal primates (Arroyo-Rodríguez and Dias, 2010; Righini et al., 2017). Such disturbance, i.e., decreased food quantity and quality, has been proposed to induce physiological stress in species including lemurs (Lemur catta, Gabriel et al., 2018; Propithecus diadema, Tecot et al., 2019), colobus (Piliocolobus tephrosceles, Chapman et al., 2006), and howler monkeys (Alouatta pigra, Behie and Pavelka, 2013). In addition, lower food availability in fragments has been related to gut bacterial diversity decreases and shifts in bacterial communities in primates, reducing the bacterial metabolic capacity to produce SCFA (Amato et al., 2013; Barelli et al., 2020), which not only provide energy to the host, but are also involved in the regulation of neuro-immunoendocrine function (Silva et al., 2020). This scenario calls for a better understanding of the additive effects of forest disturbance on changes in the gut microbiota of primates living in fragments, possibly mediated and/or exacerbated by the host’s physiological stress response triggered by deficits in food sources.
Here we aimed to explore the association between physiological stress related to anthropogenic forest disturbance and changes in gut bacterial communities of endangered black howler monkeys (A. pigra) living in forest fragments in the Yucatan peninsula, Mexico. In this region, some populations of black howler monkeys can be found in relatively large protected areas, however, several other populations inhabit fragmented landscapes characterized by a mosaic of small vegetation patches. Therefore, studying these remaining unprotected populations affected by anthropogenic disturbance can help us elucidate the role of the gut microbiota and the metabolic and physiological responses of these animals to changing environments. We assessed the degree of disturbance of fragments through differences in the forest structure (i.e., species diversity and biomass of feeding trees) commonly used as food sources in primary forests. We hypothesized that monkeys inhabiting forest fragments with decreased food availability (i.e., lower feeding tree diversity and basal area) would show higher stress levels, measured by fecal glucocorticoid metabolite (fGCM) concentrations, a non-invasive index of the HPA axis activity (Palme, 2019), and therefore, alterations in the gut microbiome. To explore this hypothesis, we tested the direct effects of habitat disturbance versus the mediating effects of stress reactions using structural equation modeling. If habitat disturbance has only direct effects, we predict that as food availability decreases, bacterial communities would decrease as well, independent of fGCM concentrations of individuals. In contrast, if microbiome change is mediated by stress imposed by the habitat condition, we predict that as food availability decreases, howler monkeys would show higher fGCM concentrations, inducing a decrease in bacterial diversity.
Materials and Methods
Study Site and Sample Collection
We studied wild black howler monkeys living in fragments of semi-deciduous tropical forest in the municipality of Escárcega, State of Campeche, Mexico (18°36′N, 90°48′W). Two groups (G1 and G2) inhabited a large fragment of 2100 ha, where there were at least other 20 groups. Groups G1 and G2 had defined territories within the fragment, but occasionally they overlapped at the boundaries of their home range. We also studied four other groups (G3–G6) that lived in small highly disturbed fragments ranging in size from <3 to 9 ha. These groups inhabited one fragment each, and they did not share the fragment with other groups or solitary individuals, nor used the landscape matrix or other fragments for feeding. Groups ranged from 4 to 12 individuals per group, with an average of 2.3 ± 1.4 adult males and 2.2 ± 0.8 adult females. Group home ranges varied from 0.8 to 14 ha (Table 1).
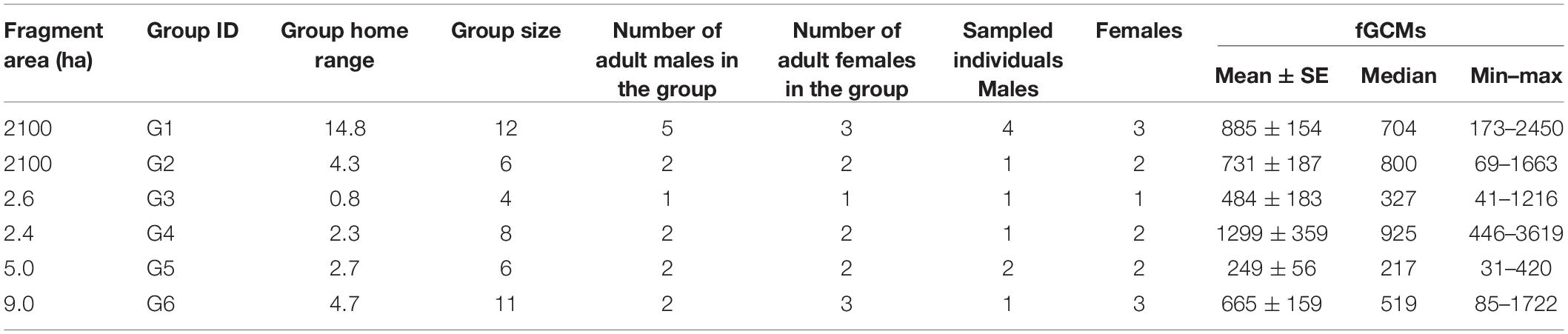
Table 1. Size of fragments, home range, number of individuals, and concentrations (ng/g) of fecal glucocorticoid metabolites (fGCMs) of six groups of black howler monkeys (Alouatta pigra) from Escárcega, State of Campeche, Mexico.
These monkey groups were part of a longer term behavioral and ecological research project of black howler monkeys living in a fragmented landscape, in which study subjects were individually recognized and monitored for more than a year (January 2011–March 2012; Martínez-Mota et al., 2017, 2021). Intense forest loss and fragmentation is occurring since the 1970s in this region, where close to 3,000,000 ha of deciduous and semi-deciduous forests have been lost between 1978 and 2000 (Díaz-Gallegos et al., 2010). At a small scale, deforestation has induced constant changes in size and shape of the studied fragments, mostly because these small vegetation patches are privately owned, and subjected to slash and burn agriculture and timber extraction for subsistence of local people. Moreover, road construction and a massive and controversial railroad megaproject (“The Maya Train”) are currently happening, threatening biodiversity and wildlife, including the black howler populations at these sites (Pérez Ortega and Gutiérrez Jaber, 2022).
Fresh fecal samples (N = 61) were collected immediately after defecation from adult male (N = 10) and female individuals (N = 13) in rainy (August and October) and dry (February and March) months (exact fecal sample sizes per group are provided in Supplementary Table 1). We sampled individuals belonging to a particular group within the same day. When we missed an individual, its feces were collected 2 or 3 days after the collection of the rest of the group. We used different days within each sampling month to complete our sampling across groups. We previously found weak to no effects of seasonality on bacterial diversity of these individuals (Martínez-Mota et al., 2021); therefore, seasonal effects are not considered as a contributing factor of microbial change in this study. Sampled individuals were at least 3 years old, and based on no signs of sickness or evident morbidity, they were considered healthy. We collected ∼3 fecal samples per individual (mean 2.7 ± 0.5 SD), and the number of sampled individuals per group is provided in Table 1. Two aliquots were obtained for each sample to be used for microbiome and fGCM analyses: 5 g of feces were stored in Falcon tubes with 96% ethanol and kept in a refrigerator at 4°C over 3–11 months until shipping to the United States for microbiome analysis. Upon arrival to the United States these samples were stored at −80°C over 6 months until processing. The other aliquot (2 g of fresh feces) was stored in 20 ml scintillation vials at −4°C during 2–3 days until fGCM extraction was conducted in the field lab.
Microbial and Glucocorticoid Analyses
Sample processing for microbial analysis were conducted at Northwestern University in the Amato lab. DNA was extracted from fecal samples using MOBio PowerSoil kits. The V4–5 hypervariable region of the bacterial/archaeal 16S rRNA gene was amplified using a two-step PCR (Earth Microbiome Project primers 515F/926R and Fluidigm Access Array primers containing sample-specific barcodes) as described in Mallott and Amato (2018). PCR products were purified and normalized using a SequalPrep Normalization Plate and sequenced on the Illumina MiSeq V3 platform to produce 2 × 250 bp paired-end reads at the University of Illinois-Chicago DNA Services Facility.
Bacterial/archaeal sequences were processed in the Quantitative Insights into Microbial Ecology 2 (QIIME 2) bioinformatics platform, version qiime2-2020.2 (Estaki et al., 2020). We first removed primers using the q2-cutadapt plugin. Later, single-end sequences were subjected to quality control including denoising, merging, and chimera removal using the DADA2 pipeline (Callahan et al., 2016). Microbial reads were then assigned as amplicon sequence variants (ASVs; Callahan et al., 2017) using the q2-dada2 plugin. Sequence taxonomic assignment was performed using the naive Bayes classifier trained on the SILVA 132 99% OTUs sequence reference (Quast et al., 2013). We also performed filtering of chloroplast, mitochondria, and doubletons. To control for the effects of variable sequencing depth, samples were rarefied to 16,600 sequences prior to downstream alpha and beta diversity analyses, and no samples were excluded by the rarefaction step.
Fecal glucocorticoid metabolites were extracted from feces in the field laboratory following the methods described in Palme et al. (2013). For each sample, 5 ml of 80% methanol was added to 0.5 g wet weight of feces. The suspension was vortex-mixed for 10 min, and then centrifuged at 1600 × g for 20 min. One milliliter of the supernatant was diluted in 9 ml of distilled water. Diluted extracts were passed slowly through solid-phase extraction (SPE) cartridges (MaxiClean Prevail C18 SPE Cartridges Alltech®). SPE cartridges were air-dried and stored at 4°C until shipping to the Department of Biomedical Sciences, University of Veterinary Medicine, Vienna, Austria.
At the University of Veterinary Medicine, steroid extracts were eluted from SPE cartridges using methanol. Concentrations of fGCMs were measured with a group specific 11-oxoetiocholanolone enzyme immunoassay (EIA) that quantifies glucocorticoid metabolites with a 5β-3α-ol-11-one structure (Möstl et al., 2002). Successful validation of this EIA to evaluate adrenocortical activity in black howler monkeys has been already conducted (Martínez-Mota et al., 2008). Sensitivity was 5 ng/g. Intra- and inter-assay coefficient of variation for a high and low concentration pool sample was 2.6 and 2.9% (intra), and 9.7 and 12.5% (inter), respectively. Concentrations are reported in ng/g wet weight.
Assessment of Feeding-Tree Diversity and Biomass in Fragments
To assess the impact of forest disturbance on food availability in fragments, we estimated the diversity of tree species used as food sources by our study groups. This information was based on a 14-month study on the feeding ecology of black howler monkeys conducted simultaneously in the largest fragment (Righini et al., 2017). We chose 15 arboreal taxa as baseline (Table 2), which represented the most consumed tree species that accounted for >85% of the howler monkey diet in a relatively less disturbed area (Righini, 2014; Righini et al., 2017). First, we established 10 Gentry’s belt transects of 50 × 2 m within each group’s home range. This technique is a reliable method since it has been shown by numerous studies in the tropics that 1000 m2 represents an adequate sample size to calculate plant species diversity within a community (Gentry, 1982). On each transect we recorded all trees with diameter at breast height >10 cm and identified each tree to species level. We then calculated species diversity and assessed equitability of feeding trees for each sampled home range, using the Shannon diversity and Pielou’s evenness indices, respectively (Magurran, 1988). These indices were calculated in the Past 3 software (Hammer et al., 2001). We also estimated food biomass per howler home range, by quantifying the basal area of feeding tree species sampled in the established transects (Table 3). Basal area is used as surrogate for tree biomass and is correlated with food productivity of primates (Chapman et al., 1992, 1994; Ganzhorn, 2003).
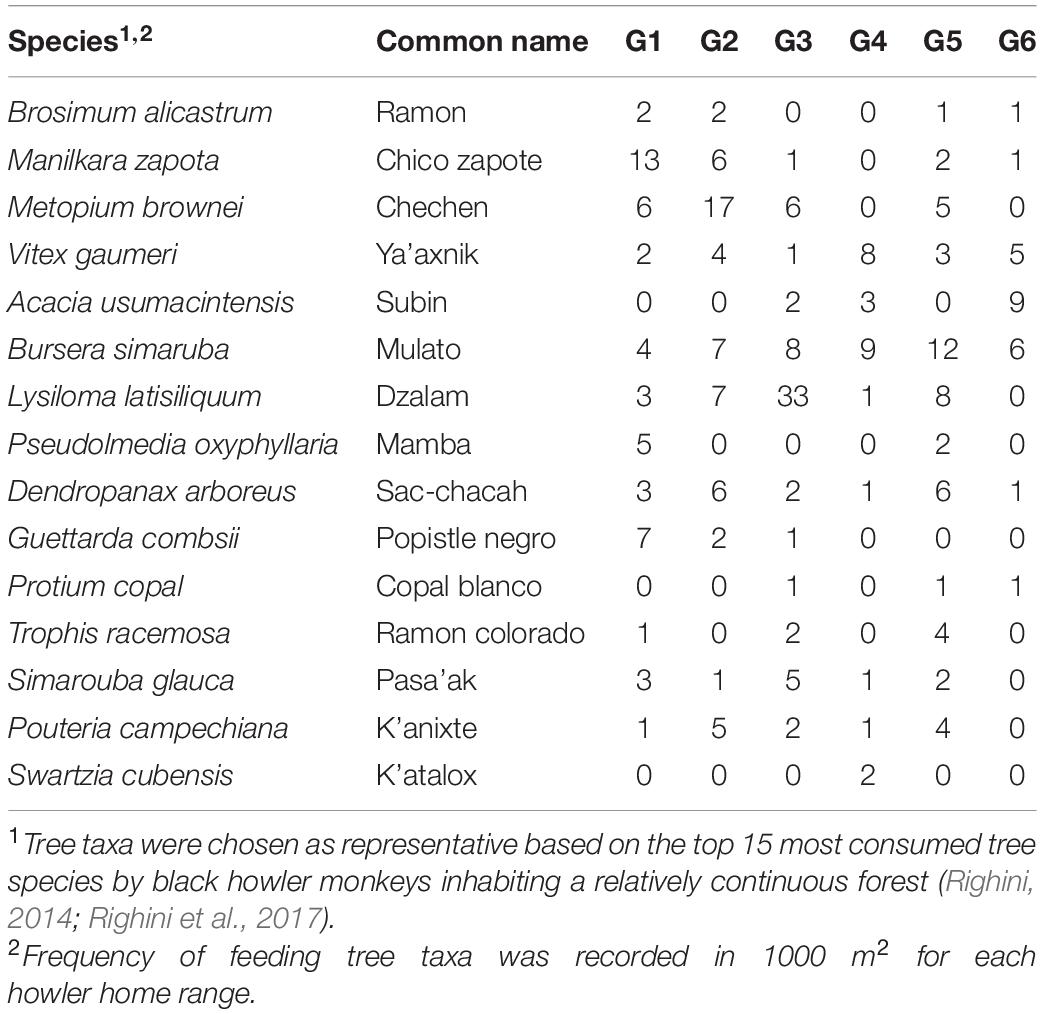
Table 2. Frequency of 15 arboreal taxa used as feeding trees by 6 groups (G1–G6) of black howler monkeys (Alouatta pigra).
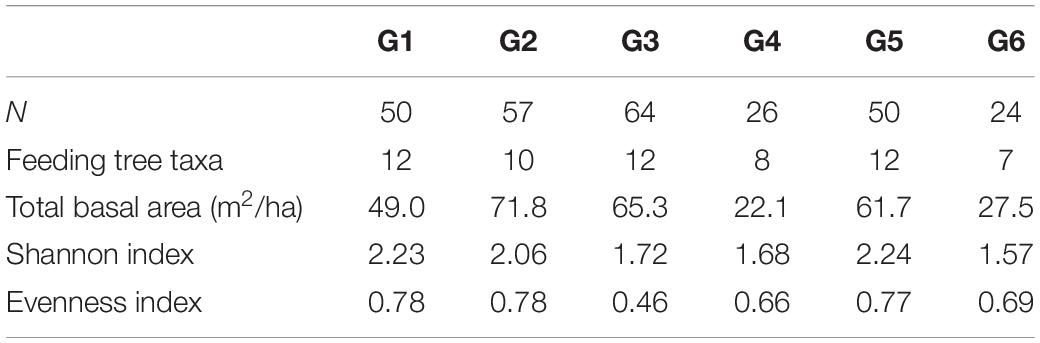
Table 3. Number of trees, species, total basal area, and diversity indices of feeding trees registered in the home range of six groups (G1–G6) of black howler monkeys (Alouatta pigra) at Escárcega, State of Campeche, Mexico.
Data Analyses
We assessed changes in bacterial alpha diversity related to physiological stress and food availability in disturbed forest fragments with generalized linear mixed models (GLMMs), using the glmmADMB package (Skaug et al., 2018) in the R software (R Core Team, 2020). Three metrics of bacterial alpha diversity were estimated for each sample in QIIME 2, including Faith’s phylogenetic diversity, Shannon diversity, and Pielou’s evenness indices; these indices were used as response variables. Faith’s phylogenetic diversity index and the Shannon index followed a normal distribution (Shapiro–Wilk test: P > 0.05); therefore GLMMs were fitted with a Gaussian distribution and identity function. Values of the evenness index range from 0 to 1, therefore, this index was fitted with a beta distribution and a logit function. fGCMs (i.e., physiological stress index), feeding tree diversity indices and basal area of feeding trees (i.e., food availability indices) were used as predictors. We tested for multi-collinearity between predictors using variance inflation factors (VIFs) in the car package (Fox and Weisberg, 2019), and found that all variables maintained low values (i.e., VIF = 1). The individual ID was set as a random factor to control for repeated measures.
For each alpha diversity metric, we first created a full model that included an interaction term between stress and food availability indices (i.e., response variable ∼ tree_diversity_shannon + tree_diversity_evenness + tree_basal_area + fGCMs + tree_diversity_shannon × fGCMs + tree_diversity_evenness × fGCMs + tree_basal_area × fGCMs + random term). We then ran a model selection command using the dredge function of the MuMIn package (Barton, 2009). All generated models are shown in Supplementary Table 2. We selected the best models based on AICc criterion, ΔAIC, and model weight (Wagenmakers and Farrell, 2004). Effects of predictors for the best model were generated with the anova function using the car package. P-values were adjusted with Benjamini–Hochberg false discovery rate (fdr, Benjamini and Hochberg, 1995). To examine in more detail causal relationships between food availability, stress, and bacterial diversity, we conducted a structural equation modeling using the lavaan package (Rosseel, 2012). In this analysis we used bacterial richness (i.e., observed ASVs) as a response variable (Supplementary Table 3).
We tested for the effects of stress and its interaction with food availability indices on bacterial community composition, using permutational multivariate analysis of variance (PERMANOVA). These analyses were run in R, using the function adonis2 implemented in the vegan (Oksanen et al., 2019) and qiimer (Bittinger, 2016) packages. Predictors were concentrations of fGCMs, feeding tree diversity, evenness, and basal area, and the interaction between these predictors. Jaccard and Bray–Curtis distance matrices were used as measures of bacterial beta diversity (Knight et al., 2018). We tested first the effects of predictors and their interactions, and later ran again a reduced model discarding non-significant interaction terms. We also tested for within-group dispersion using the betadisper function. Bacterial community shifts were visualized through non-metric multidimensional scaling (NMDS).
We analyzed bacterial taxonomic changes related to the observed community shifts using Count Regression for Correlated Observations with Beta-Binomial (corncob) analyses (Martin et al., 2020). This method tests for the effects of covariates on microbial relative abundances accounting for the correlational structure of microbiome data. For these analyses we removed ASVs present in less than 10% of samples and those with less than 20 reads. P-values were adjusted with Benjamini–Hochberg fdr to a cutoff = 0.01. These models were run using corncob (Martin et al., 2021) and phyloseq packages (McMurdie and Holmes, 2013) in R.
Permits
This research complied with legal requirements of Mexico (SEMARNAT−DGVS/09084/10) and was approved by the Institutional Animal Care and Use Committee of the University of Illinois (protocols #10054 and #10062).
Results
The 16S rRNA gene sequencing effort yielded 1,400,816 sequence reads with an average of 22,964 ± 5240 SD sequences per sample. After filtering we obtained a total of 1741 bacterial/archaeal ASVs with an average of 189 ± 32 SD ASVs per sample. The median value of fGCMs was 512 ng/g, ranging from 31 to 3619 ng/g. Mean, median, and minimum and maximum values of fGCMs per group are shown in Table 1.
Effects of Stress and Food Availability Indices on Bacterial Diversity
We found that bacterial alpha diversity metrics of black howler monkeys were influenced differently by the stress index and the three indices of food availability in forest fragments. The best models for each bacterial diversity metric are shown in Table 4. Bacterial diversity estimated through the Shannon index was predicted by the two indices of food availability (feeding tree Shannon diversity F1,56 = 19.3, fdr P < 0.001; feeding tree Pielou’s evenness F1,56 = 8.8, fdr P < 0.01); while bacterial diversity was negatively related with feeding tree diversity, it was positively related with feeding tree evenness (Figure 1A). The Faith’s phylogenetic diversity of bacteria was affected by the feeding tree diversity (F1,54 = 25.6, fdr P < 0.001) and its interaction with the stress index (F1,54 = 4.6, fdr P = 0.035); bacterial phylogenetic diversity increased with concomitant increases in diversity of feeding trees and fGCM (Figure 1B). However, feeding tree evenness had marginal effects on bacterial phylogenetic diversity (F1,54 = 3.9, fdr P = 0.053). Basal area of feeding trees (F1,56 = 13.9, fdr P < 0.001) was negatively associated with bacterial evenness (Figure 1C) and feeding tree evenness did not have significant effects on bacterial evenness as a single term (F1,56 = 2.7, fdr P = 0.10), despite the fact that it was included as predictor in the best model selection.
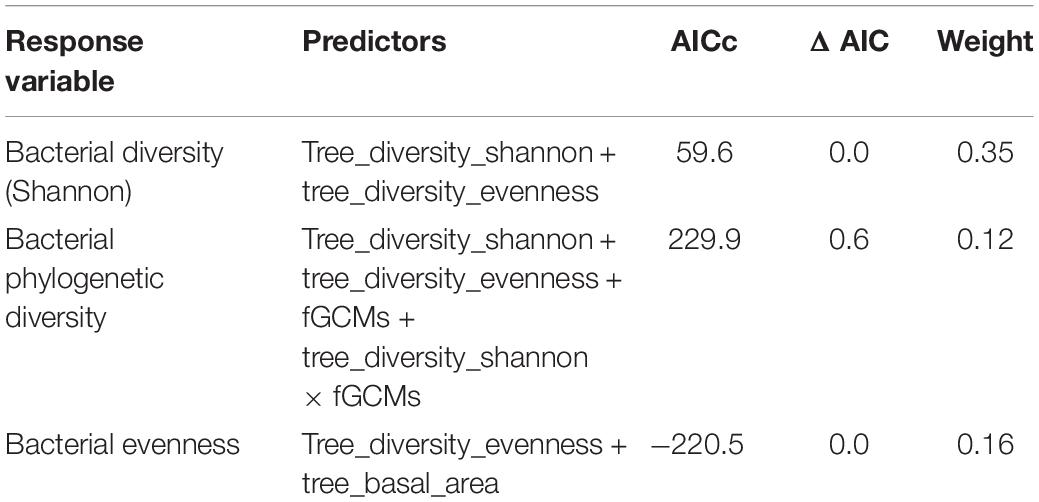
Table 4. Best model based on AICc, ΔAIC, and model weight for each alpha bacterial diversity of black howler monkeys (Alouatta pigra) inhabiting forest fragments at Escárcega, State of Campeche, Mexico.
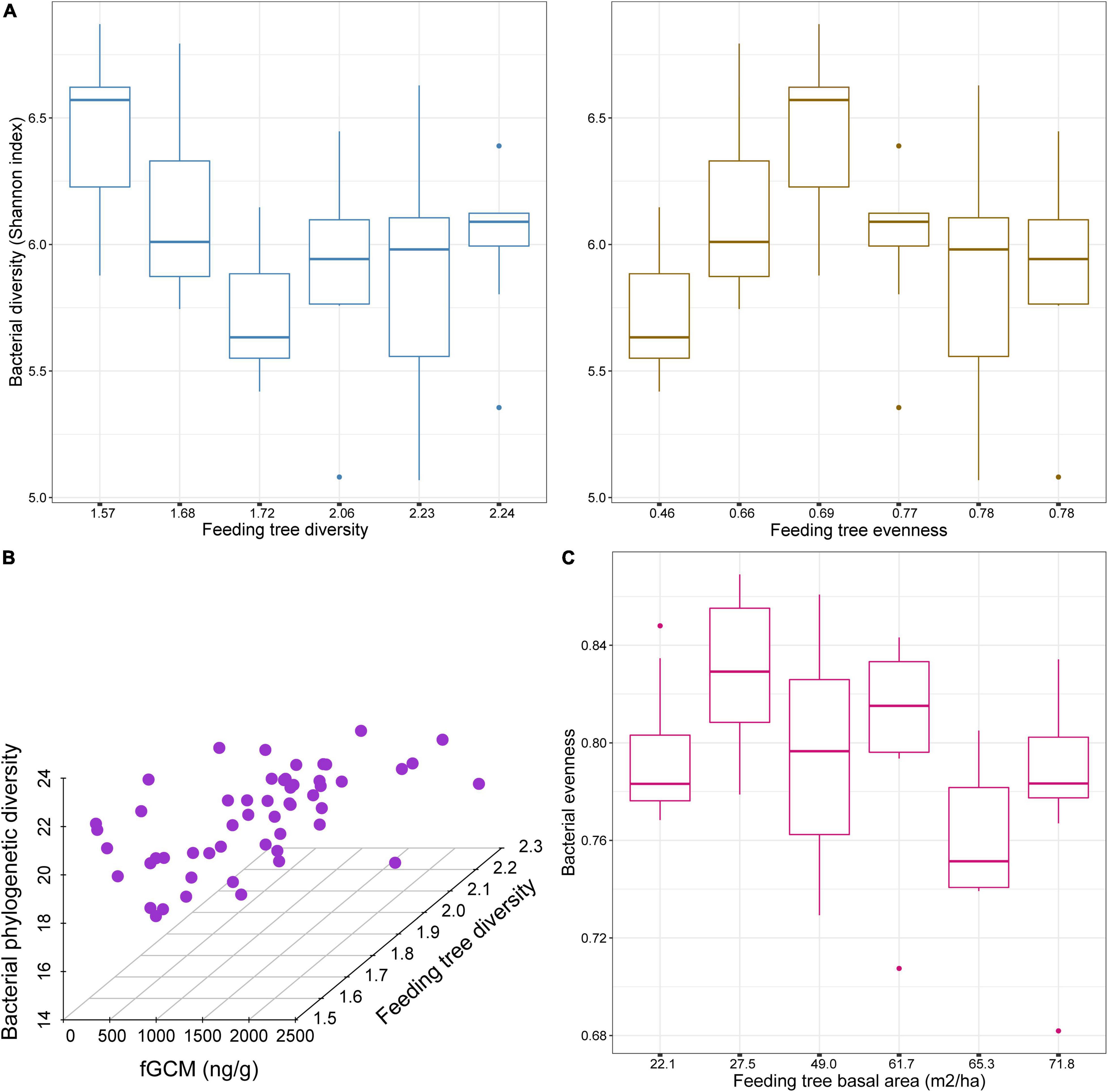
Figure 1. Changes in bacterial alpha diversity associated with variation in food availability indices and physiological stress of black howler monkeys (Alouatta pigra) inhabiting disturbed forest fragments at Escárcega, State of Campeche, Mexico. (A) Boxplots show that bacterial diversity is negatively related to feeding tree diversity estimated with the Shannon index, and positively related to feeding tree evenness. (B) Scatterplot shows the effects of an interaction between fecal glucocorticoid metabolites (fGCMs) and diversity of feeding trees estimated with the Shannon index on bacterial phylogenetic diversity. (C) Boxplot shows that bacterial evenness negatively relates to basal area of feeding trees.
Using structural equation modeling, we found that feeding tree diversity influenced bacterial richness in a direct way (estimate = −77.0, SE = 16.5, z = −4.7, P < 0.001). A negative relationship between feeding tree diversity and bacterial richness was found. Moreover, feeding tree basal area directly and negatively affected fGCMs (estimate = −0.02, SE = 0.01, z = −2.3, P = 0.02), which in turn influenced positively bacterial richness (estimate = 6.6, SE = 3.2, z = 2.1, P = 0.04); however, the effects of feeding tree diversity on bacterial richness were stronger compared to those of fGCMs (Supplementary Table 3 and Figure 2).
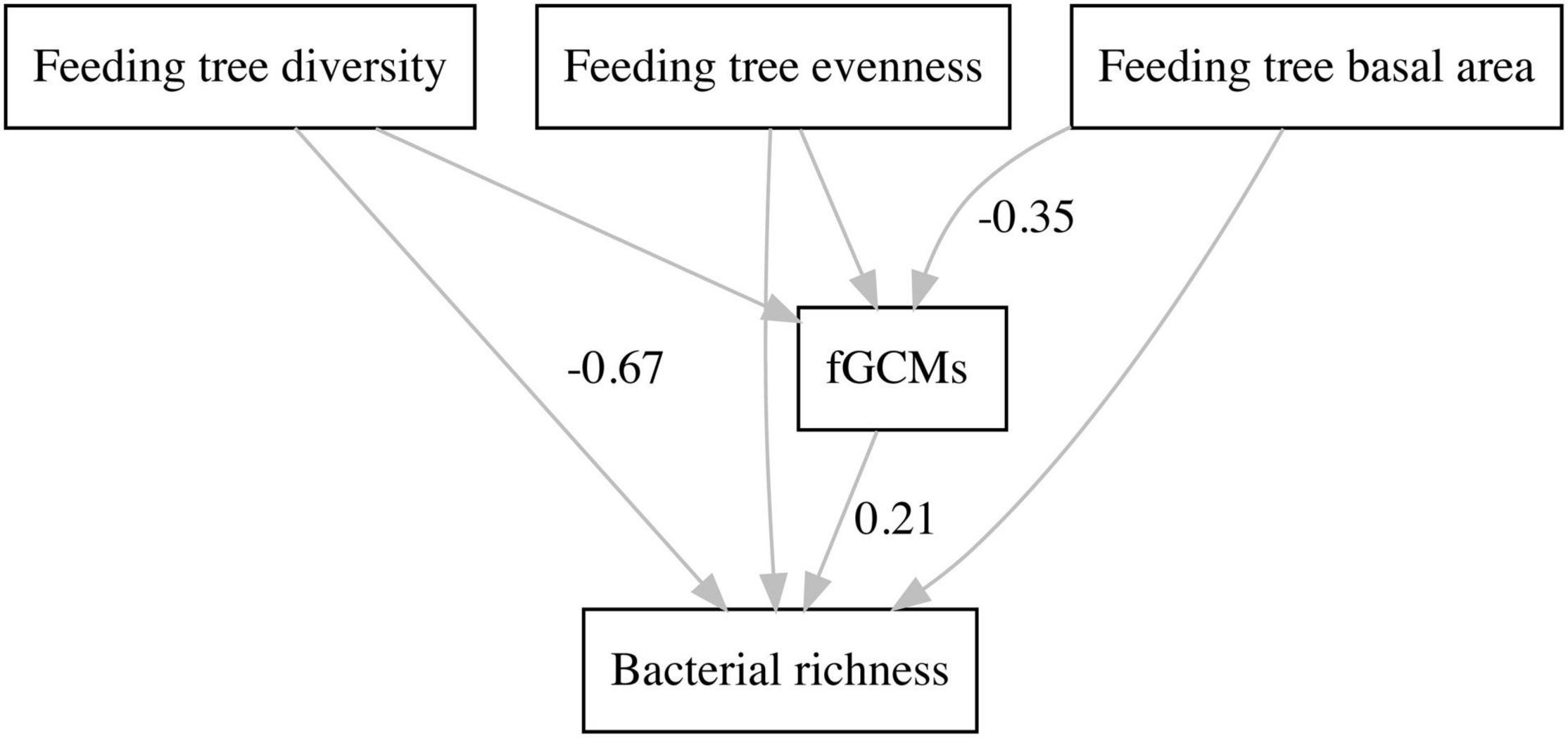
Figure 2. Path diagram from structural equation modeling, showing direct and indirect effects of food availability and physiological stress indices on bacterial richness of black howler monkeys (Alouatta pigra) inhabiting disturbed forest fragments at Escárcega, State of Campeche, Mexico. Standardized regression coefficients of significant paths are shown.
Stress and food availability indices were related to gut bacterial community shifts in black howler monkeys. PERMANOVAs using either Jaccard or Bray–Curtis distance matrices revealed that the interaction between fGCMs and basal area of feeding trees was related to bacterial community changes in these primates (Jaccard: pseudo-F1,53 = 1.4, P = 0.014, R2 = 0.021; Bray–Curtis: pseudo-F1,53 = 1.6, P = 0.018, R2 = 0.024), although the effect size was modest. Black howler monkeys inhabiting forest fragments characterized by feeding trees with lower basal area showed increased concentrations of fGCMs which influenced to some extent bacterial community structure (Figure 3). However, the interaction between fGCMs and feeding tree diversity or evenness had no effects on bacterial community shifts. After running again a reduced model discarding non-significant interactions, we found that feeding tree diversity and evenness also had significant effects on community changes, both as single terms (tree diversity: Jaccard, pseudo-F1,55 = 2.9, P = 0.001, R2 = 0.043; Bray–Curtis, pseudo-F1,55 = 3.0, P = 0.001, R2 = 0.044; tree evenness: Jaccard, pseudo-F1,55 = 2.4, P = 0.001, R2 = 0.035; Bray–Curtis, pseudo-F1,55 = 1.9, P = 0.002, R2 = 0.030; Supplementary Figures 1A,B). Within-group dispersion was not significant for fGCMs (Jaccard, P = 0.32; Bray–Curtis, P = 0.65), but significant for feeding tree diversity, evenness, and basal area (Supplementary Table 4). This indicates that the significant effects of fGCMs on community structure found in the PERMANOVAs were not due to heterogeneity of variance.
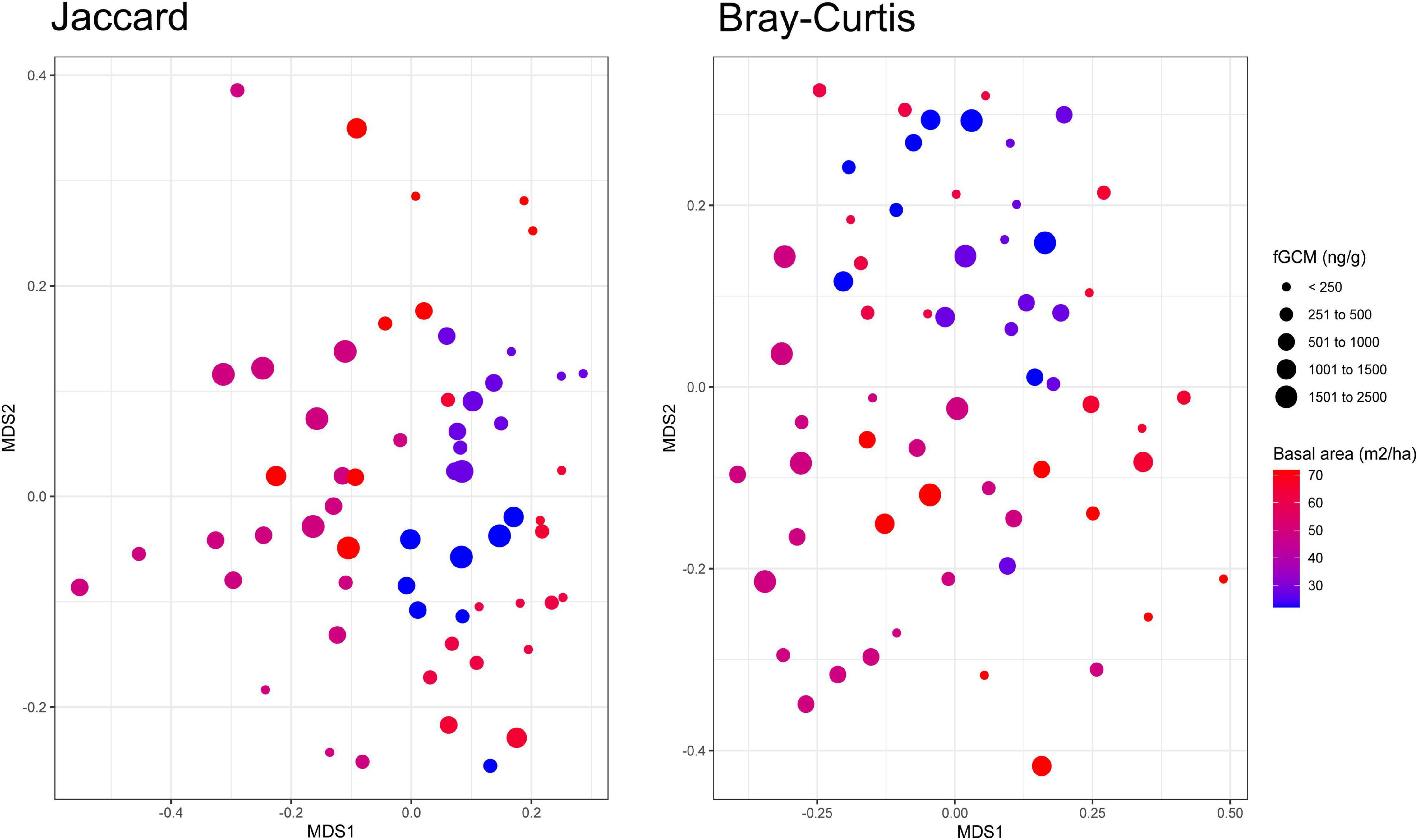
Figure 3. Bacterial community shifts associated with variation in a food availability index and physiological stress of black howler monkeys (Alouatta pigra) inhabiting disturbed forest fragments at Escárcega, State of Campeche, Mexico. Bacterial community shifts were influenced by higher stress levels of individuals inhabiting fragments with lower basal area of feeding trees. MDS plots based on Jaccard and Bray–Curtis distance matrices.
Bacterial Taxonomic Changes Related to Stress and Food Availability Indices
We found taxonomic changes in gut bacterial communities of black howler monkeys related to stress and food availability indices. Corncob analysis indicated that fGCMs significantly predicted changes in relative abundance of bacterial taxa (Estimate = −7.1e−04, SE = 2.4e−04, t-value = −2.9, fdr P < 0.05), and that the interaction between fGCMs and feeding tree basal area also influenced taxonomic change, although these effects were marginally significant (Estimate = 1.7e−05, SE = 8.8e−06, t-value = 1.9, fdr P = 0.056). Overall, howler monkeys inhabiting fragments characterized by lower feeding tree basal area showed higher fGCM levels (1000–2000 ng/g) and had higher relative abundance of ASVs belonging to Anaerostipes, Bacteroidales, Prevotellaceae, Lachnospiraceae, and Clostridiales vadin BB60 compared to individuals experiencing lower fGCMs (<500 ng/g) in areas with higher feeding tree basal area (Figure 4). Moreover, feeding tree diversity (Estimate = 16.3, SE = 3.7, t-value = 4.4, fdr P < 0.001) and evenness (Estimate = 25.8, SE = 7.2, t-value = 3.6, fdr P < 0.001) significantly predicted bacterial change but only as single terms. Howler monkeys inhabiting areas with higher feeding tree diversity showed increased relative abundance of several ASVs assigned to Bacteroidia, Clostridia, and Erysipelotrichia (Figure 5).
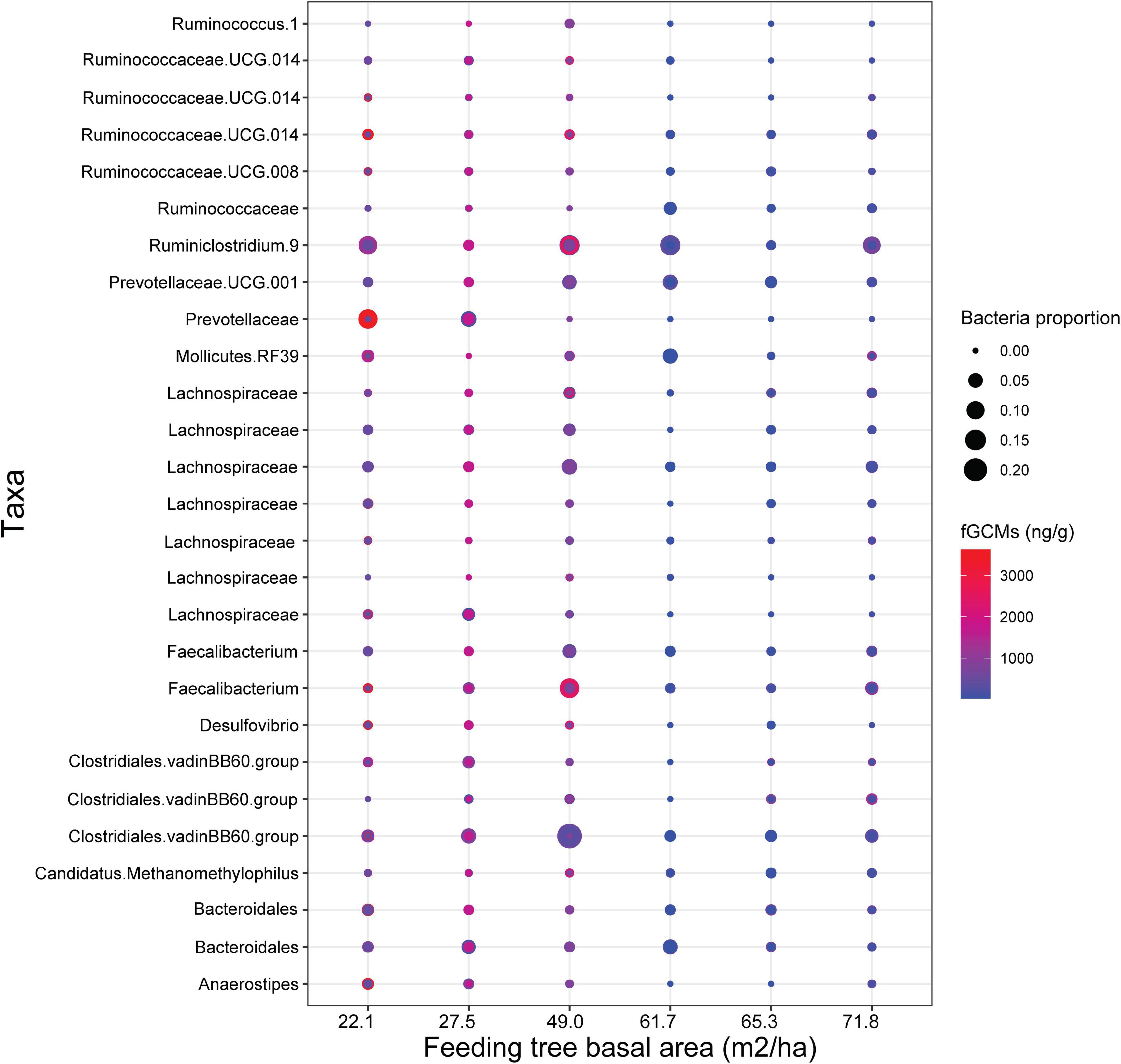
Figure 4. Changes in proportional abundance of bacterial taxa related to fecal glucocorticoid metabolites of black howler monkeys (Alouatta pigra) and to variation in feeding tree basal area. The increase in bacterial abundance is represented by size of dots, and changes in fGCM levels are represented from color transition from blue to red.
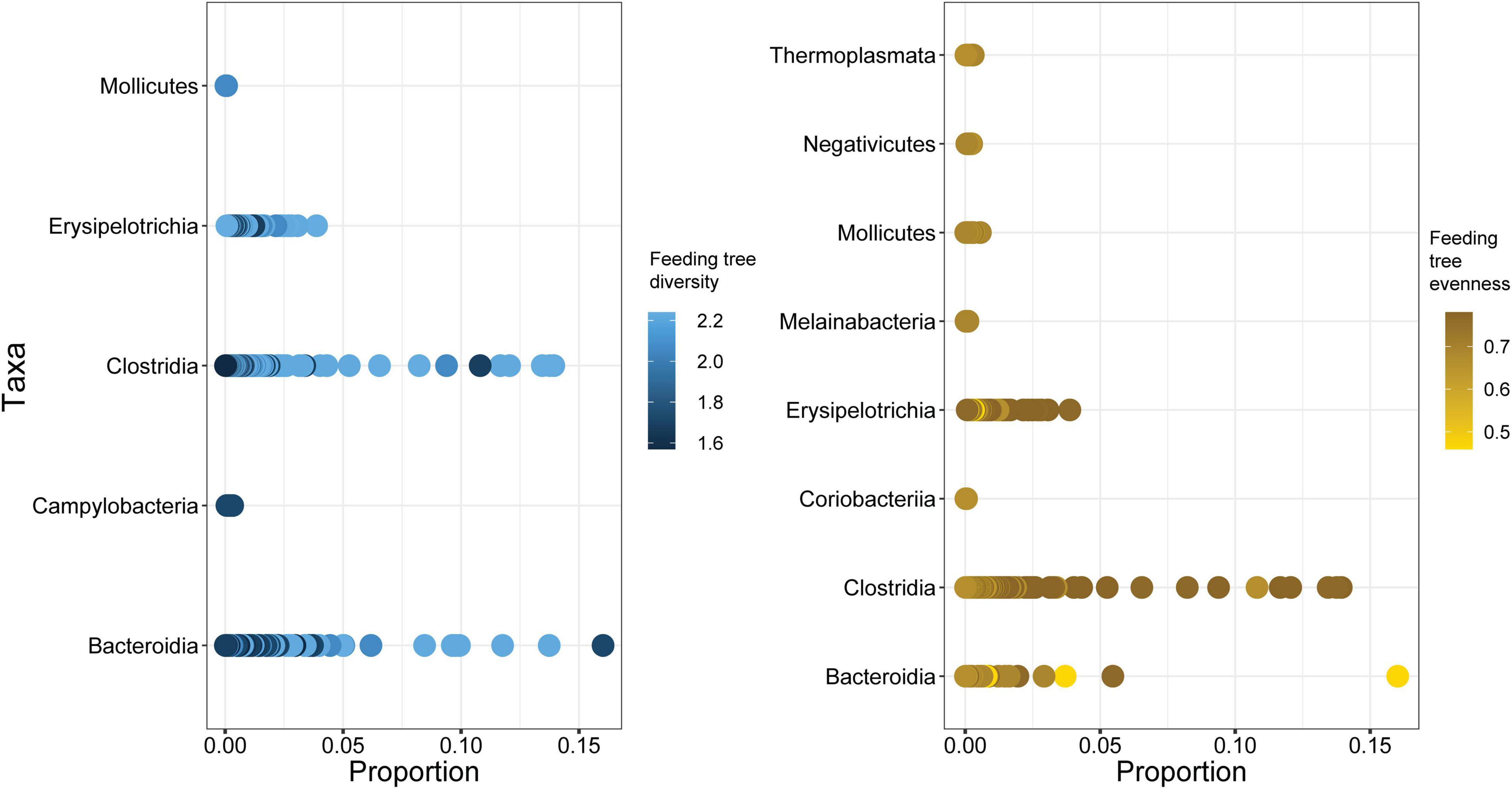
Figure 5. Changes in proportional abundance of bacterial taxa of black howler monkeys (Alouatta pigra) related to variation in measures of food availability (i.e., feeding tree diversity and evenness).
Discussion
We hypothesized that black howler monkeys inhabiting anthropogenically disturbed forest fragments would show changes in gut bacterial communities, most likely related to physiological stress imposed by deficits in food availability. To test this, we measured fGCMs as an index of physiological stress and collected data on feeding tree diversity and biomass to assess food availability and found that both alpha and beta bacterial diversity were associated with these indices. Interestingly, the interaction between a metric of food biomass (i.e., feeding tree basal area) and fGCMs significantly affected bacterial community shifts and diversity, supporting our hypothesis, although in a different direction than our prediction. Our findings show that the activation of the HPA-axis, which is a physiological response sensitive to environmental stressors such as forest disturbance (Martínez-Mota et al., 2007; Rangel-Negrín et al., 2014a; Boyle et al., 2021), contributes to some extent to structure the gut microbiome of arboreal primates in disturbed habitats.
The degree of disturbance in each forest fragment affected vegetation composition, thus the availability and abundance of food sources for black howler monkeys. This most likely influenced food and nutrient intake, impacting gut bacterial community diversity and structure of these primates. In this regard, variation in dietary breadth and macronutrient intake are contributing factors that shape gut microbiomes in human and animal hosts (David et al., 2014; Yang et al., 2020). Black howler monkeys are selective feeders that feed daily on different food items switching from fruit to leaves or other plant parts to maintain a balance between protein and non-protein energy intake (Righini, 2014; Aristizabal et al., 2017; Righini et al., 2017), and this behavioral feeding strategy induces changes not only in the composition and function of the primate gut microbiome (Amato et al., 2014, 2015), but also in the physiological stress response (Martínez-Mota et al., 2016). For example, a negative relationship between protein intake and fGCMs was found in black howler monkeys (Martínez-Mota et al., 2016), suggesting that the HPA-axis responds to fluctuations in macronutrient intake.
A limitation in our study was that we neither quantified food nor macronutrient intake to directly assess variability in energy acquisition in black howler monkeys; this approach would have allowed to address more directly the impact of nutritional deficits on stress and bacterial diversity changes. However, the structural equation modeling analysis suggests that such effects could be taking place, since there is an interplay between changes in fGCM and gut bacterial diversity in howler monkeys that inhabit forest fragments characterized by a reduction of food biomass.
Moreover, using GLMMs and structural equation modeling we found that feeding tree diversity was negatively associated with bacterial richness. This contrasts with previous findings in mammals which reported positive relationships between dietary diversity and microbial diversity (Amato et al., 2013; Barelli et al., 2020; Wastyk et al., 2021; Weinstein et al., 2021). Although the small forest fragments in our study were characterized by a low feeding tree diversity, these areas presented high abundance of lianas and vines compared to the largest fragment; these vegetation forms are associated with disturbance such as forest edges and canopy gaps (Gómez-Marín et al., 2001; Martins, 2009). Howler monkeys in these areas may have supplemented their diets with these vegetation forms increasing gut bacterial richness. In other A. pigra populations inhabiting disturbed fragments in Southern Mexico, these primates obtain the majority of their mineral intake from vines and epiphytes, which are significantly higher in Ca and P and lower in fiber than plants from less disturbed forests (Aristizabal, 2013). Thus, the contribution of the phytochemical and fiber content of vines, lianas, and epiphytes to howler monkeys’ diet in small fragments, could have affected gut bacterial communities, possibly adding to the differences found between the study groups living in contrasting habitats.
Independent of the physiological stress response, our results indicated that, at a taxonomic level, bacterial abundance was also predicted by feeding tree diversity. The relative abundance of bacteria belonging to Clostridia and Erysipelotrichia was increased in areas of higher feeding tree diversity. The metabolic activity of these bacterial taxa has recently been linked to sugar alcohol metabolism from fermentable complex carbohydrates (Tiffany et al., 2021). It is possible that howlers living in habitats which offered a higher diversity of feeding trees, had access to more variable food items rich in non-digestible fiber and sugar (e.g., young and mature leaves, ripe and unripe fruits, flowers), which can be used by these bacteria with capabilities of degrading complex carbohydrates.
The patterns we report here provide important foundational knowledge regarding the interactions between the environment, diet, stress, and the microbiome. However, we acknowledge that the relatively small sample size and sparse longitudinal sampling constrain our ability to disentangle some of the measured variables. The logistics of sampling known individuals in different forest fragments limited the quantity and type of data that we could obtain. Moving forward, studies should further explore relationships of interest reported here, choosing forest fragments representing a gradient of ecological attributes that are identified a priori and sampling individuals more frequently over time.
It will also be important to explore the extent to which the magnitude of these effects varies across different host species and the physiological pathways that contribute to this variation. Although several populations of black howler monkeys inhabit highly disturbed tropical forests (Pozo-Montuy et al., 2011; Rangel-Negrín et al., 2014b; Klass et al., 2020; Martínez-Mota et al., 2021) and are most likely exposed to environmental stressors (Martínez-Mota et al., 2007; Behie and Pavelka, 2013; Rangel-Negrín et al., 2014a), these primates might have coping mechanisms to deal with food availability deficits resulting from forest loss and fragmentation. For instance, Amato et al. (2015) found that SCFA produced by gut bacteria of howler monkeys were increased during periods of low energy intake, which suggests that microbial metabolism may support energy availability under critical periods of low food intake. This agrees with our findings which revealed that black howler monkeys showing increased fGCM levels and living in fragments characterized by lower food biomass showed increased abundance of fermenting bacteria such as Prevotellaceae, Ruminococcaceae, Lachnospiraceae, and Faecalibacterium. Given the communication between the brain and the gut (i.e., brain–gut axis, Foster et al., 2017), testing whether environmental stress perception and physiological stress responses of black howler monkeys serve as cues to their gut microbiome to respond and provide energy, or whether gut microbial changes signal a significant challenge that threatens black howler monkeys, will be important for understanding primate adaptation to changing environments. Similarly, data describing these relationships in other wild animals will allow a broader understanding of environment–microbiota-physiology dynamics.
Several studies have attempted to determine whether perceived environmental stressful conditions (e.g., habitat disturbance, food availability constraints) and the concomitant physiological stress reactions affect health and predict survival of wild animals (Romero and Wikelski, 2001). However, it is still unclear if such physiological responses result in pathologies or even fitness reduction in wildlife, including free-ranging primates (Bonier et al., 2009; Boonstra, 2013; Beehner and Bergman, 2017). Our results of the interaction effects between an index of stress and one aspect of habitat disturbance (i.e., food availability) on bacterial community shifts and richness, indicate that animal’s physiological responses to environmental challenging conditions may affect other body systems, like the gut microbiome. Whether the complex interplay between stress reactions and dysbiosis in wild animals contributes to a fitness decline or a detrimental health state should be further explored in nature.
Studies investigating the interplay between stress reactions and the gut microbiome in wildlife are still limited, but an emerging field exploring the communication between endocrine and gut microbiome systems in free ranging animals is currently developing (see Benavidez et al., 2019). Stress responses may induce intestinal disorders affecting microbial structure and/or metabolism in wild and domestic animals (Söderholm et al., 2002; Li et al., 2018; Yan et al., 2021). For instance, correlations between stress biomarkers, such as glucocorticoids, and gut bacterial abundance were found in eastern gray squirrels, pangolins, yellow-legged gulls, and western lowland gorillas (Noguera et al., 2018; Vlčková et al., 2018; Stothart et al., 2019; Yan et al., 2021); however, the size of effects varied according to the host species. Our results support this trend, in which only the relative abundance of certain bacterial taxa was associated with changes in the host’s fGCM levels.
Hypothetical models of host–microbiome interaction point out that environmental and host-related factors work in concert to sculpt bacterial communities in wild primates (Amato and Righini, 2015; Benavidez et al., 2019). Supporting this notion, here we showed that gut bacterial communities of black howler monkeys living in disturbed forest fragments could be influenced not only by food availability (as a surrogate of diet), which is a significant ecological factor contributing to shape wildlife microbiomes, but also by the host endocrine physiology.
Data Availability Statement
The datasets presented in this study can be found in online repositories. The names of the repository/repositories and accession number(s) can be found below: https://www.ncbi.nlm.nih.gov/, BioProject PRJNA798151.
Ethics Statement
The animal study was reviewed and approved by the Institutional Animal Care and Use Committee of the University of Illinois (protocols #10054 and #10062).
Author Contributions
RM-M and KA designed the investigation, collected the field data, conducted the bioinformatic analyses, and led the writing of the manuscript. NR collected the field data and wrote the manuscript. EM conducted the laboratory analysis of microbiome data and wrote the manuscript. RP supervised the laboratory analyses of glucocorticoid metabolite data and wrote the manuscript. All authors contributed to the article and approved the submitted version.
Funding
This work was supported by the Conacyt-Mexico (fellowship #229748 granted to RM-M), Scott Neotropical Fund-Cleveland Metroparks Zoo, Idea Wild, Lewis and Clark Fund-American Philosophical Society, University of Illinois, and Northwestern University.
Conflict of Interest
The authors declare that the research was conducted in the absence of any commercial or financial relationships that could be construed as a potential conflict of interest.
Publisher’s Note
All claims expressed in this article are solely those of the authors and do not necessarily represent those of their affiliated organizations, or those of the publisher, the editors and the reviewers. Any product that may be evaluated in this article, or claim that may be made by its manufacturer, is not guaranteed or endorsed by the publisher.
Acknowledgments
We thank the local community from ejido Division del Norte, Escárcega, México and El Tormento-INIFAP-Campeche for allowing us to conduct fieldwork. RM-M and NR would like to thank the undergraduate students who helped us collect samples (L. L. López Madrigal, V. Jiménez de la Cruz, and F. Mora Carrillo).
Supplementary Material
The Supplementary Material for this article can be found online at: https://www.frontiersin.org/articles/10.3389/fevo.2022.863242/full#supplementary-material
References
Amato, K. R., Leigh, S. R., Kent, A., Mackie, R. I., Yeoman, C. J., Stumpf, R. M., et al. (2014). The role of gut microbes in satisfying the nutritional demands of adult and juvenile wild, black howler monkeys (Alouatta pigra). Am. J. Phys. Anthropol. 155, 652–664. doi: 10.1002/ajpa.22621
Amato, K. R., Leigh, S. R., Kent, A., Mackie, R. I., Yeoman, C. J., Stumpf, R. M., et al. (2015). The gut microbiota appears to compensate for seasonal diet variation in the wild black howler monkey (Alouatta pigra). Microb. Ecol. 69, 434–443. doi: 10.1007/s00248-014-0554-7
Amato, K. R., and Righini, N. (2015). “The howler monkey as a model for exploring host-gut microbiota interactions in primates,” in Howler Monkeys. Adaptive Radiation, Systematics, and Morphology, eds M. M. Kowalewski, P. A. Garber, L. Cortés-Ortiz, B. Urbani, and D. Youlatos (New York, NY: Springer), 229–258.
Amato, K. R., Yeoman, C. J., Kent, A., Righini, N., Carbonero, F., Estrada, A., et al. (2013). Habitat degradation impacts black howler monkey (Alouatta pigra) gastrointestinal microbiomes. ISME J. 7, 1344–1353. doi: 10.1038/ismej.2013.16
Aristizabal, J. F. (2013). Estrategias de Forrajeo y Características Nutricionales de la Dieta Del Mono Aullador Negro (Alouatta pigra) en un Ambiente Fragmentado. dissertation/master’s thesis, Xalapa: Instituto de Ecología.
Aristizabal, J. F., Rothman, J. M., García-Feria, L. M., and Serio-Silva, J. C. (2017). Contrasting time-based and weight-based estimates of protein and energy intake of black howler monkeys (Alouatta pigra). Am. J. Primatol. 79, 1–8. doi: 10.1002/ajp.22611
Arroyo-Rodríguez, V., and Dias, P. A. D. (2010). Effects of habitat fragmentation and disturbance on howler monkeys: a review. Am. J. Primatol. 72, 1–16. doi: 10.1002/ajp.20753
Balestri, M., Barresi, M., Campera, M., Serra, V., Ramanamanjato, J. B., Heistermann, M., et al. (2014). Habitat degradation and seasonality affect physiological stress levels of Eulemur collaris in littoral forest fragments. PLoS One 9:e107698. doi: 10.1371/journal.pone.0107698
Barelli, C., Albanese, D., Stumpf, R. M., Asangba, A., Donati, C., Rovero, F., et al. (2020). The gut microbiota communities of wild arboreal and ground-feeding tropical primates are affected differently by habitat disturbance. mSystems 5:e00061-20. doi: 10.1128/mSystems.00061-20
Barton, K. (2009). MuMIn: Multi-model Inference. R Package Version 1.0.0. Available online at: http://r-forge.r-project.org/projects/mumin/
Beehner, J. C., and Bergman, T. J. (2017). The next step for stress research in primates: to identify relationships between glucocorticoid secretion and fitness. Horm. Behav. 91, 68–83. doi: 10.1016/j.yhbeh.2017.03.003
Behie, A. M., and Pavelka, M. S. M. (2013). “Interacting roles of diet, cortisol levels, and parasites in determining population density of Belizean howler monkeys in a hurricane damaged forest fragment,” in Primates in Fragments. Developments in Primatology: Progress and Prospects, eds L. Marsh and C. Chapman (New York, NY: Springer), 447–456. doi: 10.1007/978-1-4614-8839-2_30
Benavidez, K. M., Iruri-Tucker, A., Steiniche, T., and Wasserman, M. D. (2019). Primate microbial endocrinology: an uncharted frontier. Am. J. Primatol. 81:e23053. doi: 10.1002/ajp.23053
Benjamini, Y., and Hochberg, Y. (1995). Controlling the false discovery rate: a practical and powerful approach to multiple testing. J. R. Stat. Soc. Series B Stat. Methodol. 57, 289–300.
Bharwani, A., Mian, M. F., Foster, J. A., Surette, M. G., Bienenstock, J., and Forsythe, P. (2016). Structural and functional consequences of chronic psychosocial stress on the microbiome and host. Psychoneuroendocrinology 63, 217–227. doi: 10.1016/j.psyneuen.2015.10.001
Bittinger, K. (2016). Package ‘qiimer’, Version 0.9.4, 1–17. Available online at: https://CRAN.Rproject.org/package=. (qiimer).
Bonier, F., Martin, P. R., Moore, I. T., and Wingfield, J. C. (2009). Do baseline glucocorticoids predict fitness? Trends Ecol. Evol. 24, 634–642.
Boonstra, R. (2013). Reality as the leading cause of stress: rethinking the impact of chronic stress in nature. Funct. Ecol. 27, 11–23. doi: 10.1111/1365-2435.12008
Boyle, S. A., de la Sacha, N. U., Pérez, P., and Kabelik, D. (2021). Small mammal glucocorticoid concentrations vary with forest fragment size, trap type, and mammal taxa in the Interior Atlantic Forest. Sci. Rep. 11:2111. doi: 10.1038/s41598-021-81073-2
Callahan, B. J., McMurdie, P. J., and Holmes, S. P. (2017). Exact sequence variants should replace operational taxonomic units in marker-gene data analysis. ISME J. 11, 2639–2643. doi: 10.1038/ismej.2017
Callahan, B. J., McMurdie, P. J., Rosen, M. J., Han, A. W., Johnson, A. J. A., and Holmes, S. P. (2016). DADA2: high-resolution sample inference from Illumina amplicon data. Nat. Methods 13, 581–583. doi: 10.1038/nmeth.3869
Cartmill, M. (2017). “Arboreal adaptations and the origin of the order primates,” in The Functional and Evolutionary Biology of Primates (eBook), ed. R. Tuttle (New York, NY: Routledge), 97–122. doi: 10.4324/9781315132129
Chapman, C. A., Chapman, L. J., Wrangham, R., Hunt, K., Gebo, D., and Gardner, L. (1992). Estimators of fruit abundance of tropical trees. Biotropica 24, 527–531. doi: 10.2307/2389015
Chapman, C. A., Wasserman, M. D., Gillespie, T. R., Speirs, M. L., Lawes, M. J., Saj, et al. (2006). Do food availability, parasitism, and stress have synergistic effects on red colobus populations living in forest fragments? Am. J. Phys. Anthropol. 131, 525–534. doi: 10.1002/ajpa.20477
Chapman, C. A., Wrangham, R., and Chapman, L. J. (1994). Indices of habitat-wide fruit abundance in tropical forests. Biotropica 26, 160–171. doi: 10.2307/2388805
Creel, S., Fox, J. E., Hardy, A., Sands, J., Garrott, B., and Peterson, R. O. (2002). Snowmobile activity and glucocorticoid stress responses in wolves and elk. Cons. Biol. 16, 809–814. doi: 10.1046/j.1523-1739.2002.00554.x
David, L. A., Maurice, C. F., Carmody, R. N., Gootenberg, D. B., Button, J. E., Wolfe, B. E., et al. (2014). Diet rapidly and reproducibly alters the human gut microbiome. Nature 505, 559–563. doi: 10.1038/nature12820
De Palma, G., Lynch, M. D. J., Lu, J., Dang, V. T., Deng, Y., Jury, J., et al. (2017). Transplantation of fecal microbiota from patients with irritable bowel syndrome alters gut function and behavior in recipient mice. Sci. Transl. Med. 9:eaaf6397. doi: 10.1126/scitranslmed.aaf6397
Díaz-Gallegos, J. R., Mas, J. F., and Velázquez, A. (2010). Trends of tropical deforestation in Southeast Mexico. Singap. J. Trop. Geogr. 31, 180–196. doi: 10.1111/j.1467-9493.2010.00396.x
Douglas, A. E. (2018). Fundamentals of Microbiome Science. How Microbes Shape Animal Biology. Hoboken, NJ: Princeton University Press.
Estaki, M., Jiang, L., Bokulich, N. A., McDonald, D., González, A., Kosciolek, T., et al. (2020). QIIME 2 enables comprehensive end-to-end analysis of diverse microbiome data and comparative studies with publicly available data. Curr. Protoc. Bioinform. 70:e100. doi: 10.1002/cpbi.100
Estrada, A., Garber, P. A., Rylands, A. B., Roos, C., Fernandez-Duque, E., Di Fiore, A., et al. (2017). Impending extinction crisis of the world’s primates: why primates matter. Sci. Adv. 3:e1600946. doi: 10.1126/sciadv.1600946
Formenti, N., Viganó, R., Fraquelli, C., Trogu, T., Bonfanti, M., Lanfranchi, P., et al. (2018). Increased hormonal stress response of Apennine chamois induced by interspecific interactions and anthropogenic disturbance. Eur. J. Wildl. Res. 64:68. doi: 10.1007/s10344-018-1228-4
Foster, J. A., and McVey Neufeld, K. A. (2013). Gut-brain axis: how the microbiome influences anxiety and depression. Trends Neurosci. 36, 305–312.
Foster, J. A., Rinaman, L., and Cryan, J. F. (2017). Stress and the gut-brain axis: regulation by the microbiome. Neurobiol. Stress 7, 124–136.
Fox, J., and Weisberg, S. (2019). An R Companion to Applied Regression, 3rd Edn. Thousand Oaks, CA: Sage.
Gabriel, D. N., Gould, L., and Cook, S. (2018). Crowding as a primary source of stress in an endangered fragment-dwelling strepsirrhine primate. Anim. Cons. 21, 76–85. doi: 10.1111/acv.12375
Ganzhorn, J. U. (2003). “Habitat description and phenology,” in Field and Laboratory Methods in Primatology. A Practical Guide, eds J. M. Setchell and D. J. Curtis (Cambridge: Cambridge University Press), 40–56.
Gentry, A. H. (1982). “Patterns of neotropical plant species diversity,” in Evolutionary Biology, eds M. K. Hecht, B. Wallace, and G. T. Prance (Boston, MA: Springer), 1–84. doi: 10.1007/978-1-4615-6968-8_1
Gómez-Marín, F., Veá, J. J., Rodríguez-Luna, E., García-Orduña, F., Canales-Espinosa, D., Escobar, M., et al. (2001). Food resources and the survival of a group of howler monkeys (Alouatta palliata mexicana) in disturbed and restricted habitat at Los Tuxtlas, Veracruz, México. Neotrop. Prim. 9, 60–66.
Hammer, Ø, Harper, D. A., and Ryan, P. D. (2001). PAST: paleontological statistics software package for education and data analysis. Palaeontol. Electron 4:9.
Hopkins, M. E. (2011). Mantled howler (Alouatta palliata) arboreal pathway networks: relative impacts of resource availability and forest structure. Int. J. Primatol. 32, 238–258.
Klass, K., Van Belle, S., Campos-Villanueva, A., Mercado Malabet, F., and Estrada, A. (2020). Effects of variation in forest fragment habitat on black howler monkey demography in the unprotected landscape around Palenque National Park, Mexico. PeerJ 8:e9694. doi: 10.7717/peerj.9694
Kleist, N. J., Guralnick, R. P., Cruz, A., Lowry, C. A., and Francis, C. D. (2018). Chronic anthropogenic noise disrupts glucocorticoid signaling and has multiple effects on fitness in an avian community. Proc. Nat. Acad. Sci U S A. 115, E648–E657. doi: 10.1073/pnas.1709200115
Knight, R., Vrbanac, A., Taylor, B. C., Aksenov, A., Callewaert, C., Debelius, J., et al. (2018). Best practices for analysing microbiomes. Nat. Rev. Microbiol. 16, 410–422.
Li, Y., Guo, Y., Wen, Z., Jiang, X., Ma, X., and Han, X. (2018). Weaning stress perturbs gut microbiome and its metabolic profile in piglets. Sci. Rep. 8:18068. doi: 10.1038/s41598-018-33649-8
Mallott, E. K., and Amato, K. R. (2018). The microbial reproductive ecology of white-faced capuchins (Cebus capucinus). Am. J. Primatol. 80:e22896. doi: 10.1002/ajp.22896
Martin, B. D., Witten, D., and Willis, A. D. (2020). Modeling microbial abundances and dysbiosis with beta-binomial regression. Ann. Appl. Stat. 14, 94–115. doi: 10.1214/19-aoas1283
Martin, B. D., Witten, D., and Willis, A. D. (2021). Package “Corncob” Count Regression for Correlated Observations with Beta-Binomial, version 0.2.0. Available online at: https://github.com/bryandmartin/corncob.
Martínez-Mota, R., Garber, P. A., Palme, R., and Gillespie, T. R. (2017). The relative effects of reproductive condition, stress, and seasonality on patterns of parasitism in wild female black howler monkeys (Alouatta pigra). Am. J. Primatol. 9999:e22669. doi: 10.1002/ajp.22669
Martínez-Mota, R., Righini, N., Mallott, E. K., Gillespie, T. R., and Amato, K. R. (2021). The relationship between pinworm (Trypanoxyuris) infection and gut bacteria in wild black howler monkeys (Alouatta pigra). Am. J. Primatol. 83:e23330. doi: 10.1002/ajp.23330
Martínez-Mota, R., Righini, N., and Palme, R. (2016). Fluctuations in daily energy intake do not cause physiological stress in a neotropical primate living in a seasonal forest. Oecologia 182, 973–984. doi: 10.1007/s00442-016-3739-6
Martínez-Mota, R., Valdespino, C., Rivera Rebolledo, J. A., and Palme, R. (2008). Determination of fecal glucocorticoid metabolites to evaluate stress response in Alouatta pigra. Int. J. Primatol. 29, 1365–1373.
Martínez-Mota, R., Valdespino, C., Sánchez-Ramos, M. A., and Serio-Silva, J. C. (2007). Effects of forest fragmentation on the physiological stress response of black howler monkeys. Anim. Cons. 10, 374–379.
Martins, M. M. (2009). Lianas as a food resource for brown howlers (Alouatta guariba) and southern muriquis (Brachyteles arachnoides) in a forest fragment. Anim. Biodivers. Conserv. 32, 51–58.
McMurdie, P. J., and Holmes, S. (2013). phyloseq: an R package for reproducible interactive analysis and graphics of microbiome census data. PLoS One 8:e61217. doi: 10.1371/journal.pone.0061217
Messina, S., Edwards, D. P., Eens, M., and Costantini, D. (2018). Physiological and immunological responses of birds and mammals to forest degradation: a meta-analysis. Biol. Cons. 224, 223–229. doi: 10.1016/j.biocon.2018.06.002
Meyyappan, A. C., Forth, E., Wallace, C. J. K., and Milev, R. (2020). Effect of fecal microbiota transplant on symptoms of psychiatric disorders: a systematic review. BMC Psychiatry 20:299. doi: 10.1186/s12888-020-02654-5
Moloney, R. D., Desbonnet, L., Clarke, G., Dinan, T. G., and Cryan, J. F. (2014). The microbiome: stress, health and disease. Mamm. Genome 25, 49–74. doi: 10.1007/s00335-013-9488-5
Möstl, E., Maggs, J. L., Schrotter, G., Besenfelder, U., and Palme, R. (2002). Measurement of cortisol metabolites in faeces of ruminants. Vet. Res. Commun. 26, 127–139.
Noguera, J. C., Aira, M., Pérez-Lozada, M., Domínguez, J., and Velando, A. (2018). Glucocorticoids modulate gastrointestinal microbiome in a wild bird. R. Soc. Open Sci. 5:171743. doi: 10.1098/rsos.171743
Oksanen, J., Blanchet, F. G., Friendly, M., Kindt, R., Legendre, P., McGlinn, D., et al. (2019). Package ‘vegan’. Community Ecology Package, version, 2.5-6. Available online at: https://github.com/vegandevs/vegan.
Palme, R. (2019). Non-invasive measurement of glucocorticoids: advances and problems. Physiol. Behav. 199, 229–243. doi: 10.1016/j.physbeh.2018.11.021
Palme, R., Touma, C., Arias, N., Dominchin, M. F., and Lepschy, M. (2013). Steroid extraction: get the best out of faecal samples. Wien Tierarztl Monat. 100, 238–246.
Pérez Ortega, R., and Gutiérrez Jaber, I. (2022). A controversial train heads for the Maya forest. Science 375, 250–251. doi: 10.1126/science.ada0230
Pierce, J. M., and Alviña, K. (2019). The role of inflammation and the gut microbiome in depression and anxiety. J. Neurosci. Res. 97, 1223–1241. doi: 10.1002/jnr.24476
Pozo-Montuy, G., Serio-Silva, J. C., and Bonilla-Sánchez, Y. M. (2011). Influence of the landscape matrix on the abundance of arboreal primates in fragmented landscapes. Primates 51, 139–147. doi: 10.1007/s10329-010-0231-5
Quast, C., Pruesse, E., Yilmaz, P., Gerken, J., Schweer, T., Yarza, P., et al. (2013). The SILVA ribosomal RNA gene database project: improved data processing and web-based tools. Nucleic Acids Res. 41, D590–D596. doi: 10.1093/nar/gks1219
R Core Team (2020). R: A Language and Environment for Statistical Computing. Vienna: R Foundation for Statistical Computing.
Rangel-Negrín, A., Coyohua-Fuentes, A., Chavira, R., Canales-Espinosa, D., and Dias, P. A. D. (2014a). Primates living outside protected habitats are more stressed: the case of black howler monkeys in the Yucatán Peninsula. PLoS One 9:e112329. doi: 10.1371/journal.pone.0112329
Rangel-Negrín, A., Coyohua-Fuentes, A., Canales-Espinosa, D., and Dias, P. A. D. (2014b). Mammal assemblages in forest fragments and landscapes occupied by black howler monkeys. Primates 55, 345–352. doi: 10.1007/s10329-014-0415-5
Reeder, D. A., and Kramer, K. M. (2005). Stress in free-ranging mammals: integrating physiology, ecology, and natural history. J. Mammal. 86, 225–235.
Rehnus, M., Wehrle, M., and Palme, R. (2014). Mountain hares (Lepus timidus) and tourism: stress events and reactions. J. Appl. Ecol. 51, 6–12. doi: 10.1111/1365-2664.12174
Righini, N. (2014). Primate Nutritional Ecology: the Role of Food Selection, Energy Intake, and Nutrient Balancing in Mexican Black Howler Monkey (Alouatta pigra) Foraging Strategies. dissertation/doctoral thesis, Urbana, IL: University of Illinois at Urbana-Champaign.
Righini, N., Garber, P. A., and Rothman, J. M. (2017). The effects of plant nutritional chemistry on food selection of Mexican black howler monkeys (Alouatta pigra): the role of lipids. Am. J. Primatol. 79, 1–15. doi: 10.1002/ajp.22524
Romero, M. L. (2004). Physiological stress in ecology: lessons from biomedical research. Trends Ecol. Evol. 19, 249–255. doi: 10.1016/j.tree.2004.03.008
Romero, M. L., Dickens, M., and Cyr, N. (2009). The reactive scope model – a new model integrating homeostasis, allostasis, and stress. Horm. Behav. 55, 375–389. doi: 10.1016/j.yhbeh.2008.12.009
Romero, M. L., and Wikelski, M. (2001). Corticosterone levels predict survival probabilities of Galápagos marine iguanas during El Niño events. Proc. Nat. Acad. Sci. U S A. 98, 7366–7373. doi: 10.1073/pnas.131091498
Rosseel, Y. (2012). lavaan: an R package for structural equation modelling. J. Stat. Softw. 48, 1–36. doi: 10.18637/jss.v048.i02
Sapolsky, R. M. (2021). Glucocorticoids, the evolution of the stress response, and the primate predicament. Neurobiol. Stress 14:100320. doi: 10.1016/j.ynstr.2021.100320
Sheriff, M. J., Dantzer, B., Delehanty, B., Palme, R., and Boonstra, R. (2011). Measuring stress in wildlife: techniques for quantifying glucocorticoids. Oecologia 166, 869–887. doi: 10.1007/s00442-011-1943-y
Silva, Y. P., Bernardi, A., and Frozza, R. L. (2020). The role of short-chain fatty acids from gut microbiota in gut-brain communication. Front. Endocrinol. 11:25. doi: 10.3389/fendo.2020.00025
Skaug, H., Fournier, D., Nielsen, A., Magnusson, A., and Bolker, B. (2018). glmmADMB: Generalized Linear Mixed Models using ‘AD Model Builder’. Version 0.8.3.4. Available online at: http://glmmadmb.r-forge.r-project.org
Söderholm, J. D., Yang, P. C., Ceponis, P., Vohra, A., Ridell, R., Sherman, P. M., et al. (2002). Chronic stress induces mast cell-dependent bacterial adherence and initiates mucosal inflammation in rat intestines. Gastroenterology 123, 1099–1108. doi: 10.1053/gast.2002.36019
Stothart, M. R., Bobbie, C. B., Schulte-Hostedde, A. I., Boonstra, R., Palme, R., Mykytczuk, N. C. S., et al. (2016). Stress and the microbiome: linking glucocorticoids to bacterial community dynamics in wild red squirrels. Biol. Lett. 12:20150875. doi: 10.1098/rsbl.2015.0875
Stothart, M. R., Palme, R., and Newman, A. E. M. (2019). It’s what’s on the inside that counts: stress physiology and the bacterial microbiome of a wild urban mammal. Proc. R. Soc. B 286:20192111. doi: 10.1098/rspb.2019.2111
Sudo, N., Chida, Y., Aiba, Y., Sonoda, J., Oyama, N., Yu, X. N., et al. (2004). Postnatal microbial colonization programs the hypothalamic-pituitary-adrenal system for stress response in mice. J. Physiol. 558, 263–275. doi: 10.1113/jphysiol.2004.063388
Sun, L., Zhang, H., Cao, Y., Wang, C., Zhao, C., Wang, H., et al. (2017). Fluoxetine ameliorates dysbiosis in a depression model induced by chronic unpredicted mild stress in mice. Int. J. Med. Sci. 16, 1260–1270. doi: 10.7150/ijms.37322
Tecot, S. R., Irwin, M. T., and Raharison, J. L. (2019). Faecal glucocorticoid metabolite profiles in diademed sifakas increase during seasonal fruit scarcity with interactive effects of age/sex class and habitat degradation. Cons. Physiol. 7:coz001. doi: 10.1093/conphys/coz001
Tiffany, C. R., Lee, J. Y., Rogers, A. W. L., Olsan, E. E., Morales, P., Faber, F., et al. (2021). The metabolic footprint of clostridia and erysipelotrichia reveals their role in depleting sugar alcohols in the cecum. Microbiome 9:174. doi: 10.1186/s40168-021-01123-9
Trevelline, B. K., Fontaine, S. S., Hartup, B. K., and Kohl, K. D. (2019). Conservation biology needs a microbial renaissance: a call for the consideration of host associated microbiota in wildlife management practices. Proc. R. Soc. B 286:20182448. doi: 10.1098/rspb.2018.2448
Vlčková, K., Shutt-Phillips, K., Heistermann, M., Pafèo, B., Petrželková, K. J., Todd, A., et al. (2018). Impact of stress on the gut microbiome of free-ranging western lowland gorillas. Microbiology 164, 40–44. doi: 10.1099/mic.0.000587
Wagenmakers, E. J., and Farrell, S. (2004). AIC model selection using Akaike weights. Psychon. Bull. Rev. 11, 192–196.
Wastyk, H. C., Fragiadakis, G. K., Perelman, D., Dahan, D., Merrill, B. D., Yu, F. B., et al. (2021). Gut-microbiota-targeted diets modulate human immune status. Cell 184, 4137–4153. doi: 10.1016/j.cell.2021.06.019
Weinstein, S. B., Martínez-Mota, R., Stapleton, T. E., Klure, D. M., Greenhalgh, R., Orr, T. J., et al. (2021). Microbiome stability and structure is governed by host phylogeny over diet and geography in woodrats (Neotoma spp.). Proc. Nat. Acad. Sci. U S A. 118:e2108787118. doi: 10.1073/pnas.2108787118
Wingfield, J. C. (2005). The concept of allostasis: coping with capricious environment. J. Mammal. 86, 248–254. doi: 10.1644/BHE-004.1
Yan, D., Hu, D., Li, K., Li, B., Zeng, X., Chen, J., et al. (2021). Effects of chronic stress on the fecal microbiome of Malayan pangolins (Manis javanica) rescued from the illegal wildlife trade. Curr. Microbiol. 78, 1017–1025. doi: 10.1007/s00284-021-02357-4
Yang, Q., Liang, Q., Balakrishnan, B., Belobrajdic, D., Feng, Q. J., and Zhang, W. (2020). Role of dietary nutrients in the modulation of gut microbiota: a narrative review. Nutrients 12:381. doi: 10.3390/nu12020381
Keywords: arboreal primates, microbiome, 16S rRNA, physiological stress, glucocorticoids, tropical forest disturbance, Mexico
Citation: Martínez-Mota R, Righini N, Mallott EK, Palme R and Amato KR (2022) Environmental Stress and the Primate Microbiome: Glucocorticoids Contribute to Structure Gut Bacterial Communities of Black Howler Monkeys in Anthropogenically Disturbed Forest Fragments. Front. Ecol. Evol. 10:863242. doi: 10.3389/fevo.2022.863242
Received: 27 January 2022; Accepted: 22 June 2022;
Published: 07 July 2022.
Edited by:
Benjamin Jerry Ridenhour, University of Idaho, United StatesReviewed by:
Giovana Slanzon, University of Hawai‘i, United StatesIlaria Agostini, Instituto de Biología Subtropical (IBS), Argentina
Copyright © 2022 Martínez-Mota, Righini, Mallott, Palme and Amato. This is an open-access article distributed under the terms of the Creative Commons Attribution License (CC BY). The use, distribution or reproduction in other forums is permitted, provided the original author(s) and the copyright owner(s) are credited and that the original publication in this journal is cited, in accordance with accepted academic practice. No use, distribution or reproduction is permitted which does not comply with these terms.
*Correspondence: Katherine R. Amato, a2F0aGVyaW5lLmFtYXRvQG5vcnRod2VzdGVybi5lZHU=